DOI:
10.1039/D1SC00244A
(Edge Article)
Chem. Sci., 2021,
12, 9168-9175
Determination of intermediate state structures in the opening pathway of SARS-CoV-2 spike using cryo-electron microscopy†
Received
13th January 2021
, Accepted 1st June 2021
First published on 3rd June 2021
Abstract
Severe acute respiratory syndrome coronavirus 2 (SARS-CoV-2) is the cause of COVID-19, a highly infectious disease that is severely affecting our society and welfare systems. In order to develop therapeutic interventions against this condition, one promising strategy is to target spike, the trimeric transmembrane glycoprotein that the virus uses to recognise and bind its host cells. Here we use a metainference cryo-electron microscopy approach to determine the opening pathway that brings spike from its inactive (closed) conformation to its active (open) one. The knowledge of the structures of the intermediate states of spike along this opening pathway enables us to identify a cryptic pocket that is not exposed in the open and closed states. These results underline the opportunities offered by the determination of the structures of the transient intermediate states populated during the dynamics of proteins to allow the therapeutic targeting of otherwise invisible cryptic binding sites.
Introduction
The ongoing COVID-19 pandemic has emerged as one of the most challenging global health crises of modern times.1 At the time of writing, the World Health Organization (WHO) reports over 150 million confirmed cases globally, leading to over 3 million deaths. The transmembrane glycoprotein referred to as spike (S) is a key component in the SARS-CoV-2 infectious cycle, with two key functions. The first is to recognise the angiotensin-converting enzyme 2 (ACE2) receptor2 of the host cells, and the second is to fuse the viral membrane with the host cell membrane,3 thereby providing an entry point for the viral genome into the host cell. In order for spike to evade the host immune response, it is extensively coated with glycans that shield it from potential recognition.4,5 Moreover, to avoid immune response during the ACE2 recognition stage, spike exhibits a conformational masking strategy by performing a hinge-like motion in which the receptor binding domain (RBD) recognises ACE2 when it is extended out (open state), while it does not do so when it is buried inwards (closed state).6–9
The immediate response of the scientific community since early 2020 has rapidly increased our understanding of the structural biology of spike. There has been great progress, in particular through cryo-electron microscopy (cryo-EM), in determining the structure of this glycoprotein.6–8 These studies have revealed in high detail conformations of the open and closed states and of the role of the hinge regions in the opening process.6–9 The role of the dynamics of the glycans in protecting spike from antigens, in the recognition of spike to the ACE2 receptor,10,11 as well as in reducing the evolutionary pressure of the shielded amino acids, was revealed by recent studies that described their importance for regulation of the open state population and for the identification of epitope sites for potential antibodies and antivirals.4,5,12
Despite great progress in the discovery of potent antibodies13–15 targeting various epitopes of spike16 and inhibiting ACE2 recognition, identifying small molecules acting in a similar manner through rational design has been challenging. The main reasons are the structural heterogeneity and conformational masking of the RBD, the glycan shielding, and the challenges of testing of therapeutic agents against the spike trimer. For this reason, most COVID-19 small molecule studies have been focused on the discovery of inhibitors at later stages of the viral infection.
In this study we identify a potentially druggable cryptic site present in an intermediate state along the transition pathway of spike from the open to the closed states, during which glycan shielding is reduced. As we show here, this goal can be achieved by using cryo-EM to determine structural ensembles representing the dynamics of the complex. A major challenge towards this objective is to disentangle the effects of macromolecular dynamics from those of systematic and random errors on the measured data. The use of molecular simulations can greatly facilitate these efforts. However, this approach brings additional challenges, as it requires several approximations.17,18 First, the structural ensembles generated through molecular simulations depend on a force field, which is often parametrized to reproduce only specific properties with limited data. Second, the accuracy of the structural ensemble depends on the exhaustiveness of the conformational sampling, especially when high free energy barriers separate relevant conformational states. The recent development of the metainference method has enabled all these challenges to be met.19 Metainference can accurately model a thermodynamic ensemble by optimally combining prior information of the system such as physico-chemical knowledge (e.g. a force field), with potentially noisy and heterogeneous experimental data. Metainference has already been used successfully in a series of complex biological problems in combination with cryo-EM, nuclear magnetic resonance spectroscopy, and other techniques.20–22
Results
Simultaneous determination of the structure and dynamics of spike
Because of their dynamics, it is challenging to determine the regions mediating ACE2 recognition, including the RBD domain, as well as the glycans (Fig. 1A and B), although progress has been made in this direction.9–12,16 In this study, we determined a structural ensemble of the complete spike head representing the conformational space populated by this glycoprotein (Fig. 1B). In this structural ensemble, glycans are highly dynamic and assume many different conformations, thereby explaining the correspondingly low density in the electron density maps (EMD-21375). The RBD is highly dynamic, with the up RBD protomer (RBD1) showing large-scale conformational rearrangements that complicate a description in terms of a single state where the RBD points up. Such a structural heterogeneous ensemble of the RBD1 can explain a recently reported EM map (EMD-11498) where one RBD protomer shows low electron density,23 which was attributed to disordered states distinct from the open and closed states.
