DOI:
10.1039/D1AN00059D
(Communication)
Analyst, 2021,
146, 3440-3448
Diffuse reflectance infrared Fourier transform spectroscopy for a qualitative evaluation of plant leaf pigment extraction
Received
11th January 2021
, Accepted 1st April 2021
First published on 6th May 2021
Abstract
The extraction and quantification of leaf pigments are easy, fast, and cheap procedures; on the other hand, diffuse reflectance infrared Fourier transform (DRIFT) spectroscopy associated with chemometrics tools could offer new insights into leaf biochemical composition. We aimed to boost the classic leaf pigment quantification, adding leaf biochemical information derived from DRIFT spectroscopy + principal component analysis, using the same leaf pigment extract produced by the classical quantification method. We performed a dose–response experiment using P as the limiting nutrient, and maize (Zea mays L.) as a plant-test. After 45 d of growth, we evaluated the effects of P fertilization in total maize shoot biomass, P shoot accumulation, leaf pigment quantification by UV-Vis, and the evaluation of biochemical variations by DRIFT spectroscopy analysis associated with a chemometric approach in the same leaf extract used for pigment quantification. P fertilization raised biomass accumulation (∼7.4×), P uptake (∼2.3×), and total chlorophyll a and b contents (∼2.1×). DRIFT spectroscopy analysis of extracted pigments revealed an elevated content of proteins and polysaccharides at high P availability. At low P availability, we found a low efficiency of N metabolism suggested by the accumulation of inorganic N forms. DRIFT spectroscopy applied together with the classic leaf pigment extraction and quantification method is a novel and promising tool for plant nutrition studies as a DRIFT spectroscopy metabolic profile protocol.
Introduction
The quantification analysis of pigments has been reported for almost a century.1–4 Chlorophylls and carotenoids play a crucial role in the process of photosynthesis, and the concentration of leaf pigments is a parameter recurrently measured as an indicator of photosynthetic capacity,5 N content or whole plant nutrition,6,7 and plant health.8 At the laboratory scale, there are multitudes of options for pigment extractions using many organic solvents (e.g. acetone, methanol, ethanol, and dimethyl sulfoxide), solid–solution ratios, times of extraction and so on. In the last step, the concentration of pigments often follows photometrical determination.3,4,9 These procedures are easy, fast, and cheap, which help the leaf pigment quantification in plants to be widely used.
The methods for pigment extraction involve not only pigments but also several leaf compounds soluble in the organic solvent used, which until now has been barely explored by the research. The pigment extraction could give us other qualitative information or eventually semiquantitative data about the biochemical composition status, in the same line as the metabolic profile. The possibility to use the traditional leaf pigments’ extraction targeting the soft plant metabolic profile discloses a new analytical approach for a well-established method, providing a deeper level of information on a quick and cheap lab work way.
Currently, the metabolic profile studies use laborious and time and expensive reagent-consuming methods. For example, liquid chromatography–mass spectrometry (LC-MS) analysis shows high sensitivity and can identify and quantify a large number of metabolites, even low concentration secondary metabolites.10,11 However, LC-MS10 requires around 6 days for a complete analysis and shows twelve critical points throughout the process, which increases the chances of failures and discrepancies between samples. All of that together also makes it an expensive analysis, with commercial labs charging around US$ 100 per sample (e.g. Center for Environmental & Human Toxicology, University of Florida). The gas chromatography–mass spectrometry (GC-MS) method offers a good balance of sensitivity and reliability, being considerably more sensitive than nuclear magnetic resonance (NMR) and more robust than LC-MS. However, the GC-MS method12 requires ten days for final results and shows two critical points as well, including the quantification of low derivatization compounds such as sugars and aminosugars.13 All of that also makes the GC-MS method an expensive one, costing around US$ 65 per sample (e.g. West Coast Metabolomics Center, UC Davis). NMR is less sensitive than LC-MS and GC-MS but is ideal for quantifying and identifying the structure of unknown compounds in untargeted metabolomic studies.14 The NMR protocol15 is simpler than LC-MS and GC-MS, but the necessity of special reagents (e.g. D2O) and expensive equipment gives this analysis a high cost as well, around US$ 60 per sample (e.g. Chemical Instrumentation Center, Boston University). Thus, despite extensive capability to quantify and qualify the metabolites, these methods are time-consuming and of high cost.
Fourier transform infrared spectroscopy, especially diffuse reflectance infrared Fourier transform (DRIFT) spectroscopy, is a rapid and non-destructive technique that allows acquiring chemical and structural information of metabolites. The diffuse reflectance geometry requires minimum sample preparation compared to the traditional transmission geometry, which requires the production of sample pellets (e.g. mixing and pressing samples with KBr). Compared with attenuated total reflection geometry, DRIFT has the advantage of evaluating not only the surface of the sample but also a certain volume of it, as the IR beam penetrates the sample to a certain extent before being re-emitted, which improves the spectral information acquisition. DRIFT studies about mineralogy,16 adsorption,17–19 and wood20 and straw21 decomposition show the advantages of this technique. Associated with DRIFT spectroscopy, principal component analysis (PCA) is a mathematical tool for data reduction and exploratory analysis, which is useful for DRIFT data analysis. The key objective of PCA is to find a small set of principal components (PC) that describe the greatest amount of variability in these data sets, supporting an easier understanding of the original data. It allows the recognition and stressing characteristics and their correlation to the chemical properties of the sample. This and other chemometric tools such as parallel factor analysis22 and two-dimensional correlation19,23 are especially useful in the interpretation of infrared spectroscopic data and spectra due to extensive data collection and hard visual investigation.24
We aimed to boost the classic leaf pigment quantification, adding leaf biochemical information derived from DRIFT spectroscopy + principal component analysis, using the same leaf pigment extract produced by the classical quantification method. We performed a dose–response experiment revisiting a well-studied concern in plant nutrition about the low phosphate availability in highly weathered soil. Hence, we evaluated the effects of P dose fertilization in maize biomass yield or P accumulation and also presented new insights into biochemical composition from leaf pigment evaluation offered by DRIFT spectroscopy and PCA analysis.
