DOI:
10.1039/C7AN00957G
(Critical Review)
Analyst, 2017,
142, 3025-3047
Raman spectroscopy for cancer detection and cancer surgery guidance: translation to the clinics
Received
9th June 2017
, Accepted 7th July 2017
First published on 11th July 2017
Abstract
Oncological applications of Raman spectroscopy have been contemplated, pursued, and developed at academic level for at least 25 years. Published studies aim to detect pre-malignant lesions, detect cancer in less invasive stages, reduce the number of unnecessary biopsies and guide surgery towards the complete removal of the tumour with adequate tumour resection margins. This review summarizes actual clinical needs in oncology that can be addressed by spontaneous Raman spectroscopy and it provides an overview over the results that have been published between 2007 and 2017. An analysis is made of the current status of translation of these results into clinical practice. Despite many promising results, most of the applications addressed in scientific studies are still far from clinical adoption and commercialization. The main hurdles are identified, which need to be overcome to ensure that in the near future we will see the first Raman spectroscopy-based solutions being used in routine oncologic diagnostic and surgical procedures.
Introduction to the international collaboration
The authors of this review closely collaborate in the development of applications of Raman spectroscopy in oncology, at the Erasmus University Medical Center in Rotterdam, the Netherlands. This is a line of research that was initiated over 20 years ago and which has resulted in over 30 publications in the peer-reviewed literature and in 4 patent applications.
The authors form a multidisciplinary team comprised of engineers, physicists, surgeons, pathologists, and dermatologists. Their joint expertise and experience covers many aspects of the development chain of clinically relevant Raman spectroscopy applications, from accurately defining the real clinical need, to developing state-of-the-art instrumentation and classification algorithms in collaboration with industry, and their validation in (pre-)clinical trials. The team is highly motivated by the opportunities to translate results of their research into clinical practice and to have an impact on the diagnosis and treatment of patients.
|
General introduction
In 2012 the World Health Organisation (WHO) reported 14.1 million new cancer cases, 8.2 million cancer deaths and 32.6 million people living with cancer (within 5 years of diagnosis). These numbers are increasing, which motivates development of cancer treatment possibilities and technology for early detection of (pre-) malignancies.1
The high mortality rate of cancer can be reduced by early and accurate diagnosis, and by adequate surgical treatment.2
The reference standard for cancer diagnosis is histopathologic assessment of biopsies or diagnostic excisions of suspicious tissue. After biopsy/excision the tissue specimen is fixed, micro-sectioned and routinely stained with haematoxylin and eosin (H&E). The pathologist makes a diagnosis based on microscopic examination of the H&E stained section. Because only small portions of the lesional tissue are biopsied or excised for histopathological examination, there is the risk of sampling error and the pathology report remains a subjective assessment (with its inter and intra operator variability).3
Studies have demonstrated that most tumour types develop through pre-malignant stages.4,5 Therefore, the treatment of pre-malignant tissue can prevent the further development of cancer. Because the distinction between early-stage malignant, pre-malignant and benign tumours can be difficult to make, repeated biopsies/excisions are often taken. For sampling of tissue, literature reports positive predictive values as low as 22% for prostate cancer diagnosis,6 1.4% for breast cancer,7 18.5% in lung cancer screenings8 and 7–23% for melanoma diagnosis.9 Despite the risk of these unnecessary biopsies/excisions, a substantial number of early stage tumours are still missed, which increases the risk of progression to a metastatic stage.
Introduction – early diagnosis/guided biopsy
A technique that helps to achieve representative biopsies and that would enable accurate and early in vivo diagnosis is needed. This tool should detect lesions in pre-malignant/early stages and assess large tissue areas in real-time to decrease sampling errors. Several techniques have been tested for biopsy guidance, such as optical coherence tomography (OCT), white light reflectance (WLR), auto-fluorescence and Raman spectroscopy.10–14
OCT and WLR rely on the visualization of changes in tissue structure. These techniques provide little or no information about the molecular tissue composition and, therefore, generally have a low specificity.10,12
Auto-fluorescence imaging is an optical technique that detects natural fluorescence emitted by fluorophores present in the tissues (e.g. flavins, collagen or haemoglobin), after excitation by a short-wavelength light source. This emission can be captured in real-time, for example during endoscopy, and can be used for lesion detection or characterization.13,14 Auto-fluorescence imaging has shown to improve the sensitivity of detection of early cancer, like epithelial neoplasia in oesophagus and colon (sensitivities are 90% and 99%, respectively).14 It also improves the diagnostic sensitivity (from 67% to 89%) for pre-malignant stages of lung cancer (e.g., dysplasia and carcinoma in situ). It also improves the diagnostic sensitivity compared to white-light endoscopic imaging (from 67% to 89%) for pre-malignant stages of lung cancer. However, the specificity of this technique is low; a specificity of 64% for diagnosing pre-malignant stages of lung cancer, a specificity of 81% for detecting high-grade dysplasia and early cancer in Barrett's oesophagus and a specificity of 35% for detection of pre-malignant colon polyps were reported.14–16
Optical vibrational spectroscopic techniques, such as Raman spectroscopy (RS), can provide high molecular specificity. The gradual changes from healthy tissue to tumour are reflected by their Raman spectra.2,17–19 Raman spectroscopy is a technique for characterizing biological tissue in vivo, ex vivo or in vitro and for non-invasive detection of the molecular differences between tumour and healthy tissue. It does not require any labelling, reagents or other preparation of the tissue, which facilitates translation to the clinic. With the use of optical fibres many anatomical locations can be assessed in vivo.20 Raman spectroscopy-based biopsy guidance can reduce the number of false positive biopsies and increase the accuracy of cancer diagnosis, with reported overall sensitivities and specificities between 73%–100% and 66%–100%, respectively.18,21
Introduction – guided surgery
After diagnosis the primary treatment for solid tumours is often surgery. The objective of surgical treatment is resection of all malignant tissue with adequate resection margins while preserving important healthy structures. Achieving adequate surgical margins is important for disease control and survival. Residual tumour after surgery is associated with poor survival and the need for additional surgery, adjuvant chemotherapy, radiation therapy, or a combination of these.22–25 A number of studies have shown that the 5-year survival decreases significantly when tumour is not completely removed.22–25 Intraoperative guidance tools can help to achieve adequate surgery. However, there are no widely used intraoperative guidance tools available yet. Current surgical resection techniques are based on subjective methods, such as palpation and visual inspection, to judge the border between normal and cancerous tissue.
In order to support the intraoperative assessment of resection margins, frozen sections can be used.26–28 A small piece of suspicious tissue is usually sampled from the wound bed (i.e. wound-driven assessment) by the surgeon. A microscopic evaluation of the frozen section is performed by the pathologist, directing operative management.28 It has been reported that the frozen section procedure increases the rate of adequate resections and thereby decreases local recurrence and improves the survival rate.29 Even though this procedure is successful for intraoperative assessment of the resection margins, it has its limitations: (1) it is time consuming, extending the duration of surgery and anaesthesia;30 (2) it is likely prone to sampling errors because only a small fraction of the resection margin can be investigated, and (3) it can introduce histologic disruption caused by rapid freezing, which makes the analysis more difficult.31 Rosenthal et al. (2015) reported that at most 5% to 10% of the wound bed can be sampled and assessed with frozen section.32 These limitations can lead to false negative results.23,27,33–35 Consequently, there is much room for improvement in performing intraoperative assessment of the resection margins.
In order to guide oncological surgery, techniques like intraoperative real-time MRI, intraoperative ultrasound, intraoperative OCT, fluorescence and Raman spectroscopy have been investigated in operating room environments.
Real-time intraoperative MRI has been tested during surgical resection of brain cancer (i.e. glioma). Several studies have demonstrated that this modality can be used for surgery guidance and improves the extent of the tumour resection without increasing neurological deficits. This also has a positive impact on survival.36–42 Some drawbacks of intraoperative MRI include: (1) operative defects that can affect the MRI image and decrease its accuracy;43 (2) interpretation of the image by the surgeon and his capability to correlate the location of the lesion to the brain anatomy;17,43 (3) significant surgical disruptions that prolong the operation time; (4) it requires a considerable investment to implement MRI-capable operating rooms; and (5) there is a need for contrast agents.43
Intraoperative ultrasound has been used to localize the tumour, guide the resection especially among highly vascular tumours (breast, e.g.), using probes that enhance contrast.43,44 However, it is less sensitive for tumour margins and has shown lower resolution and accuracy compared to intraoperative MRI. Besides, its accuracy is affected by previous surgery, as almost any imaging modality.43,45
A portable label-free optical coherent tomography (OCT) imaging system has been tested intraoperatively to assess breast resection margins and lymph nodes ex vivo.46 The study showed promising results for real-time microscopic image-guide breast cancer surgery.
Structural-based imaging techniques (like OCT and intraoperative MRI) show promising results. Unfortunately, they lack chemical disease-specific information, which is essential for adequate tumour removal.
Fluorescence imaging is another technique that has been applied to assess surgical resection margins. The technique requires administration of fluorescence agents and is based on the detection of fluorescently labelled structures during surgery. Various studies have reported that complete resection was achieved in a significantly higher percentage when fluorescence imaging was used, as compared to only regular white light.47–50 The major disadvantages of fluorescence imaging are: (1) the need for fluorescence agents, and (2) the need to switch off the room lighting to maximize the detection of the weak fluorescence.46 In addition, translational problems have been identified, related to the need of consistent manufacturing, to costs related with toxicology studies, and to the strict procedures for submission of a new investigational drug.51 An interesting alternative would be a label-free imaging method, which avoids the risks associated with dye/drug reactions and the challenges associated with specific tumour targeting and non-specific binding.
Raman spectroscopy has also been implemented to guide oncological surgery. Several studies have demonstrated that this technique can be used for surgical guidance. For example, in brain cancer surgery, an intraoperative Raman system that measures directly brain tissue in the patient, has proven to distinguish dense and low-density cancer infiltration from benign brain tissue with a sensitivity of 93% and a specificity of 91%.52 In another study, a real-time Raman intraoperative system was used during breast cancer surgery for assessment of freshly resected specimens. This study has demonstrated that Raman spectroscopy could discriminate cancerous tissue from normal breast tissue with a sensitivity of 83% and a specificity of 93%.53
Implementing Raman spectroscopy in the clinical setting can have important benefits: (1) it can enable representative sampling for correct pathological diagnosis (biopsy guidance); (2) it can accurately assist in defining adequate resection margins during surgery; (3) it can reduce the sampling problem, during intraoperative assessment; (4) it can introduce a more objective assessment and (5) it can reduce the need for adjuvant therapies.20
In the last years, several review articles reported the advances of Raman spectroscopy with the ultimate goal the clinical application for cancer diagnosis in different anatomical locations.17,18,20,21,54–57 In these reviews the latest developments of technology adaptations for Raman cancer diagnostic applications are described. The latest clinical outcomes of Raman spectroscopy on (early) cancer detection at different sites in vitro, ex vivo and in vivo have been also reported. However, specifically the translation of Raman spectroscopic developments into oncological applications has not been addressed extensively before.
The scope of this article is to provide an analysis of the translation of R&D results, obtained in oncological applications of Raman spectroscopy, into clinical practice. We discuss problems that still need to be solved in order to bring the technique successfully to the end-users in the hospital setting. The importance of defining the clinical needs and requirements, for different applications, is also explored in this review. We have limited our review to spontaneous Raman spectroscopy applications on ex vivo and/or in vivo human tissue samples. We refer to the other recent review articles for biomedical applications of non-linear Raman spectroscopy, such as coherent and surface-enhanced Raman scattering.55,58,59
Methods
Literature search
A search was carried out using the Web of Science™ library. Web of Science™ comprises the following databases: Web of Science™ Core Collection, KCI-Korean Journal Database, MEDLINE, Russian Science Citation Index and SciELO Citation INdex. For research articles the included period was from 2006 to 2017. For review articles the included period was from 2011 to 2017. The date of the final search was on 16th of January 2017. The search filter comprehended a combination of the keyword “Raman” with two of the following keywords: “tumo(u)r”, “patient”, “biopsy”, “carcinoma”, “assessment”, “intraoperative”, “cancer”, “guidance”, “surgery”, “human”, “diagnostics” and “translation”. These keywords were searched as a topic in the article. Articles that had at least three of the topic words were considered for inclusion. Commentaries and opinion articles were excluded. Furthermore, studies on non-human samples and performed in vitro were not selected. Studies that used non-linear Raman spectroscopy were excluded. Cytological screening methods based on Raman Spectroscopy are also being developed,60,61 however to limit the scope we have chosen not to include such studies in this review. Articles that were not written in English were also discarded.
Definition of the clinical need
The clinical needs (clinical problems and their relevance) that could be solved by Raman spectroscopy applications were defined per cancer type as reported by the studies selected in this review. Furthermore, the information on clinical needs from current (inter)national guidelines and clinical articles (PubMed) were included. For clinical articles the included period from 1990 to 2017 was regarded. Moreover, opinion from pathologists and surgeons was obtained based on personal interviews.
During the interviews clinical needs for other types of cancer than those addressed in the included studies were identified and discussed.
Raman spectroscopy applications
All the eligible Raman application studies were included, regardless the cancer type addressed. The included studies were divided considering two major oncological applications of Raman spectroscopy: (A) biopsy guidance/early diagnosis and (B) surgery guidance.
Progress of the companies
To address the progress of the companies that are developing/have developed commercial Raman spectroscopy oncological applications towards implementation in the clinics, we have contacted leading companies in Raman spectroscopy to collect information. The contacted companies were: Tokyo Instruments Inc., Princeton Instruments Inc., Verisante Inc., ODS Medical Inc., Endofotonics Pte. Ltd, RiverD International B.V., EmVision LLC, Invenio Imaging Inc., Artphotonics GmbH., Carl Zeiss GmbH., Kaiser Optical systems Inc., Lambda solutions Inc., Horiba Jobin-Yvon Inc., B&W Tek Inc., Snowy Range instruments Inc., Wasatch Photonics Inc., Witec GmbH., Avantes B.V., Photonetc Inc. and Renishaw Plc. A questionnaire was sent to the companies with the following questions: (1) “Are you actively developing or collaborating in the development of oncological Raman in vivo and/or ex vivo tools for the clinics?”; (2) “Can you indicate what is the application?”; (3) “Do you have any scientific publication or can you share with us more information about the subject?”; (4) “When do you think that it will be available in the market? What is the prognosis?”, and (5) “About the translation to the clinics, what hurdles have you encountered?”.
Technology readiness level (TRL) classification of the studies
In order to provide an overview of the current status of the technology towards clinical translation we classified all the included studies based on the Technology Readiness Level (TRL).
The TRL is an index used to measure the maturity and usability of an evolving technology.62 There are 10 levels (TRL 0 to TRL 9): at TRL 0 there is just an idea, but the concept is not yet proven or not yet tested; TRL 1 is related to basic research, when the principles are observed and postulated, but not experimentally proven; TRL 2 is associated with technology development, when the concept and application have been formulated; TRL 3 consists of applied research/proof of concept, when the first laboratory tests are completed; at TRL 4 a small scale prototype has been realized, which is still working in a laboratory environment; at TRL 5 the prototype has been tested in intended environment; at TRL 6 the prototype system has been tested in the intended environment close to the expected performance; at TRL 7 use of the system has been demonstrated in an operational environment at a pre-commercial scale; TRL 8 is characterized by the first version of a commercial system, when manufacturing issues are solved; finally, at TRL 9, the technology is available for end users.
We have grouped the TRL levels of the Raman applications into 4 main categories: (A) the proof of concept has been tested in the laboratory (TLR 0–3), (B) first hardware and software have been developed, including the algorithms for detection of (pre-) malignant lesions, and experiments have been performed close to the intended environment (TLR 4), (C) the developed hardware and software were tested with an independent dataset in the intended environment and preferably at different centres (TLR 5–7), and (D) the first of a kind commercial system(s) are/will be available soon for end users (TLR 8–9).
Results
Clinical need and Raman applications
Definition of the clinical need.
Prior to the development of technological tools aiming to improve diagnosis and/or treatment of cancer, it is important to define the actual clinical needs, because various clinical problems have different needs and solutions.
Currently, two main oncological clinical needs, which could be fulfilled by Raman spectroscopy, have been identified: (A) diagnostic tools for biopsy guidance for early diagnosis of (pre-) malignant, and (B) tools for surgery guidance, which can be used for intra-operative assessment of resection margins to achieve adequate tumour resection.
