Theoretical insights into dopamine photochemistry adsorbed on graphene-type nanostructures†
Received
30th January 2024
, Accepted 30th April 2024
First published on 7th May 2024
Abstract
The equilibrium geometry structures and light absorption properties of the dopamine (DA) and dopamine-o-quinone (DAQ) adsorbed on the graphene surface have been investigated using the ground state and linear-response time-dependent density functional theories. Two types of graphene systems were considered, a rectangular form of hexagonal lattice with optimized C–C bond length as the model system for graphene nanoparticles (GrNP) and a similar system but with fixed C–C bond length (1.42 Å) as the model system for graphene 2D sheet (GrS). The analysis of the vertical excitations showed that three types of electronic transitions are possible, namely, localized on graphene, localized on the DA or DAQ, and charge transfer (CT). In the case of the graphene–DA complex, the charge transfer excitations were characterized by the molecule-to-surface (MSCT) character, whereas the graphene–DAQ was characterized by the reverse, i.e. surface-to-molecule (SMCT). The difference between the two cases is given by the presence of an energetically low-lying unoccupied orbital (LUMO+1) that allows charge transfer from the surface to the molecule in the case of DAQ. However, it was also shown that the fingerprints of excited electronic states associated with the adsorbed molecules cannot be seen in the spectrum, as they are mostly suppressed by the characteristic spectral shape of graphene.
1 Introduction
The photochemical behaviour of an organic molecule can change fundamentally when it is adsorbed on a metal or semiconductor surface.1–4 Not only do the photochemical properties of the adsorbed molecule change, but new, more complex photo-processes may also appear, such as, electron or energy transfer to metal surfaces,5,6 substrate–adsorbate photocoupling,7 surface driven photooxidation8,9 or more complex photocatalytic processes.10 Moreover, the wide range of these properties can offer several research and industrial applications, such as, development of high-performance dye-sensitized solar cells,11 building new, functionalized materials for electrode material for fuel cells and batteries,12,13 materials for 3D printing14 or creating micropatterns with desired biofunctional properties.15
Inspired by the composition of adhesive proteins in mussels, Lee and co-workers16,17 have shown that dopamine (DA) through the self-polymerization process can form thin, surface-adherent polydopamine films (PDA)18,19 onto a wide range of inorganic and organic materials, including noble metals, oxides, polymers, semiconductors, and ceramics.20–26
On the other hand, carbon-based nanomaterials are one of the most widely discussed, researched and applied synthetic nanomaterials, due to their diverse capabilities, such as excellent electronic, magnetic and optical properties. At the same time, their chemical versatility allows them to be easily manipulated in laboratory and industrial environments, to be biocompatible and to be considered as a chemically robust platform.27–32
Taking into account the properties of the individual systems presented in the previous two paragraphs, further interesting behaviour can be observed in the case of the dopamine–graphene complex. First of all, one should mention the use of graphene as a DA sensor33–37 or that of PDA for the reduction of graphene oxide,38 but their complex was also used for making polymer filters,39 to improve electrochemical performances of supercapacitors40 or Li–S batteries,41 to use as high-performance material for biosensing42,43 or as an efficient electrocatalysts44–46 as well as to apply it in cancer therapy.47 But what makes it particularly more interesting is the photochemical application of the graphene–dopamine complex,48–52 especial as photothermal therapy for cancer.53
Although DA is a relatively small molecule, its photochemical behaviour strongly dependents on its the concentration and tautomer forms as well as on the pH of the solvent environment,54,55 and it is therefore much more difficult to unambiguously identify the fingerprints of different tautomers in the experimental UV-Vis spectrum. Similarly to DA, graphene exhibits complex photochemical behaviour, as the shape of the spectrum is highly dependent on the atomic configuration and size of the graphene structure.56–62
Accurate theoretical reproduction of experimental UV-Vis spectra is a challenge in itself, even for intermediate molecules,63 especially with respect to the accurate description of the electron correlation and the response function. This is particularly true for complex, multi-component supramolecular systems.64–68 At the same time, graphene nanostructures have been successfully applied to the detection of e.g. catechol,69 where also the UV-Vis spectroscopy technique was considered for the structure recognition. In such a case, the question arises whether it is possible to identify the individual components on the basis of the resulting adsorption spectrum, or whether this resulting spectrum has any characteristic feature that suggests that the two components form a well-defined joint complex.
The aim of our study is to give a detailed description for the photochemistry behavior, and in particular, for nature of the electronic transitions induced by an electromagnetic radiation in the cases of graphene – dopamine and graphene – dopamine-o-quinone complexes using the framework of the time-dependent density functional theory, with the aim of investigating whether graphene as a tool helps to facilitate the identification of adsorbed molecules on it surface.
2 Computational details
The equilibrium geometries and normal mode vibrational frequencies of individual molecules, the graphene sheet and different molecule–graphene binary complexes were computed in the framework of density functional theory (DFT) considering the ωB97X-D3BJ exchange–correlation (XC) functional70 by including the Grimme's empirical dispersion correction scheme71,72 and using the def2-TZVPP triple-ζ basis set of the Karlsruhe group73 as implemented in the Orca program suite.74,75 The electronically excited state calculations and equilibrium geometry optimizations were computed using the time-dependent version of the same DFT framework considering the Tamm–Dancoff approximation (TDA) approximation.76 The RIJCOSX approximation77 designed to accelerate Hartree–Fock and hybrid DFT calculations were considered together with the Def2/J78 auxiliary basis set for Coulomb fitting and the def2-TZVPP/C79 auxiliary basis set for correlation fitting in the case of TD-DFT calculations. During the geometry optimization of the molecule–graphene binary complex the graphene part was kept frozen and the Partial Hessian Vibrational Analysis (PHVA) method80 has been used for obtaining the normal mode vibrational frequencies of the unconstrained part of the binary complex. The theoretical prediction of the fluorescence rate was made based on the path integral approach to the dynamics by solving Fermis Golden Rule-like equation including vibronic coupling in forbidden transitions (the so-called Herzberg–Teller effect (HT)) and Duschinsky rotations between modes of different states.81–83 The amount of transferred charge between the complex constituents was obtained based on the analysis of the charge population (Löwdin atomic charges) of a given electronically excited state density computed in the TDDFT framework. The molecular geometries were built, analyzed and further manipulated using Multiwfn84 Gabedit85 and Avogadro86 programs, while the molecular graphics were created using the GaussView87 software.
3 Results and discussion
3.1 Geometry structures
As a first step, a two-dimensional (three zig-zag and seven armchair edges) rectangular graphene nano-sheet with fully aromatic hexagonal rings (see Fig. 1) was considered in two geometrical configurations. In the first case its structure has been fully optimized in order to mimic the graphene-type nanoparticle (GrNP), while in the second case all C–C bond lengths were kept as 1.42 Å for reproducing the 2D infinite dimensional graphene sheet (GrS) geometry.88 The geometry optimization leads to an irregular honeycomb lattice, where the C–C bond length varies between 1.38–1.47 Å.
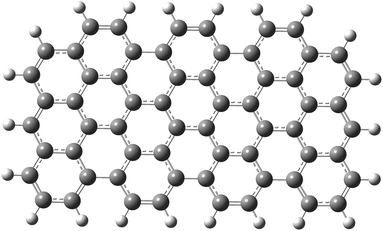 |
| Fig. 1 The molecular graphics of the graphene nanoparticle with three zig-zag and seven armchair edges. | |
In the next step, the dopamine (DA) and dopamine-o-quinone (DAQ) molecules were deposited on the GrNP-type graphene sheet and the geometry of the molecules as well as their relative position to the graphene sheet were optimized, while the geometry of the graphene sheet was was kept fixed. In the equilibrium geometry configuration, the DA molecule is slightly tilted with respect to the graphene sheet, with the O atoms of the hydroxyl group at 3.2 and 3.26 Å from the graphene plane, respectively, and the N atom of the amine group at 3.51 Å (see Fig. 2). A similar arrangement can be observed for DAQ, where the O atoms of the hydroxyl groups are 3.14 Å and 3.19 Å away, while the N atom of the amine group is 3.52 Å from the graphene plane (see Fig. 3). Adsorption energy of DA on the GrNP surface is −20.24 kcal mol−1, while that of DQ is −20.11 kcal mol−1, of which the deformation energy is only 0.41 kcal mol−1 and 0.13 kcal mol−1, respectively. As for the ground state charge distribution, there is a charge transfer of 0.068 e from the DA to the surface (called as molecule-to-surface charge transfer or MSCT), while for DAQ the charge transfer to the graphene is only 0.031 e.
