Wastewater-based protocols for SARS-CoV-2: insights into virus concentration, extraction, and quantitation methods from two years of public health surveillance†
Received
27th December 2023
, Accepted 25th May 2024
First published on 6th June 2024
Abstract
The ongoing COVID-19 pandemic has accelerated the development and application of wastewater-based disease surveillance (WBS) as a tool for public health practice. The wide variety of WBS methods currently in use hinders the ability to compare data between different laboratories and limits the potential of nationwide surveillance programs. In this study, we conducted a systematic analysis to identify among widely used concentration, extraction and quantification methods, which ones would perform well for WBS of SARS-CoV-2. We evaluated electronegative filtration, one of the traditional methods applied early in the pandemic, to other methods including direct capture, magnetic affinity particles and PEG. Our results indicated that these alternative concentration methods quantify SARS-CoV-2 just as effective if not better compared to membrane filtration. We also identified the effect that filtration flow rate, volume filtered, and bead beating parameters have on viral target recovery. The evaluation of different extraction methods demonstrated that an automatic paramagnetic bead-based method performs better than the column-based method tested. In addition, we compared the quantification between RT-qPCR and RT-dPCR, and while both perform well, we documented that RT-dPCR has a lower LOD and can provide more accurate data. Lastly, we compared three weeks of side-by-side wastewater surveillance by two different, but currently commonly applied approaches: HA filtration quantified by RT-qPCR and Ceres Nanotrap® Microbiome A Particles quantified by RT-dPCR. On average, we found a 3.6-fold difference in SARS-CoV-2 levels between the two approaches and observed that the N1
:
N2 ratio was closer to one with Nanotrap® particle concentration quantified by RT-dPCR.
Water impact
Wastewater surveillance is a useful public health tool to track SARS-CoV-2 and other disease targets in communities. This study provides important guidelines for the selection of analytical approaches for SARS-CoV-2 quantification from wastewater. Each of the key steps of the wastewater workflow (virus capture/concentration, nucleic acid extraction, and PCR quantification) are examined. Multiple protocols within each key workflow step are compared, and their performance evaluated – including several protocols that have not been previously studied and compared. In parallel with SARS-CoV-2, the study evaluated the performance of these protocols for two method control viruses (PMMoV and BCoV). The study also included a large (over 200 wastewater samples) comparison of two common wastewater monitoring workflows; virus concentration by HA filtration paired with quantification by RT-qPCR and Nanotrap® paired with quantification by RT-dPCR. The findings from these comparisons provide important guidance for the selection of methods for wastewater monitoring of SARS-CoV-2 and potentially other pathogens.
|
1. Introduction
The value of monitoring wastewater to determine the presence and quantity of pathogens, i.e., wastewater-based disease surveillance (WBS), has long been recognized.1–3 However, until the COVID-19 pandemic WBS had not been used to surveil a viral outbreak on a similar scale,4 and with generally widespread public health support. Monitoring of severe acute respiratory syndrome coronavirus 2 (SARS-CoV-2), the etiological agent of COVID-19, in wastewater began early in the pandemic in several countries, including Australia,5 Italy,6 the Netherlands,7,8 and the United States.9 Since then, wastewater surveillance of SARS-CoV-2 has expanded to over 50 countries and many universities.10 Despite the growth and expansion of SARS-CoV-2 WBS efforts worldwide, multiple challenges remain in both testing methods and data analysis. The robust assessment of SARS-CoV-2 RNA levels in wastewater requires careful attention to several key methodological steps, including selection and implementation of the virus concentration, extraction and polymerase chain reaction (PCR) quantitation protocols, as well as the molecular targets to be assessed, and quality controls to be implemented at various stages of the overall process.
Several studies have been published comparing selected available protocols for the three main processing steps: concentration, extraction and PCR quantification (reviewed in ref. 11–13). Protocols for virus concentration that have been examined include ultracentrifugation, filtration, magnetic particles, and polyethylene glycol (PEG) precipitation.14–21 Babler et al., 202214 compared electronegative (HA) filtration with magnetic bead-based concentration methods for SARS-CoV-2 and OC43 recovery, finding that both methods produced equivalent results. Advantages to membrane filtration include flexibility (variable input volumes) and relatively short processing times, while some disadvantages include limited automation options and frequent requirement of sample pretreatment. In contrast, the magnetic bead approaches offer several advantageous automation options, yet a prescribed and often restricted starting volume can be a limiting factor. Kaya et al., 202216 compared 50 different combinations of concentration and extraction protocols for bovine respiratory syncytial virus (BRSV), a proposed proxy virus for SARS-CoV-2, and found the highest recovery was achieved with ultrafiltration and Zymo-Quick RNA kit, though the authors did not include SARS-CoV-2 in their comparison. Kevill et al., 202217 evaluated aluminum-based adsorption and PEG precipitation methods and did not find a difference in SARS-CoV-2 recovery between the two. North and Bibby, 202318 recently demonstrated that virus concentration methods vary in efficiency based on the viral structure, finding that SARS-CoV-2 performed best with PEG, evaluating both the viral recovery and number of detects across replicates tested. However, North and Bibby18 concentrated 200 mL of wastewater with an overnight agitation, which may not always be suitable for wastewater surveillance and the urgency of data turnaround times.
Several studies have compared quantitative PCR (qPCR) methods.14,22,23 Babler et al., 202214 compared volcano second generation (V2G)–qPCR and reverse transcriptase (RT)-qPCR and found them to be equivalent in their quantitation. More relevant to the recent introduction of dPCR use in WBS, RT-qPCR has been compared with digital PCR (dPCR) by Ahmed et al. 202222 indicating that dPCR is 2–5 times more sensitive than qPCR in detecting SARS-CoV-2. These comparison studies of PCR and concentration methods commonly concluded that when properly conducted most of the methods evaluated can produce adequate results. The method choice for WBS applications, however, is often driven by a suite of performance metrics, including PCR inhibition, limit of detection, turnaround time, throughput, as well as supply cost and availability,14,15,19 and adequate is not necessarily acceptable or advisable for some wastewater surveillance applications (e.g., low target abundance).
Currently no widely accepted best-practice method exists for monitoring SARS-CoV-2 in wastewater, though several guidance documents have been created and resources are now available for those planning to use wastewater for pathogen surveillance.24–26 Many factors contribute to the lack of standardization and consensus, including evolving methodology, complex and varied wastewater matrices, and few head-to-head method trials, particularly with newer methods. None of the wastewater surveillance SARS-CoV-2 method comparison studies published to date has presented a thorough method comparison between several popular wastewater concentration methods, especially newer methods that may offer improved capture/concentration efficiency and higher throughput, or a comparison between RT-qPCR and new RT-dPCR quantification platforms. Moreover, for completeness those studies should include sufficient numbers of wastewater samples from different facilities to capture the different characteristics of wastewater matrices over time and the varied virus levels that can also affect the method performance.
In this study we compared several common capture/concentration protocols (HA filtration, Promega's direct capture, affinity-binding beads, and PEG) with multiple protocol variations, as well as on-column extraction and automated magnetic bead-based nucleic acid extraction, and quantitation by RT-qPCR and RT-dPCR. To assess the performance of those methods, we quantified SARS-CoV-2 genes N1 and N2, pepper mild mottle virus (PMMoV) as an endogenous human fecal marker, bovine coronavirus (BCoV) an exogenous SARS-CoV-2 surrogate, and bovine respiratory syncytial virus (BRSV) RNA as the indicator of inhibition. We also compared two surveillance methods, HA filtration quantified by RT-qPCR and affinity-binding beads (Nanotrap® particles) quantified by RT-dPCR as deployed for large-scale monitoring to assess the performance difference in these two commonly applied surveillance approaches. A large number of laboratories had begun to deploy nanotraps together with the newly recommended dPCR approach, yet many laboratories were still using the HA and qPCR combo (which is less expensive to implement and applicable to smaller scale monitoring efforts), so we had the opportunity to directly compare those two common approaches across many different wastewater matrices. The resulting dataset from this case-study allowed for normalization of the data generated by these two major workflow approaches and spoke to their overall relative performance. With 229 samples, from 45 different wastewater treatment facilities, the large sample size provided for robust conclusions of comparative performance.
We emphasize in most of these comparisons the performance of both the latest generation of affinity-binding magnetic-bead-based capture and new dPCR quantitation protocols.
2. Materials and methods
2.1 Wastewater collection
The samples used in these studies were obtained from 60 publicly owned treatment works (POTWs) across Wisconsin, USA. All were sub-samples of 24-hour flow-proportionate influent composites, collected after grit removal, between November 2020 and October 2022, except one facility that is a 24-hour time proportionate composite. Influent chemical and physical measurements were taken by POTW operators employing their routine procedures. The wastewater samples were shipped overnight at 2–10 °C. Upon receipt, 250 mL of unpasteurized wastewater was spiked with 20 μL of approximately 100
000 gc μL−1 of Calf-Guard® (Zoetis, Parsippany, NJ, USA), a cattle vaccine containing bovine coronavirus (BCoV), which serves as a wastewater viral recovery control. Studies using larger volumes of wastewater were spiked with BCoV proportionally. The BCoV-spiked influent was held at 4 °C for up to four hours, until virus concentration was performed. Prior to use as a wastewater viral recovery control, the BCoV solution was titered and the concentration in gc μL−1 was ascertained (see ESI† Methods). All BCoV-spiked samples were homogenized by liberal hand-shaking immediately before virus concentration. Pre-filtering was purposely not performed to measure the total wastewater virus content (not biased towards the solid or aqueous phase), and to provide higher throughput.
2.2 Virus capture-concentration methods
2.2.1 HA filtration.
A total of 25 mL of untreated wastewater was filtered using 0.8 μm mixed cellulose ester membrane filters (47 mm diameter; MF Millipore, Burlington, MA, USA; commonly referred to as HA filters) on a vacuum manifold, unless noted otherwise. Before use, the filters were soaked for a minimum of 1 hour in a solution of 25 mM MgCl2 After sample processing, the filters were folded in half and rolled before being placed into Lysing Matrix A 2 mL bead-bashing tubes (MP Biomedical, Irvine, CA, USA) containing 400 μL of cetrimonium bromide buffer (CTAB; Promega, Madison, WI, USA). The tubes were then immediately frozen at −80 °C for a minimum of 1 hour before cell disruption and lysis by bead beating (7 m s−1 for 90 seconds on the MPBio FastPrep-24 5G then centrifuged for 2 minutes at 9168 × g) and RNA extraction with the custom Maxwell® HT Environmental TNA kit (#AX9190, Promega, Madison, WI, USA), as described below.
2.2.2 Affinity-binding beads (Nanotrap® Microbiome A Particles, NT).
Duplicate 24-deep well plates (Thermo Fisher Scientific, Inc., Waltham, MA, USA) were prepared as follows: 5 mL of untreated wastewater was aliquoted into corresponding wells on each plate; 50 μL of Nanotrap® Enhancement Reagent 2 (#10112-30, Ceres Nanosciences, Manassas, VA, USA) was then pipetted into each aliquot, directly followed by 75 μl of affinity-binding Nanotrap® Microbiome A Particles (#44202-30, Ceres Nanosciences, Manassas, VA, USA). Virus capture and concentration was automated using a KingFisher Apex system (Thermo Fisher, Waltham, MA, USA) using the Nanotrap® Classic Wastewater Capture program (Ceres Nanosciences). The captured viruses were lysed and eluted in 300 μL of Cell Lysis Buffer (Promega) and extracted using the Maxwell® HT Environmental TNA kit as described below.