In this section we review several clinical problems in the process of diagnosis and surgical treatment of cancer.
Literature search for Raman spectroscopy applications.
Raman spectroscopy has been explored for oncological applications in numerous studies, employing various types of measurement systems, and targeting various types of cancers. This section also provides a review of the use of Raman spectroscopy ex vivo and in vivo for early diagnosis, biopsy guidance and surgery guidance of different types of cancer. Based on the search terms, 42 research papers on oncological applications of Raman spectroscopy were included. From those, 36 studies regarded early diagnosis and biopsy guidance applications and 6 studies regarded surgery guidance applications.
Breast cancer
Raman applications for early diagnosis/biopsy guidance.
Saha et al. developed a Raman spectroscopic tool for detecting microcalcifications, as an adverse sign, in breast tissue. An ex vivo study was conducted and Raman spectra were acquired in fresh stereotactic breast needle biopsies from 33 patients using a portable compact clinical multi-fibre Raman spectroscopy probe system. This first study resulted in a positive predictive value of 97% for detecting microcalcifications, demonstrating potential of Raman spectroscopy as real-time feedback tool for radiologists and to reduce unnecessary biopsies.75
Raman applications for surgery guidance.
Barman et al. looked at the utility of Raman spectroscopy as a guidance tool for mastectomy procedures. Spectra were acquired ex vivo on freshly excised specimen from a total of 33 patients. The Raman instrument was a portable clinical fibre probe system. A classification algorithm was developed to differentiate breast cancer from healthy tissue. The reported sensitivity was 62.5% with a specificity of 100%. The accuracy obtained for differentiation between normal, fibrocystic change, fibroadenoma, and breast cancer was 82.2%.76
Skin cancer
Clinical need.
The clinical diagnosis of skin cancer is conventionally based on visual inspection of morphologic characteristics of the lesions, usually supported by a dermoscope. This manner of diagnosing cancer is subjective and largely depends on the experience of the clinician.
Keratinocytic cancers.
Keratinocytic cancers, such as the basal cell carcinoma (BCC) and squamous cell carcinoma (SCC), are two of the most common cancers in fair-skinned populations.77 Only in the United Stated of America, about 3 million people are diagnosed with keratinocytic cancers per year. Although keratinocytic cancers are associated with low mortality rates both, BCC and SCC, can destruct surrounding tissue, recur and/or metastasize.78
SCC can be locally aggressive, difficult to treat and it is associated with a 0.5%–5% risk of metastasis. An effective management can be challenging in cases of patients with multiple lesions, or patients that in the past were diagnosed with keratinocytic cancers, demonstrating the need for early diagnosis.79
Standard type of treatment for keratinocytic cancers is complete removal of the lesion by (1) standard surgical excision or (2) Mohs micrographic surgery (MMS). In standard surgical excision, resection margins are examined by the pathologist after the procedure. In low risk BCC a tumour free resection margin of 3 mm is recommended, and in high risk BCC a margin of 5 mm is advised.78,80
In MMS, complete resection margins are examined by the specifically trained dermatologist and/or pathologist during the procedure, aiming to spare healthy tissue while removing completely the cancer cells. MMS leads to fewer recurrences, however this procedure is labour-intensive and time consuming.81
Therefore, a tool that could quickly detect the cancer cells at the resection margins, could guide the excision towards an adequate surgical margin and accelerate the surgical procedure.21,82
Melanoma.
Melanoma is the most commonly fatal form of skin cancer with a worldwide incidence of 232
130 and mortality rate as high as 55
488.1 Melanoma is preferably diagnosed at an early stage because of its metastatic disease. The vertical depth of growth in a primary melanoma, the Breslow thickness, is significantly correlated with metastatic propensity and thus prognosis. Melanoma with <1 mm thickness can be treated surgically with a high cure rate without reducing life expectancy (5-year survival is approximately 97%).83,84 Once the melanoma is diagnosed at an invasive stage, the survival rate decreases enormously to approximately 40% for melanomas with a Breslow thickness >4 mm.83,85 Patients with thick melanomas and signs of distant metastasis are submitted to surgery and/or immunotherapy, which places a high burden on the patient's quality of life. Moreover, the effect of current treatments on patient survival is very limited.
Even with the use of a dermoscope by an experienced clinician, the sensitivity in diagnosing melanoma is between only 68% and 96% and it is very much depending on the clinician's experience.9,86,87 Despite the large number of excised benign pigmented lesions (false positive suspicious lesions), 30% of early stage melanomas is still missed at the first clinical presentation and thereby present a risk to progress to a metastatic stage.88 Because of the severe consequences when a melanoma is missed, many suspicious pigmented skin lesions are surgically removed. The clinical positive predictive values are as low as 7% to 23% for melanoma diagnosis by dermatologists in a specialized pigmented lesion clinic.9,89 Therefore, a rapid, objective, real-time tool for clinical diagnosis could reduce the number of unnecessary excisions, guarantee early detection of melanoma, and, at the same time, could be used for lesions surveillance.
Raman applications for early diagnosis/biopsy guidance.
Investigations of Raman spectroscopy for skin cancer focus primarily on (early) detection of skin cancer and discrimination of benign from malignant and pre-malignant skin tumours.2,18,79,90–95
In 2008, Raman experiments were performed in vivo by Zhao et al. on 289 patients using a single fibre Raman probe; Raman spectra were measured from 9 different types of lesions, including BCC and SCC. The authors report a sensitivity of 91% and a specificity of 75% in differentiating malignant lesions from benign lesions. Malignant melanoma could be distinguished from pigmented benign lesions with reported sensitivity of 97% and specificity of 78%.96
In the same year, Lieber et al. used a portable Raman system with handheld probe for non-melanoma skin cancer diagnosis.97 Lieber et al. performed an in vivo study on 19 patients. Using the same handheld probe, Lieber et al. report classification of the BCC, SCC, inflamed scar tissues and normal tissues with sensitivity of 100% and specificity of 91%. The reported overall classification accuracy was 95%.98
In 2012, Lui et al. measured 518 in vivo skin lesions from 453 patients. Malignant and pre-malignant lesions could be separated from benign skin lesions using a single fibre Raman probe with a reported sensitivity of 90% and a specificity of 64%. Benign pigmented lesions could be distinguished from melanoma with reported sensitivity of 90% and specificity of 68%. Melanomas could be separated from seborrheic keratosis with a reported sensitivity of 90% and specificity of 68%.90 The specificities were 15%, 17% and 25% for a sensitivity of 99%, when three distinct discrimination tasks were investigated: melanoma vs. pigmented benign lesions, all cancers vs. benign lesions and melanoma vs. seborrheic keratosis, respectively.
In 2012, Silveira et al. reported an in vivo study using a Raman fibre probe in which they demonstrated differentiation of BCC from normal skin with an accuracy of 85%.99
Lim et al. determined the diagnostic capability of a multimodal spectral diagnosis for in vivo non-invasive disease diagnosis of melanoma and non-melanoma skin cancers. Measurements were performed with a custom-built clinical system that combines three fibre optic-based optical spectroscopy modalities: diffuse optical spectroscopy, laser-induced fluorescence spectroscopy, and Raman spectroscopy. Raman, fluorescence and reflectance spectra were acquired from 137 lesions in 76 patients. From the measured set, the number of lesions considered for the study were 100 (12 melanomas, 19 BCC, 38 SCC, 14 actinic keratosis and 17 non-melanoma pigmented lesions). When combining the three modalities, they reported a sensitivity of 100% and specificity of 100% for classifying melanoma vs. non-melanoma pigmented lesions. BCC and SCC vs. actinic keratosis were classified with a sensitivity of 95% and specificity of 71% also when combining the three modalities.100
In 2015, using a multi-fibre Raman probe, Schleusener et al. performed in vivo measurements on 104 subjects with lesions clinically suspected of being skin cancer (36 melanomas, 39 BCC and 29 SCC). Additionally, 67 measurements on benign pigmented nevi from 33 subjects were also included. Sensitivity and specificity for discriminating histopathologically confirmed melanoma from lesions clinically suspected of being melanoma (not histopathologically confirmed) were 52% and 67%, respectively. For discriminating histopathologically confirmed BCC from lesions clinically suspected of being BCC (not histopathologically confirmed) the sensitivity was 54% and specificity was 48%. The results for discriminating histopathologically confirmed SCC from lesions clinically suspected of being SCC (not histopathologically confirmed) were sensitivity of 52% and specificity of 27%. Discriminating histopathologically confirmed malignant lesions from lesions clinically suspected of being skin cancer, but not histopathologically confirmed, was not successful.93
Zhao et al., in 2015, performed an independent clinical test on a total of 645 lesions from 409 patients. The sensitivity and specificity for discriminating skin cancers and pre-cancers (including melanoma, BCC, SCC, and actinic keratoses) from benign skin disorders were 99% and 24%, respectively. This study provided an independent confirmation of in vivo skin cancer diagnosis by Raman spectroscopy.92
Santos et al. measured 82 freshly excised melanocytic lesions suspected of melanoma.89 The measurements were performed using an in-house built Raman micro spectrometer optimized for measurements on pigmented skin lesions.101 All 24 melanomas were correctly identified with a specificity of 45%. The authors indicated that Raman spectroscopy might lead to a 3-fold reduction of the ratio of unnecessary skin excisions and that their results should encourage the use of Raman spectroscopy in vivo systems by general practitioners and non-experienced dermatologists to discriminate melanoma from suspicious lesions and reduce unnecessary skin excisions.89
In 2017, Bratchenko et al. tested a combination of Raman spectroscopy with auto-fluorescence for melanoma and BCC diagnosis. They performed ex vivo measurements on 39 melanomas, 40 BCC's and respective healthy skin within the excised samples. The authors show that the combination of Raman spectroscopy with auto-fluorescence has an accuracy of 97.3% in discriminating BCC's from melanoma, whereas the determined accuracy for each modality separately is 79%.102
Raman applications for surgery guidance.
Kong et al. combined auto-fluorescence with Raman spectroscopy for intraoperative detection of BCC in skin. This approach was developed to reduce the measuring time of whole tissue sections during the Mohs surgery procedure. First, the auto-fluorescence images, which were taken at excitation wavelengths of 377 nm and 292 nm (corresponding to collagen and tryptophan excitation) were segmented. In this way, the normal dermis, which is dominated by collagen, was discarded and only the suspicious segments were sampled by Raman spectroscopy. Based on this approach, Kong et al., demonstrated that BCC could be automatically detected with a sensitivity of 95% and a specificity of 94%.94
Lung cancer
Clinical need.
Lung cancer is one of the leading causes of cancer-related deaths in the world, with an incidence of 1
824
701 and mortality rate of 1
589
925. Reasons for the high mortality rate are the fact that patients tend to be diagnosed at an advanced stage and a lack of effective treatments. Part of the diagnostic process is white light bronchoscopy (whether or not combined with auto-fluorescence) combined with tissue biopsy for definitive pathology. A problem with this technique is that it suffers from either low sensitivity or specificity103 and it is mainly accessible for centrally located lesions. Moreover, the representativeness and quality of the transbronchial and endobronchial biopsies is difficult to assess during the procedure.104
Cytologic evaluation also plays an important role in the initial evaluation and diagnosis of patients with lung cancer, especially in the evaluation of suspicious lymph nodes.105 Sampling errors and numerous mimics are the main pitfalls of the technique.105 An objective optical tool that could be adapted to bronchoscopy or incorporated in a biopsy needle would be beneficial for biopsy guidance and early diagnosis of lung cancer.
In early stage disease, surgical management is the treatment of choice. Several studies showed better 5-year survival rate for lobectomy (complete removal of the pulmonary lobe where the tumour is situated) than for sublobar resection, where less than an entire pulmonary lobe is removed.106–108 The sublobar resection procedure has been adopted in a number of centres. This procedure has been used for diagnosis and is considered sufficient for early stage disease and for non-cancerous lesions. Technical limitations that preclude negative surgical margins are a more challenging issue for sublobar resection than conventional lobectomy.108 Ideally, complete tumour resection with resection margins of ≥2 cm by sublobar resection would be favourable, maintaining lung volume and function108 while reducing the risk of local recurrence. Intraoperative frozen section has traditionally been the sole modality for achieving negative resection margins. However, in some cases, frozen sections are not representative enough to predict the final pathology. Thus, the histopathological evaluation of the frozen section is difficult, especially between non-invasive stage of the disease and minimally invasive stage.109 The difficulty to predict the predominant pattern based on frozen section has been reported to be due mostly to sampling errors.110 Moreover, the intraoperative assessment of margins is challenging owing to an underestimation of the margin distance in lung tissue. If the tumour is peripheral, it is easier to guarantee a large resection margin. However, when the tumour is centrally located it is more challenging to assure that adequate resection margins are achieved. Improvement of intraoperative assessment of resection margins is essential to amend sublobar surgery and reduce the local recurrence rate.
Raman applications for early diagnosis/biopsy guidance.
The first study that demonstrated the technical feasibility of measuring in vivo lung lesions using Raman spectroscopy was conducted in 2008 by Short et al.111 A multi-fibre Raman probe was implemented to complement white light and auto-fluorescence bronchoscopy for in vivo detection of lung cancer. Preliminary research on 26 patients demonstrated that the combination of Raman spectroscopy with white light bronchoscopy and auto-fluorescence bronchoscopy can improve the diagnostic specificity of lung cancer (reducing the number of unnecessary biopsies). With these combined technologies, the sensitivity and specificity achieved were above 90% for detection of lung cancer and high grade dysplasia lesions. The authors stated that the use of Raman spectroscopy has potential for substantially reducing the number of false-positive biopsies associated with white light bronchoscopy and auto-fluorescence bronchoscopy.112
Recently, the same group used the bronchoscopic Raman spectroscopy in vivo in 80 patients (280 samples), using signal acquisition times of 1 s. The detection of high grade dysplasia and malignant lung lesions resulted in a reported sensitivity of 90% at a specificity of 65%.113
Oesophageal cancer
Clinical need.
Oesophageal cancer has a worldwide incidence rate of 455
784 and mortality rate of 400
169.114 There are two main types of oesophageal cancer: squamous cell carcinoma and adenocarcinoma. Diagnosis is established with endoscopic guided biopsy. Oesophageal adenocarcinoma develops in sequential stages of change in the mucosa. Firstly, there is a change from a normal squamous epithelium to a columnar cell epithelium (i.e. intestinal metaplasia), known as “Barrett's oesophagus”. The “Barrett's oesophagus” is considered a pre-malignant stage because it is associated with 100 times higher risk of developing oesophageal cancer.115 Endoscopic identification of this pre-cancerous stage may enable early, minimally invasive therapeutic intervention (i.e. endomucosal resection). The greater the number of biopsies taken from the border of the lesion (at least six), the higher the diagnostic accuracy.116,117 However, the increase in the number of biopsies performed may cause an increased risk of complications, such as gastrointestinal bleeding. Furthermore, still 11.3
% of upper gastrointestinal tract cancers are missed with this technique.118 Therefore, an objective, real-time tool for biopsy guidance could reduce the number of unnecessary biopsies and improve the diagnostic accuracy.
Surgical resection is the treatment of choice in early staged oesophageal cancer. The optimal extent of oesophageal resections is still controversial, but completeness of surgical resection is an important determinant for a better outcome. There are recommendations for clearance of tumour from proximal and distal margins, but this may vary depending upon the type of tumour.119,120 The presence of tumour at a distance ≤1 mm of the circumferential resection appear to be a significant cause of local tumour recurrence and decreased survival.121,122 An intraoperative assessment of the resection margins of oesophageal excisions is essential to reduce the local recurrence rate and increase the survival rate.
Raman applications for early diagnosis/biopsy guidance.