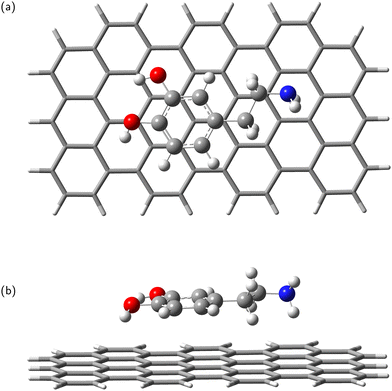 |
| Fig. 2 Dopamine adsorbed on graphene surface: top-view (a) and side-view (b). | |
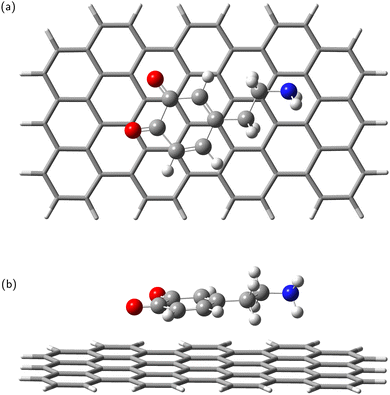 |
| Fig. 3 Dopamine adsorbed on graphene surface: top-view (a) and side-view (b). | |
3.2 Electronic excited states and UV absorption spectra
In order to gain more insight into the nature of the initial (vertical) and relaxed excited states, the first 30 electronic excited states were computed separately for each molecule and graphene sheet, and for different molecule–graphene binary complexes.
DA.
Given that the results for dopamine have already been reported,54,55 only the excited states of the graphene sheet and binary complexes will be discussed in more detail. Briefly, DA presents spectral characteristics with peak maxima at 280 nm and 218 nm (see Fig. 1 in ref. 54). However, as for the equilibrium geometry of the first excited state (S1), the C–O bonds of the DA's hydroxyl groups are shortened (from 1.377 Å and 1.362 Å to 1.360 Å and 1.348 Å) and the C–C bonds of the benzene ring are stretched, with all six bonds being longer than 1.4 Å (the bond values are 1.425, 1.403, 1.421, 1.407, 1.416 and 1.412, respectively in Å).
DAQ.
Oxidation of DA using different oxidation agents can easily lead to the quinone form,89 which induces an additional spectral peak around 390 nm in the UV absorption spectra. TDDFT calculations have shown that this additional peak comes from the contribution of the S0 → S3 electronic excitation, while the S1 and S2 excitations have nearly zero oscillator strength and do not result in any noticeable effect on the spectrum. For the electronic excited state energy values, oscillator strengths and the most intense orbital transition see Table S1 (ESI†), as well as for the UV absorption spectra see Fig. S1 from the ESI† file. The geometry optimization of the S1 electronic excited state leads mainly to the stretching of the C–C bond, to which carbon atoms are the carboxyl groups attached (from 1.553 Å to 1.625 Å).
Graphene.
Graphene is a 2D solid that has interesting physics due to its unusual electronic band structure,88 and consequently also has unusual optical properties through a wide absorption spectral region given by intraband transitions (π → π*) at low photon energies (0.0–5.0 eV in the far-infrared spectral range) and by interband transitions (π → σ*) at higher energies (over 5.0 eV from mid-infrared to ultraviolet).90,91 However, state-of-the-art theoretical calculations have shown that in order to reproduce the experimental spectrum accurately, it is necessary to take electron correlation into account.92,93 On the other hand, graphene-like materials with lower dimension (1D wires), such as graphene nanoribbons, substantially different electronic band structure and optical properties than graphene.94,95 Although with significant differences, a similar spectral profile in the UV-Vis absorption spectrum can be found for graphene nanocrystals of finite dimensions (0D structures), 10–50 nm.96,97 That is, the peak around 4.5 eV and the long decay shoulder towards lower energy values, which in turn can vary depending on the shape and size of the particle. The experimental UV absorption spectra of graphene nanoparticles60,98 showed an intense spectral peak centred at 269 nm and a very long shoulder that is over-expanded up to 700 nm (see Fig. 1 in ref. 60). At the same time, theoretical calculations based on TDDFT showed that for a 0D graphene particle with a dimension of a few nanometres, additional peaks of lower intensity can appear in the 350–700 nm interval, which is clearly influenced by the dimension of the graphene model system.61,62,99 Theoretical UV absorption spectra were computed considering the graphene nanostructure shown in Fig. 1 both in the optimized (GrNP) and fixed (GrS) geometry forms, by applying the TDDFT/ωB97X-D3BJ/def2-TZVPP level of theory. Their spectral shapes are presented in Fig. 4, while their detailed spectral features (spectral shape, wavelength lines and intensities) is shown in Fig. S2 and Table S2 in the ESI† file. Theoretical results show an intense peak around the 260–280 nm as it can be observed in the experimental spectra,60,98 but in the present analysis there are also additional peaks associated mainly with the first excited states (537 nm for GrNP and 677 nm for GrS) that are likely to depend on the dimension of the chosen graphene model system. Furthermore, it is clear that the peak observed in the experimental spectrum around 270 nm is given by the higher states in the excitation spectrum (S18 and S21 for GrNP and S17 and S19 for GrS) and the longer shoulder of 350–700 nm region comes from the lower but weakly intense excited states. By analyzing the so-called natural difference orbitals (NDO) for electronic transitions between the ground state and the different excited states, it is observed that the S0 → S1 transition is a π → π* type excitation along two consecutive C–C aromatic bonds, thus forming a zig-zag chain along the longer dimension both for the GrNP- and GrN-type graphene structures (see Table S3 in the ESI† file).
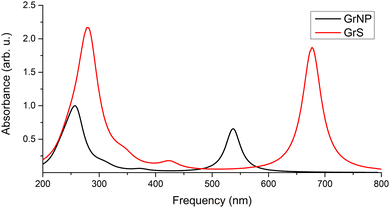 |
| Fig. 4 Theoretical UV absorption spectra of the model graphene lattice both in the optimized (GrNP) and fixed (GrS) geometry forms, computed at TDDFT/ωB97X-D3BJ/def2-TZVPP level of theory. | |
It is important to note that in the case of graphene, only a simplified model has been considered, where the 2D solid state character and the band structure have been discarded. Instead, a finite dimensional hydrocarbon structure has been adopted which results in discrete electronic states. Compared to the experimental UV spectrum of graphene, this simple model is already able reproduce the most important features of the UV spectrum. Of course, in order to accurately follow the changes in the electron structure of graphene induced by the adsorbed molecules, the simplified model described here is no longer suitable, it needs for solid-state model.
DA–graphene.