2.2.3 Polyethylene glycol (PEG) precipitation.
For PEG concentration, 38 mL of untreated influent was combined with 13.1 g of 50% PEG 8000 (w/v), 1.2 M NaCl (prepared in-house) in an ultra-centrifuge tube (Polycarbonate #355622, Beckman Coulter, Brea, CA, USA). The tube was thoroughly mixed by inversion and incubated at 4 °C for one hour. Samples were then centrifuged for two hours at 12
000 × g and 4 °C. After centrifugation, the supernatant was removed and the entire pellet was transferred to a 1.5 mL flip-top tube (Eppendorf, Hamburg, Germany) and centrifuged for 2 minutes at 10
000 × g and 4 °C. The remaining supernatant was removed from the tube and the pellet was re-suspended in 600 μL of CTAB Buffer (Promega, Madison, WI, USA) and vortexed thoroughly. Finally, 200 μL of the re-suspended pellet was transferred into 800 μL of CTAB Buffer to create a 1
:
5 dilution (done to alleviate inhibition based on preliminary experiments), which subsequently underwent total nucleic extraction using the Maxwell® HT Environmental TNA kit as described below using 250 μL of the 1
:
5 diluted sample in CTAB. After extraction, the eluted RNA was diluted 1
:
2 in 25 mM Tris HCl (Promega, Madison, WI, USA).
2.2.4 Vacuum-based Promega direct capture (DC).
Untreated wastewater was concentrated using Promega's vacuum-based direct capture protocol, i.e., Wizard® Enviro TNA kit (#A2991). Briefly, 40 mL of influent was centrifuged 1900 × g, for 15 minutes to pellet solids. The supernatant was incubated for 30 minutes with the protease solution included with the kit. The supernatant was then passed through a PureYield binding column (Promega, Madison WI, USA) and washed per manufacturer's protocol. Concentrated influent was then eluted in 500 μL of nuclease free water and extracted using the Maxwell® HT Environmental TNA kit as described below.
2.3 RNA extractions
2.3.1 Promega's Maxwell® HT Environmental TNA kit.
For samples concentrated by HA filtration, filters were thawed at room temperature for 15–20 minutes before being bead beating at a speed setting of 7 m s−1, for 90 seconds, on a MP FastPrep-24™ 5G homogenizer (MP Biomedical, Irvine, CA, USA). Samples were then spun at 9168 × g for 2 minutes at 4 °C in a Microfuge 20R centrifuge (Beckman Coulter, Brea, CA, USA). Without disturbing the pellet, 250 μL of the supernatant was pipetted from each tube and total nucleic acid was extracted in 96 well plates on a KingFisher Flex system (Thermo Fisher, Waltham, MA, USA) following the general procedure described in the custom Maxwell® HT Environmental TNA kit (#AX9190, Promega, Madison, WI, USA). Slight adjustments were made to this procedure; 400 μL of isopropanol (after adding the sample and 35 μL of resin) were added to the wells during the first step instead of adding the isopropanol until after an initial mixing step. Additionally, the elution volume was altered from 50 μL to 200 μL of Tris to generate enough RNA template for experimental and archiving purposes. For samples concentrated by Nanotrap®, the only difference from that described above, was that 300 μL of CLD lysate (instead of 250 μL of sample) was extracted on the KingFisher Flex system.
2.3.2 RNeasy PowerMicrobiome kit.
Extractions with the RNeasy PowerMicrobiome kit (#26000-50, Qiagen, Valencia, CA, USA) were performed manually following manufacturer's protocol. Briefly, wastewater samples collected on a filter (or by PEG where indicated) were lysed using bead beating (90 seconds, 7 m s−1 on MP Bio's FastPrep-24™ 5G) and PM1 buffer (a strong chemical lysis buffer), followed by an inhibitor removal, on-column RNA binding, washes and elution. The optional DNAse treatment was not applied in this study in order to retain total nucleic acids. Total nucleic acids were eluted in 200 μL of RNAse free water from the kit.
2.3.3 MagMax Viral kit.
Samples concentrated by affinity-binding beads (Nanotrap® Microbiome A Particles) indicated as being extracted using the MagMax Viral RNA isolation kit (#AM1939, ThermoFisher, Waltham, MA) were extracted following an automated protocol implemented on the KingFisher Flex. The 450 μL of lysis/binding solution from the Nanotrap®-based concentration was combined with 20 μL of the binding beads and 400 μL of isopropanol in the first step of the KingFisher Flex automated extraction protocol. Total nucleic acids were eluted in 200 μL of Elution Solution from the kit.
2.3.4 NucleoMag DNA/RNA Water kit.
Samples concentrated by affinity-binding beads (Nanotrap® Microbiome A Particles) indicated as being extracted using the NucleoMag DNA/RNA Water kit (#744220.4, Macherey-Nagel, Düren, Germany) were extracted following an automation protocol provided by Ceres Nanosciences for implementation on the KingFisher Flex. Total nucleic acids were eluted in 200 μL of RNAse free water from the kit.
All KingFisher programs are available on Figshare https://doi.org/10.6084/m9.figshare.21538143.v7.
2.4 RT-PCR
Reverse transcription PCR was used to target the N1 and N2 regions of the SARS-CoV-2 N gene by duplexing the N1 and N2 CDC assays. BCoV concentration was determined in each sample using a published assay.27 Inhibition was evaluated in a separate assay by spiking a known amount of BRSV RNA (see ESI† for more details) into the PCR mastermix and comparing the concentration obtained in each sample to the concentration obtained in the method blank. When inhibition was detected (greater than 2Cq values between sample and the method blank for qPCR or less than 50% of the expected gene copies per μL for dPCR), samples were run at a dilution, usually 1
:
2 or 1
:
5 and inhibition was reassessed. PMMoV was quantified as a fecal strength indicator in each wastewater sample using the oligos from Haramoto et al., 2013 with the exception of a probe modification to increase the melting temperature.28 All primers, probes and standard materials used are from Integrated DNA technologies (IDT) unless noted otherwise; all sequences and their final concentrations are listed in Tables S1 and S2.†
2.4.1 RT-qPCR.
Each RT-qPCR analysis included one positive and three negative controls including a no-template control (NTC), a method blank and an extraction blank, all tested in triplicate using the QuantStudio 6 pro thermocycler (Applied Biosystems, ThermoFisher, Waltham, MA). A five-point standard curve was assessed for each viral target (SARS-CoV-2 (N1, N2) and BCoV standards ranged from 10 to 100
000 gene copies per μL and PMMoV ranged from 100 to 1
000
000 gene copies per μL) to evaluate and compare the quantification and threshold cutoff with previous runs. SARS-CoV-2 (N1, N2) standard was a circular plasmid from IDT (#10006624), BCoV and PMMoV positive controls were custom single stranded DNA ultramers (Table S2†). All samples were assessed for inhibition using the BRSV assay. RT-qPCR reactions were setup in 20 μL reaction volume using UltraPlex 1 Step ToughMix (Quantabio, Beverly, MA, USA) or TaqPath 1-Step RT-qPCR master mix (ThermoFisher Scientific) at a final 1× concentration with 5 μL of template and IDT primers and probe for each assay (see Table S1† for final concentrations). RT-qPCR reactions were performed under the following cycling conditions: 50 °C for 10 min, 95 °C for 10 min, followed by 40 cycles of 95 °C for 15 s and 60 °C for 1 min. The limits of detection (LOD) and quantification (LOQ) were defined according to the MIQE guidelines29 and detailed in the ESI.† The LOD and LOQ for N1 and N2 are described in Table S3.† The range of standard curve metrics observed for RT-qPCR in this study (R2, efficiency, slope and y-intercept) are listed in Table S4.†
2.4.2 RT-dPCR.
The master mix for SARS-CoV-2 N1 and N2, BCoV, and PMMoV was prepared using the One-Step Viral RT-PCR kit (4×) (Qiagen, Germantown, MD, USA) and GT Molecular dPCR SARS-CoV-2 Wastewater Surveillance Assay kit (20×) (GT Molecular, Fort Collins, CO, USA). BRSV was determined in a separate assay using primers and probes from IDT (Table S1†). The samples were run on a QIAcuity Four Digital PCR System (Qiagen, Germantown, MD, USA). N1, N2, and BCoV were multiplexed on QIAcuity Nanoplate 26k 24-well plates in 40 μL PCR reactions at 1× final concentration of master mix with 5 μL of template while PMMoV and BRSV were singleplexed on an 8.5k 96-well nanoplate in 12 μL PCR reaction volume with 6 μL of template. The template for PMMoV RT-dPCR assays was diluted 1
:
10 for the reaction to not over saturate the dye channel. Cycling and exposure conditions are listed in Table S5.† Analysis of the dPCR results was performed on the QIAcuity Software Suite version 2.1.7.182. For each target and run, a common threshold was manually set for all samples to separate negative and positive partitions in the positive and negative controls. Threshold consistency between runs was also checked.
2.5 Equivalent sewage volume
The equivalent sewage volume can be determined per eqn (1) for each method.
Eqn (1): equivalent sewage volume
Considering that both RT-qPCR and RT-dPCR used 5 μL of undiluted template for SARS-CoV-2 quantification, the equivalent sewage volume for the primary methods detailed above are, for HA filtration using 25 mL wastewater is 0.39 mL, for Nanotrap using 10 mL of wastewater is 0.25 mL, for PEG using 38 mL of wastewater is 0.04 mL and for direct capture using 40 mL of wastewater is 1 mL.
2.6 Positive and negative controls
Method blanks were prepared and analyzed with each experiment. For HA filtration the method blank was 250 mL TE buffer (10 mM Tris, 1% EDTA, pH 7.5). For Nanotrap® concentration the method blank consisted of 250 mL 1× PBS (pH 7.4, Sigma Aldrich, St. Louis, MO, USA). Method blanks were spiked with 20 μL of titered BCoV per 250 mL of volume (same as samples) and concentrated alongside samples in each experiment. Method blanks are then extracted alongside samples in each experiment and included on each RT-PCR plate. At the extraction step, an extraction blank was introduced by replacing the sample with sterile water. Positive controls for each viral target are included in RT-PCR, 2019-nCoV_N_Positive control (IDT, Coralville, IA, USA) for qPCR, EDX SARS-CoV-2 standard (Exact Diagnostics, Fort Worth, TX, USA) for dPCR and single stranded DNA ultramers for BCoV and PMMoV (Table S2,† IDT, Coralville, IA). No template controls (NTCs) were also included on each PCR plate.
2.7 Assessment of parameters affecting filtration
2.7.1 Assessment of the influence of major HA filtration concentration parameters on recovery of viral targets.
A sample of unfiltered/unpasteurized wastewater influent, obtained from a southern Wisconsin POTW in January 2022, was used to assess the performance of different variations of the HA filtration method. A well-mixed 700 mL aliquot of influent was spiked with 56 μL of the stock titered BCoV suspension, mixed again and subsequently held at 4 °C for a minimum of 30 minutes to allow for equilibration of the BCoV spike. As described in section 2.2.1, all HA filters used in these trials were soaked in 25 mM MgCl2 (final concentration) prior to use.
2.7.1.1 HA membrane filtration baseline method metrics.
The sample was processed by HA filtration in 20 replicates of 25 mL by a single analyst using the same filter funnel and position on the vacuum manifold. Sample was inverted five times to maintain sample homogeneity before each filtration replicate. Filtration of the 25 mL aliquots was completed at a standard vacuum setting of 5.4 PSI (average flow rate 22.2 mL min−1) and the filtration time of each replicate was recorded.
2.7.1.2 HA membrane filtration speed.
To determine the impact of filtering flow rate on target concentrations, two sets of 3 replicates each of 25 mL aliquots were filtered at both lower (2.9 PSI and flow rate 5.6 mL min−1) and faster rate (10.8 PSI and flow rate 49.9 mL min−1) than the standard detailed above.
2.7.1.3 Sample volume filtered.
To assess the influence of sample volume processed on target concentrations determined with the HA filtration protocol, another sample of wastewater influent from the same POTW was collected in May 2022 and processed as follows. A homogeneous aliquot (675 mL) of the unfiltered/unpasteurized sample was spiked upon arrival in the lab with 54 μL of titered BCoV suspension. Multiple aliquots were filtered at a vacuum setting of 5.4 PSI. Aliquots were distributed as 9 filter replicates of 3 groups each, each group representing a different filtration volume of 40 mL, 25 mL, and 10 mL.
