DOI:
10.1039/C8LC01153B
(Paper)
Lab Chip, 2019,
19, 1071-1081
Formation of precisely composed cancer cell clusters using a cell assembly generator (CAGE) for studying paracrine signaling at single-cell resolution†
Received
25th October 2018
, Accepted 12th February 2019
First published on 14th February 2019
Abstract
The function and behaviour of any given cell in a healthy tissue, or in a tumor, is affected by interactions with its neighboring cells. It is therefore important to create methods that allow for reconstruction of tissue niches in vitro for studies of cell–cell signaling and associated cell behaviour. To this end we created the cell assembly generator (CAGE), a microfluidic device which enables the organization of different cell types into precise cell clusters in a flow chamber compatible with high-resolution microscopy. In proof-of-concept paracrine signalling experiments, 4-cell clusters consisting of one pancreatic β-cell and three breast cancer cells were formed. It has previously been established that extracellular ATP induces calcium (Ca2+) release from the endoplasmic reticulum (ER) to the cytosol before it is cleared back into the ER via sarcoplasmic/ER Ca2+ ATPase (SERCA) pumps. Here, ATP release from the β-cell was stimulated by depolarization, and dynamic changes in Ca2+ levels in the adjacent cancer cells measured using imaging of the calcium indicator Fluo-4. We established that changes in the concentration of cytosolic Ca2+ in the cancer cells were proportional to the distance from the ATP-releasing β-cell. Additionally, we established that the relationship between distance and cytosolic calcium changes were dependent on Ca2+-release from the ER using 5-cell clusters composed of one β-cell, two untreated cancer cells and two cancer cells pretreated with Thapsigargin (to deplete the ER of Ca2+). These experiments show that the CAGE can be used to create exact cell clusters, which affords precise control for reductionist studies of cell–cell signalling and permits the formation of heterogenous cell models of specific tissue niches.
Introduction
There is a continued need for better in vitro methods for the study of human cell clusters representing distinct tissue niches.1,2 Cells in all tissues send and receive messages in the form of direct contacts and secreted signaling molecules. These messages affect cell behavior in the broadest sense throughout animal life; from the first cell divisions during embryonic development to adult tissue homeostasis, as well as in situations of tissue damage or disease.
The heterogeneity of tumors derived from the inherent genomic instability of cancer cells influences both tumor development and responsiveness to specific treatments.3,4 Following their escape from the primary tumor, individual cancer cells can enter secondary sites. Complex heterotypic cell–cell interactions can occur between the cancer cells and the healthy cells that reside in the affected tissue,5 and the outcome of these interactions may well determine whether or not a metastasis will be established. Cancer cell responses to paracrine stimulation include cell migration,6 increased metastatic behaviour7–9 and growth, while cancer cell signaling to its surroundings increases tumor angiogenesis,10–13 immunosuppression,14 and chemoresistance.15 Consequently, elucidating the impact of paracrine signaling on cancer cells, and the communication between cancer cells and surrounding healthy cells, will aid in our understanding of tumor establishment in tissue-specific microenvironments.5,16
3D tumor spheroid models have been extensively used in basic research for experimental studies of tumor growth17–19 and drug screening.20,21 Spheroid models are especially well suited for the study of the collective behavior and interaction of cancer cells with specific non-cancer cell types. Nonetheless, it is practically speaking impossible in the currently available spheroid systems to resolve the impact of a single cell's paracrine signal on cancer cell behavior. Furthermore, the spheroid system does not permit modeling of the responsiveness of cancer cells that are acting independently of the primary tumor. For example, when cancer cells enter secondary tissue sites they encounter new cell-type specific paracrine signals, which may induce responses that are distinct from those of cells that remain at the primary tumor site. Ultimately, these responses will play a role in determining the behavior of the tumor cell at this secondary microsite, so studying such interactions will contribute to our understanding of the early stages of metastatic colonization. To study interactions with this level of resolution requires assays that permit the precise assembly of heterotypic cell groups.
A number of in vitro assays exist to study single cells.22–35 Whereas these assays represent robust tools for biological assays that focus on single cell stimulation, the same assays do not find use in models aiming to study multicellular behavior. Cell pairing assays36–41 have also been developed, that focus on the interplay between two adjacent cells, however the designs and principles used for cell pairing assays are not readily applicable for the study of larger and isolated cell assemblies. Methods have also been created for generating large multicellular clusters, in some instances with high-throughput capabilities.42–49 However, in many of these approaches the cluster composition is subject to a degree of randomness, which can complicate the interpretation of observed effects, as such clusters may not be readily compared. Optically trapping,50–52 positioning53 and manipulating54 living cells has proved to be both precise and reliable. However, the approach still relies on fluid handling components (e.g. pumps) if cell stimulation or long-term culture is to be performed and additionally relies on expensive equipment.
Assays developed for the study of detailed cell interactions in cell clusters should be capable of establishing reproducible clusters composed of exactly the same number and types of cells, with the possibility to treat the cluster after its formation and provide for a suitable environment for a cell. As many paracrine signaling events occur quickly, and are transient, the assay should also be fully compatible with analysis by high-resolution time-lapse microscopy.
Here we present a device called the cell assembly generator (CAGE), which is a microfluidic system that can be used to create precisely composed cell clusters. The CAGE can be used to form multiple identical cell clusters, containing different types of cells, which can be stimulated after formation and live imaged with high-resolution microscopy. Ca2+ is a key regulator of transcription, proliferation, migration and cell death, and Ca2+ signaling is known to be altered in cancer cells.55 Inputs that induce changes in Ca2+ signaling in cancer cells will therefore also alter the behavior of these cells. This is of particular relevance to metastatic cells that will be exposed to new environments that may result in aberrant Ca2+ signaling. In the present study the CAGE was applied to spatially resolve Ca2+ dynamics in 3 or 4 individual breast cancer cells relative to a paracrine ATP signal selectively released from a single pancreatic β-cell source. Although metastases in the pancreatic islets are known to occur,56 this should mostly be seen as a model system for the impact of ATP and purinergic signaling on breast cancer cells. ATP release is a common response in all secretory cells,57 and therefore a messenger that cancer cells will be exposed to in many locations in the body. The ability to assemble precise constellations of cell clusters and live image them at high-resolution in the isolated microenvironment afforded by the CAGE, allowed us to observe that changes in cytosolic Ca2+ in individual cancer cells was proportional to their distance from the single ATP-releasing β-cell.