Materials and methods
Greenhouse trial
An Oxisol was collected at 0.0–0.2 m depth in an uncultivated area, located in Piracicaba, state of São Paulo, Brazil (22°43′06′′ S; 47°36′31.4′′ W). Samples were air-dried and passed through a 4 mm sieve for experiments and a 2 mm sieve for chemical and physical analyses.25 The soil presented low available P (13 mg dm−3, ion exchange resin extractant), 24% of clay,25 a pH of 4.7 (0.01 M CaCl2 at a ratio of 1
:
2.5 v/v), low exchangeable Ca2+ and Mg2+ (41 and 11 mmolc dm−3, respectively, using ion exchange resin extractant), low organic C (27 g kg−1, dichromate oxidation26), and high P buffer capacity (low remaining-P, 22 mg L−1 P27). The soil clay mineralogy is mainly composed of kaolinite and gibbsite, as well as minor contents of Fe oxides such as goethite and hematite.
Soil samples of 2 dm3 were placed in plastic pots. CaCO3 and MgCO3 (Ca
:
Mg ratio of 4
:
1) were used to neutralize soil acidity to reach 50% base saturation. We added water in each pot to raise the soil water content to about 80% of the maximum water retention capacity (MWRC), maintaining the pots under incubation for 14 d. After, the soil samples were air dried and passed through a sieve with a mesh of 4.0 mm, the P fertilizer was mixed with soil samples. The P doses were 0, 30, 60, 120, 180 and 300 mg dm−3, using triple-superphosphate (20% P) as the P source, and are defined according to the remaining-P as 0, 0.25, 0.5, 1, 1.5 and 2.5 times the optimal P dose to overcome P soil availability limitation.28,29 Lastly on the same day, five maize (Zea mays L.) seeds (hybrid Dow SwB585) were sown per pot. After seedlings’ emergence (after 4 d), two plants were left per pot.
The addition of other nutrients was carried out by nutrient solution applications according to Barreto et al. (2008).29 Briefly, the doses and sources of the other nutrients corresponded to: 320 mg dm−3 of N [(NH4NO3]; 160 mg dm−3 of K (KCl); 80 mg dm−3 of S [(NH4)2SO4]; 4 mg dm−3 of Mn (MnCl2·4H2O); 4 mg dm−3 of Zn (ZnSO4·7H2O); 1.6 mg dm−3 of Fe(FeCl3·6H2O); 1.5 mg dm−3 of Cu (CuSO4·5H2O); 0.9 mg dm−3 of B (H3BO3); and 0.2 mg dm−3 of Mo (NaMoO4·2H2O). The plants were grown in a greenhouse under natural light conditions, with the temperature varying from 26 to 33 °C (day) to 16–20 °C (night), and a photoperiod (14 h day/10 h night) for 45 days. The pots were kept with the soil moisture close to 80% of the MWRC by irrigation in the upper part of the pots. The experimental design was in randomized blocks, with four replicates.
Plant sampling, determination of the contents of pigments, and P content
After 45 days of cultivation, we collected the middle third of the last totally expanded leaf, without the central vein and immediately stored at −20 °C until subjected to freeze-drying. The total leaf pigments were extracted and measured as described by Lichtenthaler et al.4 Briefly, 100 mg of dried leaf samples were weighed in polypropylene tubes (15 ml), and 10 ml of cold acetone solution (acetone/deionized water; 80% v/v) were added, gently macerated using a thin plastic pestle (∼1 min) and left to rest at −8 °C for 24 h. The homogenate was centrifuged (830g for 5 min) and the supernatant was collected. The spectrophotometer (UV5, Mettler Toledo) was previously blanked using acetone solution, then the absorbance at 663, 647, and 470 nm wavelength of acetone solution + leaf pigment was measured at 22 ± 2 °C in a crystal cuvette of 1 cm length. The pigment concentration was determined following the classical method4 using eqn (1)–(3), respectively: | Chlorophyll a (Chla) = 12.25A663 − 2.79A647; | (1) |
| Chlorophyll b (Chlb) = 21.50A647 − 5.10A663; | (2) |
and | Carotenoids = [1000A470 − 1.82(Chla) − 95.15(Chlb)]/225. | (3) |
The maize shoot was collected, dried in a forced-air circulation oven at 65 °C for 72 h, after which the shoot dry matter was weighed. The Mitscherlich equation was fit to the data of dry matter production according to eqn (4);
| ŷ = y0 + Amax × (1 − e−bx) | (4) |
in which
ŷ is the dry matter production by shoots (g pot
−1), the variable
x corresponds to the P dose added (mg dm
−3),
y0 is the dry biomass accumulated without P addition,
Amax is the maximum of dry matter production estimated (
x → +∞), and
b is a parameter related to the shape of the curve.
The plant shoot tissue digestion was performed in an open-vessel-digestion system using the mixture of nitric and perchloric acids (3
:
1), and the dosage was determined using the molybdenum blue method30 by colorimetry (UV5, Mettler Toledo).