Teh et al. studied 21 dysplastic gastric lesions and 44 normal samples from 44 patients using in vivo multi-fibre Raman probe system. A sensitivity of 95% and specificity of 91%, between dysplastic and healthy stomach tissue was found.123
Bergholt et al. measured 924 Raman spectra in vivo from normal tissue and 111 from benign ulcers from 71 patients with an endoscopic multi-fibre Raman probe system. Malignant ulcers were correctly classified with a sensitivity of 82.1% and a specificity of 95.3%.124
An endoscopic multi-fibre Raman probe system for in vivo and on-line diagnosis of gastric cancer was developed by the group of Huang.125 Using this system, they obtained a total of 2748 in vivo gastric tissue spectra from 305 patients: 2465 diagnosed as normal and 283 as cancer. The reported diagnostic accuracy for gastric cancer was 85.6% (sensitivity of 80.5% and specificity of 86.2%). Gastric cancer detection algorithms were further applied on 10 patients undergoing gastroscopy (Fig. 1). The authors report a predictive accuracy of 80.0% (sensitivity of 90.0% and specificity of 73.3%).125
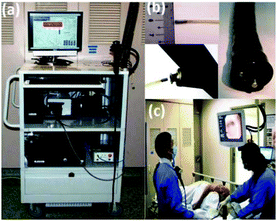 |
| Fig. 1 (a) Photograph of Raman endoscopy system in clinic; (b) insertion of the 1.8 mm Raman endoscopic probe into the working channel of an endoscope during gastroscopy; and (c) routine Raman endoscopy procedure in clinic.125 From Duraipandian et al.125 Reprinted with permission from SPIE. | |
In 2014, Bergholt et al. performed an in vivo study for diagnosis of gastric dysplasia in Barrett's oesophagus. A total of 450 patients underwent endoscopy in the upper gastrointestinal tract, Raman measurements were performed, biopsies were taken and submitted for histopathological evaluation. High grade dysplasia in Barrett's oesophagus, non-dysplastic Barrett's oesophagus and normal columnar lined epithelium could be discriminated with a reported sensitivity of 87% and a specificity of 84.7%.126
Wang et al. demonstrated that, using the in vivo endoscopic multi-fibre Raman probe system and acquisition times of 0.1–0.5 s oesophageal squamous cell carcinoma can be detected with sensitivity of 97% and specificity of 97%, based on the results from 48 patients.127
Ishigaki et al. used a micro Raman probe system to examine ex vivo early-stage (stages 0 and I) oesophageal cancer samples from 15 patients. They demonstrated a sensitivity of 81% and a specificity of 94% using leave-one-out cross validation.128
Using a Raman endoscopic multi-fibre probe during routine endoscopy examination, Lin et al. demonstrated in vivo that simultaneous use of the fingerprint and high-wavenumber part of the Raman spectrum can improve early diagnosis of gastric pre-cancers (sensitivity of 93% and specificity of 94%) in an independent dataset. A total of 48 patients were included. From this dataset, 80% of the spectra were used for training and 20% for testing. Using exclusively either the fingerprint or the high-wavenumber part, the area under the ROC curve was 0.972 and 0.928, respectively. When combining fingerprint spectral range and high-wavenumber range, the area under the ROC curve was 0.995.129
Head and neck cancer
Clinical need.
Head and neck cancer includes cancer of the oral cavity, nasopharynx, oropharynx, hypopharynx, and the larynx. Head and neck cancer has an overall incidence rate of 686
328 and a mortality rate of 375
665.114 The most frequent type of cancer in head and neck region is oral cavity squamous cell carcinoma (OCSCC). When a patient is suspected for a tumour in the head and neck region, all subsites will be examined to screen for a synchronous second primary tumour. Biopsies are taken for tissue diagnosis and for staging purposes.
Surgery is the mainstay of treatment for most oral cancers and for early stages in the other subsite. Advanced disease usually requires multimodality treatment consisting of surgery, radiation and/or chemo/biotherapy. Very recently immunotherapy was added as a promising option. An acceptable balance between remaining function and physical appearance on one side and clear margins on the other, is the main goal of surgery. Inadequate resection margins influence negatively local control and prognosis.130,131 In head and neck cancer, clear resection margins impact on disease control and survival.132,133 Achieving adequate resection margins is challenging. The lack of reliable intraoperative guidance and the proximity of tumours to vital structures are common causes of inadequate tumour resection. Recent studies show poor surgical success rates with inadequate tumour resection in oral cavity cancer surgery from 30% up to 85%.23,33,134 Intraoperative assessment by means of frozen-section is the standard procedure. However, these can only be used for soft tissue and not for bone. Additionally, only a small proportion of the resection margins can be investigated by this procedure and the selection of suspicious tissue depends on the surgeon's and/or the pathologist's experience. These factors also contribute to a high recurrence rate of oral cancer.21,135 The two primary clinical needs are early diagnosis of OCSCC and intraoperative assessment of the complete resection margins, not only for soft tissue but also for bone margins.
Raman applications for early diagnosis/biopsy guidance.
In 2014, Krishna et al. created an in vivo multi-fibre Raman probe system and measured a total of 28 healthy volunteers and 171 patients with oral lesions. Spectra were annotated, based on histology or by clinical assessment, as oral squamous cell carcinoma (OSCC), oral submucosa fibrosis (OSMF), oral leukoplakia (OLK) and normal mucosa. Each group (OSCC, OSMF, OLK and normal) was correctly classified, when applying the developed diagnostic algorithm, in 89%, 85%, 82% and 85% of the cases, respectively.136
In 2015, Guze et al. conducted an in vivo pilot study with a Raman probe and measured oral diseases from 18 patients. Benign and malignant oral lesions were classified correctly with sensitivity of 100% and specificity of 77% using a multi-fibre Raman probe. Although, a larger study group was preferable, the results indicate that Raman spectroscopy is a promising diagnostic tool.137
Raman applications for surgery guidance.
In 2015, the feasibility of identifying oral cancer tumour based on water concentration was demonstrated ex vivo, using high-wavenumber Raman spectra obtained from freshly excised resection specimens from oral cavity.135
Barroso et al. investigated how the water concentration changes across the border between tumour and the healthy surrounding tissue on ex vivo specimens from patients that underwent surgery for oral cavity squamous cell carcinoma. Measurements were performed on 20 patients using a confocal Raman microscope system. The results revealed consistent changes in the water concentration across the tumour border. Over distances of 4 to 6 mm across the tumour border, water concentrations changed from 76% ± 8% in the tumour to 54% ± 24% in the healthy surrounding tissue.138
Brain cancer
Clinical need.
The estimated incidence of brain cancer in the developed world is 7.1/100
000 with a high mortality of 5.3/100
000.1 Neuroradiology is usually sufficient as the initial diagnostic modality for brain cancer. In some cases, stereotactic biopsy is needed to decide for primary resection versus chemo-radiation. For accurate histopathological diagnosis multiple tissue samples are obtained.139 Brain biopsy may be complicated by sampling error and cerebral haemorrhage.140,141 Therefore a rapid and objective tool is needed for optimal biopsy guidance to provide one representative brain tumour tissue sample, thus minimizing the risk of cerebral haemorrhage.
Treatment of choice for most primary brain cancer is surgery.17 In low-grade gliomas the extent of tumour tissue resection is associated with improved patient outcome.141–143 Three-dimensional stereotactic navigation, 5-ALA-fluorescence and intraoperative magnetic resonance imaging (MRI) are currently used to achieve optimal surgical results.141,144 Unfortunately, distinguishing cancer from healthy tissue during surgery still is a challenge.140,145 Generally, postoperative MRI demonstrates residual tumour in 69.6% of the cases.145 The accuracy of navigation is influenced by the intent to preserve vital brain structures, inter-observer disagreement on the tumour transformation zone, and brain oedema.146 In the current practice, adoption of navigation techniques is limited owing to high costs and hampered by low evidence of survival benefit.147 An optical, intra-operative tool to guide the surgeon towards tumour tissue in stereotactic biopsy and to identify adequate resections while preserving function brain structures with resection may improve patient outcome.
Raman applications for surgery guidance.
Jermyn et al. in Montreal have developed a Raman system with a hand-held probe for intraoperative use during brain tumour resection. In an in vivo study, spectra were obtained from 17 patients with 0.2 s acquisition time (Fig. 2). Patients with grade 2 to 4 gliomas were included (66 normal spectra and 92 cancer spectra). They reported a sensitivity of 93% and a specificity of 91% in distinguishing normal brain tissue from dense cancer and normal brain invaded by cancer cells.148
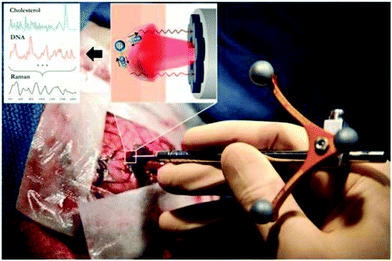 |
| Fig. 2 The handheld contact fiber-optic probe for Raman spectroscopy. The probe (Emvision, LLC) was used to interrogate brain tissue during surgery. A schematic diagram illustrated the excitation of different molecular species, such as cholesterol and DNA, to produce Raman spectra of cancer versus normal brain tissue.52 From Jermyn et al.52 reprinted with permission from the authors and AAAS. | |
Colorectal cancer
Raman applications for early diagnosis/biopsy guidance.
In 2008, Widjaja et al. measured 105 colon specimens ex vivo with an in-house built Raman probe.157 From the 105 colon specimens 41 were normal, 18 were hyperplastic polyps and 46 were adenocarcinomas. In this study, the diagnostic accuracy was 98%.157
Short et al. conducted a study using Raman spectroscopy on ex vivo colon tissue from 18 patients, measuring both the fingerprint and high-wavenumber spectral regions. The results indicated that, using the high-wavenumber region, the non-malignant and the malignant groups could be classified correctly with a specificity of 89%. The authors referred that high-wavenumber region could be used in vivo to improve the identification of neoplastic lesions.158
Bergholt et al. tested the same approach in vivo (combined fingerprint and high-wavenumber spectral regions) on adenomatous polyps measured from 50 patients using an endoscopic multi-fibre Raman probe. Adenomatous polyps were separated from hyperplastic polyps with a sensitivity of 91% and specificity of 83%. The authors demonstrated that the analysis based on the combination of fingerprint and high-wavenumber spectral regions is superior to considering either region alone.12
Cervical cancer
Clinical need.
Cervical cancer has a worldwide yearly incidence of 528
000 women and mortality of 266
000,1 in recent years incidence and mortality rates have decreased because of the emergence of screening.159 The primary method of screening is by cervical cytology with the Papanicolaou test (PAP). This has a high specificity (95–98%) but a low sensitivity (<50%).160 This sensitivity of screening is increased by testing for Human Papilloma Virus (HPV) in the cervical swab.161 Final diagnosis of cervical cancer is based on histopathology. For this colposcopy guided biopsy is recommended, with a sensitivity of 92% and specificity of 67%.73 In low resource settings visual inspection of the cervix with biopsies is used with a specificity and sensitivity of 88–92% and 10–84%.162,163 Treatment of cervical cancer is based on stage. Small tumours will be treated by surgery, and higher stages with chemoradiation. It is not to be expected that Raman spectroscopy has any value in the diagnostic or treatment of cervical cancer since the diagnostic accuracy of colposcopy guided biopsies will not be improved and precision treatment is not applicable for cervical cancer.
Raman applications for early diagnosis/biopsy guidance.
Mo et al. measured Raman spectra from 46 patients in vivo with high-wavenumber Raman spectroscopy, to investigate cervical pre-cancer diagnosis. The Raman instrument used a ball-lens fibre-optic Raman probe. Cervical dysplasia could be distinguished from normal tissue with a reported sensitivity of 94% and specificity of 98%.164
Kanter et al. performed a clinical study on a total of 43 patients that underwent colposcopy-guided biopsy to investigate dysplasia. Using a portable Raman system with a fibre-optic probe spectra were acquired of low grade squamous intraepithelial lesions, high grade squamous intraepithelial lesions, metaplastic lesions and normal tissue. The classification accuracy for discriminating diseased from normal tissue was 88%, the sensitivity was 86% and the specificity was 97%.165 The same group reported, in 2011, a sensitivity of 70% and a specificity of 83% for cervical pre-cancer diagnosis. In this study a total of 29 patients were included. Measurements were done in vivo using fibre-optic Raman probe with a ball-lens.166 To improve diagnostic accuracy, Duraipandian et al. combined high-wavenumber and fingerprint Raman spectroscopy for cervical and pre-neoplasia detection. The obtained sensitivity and specificity were 85% and 81.7%, respectively.167
Rubina et al. performed ex vivo Raman measurements on 49 abnormal cervical specimens and 45 negative control cytology samples using a fibre-optic Raman microprobe system. Abnormal lesions were classified correctly in 84.5% of the cases. The authors suggested that presence of blood in abnormal specimens was a major cause of discrimination.168,169
Vulval cancer
Clinical need.
Vulval cancer has a worldwide incidence of 1300 women and mortality of 450 women per year.170 This malignancy has a high curability in early-stage disease, but a poor prognosis for advanced-stage disease and recurrent disease. Diagnosis is based on histopathology of a biopsy of the vulva at the peripheral edge of the lesion,171 whereby underlying stroma is included and possible necrotic tissue avoided.172In vivo diagnosis and biopsy guidance can be of great value for accurate diagnosis. Surgery is the main choice of treatment. In recent years surgical management has changed, were large radical and disfiguring resections make place for conservative surgery.172 The multifocality of this type of cancer, the presence of dysplasia and/or small resection margins result inlocal recurrence rates of 23–50%.173,174 Surgical management of vulval carcinoma aims to achieve a fine balance between adequate resection margins and preservation of sexual and urinary function.172,175 An intra-operative tool for surgery guidance is therefore needed.
Raman applications for early diagnosis/biopsy guidance.
During the literature search we have noticed that Raman applications have not been greatly developed for vulval cancer. The most recent study, was developed by Frost et al.176 They have evaluated the diagnostic performance of Raman Spectroscopy for differentiating a pre-malignant vulval lesion (lichen sclerosus, LS) from other inflammatory vulval conditions in fresh vulval biopsies from 27 women. Biopsies resulted in circular discs o skin 3–4 mm in diameter (full thickness of the epidermis with underlying dermal tissue). Spectra were measured with a Raman micro-spectrometer (modified Renishaw system 1000 dispersion), which was coupled to a diode laser of 830 nm excitation wavelength and had a spot size of 15 × 17 μm. A classification model was created using principal components and multivariate linear discriminant analysis. Leave one sample out cross validation was used to validate the model. The model was able to correctly differentiate LS from other inflammatory vulval conditions with a sensitivity of 91% and specificity of 80%. The authors suggested that the study demonstrates that Raman spectroscopy has potential for in vivo non-invasive diagnosis of vulval skin conditions and when applied in the clinical setting may reduce the need for invasive tissue biopsy.177
Bladder cancer
Clinical need.
The global incidence rate for bladder cancer is 430
000 and mortality is 165
000.1 The standard for diagnosis is cystoscopy, with biopsies of suspicious lesions, and transurethral resection (TUR) to confirm the diagnosis.178 Unfortunately, small papillary bladder tumours and flat urothelial carcinoma-in situ (CIS) can easily be overlooked.179 This contributes to increased residual rates and a recurrence rate of 50% within 18 months. TUR is the standard of treatment for small (Ta) bladder tumours. Incomplete resection results in a second operation and/or increased recurrence rate, resulting in poor long-term prognosis.180–183 Thereby, high risk tumours grow fast with a mortality rate of 50%, despite treatment. Urinary markers are emerging but not able to outperform cystoscopy. These findings suggest that in vivo biopsy, biopsy guidance and surgery guidance is needed for early detection, accurate diagnosis and adequate resections, to improve patient outcome.
Raman applications for early diagnosis/biopsy guidance.
The first in vivo Raman probe that was used in bladder was an Emvision® probe with a filtered six-around-one fibre configuration. The excitation wavelength was 785 nm. The probe used had a large measurement volume (∼1 cm3) and did not have any lenses. As a result, the probe collected Raman spectra not only from the upper urothelium surface of the bladder (where transitional cell carcinoma has its origin) but also from deeper layers. During the procedure of transurethral resection of bladder tumours (TURBT), spectra were collected from suspicious and non-suspicious locations (collection times of 1 to 5 s). Bladder cancer could be distinguished from normal bladder with a reported sensitivity of 85% and a specificity of 79%. Even though the results show the possibility of discerning normal from malignant bladder tissue using Raman spectroscopy, the authors suggest that using a Raman probe that collects shallower signal from the urothelium surface of the bladder would be ideal for early diagnosis. Also the combination of auto-fluorescence with Raman spectroscopy could potentially improve the diagnostic capability, by using the sensitive auto-fluorescence modality to indicate suspicious regions for “optical biopsy” by the highly specific Raman spectroscopy modality.184,185
In 2012, a confocal Raman probe was designed by Barman et al. and it was used ex vivo for bladder cancer diagnosis in 14 patients. The confocal probe had depth of field of approximately 280 μm. The result of the confocal system had a significantly higher specificity with respect to large-volume Raman spectral data, with a sensitivity that was comparable to the large-volume Raman system.60,177
Prostate cancer
Clinical need.