Results and discussion
Design of the CAGE chip and system overview
The CAGE chip contains hydrodynamic single-cell traps that are connected to separate clustering chambers. Defined multicellular clusters are built one cell at a time by the repeated capture of individual cells in the hydrodynamic cell traps, followed by ejection and transport into the clustering chambers. The chip is composed of three traps with three adjacent clustering chambers (Fig. 1). Inlets and outlets permit cell loading and treatment administration. Note that the number of single-cell traps and corresponding clustering chambers per chip could be increased according to the needs of the user.
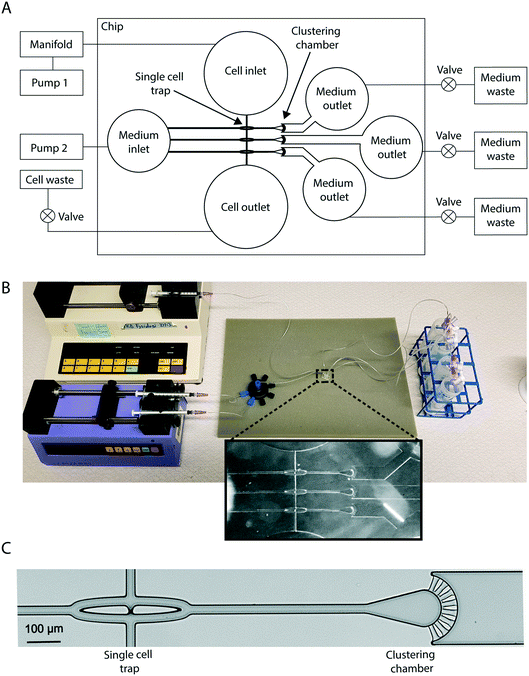 |
| Fig. 1 Schematic illustration of the system and the microfluidic chip. (A) Overview of the CAGE chip and its connections to the other system components (pumps, valves, and manifold). (B) Picture of the setup. The inset shows a magnification of the central part of the CAGE chip. (C) Image showing one of the single-cell traps in the CAGE chip together with the corresponding clustering chamber, with its eight outward radiating fluidic channels present in the fan-shaped cell restrainer. | |
System operation
To permit cluster formation the system has a cell-trapping mode and a cell-ejecting mode. These operations are completed with the aid of two external pumps connected to the cell and medium inlets, and stopcock valves connected to a cell outlet, and three medium outlets (Fig. 1). In trapping mode, flow was directed to the central trapping region of the chip by activating syringe pump 1 and opening the cell outlet, while the medium outlets were closed. The syringe pump was equipped with syringes that each contained a unique cell population for loading into the chip. The syringes were connected to a manifold that made it possible to seamlessly switch between loading of the different cell populations. Cells were pumped into the chip via the cell inlet and flowed through a microchannel towards the three aligned single-cell traps (Fig. 1).
Hydrodynamic single cell trapping
All fluidic channels in the chip were designed to be 25 μm high and 25 μm wide. These dimensions were selected so that the cells flown through the system (measured to be approximately 20 μm in diameter in suspension) go through the channels in single file. The hydrodynamic single-cell traps have a 2 μm wide pore centrally located at their base that allows for fluid flow through the trap, but is too narrow to permit cell passage (Fig. 1C and 2). A finite element method (FEM) simulation was used to demonstrate fluid flows through the trap pore as well as laterally along paths that circumvent the trap, prior to cell capture (Fig. 2A). Cells that follow the central flow path enter the cell trap and are immobilized (ESI,† Movie M1). The trapped cell plugs the trap pore, which eliminates the central flow path and ensures that cells will now circumvent the populated trap via the lateral flow paths (Fig. 2A). The single cell traps were typically occupied within seconds of the first cells entering the chip, but as the likelihood of capturing a cell is equal for all three traps the order of trap occupation varied (Fig. 2B); however, as the ejection process is only initiated once all the traps are occupied, the order of trap occupation does not influence cluster formation. Importantly, untrapped cells can be recovered unharmed via the cell outlet, which is advantageous for studies in which cell availability is limited.
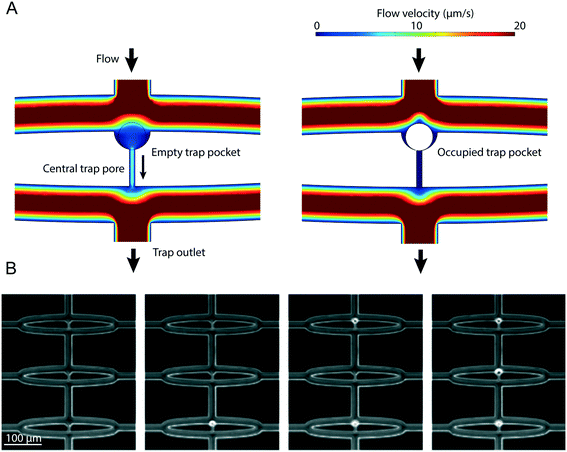 |
| Fig. 2 Overview of flow dynamics and operation of the single-cell trap. (A) FEM simulation of the flow velocities (μm s−1; color-coded) in and around the hydrodynamic single-cell trap with an empty (left) or occupied (right) trap pocket. Occupation of the trap prevents fluid flow through the centrally located trap pore. (B) Image sequence showing the trapping of three individual cells in the three serially connected traps. | |
Formation of precisely composed multicellular clusters
To eject cells from the hydrodynamic single-cell traps to the clustering chambers, pump 1 is turned off and the cell outlet valve closed, all three medium outlet valves opened and pump 2 activated (Fig. 1A). The flow from the medium inlet to the medium outlets (Fig. 3) ejects the cells from each of the three traps into the fluidic channels that lead to their respective clustering chambers (Fig. 3C, Movie M1†). The clustering chamber extends from the fluidic channel and expands to form a teardrop-shaped reservoir with a height of 25 μm (Fig. 4A). Each clustering chamber ends in a fan-shaped cell restrainer holding eight channels (each 2 μm high) that connect the chamber to the adjacent medium outlet. These channels allow for cell medium and treatment solutions to flow through the clustering chamber, but are suitably small to ensure that cells remain within the confines of the chamber (Fig. 4A). Multiple rounds of trapping and ejecting enabled the sequential formation of precisely defined cell clusters (Fig. 4B). Using multiple rounds of trapping and two different cell populations (MCF7 breast cancer cells that had been fluorescently labeled green or red) the clustering efficiency was evaluated (Fig. 4C and D). Of the 21 attempted cluster assemblies, all were successful in forming two-cell clusters of the first cell population, 86% succeeded in adding the first cell from the second cell population to form a three-cell cluster, and 67% formed complete 4-cell clusters. After the switch to the second cell population, the following malfunctions were observed in the 33% of cases that the intended 4-cell clusters were not formed (Fig. 4D). In 57% of the malfunctioning cases, leftover cells from the first population were still present in the system and occasionally re-occupied a trap, and this prevented the creation of a cluster with the desired composition. In 29% of cases the malfunction was a failure to eject the cell from the trap thus not adding it to the cluster. Finally, 14% of the malfunctions were due to two cells being trapped and ejected at once, thus adding an extra cell to the cluster. On average this would correspond to that 2 out of 3 of the clustering chambers on any given CAGE chip would contain the intended cluster constellation.
