A review of simulation experiment techniques used to analyze wildfire effects on water quality and supply†
Received
2nd February 2022
, Accepted 20th June 2022
First published on 22nd June 2022
Abstract
This review addresses the critical knowledge gap of techniques simulating combustion and heating characteristics present in natural wildfires and their use in assessing postfire impacts on water quality and quantity. Our assessment includes both laboratory and plot-scale techniques with burn and rainfall simulation components. Studies included focus on advancing understanding of changes in chemical and physical properties of soil, as well as subsequent runoff changes. Advantages of simulation experiments include: overcoming logistical challenges of collecting in situ wildfire data, reducing the high spatial variability observed in natural settings (i.e., the heterogeneity of burn intensity and the underlying vegetation and soil properties), and controlling the magnitude of key drivers of wildfire impacts. In sum, simulation experiments allow for more direct attribution of water quality and quantity responses to specific drivers than experiments conducted in situ. Drawbacks of simulation techniques include the limitation of observing only local-scale processes, the potential misrepresentation of natural settings (i.e., lack of spatial variability in vegetation, soil structure, burn intensity, etc.), uncertainty introduced through experimental error, and subsequent challenges in upscaling results to larger scales relevant for water management. This review focuses primarily on simulation techniques, with the goal of providing a foundation of knowledge for the design of future simulation experiments.
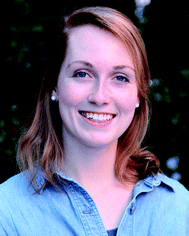 Carli P. Brucker | Carli P. Brucker received her B.S. in Mechanical Engineering from the University of Iowa and her Master’s degree from the University of Colorado Boulder where she is a current PhD candidate in Civil, Environmental, and Architectural Engineering Department. Her current research addresses the broad challenge of quantifying the impacts of wildfires on hydrologic and water quality responses in watersheds in the U.S. West. These efforts include the design and creation of laboratory-scale wildfire and rainfall simulation experiments, as well as modeling wildfire impacts on water quality and supply using physical models and machine learning techniques. |
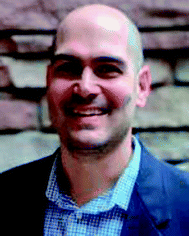 Ben Livneh | Ben Livneh received his PhD in Civil Engineering from the University of Washington-Seattle. He is currently a professor at the University of Colorado Boulder in the Civil, Environmental, and Architectural Engineering Department with a joint appointment at the Cooperative Institute for Research in Environmental Sciences. His research group explores the impacts of changing land-cover and climate on water resources. They seek to quantify changes in the mean-state and variability of hydrologic systems, identifying key processes and exploring predictability. Active areas of research include sediment transport, land-cover disturbance, hydrologic connectivity, forecasting, and estimating components of the surface water balance from space. |
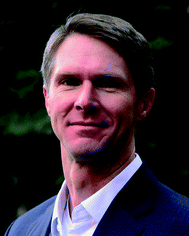 J. Toby Minear | J. Toby Minear Dr Minear received his undergraduate degree from Colorado College and his Masters and PhD degrees from the University of California, Berkeley. Before joining Cooperative Institute for Research in the Environmental Sciences, he worked as a Research Hydrologist at the United States Geological Survey at the California Water Science Center and the National Research Program. Dr Minear works on a variety of river research and hydrodynamic modeling projects including the NOAA National Water Model, is a Science Team and Cal/Val Team member for the upcoming NASA Surface Water and Ocean Topography Mission, and serves on the U.S. Subcommittee on Sedimentation. |
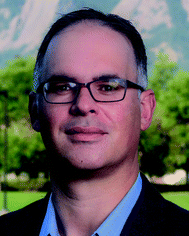 Fernando L. Rosario-Ortiz | Fernando L. Rosario-Ortiz received his D.Env. in Environmental Science and Engineering from UCLA in 2006. He is a professor in the Department of Civil, Environmental, and Architectural Engineering and the Environmental Engineering Program at the University of Colorado Boulder. His main research interests focus on environmental chemistry and water quality. |
Environmental significance
Wildfires affect water quality through increased concentrations of sediment, metals, nutrients, and dissolved organic matter in runoff. This can create hazards for drinking water treatment plants, reservoirs, and freshwater ecosystems. Laboratory- and plot-scale wildfire simulation experiments are a valuable tool used to study these effects. This review summarizes the state of the art of wildfire and rainfall simulation experiment methodologies, including the strengths and weaknesses of each technique. This knowledge can help inform researchers in the design of their own simulation experiments, ultimately informing water management efforts to prepare for and mitigate potential water-related impacts of future wildfires.
|
1 Introduction
Forested watersheds are an important source of high quality water for billions of consumers worldwide, providing nearly two-thirds of potable water in the U.S.1,2 Forests serve to capture and store precipitation, lowering the risk of flooding events.1 The natural filtration processes that occur in watersheds provide major economic benefits, saving an estimated 4.1 trillion USD per year globally in water treatment costs.2,3
Wildfires are a natural disturbance mechanism which support the long-term health of forested ecosystems,4 but also can degrade stream water quality and alter runoff generation mechanisms. Wildfire-driven increases in sediment, dissolved organic matter (DOM), nutrients, and heavy metals1,2,5–7 can necessitate investments in infrastructure and altered methods of treatment in water treatment plants,2,8–11 diminish reservoir storage due to sediment filling, and disturb freshwater ecosystems.1,12 Additionally, increased post-fire runoff generation can produce high peak flows, increasing flood risks.14 These effects (Fig. 1) occur immediately after a wildfire, but impacts can persist for up to 10 years.13
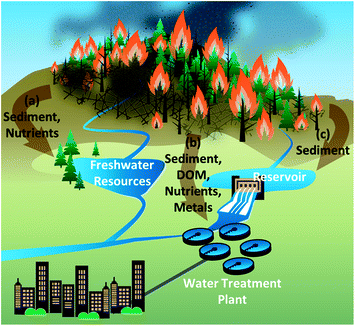 |
| Fig. 1 Conceptual illustration of the impacts of wildfire on water quality and supply. The constituent fluxes in streams are driven by burning effects, affecting human and natural systems. Increased sediment and nutrient concentrations (a) drive eutrophication and disturb freshwater resources; increased sediment, DOM, nutrient, and heavy metal concentrations (b) can exceed water treatment plant treatment capacities; and increased sediment loads (c) affect reservoir storage capacity. | |
In recent decades, an increase in wildfire size, frequency, and severity has been observed in certain forested regions—a trend predicted to continue.15–18 For example, the mean annual burn area in the Western U.S. has doubled since 1984 and is projected to have a 24–169% increase in mean burn area by midcentury.18–21 Current understanding of wildfire effects on water quality and supply is incomplete, such that research is critically needed to assist water managers in adaptation and mitigation strategies.1,7,10
Hindrances in the collection of post-wildfire in situ data—unstable terrain and road closures immediately after wildfires, lack of comparable pre-burn control data, and high natural spatial and temporal variability—have contributed to a lack of knowledge of wildfire effects.2,10,11 Additionally, post-wildfire response varies greatly regionally due to differences in soil and vegetation regimes, as well as climate.22 Thus, insights from an in situ study are generally not directly transferable to another geographic area. Limited information exists about post-wildfire water quality response, in particular, with most studies primarily focusing on DOM, nutrients, and metals—leaving the responses of microbial constituents and anthropogenic contaminants largely unknown.23
Laboratory- and plot-scale simulation experiments which replicate burning and combustion mechanisms present in natural wildfires, as well as raindrop kinetic energies and leaching effects similar to post-wildfire precipitation, offer an alternative analytical technique for estimating wildfire effects on water quality and supply.2,7,24–28 Hereafter, these two types of simulation experiments will be referred to as wildfire and rainfall simulators, respectively. These studies primarily advance understanding of burn effects on small-scale soil and water physical and chemical properties. The controlled setting of these simulation experiments can overcome logistical issues associated with in situ studies, as well as provide insight into regionally-specific drivers by allowing for more precise attribution of responses to controls. However, this type of analysis also presents new limitations, including dissimilarities to natural settings and difficulties in upscaling results to the catchment scale.24,29
2 Scope of review
The overarching scope of this review is to provide a critical assessment of existing laboratory- and plot-scale wildfire and rainfall simulation techniques used to measure the effects of wildfires on water quality and supply. Terminology is defined in Section 3, followed by a review of post-wildfire hydrologic and water quality responses in Section 4, including their implications for human and natural systems. Section 5 presents a review of simulation experiment techniques for both wildfire and rainfall processes, providing strengths and weaknesses for both laboratory- and plot-scale methods. Based on the merits of each method, recommendations for experimental design are provided in Section 6, followed by overall conclusions in Section 7.
A novel contribution of this review is the focus on methodological techniques—specifically, simulation experiments—used to generate and collect wildfire response data, rather than the data themselves.13,30,31 We include brief analyses of common hydrologic and water quality response data from reviewed simulation experiment studies (a summary of the reviewed data is provided in the ESI†). However, this is not the focus of this review due to studies' wide ranges of research goals, temporal and physical scales, and key experimental factors—making useful cross-study comparisons difficult. Overall, this review is distinct from existing reviews, which primarily summarize the state of the art of wildfire effects on hydrology,31–37 sediment transport and erosion,12,13,38–40 and streamflow concentrations of DOM and nutrients (i.e., nitrogen and phosphorous),2,41–46 as well as metals.1,30
One notable exception is Ferreira et al. (2008), which discusses limitations of common methods and techniques used to analyze hydrologic and erosional responses in post-wildfire settings, from laboratory to catchment scales. However, while Ferreira et al. (2008) does cover rainfall simulation methods, the present manuscript is the first to provide in-depth review of wildfire simulation techniques together with associated rainfall simulators. Additionally, where Ferreira et al. (2008) focuses on hydrologic and sedimentation impacts of fire, this review has an additional focus on postfire water quality impacts including DOM, nutrient, and heavy metal concentrations. Finally, as over a decade has passed since the Ferreira et al. (2008) review, the time is ripe for a fresh perspective that considers more recent research on simulation experiment techniques, drawing upon a larger pool of studies.
The studies reviewed in this paper were compiled using the search strings of “wildfire experiments”, “wildfire laboratory simulations”, and variations of those in the Google Scholar search engine. These were further filtered by laboratory- and/or plot-scale studies, and those which had research goals focused on hydrologic or water quality responses, as well as temperature profiles in soils. Studies examining post-fire air quality, wildfire behavior, and ecosystem restoration were excluded. From these studies, only those analyzing burn effects of natural fuels (i.e., litter and woody biomass), rather than human infrastructure and anthropogenic fuels (i.e., plastics and metals), were included. Additional studies were identified through the internal references across the initial set of publications. In total, 40 studies were included: 23 had a wildfire simulation component and 27 had a rainfall simulation component, with 10 studies implementing both elements.
3 Terminology
A variety of wildfire characterization terms are commonplace across studies, despite calls for standardization in recent decades.47–50 In this review, wildfire is used to describe fires which occur in a natural environment, though bushfire is an interchangeable term common in Australia. Following are the definitions for wildfire intensity and wildfire severity as used in this review, as well as other relevant wildfire and rainfall simulation terms.
Wildfire intensity: is typically a quantitative characterization of energy, such as the amount of fuel burned (e.g., units of g),22 the rate of fuel burned (e.g., units of g s−1 or W m−2),22,51 or peak temperature (e.g., units of °C).24,48,49,52–54 This allows for explicit quantifications of mild to severe burn intensities, however the lack of a standardized scale across studies makes cross-comparisons difficult.2,22,55,56
Wildfire severity: is typically a visual characterization of the response of an ecosystem (i.e., vegetation, soil, water systems, and atmosphere) to fire,5,47,50 such as ash color or amount of biomass consumed.29,57 Similar to wildfire intensity, a standard definition of a wildfire severity range from low to high does not exist across studies.38,48,58
Laboratory-scale simulations: of wildfire and rainfall are typically applied to smaller soil samples (∼0.0045–4 m2)26,59,60 using experimental apparati. These analyses occur either inside a laboratory or in a designated outdoor setting,29,61,62 limiting the size of samples to the dimensions of the equipment used.
Plot-scale simulations: of wildfire and rainfall typically occur over a larger area of ground or hillslope (∼0.5–300 m2)—either undisturbed or where a natural wildfire has already occurred.63–65 If undisturbed, a prescribed burn is typically applied to the area, then rainfall simulators used to generate runoff.66,67 These experiments are also referred to as field-scale or hillslope-scale experiments, but in this review only the term plot-scale is used.
Rainfall intensity: is the ratio of the total rain depth (e.g., units of mm) to the duration of rainfall (e.g., units of minutes, hours, or days).
Slope: is the average topographical inclination or gradient across a terrain surface (e.g., units of degrees or percent).