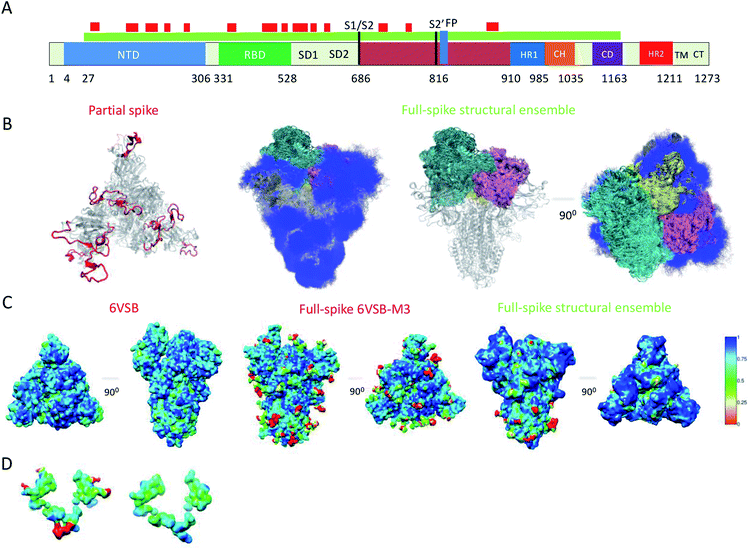 |
| Fig. 1 Simultaneous determination of the structure and dynamics of SARS-CoV-2 spike from a cryo-EM electron density map of the open state. (A) Cartoon representation of the primary sequence and respective functional domains of spike. Regions that were not previously determined (PDB: 6VSB) are shown in red. (B) Open state structural ensemble determined in this study; the different RBD protomers RBD1, RBD2 and RBD3 are coloured in cyan, purple and yellow, respectively. The conformationally heterogeneous regions shown in red in panel A are also represented. (C) Local correlation of the experimental electron density map (EMD-21375) with a map generated using the model 6VSB (left), a map derived from the full-spike 6VSB-M3 model for the open state (middle), and with a structural ensemble average map (right). (D) Local correlation of the experimental electron density map with the average electron density map corresponding to the structural ensemble for the region of glycans N717, N1098 and N1094, showing that the correlation is improved if the glycans are absent. | |
To evaluate our results, we compared the correlation of the experimental electron density map with an electron density map generated as an ensemble average over the structural ensemble, as well as with an electron density map corresponding to a single structure of the open state (PDB: 6VSB) (Fig. 1C). These comparisons revealed correlations of 0.87 and 0.85, respectively. Moreover, we derived an electron density map from a single structure of the full atomistic model of spike (6VSB-M3), including glycans and all RBD and N-terminal domain (NTD) regions, used in this study as initial conditions in our simulations (see Methods) and correlate it with the experimental electron density map.
This map generated from the 6VSB-M3 model exhibits a correlation of 0.82 to the experimental electron density map. We attribute this mismatch in correlation (Fig. 1C) to the static representation of the glycans, RBD and NTD regions in the experimental density. This analysis can also point to poor glycan type selection in the atomistic model. In particular, the structural ensemble of glycans attached to N717, N1098 and N1094 does not correlate well with the experimental electron density map (Fig. 1D). This result points to either the absence of a glycan in these positions, or a differing glycan type in the experimental conditions. The latter hypothesis corroborates a recent study supporting the glycosylation of these residues with more complex mannose glycans.5
Free energy landscape of spike
To obtain more insights into the dynamics of spike, we represented the dependence of its free energy on the deviation from the reference open and closed states (Fig. 2). The dynamics responsible for the low electron densities in the RBD region of spike found in the experimental electron density map of the open state is now rationalised by the coexistence of a plethora of states (Fig. 2). The structural ensemble contains the open state O and other open states O1 and O2, as well as nearby closed states C1, C2, C3, C4 and C5.
