DOI:
10.1039/D4MA00411F
(Perspective)
Mater. Adv., 2024, Advance Article
Polyhydroxyalkanoates in emerging recycling technologies for a circular materials economy
Received
19th April 2024
, Accepted 26th July 2024
First published on 2nd August 2024
Abstract
Circular polymer systems, specifically polyesters operating through chemical and biological technologies, are approaching a critical moment of industrial adoption and scale-up feasibility. At the same time, polyhydroxyalkanoate (PHA) production, scale-up, and resulting material development is converging toward commodity applications. The current PHA end-of-life philosophy, however, focalizes leveraging inherent biodegradability to circumvent plastic waste accumulation. If indeed a substantial replacement of incumbent single-use plastics with PHA alternatives is to be met in commercial manufacture, we emphasize the importance of linking PHA development with feasible polymer recycling technologies. In other words, a PHA materials economy is significantly more carbon- and cost-favorable when efficient mechanical (reprocessing), chemical (deconstruction, depolymerization), or biological (enzymatic) recycling is prioritized over biodegradation or composting. In this perspective, we discuss strategies for PHA recyclable-by-design principles, guidable by developing machine learning tools, as well as material compatibility with closed-loop recycling technologies. Additionally, we posit compelling life-cycle assessment incentives for adopting polymer reclamation over competing pathways. Ultimately, we hope this narrative further inspires the alignment between PHA design with growing calls for a circular material economy.
PHAs in the circular economy
Material circularity is arguably the top priority of the polymer research community, responding to growing calls for reductions in environmental, climate, and energy consequences related to plastics manufacture and consumption. Today's commodity plastics are dominated by single-use, non-renewable polyethylene (PE) and polypropylene (PP). Forecasts suggest 20% of all oil and natural gas consumption will be redirected towards supplying 1 billion tons of fossil-derived plastics by 2050.1,2 The recalcitrant nature of polyolefin materials (PE and PP, primary materials in single use packaging) in particular precludes any capability for closed-loop recycling, promoting a linear material flow dominated by landfilling, pyrolysis to fuels, and negligent discard to the natural environment at end-of-life (EoL). Models by Meys et al. project that a net zero and circular materials economy must combine both biomass and CO2 utilization with an effective recycling rate of at least 70%,3 relieving an estimated 34–53% of current energy demand from plastics manufacture. Furthermore, redesigning plastic production to be fully decoupled from fossil fuels, complemented by lower manufacture energy and high recycling rates, will substantially improve carbon footprint.4 Thus, sustainable practices at both the means (production) and ends (disposal) of polymer life-span are required for a truly circular, net-zero materials economy.
Polyesters have the potential to dominate a future bio-based and circular plastics system. Polyesters contain ester linkages that are prevalent in naturally occurring materials (e.g., cutin, suberin), which can readily enable the use of bio-based feedstocks and more energy efficient deconstruction into feedstock materials through known chemical and biological recycling techniques. Polyhydroxyalkanoates (PHAs) are a class of polyesters that have attracted significant attention over the last few decades as a “natural polymer” class that can support the transition towards a circular materials economy. PHAs are naturally occurring in Earth's biome, whereby synthesis occurs through microbial metabolic pathways, and thus the resulting polymers demonstrate high biocompatibility with environmental ecosystems.5–7 This property affords timely degradation in home-compost (“backyard”), soil, and freshwater conditions8 (depending on the PHA side chain functionality) in contrast to other synthetic “biodegradable” plastics such as poly(lactic acid) (PLA) which require specific industrial compost facilities for biological degradation.9–11 Additionally, PHAs have demonstrated polyolefin-like mechanical properties, remarkably low water vapor and oxygen permeability,12,13 and high crystallinity or melt temperatures (Tm) for processability.14,15
Another compelling aspect of PHAs lies in their versatility in synthesis, which can be achieved through either a biological or chemo-catalytic route. Each method presents distinct advantages and disadvantages, as illustrated graphically in Fig. 1 and 2. Several compelling arguments are posited for an all-PHA materials economy, including the mitigation of issues inherent in the recycling of mixed polymer products and waste streams. In a recent commentary, Quinn et al. illustrate a mono-material approach to multi-layer packaging films wherein sealant, tie, structural, and barrier layers can be accessed entirely by poly(3-hydroxybutyrate) (P3HB) with varying stereomicrostructures.16 Acknowledging that biological pathways for PHA production have an inherent limitation to the isotactic poly-(R) stereo-microstructure (Fig. 1), this mono-material approach indicates that the future of PHA manufacture will likely rely on both natural and synthetic routes. Exciting advancements in chemo-catalytic synthetic routes are poised to revolutionize the production of PHA-based polymers, offering a promising alternative to conventional petroleum-based plastics. These innovative methods hold the potential to match, or even surpass, the performance of current commodity plastics. Moreover, the integration of machine learning tools (ML) opens new avenues for designing PHA polymers with enhanced properties and performance characteristics. By leveraging ML algorithms, we can target tailored PHA backbones to exhibit advantageous attributes, further propelling the competitiveness of these sustainable materials.