4 Wildfire-driven effects on water quality and supply
4.1 Sedimentation
Wildfire-driven increases in suspended sediment in streamflow have been reported from 1 to up to 1459 times pre-disturbance levels.13 Downstream impacts of elevated sediment loading include strain on water treatment plants, reductions in reservoir storage capacity, and disruption to freshwater ecosystems.1,11,12 Increased sediment loads can require increases in water treatment plant infrastructure and monitoring.1,11,68 Sediment-driven reservoir filling reduces the storage capacity, limiting available water for municipalities, and is expensive to mitigate using dredging or tunnels.69–71 Finally, increased turbidity from wildfire-driven sediment limits sunlight needed for photosynthesizing organisms that produce oxygen and form the base of aquatic food chains, resulting in fish death.72–74
Sedimentation is enhanced by erosional effects due to loss of vegetation (both ground and canopy cover) and root structure,27,75,76 exacerbated by high runoff rates in post-fire settings. Rill erosion, for example, expands channel networks through high-flow rate runoff flowing through streamlets – the eroded soil mobilized into suspended sediment.76 These high post-fire runoff rates can be driven by increased soil water repellency75,77,78 thought to occur during heating and combustion processes in wildfires.13,43,75,79–81 Organic compounds in litter and topsoil can be volatilized and fill the pores of upper soil layers creating water repellent effects,29 further increased by the melting and redistribution of waxes and organic molecule polymerization.81
Sediment is further supplied by an accumulation of combusted vegetation and soil organic material (i.e., ash), mobilized by erosional effects.77 Initially, ash can absorb and store large amounts of rainfall (in one case, 99% for a ∼15 mm precipitation storm), thereby limiting runoff generation.79 In subsequent or larger storms when ash saturation occurs, runoff generation increases, exacerbated by increased water repellency, and ash is mobilized downstream—contributing to high sedimentation rates.79,82
4.2 Dissolved organic matter
Post-fire runoff DOM response varies widely across studies, from slight decreases in concentration to levels in the 95th percentile of pre-fire conditions.1,10,13,28,43,60,83–85 Downstream, DOM is the main substrate in the formation of carcinogenic disinfection byproducts (DBPs) during the chlorination stage of water treatment.60 Elevated levels can require water treatment plants to implement expensive alternate disinfectants, precursor (i.e., DOM) and DBP removal strategies, or even force them to shut down.84,86,87
Alterations in the load and chemistry of DOM in post-fire settings is driven by thermal reactions during burning.87,88 However, isolating wildfire-driven DOM response is challenging because of natural background sources of DOM, contributed by other hydrological, topographical, physicochemical, and microbiological processes.2,56 DOM levels monotonically decrease89 or remain the same61 with increasing burn severity in some studies, while others report peak DOM concentrations under moderate burn severity conditions.5,26,30,83,90 Lower DOM concentrations are frequently reported at high burn intensities, likely due to DOM vaporization (transformation of organic material into carbon dioxide and water vapor) at extreme temperatures.6,43,89
4.3 Nutrients
Wildfire-driven increases in the nutrients phosphorous and nitrogen have been reported up to 250 and 400 times pre-burn conditions, respectively.1,6,13 These dramatic increases in nutrients can lead to eutrophication in aquatic ecosystems.91 Eutrophication is a process where excess nutrients lead to accelerated growth of aquatic plants and benthic communities, but also algal blooms which produce toxins and deplete oxygen from the ecosystem.1,91,92 These effects, in addition to the impacts of sediment discussed earlier, result in the mortality of fish and other aquatic species, as well as loss of biodiversity.85,91,92
Many complex mechanisms contribute to changes in nutrients in post-fire settings.1,43,93–95 However, studies have generally shown higher rates of nitrogen concentrations after burning due to deposition and changes in chemical structure.1,43,93 Increases in phosphorous can result from constituent transport through increased post-wildfire sedimentation rates.68
4.4 Heavy metals
Though heavily dependent on the nature of the forest and climatic conditions, increases in dissolved heavy metal concentrations in runoff driven by wildfires can range from 2 to at least 2500 times pre-disturbance levels.30,96 These increases can exceed federal regulations, posing health hazards as some metals are carcinogenic or can cause anemia or heart failure.97 Increases in magnesium are of particular concern due to toxicity, volatility, and persistence in the environment.98,99 Iron, manganese, arsenic, chromium, aluminum, barium, and lead have all been observed at statistically significantly higher concentrations in post-fire runoff.13 Moderate to high intensity fires can alter soil properties, releasing sequestered metals in organic matter which are transported downstream by high post-fire flowrates.30,100
5 Experimental techniques to observe and quantify post-fire impacts
The strengths of wildfire and rainfall simulation experiments are in the reduction of the logistical challenges associated with collecting in situ data, the ability to generate baseline pre-fire data as well as replicate samples, and to provide greater control over factors in post-fire systems.10,13,24,29 Greater control over the timescale and number of samples collected is conducive to isolating wildfire responses from background sources.10,38 Limitations of simulation experiments include deviation from natural conditions, e.g. vegetation characteristics and lateral flow paths, as well as significant differences in hydrologic and chemical processes at different scales.7,29,67 Interpretation of results from laboratory- and plot-scale simulation experiments for catchment scale impacts should incorporate uncertainties, such as landscape heterogeneity, associated with the upscaling process. The following section will first discuss key differences in plot- versus laboratory-scale simulation techniques, then present the strengths and weaknesses of specific methodologies for wildfire and rainfall simulation experiments. Fig. 2 shows how laboratory-, plot-, and catchment-scale studies are related in terms of size, as well as common simulation apparati used at the laboratory and plot scales.
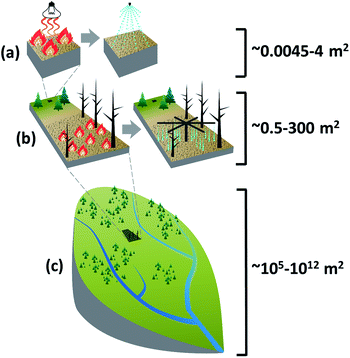 |
| Fig. 2 Illustration of laboratory-, plot-, and catchment-scale post-wildfire study areas, with (a) a heat lamp and spray nozzle being used at the laboratory scale (∼0.0045–4 m2) for wildfire and rainfall simulation, respectively; (b) a prescribed burn and rotating-boom rainfall simulator (similar to the Swanson-style rainfall simulator105) being used for plot-scale (∼0.5–300 m2) wildfire and rainfall simulation, respectively; and (c) a catchment (∼105–1012 m2) affected by wildfire. | |
5.1 Plot- versus laboratory-scale simulation techniques
Plot-scale experiments are conducted on in situ hillslopes or plots and are generally subject to higher spatial variability in soil properties and vegetation, as well as spatial and temporal variability in burning and rainfall, than laboratory-scale experiments.101 Though high variability can hinder the attribution of responses to drivers, this type of analysis also minimizes area-to-edge ratios (i.e., limiting edge effects) and allows for larger-scale vegetation (e.g., trees) and hydrologic processes to be captured.67,76 Larger-scale hydrologic processes, i.e. rill formation and other erosional processes, can be key drivers of post-wildfire sedimentation and runoff responses76 and thus important to consider in post-wildfire analyses. A wide range of replicates are typically tested in plot-scale analyses, from 0 to approximately 15.76,102
Laboratory-scale experiments typically use excavated, intact soil cores or homogenized samples which have low spatial variability, due in part to their smaller size.54,67,76,89,103 Samples are typically excavated by hammering lysimeter boxes or PVC cylinders into soil89,90 or collected from loose soil and litter on the ground—often homogenized to minimize variability.26,54,102,104 Laboratory-scale samples are often less representative of natural conditions in terms of wildfire intensity, erosional processes, vegetation type, and soil structure, but allow for precise control over experimental factors. This is conducive to more direct attribution of the impacts of burning and other factors in the system (e.g., vegetation, rainfall intensity, etc.) to observed responses.29 Another key feature of laboratory-scale analyses is they allow for the measurement of infiltration through the bottom of samples and subsurface later flows, which is difficult or impossible in plot-scale in situ analyses.29,90,104 A comparison of the pros and cons of plot- and laboratory-scale analyses are presented in Table 1. The number of replicate samples in laboratory-scale analyses typically range from 2–5.56,106
Table 1 Comparison of pros and cons of plot- and laboratory-scale simulation experiments based on the following factors: spatial and temporal variability, control over environmental factors, representation of natural environments, edge effects, and subsurface flows. An N/A entry indicates that no significant pro or con exists for that category
|
Plot-scale (∼0.5–300 m2) |
Laboratory-scale (∼0.0045–4 m2) |
Pros |
Cons |
Pros |
Cons |
Spatial and temporal variability |
Higher variability in climatic, burn, rainfall, and soil and vegetation factors similar to the natural environment |
Higher variability can hinder attribution of responses to drivers |
Lower variability allows for greater attribution of responses to drivers |
Lower variability not as representative of the natural environment |
Control over environment and experimental factors |
N/A |
Little control over climatic conditions and other factors in experimental environment |
Precise control over climatic, burn, and rainfall factors, as well as soil and vegetation characteristics |
N/A |
Representation of natural environments |
Wildfire intensity |
Able to simulate high intensity fires |
Trade-off between scale and fire intensity (i.e., high intensity typically applied on smaller scales) |
N/A |
Typically only low to moderate intensity fires simulated |
Hydrologic and erosional processes |
Allows for some larger-scale erosional processes such as rill formation |
Does not capture basin-scale erosional processes such as streambed erosion |
N/A |
Larger-scale hydrologic processes typically not represented |
Vegetation |
Larger-scale vegetation such as trees represented |
N/A |
N/A |
Typically only small-scale vegetation represented |
Soil structure |
Soil minimally disturbed or not at all |
N/A |
N/A |
Soil structure may be compromised during excavation or purposefully homogenized |
Edge effects |
Minimized area-to-edge ratio |
N/A |
N/A |
Greater edge effects |
Subsurface flows |
N/A |
Typically does not allow for measurements of subsurface flows |
Allows for the analysis of infiltration and lateral subsurface flows |
N/A |
5.2 Wildfire simulation
Prescribed and slash burns, propane torches and heat lamps, litter burns, and muffle furnaces have all been used to study the effects of burning on soil and runoff physical and chemical properties.22,26,54,67,83,90,103 The key features which differ across these techniques are the range of simulated wildfire intensities, methods of burn characterization, and spatial variability of the combustion and burn properties. These studies are tabulated in Table 2 and are described in the following sub-sections.
