DOI:
10.1039/D3LC00488K
(Paper)
Lab Chip, 2024,
24, 505-516
3D printed porous membrane integrated devices to study the chemoattractant induced behavioural response of aquatic organisms†
Received
6th June 2023
, Accepted 3rd December 2023
First published on 12th December 2023
Abstract
Biological models with genetic similarities to humans are used for exploratory research to develop behavioral screening tools and understand sensory–motor interactions. Their small, often mm-sized appearance raises challenges in the straightforward quantification of their subtle behavioral responses and calls for new, customisable research tools. 3D printing provides an attractive approach for the manufacture of custom designs at low cost; however, challenges remain in the integration of functional materials like porous membranes. Nanoporous membranes have been integrated with resin exchange using purpose-designed resins by digital light projection 3D printing to yield functionally integrated devices using a simple, economical and semi-automated process. Here, the impact of the layer thickness and layer number on the porous properties – parameters unique for 3D printing – are investigated, showing decreases in mean pore diameter and porosity with increasing layer height and layer number. From the same resin formulation, materials with average pore size between 200 and 600 nm and porosity between 45% and 61% were printed. Membrane-integrated devices were used to study the chemoattractant induced behavioural response of zebrafish embryos and planarians, both demonstrating a predominant behavioral response towards the chemoattractant, spending >85% of experiment time in the attractant side of the observation chamber. The presented 3D printing method can be used for printing custom designed membrane-integrated devices using affordable 3D printers and enable fine-tuning of porous properties through adjustment of layer height and number. This accessible approach is expected to be adopted for applications including behavioural studies, early-stage pre-clinical drug discovery and (environmental) toxicology.
Introduction
Sensory–motor integration in neuroscience involves the study of sensory input–motor output and associated complex neuronal mechanisms.1 Sensory and motor interaction provides the foundation for growth, development and learning, and defects cause sensory processing disorders.2,3 Organisms with genetic, anatomical, and physiological/biological process similarities with humans are used for conducting exploratory research in this field.4 Zebrafish (Danio rerio) are a prominent model for genetic disorders and enable studying sensory input–motor output from the genetic to the behavioural level.5 The small size, optical transparency, and rapid development of neuron circuitries make zebrafish embryos/larvae attractive for biological and toxicological studies.6–9 Likewise, planarians are popular for chemo-behavioural phenotyping to study the impact of chemicals on central and peripheral nervous systems.10–12
Behavioural studies rely on the precise control of the delivery of chemosensory, visual, acoustic/photo and/or thermal stimuli13 and unobstructed observation, preferably using a microscope. Traditional setups involve freely moving animals in an open chamber,14,15 Petri dish,16 or multi-well plate,17 with the manually controlled application of stimuli18,19 not well suited for the observation of subtle behaviour responses of mm-sized animals. Microfluidics provides an alternative that is well suited to deliver the stimuli with accuracy and control, and by using optically transparent materials, subtle responses can be readily observed and quantified.20 For example, polydimethylsiloxane (PDMS) devices have been used to partially restrain zebrafish embryos in agar gel and study the behaviour movements of the animals in response to chemical stimuli.21 Partial immobilisation and constraint of the freedom of zebrafish was also realised using a 200 μm thick PDMS membrane to study shape tail movements.20 PDMS devices are typically produced using soft lithography,22 a multistep process that relies on casting from a high resolution template. Functional integration is typically achieved by combining multiple layers that are sandwiched together through clamping, plasma bonding or gluing following alignment.20,23,24 This process is time-consuming, and the manual handling involved in assembly requires skill, reduces yield, and compromises repeatability.
3D printing is an alternative manufacturing approach that involves the transformation of computer-aided design (CAD) models into physical objects by layering structured materials in a systematic process.25 Its advantages including automation, limited capital investment, and opportunities for material integration resulted in fast adoption for the production of reaction-ware26 and related devices for chemical sciences,27 tissue engineering28–30 and microfluidics.31,32 In comparison, the uptake of 3D printing in aquatic animal behaviour studies, however, is still in its infancy.
Porous materials have many uses, including the facilitation of size-selective transport. Directly printing with nanoporous features as desirable for selective transport remains a challenge as desktop 3D printers can produce features down to only 100 μm (ref. 33) and more advanced photopolymerization-based 3D printing technologies can achieve submicron (1–100 μm) resolution.34 The printing of nanoporous materials has been used as an alternative,32 for example by the removal of inert components during post-processing to reveal a porous skeleton.27,35–37 Materials with more controlled porous properties can be obtained by polymerisation induced phase separation (PIPS).38,39 Both the exposure dose and the resin composition can be used to influence the material properties. Porous properties have been tailored using the photoinitiator concentration and porogen content to yield mean pore sizes from 20 nm to 800 nm.40 The role of the exposure dose has also been investigated, and nanoporous materials were successfully printed from PIPS resins by two-photon polymerization (TPP), decreasing the porosity from 43% to 22% by increasing the exposure power.41 Our team recently presented the printing of functionally graded porous materials by digital light projection (DLP) 3D printing using a greyscale mask, yielding materials ranging from effectively dense to materials with a porosity of 23%.42 Unfortunately, greyscale masks are accessible in only a few printers, limiting the widespread uptake of the approach.
In the current work, the influence of the repeat exposures typical for the layer-by-layer manufacturing approach as well as that of the layer height on the porous properties of materials printed from PIPS resins is presented. The formed porous membranes were integrated in devices designed to study the chemoattractant-induced behavioral response of aquatic organisms. The use of an affordable printer (<$500) with an easy-to-use interface is anticipated to lower the barrier for uptake of the new technology, and is anticipated to open opportunities for tailored designs and controlled chemical environments to study ecological responses in response to toxins and animal behavior to inform early-stage pre-clinical drug discovery.
Experimental
Materials
Commercial DLP printing resin DruckWege Clear and Black Standard-D (FEP Shop, the Netherlands) was used to print the device body, employing resin exchange with the custom PIPS resin to print the membranes separating the chambers. The PIPS resin formulation contained poly(ethylene glycol diacrylate) (PEGDA-250) (Sigma-Aldrich, NSW, Australia) as the monomer, Irgacure 819 (also known as phenylbis(2,4,6 trimethyl benzoyl) phosphine oxide (BAPO)) as the photoinitiator and methanol (Sigma-Aldrich, NSW, Australia) as the porogen. The resin formulation was prepared by combining PEGDA-250 and methanol in a ratio of 30% and 70% (v/v), before adding 1% (w/w) BAPO (0.72 mg mL−1). A 0.1 M solution of sodium fluorescein (Sigma-Aldrich, NSW, Australia) in deionised water was used to visualise small molecule transport.