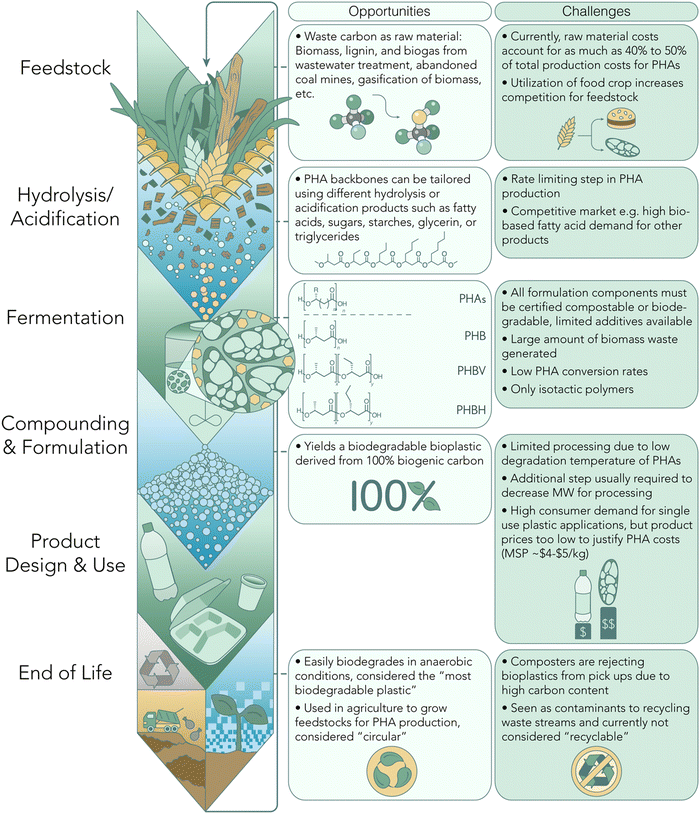 |
| Fig. 1 Current PHA life-cycle trade-offs for biologically synthesized PHAs. Challenges and opportunities corresponding with biological PHA production considering feedstock sourcing, synthetic production, application-oriented engineering, and end-of-life avenues. | |
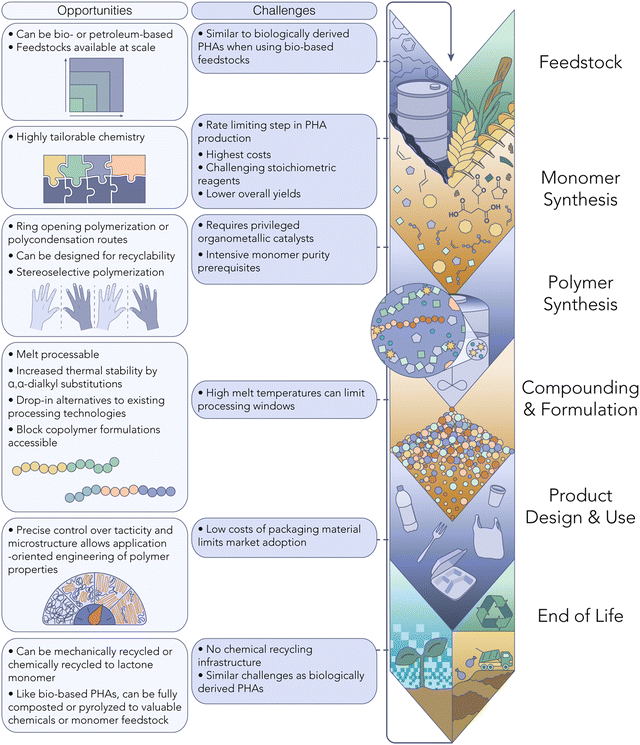 |
| Fig. 2 Current PHA life-cycle trade-offs for chemo-catalytically synthesized PHAs. Challenges and opportunities corresponding with biological PHA production considering feedstock sourcing, synthetic production, application-oriented engineering, and end-of-life avenues. | |
As the demand for natural polymers continues to rise, it becomes imperative to address EoL solutions for these materials. While PHAs currently available on the market today are often promoted as both home and industrially compostable,17 there is a pressing challenge to contend with. Many composting facilities worldwide are increasingly reluctant to accept compostable plastics due to concerns about the high carbon and oxygen content, which can disrupt the balance and health of composting processes. While biodegradability is a key advantage for PHA adoption, the plastics economy is moving towards scaling of mechanical recycling, pyrolysis, solvolysis, and enzymatic hydrolysis as primary avenues of plastic waste management.18 It is thus important to understand PHA compatibility in these growing infrastructures to achieve circularity. Recognizing the limitations of the current waste management system,19 we should consider how emerging plastic waste streams, such as PHAs, could benefit from multiple end-of-life options. Ideally, these materials should be recyclable to monomers first, compostable second, and ultimately biodegradable as a safety net.