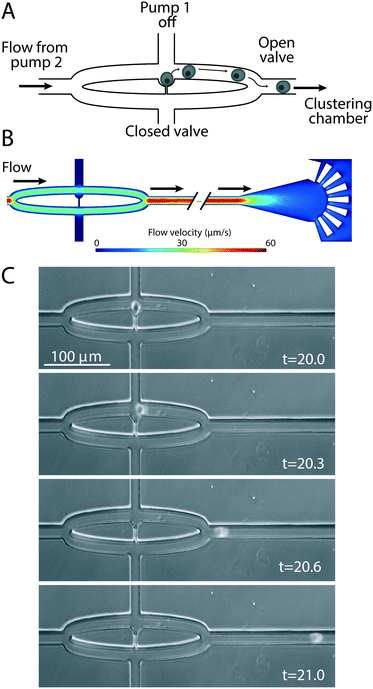 |
| Fig. 3 Ejection of cells from single-cell traps. (A) Illustration of the ejection of a trapped cell from a single-cell trap. Block arrows denote the flow paths. In the cell ejection mode, pump 1 is switched off and the cell outlet valve is closed. Pump 2 is switched on and the cell is exposed to flow from the medium inlet, which dislodges the cell from the trap and carries it towards the clustering chamber. (B) FEM simulation of the flow velocities in the single-cell trap, transport channel and clustering chamber during ejection of captured cells. (C) Time-lapse images of the cell ejection process 20 s after that flow had been established. | |
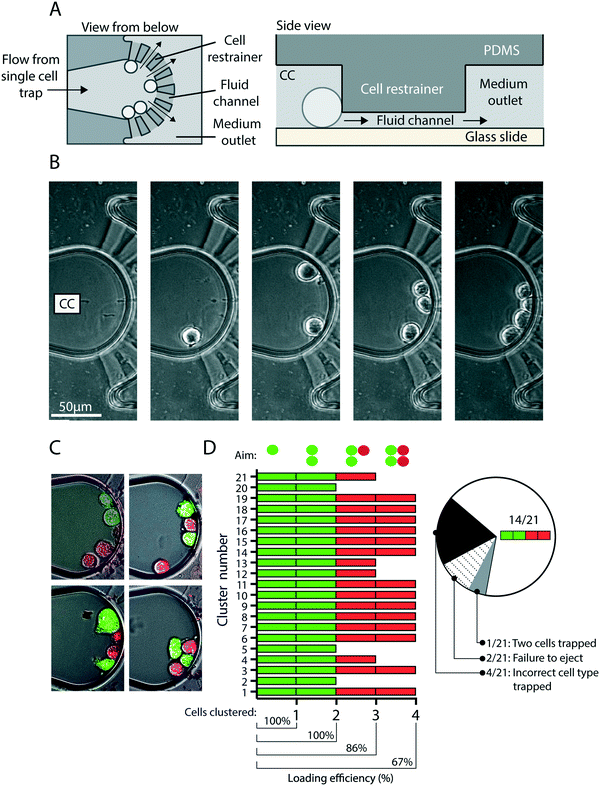 |
| Fig. 4 Formation of specific cell clusters one cell at a time. (A) Illustrations of the clustering chamber (CC) as viewed from below and from the side. The position of the cell restrainer (holding eight channels enabling perfusion but preventing cells from going through) and the medium outlet are indicated. Cells are shown as white circles, arrows indicate the direction of flow through the clustering chamber. (B) Consecutive images from the same clustering chamber. The empty chamber is filled incrementally with individual cells through cycles of trapping and ejecting, which permitted the formation of a 4-cell cluster of MCF7 cells. (C) Examples of 4-cell MCF7 cell clusters derived from two different cell populations stained green or red with Celltracker dye. (D) Quantification of the efficiency of clustering at each step in the formation of 4-cell MCF7 clusters; the green cells were loaded first, followed by the red cells (n = 21). A pie chart detailing the failure modes is shown. | |
The cell types tested in this study are adhesive and we investigated whether cell adhesion to the cover glass substrate used in the CAGE could be established within the timeframe of cluster formation (approximately 20 minutes). MCF7 cells were added to a culture dish containing a cover glass and within 15 minutes typically established a sufficient degree of attachment to resist movement caused by fluid flow (Fig. S1A†). Additionally, the conditions within the cluster chamber were compatible with longer durations of cell culture, during which more extensive cell spreading and migration were observed (Fig. S1B†).