DRIFT-spectroscopy analysis of the soluble extract
The pigment–acetone solution was used to evaluate the soluble compounds in the leaf. An aliquot of 0.25 ml from each replicate was added to 0.4 g of KCl placed in polypropylene tubes (2 ml) to produce a composite sample for each P dose. We choose KCl because it is “invisible” in the spectral range used here, cheaper than KBr, and easily found in soil science and plant nutrition laboratories around the world. The solvent evaporation step was carried out at room temperature (22 °C) in an air-flow chamber with exhaustion every 6 h, and then the tubes were stored in a desiccation chamber until analysis. Also, we performed the addition of 1 ml of pure acetone solution on KCl salt to create a “blank-KCl”.
The dried samples (pigments + KCl) were gently homogenized in the tube using a spatula for diffuse reflectance infrared Fourier transform (DRIFT) spectroscopy analysis. We collected the DRIFT spectra directly from the sample powder using a Fourier-transform infrared spectrometer (Alpha, Bruker Optics Inc., Billerica, MA, USA), equipped with a diffusive reflection element accessory (DRIFT module) and a DLATGS (deuterated L-alanine doped triglycine sulphate) detector. The spectra were acquired from 4000 to 650 cm−1 as the absorbance units, with 4 cm−1 of resolution and 32 scans were co-added per spectrum (time consumption per sample was ∼3 min). The background spectrum was measured using blank-KCl. Corrections for atmospheric interferences (water vapor and CO2), background subtraction and spectral averaging were carried out using OPUS/Mentor software.
DRIFT spectral data pre-treatments
Origin 2017 software was used for spectral processing (OriginLab Corporation, Northampton, Massachusetts, USA). The spectra were preprocessed using a combination of mean-centering and standard normal deviation, and then we applied the Savitzky–Golay smoothing process (second-order polynomial over 20 points), to eliminate some noise from electronic (e.g., electric-powered) or environmental (e.g., air humidity, temperature) oscillation over time of spectral acquisition. We normalized the spectra (minimum y value becomes 0 and the maximum becomes 1) for visual inspection. The PCA on the transposed data matrix (samples as columns and spectral bands as rows) was executed to identify changes in FTIR band absorption dependent P dose effects. The transposed matrix focuses on the variation of the spectroscopic signal within the samples, providing an estimation of the statistical weight (i.e., loadings) of the main constituents of the samples, allowing us to create a “score spectra” highlighting the main absorbance bands for each PC.31,32 For that, after the smoothing process, we selected the spectral range from 3900 to 950 cm−1 to eliminate high noise obtained close to the limit of detection of the equipment. The spectral derivatives were used to remove baseline and linear trends with the intention of eliminating both additive and multiplicative effects of artificial interferences,21,33,34 which can help disclose overlapping absorption peaks.
Results
Biomass and P accumulation
Enhanced P supply rate by fertilization incremented the dry shoot biomass (Fig. 1A). The biomass production had a good fitting to the Mitscherlich equation (ŷ = 4.35 + 27.33 × (1 − e−0.0015x); R2 = 0.95). The dry biomass increased from 4.4 g pot−1 (biomass production without P fertilization) to 32.4 g pot−1 at 300 mg dm−3 P, at the maximum production estimated (Amax = 31.7 g pot−1) without any P limitation (P dose → +∞). Concomitantly, the P content in plant tissue increases linearly (ŷ = 0.5 + 0.0023x; R2 = 0.98) following P fertilization (Fig. 1B). Both increments in maize biomass production (∼7.4×) and P uptake (∼2.3×) confirm the strongest soil P limitation for suitable plant growth.
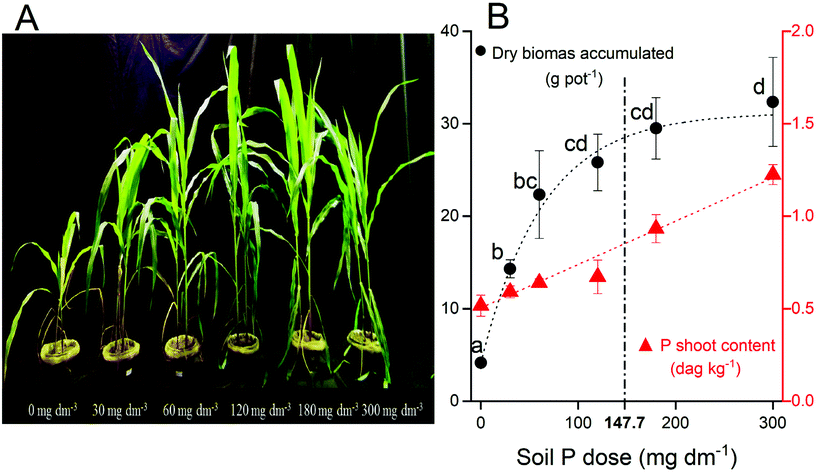 |
| Fig. 1 (A) Maize plants 45 d after seedling emergence at the harvest. (B) The dry biomass accumulated (black circle) and P contents in plant tissue (red circle) over the P fertilizer applied. The different letters above each black circle represent significant differences between treatments at P < 0.05 according to Tukey's HSD for one-way ANOVA. Dashed line represents the P dose equivalent of maximum agronomic efficiency (147.7 mg dm−3 P). Data are presented as the mean of four replicates followed by its standard error (±SE). | |
Pigment content
The pigments’ content in maize leaf rose from 0.64 to 1.22 mg g−1 for chlorophyll a (Chla), from 0.62 to 1.50 mg g−1 for chlorophyll b (Chlb), and from 0.15 to 0.50 mg g−1 for carotenoids when we compare the no P addition with the highest P dose treatments (Fig. 2). The contents reached a plateau near to the maximum agronomic efficiency (147.7 mg dm−3 P), reinforcing the optimum P dose concept.