This perspective provides a broad yet insightful discussion on the pivotal role of chemo-catalytic and biologically synthesized PHAs within the circular economy framework. We explore the opportunities and challenges inherent in both production routes, emphasizing the potential of leveraging advanced ML tools to design innovative PHA-based materials aligning with principles of polymer circularity. Additionally, we examine the significance of PHAs in the circular economy landscape, addressing their compatibility with both established and emerging recycling platforms. Furthermore, we delve into the life cycle impacts of PHAs and underscore the importance of designing plastic materials with recyclability as a central focus.
Biological PHA synthesis
It has been nearly a century since PHAs were first discovered as the intracellular product of carbon and energy storage in Bacillus megaterium.20 Since then, PHA polymers with increasing pendent chain lengths beyond that of methyl-substituted poly(3-hydroxybutyrate) (P3HB) have been discovered in the same and other bacterial strains from a range of different environments.21,22 The increasing access and variability in PHAs have evolved not only with chemical synthesis operating on strategic monomer design, but through emerging techniques for genetic modification or enzyme engineering to expand both microorganism feedstocks and products.17 These include thermoplastics spanning from short-chain length (scl) C1 (e.g., brittle P3HB) to medium-chain length (mcl) C9+ (e.g., elastomeric P3HDD).23
Notably, PHAs available on the commercial market are predominantly produced by microorganisms. When compared to the chemo-catalytic production of PHAs, various tradeoffs emerge at different stages of production (Fig. 1). One limitation to the large-scale production of PHAs lies in the bio-based feedstocks available for PHA fermentation. Currently, feedstock accounts for 40–50% of the total production costs for PHAs.24–26 Historically, these feedstocks were limited to crops that also competed in the food market. However, the PHA industry is starting to shift to utilizing waste-based feedstocks for PHA production.27–31
A variety of PHA side-chain functionality and copolymer design can be achieved by modulating the feedstock and bacterial strain used for biological synthesis. Microbial engineering by Chen and colleagues demonstrated expressed combinations of acetoacetyl-CoA, 3-ketovaleryl-CoA, and 3-ketohexanoyl-CoA affords the corresponding 3HB, 3-hydroxyvalerate (3HV), and 3-hydroxyhexanoate (3Hx) units, respectively. In tandem, these genetic modifications can yield copolymers such as poly(3-hydroxybutyrate-co-3-hydroxyvalerate) (PHBV) and poly(3-hydroxybutyrate-co-hydroxyhexanoate) (PHBHx).32,33 Copolymers are increasingly deployed in consumer items and packaging films, leveraging differences in chain packing behavior to disrupt the high 3HB crystallinity for ductile and tough materials. PHBV and PHBHx have attracted significant attention for applications in packaging, textiles, and biomedical technologies such as implants, drug delivery, and tissue engineering.34 It is worth mentioning that PHAs have also recently been applied toward technologies requiring crosslinked architectures, such as elastomers, as a result of typically low parent polymer Tg. Specifically, Cywar et al. demonstrated the microbial production of mcl-PHAs equipped with alkene functionality for chemical installment of boronic ester crosslinks, yielding vitrimers with three-fold recyclability via melt reprocessing, chemical de-networking, and biodegradability.35
Despite the remarkable diversity in possible PHA structures, biological synthesis encounters limitations in controlling tacticity, microstructure, and molar mass of the final PHA product.36,37 Biological processes typically yield PHAs with molar mass in the hundreds of kilodaltons, necessitating a degradation processing step to lower the molecular weight before further processing into products. Additionally, the melt temperatures of resulting PHAs often approach the carbon degradation point, resulting in narrow processing windows and posing challenges for effective processing.
Chemo-catalytic PHA synthesis
Some of the challenges associated with biological PHA production can be overcome via synthetic routes (Fig. 2). Obtaining PHAs through chemo-catalytic synthesis affords precise control over the polymer MW, architecture and performance profile. Specifically, the monomer and catalyst can be selected to fine-tune the polymer tacticity and stereochemistry,38 in turn affecting the microstructure, a luxury currently not available via microbial PHA production.
Substantial innovation has recently come from chemo-catalytic PHA synthesis, whereby either β-butyrolactone or 8-membered diolide (8DL) monomers are engaged in ring-opening polymerization (ROP) by organometallic coordination catalysts.39,40 While P3HB is a rather brittle material, simple synthetic substitutions at the backbone β-position have become a modern tunability handle for optimizing thermal and mechanical properties. For example, Westlie et al. demonstrated that ROP of benzyl-substituted 8DL in the presence of an Yttrium-based complex returned high-Tg P3BnB.41 Other 8DL substitutions range from C1–C4 pendents, and behave identically to natural PHAs when isotactic stereochemistry is achieved. Upon evaluating the utilization of these 8DL monomers, however, it is noticeable that the multi-step synthesis, low yields, and use of unsustainable precursors or chemical reagents (e.g., alkyl halides) are hindersome to the scalability of these systems. More optimistically, Zhou et al. recently highlighted the nature-inspired production of methylated PHAs (PHMB) from the carbonylation of abundant and inexpensive 2-butene oxide.42
As with any innovative process, the chemo-catalytic pathway to PHA production entails its share of trade-offs. Given its relatively recent emergence, there's a scarcity of studies delving into the economic and life cycle implications of synthetic PHAs. While numerous monomers suitable for PHA synthesis can be derived from bio-based sources, most are not yet produced at commercial scales. Although some commercially available monomers, like β-butyrolactone, exist, their synthesis often involves complex, multi-step procedures, thus acting as a bottleneck for chemo-catalytic PHA production. Additionally, the synthesis of highly pure monomers necessary for polyester production entails energy-intensive purification processes. Lastly, the utilization of homogeneous organometallic catalysts in many reported PHA synthesis methods presents a significant cost barrier, necessitating further innovation in catalyst design to enhance feasibility.