Based on published literature,16,43 an amino acid mixture was selected as the chemoattractant for zebrafish embryos (MEM non-essential amino acid solution, Thermo Fisher Scientific, Australia) at a concentration of 100 μM. For the planaria, a beef liver homogenate was used, dissociated in spring water using a pipette following literature precedence.19 Spring water was obtained from a supermarket (Coles brand, Coles, Australia).
3D printing and device manufacture
A Phrozen Shuffle 2018 DLP 3D printer (Phrozen Tech, Co, Ltd, Taiwan) was used with a screen resolution of 47 μm × 47 μm and build plate dimensions of 120 mm × 70 mm. The devices were 3D printed using resin exchange to change from printing in the device body in standard-D and the porous membranes in the custom PIPS resin with an optimized exposure time of 30 s per layer (optimized using a procedure by Gong et al.44). The optimised layer height was 100 μm. After printing, the device was sonicated in isopropanol for 3 h to ensure complete removal of any unpolymerized resin. Each device was mounted on a glass slide using PCR grade highly adhesive double-sided tape (ARseal 90880, Adhesives Research, Singapore) on the edges of the device, which provided a leak-tight seal. To prevent any movement of the device on the tape during handling, an epoxy adhesive (Araldite, Selleys, New Zealand) was applied around the device.
Membrane characterization
Cross sections of the membrane were imaged in a scanning electron microscope (SEM) at 4 kV using the in-lens for detecting backscattered electrons (Supra 55VP, Zeiss). Before imaging, samples were cross-sectioned in liquid nitrogen and coated with gold nanoparticles (thickness 5 nm) using a Leica ACE600 sputtering system.
Pore size distribution and average pore size were measured using a capillary flow porometer (Porometer 3GHz, Anton Paar, Australia) for quantitative measurement of pore size (average size and distribution) using both liquid and gas flow. Geometrical density was calculated from mass and volume, measured from 3D printed samples with 5 mm diameter; the thickness was measured using a digital calliper. The geometrical volume of the sample combines the volume of the skeletal structure and the air filling the pores. An ultrapycnometer (Ultrapyc 1200e, Quantachrome Instruments, Australia) was used to measure the sample's skeletal volume/true volume in the microcell at ambient temperature (21 °C) using helium as the operating gas at 19 psi. The percentage porosity (ε) was calculated from the geometrical and true volumes.
Zebrafish husbandry and embryo collection
Zebrafish husbandry, breeding and embryo collection was carried out using previously published methods.9,45 In brief, a Tecniplast zebrafish recirculating aquaculture system with a custom life support system (Tecniplast, Italy) was used to maintain Deakin wild-type zebrafish at the Deakin University Aquatics Facility, Waurn Ponds, Australia. Adult fish were maintained in 3 L tanks (30 fish per tank) with a continuous flow of aerated water (28 °C; pH 7 ± 0.5; electric conductivity: 470–530 μs m−1), with water quality parameters maintained and measured regularly (nitrates: <0.009 g L−1; nitrites: 8–12 mg L−1; and ammonia levels: <0.05 mg L−1). Breeding was used to obtain zebrafish embryos for experiments with two females and one male adult zebrafish transferred to 1 L breeding tanks with a 2 mm transparent mesh insert and the sexes were kept separate using a transparent barrier. On the next day and after the lights were turned on in the facility, the transparent barrier in the breeding tank was removed and the zebrafish were allowed one hour for mating, spawning, and fertilizing eggs. A sieve was used to collect the fertilized eggs and they were transferred into 90 mm Petri dishes filled with zebrafish aquatic water. The aquatic water in the Petri dishes was changed regularly and unfertilized eggs were removed. The embryos were incubated in the Petri dishes (80–90 embryos per Petri dish) at 28 °C and 80% relative humidity in an incubator with a photoperiod of 12 L
:
12 D (temperature cycling chamber, Labec, Australia). Unfertilized eggs and dead embryos were removed every 8 h with a water change and embryos were cultured to 96 hpf age for chemotaxis experiments. On the day of the experiments, zebrafish embryos (96 hpf) were randomly collected from the Petri dishes and transferred into the 3D printed devices for the chemotaxis experiment (6 embryos per device).
All animals used in this study were approved for use by the Deakin University Animal Ethics Committee (AEC number G11-2019) following the Australian code for the care and use of animals for scientific purposes (8th edition, National Health and Medical Research Council).
Planaria collection and husbandry
Freshwater planaria (Girardia tigrina) were collected from St Augustine's Lagoon (GPS location −38.191181, 144.313191), Waurn Ponds, Australia, and were transported to the lab for culturing. The planaria were cultured in 1 L plastic containers containing 500 mL of spring water (Coles, Australia) in the dark. Every three days, 1–2 pieces of beef liver were placed in each culture container containing the colony of planaria and after 2 hours of feeding, the liver was removed from the colonies and the water was drained. The containers were cleaned and were replenished with 500 mL spring water. Cleaning of the culture containers was repeated the day after each feeding. The planaria were kept and cultured for at least one week prior to use in the experiments and individuals that were randomly selected for chemotaxis experiments were starved for 3 days prior to being used in experiments.
Chemotaxis set up and analysis
Three devices were set up simultaneously to observe the animal's behaviour under identical conditions; devices were placed in a glass Petri dish on graph paper to define the grid reference for the observation chamber. For each device, the test animals (6 zebrafish embryos or 3 planaria) were temporarily confined to a small area in the middle of the chamber filled with spring water using a ring, with the chemoattractant dispensed in the top chamber (adjacent to row A) and spring water in the bottom chamber (adjacent to row H). The devices were placed inside a black chamber to limit the light and other external interferences. Movement of the test animals in the observation chamber was recorded using a 10 MP HD USB camera connected to a laptop for 1 h. The videos were analysed using Adobe Premiere Pro 2022 (Adobe, USA). The position frequency data were obtained manually, recording the position of each organism at 60 s intervals using the grid reference. The cumulative time intervals were calculated for each organism to determine the time spent in the top (rows A–D) and bottom half (rows E–H) of the observation chamber. The data were processed using Excel 365 (Microsoft, USA) and Origin 2020 (Origin Lab, USA) following data analysis methodologies detailed in previous reports.18,19
The chemotaxis results were statistically analysed using GraphPad Prism (v9.5). A two-way ANOVA and Tukey's multiple comparison test were used to determine if there were differences between devices (D1–D6) in the planaria and zebrafish embryo experiments. A two-way ANOVA and Sidak's multiple comparison test were used to determine if there were differences in the time spent by the planaria and zebrafish embryos in the chemoattractant side of the devices compared to the blank side. An unpaired two-tailed t-test was used to compare the cumulative chemotaxis data for planaria and zebrafish embryos. Data are presented as mean ± standard deviation and p values ≤0.05 were considered significant.