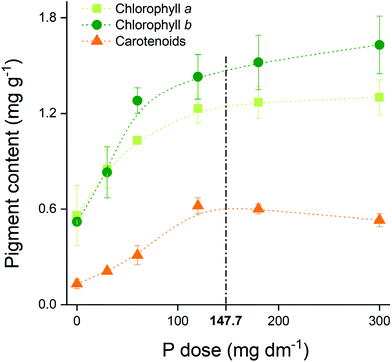 |
| Fig. 2 Pigment content in maize leaf over P doses applied. Dashed line represents the dose equivalent of maximum agronomic efficiency (147.7 mg dm−3 P). Data are presented as the mean of four replicates followed by their standard error (±SE). | |
DRIFT spectroscopy investigation
The DRIFT spectra give us some remarkable insights into the acetone extractable compounds in maize leaf (Fig. 3). The use of “acetone extractable” leaf fraction allowed us to investigate the spectra of biochemical compounds that should be hidden above the strong IR absorption band produced by dominant biochemical compounds, such as cellulose and lignin, if we used DRIFT spectroscopy analysis directly on the leaf.
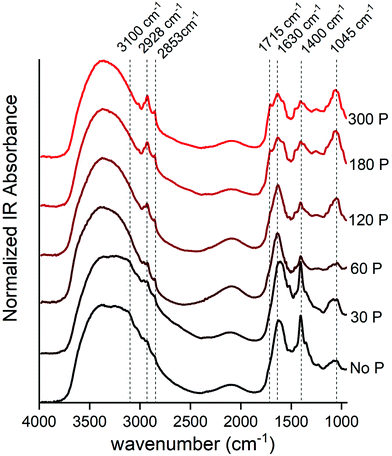 |
| Fig. 3 Normalized DRIFT spectra of maize leaf extract from acetone solution (acetone/deionized water; 80% v/v) dried on KCl salt. The P dose (mg dm−3) applied for rise from bottom (black) to upward (red). The data were stacked to improve visual analyses. The FTIR center peak assignment is: 3100 cm−1 = N–H stretch; 2920 cm−1 = symmetric methylene (–CH2–) stretching; 2850 cm−1 = symmetric methyl (–CH3) stretching; 1715 cm−1 = carbonyl double bond (C O) of carboxylic acids and symmetric C O of ester groups, primarily from lipids and fatty acids; and C O group of chlorophyll molecule; 1650 ± 50 cm−1 = asymmetric (C O) of amides associated with proteins (amide I) or carboxylate from pectin; 1400 cm−1 = ionized carboxylic acids (–COO−) of organic acids and asymmetric N–O stretch of nitrate (NO3−1) or asymmetric N–H stretching of ammonium (NH4+); 1045 ± 30 cm−1 = polysaccharides. | |
At a lower P supply, the strongest peak was visibly centered at 1400 cm−1, which could be assigned to ionized carboxylic acids (–COO−)35,36 of organic acids or inorganic N forms as NO3−
37 and NH4+.38 The second option is preferential, because the strongest peak of carboxylic acids (1715 cm−1)35,36 was not observed, and there is another distinctive peak (3100 cm−1) related to the N–H bond.38,39
The broad FTIR band has a center peak at 1045 cm−1 and its area had a strong increase as a response to P doses (Fig. 3). This region is assigned to the carbohydrate-like fingerprint region,21,40,41 such as xyloglucan (1036 cm−1),42 starch (∼1010 cm−1)43 and sucrose (1056 cm−1).44 Also, this band could be associated with the DNA backbone at 1224 (νas PO4−), 1088 (νs PO4−), 1055 (backbone ν C–O), 1021 (furanose vibration),45 or even free orthophosphate (–PO4) groups,18 because the P content in the leaf was expressive at higher P doses (Fig. 1). The visual inspection of DRIFT spectra suggested an increment in both of these peaks, which likely means an increment in both these structural and energetic polysaccharides as well as genetic material, therefore suggesting a increase in whole plant activity linked with the photosynthetic metabolism.
Other peak regions that were boosted following the P dose increment were those around 2928 and 2853 cm−1, which represent the C–H bond, mainly in hydrophobic compounds such as leaf wax and membrane lipid structures.46–48 Of note, these peaks are related to the aliphatic methylene chain, such as those found in chlorophylls and carotenoid structures, which is in agreement with the increment of total pigment content shown in Fig. 2.
The peak at 1630 cm−1 had a slight decrease from 0 to 300 mg dm−3 P. This peak is assigned to C
O vibrations of amides associated with proteins (amide I)40,42,49 or carboxylate from pectin.50 However, the peak concerning amide II (1550 cm−1) was not prominent at lower P doses, and this suggests that the contribution of protein for this band region began just at moderated P availability and P starvation. Thus, this peak likely is associated with pectin accumulation.
The slight shoulder at 1715 cm−1 could be assigned to the carbonyl double bond (C
O) of carboxylic acids35,36 and symmetric stretching C
O of ester groups, primarily from lipids and fatty acids,40 and the C
O group of the chlorophyll molecule.51 The increase of absorbance derived from these IR bands followed the total improvement of metabolic performance due to P fertilization.