2.7.1.4 Bead beating parameters.
A 1200 mL untreated, unfiltered/unpasteurized influent sample, obtained from one POTW in January 2022, and was spiked with 96 μL of BCoV suspension. The influent was aliquoted for a total of 42 replicates of 25 mL of wastewater were HA filtered as described above. For the cell lysis step using bead beating of these samples, eight different protocols were evaluated (Table 2) comparing different speeds of bead bashing (4 or 7 m s−1), the length of time the bead beating lasted (30, 60 or 90 seconds), and the centrifuge speed (9168 or 20
627 × g) used post bead beating to pellet the debris. The use of vortexing the filter in the Lysing Matrix A tube for 15 seconds as opposed to bead beating for cell lysis was also assessed. All protocols tested had 6 filter replicates with the exception of protocol 2 and 6 with 3 filter replicates (4 m s−1 speed parameter) and all filters had 3 RT-qPCR replicates. All 42 samples were extracted as described above with Promega's Maxwell® HT Environmental TNA kit. The resulting RNA was quantified for all viral targets and BRSV for PCR inhibition, using RT-qPCR.
2.8 Plots and statistical analysis
All figures and statistical analyses were done using Prism 9.3.1 (GraphPad Software, Inc., La Jolla, CA) apart from Fig. 7 and its associated statistical tests which were generated using R version 4.1.1 in addition to the regression analysis used to determined significance in Fig. 1. In bar plots, dots represent (when displayed) the individual replicates, bars represent the mean and error bars the standard deviations. Paired t-test (parametric) or Wilcoxon (non-parametric) tests were used to assess the difference of the viral recovery between two groups or methods. Correlation coefficients using Pearson (parametric) or Spearman rank correlation (non-parametric) tests were used to assess the strength and direction of the linear relationships between pairs of variables. Parametric tests were used when variables were normally distributed; otherwise, non-parametric tests were performed. One-way ANOVA with multiple comparison (Tukey post hoc test) were used to compare the difference of means among more than two treatment groups. Two-way ANOVA with multiple comparison (Tukey post hoc test) were used to compare the difference of means among more than three treatment groups. The normality of the ANOVA residuals was evaluated using Shapiro–Wilk tests and the homoscedasticity was checked visually. Where necessary, data were log
10 transformed prior to the ANOVA analysis.
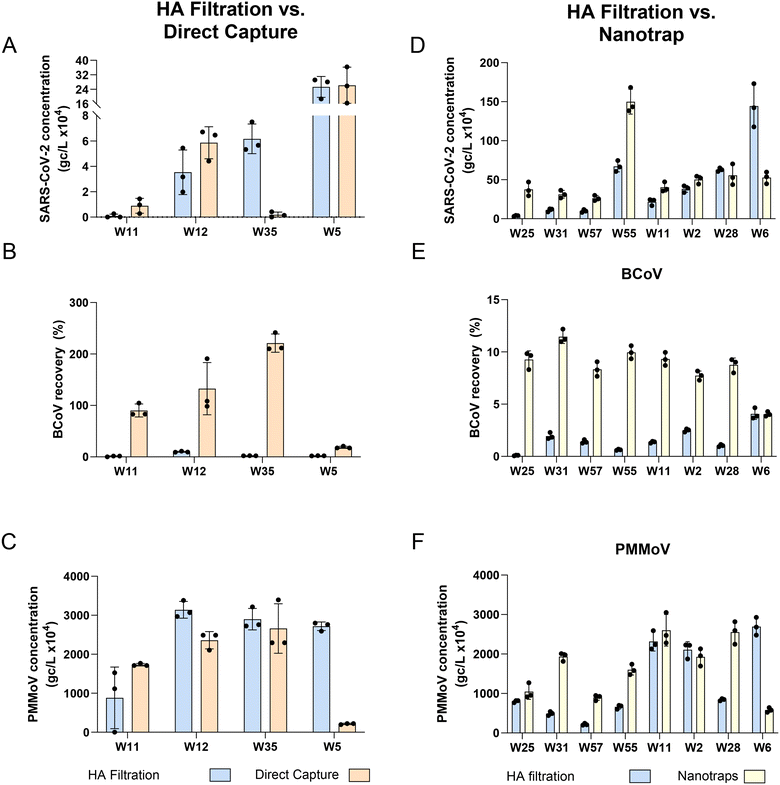 |
| Fig. 1 Comparison of virus quantification between HA filtration, direct capture, and Nanotrap®. Wastewater influents from the POTWs were processed using HA filtration (HA) vs. direct capture (DC) (panels A–C) or HA vs. Nanotrap® (NT) (panels D–F). SARS-CoV-2 concentration, gene copies per liter (A and D), BCoV percent recovery (B and E), and PMMoV concentration, gene copies per liter (C and F) were quantified by RT-qPCR. Each dot represents a PCR technical replicate and error bars represent the standard deviation of those replicates. | |
3. Results and discussion
A summary of the method comparisons performed in this study is presented in Tables 2 and S6.†
3.1 Quality control outcomes
Negative and positive controls were included on each qPCR and dPCR plate for each viral target. All positive controls met acceptance criteria, i.e., within 35% of the expected amount. Negative controls, including at least one non-template control (NTC), one method blank, and one extraction blank per plate, were all below detection. Recovery of spiked BCoV was assessed in all methods and was one of several metrics used in evaluating method performance. The range of BCoV recovery varied between concentration methods evaluated, HA filtration (0–17%), PEG (1–12%), Nanotrap® (NT) (4–13%) and direct capture (DC) (17–241%). When inhibition was detected, samples were re-quantified using a dilution (usually 1
:
2 or 1
:
5), the dilution of the sample resolved inhibition within the sample for quantification.
3.2 Virus capture/concentration methods
3.2.1 Concentration methods comparisons: HA compared with DC and NT.
Establishing a larger, more robust, wastewater surveillance program requires implementing methodological efficiencies to both increase the sensitivity and decrease the turnaround time, maximizing the utility of WBS data for public health. Here, we compared the viral concentration methods of HA filtration (HA) with two newer virus capture technologies: (a) vacuum-supported direct capture (DC), an on-column virus concentration approach from Promega and (b) affinity-binding Nanotrap® Microbiome A Particles (NT) from Ceres Nanosciences. To enhance the applicability of our findings, knowing that method's performance can be affected by different matrices,30–32 we chose influents from varied POTWs, as summarized in Table S7.†
For SARS-CoV-2, the DC method resulted in concentrations 3.3× higher on average than HA filtration (3 of 4 wastewater samples exhibited similar or higher levels) while the Nanotrap® method resulted in concentrations 2.8× higher on average than HA filtration method (7 of 8 wastewater samples exhibited similar or higher levels) (Fig. 1 and Table 2). None of the meta-data (or sewershed characteristics) associated with W35, the lone facility exhibiting lower recovery with DC compared to HA, were unusual, except for a small size of the population served. Similarly, the W6 facility exhibiting lower recovery with NT compared with HA filtration was a relatively small sewershed (10
000) with fairly normal metadata, except for a 10% industrial contribution (Table S7†). Using a linear regression model to determine if there is any significant difference in viral quantification between methods showed that the concentrations of the DC method were not significantly higher for SARS-CoV-2 (HA vs. DC: p-value = 0.78, Fig. 1A; HA vs. NT: p-value = 0.179, Fig. 1D). In contrast, for both the spiked-in internal control, BCoV, and the endogenous fecal marker, PMMoV, we observed a significant difference between the HA and DC protocols (linear regression model: BCoV p-value < 0.001, DC > HA, Fig. 1B and PMMoV p-value < 0.05, HA > DC, Fig. 1C), and between HA and NT protocols (BCoV p-value < 0.0001, NT > HA, Fig. 1E and PMMoV p-value < 0.05, NT > HA, Fig. 1F). Similarly to our findings, a recent study compared Nanotrap concentration with membrane filtration (HA) and found that concentrating a relatively small volume of wastewater for SARS-CoV-2 (10 mL) provided higher N1 concentration compared to membrane filtration of a 150 mL sample.33 To our knowledge no studies have compared the DC method with HA, however DC has been compared to other methods (PEG, concentrating pipette, adsorption–precipitation) and found to perform similarly if not better for the detection of SARS-CoV-2.34,35
We observed that only a very small fraction of our internal control BCoV was captured by the HA filtration method (range = 0.0 to 11.2% and median = 1.7%), an observation consistent with other studies (e.g., ref. 19, 36 and 37). Low surrogate viral recoveries have also been observed in published studies of other concentration methods, e.g., ultrafiltration and PEG.38,39 Therefore, the use of BCoV to “correct” recoveries of SARS-CoV-2 may not be appropriate. BCoV recovery, however, may still be used as part of a quality assurance program to identify matrices that may contribute to inhibition or method failures. Interestingly, while the NT method increased BCoV recovery several fold on average, the DC method resulted in over 100% recoveries. Further experiments would be needed to confirm these findings, but they could be the result of several mechanisms working together, including the impact of uncertainties associated with the process of BCoV titer quantification (see ESI† for details) and the DC method's ability to remove inhibitors from the samples. While BCoV failed to parallel SARS-CoV-2 sample recovery trends with these methods, indicating it is likely a poor surrogate to use, PMMoV sample recovery trends were more consistent with SARS-CoV-2 outcomes. This indicates that PMMoV may be a better control for assessing method performance alongside SARS-CoV-2 in surveillance protocols.
3.2.2 Concentration methods comparisons: HA compared with PEG.
The performance of PEG precipitation, a traditional viral concentration method, was compared with HA filtration using raw wastewater samples collected in late August of 2022 from three different Wisconsin POTWs (Fig. 2, Tables 2 and S7†): two sample replicates were processed with each concentration method and quantified by RT-qPCR for viral targets in triplicate. PEG processing resulted in higher concentrations for two of the three samples compared to HA filtration for all targets, however, the differences were not significant based on a paired t-test (p-value = 0.31, SARS-CoV-2, p-value = 0.25 BCoV and p-value = 0.22, PMMoV). Multiple studies have reported that viral recoveries from wastewater using the PEG method can vary substantially, including Pecson et al., 2021 who documented that the recovery efficiency of the entire process can vary by orders of magnitude for a given sample, depending on the specific implementation of the procedure such as inclusion of solids, pasteurization, and method of concentration.19,21,39 We have shown that NT, DC and PEG all performed relatively well at recovering viral targets from wastewater (summarized in Table 2). BCoV recoveries were significantly improved with the DC and NT concentration methods in comparison to HA. Similarly, SARS-CoV-2 and PMMoV typically returned higher concentrations with the DC and NT, but the outcome was also matrix-dependent, underscoring the importance of comparing multiple wastewater matrices in such studies. A summary of the advantages and disadvantages of these methods is presented in Table 1 and summary of wastewater sample characteristics is listed in Table S7.†
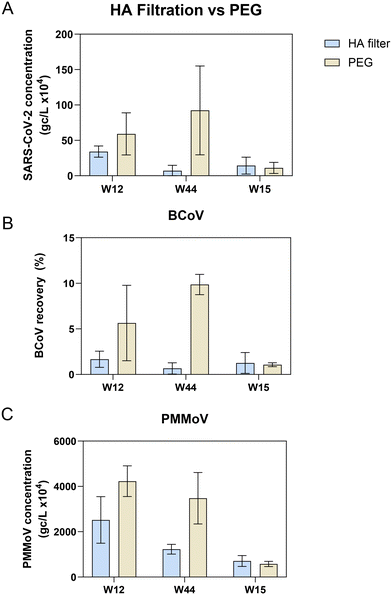 |
| Fig. 2 Comparison of virus quantification between HA filtration and PEG. Two concentration replicates were processed for each POTW sample and method. SARS-CoV-2 gene copies per liter (A), BCoV percent recovery (B), and PMMoV gene copies per liter (C) were quantified by RT-qPCR in triplicate. Error bars depict the standard deviation between the samples and PCR replicates (n = 6). | |
Table 1 Summary of concentration methods compareda
Method |
Pros |
Cons |
Synthesis of information from the following references: 11, 13, 14, 15–18, 33, 35, 39, 40 and 41.