Table 2 Summary of major wildfire simulation studies included in this review, listed in alphabetical order. The characteristics included are scale, size and shape of samples, wildfire simulation technique, peak burning temperature, duration of the burn, and a summary of key results from each study. The provided summaries are reported with respect to unburned conditions unless otherwise stated, e.g. a percent decrease indicates the percent decrease of the response after burning from the control or unburned case in the study. An N/A entry denotes an unreported study characteristic, WR is water repellency, TOC is total organic carbon, PyC is pyrogenic carbon, O horizon is organic soil horizon, Ah horizon is soil mineral horizon, KCl is potassium chloride, DOC is dissolved organic carbon, and DON is dissolved organic nitrogen
Scale |
Size and shape of samples |
Simulation technique |
Peak burn temp |
Duration of burn |
Hydrologic and water quality results |
Source |
Laboratory |
0.25 × 0.25 × 0.15 m |
Propane torch or heat lamps |
500 °C |
∼220 min |
WR, TOC, PyC significantly decreased in O horizon (98, 47, 74%, respectively) |
Badía-Villas et al., 2014 |
WR, PyC significantly decreased in upper cm Ah horizon (74, 38%, respectively) |
Laboratory |
N/A |
Muffle furnace |
100, 150, 200, 250, 300, 350, 400, 450, and 500 °C |
5, 10, 15, and 30 min |
KCl-extractable organic anion concentrations peaked between 150 and 350 °C, significantly affected by duration of heating |
Blank et al., 1994 |
Laboratory |
0.3 m diam., 0.2 m height |
Litter burn |
230–867 °C |
1–9.9 h |
Soil moisture significantly affected heat transfer (>20% moisture quenched heating lower than 2.5 cm) |
Busse, et al., 2010 |
Laboratory |
0.2 × 0.4 × 0.15 m |
Propane torch or heat lamps |
200 and 400 °C |
Until temp reached, cooled afterwards |
Degree hours (°C h measured during burning) were positively linearly related (R2 = 0.966) to sum of Na, K, Ca, and Mg cations |
Cancelo-Gonzalez, et al., 2012 |
Laboratory |
0.45 × 0.2 × 0.15 m |
Propane torch or heat lamps |
200 and 400 °C (at 1 cm depth) |
Until temp reached, cooled afterwards |
Leached cations increased significantly (114% and 183% in moderate and severe burns, respectively) |
Cancelo-Gonzalez, et al., 2013 |
Laboratory |
0.2 × 0.4 × 0.15 m |
Propane torch or heat lamps |
200 and 400 °C (at 1 cm depth) |
Until temp reached, cooled afterwards |
Al and DOC increased 19 and 21%, respectively, for moderate burns and 44 and 679% for severe burns |
Cancelo-Gonzalez, et al., 2015 |
Fe increased only at severe burns (66%) |
Laboratory |
N/A |
Muffle furnace |
225, 350, and 500 °C |
2 h |
DOC peaked at mild-moderate burns (84% increase) and was lowest for severe burns (99% decrease) |
Cawley et al., 2017 |
Laboratory |
N/A |
Muffle furnace |
149, 204, 260, 316, 371, 427, and 482 °C |
5, 10, 15, and 20 min |
Non-wettability did not increase for mild burns, increased after 10–20 min for mild-moderate burns |
Debano and Krammes, 1966 |
Impenetrability reached after 5–10 min for moderate burns, was destroyed after 10 min for severe burns |
Plot |
3 × 10.7 m |
Prescribed or slash burn |
Low intensity |
N/A |
Runoff ratio and sediment yield increases (21% and 40%, respectively) were not significantly greater than natural variability |
Emmerich and Cox, 1992 |
Plot |
0.5 m2 |
Prescribed or slash burn |
N/A |
N/A |
Infiltration significantly decreased in bunchgrass, shortgrass, and oak-dominated sites (25–36%) |
Hester et al., 1997 |
Sediment yield significantly increased for all sites (344–225,000%) |
Laboratory |
N/A |
Muffle furnace/litter burn |
Muffle: 200, 250, 300, 350, 400, 450, and 550 °C; flame: 520, 460, 350 °C |
Muffle: 2 h; flame: 200–700 s (until 5 g, 10 g, and 25 g of fuel were incinerated) |
Muffle furnace: total C, P changed monotonically with burn intensity (89% decrease and 1381% increase, respectively); total N peaked at moderate burns (385% increase), decreased at severe burns |
Hogue and Inglett, 2012 |
Litter burning: results showed no significant trends with burn intensity |
Laboratory |
0.5 × 0.5 × 0.05 m (+ litter layer) |
Muffle furnace |
225, 350, and 500 °C |
2 h |
DOC decreased significantly for mild-moderate burns (52%), DOC : DON non-significantly decreased27% |
Hohner et al., 2019 |
Laboratory |
0.48 × 0.28 × 0.05 m |
Litter burn |
250–300 °C |
Until litter was completely burned |
WR was moderate, peak runoff rates increased up to 5525%, infiltration rates decreased up to 86%, and sedimentation rates increased |
Keesstra, et al., 2014 |
Laboratory |
0.2 m diam., 0.35 m height |
Propane torch or heat lamps |
40–94 °C |
15 min |
Positive correlation (R2 = 0.90) between vesicular-arbuscular mycorrhizal colonization and soil temperature |
Klopatek et al., 1988 |
Plot |
N/A |
Prescribed or slash burn |
N/A |
N/A |
Infiltration rates and sediment yield decreased 2 and 3%, respectively, though not with statistical significance |
Knight et al., 1983 |
Laboratory/Plot |
2 × 2 m (burn box), 1.2 × 2.4 m (burn table) |
Litter burn/Propane torch or heat lamps |
650 °C (prescribed); 600 °C (burn box); 400 °C (burn table); 400 °C (propane prong) |
Until fuel burned (prescribed, burn box, burn table); 60 s (propane prong) |
Burn box, burn table, and prescribed fires had similar time–temperature profiles, but propane prong fires had most similar total heat dosages to prescribed fires |
Kral et al., 2015 |
Plot |
1 m2 |
Prescribed or slash burn |
35–563 °C |
Until done burning |
Runoff ratios and sediment yields increased 275% and 775%, respectively |
Marcos et al., 2000 |
Laboratory/Plot |
0.1 × 0.1 × 0.1 m |
Litter burn/Propane torch or heat lamps |
Low intensity |
N/A |
Root mat soils with moisture contents of 93% and 145% had a 50% and 10% probability, respectively, of sustained smouldering |
Reardon et al., 2007 |
Laboratory |
0.305 m diam. |
Propane torch or heat lamps |
100–150 °C, 250–300 °C, 400–500 °C |
N/A |
WR peaked for mild burns (moderate repellency), then decreased at moderate and severe burns (slight and no repellency, respectively) |
Robichaud and Hungerford, 2000 |
Plot |
0.83 m2 |
Prescribed or slash burn |
N/A |
N/A |
Infiltration rates for field capacity and dry conditions, respectively, significantly decreased (∼11%) and did not change |
Roundy et al., 1978 |
Sediment yields significantly increased ∼141% for all conditions |
Laboratory/Plot |
70 × 70 m (plot) |
Prescribed or slash burn/Muffle furnace |
370, 470, and 570 °C (muffle furnace) |
155, 105, and 65 s |
PyC mass decreased 7–15% for experimental fire and 1–15%, 28–63%, and 26–37% for increasing muffle furnace temperatures |
Santín et al., 2013 |
Laboratory |
0.103 m diam., 0.1 m height |
Propane torch or heat lamps |
600 °C |
5 min |
Soil moisture significantly reduced peak temperatures, duration of heating above 60 and 175 °C; rock cover reduced peak temperatures, increased heating durations |
Stoof, et al., 2011 |
Laboratory |
0.2 m diam., 0.1 m height |
Propane torch or heat lamps |
200–250 °C, 450–500 °C |
∼40 min |
Hydraulic conductivity significantly decreased for moderate burns (∼62%), increased for severe burns (∼229%) |
Wieting et al., 2017 |
TOC significantly increased for moderate burns (∼60%), decreased for severe burns (∼11%) |
5.2.1 Prescribed and slash burns.
Prescribed and slash burns are used as wildfire simulation techniques for plot-scale analysis of erosional, hydrologic, and sedimentation response to burning.63,76,107 They involve the application of an incendiary device to in situ accumulated fuels under conditions conducive to the control of a fire, i.e. low winds and high soil moisture.63,66,107–110 Either aerial or ground-based incendiary devices are used to start these fires, such as a Plastic Sphere Dispenser111 or a drip torch,112,113 respectively. Prescribed burning, also referred to as control or experimental burns,113,114 typically span a 40–200 ha (100–500 acre) area and most often burn at a low intensity, only consuming fuels with small diameters (e.g., pine needles and small branches).115 Slash burns, also referred to as pile burns,115 involve first collecting surplus woody debris (i.e., trees or brush) into a concentrated area – typically burning at a high intensity.115–117 Prescribed and slash burns are most often qualitatively characterized by visual characteristics, such as biomass consumed (i.e., wildfire severity).112,118 A wide range of responses are typical for this type of technique, with runoff increasing ∼20–300%, infiltration decreasing ∼2–40%, sediment yield increasing ∼3–200
000%, and soil chemical composition decreasing ∼7–15% with burning (typically analyzed at just one burn severity increment).63,108,113,119 However, some studies reported no statistical significance in certain results due to natural variability exceeding post-burn response.66,119
5.2.2 Propane torches and heat lamps.
Propane torches and heat lamps are common wildfire simulation techniques used in laboratory-scale analyses of burn impacts on soil and runoff chemical composition, as well as sedimentation response and changes to soil structure.61,90,106 These techniques involve a steady heat flux concentrated at a point (∼50–100 mm) on the soil surface.54,59,61,106,120,121 Propane torches typically produce peak soil surface temperatures of 500–600 °C
54,61 whereas heat lamps produce peak temperatures of 200–400 °C
90 from 5 to up to 220 minutes of exposure,54,61 representing a range in burn intensities. The temperature gradient extending down through the soil surface in these experiments is typically measured using thermocouples placed 0–15 cm below the soil surface.22,24,29,54,61,103 These studies report a wide range of significant responses to burning, with soil and water chemical constituents varying by ∼10–700% and hydraulic and water repellent properties by ∼60–200%, typically at 1–3 increments of burn intensities.62,89,121 Kral et al. (2015) showed that propane prong fires had disparate time–temperature curves compared to prescribed fires, with longer durations near peak temperatures, but the least difference in total heat dosage.
5.2.3 Litter burns.
Litter burns are a laboratory-scale wildfire simulation technique used to study burn effects on soil and runoff composition, as well as the effects of soil composition on heating profiles.29,59,103,120 The method involves igniting small amounts of litter spread evenly on top of soil samples, reaching temperatures of 230–867 °C.29,120,122 This measured amount of fuel allows for a direct and quantitative measurement of burn intensity.22,29 Additionally, similar to propane torches and heat lamps, thermocouples placed 0–15 cm below the soil surface are used to measure heating profiles during the burn process.29,59,122 Study results show substantial changes in water repellency, runoff and infiltration rates (up to a ∼6000% increase and ∼90% decrease, respectively), and sedimentation rates,29 as well as clear soil heating profiles, analyzed at typically 1–3 increments of burn intensities.59,103,120 Hogue and Inglett (2012) found significant changes in some soil chemical properties (i.e., total carbon and nitrogen) after litter burning, however no clear trend with increasing burn intensity was observed due to heterogeneity of burn residues. Kral et al. (2015) showed that litter burns had analogous time–temperature curves to prescribed fires, however often had significantly higher heat durations and dosages.
5.2.4 Muffle furnaces.
Muffle furnaces are a laboratory-scale wildfire simulation technique typically used to analyze burn impacts on soil chemical composition.26,52,113,123 Samples are placed in an oven which is typically raised to temperatures between 100-570 °C for as little as 65 seconds up to 2 hours to simulate a range of burn intensities.26,52,83,113 Results from muffle furnace studies show generally significant monotonic and parabolic-shaped trends in leached chemical constituents and water repellency, typically measured at 1–9 increments of burn intensities.22,26,52,83,113,123 Hogue and Inglett (2012) found that, unlike litter burns, muffle furnace burning did not produce heterogeneous burn residues – commonly observed in natural wildfires.
5.3 Comparison of key wildfire simulation technique characteristics
Each of the wildfire simulation experiment techniques described above has benefits and limitations which future researchers may take into consideration in their own designs. Table 3 shows a broad description the pros and cons of each technique.
Table 3 Pros and cons of wildfire simulation techniques covered in the review, as well as the studies referenced and their scales. WP1, WP2, etc. represents wildfire simulation pro 1, wildfire simulation pro 2, etc. Similarly, WC1, WC2, etc. represents wildfire simulation con 1, wildfire simulation con 2, etc
Simulation Technique |
Pros |
Cons |
Scale |
Sources |
Prescribed Fire or Slash Burn |
WP1-heterogeneous combustion similar to natural wildfires |
WC1-qualitative wildfire characterizations |
Plot |
Emmerich and Cox, 1992; Hester et al., 1997; Marcos et al. 2000; Roundy et al., 1978; Santin et al., 2013 |
WP2-similar intensity and duration as a natural wildfire |
WC2-tradeoff between intensity and size of burn |
Propane Torch or Heat Lamp |
WP3-control over burn intensity and spatial distribution |
WC3-uniformity in spatial distribution of heating |
Laboratory |
Badía-Villas et al., 2014; Cancelo-Gonzalez, et al., 2012; Cancelo-Gonzalez, et al., 2013; Cancelo-Gonzalez, et al., 2015; Klopatek et al., 1988; Robichaud and Hungerford, 2000; Stoof et al., 2011; Wieting et al., 2017 |
WP4-low variability in heating |
WP5-allowance for measurement of heating profile |
WP6-control over duration of heating |
Litter Burns |
WP1-heterogeneous combustion similar to natural wildfires |
WC4-limited to low-intensity burns |
Laboratory |
Busse, et al., 2010; Keesstra, et al., 2014 |
WP3-control over burn intensity and spatial distribution |
WP5-allowance for measurement of heating profile |
Laboratory/Plot |
Kral et al. |
Muffle Furnaces |
WP4-low variability in heating |
WC3-uniformity in spatial distribution of heating |
Laboratory |
Blank et al., 1994; Cawley et al., 2017; Debano and Krammes, 1966; Hohner et al., 2019 |
WP5-allowance for measurement of heating profile |
WC5-heating occurs from all sides |
WP6-control over duration of heating |
WP7-incremental control of burn intensity |
The following paragraphs discuss common pros of wildfire simulation experiments as listed in Table 3:
Heterogeneous combustion (WP1) is a key strength of prescribed fires and slash burns, as well as litter burns. The variability in spatial distribution of heating and volatilization created by these types of burns produce variable combustion residues analogous to natural wildfires. This variability is due to spatial heterogeneity of fuel types and amounts, as well as variable wind speed, direction, and air temperature across the burn area in plot-scale experiments.22
Similar intensity and duration to a natural wildfire (WP2) is a strength of prescribed fires and slash burns. As a comparable amount of fuel is available in a prescribed burn as a natural wildfire, prescribed fires typically match the peak temperatures and duration of a low intensity wildfire.112 Slash burns can reach the extreme temperatures reached by severe wildfires, sometimes as high as ∼2200 °C—often unachievable by laboratory-scale simulation techniques.117
Precise control over burn intensity and spatial distribution (WP3) is a strength of propane torches and heat lamps, as well as litter burns.121 This technique allows for more direct attribution of burning effects to specific intensities, given the controlled range and spatial distribution of burn intensities.29
Low variability in heating (WP4) is a benefit of propane torches and heat lamps, as well as muffle furnaces. Low spatial and temporal variability created by the consistent heating distribution of these methods is conducive to quantitative analysis, as it limits variability-driven uncertainty in responses.113
Allowance for measurement of heating profiles (WP5) is a key aspect of propane torches and heat lamps, litter burns, and muffle furnace methods. Propane torches and heat lamp methods, as well as litter burns, achieve this using thermocouples, and muffle furnaces achieve this through digital temperature readings. This allows for consistent characterization of burn intensity, conducive to quantitative post-wildfire analyses.
Control over duration of heating (WP6) is an attribute of propane torches and heat lamps, as well as muffle furnaces. This allows for further control and precision of burn intensity—sometimes characterized by burn duration.24
Incremental control over burn intensity (WP7) is a benefit unique to muffle furnaces. This feature allows for analysis of changing burn effects over a range of burn intensities—conducive to analyzing response trends with increasing burn intensity.113
The following paragraphs discuss common cons of wildfire simulation experiments as listed in Table 3:
Qualitative burn severity characterization (WC1) is a limitation for prescribed fires and slash burns.67,101 Qualitative wildfire characterizations (i.e., burn severity) are less precise than temperature or fuel measurements (i.e., burn intensity), hindering quantitative analyses and precise replication of simulated burn severities.