Principal component analysis (PCA)
The plot of the scores of PCs allowed the qualitative recognition of different groups of samples (Fig. 4). The first two principal components (PCs) explained about 93% of the total variability observed in the dataset; meanwhile PC1 did not allow for discrimination between sample groups as observed before,52,53 because all samples were located in the negative side of PC1 (data not showed), and since all samples corresponded to a similar biochemical composition of maize leaf regardless of the P dose. However, PC2 (9.49%) and PC3 (4.12%) reached a satisfactory group association, because PC2 segregated a lower P dose at positive score values, and higher P dose at negative ones (Fig. 4A). PC3 segregated the intermediates’ P doses (60 and 120 mg dm−3) at negative score values.
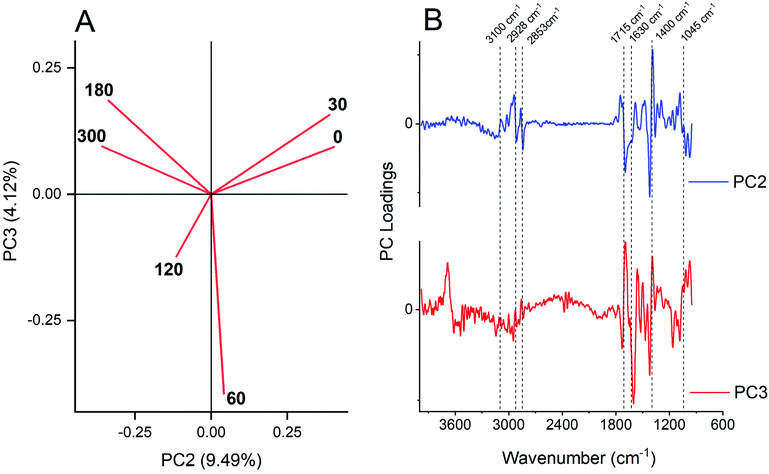 |
| Fig. 4 (A) PCA of the first derivative DRIFT spectra (3900 to 950 cm−1 range) of pigment extraction of maize leaf dependent on P dose fertilization. (B) Loading profile of PC2 (blue line) and PC3 (black line). The FTIR center peak assignment is: 3100 cm−1 = N–H stretch; 2920 cm−1 = symmetric methylene (–CH2–) stretching; 2850 cm−1 = symmetric methyl (–CH3) stretching; 1715 cm−1 = carbonyl double bond (C O) of carboxylic acids and symmetric C O of ester groups, primarily from lipids and fatty acids; and C O group of chlorophyll molecule; 1650 ± 50 cm−1 = asymmetric (C O) of amides associated with proteins (amide I) or carboxylate from pectin; 1400 cm−1 = ionized carboxylic acids (–COO−) of organic acids and asymmetric N–O stretch of nitrate (NO3−1) or asymmetric N–H stretching of ammonium (NH4+); 1045 ± 30 cm−1 = polysaccharides. | |
The loadings of the PC2 and PC3 plots vs. wavenumber (Fig. 4B) offer information about the link between scores and variables. Negative loadings for PC2, which grouped the higher P dose treatments, showed wavenumber characteristics mostly for methyl and methylene stretching groups (2920 and 2850 cm−1),46–48 protein (from 1690 to 1610 cm−1),40,42,49 –CH2 scissoring of lipids (1430 ± 10 cm−1) following 2920 cm−1 band absorbance improvement, and polysaccharides21,40,41 or even nucleic acid45 (1010 ± 10 cm−1). Of note, the positive PC2 loading at 1380 cm−1, which is associated with inorganic forms of N, reinforces the accumulation of these ions in leaf of P-starved plants.54–57
PC3 segregated in negative score, the sample spectra of the middle P dose (60 and 120 mg dm−3) (Fig. 4A) mainly by negative loadings of amides (1601 cm−1) and –CH2 scissoring of lipids (1430 ± 10 cm−1) and higher (180 and 300 mg dm−3) together with the lower dose (0 and 30 mg dm−3). However, when observing the variation on carotenoids pigments, one can state that there was a maximum at 120 mg dm−3 (Fig. 2), which could imply the PC segregation.
Discussion
The P fertilization improved strong biomass accumulation until 120 mg dm−3 P dose, and after that, there was no significant increment (P < 0.05) (Fig. 1). The maize plants, however, kept accumulating more P (Fig. 1). The maximum agronomic efficiency (0.9Amax) was reached with 147.7 mg dm−3 P, which is close to the recommendation of about 120 mg dm−3 as a suitable P dose for biomass production when related to its soil P-buffer capacity.58–60 The accumulation of P in plant tissue in dose above Amax represents a high consumption of P (Fig. 1B), which did not improve the biomass yield.