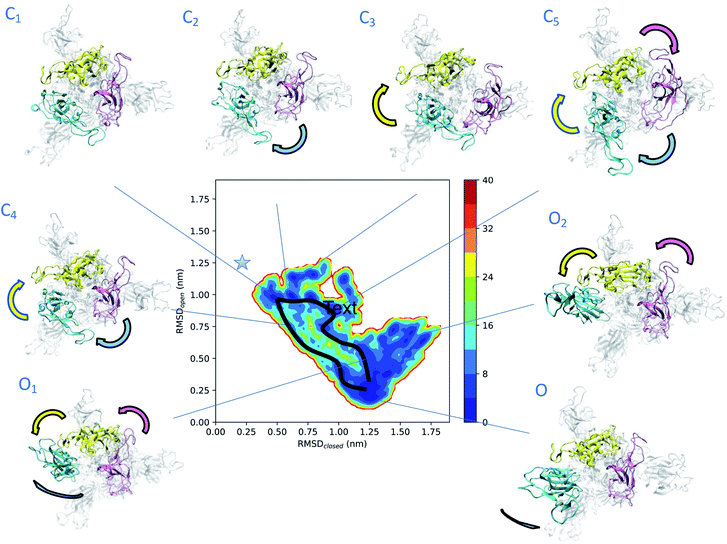 |
| Fig. 2 Determination of intermediate states in the opening transition pathways of spike. The structural ensemble of spike in the open state (Fig. 1) is represented here as a free energy landscape as a function of the structural distances (in terms of RMSD) from previously reported closed state (PDB: 6VXX) and open state (PDB: 6VSB) structures. Cyan, purple and yellow arrows indicate the typical motions of RBD1, RBD2 and RBD3, respectively. Two distinct opening pathways are drawn by black lines, while with asterisk the position of the closed state (PDB: 6VXX). The energy is given in kBT units. | |
The convergence of the simulations (Fig. S1A†) confirms the presence of distinct free energy minima associated with the intermediate states. The open state O, which is within 3 Å of PDB: 6VSB (Fig. S1B†), shows the highest population and hence represents the ground state, followed by the lower-populated excited states C1, C2, C3, C4, C5, O1 and O2. The intermediate state C1 is only 4 Å away from the closed state (Fig. 2 and S1C–E†) and reveals the presence of states that are far removed from the open state. The free energy landscape allows the identification of two possible distinct transition pathways between the open and closed states. A more frequent, low energy pathway traversing states C1, C2, C5, O1 and O2, and a less frequent, high energy pathway traversing states C1, C4, and ending up in O. The low energy pathway commences from state C1, in which the three RBDs are found in close proximity. RBD1 first loses its contacts with RBD2 and RBD3, and then performs a twisting motion around the longitudinal axis (z-axis) of spike. In state C5 both RBD2 and RBD3 also lose their contacts, before rejoining each other, while RBD1 extends up and turns outwards in state O1. Finally, in state O the up RBD1 repositions itself by turning inwards to the ground-state up conformation. The high energy pathway again starts from state C1 and commences with a simultaneous loss of contact of the RBD2 and RBD3 (purple and yellow) domains in state C4, by twisting around the longitudinal axis of spike, and then progresses towards the open state.
Identification of a cryptic site in an intermediate state along the opening transition
From a therapeutic point of view, targeting an intermediate state of spike with a small molecule could alleviate issues caused by the glycan shield protection in the open and closed states and the conformational masking of the closed state in which the RBD is buried. We leverage the structural ensemble to identify potential cryptic pocket sites that do not exist in the open or closed states. In this way (Fig. S2†), we identify a binding site present in intermediate states C1, C2, C3 and C4, near the closed state. This cryptic pocket binding site showed populations of 10%, 25%, 8% and 75% in states C1, C2, C3 and C4, respectively. The cryptic pocket site forms between the NTD and the RBD domains in down position and is situated at the vicinity of the RBD recognition site of ACE2 (residues 448 to 501). The cryptic pocket site involves polar and apolar interacting residues (Fig. 3A–D), in particular, we find interdomain interactions between NTD residues F168, Y200, P230 and RBD residues R357, Y396 and E516. Recent hydrogen/deuterium mass exchange spectrometry experiments showed that RBD and NTD residues 351–375 have high deuterium exchange propensity, suggesting their role in the hinge motion.23 Such results increase our confidence on the functional role of the RBD–NTD interface along the hinge motion opening transition.