Prostate cancer is among the most common cancers in men worldwide, with an incidence of 1
094
916 and mortality rate of 307
481.1 The diagnosis of prostate cancer is often made through transrectal ultrasound guided prostatic biopsy.186 Ten to twelve core biopsies are recommended, with >12 biopsies not being significantly more conclusive.187,188 While the majority of prostate cancer patients are treated by radical prostatectomy or radiation therapy, an increasing number of men are actively surveyed for their disease without immediate treatment. In a significant number of men, radical prostatectomy is complicated by urinary and/or erectile dysfunctions, which is related to eradication of the neurovascular bundles (NVB) localized in the dorsolateral peri-prostatic fat tissue. Operative removal of the prostate leaving the NBV intact can reduce post-operative complications. Decision-making on nerve-sparing surgery can be optimized by intra-operative assessment of the prostatic surgical margins. In practise, the urologist can only remove the prostate initially leaving the NVB in situ. In case intra-operative assessment of the prostatic surgical margin is negative, the NVB will remain intact; in case the margin is positive the urologist can remove the NVB at a second term. Although intra-operative frozen-section assessment is able to increase nerve-sparing surgery from 81% to 97%, it is labour-intensive requiring an optimized work-flow for the fast throughput of a large number of frozen sections.189 Intra-operative assessment of surgical margins using Raman spectroscopy might be a good alternative for extensive pathologic frozen sections and could be implemented at large scale in operation theatres or departments of pathology.
Raman spectroscopy applications.
Spontaneous Raman spectroscopy has not been explored yet in vivo and/or ex vivo using human prostate cancer material. Raman-based studies were developed in the last years in vitro, using cell cultures and, more recently, ex vivo using peripheral nerves of rats.177,190,191
Instrumentation
When developing instrumentation for in vivo and ex vivo applications, the main technical problems are: speed of measurement, adaptation in clinical workflow, interference of background from tissue auto-fluorescence and high instrumentation costs.
In the last years we consider that the major technological advances in Raman spectroscopic systems for clinical applications have been in: (1) detector technology, (2) fibre-optic probe design, (3) combination with other techniques, and (4) new laser opportunities for low-cost systems.
InGaAs detectors for short-wave infrared Raman spectroscopy
Until now the detection of Raman signals in the short-wave infrared (SWIR) region (>1100 nm) was constrained by limitations of the state-of-the-art detector technology for visible to NIR excitation (CCDs). An alternative in this spectral range is the indium–gallium–arsenide (InGaAs) detector, which enables detection at wavelengths well above 1100 nm. Several companies have recently moved towards the SWIR range, and have introduced InGaAs-based Raman devices (e.g. Andor™, Anton Paar™, Horiba™, B&W Tek™). Xenics™ introduced an InGaAs camera that has a read-out noise at comparable levels as a CCD detector, and that consequently can achieve shot-noise limited Raman spectra in clinically acceptable integration times.101 This development in detector technology enabled the use of longer excitation wavelengths for clinical applications on tissues with would otherwise suffer from strong laser-induced fluorescence of fluorophore-containing tissues. Santos et al. could measure melanocytic skin lesions without overwhelming tissue auto-fluorescence interference using a SWIR Raman system, with NIR 967 nm laser and an extreme low-noise InGaAs detector (Fig. 3).89
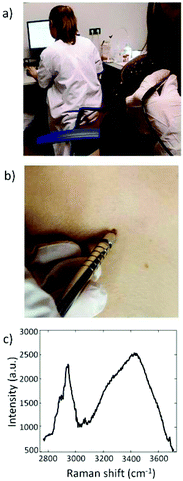 |
| Fig. 3 Implementation of SWIR Raman system in the dermatology out-patient clinic of Leiden University Medical Center for in vivo measurements (a). Photograph of a pigmented skin lesion (b). This was measured using a 976 nm laser excitation, the SWIR Raman instrument and a single fiber probe. The corresponding Raman spectrum measured in vivo is shown in (c). Exposure time: 30 s. | |
Also Patil et al. and Pence et al. have reported dispersive Raman spectroscopy of tissues with strong auto-fluorescence (liver and kidney human tissues) using 1064 nm excitation in combination with an InGaAs detector array.192,193 Laser-induced tissue fluorescence was significantly reduced compared to excitation with lasers in the visible wavelength range.
Fibre-optic probes
Fibre probes for early diagnosis.
Fibre-optic probes have enabled the use of Raman spectroscopy for in vivo clinical applications. The integration of Raman spectroscopy with other diagnostic techniques has been a major step in the development towards improved efficiency in biopsy guidance and early cancer diagnosis. Fibre probes that could be inserted into endoscopic channels enabled in vivo measurements in hollow organs or surface assessment of solid organs: oral cavity,136,137 lung,111,113 upper gastroinstestinal tract,123–127,129 colorectal,12,158 bladder,185 and cervical cancers.164–167,194 Also, handheld fibre-based systems have been used for in vivo assessment of skin cancer,88,90,91,94–96 and of brain cancers.148,195
In the last years numerous Raman probe designs have been developed, optimized and tested for clinical purposes. There has been an increased effort to tailor Raman probes to clinical needs and constraints defined by the specific clinical targets, specific pathophysiology of diseases, and specific anatomies of the region of interest (e.g. accessibility of the tissue, sample size, sampling depth).
Stevens et al. and Wang et al. have reviewed Raman fibre-optic probe designs and construction concerns, considering different oncological applications and respective clinical needs.18,196
Depth-selective fibre-optic Raman probes have been investigated for use in epithelial tissue associated with dysplasia.197 Wang et al. developed a confocal fibre-optic Raman probe coupled with a ball lens to enhance in vivo Raman measurements from gastric pre-malignant epithelial tissue during endoscopy.197,198 With a bevelled fibre-optic probe coupled with a ball lens, the authors could limit their collection depth to 300 μm. Since gastric dysplasia initially evolves in the epithelial tissue layer (∼300 μm), it is especially relevant for selective interrogation of the gastric epithelium.
Further work from the same group, featured a fully automated real-time Raman spectral diagnostics framework integrated with a multimodal image-guided Raman technique that enabled real-time in vivo cancer detection at routine endoscopy procedure, as shown in Fig. 1.199 The same fingerprint/high-wavenumber fibre-optic Raman endoscopic system was developed further by Bergholt et al. for routine clinical colonoscopy diagnosis, making use of a foot pedal control switch and an auditory feedback to the gastroenterologist.199
Using a similar probe in a hand-held confocal design, Duraipandian et al. measured in vivo fingerprint and high-wavenumber Raman spectra during colposcopy.167
Agenant et al. used a novel clinical Raman probe for sampling superficial tissue to improve in vivo diagnosis of (superficial) urothelial carcinoma. This probe had a measurement depth of 0–200 μm, which corresponds to the average urothelium depth. The probe is comprehended by 7 collection fibres, 1 excitation fibre and two component front lens.200
Fibre-optic probes for guided surgery.
In the field of brain cancer, Jermyn et al. preformed in vivo Raman measurements during human brain cancer surgery for real-time identification of invasive cancer, using a handheld contact Raman spectroscopy probe.52,195 Each measurement covered a 0.5 mm diameter tissue area with a depth sampling up to ∼1 mm in 0.2 s. During the tumour resection, Raman signals were measured in the surgical cavity. The probe is comprehended by filters that were placed directly at the tip of the optical fibres. The spectra acquired with the handheld Raman probe (Fig. 2) do indicate that there are still some challenges in developing probes and methods for correcting for spectral distortions mainly caused by the filter characteristics of the probes and subtraction of fluorescence signal backgrounds.201
A Raman system comprehended by a single fibre needle probe is currently being tested ex vivo by Head and Neck surgeons and pathologists from Erasmus University Medical Center Rotterdam, The Netherlands. This probe aims intraoperative inspection of the entire resection margin of specimens from patients that underwent surgery for oral cavity SCC (Fig. 4).
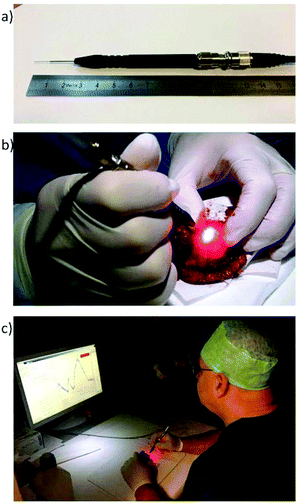 |
| Fig. 4 Single fibre needle probe for intraoperative assessment of the oral cavity SCC resection margins, developed by Artphotonics GmbH in collaboration with RiverD International B. V. and Erasmus University Medical Center, Rotterdam. (a) Close up of the single fibre needle probe. (b–c) Testing the single fibre needle probe for inspection of the freshly excised oral cavity specimens. | |
Combined Raman systems for guided surgery
In the field of dermatology, Kong et al. developed a scanning device that combines auto-fluorescence imaging and Raman spectroscopy to guide Mohs micrographic surgery in the excision/treatment of high-risk skin basal cell carcinoma.94 Although Mohs micrographic surgery provides the highest cure rates, it is a time-consuming technique (1–3 hours) needing highly trained personnel. With the purpose of reducing the duration of scanning large areas of tissue, tissue auto-fluorescence imaging is used to determine the main spatial histological features of the sample, and this information is used to select and prioritize the sampling points for Raman spectroscopy (Fig. 5).94,202
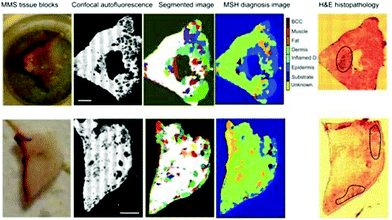 |
| Fig. 5 Multimodal spectral histopathological diagnosis of BCC in unsectioned tissue blocks as received from surgery. H&E histopathology images for adjacent sections are included for comparison (scale bars: 2 mm).94 Reprinted with permission from the authors and PNAS. | |
New lasers
Recent developments in vertical-external-cavity surface-emitting laser (VECSEL) technology have brought their characteristics in the realm of Raman spectroscopy, with special interest for low-cost hand-held devices.203 VECSELs are semiconductor lasers based on a vertical-cavity surface-emitting geometry.
These types of lasers achieve a combination of high continuous-wave output power, due to a large gain area, and a low-divergence near-diffraction-limited beam, due to the transverse mode control of the extended cavity. The advantages of VECSELs over edge-emitting semiconductor lasers are circular output beam, easy array fabrication, small size, and low cost at mass production.56,203–205
Transferability
The Raman applications described above demonstrate potential of Raman spectroscopy to affect patient care. The major requirements for translation of a Raman equipment into clinical practice are: (1) well defined clinical need, (2) patient benefit proven and/or cost benefit, (3) equipment changes clinical practice, (4) demonstrated safety, reproducibility, robustness, and reliability (e.g. large clinical trials) and (5) regulatory approval. First, it is important to verify beforehand that the developed Raman application addresses an actual clinical need and that the application can provide results of clinical relevance. This does not mean that the Raman instrument needs to give perfect results, as long as it can bring about measurable and cost effective improvements in patient care. Moreover, it is required that the instrument is introduced in the clinical workflow and is used/tested by clinicians.
Second, it is essential to demonstrate the safety, reproducibility, robustness, and reliability of the developed application in large clinical trials, to the end-users. And finally, receive approval from regulatory agencies.
Many of these aspects are now increasingly being addressed, which is a good sign of progress in the field towards clinical translation. However, for this translation continued effort is needed by searching for close collaboration and input from the medical community.
Additionally, to gain the confidence of clinicians to the potential value of Raman-based applications, it is essential to expose the results of Raman technologies over or in line with existing medical devices.2,206 This should be done in large cohorts’ studies based on patient outcomes and in direct comparison with the current gold standards.
Multidisciplinary networks are now being developed, such as the International Society for Clinical Spectroscopy (ClirSpec), European Photonics Industry Consortium (EPIC) and Raman4Clinics (EU COST Action BM1401), which target especially these translational aspects.
ClirSpec is a society, comprehended by spectroscopists and clinicians, that facilitates and promotes the translation of spectroscopy into the clinical environment, for the general benefit of patients (clirspec.org).
Raman4Clinics (EU COST Action BM1401) is a platform for scientific communication, exchange, collaboration and for new research activities, combining the partners’ expertise in technology, component, system and methodology development and medical application (http://www.raman4clinics.eu).
EPIC is a network that promotes awareness of opportunities for the European photonic industry in healthcare (http://www.epic-assoc.com).
To provide an overview of the current status of the technology towards clinical translation, we classified all reviewed R&D results, based on the TRL.
Fig. 6 (2008–2017) shows the result from the technology readiness classification of the different Raman studies mentioned in the Raman applications section.
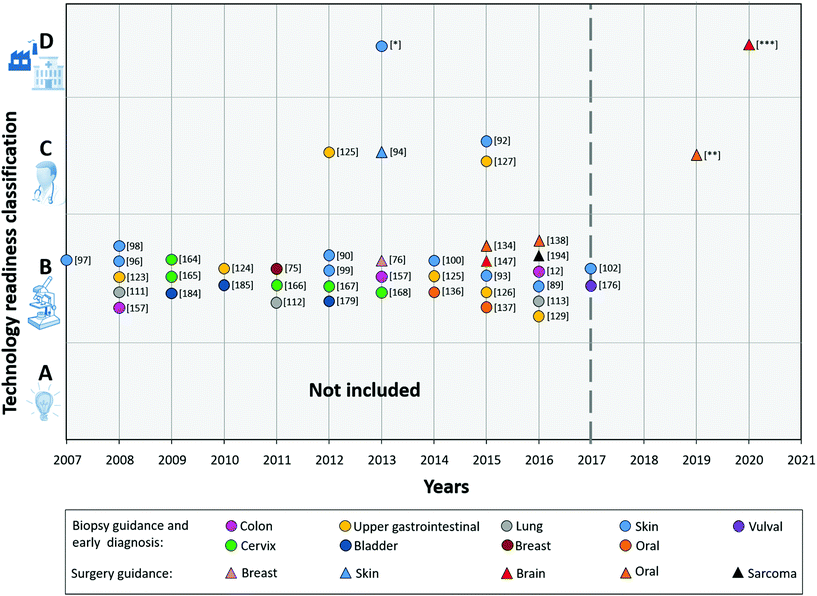 |
| Fig. 6 Illustration of the result from the technology readiness classification of the different Raman studies, mentioned in the Raman applications section. Verisante Technology Inc. is commercializing a device (Verisante Aura) for skin cancer detection [*]. In the graph is also plotted the prognosis (2017–2021) of further development/commercialization given by the companies RiverD International B.V. [**] and ODS Medical Inc. [***]. | |
From this figure, it is immediately clear that the majority of the developed applications appears to get stuck in category B (TRL 4).
These studies presented results of algorithms for (pre-) malignant tissue detection with data either collected in vivo or ex vivo with prototype Raman systems. However, validation of algorithms with an independent large dataset has not been proven yet. Some of the systems used in the analysed studies are already further towards actual clinical use, and were classified as C, such as Zhao et al., Wang et al., Kong et al., and Duraipandian et al.92,94,125,127
Development of commercial instruments for oncological applications
We realized that most of the studies do not report a fully commercial product (ready to be used in the clinic). We therefore contacted the companies that are collaborating directly with the research groups presented in the Raman applications section. We also investigated the progress/potential interest of the companies which are developing or have developed commercial Raman spectroscopic systems for oncological applications.
We received information from the following leading companies in Raman spectroscopy: Avantes B.V., B&W Tek, EmVision LLC, Invenio B.V., Horiba Jobin-Yvon Inc. (HORIBA Scientific), ODS Medical Inc., Renishaw, RiverD International B.V., Verisante Technology Inc., and WITec B.V. Based on the information received we have created a future perspective of clinical translation, which is presented in Fig. 6 (2017–2021).
Progress reported by companies.
B&W Tek has developed a Raman spectroscopic system, for the identification and qualification of cytostatics (in vitro). B&W Tek is a company that provides instruments looking for R&D partners.
Invenio Imaging Inc. is developing a stimulated Raman scattering microscope for histological examination of fresh tissue. The most recent publication was in Nature Biomedical Engineering, early 2017.207 The perspective of the company is to have the equipment ready to be commercialized later this year.
EmVision LLC does not manufacture or develop clinical systems, but the company is active in selling components, such as fibre-optic probes, to companies that are pursuing clinical systems.
HORIBA Scientific is an active member of Raman4Clinics European project and provides research and analytical Raman instruments for all kind of applications, including oncology.