While chemo-catalytic PHAs are not at the production scale of biologically synthesized PHAs, key learnings from their development have the potential to play a vital role in eliciting structure–property relationships and expediting the discovery of high-performance PHA candidates. More specifically, synthetic PHAs can greatly assist in directing biological engineering, which has also demonstrated tunability by varying the metabolic feedstock. A recent report from Li & Zhang et al. revealed that α,α-dimethyl di-substitution to β-butyrolactone, yielding P3Me2HB following ROP, circumvents thermally-triggered β-elimination, the main culprit for P3HB's low (<250 °C) thermal stability.43 Even more importantly, the geminal di-substitution promotes ring-closure to the 4-membered starting monomer during catalyzed depolymerization instead of the traditional thermodynamic trimer product, achieving a true chemically recyclable-to-monomer PHA. The achievement here is a powerful example of the potential translatability to metabolic engineering, which can subsequently select complementary renewable feedstocks with this and future target structures in mind.
Machine learning for next-generation PHA discovery
As noted at the start of this perspective, the future manufacture of PHAs is likely one that integrates both biological and chemo-catalytic routes for production. Given the immense design space available for tailored PHAs (Fig. 1 and 2), it is critical that intelligent and accurate screening strategies narrow the possibilities to the most promising formulations for synthesis and characterization. Novel approaches to map molecular features to polymer performance are being pursued via the development and application of increasingly advanced high-throughput ML tools for polymer property prediction.44–46 For example, PolyID (Fig. 3) is a multioutput, message passing neural network (MPNN) specifically designed to enable quantitative structure–property relationship (QSPR) analysis for polymers.47 From input monomer SMILES strings that encode chemical structure, in silico polymerization allows for specification of comonomer ratio (if applicable) and degree of polymerization. The PolyID MPNN, trained on ∼1800 experimental data points extracted from literature and existing polymer databases, predicts polymer performance attributes, including thermal, mechanical, and barrier properties with high accuracy (e.g., mean absolute error of ∼20 °C for Tg predictions). Additionally, PolyID was constructed to be interpretable, thus enabling not only the direct prediction of specific polymer properties, but also the development of structure–function relationships that can guide the establishment of design principles.
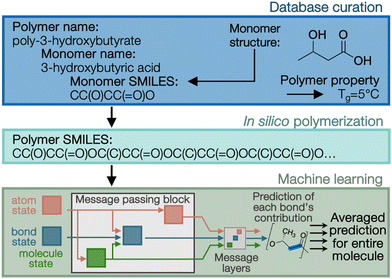 |
| Fig. 3 The main components of the PolyID machine learning tool. As illustrated, PolyID comprises three primary components: (1) a database of monomer structure(s) with mapping to polymer property (top) curated from literature, existing property databases, and experimental measurements, (2) an in silico polymerization scheme (middle), and (3) a message-passing neural network (MPNN) for high-fidelity polymer property prediction (bottom). In the context of chiral polymers such as PHAs, stereochemical information must be included at all three levels, namely the training data, the in silico polymerization, and into the MPNN, e.g. as atom or global features. | |
Given the dependence of polymer performance properties on stereochemistry, computational prediction tools must include tacticity when applied to PHAs.48–50 Experimental training databases must be assembled with extra care when incorporating data specifically from biologically produced PHAs due to their broader molecular weight distribution, co-monomer integration, and exclusive stereochemistry. ML models have been applied to PHA property prediction with some success for limited properties but have not incorporated tacticity, generally citing the scarcity of available data.51–53 Examples of how tacticity can be incorporated include atom tags at chiral centers or at the global feature level (e.g., through Pm, the probability of a meso-linkage between two repeat units). Advanced notations for specifying polymer structures such as BigSMILES,54 are another possible means for incorporating tacticity throughout the training-to-prediction workflow. Lastly, incorporating tacticity will be increasingly important as synthetic routes to PHAs allow for tailored tacticity, which is generally inaccessible biologically.