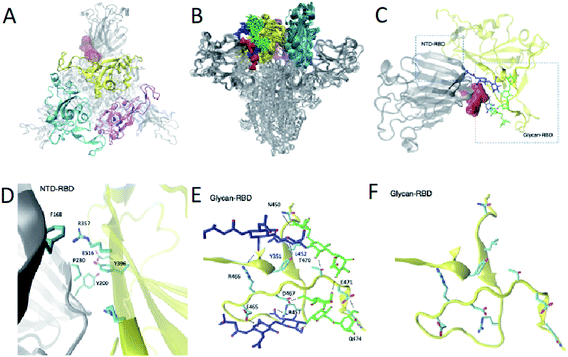 |
| Fig. 3 Identification of a cryptic pocket in an intermediate state of spike populated in the opening transition. (A) Position (shown in pink) of the cryptic pocket between the NTD and the RBD, which is present in the intermediate state C2. (B) Structural ensemble of the glycans in the NTD and RBD in the intermediate state C2, illustrating their protective role of the cryptic pocket. (C) Position of the cryptic pocket in the intermediate state C2 with the glycans highlighted. (D) Interatomic interactions between the NTD and the RBD. (E) Interatomic interactions between the glycans and the RBD. (F) Representation of RBD residues interacting with the glycans. | |
To further verify the presence of the nearby closed state intermediate states discussed earlier as well as the presence of the cryptic pocket, we determined the structural ensemble of the closing transition by performing an EMMI simulation starting from the closed state (6VXX) and using the EM data of the closed state as restraints (EMD-21452)7 (Fig. S3†). The free energy landscapes corresponding to the open (Fig. 2) and closed (Fig. S3†) states exhibit similarities and differences. The differences arise as a consequence of the fact that the electron density maps of the open and closed states are obtained by a class averaging procedure that separates the experimental information corresponding to the two states. Therefore, according to this procedure, the open and closed electron density maps report on different regions of the conformational space. Despite these differences, we found that some information about the closed state is included in the electron density map of the open state. This result is reported in Fig. 2, where we show 5 intermediate states (C1, C2, C3, C4 and C5) in the free energy landscape calculated from the electron density map of the closed state. The structure of the fully closed state, however, does not appear in this free energy landscape because the experimental information about it is excluded by the class averaging procedure. Given these differences and similarities, an important result that we report is that the same 5 intermediate states (C1, C2, C3, C4 and C5) in Fig. 2 appear also in the free energy landscape obtained using the electron density map of the closed state (Fig. S3†). As expected, however, the populations of the 5 intermediate states are different in the open and the closed free energy landscapes, because these states have different weights in the two class averages.
To verify that the presence of the cryptic pocket identified for the opening transition, we performed the pocket analysis for 100 structures of the metastable state C1 (Fig. S3†) identified in the structural ensemble of the closing transition. We were thus able to find the same binding site as in state C1 of the opening transition structural ensemble (Fig. 3 and S4†). As in the opening transition the cryptic pocket sits between glycans N165 and N234.
Glycans N165 and N234 participate in the formation of the cryptic pocket
We found that glycans N165 and N234, which were recently identified as regulators of the recognition of spike to the ACE2 receptor,10,11 participate in the formation of the cryptic pocket. They do so by interacting with the RBD (Fig. 1E and D), thus forming contacts with mostly charged and polar residues. Specifically, N234 interacts with E465, D467, R457, Y351, T470, Q474, E471 and N165 interacts with R466, Y351, L452, T470. Furthermore, glycans N165 and N234 interact with each other, corroborating the notion of glycans forming an extensive interaction network that leads to higher order structuring of the glycan shield.5
In order to assess the role of these glycans along the opening pathway, we projected the number of total contacts each glycan makes with the RBD cryptic pocket related residues described earlier (Fig. S5A†). We found that N165 forms more than 20 contacts with the cryptic pocket related residues of the RBD in states C1, C2 and C3. As the spike RBD moves towards the open state, N165 loses its interactions with the RBD, which partially regains them in state O1 with 15 contacts. Finally, in state O, N165 shows very few contacts with the RBD. The dynamics of N165 along the hinge motion, stabilizing states C1, C2, C3 and state O1 to a lesser degree, provides evidence of its regulatory activity for the hinge motion and thereby shines light on its role regulating recognition of the ACE2 receptor.10,11 In turn, glycan N234 exhibits constant binding with the RBD along the hinge motion as shown in Fig. S5B† and suggests a global stabilising role. In fact, such an important role along the hinge motion can potentially explain the reduction of spike binding affinity to ACE2 upon mutation of N234 with alanine.