Paracrine-induced calcium signaling in breast cancer cells by a single pancreatic β-cell
As mentioned above, though a relatively rare event the pancreas is targeted by metastases derived from a number of primary tumor types, including breast cancer.56 Pancreatic β-cells are an essential functional unit of the endocrine pancreas and, in response to membrane depolarization, the β-cells release insulin and other signaling molecules, including ATP. We applied the CAGE to assemble 4-cell clusters consisting of a single clonal MIN6 β-cell and three MCF7 breast cancer cells, to analyze the paracrine effects of β-cell ATP release on the Ca2+ dynamics in the adjacent cancer cells. We first defined the signaling responses of the MIN6 and MCF7 cells by conducting a number of control experiments using cells grown on cover glass, that was transferred to an open imaging chamber, superfused with medium supplemented as indicated, and analyzed by TIRF microscopy (ESI,† Fig. S2). Membrane depolarization of excitable MIN6 cells with 30 mM K+ triggered a rapid increase in the cytosolic Ca2+ concentration by voltage-dependent influx,58 which can be visualized with the Ca2+ indicator Fluo-4 (Fig. S2A†). The rise of Ca2+ triggers insulin granule fusion and release of content. Besides insulin, the granules are rich in ATP, which will bind P2Y1 purinoceptors of the β-cell surface and initiate autocrine signaling. This involves conversion of PI(4,5)P2 to diacylglycerol (DAG) and inositol triphosphate (IP3). IP3 in turn stimulates release of ER-stored Ca2+ to the cytoplasm.59 In MIN6 cells, DAG production (and consequently Ca2+ release) was blocked by the P2Y1 receptor inhibitor MRS2179 (ref. 60) demonstrating that depolarization triggers ATP release from MIN6 cells (Fig. S2B†). As expected, no pronounced changes in Fluo-4 fluorescence were observed in response to depolarization in the non-excitable MCF7 cells (Fig. S2C†). In contrast, Fluo-4 fluorescence in MCF7 cells increased in response to exogenously added ATP (as MCF7 cells do express P2Y receptors61) and decreased to below baseline once the ATP stimulus was removed (Fig. S2D and E†). Ca2+ is cleared from the cytosol back into the ER via the sarcoplasmic/ER Ca2+ ATPase (SERCA) pump.62 Application of the SERCA inhibitor Thapsigargin caused a gradual increase in Fluo-4 fluorescence in MCF7 cells (Fig. S2D and E†), this is due to passive leakage of the ion from the ER that is unmasked in the absence of SERCA activity. This eventually leads to depletion of ER-stored Ca2+, which was evident in MCF7 cells as Thapsigargin treatment rendered these cells insensitive to ATP stimulation (Fig. S2D and E†).
In the CAGE we confined a single MIN6 β-cell, serving as a paracrine source of K+-induced ATP, with three MCF7 breast cancer cells as ATP-responders (Fig. 5A). The MCF7 cells were pre-stained with the blue nuclear stain Hoechst to distinguish them from the unstained MIN6 cell (Fig. 5B), and intracellular changes in cytosolic Ca2+ monitored in all 4 cells with Fluo-4. Time-lapse imaging of Fluo-4 fluorescence in the assembled clusters was performed 20 s after depolarization with K+ and for a duration of 500 s (Fig. 5C). Following the treatment with K+ all flow was stopped in the chip for the entire duration of the imaging.
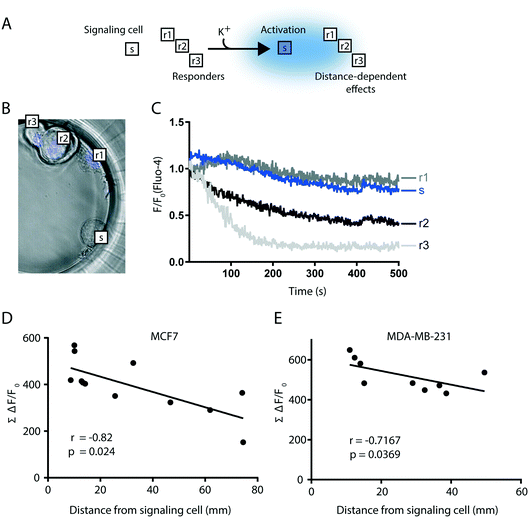 |
| Fig. 5 Formation of 4-cell clusters to study Ca2+ dynamics in MCF7 cancer cells responding to a paracrine signal derived from a single MIN6 cell. (A) Schematic representation of a 4-cell cluster comprised of a single MIN6 paracrine signaling cell (s) and three MCF7 responder cells (r). K+ stimulation induces ATP release (shown as blue gradient) from the MIN6 cell, and distance dependent effects on Ca2+ dynamics are imaged in the MCF7 cells. (B) 4-Cell cluster composed of a single MIN6 (s) and 3 MCF7 cells (r1–r3) in the CAGE. The identity of the MCF7 cells was confirmed by pre-staining with Hoechst (nuclear stain; blue). (C) Fluo-4 fluorescence was imaged for a total of 500 s, starting 20 s after K+ perfusion was initiated. Fold change in Fluo-4 fluorescence was plotted for every cell in the cluster over time. (D) Accumulated fluorescence change (AFC = ∑ΔF/Fo) was calculated for each MCF7 cell and its distance from the individual MIN6 cell present in each cluster was measured. The Fluo-4 AFC for 12 MCF7 cells from 4 independent clusters were plotted against distance (μm) to their MIN6 source and correlation analysis was performed. (E) The experiments in B–D were repeated with clusters formed from three MDA-MB-231 breast cancer cells with a single MIN6 signaling source. The Fluo-4 AFC for 9 MDA-MB-231 cells from 3 independent clusters was plotted against distance (μm) to their MIN6 source and correlation analysis was performed. The line in each plot (D and E) corresponds to a linear interpolation from all data points. | |
Direct comparison of Fluo-4 fluorescence between cells is complicated by the fact that cells exhibit differential degrees of Fluo-4 uptake. To circumvent this limitation we quantified intracellular changes in Ca2+ levels by calculating the area under the curve of Fluo-4 fluorescence in each MCF7 over time (Fig. 5C), which represents accumulated fluorescence change (AFC = ∑(ΔF/F0)). Additionally, the distance between each MCF7 responder cell and the signaling MIN6 cell was measured. We found that the greatest Fluo-4 AFC was observed in MCF7 responders in closest proximity to the MIN6 source of ATP (Fig. 5D). This effect was replicated using the breast cancer cell line MDA-MB-231 (Fig. 5E). Furthermore, correlation analysis revealed a statistically significant relationship between the distance from the MIN6 cell and the Fluo-4 AFC, for both MCF7 and MDA-MB-231 responder cells (Fig. 5D and E). In addition, by determining the basal AFC of cancer cells in clusters with no β-cells and applying that value to the previously described correlation models in Fig. 5D and E, a distance can be identified that can be used to categorize the cells into high and low responders (Fig. S3†).
Given the timing of our imaging protocol the Fluo-4 AFC represents changes to Ca2+ that occur after ATP has stimulated ER release of Ca2+ to the cytosol of MCF7 cells. Therefore, we considered that Fluo-4 AFC is predominantly a measure of Ca2+ clearance from the cytosol to the ER. To specifically investigate the importance of Ca2+ clearance in the observed distance-dependent effects (Fig. 5D and E), we again employed the SERCA inhibitor Thapsigargin. In the CAGE, 5-cell clusters composed of a single MIN6 β-cell, 2 untreated MCF7 cells, and 2 Thapsigargin-treated MCF7 cells were formed (Fig. 6A). Thapsigargin-treated cells revealed lower Fluo-4 AFC in response to MIN6-derived ATP (Fig. 6B) and while the distance from MIN6 correlated as before with Fluo-4 AFC in the untreated MCF7 cells (Fig. 6C), there was no such correlation for the Thapsigargin-treated MCF7 cells (Fig. 6D). Together these data reveal the capacity of the CAGE to facilitate detection of spatially determined differences in the dynamics of Ca2+ clearance in individual cancer cells following paracrine signaling from a single β-cell.