ODS Medical Inc. is developing an in vivo Raman tool for surgical brain tumour resection.148,208–211 The company predicts that the system will be commercialized within approximately 3 years (in 2020) following approval by regulatory bodies, including FDA.
Renishaw has been actively developing a Raman system for ex vivo analysis of tissue cells and bio fluids for clinical use. The company is upgrading fibre-optic probe designs for clinical use during oncological surgery. This company has an ex vivo Raman spectroscopy platform (InVia series, Renishaw, Gloucestershire, U.K.) for a number of life-science and clinical applications including tissue pathology, neurobiology, stem cell research. Renishaw is currently developing a fast tissue imaging platform, which is based on the RA802 Pharmaceutical Analyser, for measuring uneven tissue surfaces with a minimum of sample preparation.212–214 The new fast tissue imaging platform should be available in the summer of 2017.
RiverD International B.V. collaborates with the University of Nottingham in the development of an auto-fluorescence/Raman system for Mohs’ micrographic surgery of basal cell carcinoma. Two prototype systems are currently being tested at the University of Nottingham, in UK, and at Erasmus MC University Medical Center Rotterdam, in the Netherlands. The systems are based on the concept described by the Nottingham group.94 RiverD International B.V. is developing a Raman tool for surgical guidance of Head and Neck tumour resection, which they predict to be ready for clinical trials in 2019. Currently, an ex vivo fibre-optic probe system is being developed for intraoperative inspection of resection margins of excised tissue. The company is also collaborating in an R&D project that aims to the development of a Raman spectroscopic device for use by dermatologists and general practitioners for objective assessment of pigmented skin lesions clinically suspected of melanoma.89,101
Verisante Technology Inc. is developing in vivo Raman technology for clinical oncology applications focused on: (1) in vivo non-invasive detection of skin cancers including melanoma and non-melanoma skin cancers; (2) endoscopy detection of cancers of internal organs such as lung cancers and gastro-intestinal cancers.90,111,113,215–217 Currently, Verisante Technology Inc. is commercializing a skin cancer detection device (Verisante Aura, see Fig. 7) in Canada, Europe and Australia. Aura systems are being in use in several clinics in Canada. In Fig. 6, Verisante Aura is identified by [*].
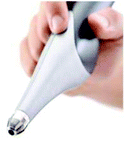 |
| Fig. 7 Verisante Aura system for skin cancer detection. Courtesy of Verisante Technology, Inc. (http://www.verisante.com/aura/medical_professional/). | |
WITec B.V. develops and commercializes confocal Raman microscope systems. The company is not actively involved in development of applications in the clinical oncology field. However, various users of the confocal Raman microscopes have applied their instruments in oncology-relevant research.218–221
Hurdles in translation reported by companies.
Some of the companies have indicated what hurdles they have encountered when translating Raman systems into the clinics. One important issue can be assuring that high-quality data can be acquired consistently and robustly under intraoperative conditions, with minimal disruption of the surgical workflow.210 It is also important to ensure that real-time high-accuracy tissue classification is provided to surgeons in an intuitive way and by simple metrics (user-friendliness).
Another referred problem is to find funding/investors that can help to bring the product into the market.
The fact that Raman spectroscopy is still a relatively unknown technique for most clinicians, was yet another hurdle mentioned. This can be partially explained by the fact that from all the scientific studies reviewed in this article only approximately 30% were published in medical oriented journals. Another explanation for Raman spectroscopy to be relatively unknown might be also partly due to this technique not being widely incorporated in the curriculum of clinicians or clinical researchers. To resolve this, more effort should be done towards publication of clinical Raman studies in medical oriented journals. Additionally, current academic leaders in the medical field, who have knowledge of clinical spectroscopy, should encourage the introduction of this subject in medical school curriculums alongside other medical imaging and diagnostic topics.
Discussion
In the last decade a strong effort has been made towards clinical implementation of Raman spectroscopy as an adjunct technique for early diagnosis, biopsy guidance and oncologic surgery guidance. The most recent studies aim to: (1) detect pre-malignant lesions,113,125,126,136,164,222 (2) detect cancer in less advanced stages,101,113,125,157,158 (3) reduce the number of unnecessary biopsies,91,96,112,113 and (4) guide surgery towards complete removal of the tumour.76,94,138,148,206 This means that Raman spectroscopy is used to detect (pre-)malignant lesions for guidance of clinical procedures. A next step would be to use Raman spectroscopy in diagnosis of (pre-)malignant lesions; i.e. differentiating between different types of cancer and grading (pre-)malignant lesions (e.g., differentiating high-grade from low-grade dysplasia).12,111,126,127,136
Raman spectroscopy may address all the clinical needs mentioned, because, in fact, all show the same fundamental problem: lack of pre- and intraoperative methods with sufficient or clinically relevant sensitivity and specificity. Raman spectroscopy is an objective technique that can add biochemical information, can be performed in vivo and can be used in real-time. Raman spectroscopy can help pathologists and surgeons to assure adequate resection margins intraoperatively.2,20,21,82,148 Consequently, the use of this technique may help to improve the surgical outcome of the patients and to decrease the need for adjuvant therapy.
Raman spectroscopy can also give an objective and reliable decision about malignancy and early malignant stages.2,20,21,82,223 This technique could help medical professionals in assessing with a clinically relevant specificity and sensitivity the location for most representative biopsies.2,21,223
In vivo and ex vivo trials that aim for detection of malignant tissue have achieved sensitivities varying between 77% and 100% and specificities varying between 45% and 100%.
Studies that aimed for detection of pre-malignant lesions presented specificities (ranging between 63% and 97.8%) and sensitivities (ranging between 70% and 93.5%). Although these numbers are not perfect they demonstrate that Raman spectroscopy can help to improve current clinical practice.
In the past, a disadvantage of Raman spectroscopy was the low measurement speed. One way to overcome this is to complement Raman spectroscopy with other techniques, such as auto-fluorescence imaging. For instance, Kong et al. and McGregor et al.94,113,224 have used auto-fluorescence imaging to quickly scan large areas of tissue for selection of the measurement locations for Raman spectroscopy, thereby reducing the time spent on redundant or non-relevant Raman measurements.94,202
Although spontaneous Raman spectroscopy is a mature technology there is still considerable development in instrumentation that further enable development towards clinical applications, in particular (1) advances in the detector technology, (2) in vivo miniature fibre-optic probes to be used as an adjunct device to conventional endoscopes for biopsy targeting or for surgery guidance, (3) combined fluorescence microscopy and Raman spectroscopy systems for guided surgery, and (4) new lasers for low-cost Raman devices.
The number of scientific groups that are working towards implementation of Raman spectroscopy devices in clinical procedures is remarkable. Most of the studies (89%) still take place at the same technology readiness level (TRL-4, or class B). In these studies, algorithms have been developed for detection of (pre-) malignant tissue based on in vivo or ex vivo measurements, under conditions that approach the intended clinical environment. Validation of these algorithms based on large independent datasets obtained in the actual clinical workflow still needs to happen.
We have observed that approximately 30% of the reviewed studies were published in medical journals. In order to raise awareness and increase acceptance of Raman technologies among clinicians and clinical researchers results of Raman cancer diagnostic studies should be presented at medical conferences and published in medical journals. Better communication between clinicians and spectroscopists will facilitate understanding of the clinical requirements and challenges. The gap between technology developers and clinicians is narrowing due to the contribution of multidisciplinary networks like ClirSpec, Raman4Clinics (EU COST Action BM1401) and EPIC. These networks also actively pursue standardisation of sample preparation, measurements, data analysis protocols, which will help to form a basis for transferability and thereby accelerate the developments discussed in this review. These networks can also play an important role in presenting Raman spectroscopy as a technology that can complement and facilitate the work of the clinicians and improve patient outcome.
In summary, based on the expectation given by the companies, we believe that within 5 years Raman-based oncological products will advance into routine clinical settings.
Acknowledgements
The authors would like to thank Martin Isabelle, Jeremy Brites, Fran Adar, Daniel Barchewitz, Edwin Weusthof, Jan Toporski, Frédéric Leblond, Eric Marple, Jay Trautman, Viacheslav Artyushenko, Katsuo Morita, Ioan Notingher, Huang Zhiwei, Haishan Zeng, and Mads Bergholt for their input and valuable comments received in the preparation of this review.
Financial support is gratefully acknowledged from the Dutch Cancer Society: EMCR2015-8027, Innovative Research Programme – Photonic Devices (Netherlands Ministry of Economic Affairs): IPD12004, FNO (Fonds Nuts Ohra), Maurits en Anna de Kock Stichting, and ATOS Medical.
Notes and references
-
World Health Organization, GLOBOCAN 2012: Estimated Cancer Incidence, Mortality and Prevalence Worldwide in 2012, http://globocan.iarc.fr, (accessed 22 March 2017).
- M. Çulha, Bioanalysis, 2015, 7, 2813–2824 CrossRef PubMed
.
- P. Mendoza, M. Lacambra, P.-H. Tan and G. M. Tse, Patholog. Res. Int., 2011, 2011, 1–8 CrossRef PubMed
.
- D. Quail and J. Joyce, Nat. Med., 2013, 19, 1423–1437 CrossRef CAS PubMed
.
- S. R. Menakuru, N. J. Brown, C. A. Staton and M. W. R. Reed, Br. J. Cancer, 2008, 99, 1961–1966 CrossRef CAS PubMed
.
- L. P. Bokhorst, X. Zhu, M. Bul, C. H. Bangma, F. H. Schröder and M. J. Roobol, BJU Int., 2012, 110, 1654–1660 CrossRef CAS PubMed
.
- M. Rominger, C. Wisgickl and N. Timmesfeld, RoFo, 2012, 184, 1144–1152 CrossRef CAS PubMed
.
- A. McWilliams, M. C. Tammemagi, J. R. Mayo, H. Roberts, G. Liu, K. Soghrati, K. Yasufuku, S. Martel, F. Laberge, M. Gingras, S. Atkar-Khattra, C. D. Berg, K. Evans, R. Finley, J. Yee, J. English, P. Nasute, J. Goffin, S. Puksa, L. Stewart, S. Tsai, M. R. Johnston, D. Manos, G. Nicholas, G. D. Goss, J. M. Seely, K. Amjadi, A. Tremblay, P. Burrowes, P. MacEachern, R. Bhatia, M.-S. Tsao and S. Lam, N. Engl. J. Med., 2013, 369, 910–919 CrossRef CAS PubMed
.
- P. Carli, F. Mannone, V. De Giorgi, P. Nardini, A. Chiarugi and B. Giannoti, Melanoma Res., 2003, 2, 179–182 CrossRef
.
- W.-C. Kuo, J. Kim, N. D. Shemonski, E. J. Chaney, D. R. Spillman and S. A. Boppart, Biomed. Opt. Express, 2012, 3, 1149–1161 Search PubMed
.
- L. P. Hariri, M. Mino-Kenudson, M. B. Applegate, E. J. Mark, G. J. Tearney, M. Lanuti, C. L. Channick, A. Chee and M. J. Suter, Chest, 2013, 144, 1261–1268 CrossRef PubMed
.
- M. S. Bergholt, K. Lin, J. Wang, W. Zheng, H. Xu, Q. Huang, J. L. Ren, K. Y. Ho, M. Teh, S. Srivastava, B. Wong, K. G. Yeoh and Z. Huang, J. Biophotonics, 2016, 9, 333–342 CrossRef CAS PubMed
.
- D. Shin, N. Vigneswaran, A. Gillenwater and R. Richards-Kortum, Future Oncol., 2010, 6, 1143–1154 CrossRef PubMed
.
- L. M. Wong Kee Song, S. Banerjee, D. Desilets, D. L. Diehl, F. A. Farraye, V. Kaul, S. R. Kethu, R. S. Kwon, P. Mamula, M. C. Pedrosa, S. A. Rodriguez and W. M. Tierney, Gastrointest. Endosc., 2011, 73, 647–650 CrossRef PubMed
.
- Y. Wang, Q. Wang, J. Feng and Q. Wu, Patient Prefer. Adherence, 2013, 7, 621–631 Search PubMed
.
- A. Nijssen, S. Koljenović, T. C. Bakker Schut, P. J. Caspers and G. J. Puppels, J. Biophotonics, 2009, 2, 29–36 CrossRef CAS PubMed
.
- B. Broadbent, J. Tseng, R. Kast, T. Noh, M. Brusatori, S. N. Kalkanis and G. W. Auner, J. Neurooncol., 2016, 130, 1–9 CrossRef PubMed
.
- W. Wang, J. Zhao, M. Short and H. Zeng, J. Biophotonics, 2015, 8, 527–545 CrossRef PubMed
.
- X. Li, Y. Wang, X. Zhang, D. Wang and J. Lin, Conf. Proc. IEEE Eng. Med. Biol. Soc., 2005, 2, 1453–1456 Search PubMed
.
- C. Kallaway, L. M. Almond, H. Barr, J. Wood, J. Hutchings, C. Kendall and N. Stone, Photodiagn. Photodyn. Ther., 2013, 10, 207–219 CrossRef CAS PubMed
.
- H. McGregor, W. Wang, M. Short and H. Zeng, Adv. Health Care Technol., 2016, 2, 13 Search PubMed
.
- A. Binahmed, R. W. Nason and A. A. Abdoh, Oral Oncol., 2007, 43, 780–784 CrossRef PubMed
.
- R. W. H. Smits, S. Koljenović, J. A. Hardillo, I. ten Hove, C. A. Meeuwis, A. Sewnaik, E. A. C. Dronkers, T. C. Bakker Schut, T. P. M. Langeveld, J. Molenaar, V. N. Hegt, G. J. Puppels and R. J. Baatenburg de Jong, Head Neck, 2016, 38, E2197–E2203 CrossRef PubMed
.
- J. K. Dillon, C. B. Brown, T. M. McDonald, D. C. Ludwig, P. J. Clark, B. G. Leroux and N. D. Futran, J. Oral Maxillofac. Surg., 2015, 73, 1182–1188 CrossRef PubMed
.
- Y. Neuzillet, M. Soulie, S. Larre, M. Roupret, G. Defortescu, T. Murez, G. Pignot, A. Descazeaud, J. J. Patard, P. Bigot, L. Salomon, P. Colin, J. Rigaud, C. Bastide, X. Durand, A. Valeri, F. Kleinclauss, F. Bruyere and C. Pfister, BJU Int., 2013, 111, 1253–1260 CrossRef PubMed
.
- S. Gokavarapu, L. M. C. Rao, M. Mahajan, N. Parvataneni, K. V. V. N. Raju and R. Chander, Br. J. Oral Maxillofac. Surg., 2015, 53, 875–879 CrossRef PubMed
.
- M. D. Williams, Curr. Oncol. Rep., 2016, 18, 54 CrossRef PubMed
.
- J. R. Miedema and H. V. Hunt, J. Gastrointestin. Liver Dis., 2010, 19, 181–185 Search PubMed
.
- T. P. Olson, J. Harter, A. Muñoz, D. M. Mahvi and T. Breslin, Ann. Surg. Oncol., 2007, 14, 2953–2960 CrossRef CAS PubMed
.
- R. Emmadi and E. L. Wiley, Int. J. Surg. Oncol., 2012, 2012, 1–9 CrossRef PubMed
.
- H. E. Trejo Bittar, P. Incharoen, A. D. Althouse and S. Dacic, Mod. Pathol., 2015, 28, 1058–1063 CrossRef PubMed
.
- E. L. Rosenthal, J. M. Warram, K. I. Bland and K. R. Zinn, Ann. Surg., 2015, 261, 46–55 CrossRef PubMed
.
- M. A. Varvares, S. Poti, B. Kenyon, K. Christopher and R. J. Walker, Laryngoscope, 2015, 125, 2298–2307 CrossRef PubMed
.
- N. D. Magee, J. R. Beattie, C. Carland, R. Davis, K. McManus, I. Bradbury, D. A. Fennell, P. W. Hamilton, M. Ennis, J. J. McGarvey and J. S. Elborn, J. Biomed. Opt., 2010, 15, 026015 CrossRef PubMed
.
- E. R. McIntosh, S. Harada, J. Drwiega, M. S. Brandwein-Gensler and J. Gordetsky, Ann. Diagn. Pathol., 2015, 19, 326–329 CrossRef PubMed
.
- C. Senft, A. Bink, K. Franz, H. Vatter, T. Gasser and V. Seifert, Lancet Oncol., 2011, 12, 997–1003 CrossRef PubMed
.