From a different point of view, namely that of the protein interacting residues with glycans N234 and N165 mentioned earlier (residues E465, D467, R457, Y351, T470, Q474, E471, R466, Y351, L452, T470) have been shown to exert a high deuterium exchange.23 This suggests an ensemble of structures with a different environment and solvent accessibility for these residues. Clearly the contact maps in Fig. S4† illustrate such a heterogeneity of interactions of the glycans with these residues in the different state along the opening transition.
Taken together, these results show that the formation of a cryptic pocket creates a vulnerability of the spike protein along the transition from the open to closed state, and simultaneously points towards a new type of cavity formation mechanism that encompasses not only the protein but also gating glycans.
Conclusions
We have determined the intermediate states along the opening pathways of the SARS-CoV2 spike glycoprotein using a metainference cryo-EM approach. The knowledge of these intermediate states has enabled us to characterise a cryptic binding site that we expect could be targeted therapeutically. These results demonstrate the opportunities offered by the combination of cryo-EM with molecular simulations and Bayesian inference to describe simultaneously the structure and dynamics of large macromolecular systems.
Materials and methods
EMMI theory
The metainference approach19 was recently extended to include cryo-EM data with the development of the cryo-EM MetaInference (EMMI) method.20 In EMMI, the cryo-EM data voxel map at a position x is represented as a Gaussian Mixture Model (GMM) ϕD(x) with ND components (data GMM) |  | (1) |
where ωD,i is the weight of the i-th component of the data GMM and G is a normalized Gaussian function centred in xD,i with a corresponding covariance matrix ΣD,i.20 EMMI quantifies the deviations between the GMMs generated from experimental data and molecular dynamics by using the following overlap function:20 | oMD,i = ∫dxϕM(x)ϕD,i(x) | (2) |
where ϕM(x) corresponds to the model GMM, in which each heavy atom of the system by one Gaussian function.20 Since EMMI deals with the heterogeneity of the system by simulating many replicas of it, the overlap between the model GMM and the data GMM is estimated over the ensemble of replicas to yield an average overlap ōMD,i. Finally, since cryo-EM maps usually contain large amounts of particle density data, EMMI samples the error in the data a posteriori, thus simplifying the total energy function to:20 |  | (3) |
where the first term corresponds to the force field and the second term provides a penalty depending on the agreement of the data with the generated models.
Simulation setup
As the initial conformation for the simulations, we started from the recently reported structure of the open state of the head of spike,24 which was provided in CHARMMGUI as fully glycosylated spike protein head-only models (residue 1–1146). We then stripped it from glycans and only considered residues 27–1146. This structure was built based on the open state structure determined using cryo-EM6 (PDB: 6VSB), and models the missing spike by performing homology modelling for the missing RBD segment using Galaxy,25 and using as a template a crystal structure of RBD binding to ACE2 (PDB: 6M0J).26 Missing structure segments are highlighted in the spike sequence in Fig. 1A. Since spike carries a glycan coat, in which each asparagine glycosylation site can host a variety of glycan types, ranging from oligomannose to higher order glycans,5 we took a conservative stance and used doGlycans27 to build an M3 glycan at each of the 16/22 solvent accessible N-linked glycosylation sites of the spike structure,7 namely at residues (N61, N122, N165, N234, N282, N331, N343, N603, N616, N657, N709, N717, N801, N1074, N1098, N1134). The M3 glycan sequence is shared among all types of glycans. We term this open state model full-spike 6VSB-M3.