Going forward, there is great opportunity for computational tools to guide novel PHA design, but several challenges must be addressed. The relative dearth of publicly available data that links polymer structure, particularly tacticity-labeled data, to performance attributes is a significant hindrance to model prediction accuracy. One significant area of interest is the a priori formulation of EoL strategies for a given PHA chemistry. That is currently a significant challenge given the limited experimental data that links chemical structure to quantified recyclability, compostability, or biodegradability metrics. Ideally, these data would be produced experimentally, though this may constitute a prohibitively large investment of resources given the number of desired polymer chemistries and properties. Molecular modeling approaches to data generation could be employed to address this need. For example, high throughput data generation can be achieved by estimations of ring strain, enthalpy, entropy, etc. from density functional theory (DFT) or hydrophobicity, molecular mobility, density, etc. from molecular dynamics (MD) simulations.55,56 The development of empirical or theoretical relationships linking more readily accessible physical properties (e.g., Tg, crystallinity, Tm) with properties that are more tedious to measure (biodegradability, recyclability) could also be especially powerful when met with modern computing power.57 Alternative approaches to getting more out of less data can also be done at the ML stage with techniques such as transfer learning wherein abundant data collected on more easily measured/estimated properties can pre-train ML models to be further refined with less abundant, experimentally-measured properties.58–60
Life cycle impact considerations for PHAs
Given the diversity of synthetic protocols for PHAs, the environmental impacts of these polymers are highly dependent on the utilized feedstock, processing conditions, and EoL waste management. Most published life cycle assessment (LCA) studies of PHA manufacturing have been conducted on the biological production of PHAs, which currently lends to all commercially available PHA materials, and this section will therefore focus primarily on this route. LCAs of biological PHA manufacturing have reported greenhouse gas (GHG) emissions ranging from −4 to 6 kg CO2 kg−1, in comparison to 1.5 to 3.5 kg CO2 kg−1 for fossil-based plastics such as polyethylene terephthalate (PET), PE, and PP.61 Most GHG emissions in the production of PHAs are associated with feedstock pretreatment and product separation, although in some cases these energy- and consumable-related impacts are counteracted by GHG credits for the biogenic carbon stored in biomass-derived PHA s.62–64
Potential EoL routes for PHAs are outlined in Fig. 4. PHAs on the market today are promoted as compostable materials. We explore a simplified model scenario in which all global plastic packaging (∼140 million metric tonnes per year; MMT per year) is converted into PHAs and composted at the EoL.65 Most organic compounds decay during composting and release ∼80% of their original carbon content as GHGs (i.e., only ∼20% is stabilized in carbon-based soil compounds).66 Composting PHAs would therefore release 230 MMT per year of CO2 according to eqn (1) and a gaseous yield of 80%. These emissions would substantially neutralize the credits received for the utilization of biogenic carbon during PHAs manufacturing. To handle this quantity of compostable material, global composting capacity would need to double from its current 110 MMT of municipal solid organic waste.67 Such an infrastructure expansion would be challenging from both investment and technical perspectives, as the lack of nitrogen or phosphorous in PHA formulations could prevent the compost from retaining a suitable carbon to nitrogen/phosphorous ratio.
|
2(C4H6O2)n + 9O2 → 8CO2 + 6H2O
| (1) |
One of the key selling points of PHAs is their biodegradability, which can mitigate the impact of short lifetimes coupled with leakage and persistence in the environment. PHA microplastic litter has been shown to have fewer physical effects on marine plants and animals than some fossil-based plastics such as polystyrene (PS), PE, and PP.
68 If PHAs were leaked to marine environments at the current global plastic leakage rate of 5–13 MMT per year and, hypothetically, fully biodegraded, 10–27 MMT of biogenic CO
2 (<0.05% of global GHG emissions) would be released according to
eqn (1).
69 Alternatively, 100% of PHA packaging could be sent to landfill, the predominant EoL pathway for plastics today.
70,71 PHA is expected to anaerobically degrade in landfill into a mixture of CO
2 and CH
4 known as landfill gas (
eqn (2)),
72 which is often vented to the atmosphere.
73 Because CH
4 is a more potent GHG, an estimated 1590 MMT CO
2 eq. per year would be released, 1300 MMT CO
2 eq. per year of which could not be offset by biogenic carbon credits. This corresponds to approximately 2.5% of global annual GHG emissions.
|
4(C4H6O2)n + 6H2O → 7CO2 + 9CH4
| (2) |
It should be emphasized that these numbers are initial estimations and based on volumes and waste management systems for non-PHA based plastics. Regardless of the hypothetical nature of these estimations, the results highlight the importance of keeping valuable biogenic carbon in circulation and avoiding landfill and composting at EoL. When considering life cycle emissions, a net-zero emissions state can be potentially achieved when combining biomass/CO
2 utilization in the production of PHAs with high recycling rates
via existing or emerging technologies such as mechanical, chemical, or enzymatic recycling.