Tradeoff between high intensities and burn coverage (WC2) is another limitation for prescribed fires and slash burns. As these burns must be managed in a safe, controlled way, larger prescribed burns (40–200 ha) are typically limited to a low burn intensity. Slash burns, which can achieve extreme temperatures, are therefore limited to smaller areas.
Uniformity in spatial heating (WC3) is considered a limitation for propane torch and heat lamp methods, as well as muffle furnaces. While this characteristic may assist in the attribution of burn effects to specific drivers, it typically does not produce the heterogeneous combustion residues present after a natural wildfire. This is a key source of uncertainty in the representativeness of burned soil structure and composition as compared to natural combustion (i.e., burning fuel).22 Some studies have addressed this shortcoming by using controlled heating methods coupled with igniting vegetation or litter on the soil surface.59,90,120
Limitations to low-intensity burns (WC4), due to safety and other logistical considerations, is a drawback of litter burns.103 The even distribution of fuels is often inconsequential in substantively heating soils, which may not exceed a low intensity burn.122
Heating from all sides (WC5) is a disadvantage of muffle furnaces. These heating mechanics are categorically different from a natural wildfire, which only heat the side of exposed soil surface.26,52
5.4 Rainfall simulation
Nozzle-based and drip-based rainfall simulators, water drop penetration time (WDPT) tests, and leaching are typically used in conjunction with one of the wildfire simulation techniques mentioned above or they are implemented in situ, over an area already burned by a wildfire.29,78,104,105,124–126 The effects of consecutive rainfall events, with drying periods anywhere from 30 minutes to 1 year, are examined by some rainfall simulation studies, as antecedent moisture content and weathering over time can greatly influence post-wildfire hydrologic response.10,64,79 Runoff collection chambers in these simulations are typically located at the lower end of a sloped plot or sample with a guard to deflect the simulated rainfall, with sampling frequencies ranging from 20 seconds to 20 minutes.29,64,76,104 To facilitate discussion, various rainfall simulation techniques are divided into four categories: fixed nozzle-based simulators, dynamic nozzle-based simulators, drip-style rainfall simulators, and WDPT tests and leaching. The key features which vary between these simulation techniques are range of rainfall intensities, precision of the droplet size and kinetic energy, and spatial distribution. Published wildfire studies which employ these techniques are listed in Table 4 and described in the following subsections.
Table 4 Summary of major rainfall simulation studies included in this review, listed in alphabetical order. The characteristics included are study subject, scale, size and shape of samples, rainfall simulation technique, rainfall intensity, duration and scheduling of the simulated rainfall, and a summary of key results from each study. The provided summaries are reported with respect to unburned conditions unless otherwise stated, e.g. a percent decrease indicates the percent decrease of the response after burning from the control or unburned case in the study. An N/A entry denotes an unreported study characteristic. WR is water repellency, TOC is total organic carbon, and DOC is dissolved organic carbon
Scale |
Size and shape of samples |
Simulation technique |
Rate of rainfall |
Duration and schedule of rainfall and drying |
Hydrologic and water quality results |
Source |
Plot |
1 m2 plots |
Dynamic nozzle-based |
79 mm h−1 |
1 h |
Runoff ratios peaked immediately post-fire (5 and 20% increases for moderate and severe burns, respectively), muted effects from older fires |
Benavides-Solorio and MacDonald, 2001 |
Sediment yield peaked for 1 year old fires (485% and 2392% increases for moderate and severe burns, respectively) |
Plot |
N/A |
Dynamic nozzle-based |
N/A |
N/A |
N/A |
Bertrand, 1961 |
Plot |
3 × 3 m |
Drip-style |
5.1–83.8 mm h−1 |
N/A |
N/A |
Blackburn et al., 1974 |
Plot |
1 × 0.5 m |
Drip-style |
20, 40, and 60 mm h−1 |
Until three temporally discrete runoff samples had been collected |
Total P increased 20% and 49% for moderate and severe burns, respectively |
Blake et al., 2010 |
Suspended sediment increased 323% and 717% for moderate and severe burns, respectively |
Laboratory |
0.2 × 0.4 × 0.15 m |
Fixed nozzle-based |
N/A |
N/A |
Degree hours (°C hrs measured during burning) were positively linearly related (R2 = 0.966) to sum of Na, K, Ca, and Mg cations |
Cancelo-Gonzalez, et al., 2012 |
Laboratory |
0.45 × 0.2 × 15 m |
Fixed nozzle-based |
75 mm h−1 |
Runoff collected in 300 mL aliquots. 3 sessions, 2 h long, 15 days apart |
Leached cations increased significantly (114% and 183% in moderate and severe burns, respectively) |
Cancelo-Gonzalez, et al., 2013 |
Laboratory |
0.2 × 0.4 × 0.15 m |
Fixed nozzle-based |
75 mm h−1 |
Runoff collected in 300 mL aliquots. 300 mm per session, 2 sessions, 2 h long, 15 days apart |
Al and DOC increased 19 and 21%, respectively, for moderate burns and 44 and 679% for severe burns |
Cancelo-Gonzalez, et al., 2015 |
Fe increased only at severe burns (66%) |
Plot |
0.24 m2 |
Fixed nozzle-based |
54.6 mm h−1 |
N/A |
N/A |
Cerda et al., 1997 |
Laboratory |
Potted plants |
Drip-style |
6.5, 8.5, and 9.9 mm h−1 |
1 h |
N/A |
Chevone et al., 1984 |
Plot |
3 × 10.7 m |
Dynamic nozzle-based |
55 and 110 mm h−1 |
45 and 15 min |
Runoff ratio and sediment yield increases (21% and 40%, respectively) were not significantly greater than natural variability |
Emmerich and Cox, 1992 |
Plot |
0.24 m2 (microplot), 8 × 2 m (plot), and <1.5 km2 (catchment) |
Fixed nozzle-based |
50.5 mm h−1 |
45–60 min |
Runoff ratios increased 343, 614, and 1746% for mild, moderate, and severe burns, respectively, for micro-plot scale; increased 14 and 16,471% for mild and severe burns, respectively, for plot scale |
Ferreira et al., 2005 |
Plot |
0.5 m2 |
Drip-style |
203 mm h−1 |
50 min |
Infiltration significantly decreased in bunchgrass, shortgrass, and oak-dominated sites (25–36%) |
Hester et al., 1997 |
Sediment yield significantly increased for all sites (344–225 000%) |
Plot |
N/A |
Fixed nozzle-based |
N/A |
N/A |
N/A |
Holland, 1969 |
Plot |
3.03 × 10.7 m |
Dynamic nozzle-based |
60 mm h−1 |
1 h event, 24 h drying period, 0.5 h event, 0.5 h drying period, 0.5 h event |
Runoff ratios and sediment yields increased 103% and 2240%, respectively |
Johansen, et al., 2001 |
Laboratory |
0.48 × 0.28 × 0.05 m |
Dynamic nozzle-based/WDPT tests and leaching |
33 mm h−1 |
4 h event, drying period in oven, then second 2 h wetting phase |
WR was moderate, peak runoff rates increased up to 5525%, infiltration rates decreased up to 86%, and sedimentation rates increased |
Keesstra et al., 2014 |
Laboratory |
1 × 0.2 × 0.075 m |
Fixed nozzle-based |
31.7 mm h−1 |
40 min |
N/A |
Kibet, et al., 2014 |
Plot |
N/A |
Drip-style |
203 mm h−1 |
30 min |
Infiltration rates and sediment yield decreased 2% and 3%, respectively, though not with statistical significance |
Knight et al., 1983 |
Plot |
1 m2 |
Fixed nozzle-based |
180 mm h−1 |
5 min |
Runoff ratios and sediment yields increased 275% and 775%, respectively |
Marcos et al., 2000 |
Plot |
0.75 × 0.75 m |
Dynamic nozzle-based |
100 mm h−1 |
1 h (three events, each 1 year apart) |
Runoff ratios increased 21% immediately post-fire, lesser values in years 1–5 |
Robichaud et al., 2016 |
Infiltration decreased 30% immediately post-fire, greater values in years 1–5 |
Sediment yield peaked 1 year post-fire (7227% increase), lesser values in years 0, 2, and 5 |
Plot |
4 × 8 m |
Fixed nozzle-based |
76 mm h−1 |
1 h (three events, the second a couple days after first event, then the third 2 h after the second) |
Runoff ratio and sedimentation rates increased ∼4000 and ∼17 000%, respectively, less for higher antecedent moisture contents |
Rosso et al., 2007 |
Plot |
0.83 m2 |
Drip-style |
83.8 mm h−1 |
1 h |
Infiltration rates, for field capacity and dry conditions, respectively, significantly decreased (∼11%) and did not change |
Roundy et al., 1978 |
Sediment yields significantly increased ∼141% for all conditions |
Plot |
10.7 × 3.05 m |
Dynamic nozzle-based |
N/A |
1 h, then 30 min 24 h later, then 30 min immediately |
Runoff ratios and sediment yields increased 432 and 845%, respectively, immediately post-fire, 391 and 690% 1 year post-fire for severe burns; little substantial changes for mild burns |
Simanton et al., 1986 |
Plot |
N/A |
Dynamic nozzle-based |
63.5 and 127 mm h−1 |
N/A |
N/A |
Swanson, 1965 |
Laboratory |
0.2 m diam., 0.1 m height |
Fixed nozzle-based/WDPT tests and leaching |
25 mm h−1 |
1 h |
Hydraulic conductivity significantly decreased for moderate burns (∼62%), increased for severe burns (∼229%) |
Wieting et al., 2017 |
TOC significantly increased for moderate burns (∼60%), decreased for severe burns (∼11%) |
Plot |
1 m2 |
Fixed nozzle-based |
69–202.5 mm h−1 |
N/A |
N/A |
Wilcox et al., 1986 |
Plot |
15 × 20 m |
Fixed nozzle-based |
35, 75, and 150 mm h−1 |
10–49 min |
Peak runoff rates and infiltration had no substantial change |
Wilson, 1999 |
Sediment yield increased 730–353% |
Plot |
0.5 m2 plots |
Dynamic nozzle-based |
75 mm h−1 |
1 h events |
With respect to removal of ash: Runoff ratios and infiltration increased 180% and decreased 32% 1 month post-burn, respectively; no change 12 months post-burn |
Woods and Balfour, 2008 |
5.4.1 Fixed nozzle-based rainfall simulators.
Fixed nozzle-based rainfall simulators have been used in both plot- and laboratory-scale analyses of wildfire impacts on soil and runoff physical and chemical changes.65,90,104,127–129 Most of these simulators use a single, stationary nozzle ∼2 m above the ground which points downward, covering areas of 0.08–1 m2.62,108 One exception is the plot-scale Field Efficient Colorado State Rainfall Simulator which has ten 3 m risers covering 300 m2, each with 1–2 nozzles pointing upwards.65,130 Nozzles are rated to produce a droplet size and kinetic energy similar to natural rainfall at a specified distance beneath the nozzle, as well as an even distribution of rainfall intensity (e.g., the FullJet® and VeeJet© nozzles produced by the Spraying Systems Company).104,127,129 Droplet sizes range from 0.8–4.0 mm and kinetic energies from 0.1–28 J m−2 mm−1, with rainfall intensities ranging from 5–203 mm h−1 and durations from 5 min to 2 h.90,108,127–129,131,132 Results from studies which implemented this type of simulator show a wide range of significant responses in runoff solute concentrations (∼20–700% increases), runoff (∼10–20
000% increases), and sediment (∼300–20
000% increases) after burning, typically measured at 1–3 increments of rainfall intensities.62,65,90,102,126 However, some studies also reported little statistical significance in runoff and infiltration rate response.65
5.4.2 Dynamic nozzle-based rainfall simulators.
Although dynamic nozzle-based rainfall simulators have been used to analyze similar wildfire effects as fixed nozzle simulators, their use is more common in plot-scale analyses.64,66,76,78,101,105,133 These simulators incorporate horizontal rotation29,105 or sweeping motions ∼3 m above the plot or samples,76,78,101,134 covering large areas of up to ∼30 m2.66 The nozzles used are rated to produce natural rainfall kinetic energy and droplet size, same as the fixed nozzle simulators, and produce rainfall intensities ranging from 33–127 mm h−1 for 15 min up to 4 hours.105 Studies with this type of simulator report wide ranges of generally significant responses in runoff (∼5–600% increases), infiltration (∼30–90% decreases), and sediment (∼40–7000% increases) after burning, analyzed at 1–2 increments of rainfall intensities.29,64,66,76,78,82,133 Studies analyzing responses several months or years after burn events tended to not see significance in results.66,76,78,82,133
5.4.3 Drip-style rainfall simulators.
Drip-style rainfall simulators have been used to study the same processes as nozzle-style simulators, typically at a plot-scale.63,124,135 In these simulators, water is channelled to a large number (∼168 to 2209) of fine tubes or needles which periodically release droplets due to gravitational forces,125,135 covering areas from 0.4–9 m2.119,135,136 This technique produces droplet sizes ranging from 2.6–3.3 mm in diameter and rainfall intensities ranging from 20–203 mm h−1 (ref. 63 and 124) for durations of 0.5–1 hour.133,136 Results from studies using this type of simulation technique show generally significant changes in runoff chemical constituents (∼20–49% increases),124 as well as infiltration rates (∼2–11% decreases) and sedimentation rates (∼3–300
000% increases) after burning, typically analyzed at 1–3 rainfall intensity increments.63,119,136
5.4.4 Water drop penetration time tests and leaching.
WDPT tests and leaching do not simulate the mechanics of rainfall, but are important laboratory-scale techniques used to assess wildfire impacts on soil water repellency and changes in chemical composition, respectively.52,61,83,89,123 WDPTs involve placing droplets of water or a water–ethanol mixture on burned soil and recording the duration of time for each drop to infiltrate as a measure of soil water repellency.61,121,137 Leaching involves dissolving water-soluble chemical constituents in burned soil or litter into water, then analyzing the water for chemical composition.26 Studies which used this type of analysis show generally significant changes after burning in water repellency and hydraulic conductivity (∼60–200% increases), as well as leached chemical constituents (∼10–60% increases and decreases).29,89
5.5 Comparison of key rainfall simulation technique characteristics
The rainfall simulation techniques described above all have benefits and limitations which future researchers may take into consideration. Table 5 shows a broad description the pros and cons of each technique.