Molecular dynamics equilibration
We set up a simulation box comprising 714
280 atoms adding ions to the experimental concentration of 150 mM NaCl.6 The protein, water and glycan force fields employed in this study are AMBER99SB-ILDN,28 TIP3P29 and GLYCAM06h.30 The system was subsequently energy minimized, equilibrated using first NPT and then NVT molecular dynamics simulations. To constrain bond lengths, we used the LINCS algorithm.31 The Lennard-Jones interactions were treated with a 1 nm cut-off, while the electrostatic interactions were treated with the Particle Mesh Ewald method using a Fourier spacing of 1.2 nm and a 1 nm cut-off for the short-range electrostatic part. Pair lists were updated every 10 fs, using a cut-off of 1 nm and a timestep of 2 fs.28 Integration of Newton's equations of motion was performed using the leap-frog algorithm,32 the velocity-rescaling thermostat33 with a coupling time constant of 0.2 ps, and the Parrinello–Rahman barostat34 for equilibration utilizing a coupling time constant of 1.0 ps during the NPT simulations. In the NPT equilibration, the positions of the Cα atoms were restrained with a constant force of 200 kJ mol−1 nm−2, the temperature was set to 310 K, the pressure to 1 atm and the simulation duration to 500 ps. In the NVT equilibration, we lifted the position restraints, simulated for 2 ns and set the temperature to 310 K without pressure coupling.
EMMI setup
Opening transition.
The experimental voxel map data (EMD-21375) were expressed as a data GMM containing 16
898 Gaussians in total, exhibiting a correlation of 0.97 with the original experimental voxel map. We extracted 32 configurations from the NVT equilibration and initiated two individual EMMI simulations, each consisting of 32 replicas with an aggregate runtime of 1 μs using PLUMED.2.6.0-dev.35 EMMI simulations were performed in the NVT ensemble using the same molecular dynamics parameters as in the equilibration step. Configurations were saved every 5 ps for post-processing. The cryo-EM restraint is calculated every 2 steps, using neighbour lists to compute the overlaps between the model and data GMMs, with a cut-off equal to 0.01 and an update frequency of 100 steps.
Closing transition.
The experimental voxel map data (EMD-21452) are expressed as a data GMM containing 12
100 Gaussians in total, exhibiting a correlation of 0.88 with the original experimental voxel map. We extracted 32 configurations from the NVT equilibration and initiated two individual EMMI simulations, each consisting of 32 replicas with an aggregate runtime of 1 μs using PLUMED.2.6.0-dev.35 EMMI simulations were performed in the NVT ensemble using the same molecular dynamics parameters as in the equilibration step. Configurations were saved every 5 ps for post-processing. The cryo-EM restraint is calculated every 2 steps, using neighbour lists to compute the overlaps between the model and data GMMs, with a cut-off equal to 0.01 and an update frequency of 100 steps.
Free energy surfaces
We removed the first 2 ns in each EMMI simulation, divided the remaining trajectory into two blocks of equal length, and projected the free energy surface along two collective variables, i.e. the root-mean-square deviations (RMSDs) from the RBD open state and closed state structures respectively (Cα atoms of residues 331–528). In the case of the open state, we use as reference the model based on PDB: 6VSB, which takes into account the missing RBD and NTD regions, whereas for the case of the closed state, we considered as reference a model based on PDB: 6VXX, which takes into account the missing RBD and NTD regions, both provided in ref. 24. Using this projection, we identified on pathway free energy minima lower than 4kBT, which we classified as metastable states, and traced their populations in each of the two-simulation blocks. These block averages and standard deviations represent a measure of convergence of our simulations (Fig. S1†). For molecular visualizations we used VMD36 and Chimera.37
Pocket analysis
The identification of potential druggable pockets was performed using the Fpocket software.38 Fpocket selects druggable sites using a scoring function that takes into account the volume, hydrophobicity and polarity of a pocket. We define druggable sites as those with an Fpocket druggability score >0.45. In order to inspect the location of these pockets in terms of residues in their direct vicinity, we considered that each residue comprising the pocket forms a pair with every other residue in the pocket and bin these pairs in a 2D histogram, referred to as a pocket-residue map (Fig. S2†). Such a projection is a dimensionality reduction used to more effectively rationalise the pocket location and should not be confused with a contact map. For each metastable state, the pocket analysis is performed for 100 structures extracted from the bottom of the corresponding free energy minimum. We provide the statistics of druggable pockets for each state in Table S1.†
Data availability
EMMI input and analysis files can be found in PLUMED-NEST (plumID:20.033) https://www.plumed-nest.org/eggs/20/033/GROMACS files and raw trajectory data for the opening transition can be found in the github repository https://github.com/vendruscolo-lab/Spike-OpeningTransitionEnsemble and in the zenobo repository https://zenodo.org/record/4289126#.YL3-fy0RrJF.