First, the GrNS form was used on graphene models. The energetic scheme of highest occupied and lowest unoccupied molecular orbitals (MO) in the energy range of [−10.0 eV to 2.5 eV] computed for the individual fragments (GrNP or DA) as well as for the joined graphene–DA binary complex is shown in Fig. 5. As can be observed from the figure, the energies of the molecular orbitals of the binary complex follow the topology of graphene much more closely than that of DA. In this sense, the binary complex is not a classical donor–acceptor system,100 since the HOMO orbital of DA is energetically much lower, and the LUMO orbital is much higher than that of graphene. Although, for occupied MOs, the DA contribution is observed starting with the HOMO−1, for unoccupied orbitals the DA contribution is only seen from the LUMO+11 upwards. For details see Fig. 5, while the frontier orbitals of DA are shown in Table S4 in the ESI† file. As the next step, the first thirty electronic excited state energies of the graphene–DA binary complex has been computed using the theoretical framework described in Section 2. Accordingly, their energy values and oscillator strengths are collected in Table 1, while the theoretical UV absorption spectra is shown in Fig. 6. In terms of light absorption intensity, the S1, S2, S10, S11, S16, S20 and S23 excited states are worth highlighting. In the UV absorption spectrum the S1 excited state gives the peak at 540 nm, S2 the hump at 377 nm, S10 and S11 the spectral shoulder at 310 nm, while S16, S20 and S23 together give the high peak at 260 nm. These electronic transitions are partly related to pure GrNP (S1, S2, S10 and S11), pure charge transfer (S16) and partly to mixed GrNP and charge-transfer (S20 and S23) electronic states, while DA alone has no significant contribution. Yet, as far as the role of DA is concerned, it is manifested by the appearance of a DA to GrNP (molecule-to-surface charge transfer or MSCT) transition (for ex. S16) alongside the pure GrNP-type transitions that diminishes the intensity of the absorption or slightly shifts the absorption peaks (e.g. from 537 nm to 540 nm in the case of S1). Of the thirty excited states, seventeen are purely GrNP-localized excitations, six have a charge-transfer character, six show a mixed form of the two types, while only one state is observed to have a typical DA excitation (S29). The detailed data on the thirty excited states mentioned above, such as the most significant molecular orbital excitations and the NDOs (or natural density orbitals) are given in Table S5 in the ESI† file. As far as the electron charge distribution after excitation is concerned, the so-called charge transfer (or CT) excitations are the most interesting electronic states. The theoretical description of these electronic excitations is not so straightforward, due to the large electron–hole separation along the molecular system.101–104 It has been shown105 that the wB97X-D exchange–correlation functional can efficiently describe not only the valence, but also the charge transfer and Rydberg electronic excitations. It is known that, CT excitations are not specific to any of the isolated molecular fragments, and during the excitation there is a large charge transfer from one fragment to another, in this particular case from DA to graphene. Accordingly, the maximum amount of charge transferred from DA to graphene is 0.798 e which is found for the S26 state. The other such states are S6, S7, S9, S16 and S28, to which charge transfers of 0.117 e, 0.397 e, 0.317 e, 0.398 e and 0.130 e have been occurred. It can be observed that a feature of the CT states, especially when accompanied by a high charge transfer, is that the coefficients of the one-electron excitations from deeper filled MO orbitals to the LUMO orbital are high compared to the other one-electron excitations (e.g., for S26 the coefficient of the HOMO−10 → LUMO excitation is 0.60). There is also a charge transfer effect in the mixed “GrNP + CT” states, but its magnitude is generally smaller than in the pure CT states, i.e. less than 0.1 e (the only exception is S23 where it is 0.114 e). The typical DA-like S29 state does not appear to be a purely DA-localized excitation either, but there is also a MSCT component in this case with a charge transfer of 0.149 e, which means that part of the DA excitation is transferred to the graphene surface. However, the high order of the DA-related electronic excitation level (29th) is not surprising, since the wavelength of the first electronic excited state calculated in the isolated state is 266 nm,54 which is close to the wavelength of the S29 excited state (240 nm). As for the UV absorption compiled spectra of the individual components and the binary system, it can be observed that graphene-like electronic transitions are the dominant components in the higher wavelength range of the spectrum (300–700 nm), while in the fingerprint of the DA appears in the lower spectral range (200–300 nm), but its intensity looks very low compared to that of graphene. From the point of view of light absorption, the CT states can be considered active, although not all of them have been assigned a large oscillator strength. The role of S9 and S16 electronic excited states should be highlighted, which could not be completely suppressed by the strong peak of graphene centred at 261 nm (S20), so that a weaker shoulder of the spectrum at 300 nm could be observed (see Fig. 6).
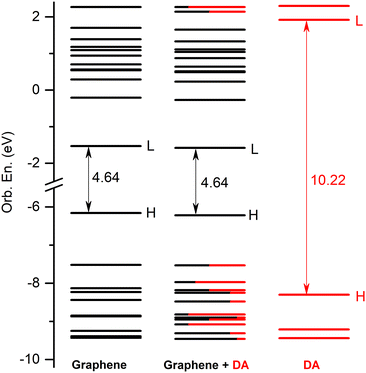 |
| Fig. 5 The molecular orbital energy scheme (in eV) of the individual, graphene and DA components and of the mixed graphene–DA binary complex (H = HOMO, L = LUMO) based on the fragment orbital contribution analysis. | |
Table 1 The first thirty electronic excited state energies (in nm), their oscillator strengths, transferred charges and electronic transition types (either located on the GrNP and on DA fragments or charge transfer states between the fragments) of the GrNP–DA binary complex, computed at TDDFT/ωB97X-V/def2-TZVPP level of theory
Si |
1 |
2 |
3 |
4 |
5 |
6 |
7 |
8 |
9 |
10 |
CT = Charge transfer character |
λ (nm) |
540 |
377 |
374 |
364 |
357 |
342 |
331 |
323 |
321 |
312 |
Osc. Str. |
1.6763 |
0.0807 |
0.0008 |
0.0006 |
0.0001 |
0.0026 |
0.0068 |
0.0085 |
0.0212 |
0.0725 |
Charge tr. (e) |
0.001 |
0.026 |
0.003 |
0.004 |
0.014 |
0.117 |
0.397 |
0.006 |
0.317 |
0.043 |
Type |
GrNP |
GrNP |
GrNP |
GrNP |
GrNP |
CT |
CT |
GrNP |
CT |
GrNP + CT |
Si |
11 |
12 |
13 |
14 |
15 |
16 |
17 |
18 |
19 |
20 |
λ (nm) |
304 |
303 |
295 |
285 |
283 |
271 |
267 |
267 |
262 |
261 |
Osc. Str. |
0.0838 |
0.0358 |
0.0348 |
0.0012 |
0.0155 |
0.2183 |
0.1371 |
0.0751 |
0.1166 |
0.7479 |
Charge tr. (e) |
0.004 |
0.003 |
0.025 |
0.040 |
0.084 |
0.398 |
0.080 |
0.028 |
0.012 |
0.084 |
Type |
GrNP |
GrNP |
GrNP |
GrNP |
GrNP + CT |
CT |
GrNP + CT |
GrNP |
GrNP |
GrNP + CT |
Si |
21 |
22 |
23 |
24 |
25 |
26 |
27 |
28 |
29 |
30 |
λ (nm) |
259 |
257 |
255 |
253 |
250 |
248 |
245 |
244 |
240 |
239 |
Osc. Str. |
0.0067 |
0.0309 |
0.7957 |
0.0243 |
0.0613 |
0.0171 |
0.0153 |
0.3103 |
0.0449 |
0.0053 |
Charge tr. (e) |
0.027 |
0.031 |
0.114 |
0.032 |
0.102 |
0.798 |
0.106 |
0.130 |
0.149 |
0.041 |
Type |
GrNP |
GrNP |
GrNP + CT |
GrNP |
GrNP + CT |
CT |
GrNP + CT |
CT |
DA + CT |
GrNP |
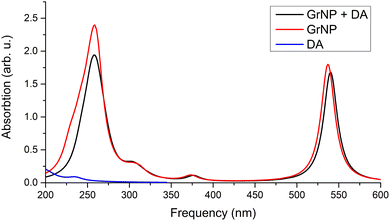 |
| Fig. 6 Theoretical UV absorption spectra of the graphene–DA binary complex computed at TDDFT/ωB97X-D3BJ/def2-TZVPP level of theory. | |
When the GrS type was used as the graphene surface, the first forty excited states were analysed rather than the first thirty. This was necessary because in the first thirty states there wasn’t any excitation that could be associated with the DA. When this interval was increased to forty, it was possible to identify the DA-related excited state as S34, which also has a mixed state coming from the locally excited DA- and MSCT-type transitions, and the amount of transferred charge is 0.272 e. In addition, three, pure CT states were also identified, S9, S17 and S25, with charge transferred from DA to GrS (MSCT) amounts of 0.816 e, 0.456 e and 0.603 e, respectively. Twelve of the thirty-six remaining excited states are pure GrS, while twenty-four are of mixed “GrS + CT” type.
DAQ–graphene.