|
Membrane filtration |
Quick processing time |
Manual operation, no automation available |
Variable input volume per filter |
Turbid samples may cause clogging; reduce volume filtered |
Easy to implement |
Requires homogenization which can effect some downstream processes (sequencing) |
Size selection bias, may require sample or filter amendments for viral capture |
|
PEG |
An inexpensive method |
Sample batch size can be limited by centrifugation step |
Able to concentrate all pathogens. No size or interaction selection bias |
Manual operation, no automation available |
|
Direct capture |
Capable of concentrating intact virions, partially intact virions and free nucleic acids |
Manual operation, no automation available |
Easy to implement; kit from vendor provides concentration to RT-PCR method |
Cumbersome to scale up in volume of sample concentrated and through-put |
|
Nanotrap Microbiome A Particles |
Automated protocol available |
Batch size limited by equipment available |
Compatible for multiple molecular analyses (PCR, sequencing, immunoassays) |
Particles have varying binding affinities for different pathogens. Some pathogens bind better with Microbiome A Particles over other particles available |
Table 2 Summary of different concentration, extraction, and quantification approaches tested
Concentration |
Extraction |
Quantification |
Figure |
Ranking based on: |
SARS-CoV-2 recovery |
Inhibition |
Throughput |
+ marks weak rating, ++ marks acceptable rating, +++ marks good rating. |
A. Concentration step approach focus |
HA filtration (Millipore) |
Maxwell® HT Environmental TNA kit (Promega) |
RT-qPCR |
Fig. 1
|
++ |
++ |
++ |
Direct capture (Qiagen) |
+++ |
++ |
+ |
|
B. Concentration step approach focus |
HA filtration (Millipore) |
Maxwell® HT Environmental TNA kit (Promega) |
RT-qPCR |
Fig. 1
|
++ |
++ |
+ |
Nanotrap® (Ceres) using Classic KF Apex program |
++ |
++ |
+++ |
|
C. Concentration step approach focus |
HA filtration (Millipore) |
Maxwell® HT Environmental TNA kit (Promega) |
RT-qPCR |
Fig. 2
|
++ |
++ |
++ |
PEG |
++ |
+ |
+ |
|
D. Nanotrap protocol detail focus |
Nanotrap® (Ceres) using Classic KF Apex program |
Maxwell® HT Environmental TNA kit (Promega) |
RT-dPCR |
Fig. S3† |
++ |
++ |
+++ |
Nanotrap® (Ceres) using Short KF Apex program |
+ |
+ |
+++ |
|
E. Extraction step approach focus |
HA filtration (Millipore) (focus on extraction kit comparison) |
RNeasy PowerMicrobiome kit (Qiagen) – column-based kit |
RT-qPCR |
Fig. S4† |
+ |
++ |
+ |
Maxwell® HT Environmental TNA kit (Promega) – paramagnetic bead-based kit |
+++ |
++ |
+++ |
PEG (focus on extraction kit comparison) |
RNeasy PowerMicrobiome kit (Qiagen) – column-based kit |
+ (except when samples are not bead-beated) |
+ |
+ |
Maxwell® HT Environmental TNA kit (Promega) – paramagnetic bead-based kit |
+++ |
+ |
++ |
|
F. Extraction step approach focus |
Nanotrap® (Ceres) using Classic KF program (focus on extraction kit comparison) |
MagMax Viral RNA isolation kit (ThermoFisher) – lysis: 65C, 5 min total mixing |
RT-dPCR |
Fig. 5
|
+++ |
++ |
++ |
NucleoMag DNA/RNA Water kit (Macherey-Nagel) – lysis: no heating step, 10 min total mixing |
++ |
+ |
++ |
Maxwell® HT Environmental TNA kit (Promega) – lysis: 85C, 35 min total mixing |
++ |
++ |
+++ |
HA filtration (Millipore) |
Maxwell® HT Environmental TNA kit (Promega) |
RT-qPCR |
Fig. 6
|
+++ |
++ |
++ |
RT-dPCR |
Fig. 6
|
++ |
++ |
++ |
HA filtration (Millipore) |
Maxwell® HT Environmental TNA kit (Promega) |
RT-qPCR |
Fig. 7
|
++ |
++ |
++ |
Nanotrap® (Ceres) using Short KF program |
RT-dPCR |
Fig. 7
|
+++ |
++ |
+++ |
3.3 Concentration methods: sensitivity to specific implementation parameters and implications for wastewater surveillance practice
In this section, we discuss the outcomes of experiments designed to test the influence of key implementation parameters within the wastewater capture/concentration methods on sensitivity and precision of viral quantification. In subsection 3.3.1, we address important variables within the HA filtration method (filter pore size, MgCl2 addition, filtration flow rate, and volume of influent sample filtered). In subsection 3.3.2, we critically examine the influence of bead beating parameters on viral quantification. Finally, in subsection 3.3.3, we compare two NT automation protocols, focusing on sensitivity and inhibition.
3.3.1 Influence of filtration parameters on viral quantification by the HA method.
Membrane filtration recovery of viruses, in theory, relies less on selection by size, but primarily on interaction of the virus particles or suspended colloids and particles with the filter by charge or electrostatic interactions (reviewed in Junter and Lebrun, 2017 and Mix, 197442,43). In neutral pH wastewater, SARS-CoV-2 virions are negatively charged.44 Commonly used cellulose-based filters (e.g., mixed cellulose ester or cellulose nitrate) are negatively charged and chemical modification of the filter and/or solution matrix is often required to allow adsorption of SARS-CoV-2 onto the filter, this method is regularly referred to as electronegative filtration.45,46 In practice, therefore, electronegative filtration usually involves either lowering the pH of the sample to impart a positive electrical double layer around the virus, and/or addition of MgCl2 to aid attachment of virus particles via salt-bridging, formation of flocs, and alteration of the charge of filter.40,45
To determine the influence of several membrane filtration implementation parameters on SARS-CoV-2 recovery and assess what suite of options might be best suited for wastewater surveillance, we performed a series of experiments. We compared two filter pore sizes, 0.8 μm and 0.45 μm, and did not find a significant difference in recovery of SARS-CoV-2 between the two pore sizes (data not shown) thus we elected to use a 0.8 μm HA filter in our wastewater experiments due to faster processing times. We also evaluated several approaches (acidification, MgCl2 addition, and soaking of the filter in 25 mM MgCl2) for enhancement of virus adsorption from wastewater to HA filters (methods detailed in ESI†). We found that there was no significant difference between SARS-CoV-2 viral recoveries with either filter treatment (dry filter vs. soaking in 25 mM MgCl2) or sample matrix alteration (acidification to pH 4.0 and or addition of MgCl2 to a final concentration of 25 mM) (Fig. S1†). Using BCoV, a study by Vadde et al., 202247 showed similar outcomes using treatment conditions comparable to those employed in this study, and their group opted to run membrane filtration with no treatment of the filter or sample. Similarly, Ahmed et al., 2020,40 observed that membrane filtration with no pretreatment or the addition of MgCl2 at a final concentration of 25 mM recovered more murine hepatitis virus than if the wastewater was acidified (though acidification resulted in less variability across sample replicates). These studies and ours suggest that mechanisms of viral capture by cellulose-based filters from wastewater may not be dominated by the charge-based filter interactions outlined previously. Instead, it may be through another mechanism, such as filter collection of viruses associated with colloids and particles in the wastewater. Further testing is needed, but this proposed alternate hypothesis would explain why particle-free solutions, e.g., method blanks, tend to see much poorer recovery for many viruses.
To our knowledge, little has been published on the effect of sample flow rate on virus recovery in HA filtration. To evaluate the effect of flow rate on measured SARS-CoV-2, BCoV and PMMoV concentrations, we varied vacuum strength to control the flow rate during filtration of influent samples. We filtered 25 mL replicate volumes of influent at three different flow rates: 5.6 mL per minute as slow (n = 3), 22.2 mL per minute as moderate (n = 20), and 49.9 mL per minute as fast (n = 3). The recovery of SARS-CoV-2, BCoV, and PMMoV are shown in Fig. 3A–C. The slower flow rate recovered significantly more (45%) SARS-CoV-2 compared to both the moderate and fast flow rates (one-way ANOVA, Tukey post hoc, p-value < 0.0001, F value (2, 74) = 4.761, Fig. 3A). The 14% higher recovery of PMMoV at the slower flow rate was also significant compared to the fastest flow rate (one-way ANOVA, Tukey post hoc, p-value < 0.001, F value (2, 75) = 0.8817, Fig. 3C). However, this slowest speed outcome was not significantly different compared to the moderate speed results. In contrast to both SARS-CoV-2 and PMMoV, recovery of the spiked recovery control (BCoV) was insensitive to flow rate, with similar recoveries observed at all examined flow rates (Fig. 3B).
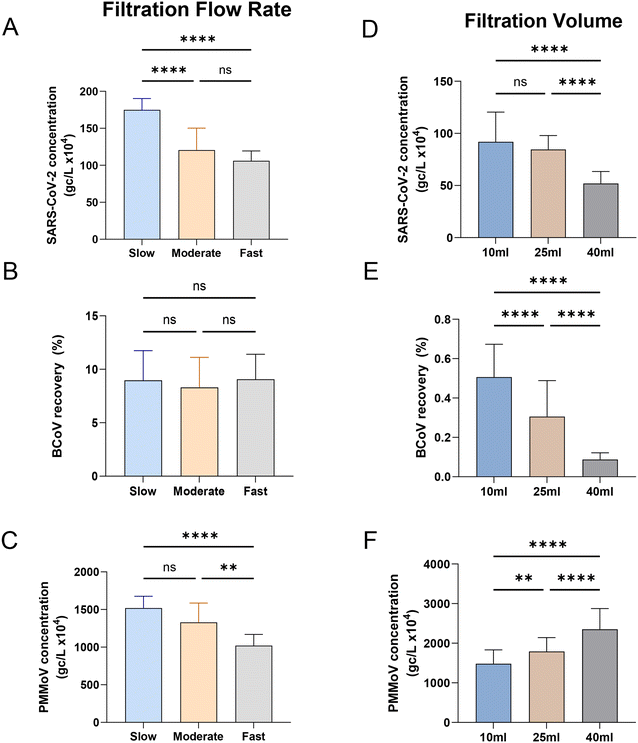 |
| Fig. 3 HA filtration: influence of filtration flow rate and volume on viral target quantification. Panels A–C: Sub-samples of the same wastewater were filtered under three different flow rates: slow – 5.6 mL min−1 (n = 3), moderate – 22.2 mL min−1 (n = 20), and fast – 49.9 mL min−1 (n = 3). Panels D–F: Different volumes of one wastewater sample were filtered at the same flow rate (n = 9). SARS-CoV-2, gene copies per liter (A and D), BCoV, percent recovery (B and E) and PMMoV, gene copies per liter (C and F), were quantified by RT-qPCR. Error bars depict standard deviation of technical replicates. Number of asterisks for each comparison indicates the significance of the Tukey post hoc tests (one-way ANOVA): P-values = **** < 0.0001, *** < 0.001, ** < 0.01, * < 0.05, ns: not significant. | |
The influence of the quantity of influent concentrated by HA filtration on virus concentration was also evaluated by filtering replicate amounts of varying volumes (Fig. 3D–F). Calculated concentrations of both SARS-CoV-2 and BCoV were significantly higher when smaller volumes were filtered, 1.8 times and 5.8 times higher on average, respectively (when 10 mL is compared to 40 mL; one-way ANOVA, Tukey post hoc, p-value < 0.001, F value (2, 78) = 5.143, Fig. 3D and F value (2, 78) = 14.51, Fig. 3E). In contrast, PMMoV exhibited the opposite trend, with calculated concentrations increasing with the volume filtered. Filtration of 25 mL generally resulted in recoveries between that measured for 10 mL and 40 mL, for all viral targets. The contrasting PMMoV outcomes suggest that the primary mechanism of PMMoV capture on the membrane filter is dissimilar to that driving SARS-CoV-2 capture or much less influenced by that process. For example, it is conceivable that PMMoV filter capture is dominated by colloid/particle interactions and that as these materials accumulate on the filter with increased sample volume, PMMoV capture efficiency is improved. Several studies have also noted an improved quantitation of viral targets with reduced filtration volumes,20,41 with several possibilities including a reduction in concentrated inhibitors, saturation/occlusion of adsorption sites as the amount filtered increases, and potential differences in the filtration speed/processing time.