- C. Senft, B. Schoenes, T. Gasser, J. Platz, A. Bink, K. Franz and V. Seifert, J. Neurosurg. Anesthesiol., 2011, 23, 241–246 CrossRef PubMed
.
- J. M. Wong, J. R. Panchmatia, J. E. Ziewacz, A. M. Bader, I. F. Dunn, E. R. Laws and A. A. Gawande, Neurosurg. Focus, 2012, 33, E16 CrossRef PubMed
.
- J.-S. Wu, Y. Mao, L.-F. Zhou, W.-J. Tang, J. Hu, Y.-Y. Song, X.-N. Hong and G.-H. Du, Neurosusrgery, 2007, 61, 935–949 CrossRef PubMed
.
- E. B. Claus, A. Horlacher, L. Hsu, R. B. Schwartz, D. Dello-Iacono, F. Talos, F. A. Jolesz and P. M. Black, Cancer, 2005, 103, 1227–1233 CrossRef PubMed
.
- D. Kuhnt, A. Becker, O. Ganslandt, M. Bauer, M. Buchfelder and C. Nimsky, Neuro. Oncol., 2011, 13, 1339–1348 CrossRef PubMed
.
- P. L. Kubben, K. J. ter Meulen, O. E. M. G. Schijns, M. P. ter Laak-Poort, J. J. van Overbeeke and H. van Santbrink, Lancet Oncol., 2011, 12, 1062–1070 CrossRef PubMed
.
- J. D. Visgauss, W. C. Eward and B. E. Brigman, Orthop. Clin. North Am., 2016, 47, 253–264 CrossRef PubMed
.
- F. Prada, A. Perin, A. Martegani, L. Aiani, L. Solbiati, M. Lamperti, C. Casali, F. Legnani, L. Mattei, A. Saladino, M. Saini and F. Dimeco, Neurosurgery, 2014, 74, 542–552 CrossRef PubMed
.
- T. Selbekk, A. S. Jakola, O. Solheim, T. F. Johansen, F. Lindseth, I. Reinertsen and G. Unsgård, Acta Neurochir., 2013, 155, 973–980 CrossRef PubMed
.
- S. J. Erickson-Bhatt, R. M. Nolan, N. D. Shemonski, S. G. Adie, J. Putney, D. Darga, D. T. McCormick, A. J. Cittadine, A. M. Zysk, M. Marjanovic, E. J. Chaney, G. L. Monroy, F. A. South, K. A. Cradock, Z. G. Liu, M. Sundaram, P. S. Ray and S. A. Boppart, Cancer Res., 2015, 75, 3706–3712 CrossRef CAS PubMed
.
- W. Stummer, U. Pichlmeier, T. Meinel, O. D. Wiestler, F. Zanella and H. J. Reulen, Lancet Oncol., 2006, 7, 392–401 CrossRef CAS PubMed
.
- G. Widhalm, B. Kiesel, A. Woehrer, T. Traub-Weidinger, M. Preusser, C. Marosi, D. Prayer, J. A. Hainfellner, E. Knosp and S. Wolfsberger, PLoS One, 2013, 8, e76988 CAS
.
- W. Stummer, J.-C. Tonn, H. M. Mehdorn, U. Nestler, K. Franz, C. Goetz, A. Bink and U. Pichlmeier, J. Neurosurg., 2011, 114, 613–623 CrossRef PubMed
.
- D. W. Roberts, P. A. Valdés, B. T. Harris, D. Ph, K. M. Fontaine, A. Hartov, X. Fan, S. Ji, D. Sc, S. S. Lollis, B. W. Pogue, F. Leblond and D. Tor, J. Neuroserg., 2011, 114, 595–603 CrossRef PubMed
.
- E. D. Agdeppa and M. E. Spilker, AAPS J., 2009, 11, 286–299 CrossRef CAS PubMed
.
- M. Jermyn, K. Mok, J. Mercier, J. Desroches, J. Pichette, K. Saint-Arnaud, L. Bernstein, M.-C. Guiot, K. Petrecca and F. Leblond, Sci. Transl. Med., 2015, 7, 274ra19–274ra19 CrossRef CAS PubMed
.
- A. S. Haka, Z. Volynskaya, J. A. Gardecki, J. Nazemi, R. Shenk, N. Wang, R. R. Dasari, M. Fitzmaurice and M. S. Feld, J. Biomed. Opt., 2009, 14, 54023 CrossRef PubMed
.
- N. Kourkoumelis, I. Balatsoukas, V. Moulia, A. Elka, G. Gaitanis and I. D. Bassukas, Int. J. Mol. Sci., 2015, 16, 14554–14570 CrossRef CAS PubMed
.
- L. A. Austin, S. Osseiran and C. L. Evans, Analyst, 2016, 141, 476–503 RSC
.
- I. Pence and A. Mahadevan-Jansen, Chem. Soc. Rev., 2016, 45, 1958–1979 RSC
.
- D. W. Shipp, F. Sinjab and I. Notingher, Adv. Opt. Photonics, 2017, 9, 315–428 CrossRef
.
- C. Krafft, I. W. Schie, T. Meyer, M. Schmitt and J. Popp, Chem. Soc. Rev., 2016, 45, 1819–1849 RSC
.
- C. H. Camp Jr. and M. T. Cicerone, Nat. Photonics, 2015, 9, 295–305 CrossRef
.
- L. T. Kerr, T. M. Lynn, I. M. Cullen, P. J. Daly, N. Shah, S. O'Dea, A. Malkin and B. M. Hennelly, Anal. Methods, 2016, 8, 4991–5000 RSC
.
- M. Isabelle, N. Stone, H. Barr, M. Vipond, N. Shepherd and K. Rogers, Spectroscopy, 2008, 22, 97–104 CrossRef CAS
.
-
European Commission, Horizon 2020; Work programme 2014–2015, 2014.
- J. M. Johnson, R. R. Dalton, S. M. Wester, J. Landercasper and P. J. Lambert, Arch. Surg., 1999, 134, 712–716 CrossRef CAS
.
- M. Wang, X. He, Y. Chang, G. Sun and L. Thabane, Breast, 2017, 31, 157–166 CrossRef PubMed
.
- W. Sun, A. Li, F. Abreo, E. Turbat-Herrera and W. D. Grafton, Diagn. Cytopathol., 2001, 24, 421–425 CrossRef CAS PubMed
.
- B. Lieske, D. Ravichandran and D. Wright, Br. J. Cancer, 2006, 95, 62–66 CrossRef CAS PubMed
.
- Y.-H. Yu, W. Wei, J.-L. Liu, R. Gupta, S. Naran, A. Buchanan, R. Fauck, J. Simpson, K. Dowlatshahi, P. Jokich, R. Schmidt, M. Bibbo, P. Dawson, W. Evans, S. Cade, G. Sterrett, D. Oliver, J. Trayne, D. Ingram, H. Sherner, E. Azavedo, G. Svane, G. Auer, W. Evans, S. Cade, H. Jay, M. Monica, L. Marce, H. Samuel, V. Jackson, H. Reynolds, P. Whiting, M. Weswood, A. Rutjes, J. Reitsma, P. Bossuyt, J. Kleijnen, W. Deville, F. Buntinx, L. Bouter, V. Montori, H. Vet, D. Windt, P. Bezemer, B. Kanchanabat, P. Kanchanapitak, W. Thanapongsathorn, A. Manomaiphiboon, E. Pisano, L. Fajardo, D. Caudry, N. Sneige, W. Frable, W. Berg, I. Tocino, S. Schnitt, J. Connolly, C. Gatsonis, B. McNeil, L. Moses, D. Shapiro, B. Littenberg, J. Lau, J. Ioannidis, E. Balk, C. Milch, N. Terrin, P. Chew, D. Salem, L. Irwig, A. Tosteson, C. Gatsonis, J. Lau, G. Colditz, T. Chalmers, F. Mosteller, S. Suzuki, T. Moro-oka, N. Choudhry, M. Westwood, P. Whiting, J. Kleijnen, M. Egger, G. D. Smith, M. Schneider, C. Minder, A. Mezzelani, L. Alasio, C. Bartoli, M. Bonoral, M. Pierotti, F. Rilke, S. Pilotti, A. Bofin, B. Ytterhus, B. Hagmar, A. Somani, J. Hwang, B. Chaiwun, G. Tse, P. Liu, P. Tan, B. Gazic, J. Pizem, M. Bracko, T. Cufer, S. Borstnar, Z. Pohar-Marinsek, M. Us-Krasovec, C. Kelten, M. Akbulut, O. Zekioglu, M. Kapkac, Y. Erhan, N. Ozdemir, B. Zhang, L. Chang, Y. Sun, J. Xu, K. Ye, S. Xu, W. Jin, R. Li, C. Cui, D. Piao, Z. Shen, A. Sapino, P. Cassoni, E. Zanon, F. Fraire, S. Croce, C. Coluccia, M. Donadio, G. Bussolati, A. Jain, M. Haisfield-Wolfe, J. Lange, N. Ahuja, N. Khouri, T. Tsangaris, Z. Zhang, C. Balch, L. Jacobs, B. Kooistra, C. Wauters, L. Strobbe, G. Jeronimo, P. Monteiro, R. Henrique, M. Dinis-Ribeiro, I. Costa, V. Costa, L. Filipe, A. Carvalho, M. Hoque, I. Pais, C. Leal, M. Teixeira, D. Sidransky, T. Sauer, K. Young, S. Thoresen, T. Sauer, K. Myrvold, J. L′mo, K. Anderssen, P. Skaane, S. Walker, F. Lumachi, M. Marzola, P. Zucchetta, F. Polistina, P. Cagnato, G. Favia, F. Bui, G. Farshid, P. Downey, P. Gill, S. Pieterse, G. Dennison, R. Anand, S. Makar, J. Pain, H. Gent, E. Sprenger, K. Doulatshahl, H. Wanebo, P. Feldman, M. Wilhelm, J. Covell, R. Binns, L. Apesteguia, L. Pina, M. Inchusta, M. Mellado, T. Franque, D. Miguel, A. Lopez-Cousillas, B. Reparaz, L. Manheimer, A. Rywlin, M. Jan, J. Mattoo, N. Salroo, S. Ahangar, P. Drew, L. Turnbull, S. Chatterjee, J. Read, P. Carleton, J. Fox, J. Monson, M. Kerin, S. Masood, E. Frykberg, G. Mclellan, J. Bullard, T. Ishikawa, Y. Hamaguchi, M. Tanabe, N. Momiyama, T. Chishima, Y. Nakatani, A. Nozawa, T. Sasaki, H. Kitamura, H. Shimada, M. Crotch-Harvey, C. Loughran, K. Leifland, U. Lagersted, G. Svane, H. Okamoto, T. Ogawara, S. Inoue, K. Kobayashi, T. Sekikawa, Y. Matsumoto, M. Rubin, K. Horiuchi, N. Joy, W. Haun, R. Read, E. Ratzer, M. Fenoglio, D. Colorado, J. Li, D. Wang, H. Yang, L. Bao, C. Liu, Q. Zhao, Y. Zhan, X. Yu, Q. Guo, P. Zhan, E. Ye, L. Gao, M. Zhang, M. Zhu, B. Lu, B. Chen, F. Sun, Y. Yan, L. Wang, Q. Liu, J. Hong, Y. Liu, M. Xu, Y. Zhang, J. Li, Z. Ji, W. Tang, Y. Zhang, C. You, J. Li, W. Chen, X. Fan, H. Hu, K. Zheng, W. Liu, Y. Hao, J. He, W. Tang, S. Tang, W. Qian, Z. Yao, W. Nan, T. Tu, Y. Lu, W. Zhang, H. Wang, Q. Zhou, L. Zhang, Y. Pan, W. Pan, J. Ma, J. Gan, D. Wu, N. Li, J. Zhang, Y. Wang, J. Li, H. Wang, L. Wei, G. Han, J. Liu, L. Wei, S. Dong, Q. Wang, G. Tao, Z. Liu, H. Liu, Y. Liu, A. He, T. Guan, P. Wang, J. Hui, T. Chen, H. Zeng, Y. Wang, Z. Jia, R. Pan, L. Ma, H. Yang, Y. Huang, Q. Shu, G. Zhang, D. Yong, K. Yu, W. Yang, J. Yang, Y. Jiang, Q. Wang, G. Wang, L. Zhou, T. Lin, P. Whiting, A. Rutjes, J. Reitsma, P. Bossuyt, J. Kleijnen, A. Glas, J. Lijmer, M. Prins, G. Bonsel, P. Bossuy, J. Deeks, R. Jaeschke, G. Guyatt, J. Lijmer, H. Lee, P. Ooi, W. Poh, C. Wong and D. Petitti, BMC Cancer, 2012, 12, 41 CrossRef PubMed
.
- M. Kazi, Suhani, R. Parshad, V. Seenu, S. Mathur and K. P. Haresh, World J. Surg., 2017, 41, 1528–1533 CrossRef PubMed
.
- M. C. van Maaren, L. de Munck, J. J. Jobsen, P. Poortmans, G. H. de Bock, S. Siesling and L. J. A. Strobbe, Breast Cancer Res. Treat., 2016, 160, 511–521 CrossRef CAS PubMed
.
- A. A. Onitilo, J. M. Engel, R. V. Stankowski and S. A. R. Doi, Clin. Med. Res., 2015, 13, 65–73 CrossRef PubMed
.
- S. Agarwal, L. Pappas, L. Neumayer, K. Kokeny and J. Agarwal, JAMA Surg., 2014, 149, 267 CrossRef PubMed
.
-
I. O. Ellis, P. Carder, S. Hales, A. H. S. Lee, S. E. Pinder, E. Rakha, T. Stephenson, S. Al-Sam, R. Deb, A. Hanby, R. Liebmann, E. Provenzano, D. Rowlands, C. A. Wells, N. Anderson, A. Girling, M. Ibrahim, E. Mallon and C. Quinn, Pathology reporting of breast disease in surgical excision specimens incorporating the dataset for histological reporting of breast cancer, 2016 Search PubMed
.
- E. L. Vos, S. Siesling, M. H. A. Baaijens, C. Verhoef, A. Jager, A. C. Voogd and L. B. Koppert, Breast Cancer Res. Treat., 2017 DOI:10.1007/s10549-017-4232-6
.
- L. Jacobs, Ann. Surg. Oncol., 2008, 15, 1271–1272 CrossRef PubMed
.
- A. Saha, I. Barman, N. C. Dingari, S. McGee, Z. Volynskaya, L. H. Galindo, W. Liu, D. Plecha, N. Klein, R. R. Dasari and M. Fitzmaurice, Biomed. Opt. Express, 2011, 2, 2792 CrossRef CAS PubMed
.
- I. Barman, N. C. Dingari, A. Saha, S. McGee, L. H. Galindo, W. Liu, D. Plecha, N. Klein, R. R. Dasari and M. Fitzmaurice, Cancer Res., 2013, 73, 3206–3215 CrossRef CAS PubMed
.
- A. Lomas, J. Leonardi-Bee and F. Bath-Hextall, Br. J. Dermatol., 2012, 166, 1069–1080 CrossRef CAS PubMed
.
- K. G. Lewis and M. A. Weinstock, Arch. Dermatol., 2004, 140, 837–842 Search PubMed
.
- E. Drakaki, T. Vergou, C. Dessinioti, A. J. Stratigos, C. Salavastru and C. Antoniou, J. Biomed. Opt., 2013, 18, 61221 CrossRef PubMed
.
- Basaalcercarcinoom - Klinische marges BCC, https://richtlijnendatabase.nl/richtlijn/basaalcelcarcinoom/conventionele_chirurgische_excisie_bcc/klinische_marges_bcc.html, (accessed 25 April 2017).
- E. van Loo, K. Mosterd, G. A. M. Krekels, M. H. Roozeboom, J. U. Ostertag, C. D. Dirksen, P. M. Steijlen, H. A. M. Neumann, P. J. Nelemans and N. W. J. Kelleners-Smeets, Eur. J. Cancer, 2014, 50, 3011–3020 CrossRef PubMed
.
- M. Jermyn, J. Desroches, K. Aubertin, K. St-arnaud, J. Madore, E. De Montigny, M. Guiot, D. Trudel, C. Brian, K. Petrecca and F. Leblond, Phys. Med. Biol., 2016, 61, R370–R400 CrossRef PubMed
.
- R. Siegel, J. Ma, Z. Zou and A. Jemal, CA–Cancer J. Clin., 2014, 64, 9–29 CrossRef PubMed
.