The P nutrition was strictly associated with the photochemistry process and energy light efficiency for biomass accumulation in our results. The P absorption benefited more the production of Chlb than the Chla as observed here and previously.61 Chlb is mostly associated with the light-harvesting complex connected to photosystem II (PSII), and the decrease of the Chl a/b ratio is probably indicative of a relative gain of photosystem II (PSII) peripheral antenna, or, alternatively, a change in photosystem stoichiometry in favor of PSII.5,61 This increment was followed by the contents of carotenoids, which play a role in the photo-protection of photosynthetic apparatus.5,61 Other molecular and physiological responses to P deficiency are well discussed in previous studies.62–64
The higher N absorption and nutrient use efficiency are supported when there is a satisfactory P supply.65 The effect of P nutrition in the metabolism of N had been described, and the N
:
P ratio has strong effects associated with root allocation, nutrient uptake, biomass turnover, and reproductive output.54–56 The increment of soluble sugar and decrease of nitrate in cabbage (Brassica oleracea L.) leaves were observed when there was proper P supply.57
Ours results and conclusion described here about P effects in plant metabolism by the DRIFT spectroscopy + PCA approach present a strong agreement with conventional metabolic profile studies. For example, the levels of carbohydrates and glycolysis intermediates in tomato leaves were extremely decreased by P deficiency,7 or citrus growing under P-deficient conditions showed increased activity of the arginine biosynthetic due to an increase in the concentration of ammonia in leaves.66 The upregulation of protein degradation observed in the P-starvation condition67 coincides with the low amide I IR band absorption (Fig. 3, 1650 ± 50 cm−1). In the end, DRIFT spectroscopy + PCA could not provide a detailed polysaccharide composition (e.g. quantities of saccharose, glucose or starch) as the traditional metabolic profile methods. However, at a very low cost (estimated to be around US$ 0.15 per sample, including costs for pigment extraction and IR analysis), with a way simpler method that requires few procedure steps, and a relatively faster analysis (2–3 days), we reached conclusions similar to traditional methods for the metabolic profile.
Conclusions
Overall, our study discloses new insights into biochemical compounds and plant nutrition status, using for the first time, DRIFT spectroscopy together with the classical plant pigment analyses. In summary, the visual inspection (Fig. 3) along with the chemometrics tool (PCA, Fig. 4) gave us considerable information that biochemical compounds are more affected by phosphate nutrition. The low efficiency of N metabolism by accumulating inorganic N forms at P starvation was highlighted as soon as the contents of protein and polysaccharides increased at high P availability. This conclusion is in agreement with a soft “metabolic profile” spectroscopic approach, which represents an upgrade in the conventional analyses for pigment quantification.
There are considerable variations in the scientific literature related to pigment extraction such as: the material used (e.g., fresh, dried, and freeze-dry), solvent (e.g., acetone 80 or 100%, methanol, and dimethyl sulfoxide), sample mix (e.g., grinding and sonication), solvent–sample ratio, contact time, and temperature, even in the equations used for pigment quantification. In this first stage, none of this variation prohibits the DRIFT analysis for the plant leaf extract. The spectroscopic method proposed here could and should be adjusted for each laboratory routine. Other multivariate analyses such as multiple linear regression and hierarchical cluster analysis could be implemented.
The DRIFT spectroscopy + PCA approach described here brings deep level information by an inexpensive and fast workflow. We strongly suggest that this new method should be incorporated in leaf pigment analyses for several plant science studies as an evaluation of other abiotic and biotic plant stresses, as a soft “plant metabolic profile” assessment.
Conflicts of interest
There are no conflicts to declare.
Acknowledgements
The first author gratefully thanks the São Paulo Research Foundation (FAPESP) (grant # 2016/05870-1, # 2016/22058-9) for the scholarship granted for this research. This study was financed in part by the Brazilian Coordination for the Improvement of Higher Education Personnel (CAPES) - #Finance Code 001, and by the Conselho Nacional de Ciência e Tecnologia - Brasil (CNPq). The authors also thank the Geotechnologies in Soil Science Group (GeoCIS/GeoSS) (https://esalqgeocis.wixsite.com/english) for the DRIFT spectroscopy analysis (FAPESP grant # 2014/22262-0).
References
- F. M. Schertz, Plant Physiol., 1928, 3, 211–216, DOI:10.1104/pp.3.2.211.
- D. I. Arnon, Plant Physiol., 1949, 24, 1–15, DOI:10.1104/pp.24.1.1.
- R. J. Porra, W. A. Thompson and P. E. Kriedemann, Biochim. Biophys. Acta, Bioenerg., 1989, 975, 384–394, DOI:10.1016/S0005-2728(89)80347-0.
- H. K. Lichtenthaler and C. Buschmann, Curr. Protoc. Food Anal. Chem., 2001, 1, F4.3.1–F4.3.8, DOI:10.1002/0471142913.faf0403s01.
- C. Hermans, G. N. Johnson, R. J. Strasser and N. Verbruggen, Planta, 2004, 220, 344–355, DOI:10.1007/s00425-004-1340-4.
- D. Zhao, K. Raja Reddy, V. G. Kakani, J. J. Read and G. A. Carter, Plant Soil, 2003, 257, 205–218, DOI:10.1023/A:1026233732507.
- J. Sung, S. Lee, Y. Lee, S. Ha, B. Song, T. Kim, B. M. Waters and H. B. Krishnan, Plant Sci., 2015, 241, 55–64, DOI:10.1016/j.plantsci.2015.09.027.
- K. Demirevska-Kepova, L. Simova-Stoilova, Z. P. Stoyanova and U. Feller, J. Plant Nutr., 2006, 29, 451–468, DOI:10.1080/01904160500524951.
- H. K. Lichtenthaler, A. Ač, M. V. Marek, J. Kalina and O. Urban, Plant Physiol. Biochem., 2007, 45, 577–588, DOI:10.1016/j.plaphy.2007.04.006.
- R. C. De Vos, S. Moco, A. Lommen, J. J. Keurentjes, R. J. Bino and R. D. Hall, Nat. Protoc., 2007, 2, 778–791, DOI:10.1038/nprot.2007.95.
- O. Fiehn, J. Kopka, P. Dörmann, T. Altmann, R. N. Trethewey and L. Willmitzer, Nat. Biotechnol., 2000, 18, 1157–1161, DOI:10.1038/81137.