Author contributions
Z. F. B. and M. V. designed research, Z. F. B. and T. L. performed research, and Z. F. B., T. L., and M. V. wrote the paper.
Conflicts of interest
There are no conflicts to declare.
Acknowledgements
The authors greatly acknowledge Tristan Croll for useful discussions. The authors would like to acknowledge ARCHER supercomputer system for the computer-time. Z. F. B. would like to acknowledge the Federation of European Biochemical Societies (FEBS) for financial support (LTF).
References
- K. G. Andersen, A. Rambaut, W. I. Lipkin, E. C. Holmes and R. F. Garry, The proximal origin of SARS-CoV-2, Nat. Med., 2020, 26, 450–452 Search PubMed.
- M. Hoffmann,
et al., SARS-CoV-2 cell entry depends on ACE2 and TMPRSS2 and is blocked by a clinically proven protease inhibitor, Cell, 2020, 181, 271–280 Search PubMed.
- F. Li, Structure, function, and evolution of coronavirus spike proteins, Annu. Rev. Virol., 2016, 3, 237–261 Search PubMed.
- Y. Watanabe,
et al., Vulnerabilities in coronavirus glycan shields despite extensive glycosylation, Nat. Commun., 2020, 11, 1–10 Search PubMed.
- Y. Watanabe, J. D. Allen, D. Wrapp, J. S. McLellan and M. Crispin, Site-specific glycan analysis of the SARS-CoV-2 spike, Science, 2020, 369, 330–333 Search PubMed.
- D. Wrapp,
et al., Cryo-em structure of the 2019-nCoV spike in the prefusion conformation, Science, 2020, 367, 1260–1263 Search PubMed.
- A. C. Walls,
et al., Structure, function, and antigenicity of the SARS-CoV-2 spike glycoprotein, Cell, 2020, 181, 281–292 Search PubMed.
- R. Yan,
et al., Structural basis for the recognition of SARS-CoV-2 by full-length human ACE2, Science, 2020, 367, 1444–1448 Search PubMed.
- B. Turoňová,
et al., In situ structural analysis of SARS-CoV-2 spike reveals flexibility mediated by three hinges, Science, 2020, 370, 203–208 Search PubMed.
- R. Henderson,
et al., Glycans on the SARS-CoV-2 spike control the receptor binding domain conformation, bioRxiv, 2020 DOI:10.1101/2020.1106.1126.173765.
- L. Casalino,
et al., Beyond shielding: The roles of glycans in the SARS-CoV-2 spike protein, ACS Cent. Sci., 2020, 6, 1722–1734 Search PubMed.
- M. I. Zimmerman,
et al., SARS-CoV-2 simulations go exascale to predict dramatic spike opening and cryptic pockets across the proteome, Nat. Chem., 2021, 1–9 Search PubMed.
- D. Zhou,
et al., Structural basis for the neutralization of SARS-CoV-2 by an antibody from a convalescent patient, Nat. Struct. Mol. Biol., 2020, 27, 950–958 Search PubMed.
- M. Yuan,
et al., A highly conserved cryptic epitope in the receptor binding domains of SARS-CoV-2 and SARS-CoV, Science, 2020, 368, 630–633 Search PubMed.
- J. Huo,
et al., Neutralizing nanobodies bind SARS-CoV-2 spike RBD and block interaction with ACE2, Nat. Struct. Mol. Biol., 2020, 27, 846–854 Search PubMed.
- M. Sikora,
et al., Map of SARS-CoV-2 spike epitopes not shielded by glycans, PLoS Comput. Biol., 2020, 17, e1008790 Search PubMed.
- M. Bonomi, G. T. Heller, C. Camilloni and M. Vendruscolo, Principles of protein structural ensemble determination, Curr. Opin. Struct. Biol., 2017, 42, 106–116 Search PubMed.