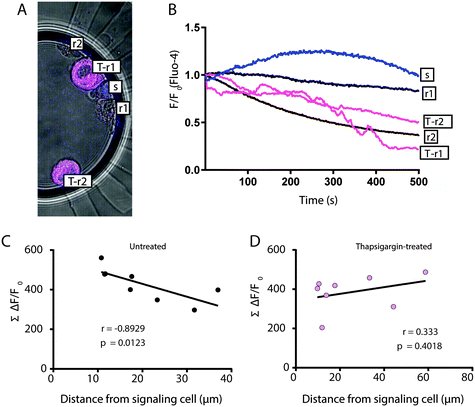 |
| Fig. 6 Formation of 5-cell clusters to determine the contribution of Ca2+ clearance to the MCF7 Ca2+ response to MIN6-derived ATP. (A) Example of a 5-cell cluster formed by the assembly of a single MIN6 signaling source (s), two untreated MCF7 cells (r1 and r2), and two Thapsigargin-treated MCF7 cells (T-r1, and T-r2). The identity of the MIN6 source cell was confirmed by pre-staining with Hoechst (presented in blue), and Thapsigargin-treated MCF7 cells were pre-stained with Celltracker dye (presented in magenta). (B) Fluo-4 fluorescence was imaged for a total of 500 s, starting 20 s after K+ perfusion was initiated. Fold change in Fluo-4 fluorescence was plotted for every cell in the cluster over time. (C and D) Accumulated fluorescence change was calculated for untreated and Thapsigargin-treated MCF7 cells and their distance from each clusters MIN6 cell was measured. The Fluo-4 AFC for 8 untreated MCF7 cells (C) and 8 Thapsigargin-treated MCF7 cells (D) from 4 independent clusters were plotted against distance (μm) to their MIN6 source and correlation analysis performed. The line in each plot corresponds to linear interpolation from all data points. | |
Whereas our main focus here was on Ca2+ clearance in cancer cells subsequent to the paracrine stimulation by MIN6-derived ATP (Fig. 5 and 6), the CAGE was also applied to visualize earlier Ca2+ signaling events, occurring during the K+ stimulation phase (Fig. S4†). In these experiments, fine-focus adjustments were occasionally required due to small movements of the cells in the chamber, caused by the fluid flow during K+ perfusion. Focus-dependent artefacts in Fluo-4 fluorescence were corrected for using Celltracker red, which served as a reporter for Ca2+-independent changes in fluorescence (Fig. S4†). This application of the CAGE is beneficial for the study of paracrine and/or autocrine signaling events that specifically occur in rapid response to a stimulus.
Manufacture of CAGE chips using 3D-printed molds
The CAGE microfluidic chip was cast from molds generated by photolithography in a cleanroom facility. This requires knowledge of microfabrication and access to relatively expensive equipment, which has limited many users in the life sciences from adopting microfluidics-based technologies that are not commercially available. To address this, we additionally cast CAGE chips from 3D-printed molds produced using a recently developed hybrid system for 3D-printing capable of resolving structures in the low micrometer range (Fig. S5†). CAGE chips cast from silanized 3D-printed molds had similar functional properties to their cleanroom-derived equivalents (Fig. S5†); however, it will be necessary to optimize surface treatments for such molds as they proved to be more susceptible to erosion. Nonetheless, it is clear that the rapid developments within the 3D-printing field soon will enable more widespread application of microfluidic methods for cell studies in general, and for high-resolution studies of tailor-made cell clusters as described here.
Conclusions
The current study describes the design and fabrication of the microfluidic CAGE chip, that can be used to create tailor-made cell clusters for reductionist studies of cell–cell signaling by live imaging. The concept described here provides a basis for modeling tissue niches, built by the sequential trapping of individual cells of interest and ejection into isolated chambers to form precisely composed assemblies that can be treated and imaged. In proof-of-principle experiments the CAGE facilitated the detection of spatially resolved differences in cytosolic Ca2+ clearance from cancer cells relative to a single β-cell serving as a paracrine signalling source. The CAGE provides researchers with the capacity to resolve subtle differences in dynamic signalling events due to the precision with which clusters can be composed and analyzed. Refining our ability to perform detailed quantification of interactions in heterotypic cellular assemblies will undoubtedly expand our knowledge of cell biology in various contexts, including the repertoire of responses elicited by cancer cells in response to signalling events in specific microenvironments.
Materials and methods
Production of the CAGE chip
The mold for production of the CAGE chip was created using photolithography and SU8 negative photoresist. Briefly, after initial surface treatment of the silica wafer, a 2 μm thick layer was created using spin coating of SU-8 2002 (MicroChem, Newton, MA, USA). A photo mask encoding mold structures for the cell restrainer was first used and the photoresist crosslinked using a 12 mJ mercury lamp and cured using a heat plate. Similarly, a second layer was created using SU-8 50 (MicroChem, Newton, MA, USA) and a second photo mask, to create 25 μm thick mold structures coding for all microfluidic channels and chambers, as well as the hydrodynamic single cell traps. Finally, the mold was hard-baked at 150 °C for 45 min.
After the fabrication of the mold, PDMS (Sylgard 184, Sigma-Aldrich Sweden AB, Stockholm, Sweden) was cast into the mold, degassed for 30 min, and cured at 80 °C for 1 h. The PDMS piece was removed from the mold and holes corresponding to the different inlets and outlets of the chip created using a 1.5 mm biopsy puncher. The PDMS piece was then bonded to a glass slide (Superfrost, VWR, Stockholm, Sweden) after corona plasma treatment (Model BD-20, Electro-Technic Products Inc., Chicago, IL, USA). Before use, the microfluidic network in the chip was primed with ethanol, and washed extensively with PBS. Gelatin coating was used exclusively in the proof of adhesion experiments (Fig. S1†), to investigate cell adhesion in a more physiological context than the one presented by untreated glass. The coating was carried out for 30 minutes with 0.1% gelatin prior to cell loading.