Results and discussion
Device and study design
A schematic representation of the 3D-printed device is shown in Fig. 1a. The device consisted of two side chambers (600 μL) separated from a central observation chamber (6000 μL) by porous membranes to facilitate the transport to initiate a chemical gradient (Fig. 1b). The chambers were filled with 600 μL and 6000 μL, respectively, using an autopipette, with the volumes calculated from the CAD design using SolidWorks. To prevent siphoning between chambers, the device was placed on a level surface.
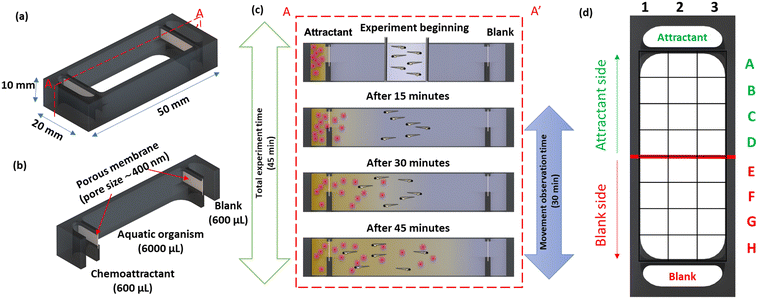 |
| Fig. 1 (a) A schematic representation of the 3D printed membrane integrated device and (b) device parameters. (c) Schematic of the process of chemotaxis response study including at experimental beginning point and after 15 minutes, 30 minutes, and 45 minutes. (d) Device segmentation using a graph paper for assessment of the organism's cumulative time and position indicated by the grid reference 1–3 (horizontal) and A–H (vertical). | |
For the study with the planarians, the membranes also served as a physical barrier to prevent cells and debris in the liver homogenate from entering the observation chamber and obscuring the view. A graphical representation of the chemoattractant induced behavioural response study is shown in Fig. 1c.
3D printing porous membrane integrated device
The printer used in this work used an LCD screen to selectively block UV light from the light source to prevent local irradiation of the photoresin. LCD based 3D printers provide a budget alternative to digital micromirror array printers and typically provide a lower resolution of ∼50 μm × 50 μm pixels, in comparison with the 30 μm × 30 μm typical for micromirror array systems. The light intensity is also about an order of magnitude lower, leading to typical exposure time of tens of seconds rather than seconds. The Phrozen Shuffle used in this work had a purchase price of US$750 in 2019, but as production stopped following the release of a new model it currently retails for significantly less.
During printing, the UV light from the source irradiates the resin in the resin tank through the transparent areas of the LCD screen, initiating free radical polymerization (Fig. 2a) and attachment of the print to the pick. The resin includes a monomer and a photoinitiator, often complemented by a photoabsorber to enhance resolution, particularly important for printing voids.44 Here, a print-pause-print (PPP) method was combined with resin exchange to integrate the porous membranes, as schematically represented in Fig. 2b. The device body was printed in a commercially sourced resin, with the two membranes formed from a purpose-developed formulation. All layers were printed at a layer exposure time of 30 s, defined by the optimised exposure time of commercial resin as layer exposure times cannot be changed during the print process. The photoinitiator concentration in the custom formulation was chosen to match the exposure time of the commercially sourced resin. Four resin exchanges were required to integrate the two membranes. By leaving the devices on the pick during resin exchange, alignment of the membrane and device components is ensured; during the manual exchange of the resin bath, the relative orientation of the pick and LCD screen remains untouched. Under optimized conditions, 12 devices could be printed in the build space in 45 min – excluding post processing – for a material cost of ∼US$1 each.
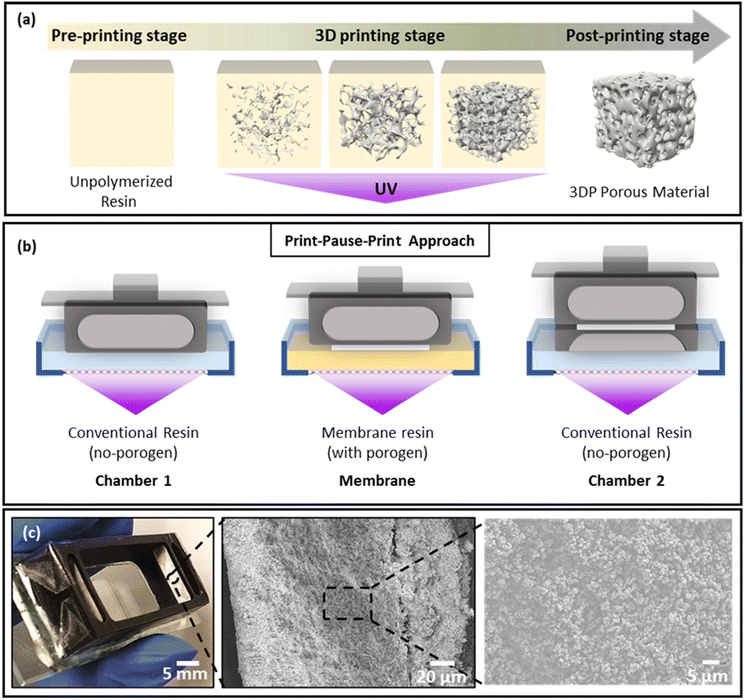 |
| Fig. 2 (a) Schematic of the formation of the 3D printed porous membrane from the PIPS resin (b) print-pause-print fabrication process for the membrane integrated device showing the lowering of the pick into the resin tank. During the sequential resin exchange process the resin tank was replaced after printing chamber 1 from the commercially sourced standard D resin with a tank containing the PIPS resin to print the membrane. After swapping back to the standard D resin tank, the study chamber was printed, and the resin exchange process was repeated for printing the second membrane to complete the process printing the third chamber in standard D resin. Prints were rinsed using isopropanol and dried between exchanges while remaining attached to the build plate. (c) 3D printed device and SEM images of the membrane. | |
Biocompatibility has been a concern, particularly in DLP 3D printing, as most commonly used resin ingredients display significant toxicity.46 Incomplete polymerization can lead to these compounds leaching out of the device, negatively impacting on bioassays. Despite a significant body of literature, biocompatibility and toxicity can be a contested issue.47 Here, the viability of the zebrafish embryos and planarians was confirmed using the DruckWege Clear and Black Standard-D empirically. Zebrafish embryos and planarians were left in a device for 5 h (5 times the duration of the experiment), after which no behavioural change or death was observed. While the standard D resin is not marketed as biocompatible, it contains cyanoacrylate monomers/oligomers that are also used as a bioadhesive48 and BAPO as a photoinitiator which has reasonable biocompatibility.47,49 Following literature recommendations for leaching out unpolymerized resin, devices were postprocessed by sonication in isopropanol for 3 h.