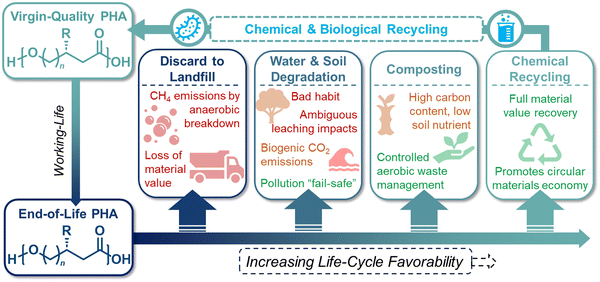 |
| Fig. 4 PHA end-of-life considerations. Avenues for waste-stream PHAs by increasing life-cycle favorability. It is important to note that the principles here are not directly translatable to other biopolymers such as PLA, for which landfilling is preferable to environmental discard. | |
Mechanical recycling of PHAs
Mechanical recycling represents the state-of-the-art of post-consumer plastic waste recycling today. This process involves meticulously sorting mixed plastics into their respective chemical families, followed by shredding, washing, melt extrusion, and pelletization to yield recycled plastic pellets for further processing into various products. However, challenges arise when considering the recyclability of PHAs as they are prone to thermal degradation during processing, potentially limiting their suitability for mechanical recycling. For example, mechanical recycling studies for P3HB have reported a notable decrease in physical properties after two reprocessing cycles.74 Moreover, due to the brittleness and costliness of biologically synthesized P3HB, they are often blended with other polymers to enhance overall properties rather than being used in their pure form.75 Consequently, research on pure PHA recycling is limited. However, mechanical recycling studies on PHA blends, such as PHBV blends with PLA, suggest that PLA can stabilize the blends and mitigate thermal degradation of the PHA component.76 Nevertheless, the complexity of PHA blends poses challenges for downstream mechanical recycling, potentially complicating the recycling process as formulations become more intricate.
Chemical recycling of PHAs
Chemical recycling of polymers, in which monomers can be refurbished for re-polymerization back to virgin-quality polymer, is considered a closed-loop process. Despite PHA facile biodegradability in ambient environments, efficient chemical recycling that would enable the recirculation of biogenic carbon would likely be favored from an LCA perspective. Furthermore, conversion of PHAs back into microbial feedstocks can mitigate the 3–12× higher cost (compared to incumbent plastics) of producing biological PHAs, where feedstocks dominate the cost of manufacture.77 Most examples of PHA chemical recycling describe methods for monomer upgrading to higher-value products (referred to as “upcycling”) as preferential to re-polymerization through biological or chemical means. This is because under typical depolymerization conditions established for polyesters, PHAs can return oligomers or linear α,β-unsaturated acids.78,79 The incentives for re-polymerization are thus severely reduced by lack of depolymerization selectivity and subsequent separations and purification requisites.
With rapidly growing interest in chemical deconstruction or degradation of polyesters, specifically scalable solvolysis technologies, it is important to consider implications for PHA deconstruction. Glycolysis, hydrolysis, and methanolysis are all well-known ester degrading chemistries that are attracting significant investment to scale and commercialize for polyester recycling. Reported and predicted compounds from PHA feedstocks in common solvolysis processes are summarized in Fig. 5, though by no means exhaustive due to variations with PHA feedstock, reaction conditions, and deconstruction catalysis.79–82 Rather than focusing on recovering these compounds in high purities for chemo-catalytic polymerization back into PHAs, which is the process that has been widely adopted for fossil-based polyesters such as PET, research into using molecules from deconstruction as carbon sources for biological PHA synthesis should be explored to decrease PHA production costs and close the loop in its polymer lifecycle. In some cases, this may require additional chemical or biological conversions to produce microbially digestible feedstocks.83–86 As an example, deconstruction during high temperature methanolysis of PHBV promotes product mixtures including a significant fraction of unsaturated methyl crotonate (MC) and methyl 4-pentenoate (MP). MC and MP, however, can be readily hydrogenated over simple supported catalysts such as Pd/C to form their corresponding saturated methyl esters, methyl butyrate and methyl valerate, respectively. These compounds can then be fed to microbes, as it is well established that volatile fatty acids, and butyrate in particular, are ideal substrates for PHA production.87,88 To demonstrate the feasibility of doing so, we performed model hydrogenation reactions of both MC and MP as described in Fig. 6A, obtaining saturated analogues with quantitative conversion by gas chromatography–mass spectrometry (GC–MS) (Fig. 6B and C).