Cell culture
MCF7 breast cancer cells, MDA-MB-231 breast cancer cells (ATCC, Manassas, VA, USA) and MIN6 insulinoma β-cells (a gift from Prof. Ken-ichi Yamamura63) were grown in Dulbecco's Modified Eagle's medium (DMEM; Thermo Fisher Scientific, Uppsala, Sweden) supplemented with 10% fetal bovine serum (FBS; Thermo Fisher Scientific).
CAGE operation
Syringe pumps (Harvard Apparatus Model 22 Syringe Pump 980532, Holliston, MA, USA) fitted with 1 ml syringes (VWR) were used for the operation of the CAGE chip. Polyethylene tubing with an inner diameter of 0.8 mm (VWR) was used for connecting the pumps to the inlets and outlets of the chip, to stopcock valves (3-way stopcock, Cole Palmer, BergmanLabora AB, Danderyd, Sweden) and to a 9-port microfluidic manifold (Darwin Microfluidics, Paris, France). Cells were loaded at a concentration of 106 cells per ml. Flow speeds for both the capture and the ejection modes were selected to ensure fast loading without compromising the function of the device or the integrity of the cells. A flow speed of 5 μl min−1 was used for single-cell trapping, and a flow speed of 15 μl min−1 was used for ejection of cells from the single-cell traps into the clustering chambers. Using the aforementioned flow speeds, the formation of 4-cell clusters was done in under 20 minutes. No temperature control was used for the duration of the experiments.
Flow simulations
All flow simulations were performed using the Comsol Multiphysics Modeling Software (Comsol, LA, CA, USA) using a creeping flow model and approximating for shallow channels.
Cell treatments
Prior to seeding in the CAGE chip cells were detached from culture flasks using Trypsin (Trypsin–EDTA, Thermo Fisher Scientific), pelleted by centrifugation, and then suspended in DMEM, 10% FBS, supplemented with 1% penicillin–streptomycin-glutamine (Thermo Fisher Scientific), referred to hereafter as imaging medium. Where specified cells were labeled with 1 μM Celltracker red or green (Thermo Fisher Scientific), with or without 1 mM Hoechst 33342 nuclear stain according to the manufacturer's instructions, followed by pelleting by mild centrifugation and media exchange (wash). For paracrine model experiments cell suspensions were incubated with 1 μM Fluo-4 (F23917, Thermo Fisher Scientific) for 45 min, then washed and resuspended in imaging medium and incubated for a further 1 h. Where indicated, cells were additionally treated with 1 μM Thapsigargin for 5 min prior to loading the cells into the CAGE chip. In the CAGE, membrane depolarization of the MIN6 cell was achieved with a perfusion of 100 mM KCl (50 μl min−1 for 5 seconds). To avoid microparticles aggregating in the cluster chambers all reagents were filtered before use using 0.25 μm pore size syringe filters (#Z741969 Sigma-Aldrich Sweden AB, Stockholm, Sweden).
Microscopy
All imaging was performed within 4 hours after obtaining appropriately stained single-cell suspensions. Imaging was carried out using either an Axiovert 200M fluorescent microscope or an LSM 700 confocal microscope (Carl Zeiss, Jena, Germany) and the AxioVision or Zen software (Carl Zeiss). Images were collected at a rate of 1 image per second for the signaling experiments.
Data analysis and statistics
Fluo-4 fluorescence was analyzed using ImageJ software. Individual cells were selected as regions of interest (ROI) and the average fluorescence value of each ROI was extracted from time-lapse series using the ImageJ multimeasure function. Background fluorescence (average fluorescence from a ROI outside of the clustering chamber) was subtracted from all time points. Fluorescence fold change was calculated by dividing the fluorescence of every cell in every frame, by each cell's fluorescence in the first frame. This permitted comparison between cells, despite differences in absolute fluorescence due to the fact that individual cells exhibited different degrees of Fluo-4 uptake. Accumulated fluorescent change was calculated as the area under the curve of the fluorescent fold change over time. To calculate the area a numerical trapezoid integration was performed. The distance between cells was calculated based on the distance between the epicenters of the regions of interest, as measured using ImageJ. In Fig. S4,† to correct for fine focus adjustments due to turbulence from KCl (K+) perfusion, cells were co stained with Celltracker red, which served as a reference signal. All Fluo-4 reference values were then expressed as a ratio of Fluo-4 to Celltracker red fluorescence. Population means and standard deviations were calculated using Excel (Microsoft). The correlation analysis between accumulated fluorescence in responder cells and distance from the ATP-secreting MIN6 cell was performed in MATLAB (MathWorks Inc., Natick, MA, USA) using a Spearman's correlation test. The linear interpolation lines were calculated in Excel to represent the data trends.
Conflicts of interest
The authors have no conflicts of interest to declare.
Acknowledgements
We thank Carl Andrén Lundahl for technical assistance. This study was funded by grants to JK from the Cancerfonden (CAN 2017/703), to NFK from the European Union's Horizon 2020 research and innovation program under the Marie Sklodowska-Curie grant agreement 642866, and to OI-H from the Swedish Research Council (MH2015-03087) and the Göran Gustafsson Foundation. Gradientech AB provided support in the form of salaries for Nikos Fatsis-Kavalopoulos, but did not have any additional role in the study design, data collection and analysis, decision to publish, or preparation of the manuscript. 3D printing for system design development was performed at U-PRINT: Uppsala University's 3D-printing facility at the Disciplinary Domain of Medicine and Pharmacy.
References
- J. P. Gillet, S. Varma and M. M. Gottesman, J. Natl. Cancer Inst., 2013, 105, 452–458 CrossRef PubMed.
- P. Horvath, N. Aulner, M. Bickle, A. M. Davies, E. Del Nery, D. Ebner, M. C. Montoya, P. Ostling, V. Pietiainen, L. S. Price, S. L. Shorte, G. Turcatti, C. von Schantz and N. O. Carragher, Nat. Rev. Drug Discovery, 2016, 15, 751–769 CrossRef CAS PubMed.
- R. A. Burrell, N. McGranahan, J. Bartek and C. Swanton, Nature, 2013, 501, 338 CrossRef CAS PubMed.
- C. A. Klein, Nature, 2013, 501, 365 CrossRef CAS PubMed.
- S. S. McAllister and R. A. Weinberg, Nat. Cell Biol., 2014, 16, 717 CrossRef CAS PubMed.