The energetic scheme of highest occupied and lowest unoccupied MOs in the energy range of [−10.0 eV to 2.5 eV] computed for the individual fragments (GrNP or DAQ) as well as for the joined graphene–DAQ binary complex is shown in Fig. 7, while the frontier orbitals of DAQ are shown in Table S6 in the ESI† file. In almost the same way as in the previous case, especially as far as the occupied orbitals are concerned, the energies of the molecular orbitals of the binary complex follow the topology of graphene much better than those of DAQ. As for the unoccupied orbitals, the situation changes in that the DAQ also has a low virtual orbitals (LUMO, see third column of energy levels at Fig. 7) which in turn can affect the nature of the electronic excitation. Similarly as in the previous case, the first 30 excited states were calculated for the DAQ–graphene binary complex. Accordingly, their energy values and oscillator strengths are collected in Table 2, while the theoretical UV absorption spectra is shown in Fig. 8. The two large peaks in the spectrum are given by S1, S24 and S25 excited states. The other two smaller peaks are defined by the S3 and S4 states, while the second larger peak is defined by the S14 and S15 states. As regards the nature of the excited states, unlike the graphene–DA system, in the present case the first three excited states are of a different nature than those seen in the previous case. There, all three were of pure graphene type, whereas here the first is of mixed “GrNP + CT” type, the second is of DAQ type and the third is of pure CT type. What is also very surprising is that not only MSCT-type charge transfer can be observed, but also charge transfer in the opposite direction, i.e. surface-to-molecule charge transfer (or SMCT). This reverse direction is manifested in the fact that the value of the transferred charge is negative. If one considers that in the ground state 0.031 e charge migrated from DAQ to graphene, and for the S1 excited state it is −0.034 e, then it can be concluded that in fact 0.065 e charge was transferred from graphene to DAQ during the excitation. Overall, eighteen of the first thirty excited states were found to be related to pure GrNP, four to mixed “GrNP + CT”, five to pure CT and the remaining three to DAQ fragment excitation. Considering, in general, the charge transfer value obtained for the ground state (i.e. 0.031 e), only the S22 and S24 states are the ones where very little additional charge amount is transferred from the DAQ to the graphene surface, 0.004 e and 0.022 e respectively, the other twenty-eight cases show SMCT type charge transfer effects. Furthermore, the five CT electronic states are S3, S16, S18, S21 and S30, with charges of −0.836 e, −0.698 e, −0.756 e, −0.799 e and −0.749 e migrating from the graphene surface to the DAQ molecule.
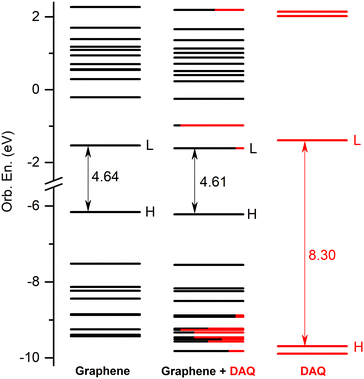 |
| Fig. 7 The molecular orbital energy scheme (in eV) of the individual, graphene and DAQ components and of the mixed graphene–DAQ binary complex (H = HOMO, L = LUMO) based on the fragment orbital contribution analysis. | |
Table 2 The first thirty electronic excited state energies (in nm), their oscillator strengths, transferred charges and electronic transition types (either located on the GrNP and on DAQ fragments or charge transfer states between the fragments) of the GrNP–DAQ binary complex, computed at TDDFT/ωB97X-V/def2-TZVPP level of theory
Si |
1 |
2 |
3 |
4 |
5 |
6 |
7 |
8 |
9 |
10 |
CT = Charge transfer character. |
λ (nm) |
547 |
518 |
472 |
376 |
374 |
364 |
357 |
340 |
332 |
326 |
Osc. Str. |
1.5631 |
0.0010 |
0.1244 |
0.0691 |
0.0054 |
0.0053 |
0.0014 |
0.0044 |
0.0068 |
0.0026 |
Charge tr. (e) |
−0.034 |
0.050 |
−0.836 |
0.023 |
0.022 |
0.025 |
0.028 |
0.021 |
−0.024 |
0.000 |
Type |
GrNP + CT |
DAQ |
CT |
GrNP |
GrNP |
GrNP |
GrNP |
GrNP |
DAQ |
GrNP |
Si |
11 |
12 |
13 |
14 |
15 |
16 |
17 |
18 |
19 |
20 |
λ (nm) |
325 |
323 |
314 |
304 |
304 |
297 |
294 |
288 |
285 |
281 |
Osc. Str. |
0.0000 |
0.0146 |
0.0980 |
0.0488 |
0.0472 |
0.0202 |
0.0209 |
0.0081 |
0.0159 |
0.0056 |
Charge tr. (e) |
0.050 |
0.021 |
0.000 |
0.000 |
0.014 |
−0.698 |
−0.084 |
−0.756 |
−0.048 |
0.018 |
Type |
DAQ |
GrNP |
GrNP |
GrNP |
GrNP |
CT |
GrNP + CT |
CT |
GrNP + CT |
GrNP |
Si |
21 |
22 |
23 |
24 |
25 |
26 |
27 |
28 |
29 |
30 |
λ (nm) |
277 |
268 |
265 |
263 |
263 |
259 |
258 |
256 |
253 |
250 |
Osc. Str. |
0.0270 |
0.0957 |
0.0026 |
0.7773 |
0.5549 |
0.1103 |
0.0246 |
0.1413 |
0.1526 |
0.0598 |
Charge tr. (e) |
−0.799 |
0.035 |
−0.015 |
0.053 |
0.014 |
0.014 |
0.020 |
0.029 |
0.015 |
−0.749 |
Type |
CT |
GrNP |
GrNP + CT |
GrNP |
GrNP |
GrNP |
GrNP |
GrNP |
GrNP |
CT |
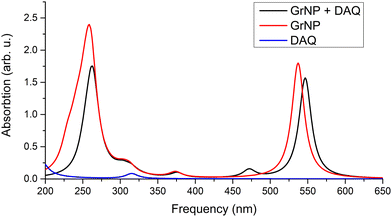 |
| Fig. 8 Theoretical UV absorption spectra of the graphene–DAQ binary complex computed at TDDFT/ωB97X-D3BJ/def2-TZVPP level of theory. | |
When the excitations between the canonical MO orbitals are analyzed for these CT excitations, it can be seen that there is always a transition with a large weight in the individual MO excitation scheme from certain occupied orbital to the same LUMO+1 orbital. Namely, for S3 is H → L+1 (93%), for S16 is H−1 → L+1 (64%), for S18 is H−4 → L+1 (61%), for S21 is H−2 → L+1 (77%) and for S30 is H−5 → L+1 (50%) excitation, respectively. A similar excitation scheme is true for DAQ-like excitations, with the difference that the excitation starts from even lower MO orbitals and goes to L+1. Namely, for S2 is H−9 → L+1 (54%), for S9 is H−8 → L+1 (26%) and for S11 is H−19 → L+1 (63%) excitation, respectively.
The detailed data on the thirty excited states mentioned above, such as the most significant molecular orbital excitations and the NDOs are given in Table S7 in the ESI† file. Concerning the UV-Vis absorption spectrum in particular, it can be observed that the spectrum of the graphene–DAQ complex is very similar to that of graphene. Difference is made by the peak obtained for the S3 CT-type excited state (around 472 nm), which cannot be seen in the graphene spectrum (see Fig. 8). As for the DAQ, some contribution from S9 (332 nm) can be observed in the UV spectrum, however, the absorption intensity associated with this electronic transition is quite small.
No significant change in the nature of the excited states was observed when instead of the GrNP form the GrS-type graphene structure was considered. For example, the nature of the S1, S2 and S3 excited states remains the same, except that the excitation wavelengths are shifted to higher (lower energy) values. That is, instead of 547 nm, 518 nm and 472 nm, values of 686 nm, 530 nm and 516 nm were obtained.