For printing the porous membranes separating the chambers, PEGDA250 was selected as the monomer owing to the reported biocompatibility of its DLP printed polymers49 and suitability for the formation of porous structures by PIPS.50 Methanol was selected as the porogen and BAPO as the photoinitiator, matching the photoinitiator used in the commercial resin. The porous material is formed in a process where oligomers eventually become immiscible in the resin and undergo phase separation, precipitating as nuclei for further growth, as shown in Fig. 2a. The formed globules self-assemble to form a continuous structure, leaving a porous network after removal of the porogen (Fig. 2a). The porous properties of PIPS materials depend on the resin formulation and polymerization conditions and we demonstrated tailoring porosity using greyscale masks42 and resin composition,42 focusing in this work on the role of layer height and layer number.
The printed device was mounted on a clean glass microscope slide using double-sided tape. Mounting the device on the glass slide using resin was also evaluated but incomplete removal of resin components during the wash negatively impacted on the viability of the test animals. To firmly mount the device in place, epoxy glue was used; the leak-tight seal between the print and the tape prevented direct contact between the glue and the observation chamber, as confirmed by viability testing. A photograph of the membrane-integrated device for chemoattractant induced behavioural response study is shown in Fig. 2c, and SEM images of the membrane revealing the porous network of globular structures are shown in Fig. 2c (center and right).
Membrane optimization
Based on the demonstrated impact of changes in light intensity on the porous properties,42 the effect of layer by layer manufacturing by photopolymerization was studied, investigating the effect of the layer thickness and layer number on the pore size, porosity and density of printed membranes.
The effect of layer thickness and number on the material properties was investigated because owing to the light absorption by the resin components, the light dose drops across a layer in a manner where previously printed layers will receive a subsequent dose during exposure of another layer, and for printing of voids and microchannels, the light dose needs to be carefully managed to prevent the void being filled.51
Layer thickness
The layer thickness can be set in the 3D printing software and controls the distance the stage is lifted in the Z-direction between print layers. To investigate the effect of layer thickness on the average pore size, the pore size distribution and porosity and density of membranes consisting of 2 print layers were printed with layer heights of 100, 150, and 200 μm, using optimized exposure times of 30, 47 and 65 s, respectively. The Phrozen Shuffle has a minimum layer height of 10 μm, but we experimentally determined that repeatability was poor for layer heights <50 μm and membranes printed with a thickness of <100 μm were brittle and difficult to remove from the build plate. Two print layers were required to repeatably yield complete structures strong enough for characterisation. Because even under optimized conditions layers with height >200 μm suffered from delamination when multiple layers were printed, this was taken as the maximum layer thickness.
As shown in Fig. 3a, as the layer thickness increased, the average pore size decreased from ∼450 nm to ∼290 nm and ∼210 nm for layer heights of 100, 150 and 200 μm, respectively, accompanied by a narrowing in pore size distribution from 600–400 nm (100 μm) to 400–250 nm (150 μm) and 250–200 nm (200 μm). Similarly, the porosity of the membranes reduced from 61% to 50% and 45% for 100, 150, and 200 μm layer thickness, while the density increased to 0.50, 0.63 and 0.70 g cm−3, respectively (Fig. 3b). As the layer thickness increases, the optimised exposure time increases, and with that the irradiation dose. In resins without porogen, thicker layers have been correlated to increased degree of conversion and reduced stiffness.52,53 When printing with a PIPS resin, the more extensive crosslinking led to a denser network with fewer and narrower pores, as evidenced by the pore size measurement. For the behavioural studies, the membranes serve to introduce the chemoattractant in a controlled manner, without size-selective exclusion of proteins and other macromolecules from the liver extract; the membrane of 100 μm was selected because of its high porosity, good repeatability and symmetrical pore size distribution.
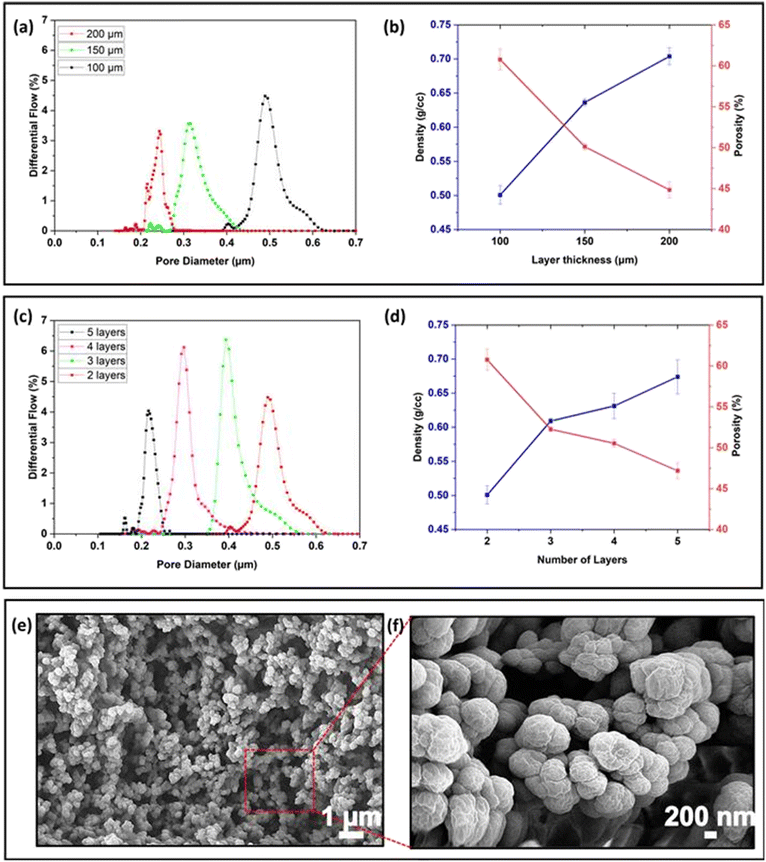 |
| Fig. 3 (a) Average pore size/distribution and (b) porosity/density under the different layer thicknesses. (c) Average pore size/distribution and (d) porosity/density under the different layer numbers. (e and f) SEM images of the porous structure of the membrane under the optimal printing conditions. | |
Layer number
When printing voids, resin removal is complicated by oligomers formed by unwanted exposure doses during the printing of subsequent layers. To investigate the impact of additional exposure doses from printing subsequent layers on the porous properties, membranes were printed with an individual layer height of 100 μm from 2 layers (the minimum number required for a stable structure) to 5 layers (5 × 100 μm).