- N. M. Hogan, M. R. Joyce, J. M. Murphy, F. P. Barry, T. O'Brien, M. J. Kerin and R. M. Dwyer, Biochem. Biophys. Res. Commun., 2013, 435, 574–579 CrossRef CAS PubMed.
- A. Patsialou, J. Wyckoff, Y. Wang, S. Goswami, E. R. Stanley and J. S. Condeelis, Cancer Res., 2009, 69, 9498–9506 CrossRef CAS PubMed.
- C. Grange, M. Tapparo, F. Collino, L. Vitillo, C. Damasco, M. C. Deregibus, C. Tetta, B. Bussolati and G. Camussi, Cancer Res., 2011, 71, 5346–5356 CrossRef CAS PubMed.
- M. Dohadwala, R. K. Batra, J. Luo, Y. Lin, K. Krysan, M. Põld, S. Sharma and S. M. Dubinett, J. Biol. Chem., 2002, 277, 50828–50833 CrossRef CAS PubMed.
- C. Folkins, Y. Shaked, S. Man, T. Tang, C. R. Lee, Z. Zhu, R. M. Hoffman and R. S. Kerbel, Cancer Res., 2009, 69, 7243–7251 CrossRef CAS PubMed.
- B. Beck, G. Driessens, S. Goossens, K. K. Youssef, A. Kuchnio, A. Caauwe, P. A. Sotiropoulou, S. Loges, G. Lapouge, A. Candi, G. Mascre, B. Drogat, S. Dekoninck, J. J. Haigh, P. Carmeliet and C. Blanpain, Nature, 2011, 478, 399–403 CrossRef CAS PubMed.
- J. Kreuger and M. Phillipson, Nat. Rev. Drug Discovery, 2016, 15, 125–142 CrossRef CAS PubMed.
- M. De Palma, D. Biziato and T. V. Petrova, Nat. Rev. Cancer, 2017, 17, 457 CrossRef CAS PubMed.
- J. Wei, J. Barr, L. Y. Kong, Y. Wang, A. Wu, A. K. Sharma, J. Gumin, V. Henry, H. Colman, W. Priebe, R. Sawaya, F. F. Lang and A. B. Heimberger, Mol. Cancer Ther., 2010, 9, 67–78 CrossRef CAS PubMed.
- A. S. Cazet, M. N. Hui, B. L. Elsworth, S. Z. Wu, D. Roden, C. L. Chan, J. N. Skhinas, R. Collot, J. Yang, K. Harvey, M. Z. Johan, C. Cooper, R. Nair, D. Herrmann, A. McFarland, N. Deng, M. Ruiz-Borrego, F. Rojo, J. M. Trigo, S. Bezares, R. Caballero, E. Lim, P. Timpson, S. O'Toole, D.
N. Watkins, T. R. Cox, M. S. Samuel, M. Martin and A. Swarbrick, Nat. Commun., 2018, 9, 2897 CrossRef PubMed.
- M. M. Mueller and N. E. Fusenig, Nat. Rev. Cancer, 2004, 4, 839–849 CrossRef CAS PubMed.
- J. B. Kim, R. Stein and M. J. O'Hare, Breast Cancer Res. Treat., 2004, 85, 281–291 CrossRef PubMed.
- C. Wang, Z. Tang, Y. Zhao, R. Yao, L. Li and W. Sun, Biofabrication, 2014, 6, 022001 CrossRef PubMed.
- G. Benton, G. DeGray, H. K. Kleinman, J. George and I. Arnaoutova, PLoS One, 2015, 10, e0123312 CrossRef PubMed.
- K. Kwapiszewska, A. Michalczuk, M. Rybka, R. Kwapiszewski and Z. Brzózka, Lab Chip, 2014, 14, 2096–2104 RSC.
- M. Vinci, S. Gowan, F. Boxall, L. Patterson, M. Zimmermann, W. Court, C. Lomas, M. Mendiola, D. Hardisson and S. A. Eccles, BMC Biol., 2012, 10, 29 CrossRef CAS PubMed.
- H. S. Kim, T. P. Devarenne and A. Han, Lab Chip, 2015, 15, 2467–2475 RSC.
- L. Bell, A. Seshia, D. Lando, E. Laue, M. Palayret, S. F. Lee and D. Klenerman, Sens. Actuators, B, 2014, 192, 36–41 CrossRef CAS PubMed.
- K. A. Ohiri, S. T. Kelly, J. D. Motschman, K. H. Lin, K. C. Wood and B. B. Yellen, Lab Chip, 2018, 18, 2124–2133 RSC.
- P. Occhetta, M. Licini, A. Redaelli and M. Rasponi, Med. Eng. Phys., 2016, 38, 33–40 CrossRef PubMed.
- A. K. White, M. VanInsberghe, O. I. Petriv, M. Hamidi, D. Sikorski, M. A. Marra, J. Piret, S. Aparicio and C. L. Hansen, Proc. Natl. Acad. Sci. U. S. A., 2011, 108, 13999–14004 CrossRef CAS PubMed.
- S. L. Stott, C. H. Hsu, D. I. Tsukrov, M. Yu, D. T. Miyamoto, B. A. Waltman, S. M. Rothenberg, A. M. Shah, M. E. Smas, G. K. Korir, F. P. Floyd, A. J. Gilman, J. B. Lord, D. Winokur, S. Springer, D. Irimia, S. Nagrath, L. V. Sequist, R. J. Lee, K. J. Isselbacher, S. Maheswaran, D. A. Haber and M. Toner, Proc. Natl. Acad. Sci. U. S. A., 2010, 107, 18392–18397 CrossRef CAS PubMed.
- A. R. Wheeler, W. R. Throndset, R. J. Whelan, A. M. Leach, R. N. Zare, Y. H. Liao, K. Farrell, I. D. Manger and A. Daridon, Anal. Chem., 2003, 75, 3581–3586 CrossRef CAS PubMed.
- H. Yin and D. Marshall, Curr. Opin. Biotechnol., 2012, 23, 110–119 CrossRef CAS PubMed.
- S. Kobel, A. Valero, J. Latt, P. Renaud and M. Lutolf, Lab Chip, 2010, 10, 857 RSC.
- D. Di Carlo, N. Aghdam and L. P. Lee, Anal. Chem., 2006, 78, 4925–4930 CrossRef CAS PubMed.