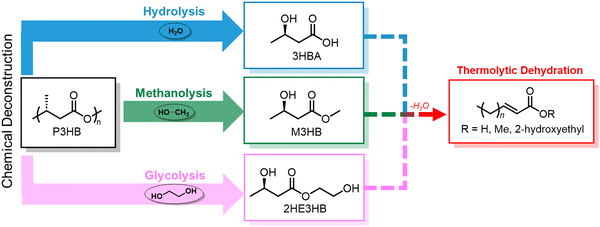 |
| Fig. 5 Solvolysis pathways for P3HB and anticipated products. Schematic for chemical deconstruction of model P3HB by hydrolysis (top, blue), methanolysis (middle, green), and glycolysis (bottom, pink) and corresponding expected products 3-hydroxybutyric acid (3HBA), methyl-3-hydroxybutyrate (M3HB), and 2-hexylethyl-3-hydroxybutyrate (2HE3HB), respectively. Also included are possible, reported unsaturated crotonate side-products generated by thermolytic dehydration (right, red) of the β-hydroxyl. | |
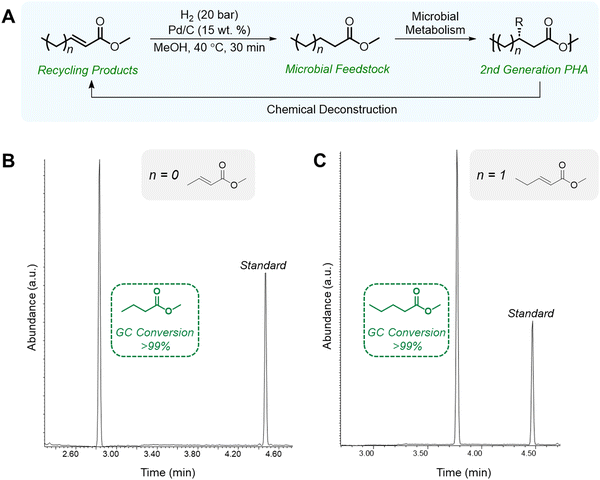 |
| Fig. 6 Tandem Chemical and Biological Sequence for Circular PHAs. (A) General schematic for funneling unsaturated products from PHA solvolysis to second-generation PHA materials via catalyzed hydrogenation and subsequent microbial metabolism. (B) GC–MS trace demonstrating the purity achievable for hydrogenation products from unsaturated methyl crotonate and (C) unsaturated methyl 4-pentenoate, along with an internal standard. GC–MS methods are as follows: 70 °C for 1 min followed by 20 °C min−1 ramp to 150 °C for 1 min, 1 μL injection, 250 °C inlet, 20 : 1 split, 3 mL min−1 flow rate (He), conducted on a J&W 122-1334 DB-624 column (30 μm × 250 μm ×1.4 μm). | |
The variety and heterogeneity of products that result from the chemical recycling of biologically produced PHAs has led to further motivation towards producing synthetic PHAs with altered and non-traditional structures aiming to maximize chemical recyclability and selectivity of depolymerization back to monomers. In a more promising direction, a recent advancement in chemical PHA synthesis has shown that by installing a geminal dimethyl group at the alpha-position of β-butyrolactone, the resulting P3HMe2B can be considered fully circular; it can be selectively depolymerized to either lactone or hydroxyacid monomer, which can then be re-polymerized through ROP or step-growth polycondensation, respectively.89 Nonetheless, the focus of P3HB EoL management has been pyrolytic degradation to selectively yield crotonic acid, considered a platform chemical. It can be used in coatings, paints, textiles, and adhesives, where the main industrial use is in co-polymers with vinyl acetate.90,91
Enzymatic recycling PHAs
Large scale biomass turnover is dependent on a wide range of microorganisms that have evolved to produce enzymes that penetrate and deconstruct polyesters.92 While some of these enzymes have long been known to cleave ester bonds in synthetic polyesters,93 the discovery of a bacterium with the remarkable ability to utilize PET as a sole carbon and energy source94 spawned multiple campaigns worldwide to apply advanced discovery and engineering approaches for improved enzymes for the conversion of waste polyesters to their respective monomers.95–101 In other microorganisms, PHAs are utilized directly as carbon and energy storage, and thus have a unique suite of enzymes for both their synthesis and depolymerization.102 Combined with the identification of multiple organisms that secrete PHA depolymerases, there is a large natural diversity of intracellular and extracellular enzymes available to characterize and adapt for industrial recycling processes.103,104 After composting, enzymatic hydrolysis is the most well studied downstream recycling process reported for PHA materials with hundreds of reported enzymes in the literature that can break down PHA polymers to monomer constituents.103,105
Several companies (e.g., Carbios, Samasara Eco, Protein Evolution Inc.) are engaged in the enzymatic deconstruction of waste PET packaging and textiles at industrial scale, with a 50
000 metric-ton per year facility currently under construction in France. While this scale has not yet been realized for PHAs, the potential for low energy, low waste, enzymatic process development remains an attractive option for the conversion of EoL PHAs back to monomers or microbial feedstocks. The drive for PHAs with material properties that match or exceed those of current polyolefins creates an opportunity to mitigate some of the current challenges for mixed-plastics recycling.16 The scale-up of technologies that can depolymerize and process a range of mixed polyesters will not only support the transition from fossil-based PET to recycled PET, but it will accelerate recycling technologies that can maintain compatibility towards new PHAs.
With respect to circularity, we note that engineered enzymes, when combined with chemical approaches, offer alternative routes towards a wide spectrum of monomer synthesis and bespoke modification for PHAs with enhanced material properties. Alternatives to fermentation processes could take advantage of advances in cell-free processes and scale-up, offering potential benefits from a technoeconomic perspective.106 While these developments further embolden an exciting materials engineering space, the impact of selective modifications on the biodegradative properties of new PHAs should be considered and tested carefully.11 Biological deconstruction of polymers, in both controlled and environmental scenarios, is intrinsically linked to the designed polymer properties.17 Thus, parallel research avenues that pair novel synthesis routes with EoL deconstruction solutions, ideally guided by integrated LCA, will hopefully help to avoid unforeseen negative environmental impacts in the future, and ensure true circularity.