- J. Lisec, N. Schauer, J. Kopka, L. Willmitzer and A. R. Fernie, Nat. Protoc., 2006, 1, 387–396, DOI:10.1038/nprot.2006.59.
- D. J. Beale, F. R. Pinu, K. A. Kouremenos, M. M. Poojary, V. K. Narayana, B. A. Boughton, K. Kanojia, S. Dayalan, O. A. H. Jones and D. A. Dias, Metabolomics, 2018, 14, 152, DOI:10.1007/s11306-018-1449-2.
- J. L. Markley, R. Brüschweiler, A. S. Edison, H. R. Eghbalnia, R. Powers, D. Raftery and D. S. Wishart, Curr. Opin. Biotechnol., 2017, 43, 34–40, DOI:10.1007/s11306-018-1449-2.
- H. K. Kim, Y. H. Choi and R. Verpoorte, Nat. Protoc., 2010, 5, 536–549, DOI:10.1038/nprot.2009.237.
- E. Yusiharni and R. Gilkes, Appl. Clay Sci., 2012, 64, 61–74, DOI:10.1016/J.CLAY.2011.12.005.
- M. S. C. Barreto, E. J. Elzinga and L. R. F. Alleoni, Environ. Pollut., 2020, 262, 114196, DOI:10.1016/j.envpol.2020.114196.
- M. S. C. Barreto, E. J. Elzinga and L. R. F. Alleoni, Soil Sci. Soc. Am. J., 2020, 84, 57–67, DOI:10.1002/saj2.20005.
- M. S. C. Barreto, E. J. Elzinga and L. R. F. Alleoni, Sci. Rep., 2020, 10, 13441, DOI:10.1038/s41598-020-70201-z.
- X. Colom, F. Carrillo, F. Nogués and P. Garriga, Polym. Degrad. Stab., 2003, 80, 543–549, DOI:10.1016/S0141-3910(03)00051-X.
- L. G. Pimentel, M. S. C. Barreto, D. M. da Silva Oliveira, M. R. Cherubin, J. A. M. Demattê, C. E. P. Cerri and C. C. Cerri, BioEnergy Res., 2019, 12, 909–919, DOI:10.1007/s12155-019-10024-7.
- V. L. Skrobot, C. de Sousa Santos and J. W. Batista Braga, Energy Fuels, 2019, 33, 6170–6176, DOI:10.1021/acs.energyfuels.9b01001.
- I. Noda, Spectrochim. Acta, Part A, 2017, 187, 119–129, DOI:10.1016/J.SAA.2017.06.034.
- R. Kumar and V. Sharma, TrAC, Trends Anal. Chem., 2018, 105, 191–201, DOI:10.1016/j.trac.2018.05.010.
- M. Pansu and J. Gautheyrou, Exp. Agric., 2007, 43, 401, DOI:10.1017/S0014479707005042.
- A. Walkley and I. A. Black, Soil Sci., 1934, 37, 29–38, DOI:10.1097/00010694-193401000-00003.
-
M. E. C. Claessen, W. D. O. Barreto, J. L. De Paula and M. N. Duarte, Manual de Métodos de Análise de Solo, 1997, vol. 2 Search PubMed.
- J. V. dos Reis, V. Víctor Hugo Alvarez, R. D. Durigan, R. B. Paulucio and R. B. Cantarutti, Rev. Bras. Cienc. Solo, 2020 DOI:10.36783/18069657rbcs20190113.
- M. S. C. Barreto, E. M. Mattiello, W. O. Santos, L. C. A. Melo, L. Vergütz and R. F. Novais, J. Environ. Manage., 2018, 208, 1–7, DOI:10.1016/J.JENVMAN.2017.11.075.
- J. Murphy and J. P. Riley, Anal. Chim. Acta, 1962, 27, 31–36, DOI:10.1016/S0003-2670(00)88444-5.
- L. López-Merino, N. Silva Sánchez, J. Kaal, J. A. López-Sáez and A. Martínez Cortizas, Global Planet Change, 2012, 92–93, 58–70, DOI:10.1016/j.gloplacha.2012.04.003.
- M. Traoré, J. Kaal and A. Martínez Cortizas, Spectrochim. Acta, Part A, 2016, 153, 63–70, DOI:10.1016/j.saa.2015.07.108.
- Å. Rinnan, F. van den Berg and S. B. Engelsen, TrAC, Trends Anal. Chem., 2009, 28, 1201–1222, DOI:10.1016/j.trac.2009.07.007.
- C. Zhou, W. Jiang, B. K. Via, O. Fasina and G. Han, Carbohydr. Polym., 2015, 121, 336–341, DOI:10.1016/j.carbpol.2014.11.062.
- W. G. Gao, X. C. Liu and M. F. Chen, RSC Adv., 2017, 7, 41011–41016, 10.1039/C7RA04587E.
- M. R. Noerpel and J. J. Lenhart, J. Colloid Interface Sci., 2015, 460, 36–46, DOI:10.1016/j.jcis.2015.08.028.
- E. Choe, F. van der Meer, D. Rossiter, C. van der Salm and K.-W. Kim, Water, Air, Soil Pollut., 2010, 206, 129–137, DOI:10.1007/s11270-009-0091-z.
- M. K. Deb and D. Verma, Microchim. Acta, 2010, 169, 23–31, DOI:10.1007/s00604-010-0308-2.
- T. P. Chopra, R. C. Longo, K. Cho and Y. J. Chabal, Surf. Sci., 2016, 650, 285–294, DOI:10.1016/j.susc.2016.01.002.