The filtration speed had been shown to affect SARS-CoV-2 measurements in a study by Hayes et al., 2022,48 where adsorption of a virus surrogate for SARS-CoV-2 improved with increasing filter contact time with an HA filter. PMMoV, the endogenous fecal marker behaved similarly to SARS-CoV-2 in these experiments, suggesting that it could be used to correct for speed-based filtration biases. BCoV recovery was insensitive to flow rate in our experiments rendering it a nonviable control for normalization of filtration speed-based biases. Similar outcomes were reported by Jafferali et al., 202149 who compared ultrafiltration and membrane filtration methods, measuring SARS-CoV-2, BCoV and PMMoV, and found that PMMoV as an internal standard is sufficient to determine relative recovery and normalize between samples in contrast to BCoV.
3.3.2 Influence of bead beating parameters on viral quantification.
After concentration of the raw wastewater by HA filtration, disruption and lysis of the filter-collected virus cells via bead beating is typically required prior to extraction of nucleic acids. Bead beating is applied in a broad range of microbial cell extraction methods making our findings potentially relevant to other viral targets. Moreover, it is also critical for nucleic acid recovery in many bacterial and fungal extraction protocols. However, it is less clear whether our findings are directly applicable to those targets. To assess the impact of energy imparted during bead beating of filters on viral target recovery, we compared four different bead beating protocols which incorporated specific combinations of the following parameters: speed (vortexing for 30 seconds or bead bashing at a rotor speed of 4 m s−1 or 7 m s−1 in the MP Bio FastPrep-24 5G), duration (30 seconds or 90 seconds) and the use of two different centrifugation speeds, 9168 × g (Fig. 4 and Table 2) and 20
627 × g (Fig. S2†) applied after bead beating in the Lysing Matrix A tubes. A one-way ANOVA with multiple comparisons (Tukey's post hoc) was used to determine the significance indicated in Fig. 4 and S2.† Centrifugation speed had minimal impact on quantitation for all virus targets, compared to the influence of bead beating speed and duration, i.e., the patterns of viral quantification observed in Fig. 4A–C (speed of 9168 × g) were similar to those in Fig. S2A–C† (speed of 20
627 × g). For all targets, the protocol that resulted in the highest virus quantification was bead beating at a rotor speed of 7 m s−1 for 90 seconds (the protocol with the greatest energy input), while simply vortexing (the protocol with the lowest energy input) returned the lowest viral concentrations (Fig. 4A and C). This finding was somewhat surprising, since RNA is considered a more labile (energy vulnerable) nucleic acid. However, it has been shown that SARS-CoV-2 RNA preferentially associates with solids50–52 and the longer time and higher speed of bead beating may be necessary to facilitate effective extraction of the RNA from the solids. This characteristic may also explain the pattern of PMMoV quantities across the different protocols, which was nearly identical to that of SARS-CoV-2, with protocol 4 (90 seconds of bead bashing at 7 m s−1 speed) showing the highest concentrations (Fig. 4C). In contrast, the recovery of the exogenous BCoV spike was essentially insensitive to bead bashing variables (Fig. 4B). It should be noted that the relationship between PMMoV and SARS-CoV-2 observed here was also present in filtration flow rate experiment (Fig. 3A) suggesting that PMMoV could in some cases serve as an effective processing control, while BCoV would be less suitable, particularly in HA filtration methods.36
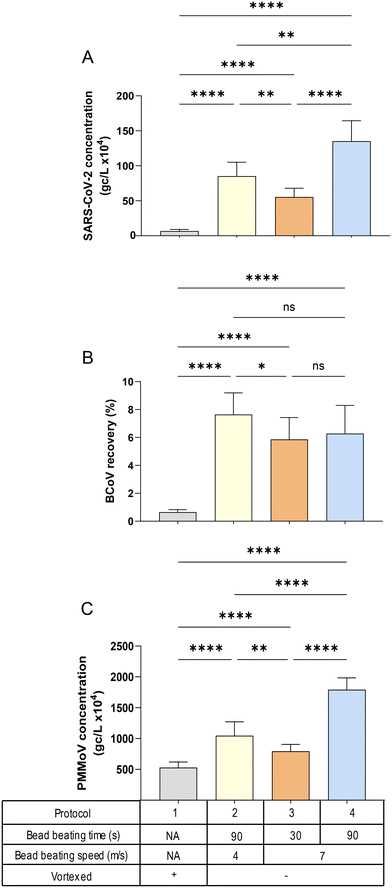 |
| Fig. 4 Influence of bead beating parameters on viral target quantification. Effect of bead beating duration and speed, performed prior to centrifugation at 9168 × g and supernatant extraction. Viral targets SARS-CoV-2, gene copies per liter (A), BCoV percent recovery (B), and PMMoV, gene copies per liter (C) were quantified by RT-qPCR. Each protocol tested had six replicate filters except protocol 2, with three replicate filters. Bar height represents means, while error bars depict the standard deviations. Symbols above groups indicate the significance of the Tukey post hoc tests (one-way ANOVA): P-values = **** < 0.0001, *** < 0.001, ** < 0.01, * < 0.05, ns: not significant. | |
3.3.3 Nanotrap® Microbiome Particle method: specific protocol implementation and effect on inhibition.
Unlike the HA filtration and PEG methods, which are challenging to automate and scale-up, the Nanotrap® Microbiome Particle concentration approach can be automated, allowing for a faster turnaround time and higher throughput. To minimize the sample processing time, the company that developed the Nanotrap® method modified the “classic” automated KingFisher programs to shorten certain steps within the method and promulgated a “short” protocol. We compared the performance of two Nanotrap® concentration protocols, the classic protocol (at ∼58 minutes total length) and short concentration protocol (∼33 minutes, further details in the ESI† Methods), focusing on virus (SARS-CoV-2, PMMoV and BCoV) concentration and inhibition performance metrics from eight raw wastewater samples (Fig. S3†). For SARS-CoV-2 and PMMoV, the measured concentrations correlated well between the two protocols (Pearson correlation, r = 0.97, R2 = 0.93, p-value < 0.001, Fig. S3A† and, r = 0.87, R2 = 0.75, p-value < 0.01 respectively, Fig. S3C†). For BCoV, the correlation between the classic and short protocols was of R2 = 0.50, (p-value < 0.05, Pearson correlation, r = 0.71, Fig. S3B†). In assessing inhibition by the BRSV assay, we observed a notable difference in inhibition handling between the long and short NT protocols. The number of samples exhibiting inhibition was much greater (five of the eight samples tested) using the short protocol, compared with the classic protocol (none were inhibited) (Fig. S3D†). Investigation of the differences between the protocols revealed that a longer period was allowed for collection of the magnetic beads after mixing in the long protocol version (3 counts for 3 seconds in the short protocol vs. 5 counts for 3 seconds in mixing steps or 5 seconds in the elution step). This change, while seemingly minor, may allow for improved particle separation from the wastewater at each step of the protocol, carrying over less matrix and thus less inhibitors into extraction. An additional difference is present in the pause step between mixes, the long protocol will pause from mixing for 105 seconds while the short protocol will pause for 45 seconds. The longer pause may allow for enhanced interaction of Nanotrap® particles with the viral particles. These differences combined with the longer bead collection steps are likely contributing to the reduced frequency of observed inhibition. Further experiments focused on inhibition may be required to fully understand the mechanisms that result in inhibition contrasts between the two protocols. With the improved inhibition handling observed with the long protocol, an NT automation approach with longer collection steps is recommended for routine use.
3.4 Extraction method evaluation
Extraction of nucleic acids is the second key laboratory step in processing wastewater for pathogen surveillance and can have a major impact on the performance and robustness of results obtained from molecular assays.53–55 In general, extraction of nucleic acids can be performed with either a column-based or paramagnetic bead-based method. Lewandowski et al. compared a column kit to automated bead-based kits using Hazara virus and found that at higher viral loads (106 gc per L) the recovery of RNA measured by RT-qPCR was similar. However, at lower viral concentrations (104 gc per L), they saw more variability in viral recovery particularly when TritonX-100 was used in the extraction method, though inhibition was not assessed in this study.53 Verheyen et al., 201254 found that in comparing automated extraction kits, each kit gave comparable RT-qPCR results; however, inhibitory substances in more complex samples such as stool impaired the quantitation.
To assess and compare the performance of both column and bead-based methods for SARS-CoV-2 one raw wastewater sample collected in July 2021 was concentrated in duplicate either by HA filtration or PEG and extracted with RNeasy PowerMicrobiome (a column-based kit) and Maxwell HT Environmental TNA kit on the KingFisher platform (a paramagnetic bead-based kit). SARS-CoV-2 quantitation of each replicate was carried out in triplicate by RT-qPCR. Samples extracted with the automated paramagnetic bead-based kit showed substantially higher amounts of SARS-CoV-2 compared to the manual extraction with the column-based kit (Fig. S4,†p-value < 0.001 for all extraction kit comparisons with the exception of the non-bead beated PEG samples, p-value = 0.89 using a one-way ANOVA with multiple comparisons, Tukey post hoc). Since the automated paramagnetic bead-based extraction using the Maxwell HT Environmental TNA kit out-performed the manual on-column approach in our hands, we further sought to identify the relative performance of several other paramagnetic bead-based kits on the KingFisher Flex platform for extraction of viral targets in wastewater surveillance. Using raw wastewater collected in September 2022 from POTWs across Wisconsin, concentrated by Nanotrap® particles, we compared the performance of three paramagnetic bead extraction kits: MagMax Viral, NucleoMag DNA/RNA and HT Environmental TNA (Fig. 5). Substantially higher SARS-CoV-2 concentrations were measured across the wastewater samples when extracted using the MagMax Viral (on average 43.5% higher) or HT Environmental TNA (on average 39.5% higher) kits compared to the NucleoMag DNA/RNA kit (Fig. 5A). Spiked-in BCoV recovery varied more widely both between the kits and across samples (Fig. 5B). PMMoV recovery was much more similar across all three kits with samples prepared with the MagMax and Environmental TNA kits returning somewhat higher PMMoV concentrations than those prepared with the NucleoMag kit (Fig. 5C). Additionally, we noted four of the ten samples exhibited inhibition using the BRSV assay with the NucleoMag kit compared to the other two kits tested.