3.3 Excited state relaxation dynamics
The relaxation dynamics of electronic excited states on metal or semiconductor 2D surface looks a very complex phenomena.106–109 Since excited states can be localize on one or the other constituent of the molecule–surface complex, or charges can easily migrate from the molecule to the surface or vice versa, relaxation processes can also occur either on the components separately or even inside the binary system. Accordingly, it was already shown, that non-radiative relaxation can occur either on the graphene surface110 itself or in the binary complex,111–118 but at the same time, excited states in the case of slightly modified GrNP can also decay via fluorescence phenomena.119,120 Given that the relatively moderate size of the chosen graphene model (62 carbon and 22 hydrogen atoms) does not require the use of, e.g., a solid-state based physical model, in the present case the binary molecule–graphene complex will be considered as a macromolecular system with two interacting constituents and treated with the ordinary DFT and TDDFT methods suitable for molecular systems. Second, non-radiative phenomena were also not considered, as they require a very complex theoretical framework and huge computation resources to describe them. Third, as a further approximation, the dynamics of the surface (geometry deformation) has been neglected, because we are more interested in the dynamics of the adsorbed molecule. This was achieved by keeping the atoms of the surface fixed during the normal mode vibration and excited state geometry optimization calculations. In order to follow the relaxation of the higher excited states without considering the internal conversion phenomena between adjacent electronic states, the “root following” condition was imposed during the geometry relaxation.
DA–graphene.
Since, different constraints for the geometries were applied, only the pure CT and DA-related excited states were investigated, except for S1. (i) S1 – during the geometry optimization, the geometrical parameters of the DA molecule did not change, only the relative orientation of the molecule with respect to the graphene plane. However, this change is also very minimal, e.g. the inter-planar distance between the DA and graphene will be only 0.002 Å shorter. The absorption wavelength of S1 remains unchanged after the geometric optimization, at 540 nm. Furthermore, the amount of charge transfer induced initially by the vertical excitation comes back after optimization to the ground state value of 0.069 e. It is well known that an important moment in the relaxation of excited states is when the system reaches its first excited state. From there it can reach the S0 ground state through different relaxation channels (radiative or even non-radiative). From this point of view, it would be interesting to investigate the role of DA in a possible fluorescence deactivation mechanism. To this end, both the ground and the first excited state normal mode vibrations have been computed by considering only the vibrations of the DA. For this purpose, the Partial Hessian Vibrational Analysis (PHVA) method80 was applied. In this way, the role of the DA on the fluorescence rate of the DA–graphene complex through the vibronic coupling can be estimated. The fluorescence rate has been computed using the both the Frank–Condon (FC) and Hertzberg–Teller (HT) approximations, but for vibrations, the effects of intermolecular modes have been considered separately. It was observed that without the contribution of the intermolecular modes the fluorescence rate was 8.48 × 108 s−1 (i.e. lifetime 1.18 ns) and the whole rate is given by the FC approximation, while when the intermolecular modes were included the rate was an order of magnitude higher (i.e. the rate was 6.54 × 109 s−1 and the lifetime 0.15 ns) and for the fluorescence rate a contribution of 10% comes from the HT effects. This may indicate that adsorbed DA molecules can accelerate the deactivation process through the vibronic coupling with the graphene surface; (ii) S7 – this is an MSCT state whose geometric optimization brings the OH fragments of the DA closer to the graphene surface, with one O at 3.11 Å (Δ = −0.08 Å) and the other at 3.23 Å (Δ = −0.03 Å) from the surface. In addition, each C–O bond becomes 0.01 Å shorter and the aromatic character of the benzene ring is slightly distorted by two C–C bonds becoming 0.01 Å longer and the other four with 0.005 Å shorter. In this way the value of the charge transferred is reduced from 0.397 e to 0.250 e, but the order of the excited state remains 7th; (iii) S9 – in this case no significant change in the geometry of the DA was found, only the relative position of the DA changed slightly where the DA moving away from the graphene by 0.12 Å. Similar to S1 state, the amount of charge transfer induced initially by the vertical excitation comes back after optimization to the ground state value of 0.064 e, the order of the excited state becomes 3th; (iv) S16 – in this case, is observed that the DA is tilted so that one of the OH and NH2 groups comes a little closer to the surface (Δ (O⋯GrNP) = −0.06 Å and Δ (N⋯GrNP) = −0.04 Å), but the DA does not suffer internal geometry changes. On the other hand, there is a significant change in the magnitude of the transferred charge, since it was initially only 0.398 e, but increases to 0.624 e during relaxation, and the order of the excited state changes from 16 to 5; (v) S26 – as in the S16 case, there is only a slight tilt, the DA's own geometry remains unchanged. What is different from the previous case, however, is the decrease in charge transfer from 0.798 e to 0.291 e. The order of the excited state decreases to 19; (vi) S29 – this excited state is interesting from the point of view of whether the geometric changes of the DA during relaxation are similar to the geometric changes obtained for the S1 state of the isolated DA. Analyzing the lengths of the C–O and aromatic C–C bonds, it is found that they do not differ substantially, one of the C–O bonds being shorter by only 0.01 Å but most importantly, the length of the aromatic C–C bonds does not exceed 1.4 Å which should be between 1.4 and 1.42 for the S1 state equilibrium geometry of the isolated DA (see bond distance values of S1 equilibrium geometry given in Section 3.2). Accordingly, it can be said that, the relaxation of the DA lying on the graphene surface, after the characteristic DA-type vertical excitation, does not follow the geometric deformation observed for its S1 state in its isolated case, but rather, it is more similar to the bond values found for S0 ground state geometry. During the geometric relaxation, DA comes closer to the surface through its OH fragments (on average by 0.08 Å), while the NH2 fragment moves away (by 0.09 Å). The charge transfer effects increase from 0.149 e to 0.217 e and the final order of the excited state is 26th.
DAQ–graphene.
Similar to DA, only the relaxations of S1, locally excited DAQ and CT-type excited states were investigated. (i) S1 – in contrast to the case of DA, where no geometry changes were observed in the DA molecule itself during the excitation at S1 state, significant changes were observed for DAQ. The most significant changes are seen for the C
O bonds, where they change from 1.206 Å and 1.208 Å in the ground state to 1.242 Å and 1.244 Å in the S1 state. These changes also affect the hexagonal ring configuration, in particular the C–C bond to which the carbonyl groups are attached. Namely, from 1.553 Å the bond length is shorten to 1.505 Å. The relative position of DAQ with respect to the plane of graphene also changes, with the O atoms of the carbonyl groups approaching the graphene from 3.14 Å and 3.15 Å to 2.84 Å and 2.85 Å while the distance of NH2 from the plane of graphene remains almost unchanged (3.51 Å). After geometric optimization, the absorption wavelength of S1 also changes significantly to 651 nm. The charge transfer value obtained during vertical excitation also jumps enormously, from −0.034 e to −0.736 e, clearly suggesting that during geometry relaxation the localized excitation character on the GrNP diminishes and the CT-type excitation character increases. Since the geometry deformation for DAQ in the S1 excited state is significant compared to the ground state geometry, the method used for DA to determine the fluorescence rate in the framework of the harmonic oscillator approach is no longer valid. Therefore, in this case, neither the fluorescence rate nor the lifetime was computed; (ii) S2 – S2 is considered mostly locally excited state on DAQ, where an additional slight charge transfer occurs from the DAQ to the graphene surface. Geometry optimization with the root following constrain leads to a slight detachment of carbonyl groups, from 3.14 Å and 3.19 Å to 3.19 Å and 3.26 Å, respectively. The equilibrium geometry will also be S1 as the final state order, but this S1 state is only a local minimum compared to the geometry obtained in the previous S1 state optimization, with a conformational energy difference of 1.404 kcal mol−1. Also, the geometry changes observed in the DAQ are more typical to the S1 equilibrium geometry of the isolated DAQ, i.e. the length of the C–C bond from the six-membered ring, which includes the carbonyl groups, is not shortened but stretched, from 1.553 Å to 1.616 Å. The amount of transferred charge remains unchanged, namely +0.050 e transferred from DAQ to the graphene surface. (iii) S3 – considering the root following condition, the geometry optimization of the S3 state leads to the same equilibrium geometry as found for S1. For geometry relaxation and charge transfer effects, see the results presented for the S1 case; (iv) S16 – for this case, it was found that the carbonyl groups move closer (Δ (O⋯GrNP) = −0.14 Å to the graphene surface relative to their ground state geometry, while the amine group moves away (Δ (N⋯GrNP) = +0.07 Å)), so that the relative position of the DAQ becomes more tilted. The internal geometry of the DAQ also changes slightly, e.g. the C
O bonds slightly increase (≈0.02 Å) and the C–C bond from the hexagonal ring containing these carbonyl groups decreases by the same difference. Furthermore, the amount of transferred charge decreases from −0.698 e to −0.324 e and the final order of the excited state is the 14th; (v) S18 – the equilibrium geometry is identical to that found for S16, the value of the transferred charge is similarly reduced from −0.756 e to −0.315 e, and the final order of the excited state is also the 14th; (vi) S21 – the only change compared to the previous two cases is that, like the carbonyl groups, the amine group is come closer to the surface, but the trend is similar. Namely, the transferred charge decreases from −0.799 e to −0.473 e, and the final order of the state becomes the 18th.