- C. M. Balch, J. E. Gershenwald, S. J. Soong, J. F. Thompson, M. B. Atkins, D. R. Byrd, A. C. Buzaid, A. J. Cochran, D. G. Coit, S. Ding, A. M. Eggermont, K. T. Flaherty, P. A. Gimotty, J. M. Kirkwood, K. M. McMasters, M. C. Mihm, D. L. Morton, M. I. Ross, A. J. Sober and V. K. Sondak, J. Clin. Oncol., 2009, 27, 6199–6206 CrossRef PubMed
.
- P. V. Dickson and J. E. Gershenwald, Surg. Oncol. Clin. N. Am., 2011, 20, 1–17 CrossRef PubMed
.
- H. Kittler, H. Pehamberger, K. Wolff and M. Binder, Lancet Oncol., 2002, 3, 159–165 CrossRef CAS PubMed
.
- C. A. Morton and R. M. Mackie, Br. J. Dermatol., 1998, 138, 283–287 CrossRef CAS PubMed
.
- J. I. van der Rhee, W. Bergman and N. A. Kukutsch, Acta Derm.-Venereol., 2011, 91, 428–431 CrossRef PubMed
.
- I. P. Santos, P. J. Caspers, T. C. Bakker Schut, R. van Doorn, V. Noordhoek Hegt, S. Koljenović and G. J. Puppels, Anal. Chem., 2016, 88, 7683–7688 CrossRef CAS PubMed
.
- H. Lui, J. Zhao, D. McLean and H. Zeng, Cancer Res., 2012, 72, 2491–2500 CrossRef CAS PubMed
.
- C. Lieber, S. K. Majumder, D. Billheimer, D. L. Ellis and A. Mahadevan-Jansen, J. Biomed. Opt., 2008, 13, 24013 CrossRef PubMed
.
- J. Zhao, H. Lui, S. Kalia and H. Zeng, Anal. Bioanal. Chem., 2015, 407, 8373–8379 CrossRef CAS PubMed
.
- J. Schleusener, P. Gluszczynska, C. Reble, I. Gersonde, J. Helfmann, J. W. Fluhr, J. Lademann, J. Röwert-Huber, A. Patzelt and M. C. Meinke, Exp. Dermatol., 2015, 24, 767–772 CrossRef PubMed
.
- K. Kong, C. J. Rowlands, S. Varma, W. Perkins, I. H. Leach, A. A. Koloydenko, H. C. Williams and I. Notingher, Proc. Natl. Acad. Sci. U. S. A., 2013, 110, 15189–15194 CrossRef CAS PubMed
.
- K. Eberhardt, C. Stiebing, C. Matthäus, M. Schmitt and J. Popp, Expert Rev. Mol. Diagn., 2015, 15, 773–787 CAS
.
- J. Zhao, H. Lui, D. I. McLean and H. Zeng, Conf. Proc. IEEE Eng. Med. Biol. Soc., 2008, 2008, 3107–3109 Search PubMed
.
- C. Lieber and A. Mahadevan-Jansen, Opt. Express, 2007, 15, 11874–11882 CrossRef CAS PubMed
.
- C. A. Lieber, S. K. Majumder, D. L. Ellis, D. D. Billheimer and A. Mahadevan-Jansen, Lasers Surg. Med., 2008, 40, 461–467 CrossRef PubMed
.
- L. Silveira Jr., F. L. Silveira, B. Bodanese, M. T. T. Pacheco and R. A. Zângaro, Proc. SPIE, 2012, 8207, 82070X CrossRef
.
- L. Lim, B. Nichols, M. R. Migden, N. Rajaram, J. S. Reichenberg, M. K. Markey, M. I. Ross and J. W. Tunnell, J. Biomed. Opt., 2014, 19, 117003 CrossRef PubMed
.
- I. P. Santos, P. J. Caspers, T. Bakker Schut, R. van Doorn, S. Koljenović and G. J. Puppels, J. Raman Spectrosc., 2015, 652–660 CrossRef CAS
.
- I. A. Bratchenko, D. N. Artemyev, O. O. Myakinin, Y. A. Khristoforova, A. A. Moryatov, S. V. Kozlov and V. P. Zakharov, J. Biomed. Opt., 2017, 22, 27005 CrossRef PubMed
.
- J. Sun, D. H. Garfield, B. Lam, J. Yan, A. Gu, J. Shen and B. Han, J. Thorac. Oncol., 2011, 6, 1336–1344 CrossRef PubMed
.
-
P. Jain, S. Hadique and A. C. Mehta, in Interventional Bronchoscopy, Humana Press, Totowa, NJ, 2013, vol. 100, pp. 15–44 Search PubMed
.
- M. O. Idowu and C. N. Powers, Int. J. Clin. Exp. Pathol., 2010, 3, 367–385 Search PubMed
.
- R. J. Ginsberg and L. V. Rubinstein, Ann. Thorac. Surg., 1995, 60, 615–623 CrossRef CAS PubMed
.
- R. J. Landreneau, D. J. Sugarbaker, M. J. Mack, S. R. Hazelrigg, J. D. Luketich, L. Fetterman, M. J. Liptay, S. Bartley, T. M. Boley, R. J. Keenan, P. F. Ferson, R. J. Weyant, K. S. Naunheim, J. B. Mark, J. R. Benfield, R. Cohen and A. N. Thomas, J. Thorac. Cardiovasc. Surg., 1997, 113, 691–700 CrossRef CAS PubMed
.
- J. D. Blasberg, H. I. Pass and J. S. Donington, J. Thorac. Oncol., 2010, 5, 1583–1593 CrossRef PubMed
.
- S. Liu, R. Wang, Y. Zhang, Y. Li, C. Cheng, Y. Pan, J. Xiang, Y. Zhang, H. Chen and Y. Sun, J. Clin. Oncol., 2016, 34, 307–313 CrossRef PubMed
.
- Y.-C. Yeh, J. Nitadori, K. Kadota, A. Yoshizawa, N. Rekhtman, A. L. Moreira, C. S. Sima, V. W. Rusch, P. S. Adusumilli and W. D. Travis, Histopathology, 2015, 66, 922–938 CrossRef PubMed
.
- M. A. Short, S. Lam, A. McWilliams, J. Zhao, H. Lui and H. Zeng, Opt. Lett., 2008, 33, 711–713 CrossRef CAS PubMed
.
- M. A. Short, S. Lam, A. M. McWilliams, D. N. Ionescu and H. Zeng, J. Thorac. Oncol., 2011, 6, 1206–1214 CrossRef PubMed
.
- H. C. McGregor, M. A. Short, A. McWilliams, T. Shaipanich, D. N. Ionescu, J. Zhao, W. Wang, G. Chen, S. Lam and H. Zeng, J. Biophotonics, 2016, 10, 98–110 CrossRef PubMed
.
- J. Ferlay, I. Soerjomataram, R. Dikshit, S. Eser, C. Mathers, M. Rebelo, D. M. Parkin, D. Forman and F. Bray, Int. J. Cancer, 2015, 136, E359–E386 CrossRef CAS PubMed
.
- J. Rees, P. Lao-Sirieix, A. Wong and R. Fitzgerald, Cochrane Database Syst. Rev., 2011, 2010–2012 Search PubMed
.
- N. Lal, D. K. Bhasin, A. K. Malik, N. M. Gupta, K. Singh and S. K. Mehta, Gut, 1992, 33, 724–726 CrossRef CAS PubMed
.
- Y. Choi, H. S. Choi, W. K. Jeon, B. I. Kim, D. Il Park, Y. K. Cho, H. J. Kim, J. H. Park and C. Il Sohn, J. Korean Med. Sci., 2012, 27, 36–39 CrossRef PubMed
.
- S. Menon and N. Trudgill, Endosc. Int. Open, 2014, 2, E46–E50 CrossRef PubMed
.
- S. Tsutsui, H. Kuwano, M. Watanabe, M. Kitamura and K. Sugimachi, Ann. Surg., 1995, 222, 193–202 CrossRef CAS PubMed
.
- A. G. Casson, S. J. Darnton, S. Subramanian and L. Hiller, Ann. Thorac. Surg., 2000, 69, 205–209 CrossRef CAS PubMed
.
- P. M. Sagar, D. Johnston, M. J. McMahon, M. F. Dixon and P. Quirke, Br. J. Surg., 1993, 80, 1386–1388 CrossRef CAS PubMed
.
- S. P. Dexter, H. Sue-Ling, M. J. McMahon, P. Quirke, N. Mapstone and I. G. Martin, Gut, 2001, 48, 667–670 CrossRef CAS PubMed
.
- S. K. Teh, W. Zheng, K. Y. Ho, M. Teh, K. G. Yeoh and Z. Huang, Br. J. Cancer, 2008, 98, 457–465 CrossRef CAS PubMed
.
- M. S. Bergholt, W. Zheng, K. Lin, K. Y. Ho, M. Teh, K. G. Yeoh, J. B. Y. So and Z. Huang, Analyst, 2010, 135, 3162–3168 RSC
.
- S. Duraipandian, M. S. Bergholt, W. Zheng, K. Y. Ho, M. Teh, K. G. Yeoh, J. B. Y. So, A. Shabbir and Z. Huang, J. Biomed. Opt., 2012, 17, 81418 CrossRef PubMed
.
- M. S. Bergholt, W. Zheng, K. Y. Ho, K. G. Yeoh, M. Teh, J. B. Y. So and Z. Huang, Proc. SPIE, 2014, 8939, 89390M CrossRef
.
-
K. Lin, J. Wang, M. Sylvest Bergholt, W. Zheng, H. Xu, J. Ren, K. Yu Ho, M. Teh, K. Guan Yeoh and Z. Huang, in Proc. of SPIE, ed. M. J. Suter, S. Lam, M. Brenner, G. J. Tearney and T. D. Wang, Nature Publishing Group, 2015, vol. 9304, p. 93040V Search PubMed
.
- M. Ishigaki, Y. Maeda, A. Taketani, B. B. Andriana, R. Ishihara, K. Wongravee, Y. Ozaki and H. Sato, Analyst, 2015, 141, 1027–1033 RSC
.
- J. Wang, K. Lin, W. Zheng, K. Yu Ho, M. Teh, K. Guan Yeoh and Z. Huang, Sci. Rep., 2015, 5, 12957 CrossRef CAS PubMed
.
- P. J. Slootweg, G. J. Hordijk, Y. Schade, R. J. J. Van Es and R. Koole, Oral Oncol., 2002, 38, 500–503 CrossRef PubMed
.
- N. Al-Rajhi, Y. Khafaga, J. El-Husseiny, M. Saleem, W. Mourad, A. Al-Otieschan and A. Al-Amro, Oral Oncol., 2000, 36, 508–514 CrossRef CAS PubMed
.
-
T. Helliwell and J. Woolgar, Dataset for histopathology reporting of mucosal malignancies of the nasal cavities and paranasal sinuses, 2013 Search PubMed
.
-
T. Helliwell and J. Woolgar, Dataset for histopathology reporting of mucosal malignancies of the larynx, 2013 Search PubMed
.
- J. Hoffmannová, R. Foltán, M. Vlk, M. Šipoš, E. Horká, G. Pavlíková, R. Kufa, O. Bulík and J. Šedý, Int. J. Oral Maxillofac. Surg., 2010, 39, 561–567 CrossRef PubMed
.
- E. M. Barroso, R. W. H. Smits, T. C. B. Schut, I. Ten Hove, J. A. Hardillo, E. B. Wolvius, R. J. Baatenburg De Jong, S. Koljenović and G. J. Puppels, Anal. Chem., 2015, 87, 2419–2426 CrossRef CAS PubMed
.
- H. Krishna, S. K. Majumder, P. Chaturvedi, M. Sidramesh and P. K. Gupta, J. Biophotonics, 2014, 7, 690–702 CrossRef CAS PubMed
.
- K. Guze, H. C. Pawluk, M. Short, H. Zeng, J. Lorch, C. Norris and S. Sonis, Head Neck, 2015, 37, 511–517 CrossRef PubMed
.
- E. M. Barroso, R. W. H. Smits, C. G. F. Van Lanschot, P. J. Caspers, I. Ten Hove, H. Mast, A. Sewnaik, J. Hardillo, C. Meeuwis, R. Verdijk, V. N. Hegt, R. J. Baatenburg De Jong, E. B. Wolvius, T. C. Bakker Schut, S. Koljenović and G. J. Puppels, Cancer Res., 2016, 76, 5945–5953 CrossRef CAS PubMed
.
-
E. T. Wong and J. K. Wu, Clinical presentation and diagnosis of brain tumors, https://www.uptodate.com/contents/clinical-presentation-and-diagnosis-of-brain-tumors?source=search_result&search=brain cancer&selectedTitle=1~150#H22, (accessed 12 May 2017).
- T. Hollon, S. Lewis, C. W. Freudiger, X. Sunney Xie and D. A. Orringer, Neurosurg. Focus, 2016, 40, E9 CrossRef PubMed
.
- S. Koljenović, L.-P. Choo-Smith, T. C. Bakker Schut, J. M. Kros, H. J. van den Berge and G. J. Puppels, Lab. Invest., 2002, 82, 1265–1277 CrossRef
.
- N. F. Marko, R. J. Weil, J. L. Schroeder, F. F. Lang, D. Suki and R. E. Sawaya, J. Clin. Oncol., 2014, 32, 774–782 CrossRef PubMed
.
- N. Sanai, S. Chang and M. Berger, J. Neurosurg., 2011, 115, 945–947 CrossRef PubMed
.
- F. K. Lu, D. Calligaris, O. I. Olubiyi, I. Norton, W. Yang, S. Santagata, X. S. Xie, A. J. Golby and N. Y. R. Agar, Cancer Res., 2016, 76, 3451–3462 CrossRef CAS PubMed
.
- F. Albert, M. Forsting, K. Sartor, H. Adams and S. Kunze, Neurosurgery, 1994, 34, 45–61 CAS
.
- S. Ulmer, World J. Radiol., 2014, 6, 538–543 CrossRef PubMed
.
- M. Ji, S. Lewis, S. Camelo-Piragua, S. H. Ramkissoon, M. Snuderl, S. Venneti, A. Fisher-Hubbard, M. Garrard, D. Fu, A. C. Wang, J. A. Heth, C. O. Maher, N. Sanai, T. D. Johnson, C. W. Freudiger, O. Sagher, X. S. Xie and D. A. Orringer, Sci. Transl. Med., 2015, 7, 309ra163–309ra163 CrossRef PubMed
.
-
M. Jermyn, K. Mok, J. Mercier, J. Desroches, J. Pichette, K. Saint-Arnaud, M.-C. Guiot, K. Petrecca and F. Leblond, in Science Translational Medicine, ed. R. R. Alfano and S. G. Demos, 2015, vol. 7, p. 93180D Search PubMed
.
- M. B. Wallace and R. Keisslich, Gastroenterology, 2010, 138, 2140–2150 CrossRef PubMed
.
- B. Bressler, L. F. Paszat, Z. Chen, D. M. Rothwell, C. Vinden and L. Rabeneck, Gastroenterology, 2007, 132, 96–102 CrossRef PubMed
.
- E. Quintero, C. Hassan, C. Senore and Y. Saito, Gastroenterol. Res. Pract., 2012, 2012 Search PubMed
, 846985.
- A. Stallmach, C. Schmidt, A. Watson and R. Kiesslich, J. Biophotonics, 2011, 4, 482–489 CrossRef PubMed
.
- W. R. Kessler, T. F. Imperiale, R. W. Klein, R. C. Wielage and D. K. Rex, Endoscopy, 2011, 43, 683–691 CrossRef CAS PubMed
.
- H. Nelson, N. Petrelli, A. Carlin and E. Al, J. Natl. Cancer Inst., 2001, 93, 591 Search PubMed
.
- R. Amri, L. G. Bordeianou, P. Sylla and D. L. Berger, JAMA Surg., 2015, 150, 890–898 CrossRef PubMed
.
- J. B. O'Connell, M. A. Maggard and C. Y. Ko, J. Natl. Cancer Inst., 2004, 96, 1420–1425 CrossRef PubMed
.
- E. Widjaja, W. Zheng and Z. Huang, Int. J. Oncol., 2008, 32, 653–662 CAS
.
- M. A. Short, I. T. Tai, D. Owen and H. Zeng, Opt. Express, 2013, 21, 5025–5034 CrossRef CAS PubMed
.