Table 5 Pros and cons of rainfall simulation techniques covered in the review, as well as the studies referenced and their scales. RP1, RP2, etc. represents rainfall simulation pro 1, rainfall simulation pro 2, etc. Similarly, RC1, RC2, etc. represents rainfall simulation con 1, rainfall simulation con 2, etc
Simulation Technique |
Pros |
Cons |
Scale |
Sources |
Fixed Nozzle-Based Rainfall Simulators |
RP1-simplicity in design |
RC1-small area of coverage |
Laboratory |
Cancelo-Gonzalez, et al., 2012; Cancelo-Gonzalez, et al., 2013; Cancelo-Gonzalez, et al., 2015; Kibet, et al., 2014 |
RP2-transportability and adaptability to steep terrains |
RC2-rain kinetic energies lower than natural rain |
RP3-intensities and droplet sizes similar to natural rainfall |
|
Plot |
Cerda et al., 1997; ferreira et al., 2005; Holland, 1969; Marcos et al., 2000; Rosso et al., 2007; Wilcox et al., 1986; Wilson, 1999 |
Dynamic Nozzle-Based Rainfall Simulators |
RP3-intensities and droplet sizes similar to natural rainfall |
RC3-complexity and expense of design |
Laboratory |
Keesstra et al., 2014; |
RP4-large area of coverage |
RC4-difficulty in transportation |
Plot |
Benavides-Solorio and MacDonald, 2001; Emmerich and Cox, 1992; Johansen, et al., 2001; Robichaud et al., 2016; Simanton et al., 1986; Swanson, 1965; Woods and balfour, 2008; betrand, 1961 |
RP5-variability in droplet dist. similar to natural rainfall |
|
Drip-Style Rainfall Simulators |
RP3-intensities and droplet sizes similar to natural rainfall |
RC3-complexity and expense of design |
Laboratory |
Chevone et al., 1984 |
RP6-increased precision in droplet size |
RC4-difficulty in transportation |
Plot |
Blackburn et al., 1974; blake et al., 2010; Chevone et al., 1984; Hester et al., 1997; Knight et al., 1983; Roundy et al., 1978 |
WDPT Tests or Leaching |
RP1-Simplicity in design |
RC1-small area of coverage |
Laboratory |
Badía-Villas et al., 2014; blank et al., 1994; Cawley et al., 2017; Debano and Krammes, 1966; Hogue and inglett, 2012; Hohner et al., 2019; Robichaud and Hungerford, 2000; Wang et al., 2015; Wieting et al., 2017 |
RP7-direct measurement of water repellency and quality |
RC5-No rainfall impact on soil surface |
|
The following paragraphs discuss common pros of rainfall simulation experiments as listed in Table 5:
Simplicity in design (RP1) is a key benefit of fixed nozzle-based rainfall simulators, WDPT tests, and leaching.90,127,129,130 A stationary nozzle is a relatively simple and inexpensive mechanism to construct or purchase, and is often sufficient in terms of coverage area and semblance to natural rainfall. WDPT tests and leaching typically only require simple laboratory equipment.
Transportability and adaptability to steep terrains (RP2) is a benefit of fixed nozzle-based rainfall simulators. This allows these simulators to be tested on otherwise inaccessible sampling locations and on steep terrains up to 45°.65,127,129
Rainfall intensities and droplet sizes similar to natural rainfall (RP3) is a key benefit of fixed nozzle-based, dynamic nozzle-based, and drip-style rainfall simulators. The ranges of intensities and droplet sizes for these types of simulators make them representative of typical natural precipitation.90,108,127–129
A large area of coverage (RP4) is a benefit unique to dynamic nozzle-based rainfall simulators. Larger plot-scale analyses allow for larger-scale hydrologic processes to occur which cannot be observed on smaller scales,138–140 and minimizes edge effects.67
Spatial and temporal variability in droplet distribution similar to natural rainfall (RP5) is an important attribute of dynamic nozzle-based rainfall simulators. The horizontal rotation and sweeping motion of the nozzles used in these simulators may create spatial and temporal variability which is more representative of natural rainfall than stationary nozzles.125
Increased control and precision of droplet size (RP6) is a benefit of drip-style rainfall simulators. Droplet size can be altered by changing the gage of the tubes and needles used, allowing for control over droplet diameters125 and subsequently the kinetic energy of raindrops produced.
Direct measurement of water repellency and chemical changes (RP7) is a benefit unique to WDPT tests and leaching. This can allow for more precise attribution of burn effects, as opposed to the indirect measurements through runoff generation and chemical composition in other rainfall simulation techniques.26,89,123
The following paragraphs discuss common cons of rainfall simulation experiments as listed in Table 5:
A small area of coverage (RC1) is a limitation of many fixed nozzle-based rainfall simulators, WDPT tests, and leaching.127 The smaller area covered limits analyses to the laboratory- or smaller plot-scale for fixed nozzle-based rainfall simulators, and typically only laboratory-scale samples for WDPT tests and leaching. This often means that these simulation experiments do not capture larger-scale hydrologic processes, such as rill erosion (as discussed in Section 5.1).
Rainfall kinetic energies lower than typical natural rainfall (RC2) is a limitation for fixed nozzle-based rainfall simulators. Rainfall kinetic energies for these simulators tend to be lower than natural rainfall due to simulated droplets often not reaching terminal velocity before impact, as nozzle heights are constrained by equipment.127
Complexity and expense of design (RC3) are limitations for dynamic nozzle-based rainfall simulators and drip-style rainfall simulators, which may result in logistical and financial challenges for studies.
Difficulty in transportation (RC4) is another drawback for dynamic nozzle-based rainfall simulators and drip-style rainfall simulators. This can limit plot-scale study sites to ones with accessible roads, as well as relatively flat terrain.
Lack of rainfall impact on soils (RC5), and therefore lack of droplet kinetic energy, is a limitation of WDPT tests and leaching. These methods do not capture the physical processes of rainfall impact on soil surface and therefore cannot simulate natural constituent transport through runoff.26
6 Recommendations for future study design
Decisions about which experimental methodologies to choose should largely depend on study scope: the hydrologic or chemical responses being analyzed, as well as the temporal and physical scales of the analysis. Researchers should also carefully consider the geographical setting of their study and incorporate specific regional characteristics into their experimental design, such as soil type, vegetation cover, climate regimes, and terrain slopes. In general, we recommend that experimental design elements should be optimized based on their strengths in analyzing important study elements, while weighing time, logistic, and financial constraints.
For example, a study analyzing the effects of different vegetation types on post-wildfire hydrologic processes may want to focus on plot-scale techniques in order to capture larger-scale erosional processes such as rill erosion. In such a scenario, a prescribed burn method could provide the necessary burning scale, burn the intended fuel type (i.e., the different types of vegetation in question), and also partially represent heterogeneous combustion patterns, intact soil structure, and larger-scale vegetation present in a natural wildfire. In this example, a dynamic nozzle-based rainfall simulator may be the best choice of rainfall simulator to allow for a large area of coverage. Alternatively, if the interaction between burn intensity and vegetation characteristics was of primary interest in the above example, then a more appropriate experimental set-up may use a burn simulation technique which allows for greater incremental control over wildfire intensity. Heat lamps, for example, allow for analysis of targeted vegetation burning at specific intensity levels. However, this type of analysis sacrifices some representation of natural burning, due to low spatial variability in combustion. Additionally, as with any laboratory method, the soil sampling process involved in heat lamp simulation techniques introduces edge effects, potentially disturbed soil structure, and can only represent small-scale hydrologic processes and vegetation. A fixed nozzle-based rainfall simulator may be the best choice in this scenario, as only a small area of coverage would be required.
Researchers should also take the results from previous simulation studies into consideration for their study designs. For example, plot-scale studies in this review which implemented prescribed burning tended to most frequently produce results that were not statistically significant relative to control samples. This is likely due to a combination of the spatial heterogeneity of burn intensities in prescribed burning methods and the high variability of plot-scale natural settings typically subjected to prescribed burning. Conversely, laboratory-scale studies which used muffle furnace heating tended to produce results that were highly statistically significant and were able to assess responses at a high number (up to nine) of burn intensity increments. This level of granularity allowed these studies to infer a more fundamental character of the heating effect, for example a monotonic versus negative parabolic response to heating. However, these types of analyses were typically limited to water quality constituents and water repellency, as larger-scale erosional and hydrologic responses were not captured.
Using precedents set by previous simulation studies as a guide, researchers may choose appropriate methods to fit their research goals. To aid discussion, we categorize strengths and weaknesses of simulation experiments into four important factors: (1) representation of natural processes and settings, (2) analysis of multiple post-wildfire water quality and supply drivers simultaneously and independently, (3) observation of responses on different temporal and spatial scales, and (4) mitigation of uncertainty of results. The following paragraphs discuss the tradeoffs that exist between these four key design factors.
6.1 Representation of natural processes
Replicating natural processes improves the representativeness of experimental results—furthering understanding of wildfire effects on soil and runoff characteristics. However, this goal must be weighed against logistical challenges of in situ collection, as well as the increased spatial and temporal variability inherent with natural features—which can create difficulties in attribution. Studies typically address these tradeoffs by only choosing natural or unperturbed features most important to the subject and scale of the study. For example, Hogue and Inglett (2012) examined carbon and nitrogen concentrations in naturally combusted residue, using litter burning with spatially variable combustion to replicate natural wildfire mechanisms.22 Similarly, Benavides-Solorio and MacDonald (2005) and Johansen et al. (2001) analyzed wildfire's role in increased rill erosion, a plot-scale erosional process, by employing plot-scale wildfire and rainfall simulation techniques. Therefore, future studies are recommended to first identify the subject and scale(s) of greatest interest, then focus efforts on replicating natural processes for those elements.
6.2 Incorporation of multiple key drivers
Incorporation of multiple drivers—i.e. burn severity, rainfall intensity, terrain slope, vegetation type, and soil characteristics—at multiple increments and categories, is often sought to gain a more comprehensive understanding of their relative importance and system interactions. However, studies must evaluate the benefits of including these characteristics, since they can limit the number of replicate samples useful for characterizing uncertainty, as well as require large numbers of samples to be collected. Most studies in this review include burn severity and rainfall intensity in their analyses.7,13,38,79,90 Soil structure and composition, terrain slope, climate, vegetation type, and antecedent moisture content are less commonly incorporated, but can have comparable impacts on hydrology and water quality.93 For example, Johansen et al. (2001) incorporated the percentage of bare soil into their analysis of post-wildfire sedimentation, finding that this driver had a strong correlation with sediment generation in addition to burn severity. Factors involved in wildfire prevention, suppression, and mitigation of effects (i.e., mechanical thinning, mulching, and chemical fire-retardants) are also less commonly incorporated, though could provide insights important to fire management efforts. Understanding how each driver impacts hydrology and water quality independently and jointly can also assist in the creation of catchment-scale predictive models, which typically incorporate multiple drivers as model parameters. Benavides-Solorio and MacDonald (2005), for example, used fire severity, percent bare soil, rainfall erosivity, soil water repellency, and soil texture as model parameters to predict post-wildfire sedimentation.
Responses systematically tested over ranges of drivers allows for an understanding of the shape of the response function (e.g., monotonic, parabolic, etc.). Studies typically use only ∼2–3 increments of burn severity and rainfall intensity due to logistical and time constraints.83,90,121,124 However, a higher number of increments proved to be important in Hohner et al. (2019). Here, soil samples were heated in a muffle furnace at five temperature increments ranging from 150–550 °C, finding that water extractable organic carbon and nitrogen had a roughly negative parabolic relationship with temperature, peaking around 250–350 °C.26 We recommend that for a given number of total samples, future studies carefully consider the tradeoffs between the number of increments and the number of replicates.