As shown in Fig. 3c, as the layer number increased from 2 to 5 layers, the average pore size reduced from ∼490 nm (2 layers) to ∼400 nm (3 layers), ∼295 nm (4 layers), and ∼210 nm (5 layers). The pore size distribution gradually reduced from 620 to 420 nm for 2 layers, 560 to 330 for 3 layers, 410 to 290 nm for 4 layers and 250 to 190 nm for 5 layers. Similarly, porosity decreased from 61% (2 layers) and 52% (3 layers) to 51% (4 layers) and 47% (5 layers), while density increased from 0.50 (2 layers), 0.60 (3 layers), 0.63 (4 layers), and 0.68 g cm−3 (5 layers) accordingly (Fig. 3b). These data suggest that an additional light dose is received by a printed layer during the printing of subsequent layers, leading to more extensive crosslinking. Characterising this effect by determining the pore distribution along the z-axis of the prints is challenging, as characterisation methods typically provide the smallest point (porometry) or average of a ground sample (porosimetry) pore size in the sample. No inhomogeneities within layers or between layers were observed by scanning electron microscopy of prints in PIPS resins.40
Based on the practical difficulty of handling the device containing the fragile 200 μm membrane, a 300 μm thick membrane formed from 3 print layers was selected for embedding in the chemoattractant induced behavioural response study device. These membranes with 52% porosity, average pore size of 400 nm (ranging from 330 to 560 nm) and a density of 0.60 g cm−3 were robust enough to allow for handling of the chemoattractant induced behavioural response device. The pores were sufficiently large to allow for the diffusion of proteins and macromolecules from the liver homogenate used in the planarian study while obstructing the transport of cells and debris from the chemoattractant into the observation chamber. If smaller pores would be desirable, for example to have a molecular weight cut-off, the resin formulation can be changed, for example by decreasing the porogen content.42 While increasing the layer number provides a simple means to decrease the pore size, it also suggests variations in porous properties along the z-axis for complex structures. Based on the successful use of photoabsorbers44 and optical filters54 to minimise oligomer formation in voids, these approaches may also be effective ways to minimise the decrease in mean pore size when printing multi-layer structures.
Chemoattractant induced behavioural response study
The experimental station for the chemoattractant induced behavioural response study using zebrafish embryos and planarians is shown in Fig. 4a; the devices were positioned on graph paper to provide a grid reference as detailed in Fig. 4b. The device was designed to prioritise a large (6 mL) observation chamber with relatively small (600 μL) reservoirs; depletion of the source reservoir was not a concern as the response study does not require an extended time frame. The experimental period is defined by the interval the chemical gradient is present in the observation chamber. The liquid levels in all chambers were matched to prevent hydrodynamic flow and the device was placed on a level surface, making the transport of the fluorescein through the small pores a diffusion-based process; based on a small molecule diffusion constant of 4.10−6 cm2 s−1, fluorescein will cross the 300 μm thick membrane in 2 min. In the open observation chamber its distribution in the chamber is expected be the result of diffusion and convection. Using fluorescein (100 mM) as a visual marker for small molecule transport, the time-lapse images presented in Fig. 4c confirm that after 15 min, the dye can be observed at the top of the observation chamber, making this a good starting point for release of the organisms. After 45 min, the fluorescein reached the middle of the observation chamber, and after 1 h, the fluorescein was distributed across the chamber. Both the fast progression of fluorescein into the chamber (small molecule diffusion in 45 min is ∼1.5 mm) and the uneven appearance of the fluorescein front confirm that its transport in the observation chamber is a combination of diffusion and convection. More information including the diffusion distance over time and a colour contour plot of the observed spread of fluorescein are provided in the ESI,† Fig. S1. The interval between 15 and 45 min after introducing the chemoattractant was selected as an experimental window for studying the chemotaxis-induced response from the centre of the observation chamber.
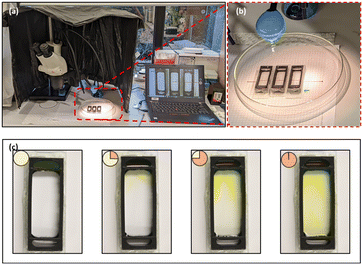 |
| Fig. 4 (a) The experimental station for the chemotaxis response study using zebrafish embryos and planaria in the 3D printed device. (b) Amplified image for the device arrangement on the graph paper for the quantificational analysis. (c) Fluorescein transport study to determine the effective chemotaxis-induced response period. | |
Zebrafish embryos
Six zebrafish embryos were placed in the movement-restricting ring positioned in the centre of the observation chamber of each device (Fig. 5a), and the amino acids and spring water were loaded in the chemoattractant and blank reservoir, respectively. After de-stressing for 15 min in the dark, the restricting ring was slowly removed using needle nose forceps, taking care to minimise fluidic disturbances in the study chamber. Immediately, migration of the embryos towards the attractant was observed. The average time spent in the chemoattractant side of the chamber was significantly higher compared to that in the blank side of the chamber (non-chemoattractant side), with 25.63 ± 3.64, 21.77 ± 3.34, 24.83 ± 4.00, 25.93 ± 4.06, 28.13 ± 3.44, and 27.09 ± 2.12 min for devices D1 to D6, respectively (Fig. 5b). Control experiments confirmed no directed migration in the absence of the chemoattractant. With a coefficient of variation of 10%, device to device repeatability was good. Combining the results from each device also demonstrated that zebrafish embryos spent a significantly greater amount of time in the chemoattractant side of the devices (25.53 ± 3.58 min) compared to that in the blank side (4.47 ± 3.58 min; Fig. S1a, ESI†). The significantly greater presence in the attractant side of the chamber confirmed that the chemical gradient was generated through the membrane in the device and the zebrafish embryos recognized the concentration gradient of chemicals, moving to a higher concentration of the attractant. This result correlates well with previously published work.43
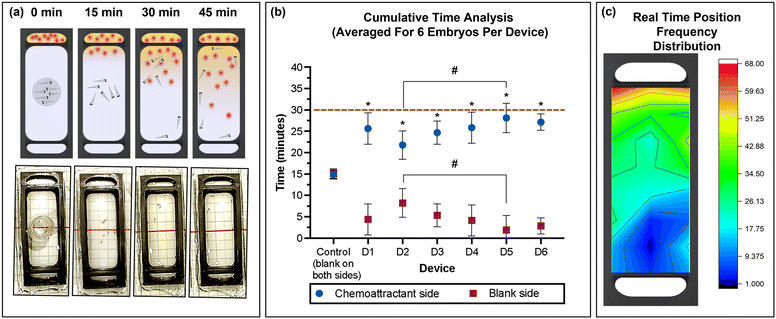 |