- L. Peirson, D. Fitzpatrick-Lewis, D. Ciliska and R. Warren, Syst. Rev., 2013, 2, 35 CrossRef PubMed
.
- K. Nanda, D. C. Mccrory, E. R. Myers, L. A. Bastian, V. Hasselblad, J. D. Hickey and D. B. Matchar, Ann. Intern Med., 2000, 132, 810–819 CrossRef CAS PubMed
.
- J. Cuzick, C. Clavel, K.-U. Petry, C. J. L. M. Meijer, H. Hoyer, S. Ratnam, A. Szarewski, P. Birembaut, S. Kulasingam, P. Sasieni and T. Iftner, Int. J. Cancer, 2006, 119, 1095–1101 CrossRef CAS PubMed
.
- R. A. Mustafa, N. Santesso, R. Khatib, A. A. Mustafa, W. Wiercioch, R. Kehar, S. Gandhi, Y. Chen, A. Cheung, J. Hopkins, B. Ma, N. Lloyd, D. Wu, N. Broutet and H. J. Schünemann, Internet J. Gynecol. Obstet., 2016, 132, 259–265 CrossRef PubMed
.
- Z. Eftekhar, P. Rahimi-Moghaddam, F. Yarandi and R. Brojerdi, Asian Pac. J. Cancer Prev., 2005, 6, 69–71 Search PubMed
.
- J. Mo, W. Zheng, J. J. H. Low, J. Ng, A. Ilancheran and Z. Huang, Anal. Chem., 2009, 81, 8908–8915 CrossRef CAS PubMed
.
- E. M. Kanter, E. Vargis, S. Majumder, M. D. Keller, E. Woeste, G. G. Rao and A. Mahadevan-Jansen, J. Biophotonics, 2009, 2, 81–90 CrossRef CAS PubMed
.
- S. Duraipandian, W. Zheng, J. Ng, J. J. H. Low, A. Ilancheran and Z. Huang, Analyst, 2011, 136, 4328 RSC
.
- S. Duraipandian, W. Zheng, J. Ng, J. J. H. Low, A. Ilancheran and Z. Huang, Anal. Chem., 2012, 84, 5913–5919 CrossRef CAS PubMed
.
- I. R. M. Ramos, A. Malkin and F. M. Lyng, BioMed. Res. Int., 2015, 2015, 1–9 CrossRef PubMed
.
- S. Rubina, M. Amita, K. D. Kedar, R. Bharat and C. M. Krishna, Vib. Spectrosc., 2013, 68, 115–121 CrossRef CAS
.
- Vulval cancer mortality statistics, http://www.cancerresearchuk.org/health-professional/cancer-statistics/statistics-by-cancer-type/vulval-cancer/mortality#heading-Zero, (accessed 4 April 2017).
- R. Wessels, D. M. de Bruin, D. J. Faber, H. H. van Boven, A. D. Vincent, T. G. van Leeuwen, M. van Beurden and T. J. M. Ruers, J. Biomed. Opt., 2012, 17, 116022 CrossRef PubMed
.
- T. H. Dellinger, A. A. Hakim, S. J. Lee, M. T. Wakabayashi, R. J. Morgan and E. S. Han, J. Natl. Compr. Cancer Network, 2017, 15, 121–128 CrossRef
.
- J. M. Heaps, Y. S. Fu, F. J. Montz, N. F. Hacker and J. S. Berek, Gynecol. Oncol., 1990, 38, 309–314 CrossRef CAS PubMed
.
- J. K. Chan, V. Sugiyama, H. Pham, M. Gu, J. Rutgers, K. Osann, M. K. Cheung, M. L. Berman and P. J. DiSaia, Gynecol. Oncol., 2007, 104, 636–641 CrossRef PubMed
.
- R. Wessels, M. van Beurden, D. M. de Bruin, D. J. Faber, A. D. Vincent, J. Sanders, T. G. van Leeuwen and T. J. M. Ruers, Int. J. Gynecol. Cancer, 2015, 25, 112–118 CrossRef PubMed
.
- J. Frost, L. Ludeman, K. Hillaby, R. Gornall, G. Lloyd, C. Kendall, A. C. Shore and N. Stone, Analyst, 2017, 142, 1200–1206 RSC
.
- T. Minamikawa, Y. Harada and T. Takamatsu, Sci. Rep., 2015, 5, 17165 CrossRef CAS PubMed
.
- P. E. Clark, N. Agarwal, M. C. Biagioli, M. A. Eisenberger, R. E. Greenberg, H. W. Herr, B. A. Inman, D. A. Kuban, T. M. Kuzel, S. M. Lele, J. Michalski, L. C. Pagliaro, S. K. Pal, A. Patterson, E. R. Plimack, K. S. Pohar, M. P. Porter, J. P. Richie, W. J. Sexton, W. U. Shipley, E. J. Small, P. E. Spiess, D. L. Trump, G. Wile, T. G. Wilson, M. Dwyer, M. Ho and National Comprehensive Cancer Network (NCCN), J. Natl. Compr. Cancer Network, 2013, 11, 446–475 CrossRef CAS
.
- I. Barman, N. C. Dingari, G. P. Singh, R. Kumar, S. Lang and G. Nabi, Anal. Bioanal. Chem., 2012, 404, 3091–3099 CrossRef CAS PubMed
.
- D. Jocham, H. Stepp and R. Waidelich, Eur. Urol., 2008, 53, 1138–1150 CrossRef CAS PubMed
.
- L. M. Seltz and S. D. Herrell, Curr. Opin. Urol., 2016, 26, 259–263 CrossRef PubMed
.
- Z. A. Dotan, K. Kavanagh, O. Yossepowitch, M. Kaag, S. Olgac, M. Donat and H. W. Herr, J. Urol., 2007, 178, 2308–2313 CrossRef PubMed
.
- G. Novara, R. S. Svatek, P. I. Karakiewicz, E. Skinner, V. Ficarra, Y. Fradet, Y. Lotan, H. Isbarn, U. Capitanio, P. J. Bastian, W. Kassouf, H. M. Fritsche, J. I. Izawa, D. Tilki, C. P. Dinney, S. P. Lerner, M. Schoenberg, B. G. Volkmer, A. I. Sagalowsky and S. F. Shariat, J. Urol., 2010, 183, 2165–2170 CrossRef PubMed
.
-
M. C. M. Grimbergen, C. F. P. van Swol, R. O. P. Draga, P. van Diest, R. M. Verdaasdonk, N. Stone and J. H. L. R. Bosch, in Proc. SPIE, ed. N. Kollias, B. Choi, H. Zeng, R. S. Malek, B. J. Wong, J. F. R. Ilgner, K. W. Gregory, G. J. Tearney, L. Marcu, H. Hirschberg and S. J. Madsen, 2009, vol. 7161, p. 716114 Search PubMed
.
- R. O. P. Draga, M. C. M. Grimbergen, P. L. M. Vijverberg, C. F. P. Van Swol, T. G. N. Jonges, J. A. Kummer and J. L. H. Ruud Bosch, Anal. Chem., 2010, 82, 5993–5999 CrossRef CAS PubMed
.
- E. Ruijter, C. Van De Kaa, G. Miller, D. Ruiter, F. Debruyne and J. Schalken, Endocr. Rev., 1999, 20, 22–45 CrossRef CAS PubMed
.
- K. Eichler, S. Hempel, J. Wilby, L. Myers, L. M. Bachmann and J. Kleijnen, J. Urol., 2006, 175, 1605–1612 CrossRef PubMed
.
- N. Mottet, J. Bellmunt, M. Bolla, E. Briers, M. G. Cumberbatch, M. De Santis, N. Fossati, T. Gross, A. M. Henry, S. Joniau, T. B. Lam, M. D. Mason, V. B. Matveev, P. C. Moldovan, R. C. N. van den Bergh, T. Van den Broeck, H. G. van der Poel, T. H. van der Kwast, O. Rouvière, I. G. Schoots, T. Wiegel and P. Cornford, Eur. Urol., 2016, 71, 1–12 Search PubMed
.
- T. Schlomm, P. Tennstedt, C. Huxhold, T. Steuber, G. Salomon, U. Michl, H. Heinzer, J. Hansen, L. Budäus, S. Steurer, C. Wittmer, S. Minner, A. Haese, G. Sauter, M. Graefen and H. Huland, Eur. Urol., 2012, 62, 333–340 CrossRef PubMed
.
- M. Li, S. R. Banerjee, C. Zheng, M. G. Pomper and I. Barman, Chem. Sci., 2016, 7, 6779–6785 RSC
.
- R. E. Kast, S. C. Tucker, K. Killian, M. Trexler, K. V. Honn and G. W. Auner, Cancer Metastasis Rev., 2014, 33, 673–693 CrossRef CAS PubMed
.
- C. A. Patil, I. J. Pence, C. A. Lieber and A. Mahadevan-Jansen, Opt. Lett., 2014, 39, 303–306 CrossRef CAS PubMed
.
- I. J. Pence, C. A. Patil, C. A. Lieber and A. Mahadevan-Jansen, Biomed. Opt. Express, 2015, 6, 2724–2737 CrossRef CAS PubMed
.
- S. Duraipandian, W. Zheng, J. Ng, J. J. H. Low, A. Ilancheran and Z. Huang, J. Biomed. Opt., 2013, 18, 67007 CrossRef PubMed
.
- J. Desroches, M. Jermyn, K. Mok, C. Lemieux-Leduc, J. Mercier, K. St-Arnaud, K. Urmey, M.-C. Guiot, E. Marple, K. Petrecca and F. Leblond, Biomed. Opt. Express, 2015, 6, 2380–2397 Search PubMed
.
- O. Stevens, I. E. Iping Petterson, J. C. C. Day and N. Stone, Chem. Soc. Rev., 2016, 45, 1919–1934 RSC
.
- J. Wang, M. S. Bergholt, W. Zheng and Z. Huang, Opt. Lett., 2013, 38, 2321–2323 CrossRef CAS PubMed
.
- J. Wang, K. Lin, W. Zheng, K. Y. Ho, M. Teh, K. G. Yeoh and Z. Huang, Anal. Bioanal. Chem., 2015, 407, 8303–8310 CrossRef CAS PubMed
.
- S. Duraipandian, M. S. Bergholt, W. Zheng, K. Y. Ho, M. Teh, K. G. Yeoh, J. B. Y. So, A. Shabbir and Z. Huang, J. Biomed. Opt., 2012, 17, 81418 CrossRef PubMed
.
- M. Agenant, M. Grimbergen, R. Draga, E. Marple, R. Bosch and C. van Swol, Biomed. Opt. Express, 2014, 5, 1203–1216 CrossRef PubMed
.
- G. J. Puppels, Cancer Res., 2017, 77, 2775–2778 CrossRef PubMed
.
- S. Takamori, K. Kong, S. Varma, I. Leach, H. C. Williams and I. Notingher, Biomed. Opt. Express, 2015, 6, 98 CrossRef PubMed
.
- I. P. Santos, A. van der Lee, X. Gu, P. J. Caspers, T. C. Bakker Schut, R. van Doorn, V. Noordhoek Hegt, S. Koljenović and G. J. Puppels, J. Raman Spectrosc., 2017, 48, 872–877 CrossRef CAS
.
-
M. Kuznetsov, in Semiconductor Disk Lasers. Physics and Technology, ed. O. G. Okhotnikov, WILEY-VCH, Weinheim, 2010 Search PubMed
.
- W. P. Pallmann, C. A. Zaugg, M. Mangold, V. J. Wittwer, H. Moench, S. Gronenborn, M. Miller, B. W. Tilma, T. Südmeyer and U. Keller, Opt. Express, 2012, 20, 24791 CrossRef CAS PubMed
.
- J. Q. Nguyen, Z. S. Gowani, M. O'Connor, I. J. Pence, T. Q. Nguyen, G. E. Holt, H. S. Schwartz, J. L. Halpern and A. Mahadevan-Jansen, Lasers Surg. Med., 2016, 48, 774–781 CrossRef PubMed
.
- D. A. Orringer, B. Pandian, Y. S. Niknafs, T. C. Hollon, J. Boyle, S. Lewis, M. Garrard, S. L. Hervey-Jumper, H. J. L. Garton, C. O. Maher, J. A. Heth, O. Sagher, D. A. Wilkinson, M. Snuderl, S. Venneti, S. H. Ramkissoon, K. A. McFadden, A. Fisher-Hubbard, A. P. Lieberman, T. D. Johnson, X. S. Xie, J. K. Trautman, C. W. Freudiger and S. Camelo-Piragua, Nat. Biomed. Eng., 2017, 1, 27 CrossRef
.
- K. St-Arnaud, K. Aubertin, M. Strupler, M. Jermyn, K. Petrecca, D. Trudel and F. Leblond, Opt. Lett., 2016, 41, 4692–4695 CrossRef CAS PubMed
.
- M. Jermyn, J. Desroches, J. Mercier, K. Saint-Arnaud, M.-C. Guiot, F. Leblond and K. Petrecca, Biomed. Opt. Express, 2016, 7, 247–248 Search PubMed
.
- J. Desroches, A. Laurence, M. Jermyn, M. Pinto, M.-A. Tremblay, K. Petrecca and F. Leblond, Analyst, 2017, 142, 1185–1191 RSC
.
- M. Jermyn, J. Desroches, J. Mercier, M.-A. Tremblay, K. St-Arnaud, M.-C. Guiot, K. Petrecca and F. Leblond, J. Biomed. Opt., 2016, 21, 94002 CrossRef PubMed
.
- M. Isabelle, J. Dorney, A. Lewis, G. R. Lloyd, O. Old, N. Shepherd, M. Rodriguez-Justo, H. Barr, K. Lau, I. Bell, S. Ohrel, G. Thomas, N. Stone and C. Kendall, Faraday Discuss., 2016, 187, 87–103 RSC
.
- R. Gaifulina, A. T. Maher, C. Kendall, J. Nelson, M. Rodriguez-Justo, K. Lau and G. M. Thomas, Int. J. Exp. Pathol., 2016, 97, 337–350 CrossRef CAS PubMed
.
-
K. Lau, M. Isabelle, G. R. Lloyd, O. Old, N. Shepherd, I. M. Bell, J. Dorney, A. Lewis, R. Gaifulina, M. Rodriguez-Justo, C. Kendall, N. Stone, G. Thomas and D. Reece, in Biomedical Vibrational Spectroscopy, ed. A. Mahadevan-Jansen and W. Petrich, 2016, vol. 9704, p. 97040B Search PubMed
.
- M. A. Short, W. Wang, I. T. Tai and H. Zeng, J. Biophotonics, 2016, 9, 44–48 CrossRef CAS PubMed
.
- J. Zhao, H. Lui, D. I. McLean and H. Zeng, Appl. Spectrosc., 2007, 61, 1225–1232 CrossRef CAS PubMed
.
- J. Zhao, H. Lui, D. I. McLean and H. Zeng, Skin Res. Technol., 2008, 14, 484–492 CrossRef PubMed
.
- H. Abramczyk, B. Brozek-Pluska, J. Surmacki, J. Musial and R. Kordek, Analyst, 2014, 139, 5547–5559 RSC
.
- L. Mavarani, D. Petersen, S. F. El-Mashtoly, A. Mosig, A. Tannapfel, C. Kötting and K. Gerwert, Analyst, 2013, 138, 4035–4039 RSC
.
- T. Tolstik, C. Marquardt, C. Matthäus, N. Bergner, C. Bielecki, C. Krafft, A. Stallmach and J. Popp, Analyst, 2014, 139, 6036–6043 RSC
.
- G. Lech, R. Słotwiński, M. I. Słodkowski and I. W. Krasnodębski, World J. Gastroenterol., 2016, 22, 1745–1755 CrossRef CAS PubMed
.
- J. Wang, K. Lin, W. Zheng, K. Ho, M. Teh, K. Yeoh and Z. Huang, Faraday Discuss., 2015, 187, 377–392 RSC
.
- S. Devpura, K. N. Barton, S. L. Brown, O. Palyvoda, S. Kalkanis, V. M. Naik, F. Siddiqui, R. Naik and I. J. Chetty, Med. Phys., 2014, 41, 50901 CrossRef PubMed
.
- K. Kong, F. Zabar, E. Rakha, I. Ellis, A. Koloydenko and I. Notingher, Phys. Med. Biol., 2014, 59, 6141–6152 CrossRef PubMed
.
Footnote |
† These authors contributed equally to this work. |
|
This journal is © The Royal Society of Chemistry 2017 |