4 Conclusions
In the present work, the equilibrium geometry structures and light absorption properties of the dopamine (DA) and dopamine-o-quinone (DAQ) adsorbed on the graphene surface has been investigated using the ground state and linear-response time-dependent density functional theories. Two types of graphene nanostructures were considered, one of them with optimized C–C bond length as the model system for graphene nanoparticles (GrNP), while the second being a similar system but with fixed C–C bond length (1.42 Å) as the model system for graphene 2D sheet (GrS). For both the GrNP and GrS model systems, the theoretical UV-Vis spectra show a more intense peak around 270 nm, which is consistent with the experimental results and is due to higher electronic transitions. It has been shown that the simplified graphene model consists in a finite-dimensional aromatic hydrocarbon system with discrete electronic states, can reproduce the main features of the experimental UV spectrum of graphene and, as a surface model, can act as a proper environment in interaction with the adsorbed molecules. Analyzing the natural difference orbital profiles characteristic for the vertical electronic excitation three types of electronic transitions were observed in the cases of graphene–DA and graphene–DAQ binary complexes. Namely, locally excited on graphene, locally excited on the molecule and charge transfer (CT) states characteristic for the charge migration induced by the excitation. For CT states, two different types of states have been identified, one when the charge is transferred from the molecule to the surface, called molecule-to-surface CT (or MSCT), and the other when the transfer occurs from the surface to the molecule, called surface-to-molecule CT (or SMCT). MSCT is characteristic of DA, while SMCT is characteristic of DAQ, and the difference between the two cases are given by the presence of an energetically low-lying unoccupied orbital (LUMO+1) that allows charge transfer from the surface to the molecule in the case of DAQ. Analyzing the spectral profiles of the UV-Vis absorption spectra, it was found that, the fingerprints of excited electronic states associated with the adsorbed molecules on the graphene surface cannot be seen in the spectrum, as they are mostly suppressed by the characteristically broad spectral shape of graphene, which is given by the lower-lying (typically the first ten) electronically excited states. As far as the relaxation dynamics of the excited states are concerned, two different scenarios were obtained for the two molecules, DA and DAQ. In the case of DA, it is observed that the molecule itself does not suffer any geometric change after the electronic excitation, only its relative position with respect to the surface changes and, of course, the quality of the CT states. The opposite effect is true for DAQ, that is, the ground-state geometry of DAQ changes substantially during the geometry relaxation of locally-DAQ and CT-type excited states. This different behavior is possible because the hydroxyl groups in the dopamine molecule have been replaced by carbonyls.
Conflicts of interest
There are no conflicts to declare.
Acknowledgements
This research was funded by the UEFISCDI public institution under the Romanian Ministry of Education, project code: PN-III-P4-ID-PCE-2020-0770. We are also grateful for the financial support from Romanian Ministry of Research, Innovation and Digitization through Programme 1 – Development of the National Research and Development System, Subprogram 1.2 – Institutional Performance – Funding Projects for Excellence in RDI, contract no. 37PFE/30.12.2021. The authors thank INCDTIM, Cluj-Napoca Data Center, for providing computer facilities.
Notes and references
- J. T. Yates and H. Petek, Chem. Rev., 2006, 106, 4113–4115 CrossRef CAS.
- Y. Matsumoto, Bull. Chem. Soc. Jpn., 2007, 80, 842–855 CrossRef CAS.
- C. Sousa, S. Tosoni and F. Illas, Chem. Rev., 2013, 113, 4456–4495 CrossRef CAS PubMed.
- J. Zhao, M. Feng, D. B. Dougherty, H. Sun and H. Petek, ACS Nano, 2014, 8, 10988–10997 CrossRef CAS PubMed.
-
J. L. Gray, The Physics of the Solar Cell, John Wiley & Sons, Ltd, 2010, pp. 82–129 Search PubMed.
- S. M. Brülls, V. Cantatore, Z. Wang, P. L. Tam, P. Malmberg, J. Stubbe, B. Sarkar, I. Panas, J. Mårtensson and S. Eigler, Chem. – Eur. J., 2020, 26, 6694–6702 CrossRef PubMed.
- C. Frischkorn and M. Wolf, Chem. Rev., 2006, 106, 4207–4233 CrossRef CAS PubMed.
- T. L. Thompson and J. T. Yates, Chem. Rev., 2006, 106, 4428–4453 CrossRef CAS PubMed.
- Á. Morales-García, F. Viñes, C. Sousa and F. Illas, J. Phys. Chem. Lett., 2023, 14, 3712–3720 CrossRef PubMed.
- Q. Guo, C. Zhou, Z. Ma, Z. Ren, H. Fan and X. Yang, Ann. Rev. Phys. Chem., 2018, 69, 451–472 CrossRef CAS PubMed.
- K. Sharma, V. Sharma and S. S. Sharma, Nanoscale Res. Lett., 2018, 13, 381 CrossRef PubMed.
- J. Kramer, S. Soukiazian, S. Mahoney and J. Hicks-Garner, J. Power Sources, 2012, 210, 122–128 CrossRef CAS.
- N. N. Sakhadeo and T. U. Patro, Ind. Eng. Chem. Res., 2022, 61, 5366–5387 CrossRef CAS.
- A. Bandyopadhyay, K. D. Traxel and S. Bose, Mater. Sci. Eng., R, 2021, 145, 100609 CrossRef PubMed.
- Y. Wang, G. Zheng, N. Jiang, G. Ying, Y. Li, X. Cai, J. Meng, L. Mai, M. Guo, Y. S. Zhang and X. Zhang, Nat. Rev. Methods Primers, 2023, 3, 68 CrossRef CAS.
- H. Lee, S. M. Dellatore, W. M. Miller and P. B. Messersmith, Science, 2007, 318, 426–430 CrossRef CAS PubMed.
- J. H. Ryu, P. B. Messersmith and H. Lee, ACS Appl. Mater. Interfaces, 2018, 10, 7523–7540 CrossRef CAS PubMed.
- J. Liebscher, R. Mrówczyński, H. A. Scheidt, C. Filip, N. D. Hădade, R. Turcu, A. Bende and S. Beck, Langmuir, 2013, 29, 10539–10548 CrossRef CAS PubMed.
- Q. Lyu, N. Hsueh and C. L. L. Chai, Polym. Chem., 2019, 10, 5771–5777 RSC.
- Y. Ding, L.-T. Weng, M. Yang, Z. Yang, X. Lu, N. Huang and Y. Leng, Langmuir, 2014, 30, 12258–12269 CrossRef CAS PubMed.
- V. Ball, Front. Bioeng. Biotechnol., 2018, 6, 109 CrossRef PubMed.
- H. A. Lee, E. Park and H. Lee, Adv. Mater., 2020, 1907505 CrossRef CAS PubMed.
- K. Lee, M. Park, K. G. Malollari, J. Shin, S. M. Winkler, Y. Zheng, J. H. Park, C. P. Grigoropoulos and P. B. Messersmith, Nat. Commun., 2020, 11, 4848 CrossRef CAS PubMed.
- D. Aguilar-Ferrer, J. Szewczyk and E. Coy, Catal. Today, 2022, 397–399, 316–349 CrossRef CAS.
- Z. Qin, D. Li, Y. Ou, S. Du, Q. Jiao, J. Peng and P. Liu, Crystals, 2023, 13, 976 CrossRef CAS.
- M. L. Alfieri, L. Panzella and A. Napolitano, Eur. J. Org. Chem., 2024, e202301002 CrossRef CAS.