- M. Bonomi and M. Vendruscolo, Determination of protein structural ensembles using cryo-electron microscopy, Curr. Opin. Struct. Biol., 2019, 56, 37–45 Search PubMed.
- M. Bonomi, C. Camilloni, A. Cavalli and M. Vendruscolo, Metainference: A bayesian inference method for heterogeneous systems, Sci. Adv., 2016, 2, e1501177 Search PubMed.
- M. Bonomi, R. Pellarin and M. Vendruscolo, Simultaneous determination of protein structure and dynamics using cryo-electron microscopy, Biophys. J., 2018, 114, 1604–1613 Search PubMed.
- L. Eshun-Wilson,
et al., Effects of α-tubulin acetylation on microtubule structure and stability, Proc. Natl. Acad. Sci. U. S. A., 2019, 116, 10366–10371 Search PubMed.
- Z. F. Brotzakis, M. Vendruscolo and P. Bolhuis, A method of incorporating rate constants as kinetic constraints in molecular dynamics simulations, Proc. Natl. Acad. Sci. U. S. A., 2021, 118, e2012423118 Search PubMed.
- Z. Ke,
et al., Structures and distributions of SARS-CoV-2 spike proteins on intact virions, Nature, 2020, 588, 498–502 Search PubMed.
- W. Hyeonuk,
et al., Developing a fully-glycosylated full-length SARS-CoV-2 spike protein model in a viral membrane, J. Phys. Chem. B, 2020, 124(33), 7128–7137 Search PubMed.
- J. Ko, H. Park and C. Seok, Galaxytbm: Template-based modeling by building a reliable core and refining unreliable local regions, BMC Bioinf., 2012, 13, 198 Search PubMed.
- J. Lan,
et al., Structure of the SARS-CoV-2 spike receptor-binding domain bound to the ACE2 receptor, Nature, 2020, 581, 215–220 Search PubMed.
- R. Danne,
et al., Doglycans–tools for preparing carbohydrate structures for atomistic simulations of glycoproteins, glycolipids, and carbohydrate polymers for gromacs, J. Chem. Inf. Model., 2017, 57, 2401–2406 Search PubMed.
- K. Lindorff-Larsen,
et al., Improved side-chain torsion potentials for the Amber ff99sb protein force field, Proteins, 2010, 78, 1950–1958 Search PubMed.
- W. L. Jorgensen, J. Chandrasekhar, J. D. Madura, R. W. Impey and M. L. Klein, Comparison of simple potential functions for simulating liquid water, J. Chem. Phys., 1983, 79, 926–935 Search PubMed.
- K. N. Kirschner,
et al., Glycam06: A generalizable biomolecular force field. Carbohydrates, J. Comput. Chem., 2008, 29, 622–655 Search PubMed.
- B. Hess, H. Bekker, H. J. Berendsen and J. G. Fraaije, LINCS: A linear constraint solver for molecular simulations, J. Comput. Chem., 1997, 18, 1463–1472 Search PubMed.
-
D. Frenkel and B. Smit, Understanding molecular simulation: From algorithms to applications, Elsevier, 2001, vol. 1 Search PubMed.
- G. Bussi, D. Donadio and M. Parrinello, Canonical sampling through velocity rescaling, J. Chem. Phys., 2007, 126, 014101 Search PubMed.
- M. Parrinello and A. Rahman, Polymorphic transitions in single crystals: A new molecular dynamics method, J. Appl. Phys., 1981, 52, 7182–7190 Search PubMed.
- G. A. Tribello, M. Bonomi, D. Branduardi, C. Camilloni and G. Bussi, Plumed 2: New feathers for an old bird, Comput. Phys. Commun., 2014, 185, 604–613 Search PubMed.
- W. Humphrey, A. Dalke and K. Schulten, VMD: Visual molecular dynamics, J. Mol. Graphics, 1996, 14, 33–38 Search PubMed.
- E. F. Pettersen,
et al., Ucsf chimera—a visualization system for exploratory research and analysis, J. Comput. Chem., 2004, 25, 1605–1612 Search PubMed.
- V. Le Guilloux, P. Schmidtke and P. Tuffery, Fpocket: An open source platform for ligand pocket detection, BMC Bioinf., 2009, 10, 1–11 Search PubMed.
Footnote |
† Electronic supplementary information (ESI) available. See DOI: 10.1039/d1sc00244a |
|
This journal is © The Royal Society of Chemistry 2021 |