| Fig. 5 (a) Schematic representation and photographic images of the 3D printed device used for the chemotaxis response study using zebrafish embryos. (b) Average (mean ± standard deviation) cumulative time spent in the chemoattractant side in rows A–D (blue) or at the blank side in rows E–H (red) for the six embryos per device for six devices (D1–D6) and control (position of rows A–G indicated in the grid reference in Fig. 1d). Dashed line represents total experimental time. Asterisks (*) denote significant differences between time spent in the chemoattractant side vs. the blank side (two-way ANOVA, Tukey's multiple comparisons test) in each device and the hash signs (#) denote significant differences between devices (two-way ANOVA, Sidak's multiple comparisons test). Values are mean ± standard deviation and each experiment was repeated 6 times (n = 6 for each data point). (c) Heat map of the position frequency distribution for six embryos during the 30 min chemotaxis observational study. | |
The real-time position of the embryos was recorded in one-minute intervals, and the frequency at each position was visualized as a heat map (Fig. 5c). The red/yellow colour near the chemoattractant reservoir indicates that the embryos spend more time in the region with higher amino acid concentration than in the region with low or no amino acids, with a strong preference of residing close to the source near the membrane. After 30 min, the migration returned to a random pattern also observed during control experiments with only spring water (no chemoattractant). This agrees with the increasing homogeneity in the yellow colour observed using fluorescein to visualize small molecule transport (Fig. 4c), anticipating that convective mixing in the observation chamber may be enhanced by the swimming of the zebrafish embryos. During the control experiment spring water was dispensed in both side chambers, demonstrating non-directional and random movement of the embryos from t = 15 min (results not shown). The difference in response in the presence and absence of the chemical stimulus demonstrates that zebrafish embryos as young as four days post fertilization (dpf) have developed chemosensory response elements, which is consistent with other previous studies.16
Planarians
Planarians are known to respond to chemical stimuli even when combined with other stimuli such as light and temperature.19 In this study, a beef liver homogenate was used to study response of the planaria. Akin to the zebrafish embryos, the planarians were introduced in a movement restricting ring in the centre of the observation chamber, and the homogenate was introduced into the attractant chamber with spring water dispensed into the bottom chamber as control. After 15 min of de-stressing, the restricting ring was slowly removed using needle nose forceps, taking care to minimise fluidic disturbances in the study chamber. The migration of the released planarians was recorded for the following 30 min. The average time spent in the chemoattractant side of the chamber by planaria was significantly higher compared to that in the blank side of the chamber (spring water), with 24.45 ± 4.83, 25.89 ± 4.94, 25.20 ± 3.78, 22.59 ± 3.91, 28.56 ± 1.8 and 27.29 ± 1.2 min for devices D1 to D6, respectively (Fig. 6b). Combining the results from each device also demonstrated that planaria spent a significantly greater amount of time in the chemoattractant side of the devices (26.01 ± 3.31 min) compared to that in the blank side (3.99 ± 3.31 min; Fig. S1b, ESI†). Control experiments confirmed no directed migration in the absence of the chemoattractant.
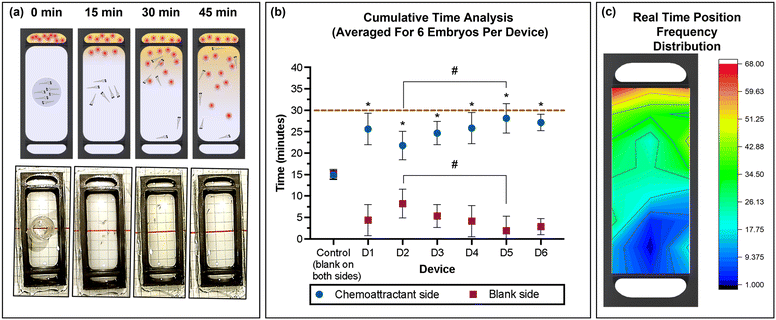 |
| Fig. 6 (a) Schematic representation and photographic images of the 3D printed device used for the chemoattractant response study using planarians. (b) Average (mean ± standard deviation) cumulative time spent in the chemoattractant side in rows A–D (blue) or at the blank side in rows E–H (red) for the three planarians per device for six devices (D1–D6) and control (position of rows indicated in the grid reference in Fig. 1d). Dashed line represents total experimental time. Asterisks (*) denote significant differences between time spent in the chemoattractant side vs. the blank side (two-way ANOVA, Tukey's multiple comparisons test) in each device, and hash signs (#) denote significant differences between devices (two-way ANOVA, Sidak's multiple comparisons test). Values are mean ± standard deviation and each experiment was repeated six times (n = 3 for each data point). (c) Heat map of the position frequency distribution for three planarians during the 30 min chemoattractant response study. | |
The real-time position frequency demonstrated that the planaria had a greater tendency to move towards the region with the highest chemoattractant concentration as shown by the heat map in Fig. 6c. In Fig. 6c, the red and yellow colour near the chemoattractant chamber visualize this higher frequency of the planarians present in that area, suggesting that the planarians detect the concentration gradient of compounds present in the homogenized liver and move towards the higher concentration. The control experiment conducted with water in both chambers showed random movement within the observation chamber, confirming that the behavioural difference was caused by the chemical stimulus.
The proposed method is cost-effective and provides flexibility in design by employing a print-pause-print approach using a PIPS resin to print nanoporous membranes directly into the device. This eliminates the need for manual alignment and additional processing for sealing/bonding typical for the incorporation of commercially sourced membranes into a fluidic device.32 The porous properties of the membrane tailor its transport behaviour, and here the insight is provided that the mean pore size and porosity decrease with increasing layer height and layer number, allowing for adjustment of porous properties using the printer settings. The membrane-integrated devices were used to generate a repeatable chemical gradient to study the chemoattractant induced response of free-swimming animals such as zebrafish embryos and planaria. The chemoattractant enters the study chamber from the source chamber and is distributed through the chamber by diffusion and convection. The membrane-integrated device provides a significant experimental advance in comparison with the manual transfer of chemoattractant-infused agar into the study chamber containing the planarian19 or zebrafish. It should be noted that the current system has a limited experimental window of 30 min before the gradient is dissipated, which is shorter than the time frames used to study behaviour in static gradients.55 However, static systems require continuous replenishment of source and sink, which adds complexity to the experimental setup.