6.3 Analyses at multiple spatial scales
A limitation of single-scale wildfire and rainfall simulation experiments is the lack of consideration for how properties and processes at one scale may effect water quality and supply responses at larger scales.67 Incorporating multiple physical scales in simulation experiments can provide insight into upscaling operators which can inform catchment-scale predictions. This is particularly important in sedimentation analyses, as geomorphic and erosional processes vary greatly from the laboratory-scale to the catchment-scale.27 Post-fire sedimentation mechanisms such as streambed erosion may be entirely missing, even from plot-scale analyses. However, understanding how mechanisms (e.g., rill erosion, streambed erosion, etc.) are introduced and change at increasing scales can allow for indirect estimation and inference about catchment-scale response.
Simulating multiple physical scales is challenging in a laboratory setting due to fixed equipment size.29,89,90,106,122 Multi-scale analysis is also uncommon in plot-scale studies. However, Ferreira et al. (2005) analyzed sediment and runoff in post-fire plots on a microplot- (<1 m2), plot- (16 m2), and catchment-scale (<1.5 km2), allowing for comparison of results across varying scales. We recommend that future studies consider analyzing post-fire responses at more than one scale, if feasible with their study design and logistical and financial limitations.
6.4 Uncertainty quantification
Uncertainty is most commonly estimated in experimental systems by testing multiple replicate samples, or uniform samples tested under the same conditions.29,80 Quantifying uncertainty can be useful in differentiating the water quality responses of different drivers and can help inform upscaling of results to the catchment scale.141,142 High spatial and temporal variability, albeit representative of natural systems, can introduce additional uncertainty due to difficulties in the attribution of responses to specific drivers. Thus, uncertainty analysis and mitigation efforts must consider both replicate uncertainty, as well as the role of natural variability on attribution uncertainty. In Keesstra et al. (2014), for example, soil samples were homogenized—reducing uncertainty from spatially variable soil structure and vegetation, but decreasing the samples' semblance of a natural environment. We recommend opting for greater numbers of replicate samples to quantify uncertainty, while weighing incorporation of multiple drivers at different increments and scales—which may constrain the feasible number of replicates across each study dimension.
7 Conclusion
This review provides a synthesis of knowledge on wildfire and rainfall simulation techniques used to understand the impacts of wildfire on water quality and supply. Wildfire and rainfall simulation techniques offer solutions to logistical challenges faced in the collection of in situ data, including potentially dangerous post-fire environments, expensive fieldwork expeditions, and lack of control data. However, each technique has unique strengths and weaknesses. Plot-scale analyses are often able to capture a higher spatial variability more representative of natural settings than laboratory-scale analyses, as well as simulate larger-scale hydrologic processes (i.e., erosion). Yet, attribution of responses to specific drivers is often difficult due to high variability of conditions within and across plots.
Laboratory-scale analyses can more precisely control factors in the simulated system, limiting variability and allowing for drivers to be tested at a range of increments. This allows for a more direct attribution of the role of each driver on system responses, independently and jointly across ranges of values. Laboratory-scale experiments also have the benefit of more precise measurements (e.g., using thermocouples to measure temperature profiles) and control over drivers (e.g., muffle furnaces which can be set to exact temperatures for exact durations), which assists in the quantitative analysis of results. The downsides of laboratory-scale experiments are that they are less representative of a natural wildfire system, due to limited spatial variability and scale—meaning only small-scale hydrologic processes can be analyzed.
Common design considerations across these studies include representation of natural processes, incorporation of multiple key drivers, analysis at multiple spatial scales, and uncertainty quantification. As studies are limited by time, resources, and logistical constraints, prioritization of these design considerations in future studies must be made based on scale, scope, and subject matter. Representation of natural processes can increase variability, and therefore increase uncertainty in results. Similarly, increased complexities in the study design, such as incorporation of multiple drivers and spatial scales, can decrease the amount of replicate samples at each condition, thereby limiting a robust quantification of uncertainty. Thus, future studies must weigh which design considerations are important for each aspect of their experiment, focusing resources on realistic representation of the key drivers or constituents of interest.
This review seeks to support the advancement of knowledge in the field of wildfire impacts on water quality and supply. These findings may be informative for future practitioners, as well as for water management efforts in mitigation and adaptation strategies for wildfire impacts. As wildfires continue to represent an increasing threat to water quality and supply, developing advanced techniques to provide further understanding of wildfire effects will become increasingly essential.
Author contributions
Carli Brucker: conceptualization, writing—original draft, writing—review and editing. Ben Livneh: conceptualization, writing—review and editing. J. Toby Minear: conceptualization, writing—review and editing. Fernando L. Rosario-Ortiz: conceptualization, writing—review and editing.
Conflicts of interest
There are no conflicts to declare.
Acknowledgements
This work is supported by funding through the U.S. Environmental Protection Agency, the Cooperative Institute for Research in Environmental Sciences (CIRES) Graduate Student Research Award, part of the NOAA Cooperative Agreement with CIRES, NA17OAR4320101, the 2020 CIRES Innovative Research Program, and the Western Water Assessment, NA21OAR4310309. The authors acknowledge Dr Fangfang Yao, for providing proofreading assistance and Aaron Heldmyer, for assistance in compiling literature.
References
- K. D. Bladon, M. B. Emelko, U. Silins and M. Stone, Wildfire and the Future of Water Supply, Environ. Sci. Technol., 2014, 48, 8936–8943 CrossRef CAS PubMed.
- A. K. Hohner, C. C. Rhoades, P. Wilkerson and F. L. Rosario-Ortiz, Wildfires Alter Forest Watersheds and Threaten Drinking Water Quality, Acc. Chem. Res., 2019, 52(5), 1234–1244 CrossRef CAS PubMed.
- R. Costanza, R. d'Arge, R. de Groot, S. Farber, M. Grasso, B. Hannon, K. Limburg, S. Naeem, R. V. O'Neill, J. Paruelo, R. G. Raskin, P. Sutton and M. van den Belt, The value of the world's ecosystem services and natural capital, Nature, 1997, 387, 253–260 CrossRef CAS.
- T. He, C. M. Belcher, B. B. Lamont and S. L. Lim, A 350-million-year legacy of fire adaptation among conifers, J. Ecol., 2016, 104, 352–363 CrossRef.
- M. Jian, M. Berli and T. A. Ghezzehei, Soil Structural Degradation During Low-Severity Burns, Geophys. Res. Lett., 2018, 45, 5553–5561 CrossRef.
- C. C. Rhoades, A. T. Chow, T. P. Covino, T. S. Fegel, D. N. Pierson and A. E. Rhea, The Legacy of a Severe Wildfire on Stream Nitrogen and Carbon in Headwater Catchments, Ecosystems, 2019, 22, 643–657 CrossRef CAS.
- P. R. Robichaud, Measurement of post-fire hillslope erosion to evaluate and model rehabilitation treatment effectiveness and recovery, Int. J. Wildland Fire, 2005, 14, 475–485 CrossRef.
- W. C. Becker, A. Hohner, F. Rosario-Ortiz and J. DeWolfe, Preparing for Wildfires and Extreme Weather: Plant Design and Operation Recommendations, J.–Am. Water Works Assoc., 2018, 110, 32–40 CrossRef.
-
W. Raseman, J. Kasprzyk, W. Kleiber and R. Balaji, Uncertainty in drinking water supplies: exploring stochastic source water quality generation methods, Int. Congr. Environ. Model. Softw., 2018 Search PubMed.
- S. F. Murphy, J. H. Writer, R. B. McCleskey and D. A. Martin, The role of precipitation type, intensity, and spatial distribution in source water quality after wildfire, Environ. Res. Lett., 2015, 10, 084007 CrossRef.
- J. H. Writer, A. Hohner, J. Oropeza, A. Schmidt, K. M. Cawley and F. L. Rosario-Ortiz, Water treatment implications after the High Park Wildfire, Colorado, J.–Am. Water Works Assoc., 2014, 106, E189–E199 CrossRef.
- J. A. Moody and D. A. Martin, Synthesis of sediment yields after wildland fire in different rainfall regimes in the western United States, Int. J. Wildland Fire, 2009, 18, 96–115 CrossRef.
- H. G. Smith, G. J. Sheridan, P. N. J. Lane, P. Nyman and S. Haydon, Wildfire effects on water quality in forest catchments: A review with implications for water supply, J. Hydrol., 2011, 396, 170–192 CrossRef CAS.
- D. J. Brogan, P. A. Nelson and L. H. MacDonald, Reconstructing extreme post-wildfire floods: a comparison of convective and mesoscale events, Earth Surf. Processes Landforms, 2017, 42, 2505–2522 CrossRef.
-
O. Edenhofer, et al., Climate Change 2014: Mitigation of Climate Change, Cambridge University Press, 2015 Search PubMed.
- J. R. Marlon, P. J. Bartlein, M. K. Walsh, S. P. Harrison, K. J. Brown, M. E. Edwards, P. E. Higuera, M. J. Power, R. S. Anderson, C. Briles, A. Brunelle, C. Carcaillet, M. Daniels, F. S. Hu, M. Lavoie, C. Long, T. Minckley, P. J. H. Richard, A. C. Scott, D. S. Shafer, W. Tinner, C. E. Umbanhowar and C. Whitlock, Wildfire responses to abrupt climate change in North America, Proc. Natl. Acad. Sci. U. S. A., 2009, 106, 2519–2524 CrossRef CAS PubMed.
- A. Sommerfeld, C. Senf, B. Buma, A. W. D'Amato, T. Després, I. Díaz-Hormazábal, S. Fraver, L. E. Frelich, Á. G. Gutiérrez, S. J. Hart, B. J. Harvey, H. S. He, T. Hlásny, A. Holz, T. Kitzberger, D. Kulakowski, D. Lindenmayer, A. S. Mori, J. Müller, J. Paritsis, G. L. W. Perry, S. L. Stephens, M. Svoboda, M. G. Turner, T. T. Veblen and R. Seidl, Patterns and drivers of recent disturbances across the temperate forest biome, Nat. Commun., 2018, 9, 4355 CrossRef PubMed.
- D. V. Spracklen, L. J. Mickley, J. A. Logan, R. C. Hudman, R. Yevich, M. D. Flannigan and A. L. Westerling, Impacts of climate change from 2000 to 2050 on wildfire activity and carbonaceous aerosol concentrations in the western United States, J. Geophys. Res.: Atmos., 2009, 114(D20) DOI:10.1029/2008JD010966.
- B. J. Harvey, Human-caused climate change is now a key driver of forest fire activity in the western United States, Proc. Natl. Acad. Sci. U. S. A., 2016, 113, 11649–11650 CrossRef CAS PubMed.
- Y. Liu, J. Stanturf and S. Goodrick, Trends in global wildfire potential in a changing climate, For. Ecol. Manage., 2010, 259, 685–697 CrossRef.
- X. Yue, L. J. Mickley, J. A. Logan and J. O. Kaplan, Ensemble projections of wildfire activity and carbonaceous aerosol concentrations over the western United States in the mid-21st century, Atmos. Environ., 2013, 77, 767–780 CrossRef CAS PubMed.
- B. A. Hogue and P. W. Inglett, Nutrient release from combustion residues of two contrasting herbaceous vegetation types, Sci. Total Environ., 2012, 431, 9–19 CrossRef CAS PubMed.
- R. Valenca, K. Ramnath, T. M. Dittrich, R. E. Taylor and S. K. Mohanty, Microbial quality of surface water and subsurface soil after wildfire, Water Res., 2020, 175, 115672 CrossRef CAS PubMed.
- J. Cancelo-González, N. Barros, M. E. Rial-Rivas and F. Díaz-Fierros, Assessment of the impact of soil heating on soil cations using the degree-hours method, Span. J. Soil Sci., 2012, 2(3) DOI:10.3232/SJSS.2012.V2.N3.04.
- M. F. Cotrufo, C. M. Boot, S. Kampf, P. A. Nelson, D. J. Brogan, T. Covino, M. L. Haddix, L. H. MacDonald, S. Rathburn, S. Ryan-Bukett, S. Schmeer and E. Hall, Redistribution of pyrogenic carbon from hillslopes to stream corridors following a large montane wildfire, Global Biogeochem. Cycles, 2016, 30, 1348–1355 CrossRef CAS.
- A. K. Hohner, R. S. Summers and F. L. Rosario-Ortiz, Laboratory simulation of postfire effects on conventional drinking water treatment and disinfection byproduct formation, AWWA Water Sci., 2019, 1, e1155 CAS.
- S. K. Kampf, D. J. Brogan, S. Schmeer, L. H. MacDonald and P. A. Nelson, How do geomorphic effects of rainfall vary with storm type and spatial scale in a post-fire landscape?, Geomorphology, 2016, 273, 39–51 CrossRef.
- P. J. Wilkerson and F. L. Rosario-Ortiz, Impact of simulated wildfire on disinfection byproduct formation potential, AWWA Water Sci., 2021, 3, e1217 CAS.
- S. D. Keesstra, J. Maroulis, E. Argaman, A. Voogt and L. Wittenberg, Effects of controlled fire on hydrology and erosion under simulated rainfall, Cuad. Investig., 2014, 40, 269–294 Search PubMed.
- J. Abraham, K. Dowling and S. Florentine, Risk of post-fire metal mobilization into surface water resources: A review, Sci. Total Environ., 2017, 599–600, 1740–1755 CrossRef CAS PubMed.
- L. F. DeBano, The role of fire and soil heating on water repellency in wildland environments: a review, J. Hydrol., 2000, 231–232, 195–206 CrossRef.