- M. L. Mueller, X. Yan, J. A. McGuire and L. S. Li, Nano Lett., 2010, 10, 2679–2682 CrossRef CAS PubMed.
- S. Kaniyankandy, S. N. Achary, S. Rawalekar and H. N. Ghosh, J. Phys. Chem. C, 2011, 115, 19110–19116 CrossRef CAS.
- F. R. Baptista, S. A. Belhout, S. Giordani and S. J. Quinn, Chem. Soc. Rev., 2015, 44, 4433–4453 RSC.
- V. Strauss, R. A. S. er, F. Hauke, A. Hirsch and D. M. Guldi, J. Am. Chem. Soc., 2015, 137, 13079–13086 CrossRef CAS PubMed.
- S. Zhu, Y. Song, J. Wang, H. Wan, Y. Zhang, Y. Ning and B. Yang, Nano Today, 2017, 13, 10–14 CrossRef CAS.
- X.-M. Huang, L.-Z. Liu, S. Zhou and J.-J. Zhao, Front. Phys., 2020, 15, 33301 CrossRef.
- A. Pandikumar, G. T. S. How, T. P. See, F. S. Omar, S. Jayabal, K. Z. Kamali, N. Yusoff, A. Jamil, R. Ramaraj, S. A. John, H. N. Lim and N. M. Huang, RSC Adv., 2014, 4, 63296–63323 RSC.
- Y. Luo, L. Ma, X. Zhang, A. Liang and Z. Jiang, Nanoscale Res. Lett., 2015, 10, 230 CrossRef PubMed.
- Z. Hsine, R. Mlika, N. Jaffrezic-Renault and H. Korri-Youssoufi, Chemosensors, 2022, 10, 249 CrossRef CAS.
- M. Abrantes, D. Rodrigues, T. Domingues, S. S. Nemala, P. Monteiro, J. Borme, P. Alpuim and L. Jacinto, J. Nanobiotechnol., 2022, 20, 495 CrossRef CAS PubMed.
- M. Chatterjee, P. Nath, S. Kadian, A. Kumar, V. Kumar, P. Roy, G. Manik and S. Satapathi, Sci. Rep., 2022, 12, 9061 CrossRef CAS PubMed.
- C. Silva, F. Simon, P. Friedel, P. Pötschke and C. Zimmerer, Nanomaterials, 2019, 9, 902 CrossRef CAS PubMed.
- P. S. Kasbe, H. Gade, S. Liu, G. G. Chase and W. Xu, ACS Appl. Bio Mater., 2021, 4, 5180–5188 CrossRef CAS PubMed.
- W. Li, W. Yang, M. Wu, M. Zhao and X. Lu, Electrochim. Acta, 2022, 426, 140776 CrossRef CAS.
- L. Zhou, Y. Zong, Z. Liu and A. Yu, Renewable Energy, 2016, 96, 333–340 CrossRef CAS.
- A. Jedrzak, T. Rebiś, M. Nowicki, K. Synoradzki, R. Mrówczyński and T. Jesionowski, Appl. Surf. Sci., 2018, 455, 455–464 CrossRef CAS.
- F. Vulcano, A. Kovtun, C. Bettini, Z. Xia, A. Liscio, F. Terzi, A. Heras, A. Colina, B. Zanfrognini, M. Melucci, V. Palermo and C. Zanardi, 2D Mater., 2020, 7, 024007 CrossRef CAS.
- R. Mrówczyński, A. Bunge and J. Liebscher, Chem. – Eur. J., 2014, 20, 1–8 CrossRef PubMed.
- D.-D. Wang, X. Gao, L. Zhao, J. Zhou, S. Zhuo, Z. Yan and W. Xing, RSC Adv., 2018, 8, 16044–16051 RSC.
- D. Damberga, V. Fedorenko, K. Grundšteins, S. Altundal, A. Šutka, A. Ramanavićius, E. Coy, R. Mrówczyński, I. Iatsunskyi and R. Viter, Nanomaterials, 2020, 10, 2438 CrossRef CAS PubMed.
- R. Mrówczyński, ACS Appl. Mater. Interfaces, 2018, 10, 7541–7561 CrossRef PubMed.
- X. Xie, C. Mao, X. Liu, L. Tan, Z. Cui, X. Yang, S. Zhu, Z. Li, X. Yuan, Y. Zheng, K. W. K. Yeung, P. K. Chu and S. Wu, ACS Cent. Sci., 2018, 4, 724–738 CrossRef CAS PubMed.
- C. Wu, X. Guan, J. Xu, Y. Zhang, Q. Liu, Y. Tian, S. Li, X. Qin, H. Yang and Y. Liu, Biomaterials, 2019, 205, 106–119 CrossRef CAS PubMed.
- S. Mei, X. Xu, R. D. Priestley and Y. Lu, Chem. Sci., 2020, 11, 12269–12281 RSC.
- I. Zmerli, J.-P. Michel and A. Makky, Multifunct. Mater., 2021, 4, 022001 CrossRef CAS.
- R. Chen, B. Lin and R. Luo, Heliyon, 2022, 8, e12105 CrossRef CAS PubMed.
- M. Wang, B. Li, Y. Du, H. Bu, Y. Tang and Q. Huang, RSC Adv., 2021, 11, 8420–8429 RSC.
- A. Falamas, A. Petran, A.-M. Hada and A. Bende, Int. J. Mol. Sci., 2022, 23, 5483 CrossRef CAS PubMed.
- K. Hirata, K.-I. Kasai, K. Yoshizawa, G. Grégoire, S.-I. Ishiuchi and M. Fujii, Phys. Chem. Chem. Phys., 2022, 24, 10737–10744 RSC.
- S. Chopra and L. Maidich, RSC Adv., 2014, 4, 50606–50613 RSC.
- H. Vovusha and B. Sanyal, RSC Adv., 2015, 5, 4599–4608 RSC.
- E. Gruber, R. A. Wilhelm, R. Pétuya, V. Smejkal, R. Kozubek, A. Hierzenberger, B. C. Bayer, I. Aldazabal, A. K. Kazansky, F. Libisch, A. V. Krasheninnikov, M. Schleberger, S. Facsko, A. G. Borisov, A. Arnau and F. Aumayr, Nat. Commun., 2016, 7, 13948 CrossRef CAS PubMed.
- Y. Miyamoto and A. Rubio, J. Phys. Soc. Jpn., 2018, 87, 041016 CrossRef.
- M. Cayambe, C. Zambrano, T. Tene, M. Guevara, G. T. Usca, H. Brito, R. Molina, D. Coello-Fiallos, L. S. Caputi and C. V. Gomez, Mater. Today: Proc., 2021, 37, 4027–4030 CAS.
- M. A. Jabed, J. Zhao, D. Kilin and T. Yu, J. Phys. Chem. C, 2021, 125, 14979–14990 CrossRef CAS.
- S. Özönder, C. Ünlü, C. Güleryüz and L. Trabzon, ACS Omega, 2023, 8, 2112–2118 CrossRef PubMed.
- P. P. Fehér, Á. Madarász and A. Stirling, Chem. Methods, 2023, 3, e202200069 CrossRef.
- N. A. Besley, Chem. Phys. Lett., 2004, 390, 124–129 CrossRef CAS.
- N. A. Besley and A. J. Blundy, J. Phys. Chem. B, 2006, 110, 1701–1710 CrossRef CAS PubMed.
- A. D. Laurent, C. Adamo and D. Jacquemin, Phys. Chem. Chem. Phys., 2014, 16, 14334–14356 RSC.
- J. M. Herbert, X. Zhang, A. F. Morrison and J. Liu, Acc. Chem. Res., 2016, 49, 931–941 CrossRef CAS PubMed.
-
N. S. Hill and M. L. Coote, in Annu. Rep. Comput. Chem., ed. D. A. Dixon, Elsevier, 2019, vol. 15, pp. 203–285 Search PubMed.
- M. Coros, F. Pogăcean, L. Măgerusan, M.-C. Roşu, A. S. Porav, C. Socaci, A. Bende, R.-I. Stefan-van Staden and S. Pruneanu, Sens. Actuators, B, 2018, 256, 665–673 CrossRef CAS.