Conclusions and future outlook
The printing of 3D objects in a layer-by-layer manner is fundamental for 3D printing. The porous properties of materials printed from porogen-containing resins depend on the exposure dose. The height of each printed layer as well as the printing of subsequent layers impact on the exposure dose. Characterisation of nanoporous membranes printed from PIPS resins with increasing layer height and layer number showed a significant decrease in mean pore size, porosity and density with increasing layer thickness and number of layers. The average pore size decreased from ∼450 nm to ∼290 nm and ∼210 nm for layer heights of 100, 150 and 200 μm, respectively, with corresponding decreases in porosity of 61% to 50% and 45%. Increasing from 2 to 5 layers, the average pore size reduced from ∼490 nm to ∼210 nm, while porosity decreased from 61% to 47%. The membranes for the chemoattractant induced behavioural response study were printed using three 100 μm layers to enable diffusion-driven transport of small and large molecules while preventing particulate matter from the nutrient source from obscuring observation. Chemoattractant induced behavioural response studies were conducted over 30 min, and the devices allowed for quantitative evaluation of the response with good repeatability between devices and minimal manual handling. Combined results from six devices demonstrated that zebrafish embryos spent a significantly greater amount of time in the chemoattractant side of the devices (25.53 ± 3.58 min) compared to that in the blank side (4.47 ± 3.58 min). Similarly, planarians spent a significantly greater amount of time in the chemoattractant side of the devices (26.01 ± 3.31 min) compared to that in the blank side (3.99 ± 3.31 min). Using the optimized manufacturing method, 12 devices were fabricated in 45 min without the need for manual alignment. With the printer cost of ≪US$1000 and a material cost of <US$1 per device, in-house manufacturing of custom membrane-integrated fluidic devices is accessible to most laboratories, enabling novel, exploratory sensory–motor response studies using sophisticated designs. Future applications of custom membrane-integrated devices are anticipated to range from early-stage pre-clinical drug discovery and toxicology studies to environmental research.
Author contributions
HB: formal analysis, investigation, methodology, validation visualization, writing – original draft. AS: formal analysis, investigation, methodology, validation visualization, writing – review & editing. SL: formal analysis, investigation, methodology, validation visualization, writing – review & editing. LD: conceptualization, formal analysis, funding acquisition, investigation, methodology, project administration, resources, supervision, visualization, writing – review & editing. ED: conceptualization, investigation, methodology, supervision, writing – review & editing. RA: conceptualization, investigation, methodology, supervision, writing – review & editing. DY: conceptualization, formal analysis, investigation, methodology, project administration, resources, supervision, visualization, writing – review & editing. RG: conceptualization funding acquisition, methodology project administration, resources, supervision, visualization writing – review & editing.
Conflicts of interest
There are no conflicts to declare.
Acknowledgements
Telisia Jayne Smith and Kamal Poudel (Deakin University) are acknowledged for helping with the zebrafish embryo collection and husbandry. Dr Antoine Dujon and Ella Louise Bowley (Deakin University) are acknowledged for assistance with planaria collection and husbandry. HKB acknowledges the receipt of a scholarship from Deakin University's Faculty of Science, Engineering, and Built Environment and the Institute of Frontier Materials. LFD acknowledges Khalifa University through project RC2-2019-007. DY acknowledges an Alfred Deakin Postdoctoral Fellowship from Deakin University.
References
- H. Fotowat and F. Gabbiani, Annu. Rev. Neurosci., 2011, 34, 1–19 CrossRef CAS PubMed.
- J. Case-Smith, L. L. Weaver and M. A. Fristad, Autism, 2014, 19, 133–148 CrossRef PubMed.
- J. P. Owen, E. J. Marco, S. Desai, E. Fourie, J. Harris, S. S. Hill, A. B. Arnett and P. Mukherjee, NeuroImage Clin., 2013, 2, 844–853 CrossRef PubMed.
- R. J. Egan, C. L. Bergner, P. C. Hart, J. M. Cachat, P. R. Canavello, M. F. Elegante, S. I. Elkhayat, B. K. Bartels, A. K. Tien, D. H. Tien, S. Mohnot, E. Beeson, E. Glasgow, H. Amri, Z. Zukowska and A. V. Kalueff, Behav. Brain Res., 2009, 205, 38–44 CrossRef CAS PubMed.
- H. A. Burgess and M. Granato, J. Neurosci., 2007, 27, 4984 CrossRef CAS PubMed.
- E. M. Wielhouwer, S. Ali, A. Al-Afandi, M. T. Blom, M. B. Olde Riekerink, C. Poelma, J. Westerweel, J. Oonk, E. X. Vrouwe, W. Buesink, H. G. J. vanMil, J. Chicken, R. van't Oever and M. K. Richardson, Lab Chip, 2011, 11, 1815–1824 RSC.
- Y. Li, F. Yang, Z. Chen, L. Shi, B. Zhang, J. Pan, X. Li, D. Sun and H. Yang, PLoS One, 2014, 9, e94792 CrossRef.
- W. Wang, X. Liu, D. Gelinas, B. Ciruna and Y. Sun, PLoS One, 2007, 2, e862 CrossRef PubMed.
- A. Priyam, P. P. Singh, L. O. B. Afonso and A. G. Schultz, Environ. Sci.: Nano, 2022, 9, 1364–1380 RSC.
- Y. Umesono, J. Tasaki, K. Nishimura, T. Inoue and K. Agata, Eur. J. Neurosci., 2011, 34, 863–869 CrossRef.
- D. Blackiston, T. Shomrat, C. L. Nicolas, C. Granata and M. Levin, PLoS One, 2010, 5, e14370 CrossRef.
- C. Hicks, D. Sorocco and M. Levin, J. Neurobiol., 2006, 66, 977–990 CrossRef.
- F. Yang, Z. Chen, J. Pan, X. Li, J. Feng and H. Yang, Biomicrofluidics, 2011, 5, 024115 CrossRef PubMed.
- K. B. Tierney, Biochim. Biophys. Acta, Mol. Basis Dis., 2011, 1812, 381–389 CrossRef CAS PubMed.
- F. Kermen, L. Darnet, C. Wiest, F. Palumbo, J. Bechert, O. Uslu and E. Yaksi, BMC Biol., 2020, 18, 66 CrossRef PubMed.
- S. M. Lindsay and R. G. Vogt, Chem. Senses, 2004, 29, 93–100 CrossRef PubMed.
- F. Ahmad, L. P. J. J. Noldus, R. A. J. Tegelenbosch and M. K. Richardson, Behaviour, 2012, 149, 1241–1281 Search PubMed.
- K. J. Herrera, T. Panier, D. Guggiana-Nilo and F. Engert, Curr. Biol., 2021, 31, 782–793.e783 CrossRef CAS PubMed.
- T. Inoue, H. Hoshino, T. Yamashita, S. Shimoyama and K. Agata, Zoological Lett., 2015, 1, 7 CrossRef PubMed.
- A. Nady, A. R. Peimani, G. Zoidl and P. Rezai, Lab Chip, 2017, 17, 4048–4058 RSC.
- R. Candelier, M. Sriti Murmu, S. Alejo Romano, A. Jouary, G. Debrégeas and G. Sumbre, Sci. Rep., 2015, 5, 12196 CrossRef CAS PubMed.
- D. B. Weibel, W. R. DiLuzio and G. M. Whitesides, Nat. Rev. Microbiol., 2007, 5, 209–218 CrossRef CAS PubMed.