- B. A. Ebel and J. A. Moody, Synthesis of soil-hydraulic properties and infiltration
timescales in wildfire-affected soils, Hydrol. Processes, 2017, 31, 324–340 CrossRef.
- F.-N. Robinne, C. Miller, M.-A. Parisien, M. B. Emelko, K. D. Bladon, U. Silins and M. Flannigan, A Global Index for Mapping the Exposure of Water Resources to Wildfire, Forests, 2016, 7, 22 CrossRef.
- R. A. Shakesby and S. H. Doerr, Wildfire as a hydrological and geomorphological agent, Earth-Sci. Rev., 2006, 74, 269–307 CrossRef.
- I. Stavi, Wildfires in Grasslands and Shrublands: A Review of Impacts on Vegetation, Soil, Hydrology, and Geomorphology, Water, 2019, 11, 1042 CrossRef CAS.
- C. J. Williams, F. B. Pierson, P. R. Robichaud and J. Boll, Hydrologic and erosion responses to wildfire along the rangeland–xeric forest continuum in the western US: a review and model of hydrologic vulnerability, Int. J. Wildland Fire, 2014, 23, 155–172 CrossRef.
- J. Wu, J. E. M. Baartman and J. P. Nunes, Comparing the impacts of wildfire and meteorological variability on hydrological and erosion responses in a Mediterranean catchment, Land Degrad. Dev., 2020, 32(2) DOI:10.1002/ldr.3732.
- J. A. Moody, R. A. Shakesby, P. R. Robichaud, S. H. Cannon and D. A. Martin, Current research issues related to post-wildfire runoff and erosion processes, Earth-Sci. Rev., 2013, 122, 10–37 CrossRef.
- J. B. Sankey, J. Kreitler, T. J. Hawbaker, J. L. McVay, M. E. Miller, E. R. Mueller, N. M. Vaillant, S. E. Lowe and T. T. Sankey, Climate, wildfire, and erosion ensemble foretells more sediment in western USA watersheds, Geophys. Res. Lett., 2017, 44, 8884–8892 CrossRef.
- R. A. Shakesby, Post-wildfire soil erosion in the Mediterranean: Review and future research directions, Earth-Sci. Rev., 2011, 105, 71–100 CrossRef.
- J. A. González-Pérez, F. J. González-Vila, G. Almendros and H. Knicker, The effect of fire on soil organic matter—a review, Environ. Int., 2004, 30, 855–870 CrossRef PubMed.
- J. Holden, P. J. Chapman, S. M. Palmer, P. Kay and R. Grayson, The impacts of prescribed moorland burning on water colour and dissolved organic carbon: A critical synthesis, J. Environ. Manage., 2012, 101, 92–103 CrossRef CAS PubMed.
- G. G. Ice, D. G. Neary and P. W. Adams, Effects of Wildfire on Soils and Watershed Processes, J. For., 2004, 102, 16–20 Search PubMed.
- G. A. Martín and F. J. G. Vila, Wildfires, soil carbon balance and resilient organic matter in Mediterranean ecosystems: a review, Span. J. Soil Sci., 2012, 2, 8–33 Search PubMed.
- D. Olefeldt, M. R. Turetsky and C. Blodau, Altered Composition and Microbial versus UV-Mediated Degradation of Dissolved Organic Matter in Boreal Soils Following Wildfire, Ecosystems, 2013, 16, 1396–1412 CrossRef CAS.
- Q. Wang, M. Zhong and S. Wang, A meta-analysis on the response of microbial biomass, dissolved organic matter, respiration, and N mineralization in mineral soil to fire in forest ecosystems, For. Ecol. Manage., 2012, 271, 91–97 CrossRef.
- A. Bento-Gonçalves, A. Vieira, X. Úbeda and D. Martin, Fire and soils: Key concepts and recent advances, Geoderma, 2012, 191, 3–13 CrossRef.
- J. E. Keeley, Fire intensity, fire severity and burn severity: a brief review and suggested usage, Int. J. Wildland Fire, 2009, 18, 116–126 CrossRef.
- L. B. Lentile, Z. A. Holden*, A. M. S. Smith*, M. J. Falkowski, A. T. Hudak, P. Morgan, S. A. Lewis, P. E. Gessler and N. C. Benson, Remote sensing techniques to assess active fire characteristics and post-fire effects, Int. J. Wildland Fire, 2006, 15, 319–345 CrossRef.
-
A. Parson, P. R. Robichaud, S. A. Lewis, C. Napper and J. T. Clark, Field Guide for Mapping Post-fire Soil Burn Severity, Gen Tech Rep RMRS-GTR-243, Fort Collins CO US Dep, Agric. For. Serv. Rocky Mt. Res. Stn. 49 P, 2010, vol. 243, DOI:10.2737/RMRS-GTR-243.
- J. M. Moreno and W. C. Oechel, A simple method for estimating flre intensity after a burn in California chaparral, Acta Oecol., 1989, 13 Search PubMed.
- R. R. Blank, F. Allen and J. A. Young, Extractable Anions in Soils following Wildfire in a Sagebrush-Grass Community, Soil Sci. Soc. Am. J., 1994, 58, 564–570 CrossRef CAS.
-
C. Chandler, P. Cheney, P. Thomas, L. Trabaud and D. Williams, Fire in forestry. Volume 1. Forest fire behavior and effects. Volume 2. Forest fire management and organization, John Wiley & Sons, Inc., New York, 1983 Search PubMed.
- C. R. Stoof, A. De Kort, T. F. A. Bishop, D. Moore, J. G. Wesseling and C. J. Ritsema, How Rock Fragments and Moisture Affect Soil Temperatures during Fire, Soil Sci. Soc. Am. J., 2011, 75, 1133–1143 CrossRef CAS.
- K. Friedrich, R. L. Grossman, J. Huntington, P. D. Blanken, J. Lenters, K. D. Holman, D. Gochis, B. Livneh, J. Prairie, E. Skeie, N. C. Healey, K. Dahm, C. Pearson, T. Finnessey, S. J. Hook and T. Kowalski, Reservoir Evaporation in the Western United States: Current Science, Challenges, and Future Needs, Bull. Am. Meteorol. Soc., 2018, 99, 167–187 CrossRef.
- J.-J. Wang, R. A. Dahlgren and A. T. Chow, Controlled Burning of Forest Detritus Altering Spectroscopic Characteristics and Chlorine Reactivity of Dissolved Organic Matter: Effects of Temperature and Oxygen Availability, Environ. Sci. Technol., 2015, 49, 14019–14027 CrossRef CAS PubMed.
- P. Nyman, G. J. Sheridan, H. G. Smith and P. N. J. Lane, Modeling the effects of surface storage, macropore flow and water repellency on infiltration after wildfire, J. Hydrol., 2014, 513, 301–313 CrossRef.
- C. C. Hardy, Wildland fire hazard and risk: Problems, definitions, and context, For. Ecol. Manage., 2005, 211, 73–82 CrossRef.
- K. C. Kral, R. F. Limb, T. J. Hovick, D. A. McGranahan, A. L. Field and P. L. O'Brien, Simulating Grassland Prescribed Fires Using Experimental Approaches, Fire Ecol., 2015, 11, 34–44 CrossRef.
- X. Wang, Y. Mao, S. Tang, H. Yang and Y. F. Xie, Disinfection byproducts in drinking water and regulatory compliance: A critical review, Front. Environ. Sci. Eng., 2015, 9, 3–15 CrossRef CAS.
- D. Badía-Villas, J. A. González-Pérez, J. M. Aznar, B. Arjona-Gracia and C. Martí-Dalmau, Changes in water repellency, aggregation and organic matter of a mollic horizon burned in laboratory: Soil depth affected by fire, Geoderma, 2014, 213, 400–407 CrossRef.
- J. Cancelo-González, D. M. Prieto, F. Díaz-Fierros and M. T. Barral, Fe and Al leaching in soils under laboratory-controlled burns, Span. J. Soil Sci., 2015, 5(1) DOI:10.3232/SJSS.2015.V5.N1.08.
- J. W. Hester, T. L. Thurow and C. A. Taylor, Hydrologic characteristics of vegetation types as affected by prescribed burning, J. Range Manag., 1997, 50, 199–204 CrossRef.
- M. P. Johansen, T. E. Hakonson and D. D. Breshears, Post-fire runoff and erosion from rainfall simulation: contrasting forests with shrublands and grasslands, Hydrol. Processes, 2001, 15, 2953–2965 CrossRef.
- C. J. Wilson, Effects of logging and fire on runoff and erosion on highly erodible granitic soils in Tasmania, Water Resour. Res., 1999, 35, 3531–3546 CrossRef.
- W. E. Emmerich and J. R. Cox, Hydrologic characteristics immediately after seasonal burning on introduced and native grasslands, J. Range Manag., 1992, 45, 476–479 CrossRef.
- A. J. D. Ferreira, C. O. A. Coelho, C. J. Ritsema, A. K. Boulet and J. J. Keizer, Soil and water degradation processes in burned areas: Lessons learned from a nested approach, Catena, 2008, 74, 273–285 CrossRef.
- M. B. Emelko, U. Silins, K. D. Bladon and M. Stone, Implications of land disturbance on drinking water treatability in a changing climate: Demonstrating the need for “source water supply and protection” strategies, Water Res., 2011, 45, 461–472 CrossRef CAS PubMed.
- J. Fan and L. Morris Gregory, Reservoir Sedimentation. II: Reservoir Desiltation and Long-Term Storage Capacity, J. Hydraul. Eng., 1992, 118, 370–384 CrossRef.
- J. T. Minear and G. M. Kondolf, Estimating reservoir sedimentation rates at large spatial and temporal scales: A case study of California, Water Resour. Res., 2009, 45(12) DOI:10.1029/2007WR006703.
-
J. A. Moody and D. A. Martin, Wildfire Impacts on Reservoir Sedimentation in the Western United States, Proceedings of the Ninth International Symposium on River Sedimentation, 2004, pp. 1095–1102 Search PubMed.
- P. Kemp, D. Sear, A. Collins, P. Naden and I. Jones, The impacts of fine sediment on riverine fish, Hydrol. Processes, 2011, 25, 1800–1821 CrossRef.
- J. O'Laughlin, Conceptual model for comparative ecological risk assessment of wildfire effects on fish, with and without hazardous fuel treatment, For. Ecol. Manage., 2005, 211, 59–72 CrossRef.
- B. Rieman and J. Clayton, Wildfire and Native Fish: Issues of Forest Health and Conservation of Sensitive Species, Fisheries, 1997, 22, 6–15 CrossRef.
- S. S. Larson-Nash, P. R. Robichaud, F. B. Pierson, C. A. Moffet, C. J. Williams, K. E. Spaeth, R. E. Brown and S. A. Lewis, Recovery of small-scale infiltration and erosion after wildfires, J. Hydrol. Hydromech., 2018, 66, 261–270 CrossRef.
- P. R. Robichaud, J. W. Wagenbrenner, F. B. Pierson, K. E. Spaeth, L. E. Ashmun and C. A. Moffet, Infiltration and interrill erosion rates after a wildfire in western Montana, USA, Catena, 2016, 142, 77–88 CrossRef.
- P. N. J. Lane, G. J. Sheridan and P. J. Noske, Changes in sediment loads and discharge from small mountain catchments following wildfire in south eastern Australia, J. Hydrol., 2006, 331, 495–510 CrossRef.
- J. Benavides-Solorio and L. H. MacDonald, Post-fire runoff and erosion from simulated rainfall on small plots, Colorado Front Range, Hydrol. Processes, 2001, 15, 2931–2952 CrossRef.
- B. A. Ebel, J. A. Moody and D. A. Martin, Hydrologic conditions controlling runoff generation immediately after wildfire, Water Resour. Res., 2012, 48(3) DOI:10.1029/2011WR011470.
- J. de D. Benavides-Solorio and L. H. MacDonald, Measurement and prediction of post-fire erosion at the hillslope scale, Colorado Front Range, Int. J. Wildland Fire, 2005, 14, 457–474 CrossRef.
- S. H. Doerr, R. A. Shakesby, W. H. Blake, C. J. Chafer, G. S. Humphreys and P. J. Wallbrink, Effects of differing wildfire severities on soil wettability and implications for hydrological response, J. Hydrol., 2006, 319, 295–311 CrossRef.
- S. W. Woods and V. N. Balfour, The effect of ash on runoff and erosion after a severe forest wildfire, Montana, USA, Int. J. Wildland Fire, 2008, 17, 535–548 CrossRef.
- K. M. Cawley, A. K. Hohner, D. C. Podgorski, W. T. Cooper, J. A. Korak and F. L. Rosario-Ortiz, Molecular and Spectroscopic Characterization of Water Extractable Organic Matter from Thermally Altered Soils Reveal Insight into Disinfection Byproduct Precursors, Environ. Sci. Technol., 2017, 51, 771–779 CrossRef CAS PubMed.
- A. K. Hohner, K. Cawley, J. Oropeza, R. S. Summers and F. L. Rosario-Ortiz, Drinking water treatment response following a Colorado wildfire, Water Res., 2016, 105, 187–198 CrossRef CAS PubMed.
- T. Meixner, Wildfire Impacts on Water Quality, Southwest Hydrol., 2004, 2 Search PubMed.