- J.-D. Chai and M. Head-Gordon, Phys. Chem. Chem. Phys., 2008, 10, 6615–6620 RSC.
- S. Grimme, J. Antony, S. Ehrlich and H. Krieg, J. Chem. Phys., 2010, 132, 154104 CrossRef PubMed.
- S. Grimme, S. Ehrlich and L. Goerigk, J. Comput. Chem., 2011, 32, 1456–1465 CrossRef CAS PubMed.
- F. Weigend and R. Ahlrichs, Phys. Chem. Chem. Phys., 2005, 7, 3297–3305 RSC.
- F. Neese, Wiley Interdiscip. Rev.: Comput. Mol. Sci., 2022, 12, e1606 Search PubMed.
- F. Neese, F. Wennmohs, U. Becker and C. Riplinger, J. Chem. Phys., 2020, 152, 224108 CrossRef CAS PubMed.
- S. Hirata and M. Head-Gordon, Chem. Phys. Lett., 1999, 314, 291–299 CrossRef CAS.
- F. Neese, F. Wennmohs, A. Hansen and U. Becker, Chem. Phys., 2009, 356, 98–109 CrossRef CAS.
- F. Weigend, Phys. Chem. Chem. Phys., 2006, 8, 1057–1065 RSC.
- A. Hellweg, C. Hättig, S. Höfener and W. Klopper, Theor. Chem. Acc., 2007, 117, 587–597 Search PubMed.
- H. Li and J. H. Jensen, Theor. Chem. Acc., 2002, 107, 211–219 Search PubMed.
- B. de Souza, F. Neese and R. Izsák, J. Chem. Phys., 2018, 148, 034104 CrossRef PubMed.
- Q. Peng, Y. Yi, Z. Shuai and J. Shao, J. Chem. Phys., 2007, 126, 114302 CrossRef PubMed.
- R. R. Valiev, V. Cherepanov, R. Nasibullin, D. Sundholm and T. Kurtén, Phys. Chem. Chem. Phys., 2019, 21, 18495–18500 RSC.
- T. Lu and F. Chen, J. Comput. Chem., 2012, 33, 580–592 CrossRef CAS PubMed.
- A.-R. Allouche, J. Comput. Chem., 2011, 32, 174–182 CrossRef CAS PubMed.
- M. D. Hanwell, D. E. Curtis, D. C. Lonie, T. Vandermeersch, E. Zurek and G. R. Hutchison, J. Cheminf., 2012, 4, 17 CAS.
-
R. Dennington, T. Keith and J. Millam, Gaussview 6.1.1, Semichem Inc., Shawnee Mission, KS, 2009 Search PubMed.
- A. H. Castro-Neto, F. Guinea, N. M. R. Peres, K. S. Novoselov and A. K. Geim, Rev. Mod. Phys., 2009, 81, 109–162 CrossRef CAS.
- L. Khalafi, M. Rafiee and S. Fathi, Spectrochim. Acta, Part A, 2014, 118, 695–701 CrossRef CAS PubMed.
- K. F. Mak, M. Y. Sfeir, Y. Wu, C. H. Lui, J. A. Misewich and T. F. Heinz, Phys. Rev. Lett., 2008, 101, 196405 CrossRef PubMed.
- K. F. Mak, L. Ju, F. Wang and T. F. Heinz, Solid State Commun., 2012, 152, 1341–1349 CrossRef CAS.
- L. Yang, J. Deslippe, C.-H. Park, M. L. Cohen and S. G. Louie, Phys. Rev. Lett., 2009, 103, 186802 CrossRef PubMed.
- P. E. Trevisanutto, M. Holzmann, M. Côté and V. Olevano, Phys. Rev. B: Condens. Matter Mater. Phys., 2010, 81, 121405 CrossRef.
- R. Denk, M. Hohage, P. Zeppenfeld, J. Cai, C. A. Pignedoli, H. Söde, R. Fasel, X. Feng, K. Müllen, S. Wang, D. Prezzi, A. Ferretti, A. Ruini, E. Molinari and P. Ruffieux, Nat. Commun., 2014, 5, 4253 CrossRef CAS PubMed.
- Y. Ge and T. S. Fisher, Front. Nanotechnol., 2022, 4, 999292 CrossRef.
- S. Kim, S. W. Hwang, M.-K. Kim, D. Y. Shin, D. H. Shin, C. O. Kim, S. B. Yang, J. H. Park, E. Hwang, S.-H. Choi, G. Ko, S. Sim, C. Sone, H. J. Choi, S. Bae and B. H. Hong, ACS Nano, 2012, 6, 8203–8208 CrossRef CAS PubMed.
- Z. Jin, P. Owour, S. Lei and L. Ge, Curr. Opin. Colloid Interface Sci., 2015, 20, 439–453 CrossRef CAS.
- X. E. Zhao, C. H. Lei, Y. H. Wang, F. Qu, S. Y. Zhu, H. Wang and J. M. You, RSC Adv., 2016, 6, 72670–72675 RSC.
- D. K. Rai and A. Shukla, Sci. Rep., 2019, 9, 7958 CrossRef PubMed.
- T. Tasnim, M. J. Ayodele and S. P. Pitre, J. Org. Chem., 2022, 87, 10555–10563 CrossRef CAS PubMed.
- S. Kümmel, Adv. Energy Mater., 2017, 7, 1700440 CrossRef.
- N. T. Maitra, Annu. Rev. Phys. Chem., 2022, 73, 117–140 CrossRef CAS PubMed.
- D. Mester and M. Kállay, J. Chem. Theory Comput., 2022, 18, 1646–1662 CrossRef CAS PubMed.
- J. M. Herbert, Phys. Chem. Chem. Phys., 2024, 26, 3755–3794 RSC.
- J. Liang, X. Feng, D. Hait and M. Head-Gordon, J. Chem. Theory Comput., 2022, 18, 3460–3473 CrossRef CAS PubMed.
- P. Avouris and B. N. J. Persson, J. Phys. Chem., 1984, 88, 837–848 CrossRef CAS.
- H. Petek, J. Chem. Phys., 2012, 137, 091704 CrossRef PubMed.
- G. Floß, G. Granucci and P. Saalfrank, J. Chem. Phys., 2012, 137, 234701 CrossRef PubMed.
- H.-T. Chen, J. Chen, D. V. Cofer-Shabica, Z. Zhou, V. Athavale, G. Medders, M. F. S. J. Menger, J. E. Subotnik and Z. Jin, J. Chem. Theory
Comput., 2022, 18, 3296–3307 CrossRef CAS PubMed.
- S. Chen, N. Ullah, Y. Zhao and R. Zhang, ChemPhysChem, 2019, 20, 1–6 CrossRef.
- W. Ouyang, W. Dou and J. E. Subotnik, J. Chem. Phys., 2015, 142, 084109 CrossRef PubMed.
- W. Dou, A. Nitzan and J. E. Subotnik, J. Chem. Phys., 2015, 142, 084110 CrossRef PubMed.
- W. Dou, A. Nitzan and J. E. Subotnik, J. Chem. Phys., 2015, 142, 234106 CrossRef PubMed.
- W. Dou, A. Nitzan and J. E. Subotnik, J. Chem. Phys., 2015, 143, 189902 CrossRef PubMed.
- J. Chen, Z. Jin, W. Dou and J. Subotnik, J. Phys. Chem. C, 2021, 125, 2884–2899 CrossRef CAS.
- Z. Jin and J. E. Subotnik, J. Chem. Theory Comput., 2021, 17, 614–626 CrossRef CAS PubMed.
- A. Bende, A.-A. Farcas, A. Falamas and A. Petran, Phys. Chem. Chem. Phys., 2022, 24, 29165–29175 RSC.
- A. Bende and A.-A. Farcas, Int. J. Mol. Sci., 2023, 24, 2906 CrossRef CAS PubMed.
- C. H. Lui, K. F. Mak, J. Shan and T. F. Heinz, Phys. Rev. Lett., 2010, 105, 127404 CrossRef PubMed.
- J. Wang, S. Cao, Y. Ding, F. Ma, W. Lu and M. Sun, Sci. Rep., 2016, 6, 24850 CrossRef CAS PubMed.
|
This journal is © the Owner Societies 2024 |