- J.-H. Kang, Y. T. Kim, K. Lee, H.-M. Kim, K. G. Lee, J. Ahn, J. Lee, S. J. Lee and K.-B. Kim, Nanoscale, 2020, 12, 5048–5054 RSC.
- J. de Jong, R. G. H. Lammertink and M. Wessling, Lab Chip, 2006, 6, 1125–1139 RSC.
- S. Waheed, J. M. Cabot, N. P. Macdonald, T. Lewis, R. M. Guijt, B. Paull and M. C. Breadmore, Lab Chip, 2016, 16, 1993–2013 RSC.
- P. J. Kitson, S. Glatzel, W. Chen, C.-G. Lin, Y.-F. Song and L. Cronin, Nat. Protoc., 2016, 11, 920–936 CrossRef CAS PubMed.
- B. C. Gross, J. L. Erkal, S. Y. Lockwood, C. Chen and D. M. Spence, Anal. Chem., 2014, 86, 3240–3253 CrossRef CAS PubMed.
- S. J. Hollister, Nat. Mater., 2005, 4, 518–524 CrossRef CAS PubMed.
- X. Li, R. Cui, L. Sun, K. E. Aifantis, Y. Fan, Q. Feng, F. Cui and F. Watari, Int. J. Polym. Sci., 2014, 2014, 829145 Search PubMed.
- S. A. Skoog, P. L. Goering and R. J. Narayan, J. Mater. Sci.: Mater. Med., 2014, 25, 845–856 CrossRef CAS PubMed.
- Y. Zhou, J. Biomed. Sci., 2017, 24, 80 CrossRef PubMed.
- H. K. Balakrishnan, E. H. Doeven, A. Merenda, L. F. Dumée and R. M. Guijt, Anal. Chim. Acta, 2021, 1185, 338796 CrossRef CAS PubMed.
- H. Kalathil Balakrishnan, F. Badar, E. Doeven, J. Novak, A. Merenda, L. Dumee, J. Loy and R. M. Guijt, Anal. Chem., 2021, 93(1), 350–366 CrossRef.
- V. Hahn, P. Kiefer, T. Frenzel, J. Qu, E. Blasco, C. Barner-Kowollik and M. Wegener, Adv. Funct. Mater., 2020, 30, 1907795 CrossRef CAS.
- F. Li, P. Smejkal, N. P. Macdonald, R. M. Guijt and M. C. Breadmore, Anal. Chem., 2017, 89, 4701–4707 CrossRef CAS PubMed.
- D. W. Johnson, C. Sherborne, M. P. Didsbury, C. Pateman, N. R. Cameron and F. Claeyssens, Adv. Mater., 2013, 25, 3178–3181 CrossRef CAS PubMed.
- X. Mu, T. Bertron, C. Dunn, H. Qiao, J. Wu, Z. Zhao, C. Saldana and H. J. Qi, Mater. Horiz., 2017, 4, 442–449 RSC.
- Z. Dong, M. Vuckovac, W. Cui, Q. Zhou, R. H. A. Ras and P. A. Levkin, Adv. Mater., 2021, 33, 2106068 CrossRef CAS PubMed.
- F. Mayoussi, E. H. Doeven, A. Kick, A. Goralczyk, Y. Thomann, P. Risch, R. M. Guijt, F. Kotz, D. Helmer and B. E. Rapp, J. Mater. Chem. A, 2021, 9, 21379–21386 RSC.
- H. Kalathil Balakrishnan, S. Lee, L. Dumee, E. H. Doeven, R. Alexander, D. Yuan and R. M. Guijt, Nanoscale, 2023, 15, 10371–10382 RSC.
- F. Mayer, D. Ryklin, I. Wacker, R. Curticean, M. Čalkovský, A. Niemeyer, Z. Dong, P. A. Levkin, D. Gerthsen, R. R. Schröder and M. Wegener, Adv. Mater., 2020, 32, 2002044 CrossRef CAS PubMed.
- H. K. Balakrishnan, L. F. Dumée, A. Merenda, C. Aubry, D. Yuan, E. H. Doeven and R. M. Guijt, Small Struct., 2023, 4, 2200314 CrossRef CAS.
- A. Shahriari, L. S. Khara, W. T. Allison and K. B. Tierney, Behaviour, 2021, 158, 355–375 Search PubMed.
- H. Gong, B. P. Bickham, A. T. Woolley and G. P. Nordin, Lab Chip, 2017, 17, 2899–2909 RSC.
- A. Priyam, P. P. Singh, L. O. B. Afonso and A. G. Schultz, NanoImpact, 2022, 25, 100387 CrossRef CAS PubMed.
- M. Carve and D. Wlodkowic, Micromachines, 2018, 9, 91 CrossRef PubMed.
- B. Zeng, Z. Cai, J. Lalevée, Q. Yang, H. Lai, P. Xiao, J. Liu and F. Xing, Toxicol. In Vitro, 2021, 72, 105103 CrossRef CAS PubMed.
- J. M. Korde and B. Kandasubramanian, Biomater. Sci., 2018, 6, 1691–1711 RSC.
- A. Urrios, C. Parra-Cabrera, N. Bhattacharjee, A. M. Gonzalez-Suarez, L. G. Rigat-Brugarolas, U. Nallapatti, J. Samitier, C. A. DeForest, F. Posas, J. L. Garcia-Cordero and A. Folch, Lab Chip, 2016, 16, 2287–2294 RSC.
- Y. Li, H. D. Tolley and M. L. Lee, Anal. Chem., 2009, 81, 9416–9424 CrossRef CAS PubMed.
- C. Achille, C. Parra-Cabrera, R. Dochy, H. Ordutowski, A. Piovesan, P. Piron, L. Van Looy, S. Kushwaha, D. Reynaerts, P. Verboven, B. Nicolaï, J. Lammertyn, D. Spasic and R. Ameloot, Adv. Mater., 2021, 33, 2008712 CrossRef CAS.
- M. Reymus, N. Luemkemann and B. Stawarczyk, Int. J. Comput. Dent., 2019, 231–237 Search PubMed.
- L. Schittecatte, V. Geersten, D. Bonamy, T. Nguyen and P. Guenoun, MRS Commun., 2023, 13, 357–377 CrossRef CAS.
- P. J. E. M. van der Linden, A. M. Popov and D. Pontoni, Lab Chip, 2020, 20, 4128–4140 RSC.
- P. Hu, K. L. Ly, L. P. H. Pham, A. E. Pottash, K. Sheridan, H.-C. Wu, C.-Y. Tsao, D. Quan, W. E. Bentley, G. W. Rubloff, H. O. Sintim and X. Luo, Lab Chip, 2022, 22, 3203–3216 RSC.
|
This journal is © The Royal Society of Chemistry 2024 |