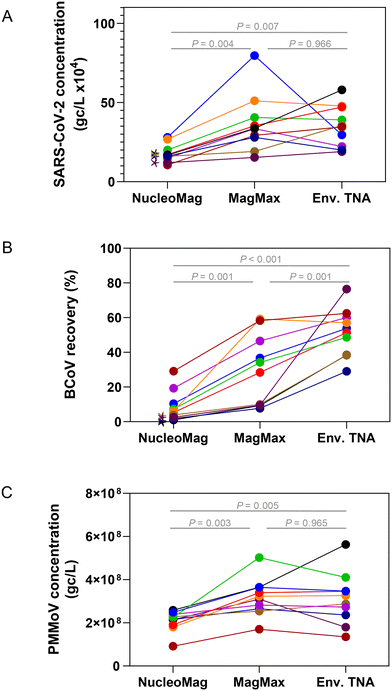 |
| Fig. 5 Comparison of virus quantification from three magnetic bead based automated extraction kits. Virus quantity extracted from ten different POTW influents when using either MagMax Viral, NucleoMag DNA/RNA water, or Promega Environmental TNA extraction kit. RT-dPCR was used to quantify SARS-CoV-2, gene copies per liter (A), BCoV, percent recovery (B) and PMMoV, gene copies per liter (C). All extractions were performed on a Kingfisher Flex automated system. SARS-CoV-2 and BCoV were tested at 1 : 2 dilution. The asterisks indicate samples that were inhibited at this dilution. Data points are single dPCR reps. | |
Extraction methods/kits generally incorporate three steps, 1) cell lysis by mechanical, chemical and/or enzymatic means, 2) cell debris and impurity removal, and 3) nucleic acid isolation. The lysis step is implemented differently in the three magnetic bead-based kits; with the kit protocol differences focused primarily on the mechanical extraction steps on the KingFisher Flex platform. The MagMax Viral kit heats at 65 °C in the lyse and bind step along with a 5-minute fast mix. The HT Environmental kit heats at 85 °C in the lyse and bind steps along with a series of mixing steps (for a total of 30 minutes between medium mixing and pausing). The NucleoMag kit does not incorporate heating in the initial lysis and bind step, it performs a loop-based mixing at 30 seconds intervals between mixing and pausing. These variations can affect lysis and inhibitor removal efficiency, as previously reported55 and likely explain differences in viral target quantities observed in Fig. 5. We hypothesize that heating during the lyse steps contributes to better lysis56 but further studies are required to confirm this. Based on these results an automated paramagnetic bead-based extraction utilizing a heating step during lysis such as employed in the MagMax Viral or HT Environmental kit may perform the best for viral nucleic acid recovery from concentrated raw wastewater for quantitative PCR.
3.5 PCR method comparison: RT-qPCR versus RT-dPCR
We directly compared, using the same TNA preparation, the quantified amount and precision of RT-qPCR and RT-dPCR for SARS-CoV-2, BCoV and PMMoV (Fig. 6). The TNA was obtained through viral concentration by HA filtration, homogenization by bead beating (method section 2.2.1), and extraction using the Promega Maxwell® HT Environmental TNA kit on the Kingfisher Flex automation platform (method section 2.3.1). Paired t-tests indicated significant differences between the concentrations determined on the two PCR platforms for all targets (p-value < 0.01). The levels of SARS-CoV-2 (average of N1 and N2 target) were significantly higher (4.4× higher on average) in qPCR compared with dPCR (Fig. 6A). However, qPCR and dPCR measurements obtained for the virus recovery control (BCoV) were more similar (Fig. 6B). Several published studies corroborate our findings that RT-qPCR quantification may return higher concentrations of SARS-CoV-2 targets in comparison to the RT-dPCR in wastewater applications (e.g., Hinkle et al., 202257). The difference may be associated with circular plasmid standards overestimation (Hou et al. 2010),58 directly affecting the estimated N1 and N2 RT-qPCR concentrations. PMMoV outcomes, like those of SARS-CoV-2, showed a striking difference between qPCR and dPCR (Fig. 6C); however, the observed difference was in the opposite direction to that of SARS-CoV-2, with dPCR data on average 6-fold higher than qPCR. This may be due in part to the fact that, unlike SARS-CoV-2 standards on qPCR (circular plasmid), DNA ultramers (linear ssDNA) were used as PMMoV standards. Moreover, the extremely high abundance of the PMMoV template in wastewater extracts, can lead to underestimation of target quantity in the RT-qPCR reaction, i.e., PCR components can become rate-limiting during PCR reaction. For this reason, along with the potential for saturation of the fluorescent signal on the nanoplates, samples for PMMoV measurement were diluted 1
:
10 prior to RT-dPCR quantification. It should be noted that a similar dilution of template prior to the PMMoV quantification via qRT-PCR resulted in only a minor increase in the levels of PMMoV measured (less than 10%; data not shown), thus suggesting that the dPCR technology may be better suited to quantify abundant targets (assuming that template levels can be adjusted for dPCR quantification). Combined with the fact that the dPCR demonstrated more than an order of magnitude lower LOD and LOQ values (Table S3†), we conclude that the Qiagen's dPCR platform can provide improved performance over a wider range of template concentrations compared to qPCR.
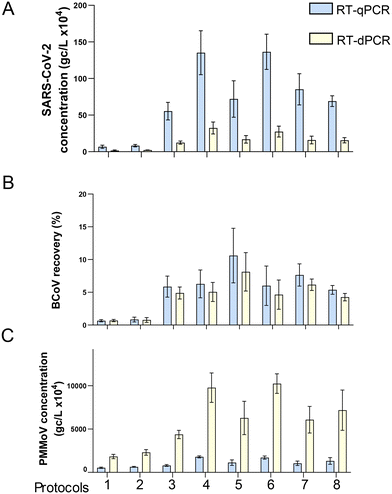 |
| Fig. 6 Comparison RT-qPCR and RT-dPCR for three viral gene targets. One sample was processed using different HA filtration protocols (see Table S6†). The same RNA extracts were then quantified by both RT-qPCR and RT-dPCR for SARS-CoV-2, gene copies per liter (A), BCoV percent recovery (B), and PMMoV, gene copies per liter (C). Error bars shown are the standard deviation of technical replicates (n = 6, except protocols 2 and 6, n = 3). | |
Quantification accuracy of dPCR may also be enhanced over qPCR due to the inherent lack of standard curve bias (dPCR does not require standard curves), and improved performance with complex/inhibited matrices.59 Most studies to date comparing the dPCR and qPCR technologies have employed BioRad droplet digital PCR (ddPCR) with data indicating that this digital technology variant can be superior to qPCR, providing improved signal to noise, resulting in lower LODs, and less susceptibility to inhibition, especially in samples with a low-level target or challenging matrix.23,60 Recent studies comparing the Qiacuity RT-dPCR platform and RT-qPCR confirm our results, indicating that the digital technology results in a significantly better signal to noise (lower LOD).22,57 The published data on relative inhibition handling of the two platforms in wastewater application is more mixed. Ahmed et al., 202222 reported that dPCR was more resistant to inhibition; however, D'Aoust et al., 2021 reported the opposite in a primary sludge application.61 Rački et al., 201459 reported similar levels of inhibition in qPCR and dPCR analysis of PMMoV. Even though traditional qPCR assays can provide adequate sensitivity and comparable reproducibility to dPCR with careful experimental design and execution (reviewed by Taylor et al., 2019), there are some target nucleic acid ranges, sample types and parameters that are challenging for qPCR.62 More importantly, the universal adoption of dPCR platform could help with cross-laboratory comparisons of WBS data, given the inherent lack of a standard curve bias.
3.6 Side-by-side comparison of two complete processing methods
In 2020, we implemented HA filtration (HA) concentration with RT-qPCR to quantify SARS-CoV-2 levels from wastewater. Due to the lack of sensitivity for SARS-CoV-2, the RNA degradation (which was an issue for sequencing), and the poor throughput, we decided to switch to a more satisfactory workflow, i.e., Nanotrap® Microbiome A particle (NT) concentration with RT-dPCR. During the transition between the two workflows that took place near the end of the first Omicron wave, we processed a total of 229 wastewater samples from 45 POTWs across Wisconsin for three weeks using the two separate complete wastewater protocols: (1) HA/RT-qPCR and (2) NT/RT-dPCR (Fig. 7). For both concentration methods tested the same extraction protocol (Promega's Environmental TNA kit) was used since we did not observe that another kit substantially improved SARS-CoV-2 recoveries. Out of the 229 wastewater samples, six samples processed using the HA/RT-qPCR workflow had N1 or N2 concentrations below the limit of detection (LOD) and sixteen were below the limit of quantification (LOQ). In contrast, only two samples processed by NT/RT-dPCR were below the LOD and nine were below the LOQ, suggesting that the Nanotrap®/RT-dPCR approach, as implemented, was marginally more sensitive (improved signal/noise) resulting in improved N1/N2 signal recovery.
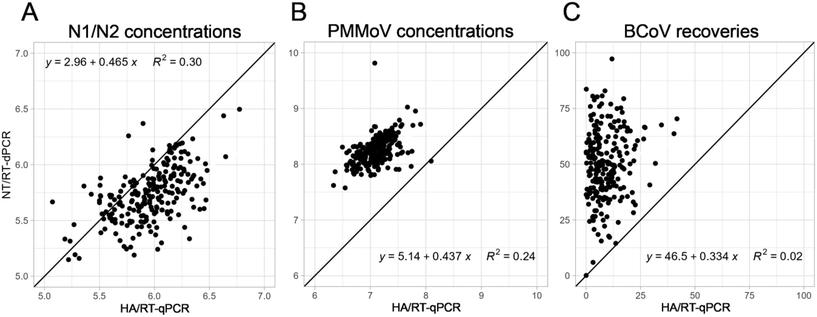 |
| Fig. 7 Comparison of three viral targets in two complete processing methods. Plots represent SARS-CoV-2 (A) and PMMoV (B) concentrations, gene copies per liter and BCoV (C) percent recovery for 229 wastewater samples processed by two common wastewater protocols: (1) concentration by HA filtration and quantified by RT-qPCR or (2) concentration by Nanotrap® capture and quantified by RT-dPCR. Black lines depict the 1 : 1 ratio. SARS-CoV-2 and PMMoV concentrations shown on log 10 scale. | |
SARS-CoV-2 concentrations obtained using the two complete approaches were moderately positively correlated (Spearman rank correlation coefficient, rho = 0.55, Fig. 7A). Concentrations obtained by HA/RT-qPCR were significantly higher (on average 3.6× higher) than the ones obtained using NT/dPCR (Wilcoxon paired-test, P < 0.001). Although the relative impact of the concentration and quantification factors could not be directly teased apart in this comparison, based on our previous experiments (Fig. 1, 2, and 6), we hypothesize that the difference was associated with the RT-qPCR estimating SARS-CoV-2 levels 4.4× higher than RT-dPCR (Fig. 6A). In an experiment designed to test this hypothesis, where we ran the same set of standards used to prepare standard curves for N1/N2 and PMMoV on both PCR platforms, we confirmed that qPCR was indeed overestimating SARS-CoV-2 target abundances. In contrast, in the concentration step, we observed that SARS-CoV-2 recoveries were overall better with NT than HA filtration (2.7× Fig. 1D and 1.5× Fig. 2A).
Interestingly, in this large methods comparison, we observed that the N1
:
N2 ratio was markedly improved with NT/RT-dPCR compared to HA/RT-qPCR (median ratios of 0.98 and 0.73, respectively). The difference in the N-ratio could be associated with the quantification method itself. Indeed, qPCR quantifies concentrations based on the fluorescence level emitted by the probe. Thus, mutations in the primer and probe sites could decrease the assay's sensitivity and lead to underestimating the target. However, dPCR concentrations are based on the number of positive and negative partitions, where the fluorescence intensity is less critical as long as the signal in positive partitions is high enough to be adequately distinguished from negative partitions. Consequently, the mutation at the 5′ end of the N1 probe in Omicron lineages (N:P13L) could potentially lessen the sensitivity of the N1 probe and decrease the fluorescence measured in the PCR reaction,63 having a stronger effect on RT-qPCR results than RT-dPCR. For comparison, during the Delta wave (where this mutation is not present), the average N1
:
N2 ratio obtained by qPCR in our statewide wastewater surveillance program was 1.23 (July–October 2021, n = 1341 samples).