- A. C. Rowat, J. C. Bird, J. J. Agresti, O. J. Rando and D. A. Weitz, Proc. Natl. Acad. Sci. U. S. A., 2009, 106, 18149–18154 CrossRef CAS PubMed.
- S. L. Faley, M. Copland, D. Wlodkowic, W. Kolch, K. T. Seale, J. P. Wikswo and J. M. Cooper, Lab Chip, 2009, 9, 2659–2664 RSC.
- C. Ionescu-Zanetti, R. M. Shaw, J. Seo, Y.-N. Jan, L. Y. Jan and L. P. Lee, Proc. Natl. Acad. Sci. U. S. A., 2005, 102, 9112–9117 CrossRef CAS PubMed.
- W.-H. Tan and S. Takeuchi, Proc. Natl. Acad. Sci. U. S. A., 2007, 104, 1146–1151 CrossRef CAS PubMed.
- A. M. Skelley, O. Kirak, H. Suh, R. Jaenisch and J. Voldman, Nat. Methods, 2009, 6, 147–152 CrossRef CAS PubMed.
- P. J. Lee, P. J. Hung, R. Shaw, L. Jan and L. P. Lee, Appl. Phys. Lett., 2005, 86, 223902 CrossRef.
- J.-P. Frimat, M. Becker, Y.-Y. Chiang, U. Marggraf, D. Janasek, J. G. Hengstler, J. Franzke and J. West, Lab Chip, 2011, 11, 231–237 RSC.
- B. Dura, M. M. Servos, R. M. Barry, H. L. Ploegh, S. K. Dougan and J. Voldman, Proc. Natl. Acad. Sci. U. S. A., 2016, 113, E3599–E3608 CrossRef CAS PubMed.
- B. Dura, S. K. Dougan, M. Barisa, M. M. Hoehl, C. T. Lo, H. L. Ploegh and J. Voldman, Nat. Commun., 2015, 6, 5940 CrossRef CAS PubMed.
- J.-P. Frimat, M. Becker, Y.-Y. Chiang, U. Marggraf, D. Janasek, J. G. Hengstler, J. Franzke and J. West, Lab Chip, 2011, 11, 231–237 RSC.
- W. Espulgar, Y. Yamaguchi, W. Aoki, D. Mita, M. Saito, J.-K. Lee and E. Tamiya, Sens. Actuators, B, 2015, 207, 43–50 CrossRef CAS.
- S.-Y. C. Chen, P. J. Hung and P. J. Lee, Biomed. Microdevices, 2011, 13, 753–758 CrossRef CAS PubMed.
- A. Valero, F. Merino, F. Wolbers, R. Luttge, I. Vermes, H. Andersson and A. van den Berg, Lab Chip, 2005, 5, 49 RSC.
- J. T. Nevill, R. Cooper, M. Dueck, D. N. Breslauer and L. P. Lee, Lab Chip, 2007, 7, 1689 RSC.
- L. Y. Wu, D. Di Carlo and L. P. Lee, Biomed. Microdevices, 2008, 10, 197–202 CrossRef CAS PubMed.
- P. J. Hung, P. J. Lee, P. Sabounchi, N. Aghdam, R. Lin and L. P. Lee, Lab Chip, 2005, 5, 44 RSC.
- S. Faley, K. Seale, J. Hughey, D. K. Schaffer, S. VanCompernolle, B. McKinney, F. Baudenbacher, D. Unutmaz and J. P. Wikswo, Lab Chip, 2008, 8, 1700–1712 RSC.
- S. L. Gac, E. Zwaan, A. van den Berg and C. D. Ohl, Lab Chip, 2007, 7, 1666–1672 RSC.
- S. Dochow, C. Krafft, U. Neugebauer, T. Bocklitz, T. Henkel, G. Mayer, J. Albert and J. Popp, Lab Chip, 2011, 11, 1484–1490 RSC.
-
S. C. Grover, A. G. Skirtach, R. C. Gauthier and C. P. Grover, Automated single-cell sorting system based on optical trapping, SPIE, 2001 Search PubMed.
- M.-C. Zhong, X.-B. Wei, J.-H. Zhou, Z.-Q. Wang and Y.-M. Li, Nat. Commun., 2013, 4, 1768 CrossRef PubMed.
- M. K. Kreysing, T. Kießling, A. Fritsch, C. Dietrich, J. R. Guck and J. A. Käs, Opt. Express, 2008, 16, 16984–16992 CrossRef CAS PubMed.
- J. Guck, R. Ananthakrishnan, H. Mahmood, T. J. Moon, C. C. Cunningham and J. Käs, Biophys. J., 2001, 81, 767–784 CrossRef CAS PubMed.
- C. Cui, R. Merritt, L. Fu and Z. Pan, Acta Pharm. Sin. B, 2017, 7, 3–17 CrossRef PubMed.
- M. A. Alzahrani, N. Schmulewitz, S. Grewal, F. V. Lucas, K. O. Turner, J. T. McKenzie, J. J. Sussman and S. A. Ahmad, J. Surg. Oncol., 2012, 105, 156–161 CrossRef PubMed.
- J. Estevez-Herrera, N. Dominguez, M. R. Pardo, A. Gonzalez-Santana, E. W. Westhead, R. Borges and J. D. Machado, Proc. Natl. Acad. Sci. U. S. A., 2016, 113, E4098–E4106 CrossRef CAS PubMed.
- T. Andersson, C. Betsholtz and B. Hellman, Acta Endocrinol., 1981, 96, 87–92 CAS.
- H. Streb, R. F. Irvine, M. J. Berridge and I. Schulz, Nature, 1983, 306, 67–69 CrossRef CAS PubMed.
- A. Wuttke, Q. Yu and A. Tengholm, J. Biol. Chem., 2016, 291, 14986–14995 CrossRef CAS PubMed.
- N. White and G. Burnstock, Trends Pharmacol. Sci., 2006, 27, 211–217 CrossRef CAS PubMed.
- J. Lytton, M. Westlin and M. R. Hanley, J. Biol. Chem., 1991, 266, 17067–17071 CAS.
- J. Miyazaki, K. Araki, E. Yamato, H. Ikegami, T. Asano, Y. Shibasaki, Y. Oka and K. Yamamura, Endocrinology, 1990, 127, 126–132 CrossRef CAS PubMed.
Footnote |
† Electronic supplementary information (ESI) available. See DOI: 10.1039/c8lc01153b |
|
This journal is © The Royal Society of Chemistry 2019 |