- M. Giordano, M. Kansiz, P. Heraud, J. Beardall, B. Wood and D. McNaughton, J. Phycol., 2001, 37, 271–279, DOI:10.1046/j.1529-8817.2001.037002271.x.
- J. Yang and H. E. Yen, Plant Physiol., 2002, 130, 1032–1042, DOI:10.1104/pp.004325.
- S. Sharma and K. N. Uttam, Vib. Spectrosc., 2017, 92, 135–150, DOI:10.1016/J.VIBSPEC.2017.06.004.
- F. J. Warren, M. J. Gidley and B. M. Flanagan, Carbohydr. Polym., 2016, 139, 35–42, DOI:10.1016/j.carbpol.2015.11.066.
- J. Garrigues, Talanta, 2000, 51, 247–255, DOI:10.1016/S0039-9140(99)00258-1.
- M. P. Schmidt and C. E. Martínez, Environ. Sci. Technol., 2018, 52, 4079–4089, DOI:10.1021/acs.est.7b06173.
- B. Ribeiro da Luz, New Phytol., 2006, 172, 305–318, DOI:10.1111/j.1469-8137.2006.01823.x.
- M. D. Guillén and N. Cabo, J. Am. Oil Chem. Soc., 1997, 74, 1281–1286, DOI:10.1007/s11746-997-0058-4.
- E. J. Martínez, M. V. Gil, C. Fernandez, J. G. Rosas and X. Gómez, PLoS One, 2016, 11, e0153139, DOI:10.1371/journal.pone.0153139.
- M. P. Schmidt and C. E. Martínez, Langmuir, 2016, 32, 7719–7729, DOI:10.1021/acs.langmuir.6b00786.
- A. Chatjigakis, C. Pappas, N. Proxenia, O. Kalantzi, P. Rodis and M. Polissiou, Carbohydr. Polym., 1998, 37, 395–408, DOI:10.1016/S0144-8617(98)00057-5.
- A. A. Zabelin, K. V. Neverov, A. A. Krasnovsky, V. A. Shkuropatova, V. A. Shuvalov and A. Y. Shkuropatov, Biochim. Biophys. Acta, Bioenerg., 2016, 1857, 782–788, DOI:10.1016/j.bbabio.2016.03.029.
- H. Chen, C. Ferrari, M. Angiuli, J. Yao, C. Raspi and E. Bramanti, Carbohydr. Polym., 2010, 82, 772–778, DOI:10.1016/j.carbpol.2010.05.052.
- S. Genest, R. Salzer and G. Steiner, Anal. Bioanal. Chem., 2013, 405, 5421–5430, DOI:10.1007/s00216-013-6967-1.
- F. Buwalda and M. Warmenhoven, J. Exp. Bot., 1999, 50, 813–821, DOI:10.1093/jxb/50.335.813.
- C. C. de Groot, L. F. M. Marcelis, R. van den Boogaard, W. M. Kaiser and H. Lambers, Plant Soil, 2003, 248, 257–268, DOI:10.2135/cropsci2016.11.0970.
- S. Güsewell, New Phytol., 2004, 164, 243–266, DOI:10.1111/j.1469-8137.2004.01192.x.
- X. Yang, M. Gu, Y. Kang and X. Feng, J. Plant Nutr. Soil Sci., 2012, 175, 582–594, DOI:10.1002/jpln.201100237.
- P. B. Leite, V. V. H. Alvarez, N. F. de Barros, J. C. L. Neves, M. A. Guarçoni and A. Zanão Júnior, Rev. Bras. Cienc. Solo, 2009, 33, 1311–1322, DOI:10.1590/S0100-06832009000500024.
- H. Q. Santos, D. M. Fonseca, R. B. Cantarutti, V. V. H. Alvarez and D. Nascimento Júnior, Rev. Bras. Cienc. Solo, 2002, 26, 173–182, DOI:10.1590/S0100-06832002000100018.
- D. A. Rogeri, C. Gianello, L. Bortolon and M. B. Amorim, Rev. Bras. Cienc. Solo, 2016 DOI:10.1590/18069657rbcs20140535.
- M. Plesniǎr, R. Kastori, N. Petrović and D. Panković, J. Exp. Bot., 1994, 45, 919–924, DOI:10.1093/jxb/45.7.919.
- T. C. Bang, S. Husted, K. H. Laursen, D. P. Persson and J. K. Schjoerring, New Phytol., 2021, 229, 2446–2469, DOI:10.1111/nph.17074.
- D. Gutiérrez-Alanís, J. O. Ojeda-Rivera, L. Yong-Villalobos, L. Cárdenas-Torres and L. Herrera-Estrella, Trends Plant Sci., 2018, 23, 721–730, DOI:10.1016/j.tplants.2018.04.006.
- K. Zhang, H. Liu, P. Tao and H. Chen, PLoS One, 2014, 9, e98215, DOI:10.1371/journal.pone.0098215.
- J. M. Ruiz, L. Romero, R. S. Martn and R. Briones, J. Sci. Food Agric., 2000, 80, 2069–2073, DOI:10.1002/1097-0010(200011)80
:
14<2069::AID-JSFA749>3.0.CO;2-7.
- E. Rabe and C. J. Lovatt, Plant Physiol., 1986, 81, 774–779, DOI:10.1104/pp.81.3.774.
- T. W. Rufty, C. T. MacKown and D. W. Israel, Plant Physiol., 1990, 94, 328–333, DOI:10.1104/pp.94.1.328.
|
This journal is © The Royal Society of Chemistry 2021 |