Like SARS-CoV-2, PMMoV concentrations obtained using NT/RT-dPCR moderately correlated with the concentrations obtained using HA/RT-qPCR (rho = 0.52, Fig. 7B). Unlike SARS-CoV-2, the NT/RT-dPCR approach resulted in significantly greater virus concentrations (Wilcoxon paired-test, P < 0.001) with a signal 14.7× higher on average compared with the HA/RT-qPCR. This large difference could result from the enhanced recovery observed using NT compared HA (2.1× and 1.6×, Fig. 1F and 2C, respectively) coupled with the 5.1× higher PMMoV concentrations determined by dPCR compared with RT-qPCR (Fig. 6C). As previously discussed, the lower RT-qPCR concentrations could come from a bias induced by the standard material (linear dsDNA for PMMoV versus circular dsDNA for SARS-CoV-2), or resource limitation in the qPCR. Moreover, samples were diluted to 1
:
10 for RT-dPCR reactions to avoid saturating the signal, which inherently diluted potential inhibitors.
BCoV recovery was also dramatically and significantly higher using NT/RT-dPCR compared to HA/RT-qPCR (Wilcoxon paired-test, P < 0.001), with median recoveries of 49% and 7%, respectively (Fig. 7C). This large difference is most likely the result of the poor capture of this exogenous viral target by the filter membrane, as previously discussed. No clear correlation (rho = 0.15) between HA/RT-qPCR and NT/RT-dPCR was observed in the BCoV data, which further points to the problematic use of BCoV as a virus recovery control, particularly with HA filtration approaches.
4. Conclusion
Two years of wastewater surveillance has provided a wealth of information on the performance of several new virus processing protocols. The findings from our protocol and method comparisons provide important considerations for selection of monitoring approaches for SARS-CoV-2 and potentially other pathogens. Our study corroborates some findings from previously published method comparisons and presents new direct comparative assessments of protocols for three major viral wastewater processing steps; concentration, extraction and quantification. We document that the selection of virus concentration method, nucleic acid extraction protocol and PCR platform will each have substantive impacts on overall method performance and outcomes. However, it is important to keep in mind that a single workflow may not be best suited for all viral targets and our study provides a framework for comprehensive evaluation of workflows for new targets. In this study, we show that for most wastewater matrices the Nanotrap® concentration method provides enhanced viral recoveries, improves sensitivity, and is easily automated. For extraction, we find that a protocol that use of either the MagMax Viral or HT Environmental TNA paramagnetic bead kits will provide greater viral nucleic acid recovery when combined with the Nanotrap® concentration. In comparison with qPCR, our data show that quantitation with the dPCR platform will provide improved signal to noise and greater sensitivity, a finding that is likely to be relatively pathogen agnostic. This study also provides a detailed and broad-based assessment of the performance of a matrix spiked in virus (BCoV) for SARS-CoV-2 recovery control. We incorporated BCoV in the suite of different processing methods evaluated, providing a comprehensive evaluation of its utility, and overall, we find it lacking in many key aspects, and generally unsuitable for a matrix spiked recover control. From our findings, PMMoV may provide more utility as a matrix control for SARS-CoV-2 (and potentially other endogenous viruses) as it mimics SARS-CoV-2 trends throughout the analyses performed in this study.
Conflicts of interest
The authors have no conflicts to declare.
Acknowledgements
This work was supported by the University of Wisconsin–Madison, Office of the Vice Chancellor for Research and Graduate Education with funding from the Wisconsin Alumni Research Foundation, Award number AAH9335, WDHS COVID-19 WASTEWTR SURVEIL, DHS Grant Agreement No.: 435100-G20-COVID-19RES-02, WDHS ELC WASTEWATER SURVEILLAN, DHS Grant Agreement No.: 435100-G21-WASTEWATER-00, and WDHS ELC ENH DETECT EXPANSION, DHS Grant Agreement No.: 435100-G21-COVIDELC-00. We thank Jonathan Meiman, Ian Pray and Nathan Kloczko from Wisconsin DHS and Dr. Sandra McLellan's lab for insightful discussions and sharing of knowledge. We also thank the wastewater treatment operators who provide the influents for these studies.
References
- L. Heijnen and G. Medema, Surveillance of Influenza A and the pandemic influenza A (H1N1) 2009 in sewage and surface water in the Netherlands, J. Water Health, 2011, 9(3), 434–442 CrossRef CAS PubMed
.
- J. D. Trask, J. R. Paul and Technical Assistance of John T. Riordan, Periodic examination of sewage for the virus of poliomyelitis, J. Exp. Med., 1942, 75(1), 1–6 CrossRef CAS PubMed
.
- E. Zuccato, C. Chiabrando, S. Castiglioni, D. Calamari, R. Bagnati and S. Schiarea,
et al., Cocaine in surface waters: a new evidence-based tool to monitor community drug abuse, Environ. Health, 2005, 4(1), 14 CrossRef PubMed
.
- D. Polo, M. Quintela-Baluja, A. Corbishley, D. L. Jones, A. C. Singer and D. W. Graham,
et al., Making waves: Wastewater-based epidemiology for COVID-19 – approaches and challenges for surveillance and prediction, Water Res., 2020, 186, 116404 CrossRef CAS PubMed
.
- W. Ahmed, N. Angel, J. Edson, K. Bibby, A. Bivins and J. W. O'Brien,
et al., Cocaine First confirmed detection of SARS-CoV-2 in untreated wastewater in Australia: A proof of concept for the wastewater surveillance of COVID-19 in the community, Sci. Total Environ., 2020, 728, 138764 CrossRef CAS PubMed
.
- G. La Rosa, M. Iaconelli, P. Mancini, G. Bonanno Ferraro, C. Veneri and L. Bonadonna,
et al., Cocaine First detection of SARS-CoV-2 in untreated wastewaters in Italy, Sci. Total Environ., 2020, 736, 139652 CrossRef CAS PubMed
.
- W. Lodder and A. M. De Roda Husman, SARS-CoV-2 in wastewater: potential health risk, but also data source, Lancet Gastroenterol. Hepatol., 2020, 5(6), 533–534 CrossRef PubMed
.
- G. Medema, F. Been, L. Heijnen and S. Petterson, Implementation of environmental surveillance for SARS-CoV-2 virus to support public health decisions: Opportunities and challenges, Curr. Opin. Environ. Sci. Health, 2020, 17, 49–71 CrossRef PubMed
.
- S. P. Sherchan, S. Shahin, L. M. Ward, S. Tandukar, T. G. Aw and B. Schmitz,
et al., Cocaine First detection of SARS-CoV-2 RNA in wastewater in North America: A study in Louisiana, USA, Sci. Total Environ., 2020, 743, 140621 CrossRef CAS PubMed
.
- C. C. Naughton, F. A. Roman, A. G. F. Alvarado, A. Q. Tariqi, M. A. Deeming and K. F. Kadonsky,
et al., Cocaine Show us the data: global COVID-19 wastewater monitoring efforts, equity, and gaps, FEMS Microbes, 2023, 17(4), xtad003 CrossRef PubMed
.
- N. Alygizakis, A. N. Markou, N. I. Rousis, A. Galani, M. Avgeris and P. G. Adamopoulos,
et al., Cocaine Analytical methodologies for the detection of SARS-CoV-2 in wastewater: Protocols and future perspectives, TrAC, Trends Anal. Chem., 2021, 134, 116125 CrossRef CAS PubMed
.
- M. Hamouda, F. Mustafa, M. Maraqa, T. Rizvi and A. Aly Hassan, Wastewater surveillance for SARS-CoV-2: Lessons learnt from recent studies to define future applications, Sci. Total Environ., 2021, 759, 143493 CrossRef CAS PubMed
.
- X. Zheng, Y. Deng, X. Xu, S. Li, Y. Zhang and J. Ding,
et al., Cocaine Comparison of virus concentration methods and RNA extraction methods for SARS-CoV-2 wastewater surveillance, Sci. Total Environ., 2022, 824, 153687 CrossRef CAS PubMed
.
- K. M. Babler, A. Amirali, M. E. Sharkey, S. L. Williams, M. M. Boone and G. A. Cosculluela,
et al., Comparison of Electronegative Filtration to Magnetic Bead-Based Concentration and V2G-qPCR to RT-qPCR for Quantifying Viral SARS-CoV-2 RNA from Wastewater, ACS ES&T Water, 2022, 2(11), 2004–2013 Search PubMed
.
- M. Hasing, J. Yu, Y. Qiu, R. Maal-Bared, S. Bhavanam and B. Lee,
et al., Comparison of Detecting and Quantitating SARS-CoV-2 in Wastewater Using Moderate-Speed Centrifuged Solids versus an Ultrafiltration Method, Water, 2021, 13(16), 2166 CrossRef CAS
.
- D. Kaya, D. Niemeier, W. Ahmed and B. V. Kjellerup, Evaluation of multiple analytical methods for SARS-CoV-2 surveillance in wastewater
samples, Sci. Total Environ., 2022, 808, 152033 CrossRef CAS PubMed
.
- J. L. Kevill, C. Pellett, K. Farkas, M. R. Brown, I. Bassano and H. Denise,
et al., A comparison of precipitation and filtration-based SARS-CoV-2 recovery methods and the influence of temperature, turbidity, and surfactant load in urban wastewater, Sci. Total Environ., 2022, 808, 151916 CrossRef CAS PubMed
.
- D. North and K. Bibby, Comparison of viral concentration techniques for native fecal indicators and pathogens from wastewater, Sci. Total Environ., 2023, 905, 167190 CrossRef CAS PubMed
.
- B. M. Pecson, E. Darby, C. N. Haas, Y. M. Amha, M. Bartolo and R. Danielson,
et al., Reproducibility and sensitivity of 36 methods to quantify the SARS-CoV-2 genetic signal in raw wastewater: findings from an interlaboratory methods evaluation in the U.S, Environ. Sci.: Water Res. Technol., 2021, 7(3), 504–520 RSC
.
- B. Peinado, L. Martínez-García, F. Martínez, L. Nozal and M. B. Sánchez, Improved methods for the detection and quantification of SARS-CoV-2 RNA in wastewater, Sci. Rep., 2022, 12(1), 7201 CrossRef CAS PubMed
.
- A. Pérez-Cataluña, E. Cuevas-Ferrando, W. Randazzo, I. Falcó, A. Allende and G. Sánchez, Comparing analytical methods to detect SARS-CoV-2 in wastewater, Sci. Total Environ., 2021, 758, 143870 CrossRef PubMed
.
- W. Ahmed, W. J. M. Smith, S. Metcalfe, G. Jackson, P. M. Choi and M. Morrison,
et al., Comparison of RT-qPCR and RT-dPCR Platforms for the Trace Detection of SARS-CoV-2 RNA in Wastewater, ACS ES&T Water, 2022, 2(11), 1871–1880 Search PubMed
.
- M. Ciesielski, D. Blackwood, T. Clerkin, R. Gonzalez, H. Thompson and A. Larson,
et al., Assessing sensitivity and reproducibility of RT-ddPCR and RT-qPCR for the quantification of SARS-CoV-2 in wastewater, J. Virol. Methods, 2021, 297, 114230 CrossRef CAS PubMed
.
- A. Keshaviah, I. Huff, X. C. Hu, V. Guidry, A. Christensen and S. Berkowitz, Separating Signal from Noise in Wastewater Data: An Algorithm to Identify Community-Level COVID-19 Surges. Public and Global Health, Proc. Natl. Acad. Sci. U. S. A., 2023, 120, e2216021120 CrossRef CAS PubMed
.
-
Association of Public Health Laboratories (APHL), SARS-CoV-2 Wastewater Surveillance Testing Guide for Public Health Laboratories, March 2022, https://www.aphl.org/aboutAPHL/publications/Documents/EH-2022-SARSCoV2-Wastewater-Surveillance-Testing-Guide.pdf Search PubMed.
-
L. Hopkins, K. Ensor and L. B. Stadler, City of Houston, Wastewater Epidemiology for SARS-CoV-2, Houston Wastewater Epidemiology (HWE) Best Practices, Houston Health Department, Houston Public Works and Rice University, 2022, https://www.hou-wastewater-epi.org/sites/g/files/bxs4786/files/2022-06/COH-Wastewater-Epi-Best-Practices.pdf Search PubMed.