- G. Hua and D. A. Reckhow, Comparison of disinfection byproduct formation from chlorine and alternative disinfectants, Water Res., 2007, 41, 1667–1678 CrossRef CAS PubMed.
- J.-J. Wang, R. A. Dahlgren, M. S. Erşan, T. Karanfil and A. T. Chow, Wildfire Altering Terrestrial Precursors of Disinfection Byproducts in Forest Detritus, Environ. Sci. Technol., 2015, 49, 5921–5929 CrossRef CAS PubMed.
- E. M. Thurman, Y. Yu, I. Ferrer, K. A. Thorn and F. L. Rosario-Ortiz, Molecular Identification of Water-Extractable Organic Carbon from Thermally Heated Soils: C-13 NMR and Accurate Mass Analyses Find Benzene and Pyridine Carboxylic Acids, Environ. Sci. Technol., 2020, 54, 2994–3001 CrossRef CAS PubMed.
- C. Wieting, B. A. Ebel and K. Singha, Quantifying the effects of wildfire on changes in soil properties by surface burning of soils from the Boulder Creek Critical Zone Observatory, J. Hydrol.: Reg. Stud., 2017, 13, 43–57 Search PubMed.
- J. Cancelo-González, M. E. Rial-Rivas and F. Díaz-Fierros, Effects of fire on cation content in water: a laboratory simulation study, Int. J. Wildland Fire, 2013, 22, 667–680 CrossRef.
- D. J. Conley, H. W. Paerl, R. W. Howarth, D. F. Boesch, S. P. Seitzinger, K. E. Havens, C. Lancelot and G. E. Likens, Controlling Eutrophication: Nitrogen and Phosphorus, Science, 2009, 323, 1014–1015 CrossRef CAS PubMed.
- C. N. Spencer, K. O. Gabel and F. R. Hauer, Wildfire effects on stream food webs and nutrient dynamics in Glacier National Park, USA, For. Ecol. Manage., 2003, 178, 141–153 CrossRef.
- G. Certini, Effects of fire on properties of forest soils: a review, Oecologia, 2005, 143, 1–10 CrossRef PubMed.
- E. Simon, S.-D. Choi and M.-K. Park, Understanding the fate of polycyclic aromatic hydrocarbons at a forest fire site using a conceptual model based on field monitoring, J. Hazard. Mater., 2016, 317, 632–639 CrossRef CAS PubMed.
- U. Choromanska and T. H. DeLuca, Microbial activity and nitrogen mineralization in forest mineral soils following heating: evaluation of post-fire effects, Soil Biol. Biochem., 2002, 34, 263–271 CrossRef CAS.
-
A. Ranalli and M. Stevens, Streamwater Quality Data from the 2002 Hayman, Hinman, and Missionary Ridge Wildfires, Colorado, 2003, p. 2004 Search PubMed.
- S. Chowdhury, M. A. J. Mazumder, O. Al-Attas and T. Husain, Heavy metals in drinking water: Occurrences, implications, and future needs in developing countries, Sci. Total Environ., 2016, 569–570, 476–488 CrossRef CAS PubMed.
- X. Huang, M. Li, H. R. Friedli, Y. Song, D. Chang and L. Zhu, Mercury Emissions from Biomass Burning in China, Environ. Sci. Technol., 2011, 45, 9442–9448 CrossRef CAS PubMed.
- C. Wiedinmyer and H. Friedli, Mercury Emission Estimates from Fires: An Initial Inventory for the United States, Environ. Sci. Technol., 2007, 41, 8092–8098 CrossRef CAS PubMed.
- G. R. Aiken, H. Hsu-Kim and J. N. Ryan, Influence of Dissolved Organic Matter on the Environmental Fate of Metals, Nanoparticles, and Colloids, Environ. Sci. Technol., 2011, 45, 3196–3201 CrossRef CAS PubMed.
- V. Balfour and S. Woods, Causes of Variability in the Effects of Vegetative Ash on Post-Fire Runoff and Erosion, AGU Fall Meet. Abstr., 2008, 11, H11C–H0778 Search PubMed.
- R. Rosso, M. C. Rulli and D. Bocchiola, Transient catchment hydrology after wildfires in a Mediterranean basin: runoff, sediment and woody debris, Hydrol. Earth Syst. Sci. Discuss., 2007, 11, 125–140 CrossRef.
- M. D. Busse, C. J. Shestak, K. R. Hubbert and E. E. Knapp, Soil Physical Properties Regulate Lethal Heating during Burning of Woody Residues, Soil Sci. Soc. Am. J., 2010, 74, 947–955 CrossRef CAS.
- L. C. Kibet, L. S. Saporito, A. L. Allen, E. B. May, P. J. A. Kleinman, F. M. Hashem and R. B. Bryant, A Protocol for Conducting Rainfall Simulation to Study Soil Runoff, J. Visualized Exp., 2014,(86) DOI:10.3791/51664.
- N. P. Swanson, Rotating-Boom Rainfall Simulator, Trans. ASAE, 1965, 8, 0071–0072 Search PubMed.
- C. C. Klopatek, L. F. Debano and J. M. Klopatek, Effects of simulated fire on vesicular-arbuscular mycorrhizae in pinyon-juniper woodland soil, Plant Soil, 1988, 109, 245–249 CrossRef.
- P. M. Fernandes and H. S. Botelho, A review of prescribed burning effectiveness in fire hazard
reduction, Int. J. Wildland Fire, 2003, 12, 117 CrossRef.
- E. Marcos, R. Tarrega and E. Luis-Calabuig, Comparative Analysis of Runo and Sediment Yield with a Rainfall Simulator After Experimental Fire, Arid Soil Res. Rehabil., 2000, 14, 293–307 CrossRef.
- P. R. Robichaud and T. A. Waldrop, A Comparison of Surface Runoff and Sediment Yields from Low- and High-Severity Site Preparation Burns1, J. Am. Water Resour. Assoc., 1994, 30, 27–34 CrossRef.
-
B. R. West, Prescribed burning and wildfire (Fire as a tool in saltcedar management), 1917 Search PubMed.
-
Wildland Fire, https://www.nps.gov/articles/what-is-a-prescribed-fire.htm, (accessed 13 January 2021).
- R. S. Arkle and D. S. Pilliod, Prescribed fires as ecological surrogates for wildfires: A stream and riparian perspective, For. Ecol. Manage., 2010, 259, 893–903 CrossRef.
- C. Santín, S. H. Doerr, C. Preston and R. Bryant, Consumption of residual pyrogenic carbon by wildfire, Int. J. Wildland Fire, 2013, 22, 1072–1077 CrossRef.
- H. H. Chapman, Prescribed Burning Versus Public Forest Fire Services, J. For., 1947, 45, 804–808 Search PubMed.
- M. S. Robinson, T. R. Anthony, S. R. Littau, P. Herckes, X. Nelson, G. S. Poplin and J. L. Burgess, Occupational PAH Exposures during Prescribed Pile Burns, Ann. Occup. Hyg., 2008, 52, 497–508 CAS.
- P. J. Fornwalt and C. C. Rhoades, Rehabilitating Slash Pile Burn Scars in Upper Montane Forests of the Colorado Front Range, Nat. Area J., 2011, 31, 177–182 CrossRef.
- A. K. Shahlaee, W. L. Nutter, E. R. Burroughs and L. A. Morris, Runoff and Sediment Production from Burned Forest Sites in the Georgia Piedmont1, J. Am. Water Resour. Assoc., 1991, 27, 485–493 CrossRef.
- M. C. Carter and C. Darwin Foster, Prescribed burning and productivity in southern pine forests: a review, For. Ecol. Manage., 2004, 191, 93–109 CrossRef.
- B. A. Roundy, W. H. Blackburn and R. E. Eckert, Influence of Prescribed Burning on Infiltration and Sediment Production in the Pinyon-Juniper Woodland, Nevada, J. Range Manage., 1978, 31, 250–253 CrossRef.
- J. Reardon, R. Hungerford and K. Ryan, Factors affecting sustained smouldering in organic soils from pocosin and pond pine woodland wetlands, Int. J. Wildland Fire, 2007, 16, 107 CrossRef.
- P. R. Robichaud and R. D. Hungerford, Water repellency by laboratory burning of four northern Rocky Mountain forest soils, J. Hydrol., 2000, 231–232, 207–219 CrossRef.
- M. D. Busse, K. R. Hubbert, G. O. Fiddler, C. J. Shestak, R. F. Powers, M. D. Busse, K. R. Hubbert, G. O. Fiddler, C. J. Shestak and R. F. Powers, Lethal soil temperatures during burning of masticated forest residues, Int. J. Wildland Fire, 2005, 14, 267–276 CrossRef.
- L. F. Debano and J. S. Krammes, WATER REPELLENT SOILS AND THEIR RELATION TO WILDFIRE TEMPERATURES, Bull. Int. Assoc. Sci. Hydrol., 1966, 11, 14–19 CrossRef.
- W. H. Blake, S. P. Theocharopoulos, N. Skoulikidis, P. Clark, P. Tountas, R. Hartley and Y. Amaxidis, Wildfire impacts on hillslope sediment and phosphorus yields, J. Soils Sediments, 2010, 10, 671–682 CrossRef CAS.
- B. I. Chevone, Y. S. Yang, W. E. Winner, I. Storks-Cotter and S. J. Long, A Rainfall Simulator for Laboratory Use in Acidic Precipitation Studies, J. Air Pollut. Control Assoc., 1984, 34, 355–359 CrossRef CAS.
- A. J. D. Ferreira, C. O. A. Coelho, A. K. Boulet, G. Leighton-Boyce, J. J. Keizer and C. J. Ritsema, Influence of burning intensity on water repellency and hydrological processes at forest and shrub sites in Portugal, Soil Res., 2005, 43, 327–336 CrossRef.
- A. Cerdà, S. Ibáñez and A. Calvo, Design and operation of a small and portable rainfall
simulator for rugged terrain, Soil Technol., 1997, 11, 163–170 CrossRef.
- T. Dunne, W. E. Dietrich and M. J. Brunengo, Simple, portable equipment for erosion experiments under artificial rainfall, J. Agric. Eng. Res., 1980, 25, 161–168 CrossRef.
- B. P. Wilcox, M. K. Wood, J. T. Tromble and T. J. Ward, A hand-portable single nozzle rainfall simulator designed for use on steep slopes, Rangel. Ecol. Manag., 1986, 39, 375–377 CrossRef.
-
M. Holland, Design and testing of rainfall system, CER, 1969, vol. 69/70, 1ss. 21 Search PubMed.
-
FullJet Full Cone Spray Nozzles, https://www.spray.com/products/nozzle-and-spray-system-brands/fulljet-full-cone-spray-nozzles, (accessed 18 September 2020).
-
VeeJet Flat Spray Nozzles, https://www.spray.com/products/nozzle-and-spray-system-brands/veejet-flat-spray-nozzles, (accessed 18 September 2020).
-
J. R. Simanton, G. D. Wingate and M. A. Weltz, Runoff and sediment from a burned sagebrush community, Gen. Tech. Rep. RM-Rocky Mt. for. Range Exp. Stn. US Dep. Agric. for. Serv. USA, 1990, 1ss. 191, pp. 180–185 Search PubMed.
-
A. R. Bertrand, Design and Operation of the Purdue Sprinkling Infiltrometer, 1961, vol. 723 Search PubMed.
- W. H. Blackburn, R. O. Meeuwig and C. M. Skau, A mobile infiltrometer for use on rangeland, Rangel. Ecol. Manag., 1974, 27, 322–323 CrossRef.
- R. W. Knight, W. H. Blackburn and C. J. Scifres, Infiltration Rates and Sediment Production following Herbicide/Fire Brush Treatments, J. Range Manage., 1983, 36, 154–157 CrossRef.
-
L. F. DeBano, Water Repellent Soils: A State-Of-The-Art, U.S. Department of Agriculture, Forest Service, Pacific Southwest Forest and Range Experiment Station, 1981 Search PubMed.
- C. E. Fraser, N. McIntyre, B. M. Jackson and H. S. Wheater, Upscaling hydrological processes and land management change impacts using a metamodeling procedure, Water Resour. Res., 2013, 49, 5817–5833 CrossRef.
- Y. Hamed, J. Albergel, Y. Pépin, J. Asseline, S. Nasri, P. Zante, R. Berndtsson, M. El-Niazy and M. Balah, Comparison between rainfall simulator erosion and observed reservoir sedimentation in an erosion-sensitive semiarid catchment, Catena, 2002, 50, 1–16 CrossRef.
- Y. Le Bissonnais, H. Benkhadra, V. Chaplot, D. Fox, D. King and J. Daroussin, Crusting, runoff and sheet erosion on silty loamy soils at various scales and upscaling from m2 to small catchments, Soil Tillage Res., 1998, 46, 69–80 CrossRef.
- Y. Liu and H. V. Gupta, Uncertainty in hydrologic modeling: Toward an integrated data assimilation framework, Water Resour. Res., 2007, 43(7) DOI:10.1029/2006WR005756.
- T. Wagener and H. V. Gupta, Model identification for hydrological forecasting under uncertainty, Stoch. Environ. Res. Risk Assess., 2005, 19, 378–387 CrossRef.
|
This journal is © The Royal Society of Chemistry 2022 |
Click here to see how this site uses Cookies. View our privacy policy here.