- N. Decaro, G. Elia, M. Campolo, C. Desario, V. Mari and A. Radogna,
et al., Detection of bovine coronavirus using a TaqMan-based real-time RT-PCR assay, J. Virol. Methods, 2008, 151(2), 167–171 CrossRef CAS PubMed
.
- E. Haramoto, M. Kitajima, N. Kishida, Y. Konno, H. Katayama and M. Asami,
et al., Occurrence of Pepper Mild Mottle Virus in Drinking Water Sources in Japan, Appl. Environ. Microbiol., 2013, 79(23), 7413–7418 CrossRef CAS PubMed
.
- S. A. Bustin, V. Benes, J. A. Garson, J. Hellemans, J. Huggett and M. Kubista,
et al., The MIQE Guidelines: Minimum Information for Publication of Quantitative Real-Time PCR Experiments, Clin. Chem., 2009, 55(4), 611–622 CrossRef CAS PubMed
.
- R. E. Beattie, A. D. Blackwood, T. Clerkin, C. Dinga and R. T. Nobel, Evaluating the impact of sample storage, handling and technical ability on the decay and recovery of SARS-CoV-2 in wastewater, PLoS One, 2022, 16(6), e0270659 CrossRef PubMed
.
- S. Ciannella, C. Gonzalez-Fernandez and J. Gomez-Pastora, Recent progress on wastewater-based epidemiology for COVID-10 surveillance: A systematic review of analytical procedures and epidemiological modeling, Sci. Total Environ., 2023, 878, 162953 CrossRef CAS PubMed
.
- M. T. Flood, J. Sharp, J. Bruggink, M. Cormier, B. Gomes, I. Oldani, L. Zimmy and J. B. Bose, Understanding the efficacy of wastewater surveillance for SARS-CoV-2 in two diverse communities, PLoS One, 2023, 18(8), e0289343 CrossRef CAS PubMed
.
- P. Liu, L. Guo, M. Cavallo, C. Cantrell, S. P. Hilton and A. Nguyen,
et al., Comparison of Nanotrap® Microbiome A Particles, membrane filtration, and skim milk workflows for SARS-CoV-2 concentration in wastewater, Front. Microbiol., 2023, 14, 1215311 CrossRef PubMed
.
- M. S. Angga, B. Malla, S. Raya, M. Kitajima and E. Haramoto, Optimization and performance evaluation of an automated filtration method for the recovery of SARS-CoV-2 and other viruses in wastewater, Sci. Total Environ., 2023, 882, 163487 CrossRef CAS PubMed
.
- I. Girón-Guzmán, A. Díaz-Reolid, E. Cuevas-Ferrando, I. Falcó, P. Cano-Jiménez and I. Comas,
et al., Evaluation of two different concentration methods for surveillance of human viruses in sewage and their effects on SARS-CoV-2 sequencing, Sci. Total Environ., 2023, 862, 160914 CrossRef PubMed
.
- S. Feng, A. Roguet, J. S. McClary-Gutierrez, R. J. Newton, N. Kloczko and J. G. Meiman,
et al., Evaluation of Sampling, Analysis, and Normalization Methods for SARS-CoV-2 Concentrations in Wastewater to Assess COVID-19 Burdens in Wisconsin Communities, ACS ES&T Water, 2021, 1(8), 1955–1965 Search PubMed
.
- R. Gonzalez, K. Curtis, A. Bivins, K. Bibby, M. H. Weir and K. Yetka,
et al., COVID-19 surveillance in Southeastern Virginia using wastewater-based epidemiology, Water Res., 2020, 186, 116296 CrossRef CAS PubMed
.
- D. Gerrity, K. Papp, M. Stoker, A. Sims and W. Frehner, Early-pandemic wastewater surveillance of SARS-CoV-2 in Southern Nevada: Methodology, occurrence, and incidence/prevalence considerations, Water Res.: X, 2021, 10, 100086 CAS
.
- Z. W. LaTurner, D. M. Zong, P. Kalvapalle, K. R. Gamas, A. Terwilliger and T. Crosby,
et al., Evaluating recovery, cost, and throughput of different concentration methods for SARS-CoV-2 wastewater-based epidemiology, Water Res., 2021, 197, 117043 CrossRef CAS PubMed
.
- W. Ahmed, P. M. Bertsch, A. Bivins, K. Bibby, K. Farkas and A. Gathercole,
et al., Comparison of virus concentration methods for the RT-qPCR-based recovery of murine hepatitis virus, a surrogate for SARS-CoV-2 from untreated wastewater, Sci. Total Environ., 2020, 739, 139960 CrossRef CAS PubMed
.
- M. A. I. Juel, N. Stark, B. Nicolosi, J. Lontai, K. Lambirth and J. Schlueter,
et al., Performance evaluation of virus concentration methods for implementing SARS-CoV-2 wastewater based epidemiology emphasizing quick data turnaround, Sci. Total Environ., 2021, 801, 149656 CrossRef CAS PubMed
.
- G. A. Junter and L. Lebrun, Cellulose-based virus-retentive filters: a review, Rev. Environ. Sci. Biotechnol., 2017, 16(3), 455–489 CrossRef CAS PubMed
.
- T. W. Mix, The physical chemistry of membrane-virus interactions, Dev. Ind. Microbiol., 1974, 15, 136–142 CAS
.
- M. Luisetto, G. Tarro, E. Khaled, K. Farhan Ahmad, A. Yesvi and B. Nili,
et al., Coronavirus COVID-19 surface properties: Electrical charges status, International Journal of Clinical Microbiology and Biochemical Technology, 2021, 4(1), 016–027 CrossRef
.
- J. Lukasik, T. M. Scott, D. Andryshak and S. R. Farrah, Influence of Salts on Virus Adsorption to Microporous Filters, Appl. Environ. Microbiol., 2000, 66(7), 2914–2920 CrossRef CAS PubMed
.
- L. A. Ikner, C. P. Gerba and K. R. Bright, Concentration and Recovery of Viruses from Water: A Comprehensive Review, Food Environ. Virol., 2012, 4(2), 41–67 CrossRef PubMed
.
- K. K. Vadde, H. Al-Duroobi, D. C. Phan, A. Jafarzadeh, S. V. Moghadam and A. Matta,
et al., Assessment of Concentration, Recovery, and Normalization of SARS-CoV-2 RNA from Two Wastewater Treatment Plants in Texas and Correlation with COVID-19 Cases in the Community, ACS ES&T Water, 2022, 2(11), 2060–2069 Search PubMed
.
- E. K. Hayes, C. L. Sweeney, M. Fuller, G. B. Erjavec, A. K. Stoddart and G. A. Gagnon, Operational Constraints of Detecting SARS-CoV-2 on Passive Samplers using Electronegative Filters: A Kinetic and Equilibrium Analysis, ACS ES&T Water, 2022, 2(11), 1910–1920 Search PubMed
.
- M. H. Jafferali, K. Khatami, M. Atasoy, M. Birgersson, C. Williams and Z. Cetecioglu, Benchmarking virus concentration methods for quantification of SARS-CoV-2 in raw wastewater, Sci. Total Environ., 2021, 755, 142939 CrossRef CAS PubMed
.
- S. Balboa, M. Mauricio-Iglesias, S. Rodriguez, L. Martínez-Lamas, F. J. Vasallo and B. Regueiro,
et al., The fate of SARS-COV-2 in WWTPS points out the sludge line as a suitable spot for detection of COVID-19, Sci. Total Environ., 2021, 772, 145268 CrossRef CAS PubMed
.
- K. E. Graham, S. K. Loeb, M. K. Wolfe, D. Catoe, N. Sinnott-Armstrong and S. Kim,
et al., SARS-CoV-2 RNA in Wastewater Settled Solids Is Associated with COVID-19 Cases in a Large Urban Sewershed, Environ. Sci. Technol., 2021, 55(1), 488–498 CrossRef CAS PubMed
.
- S. Kim, L. C. Kennedy, M. K. Wolfe, C. S. Criddle, D. H. Duong and A. Topol,
et al., SARS-CoV-2 RNA is enriched by orders of magnitude in primary settled solids relative to liquid wastewater at publicly owned treatment works, Environ. Sci.: Water Res. Technol., 2022, 8(4), 757–770 RSC
.
- K. Lewandowski, A. Bell, R. Miles, S. Carne, D. Wooldridge and C. Manso,
et al., The Effect of Nucleic Acid Extraction Platforms and Sample Storage on the Integrity of Viral RNA for Use in Whole Genome Sequencing, J. Mol. Diagn., 2017, 19(2), 303–312 CrossRef CAS PubMed
.
- J. Verheyen, R. Kaiser, M. Bozic, M. Timmen-Wego, B. K. Maier and H. H. Kessler, Extraction of viral nucleic acids: Comparison of five automated nucleic acid extraction platforms, J. Clin. Virol., 2012, 54(3), 255–259 CrossRef CAS PubMed
.
- G. Yang, D. E. Erdman, M. Kodani, J. Kools, M. D. Bowen and B. S. Fields, Comparison of commercial systems for extraction of nucleic acids from DNA/RNA respiratory pathogens, J. Virol. Methods, 2011, 171(1), 195–199 CrossRef CAS PubMed
.
- M. N. Emaus, M. Varona, D. R. Eitzmann, S. A. Hsieh, V. R. Zeger and J. L. Anderson, Nucleic acid extraction: Fundamentals of sample preparation methodologies, current advancements, and future endeavors, TrAC, Trends Anal. Chem., 2020, 130, 115985 CrossRef CAS
.
-
A. Hinkle, H. D. Greenwald, M. Metzger, M. Thornton, L. C. Kennedy and K. Loomis, et al., Comparison of RT-qPCR and Digital PCR Methods for Wastewater-Based Testing of SARS-CoV-2. Infectious Diseases (except HIV/AIDS), medRxiv, 2022, preprint, DOI:10.1101/2022.06.15.22276459.
- Y. Hou, H. Zhang, L. Miranda and S. Lin, PLoS One, 2010, 5, e9545 CrossRef PubMed
.
- N. Rački, T. Dreo, I. Gutierrez-Aguirre, A. Blejec and M. Ravnikar, Reverse transcriptase droplet digital PCR shows high resilience to PCR inhibitors from plant, soil and water samples, Plant Methods, 2014, 10(1), 42 CrossRef PubMed
.
- S. C. Taylor, G. Laperriere and H. Germain, Droplet Digital PCR versus qPCR for gene expression analysis with low abundant targets: from variable nonsense to publication quality data, Sci. Rep., 2017, 7(1), 2409 CrossRef PubMed
.
- P. M. D'Aoust, E. Mercier, D. Montpetit, J. J. Jia, I. Alexandrov and N. Neault,
et al., Quantitative analysis of SARS-CoV-2 RNA from wastewater solids in communities with low COVID-19 incidence and prevalence, Water Res., 2021, 188, 116560 CrossRef PubMed
.
- S. C. Taylor, K. Nadeau, M. Abbasi, C. Lachance, M. Nguyen and J. Fenrich, The Ultimate qPCR Experiment: Producing Publication Quality, Reproducible Data the First Time, Trends Biotechnol., 2019, 37(7), 761–774 CrossRef CAS PubMed
.
- M. K. Schussman, A. Roguet, A. Schmoldt, B. Dinan and S. L. McLellan, Wastewater surveillance using ddPCR accurately tracked Omicron emergence due to altered N1 probe binding efficiency, Environ. Sci.: Water Res. Technol., 2022, 8(10), 2190–2195 RSC
.
Footnotes |
† Electronic supplementary information (ESI) available. See DOI: https://doi.org/10.1039/d3ew00958k |
‡ These authors contributed equally. |
§ Present address: Minnesota Department of Health Public Health Laboratory, 601 N. Robert St. St. Paul, MN 55155 United States of America. |
|
This journal is © The Royal Society of Chemistry 2024 |
Click here to see how this site uses Cookies. View our privacy policy here.