Future outlooks
We see the development of both chemical (solvolysis, depolymerization) and biological (enzymatic) polyester recycling technologies as an opportunity complementing emerging chemo-catalytic and biological PHA production pathways. While composting and biodegradation are simple and preferred to conventional incineration and landfilling waste management, issues relating to capacity, compost health, and emissions may become prevalent with increasing volume of PHA production and subsequent post-consumer waste. We thus emphasize two major points in the future development of PHA materials: (1) a circular materials economy will require efforts in aligning current and future application-oriented PHAs with reclamation and recirculation principles as a top priority, and (2) avenues of PHA production must maintain a high degree of convergency when considering structural derivation for application-specific needs. When practiced, these principles both mitigate waste-stream complexity following recycling and promote the sequential waste management of recycling-first, composting-second, and environmental degradation-third. To these ends, we note practical (potentially linked) chemical and biological pathways for funneling recycling products to serve as feedstocks in next-life materials. ML prediction platforms and accompanied experimental feedback loops can play a significant role in the expedited discovery, design, and synthetic feasibility of functional and circular PHA-based materials, but more work is necessary to account for key structural factors such as stereomicrostructures. Overall, global efforts amassed on computational, experimental, and waste management fronts indeed support PHA adoption to the commercial landscape and must be united to translate these materials to scalable recycling infrastructures. It is our hope that this perspective will aid in coupling green chemistry principles between polymer and waste-stream as the field continues to innovate.
Data availability
All primary research results and data are presented in this manuscript. No software or code have been included as part of this perspective.
Conflicts of interest
The authors declare no competing interests.
Acknowledgements
Funding was provided by the U.S. Department of Energy, Office of Energy Efficiency and Renewable Energy, Advanced Materials and Manufacturing Technologies Office (AMMTO), and Bioenergy Technologies Office (BETO). This work was performed as part of the Bio-Optimized Technologies to keep Thermoplastics out of Landfills and the Environment (BOTTLE) Consortium and was supported by AMMTO and BETO under contract DEAC36-08GO28308 with the National Renewable Energy Laboratory (NREL), operated by Alliance for Sustainable Energy, LLC. The views expressed in the article do not necessarily represent the views of the DOE or the U.S. Government. The U.S. Government retains and the publisher, by accepting the article for publication, acknowledges that the U.S. Government retains a nonexclusive, paid-up, irrevocable, worldwide license to publish or reproduce the published form of this work, or allow others to do so, for U.S. Government purposes. The authors thank K. M. Shebek (Northwestern University), M. R. Nimlos (NREL), and J. N. Law (NREL) for their helpful discussions and insight on the machine learning aspect of this perspective. The authors also thank Scivetica/Rita Clare for graphics support.
References
- E. Y. X. Chen, Nat. Sustainability, 2023, 6, 1140–1141 CrossRef.
- W. E. Forum, E. M. Foundation and M. Company, The New Plastics Economy: Rethinking the future of plastics, 2016 Search PubMed.
- R. Meys, A. Kätelhön, M. Bachmann, B. Winter, C. Zibunas, S. Suh and A. Bardow, Science, 2021, 374, 71–76 CrossRef CAS PubMed.
- F. Vidal, E. R. van der Marel, R. W. F. Kerr, C. McElroy, N. Schroeder, C. Mitchell, G. Rosetto, T. T. D. Chen, R. M. Bailey, C. Hepburn, C. Redgwell and C. K. Williams, Nature, 2024, 626, 45–57 CrossRef CAS PubMed.
- F. M. de Souza and R. K. Gupta, ACS Omega, 2024, 9, 8666–8686 CrossRef CAS PubMed.
- D. Vicente, D. N. Proença and P. V. Morais, Int. J. Environ. Res. Public Health, 2023, 20, 2959 CrossRef CAS PubMed.
- S. A. Acharjee, P. Bharali, B. Gogoi, V. Sorhie, B. Walling and Alemtoshi, Water, Air, Soil Pollut., 2022, 234, 21 CrossRef PubMed.
- M. S. Kim, H. Chang, L. Zheng, Q. Yan, B. F. Pfleger, J. Klier, K. Nelson, E. L. W. Majumder and G. W. Huber, Chem. Rev., 2023, 123, 9915–9939 CrossRef CAS PubMed.
- C. Gioia, G. Giacobazzi, M. Vannini, G. Totaro, L. Sisti, M. Colonna, P. Marchese and A. Celli, ChemSusChem, 2021, 14, 4167–4175 CrossRef CAS PubMed.
- A. Chamas, H. Moon, J. Zheng, Y. Qiu, T. Tabassum, J. H. Jang, M. Abu-Omar, S. L. Scott and S. Suh, ACS Sustainable Chem. Eng., 2020, 8, 3494–3511 CrossRef CAS.
- T. Narancic, S. Verstichel, S. Reddy Chaganti, L. Morales-Gamez, S. T. Kenny, B. De Wilde, R. Babu Padamati and K. E. O’Connor, Environ. Sci. Tech., 2018, 52, 10441–10452 CrossRef CAS PubMed.
- N. Follain, C. Chappey, E. Dargent, F. Chivrac, R. Crétois and S. Marais, J. Phys. Chem. C, 2014, 118, 6165–6177 CrossRef CAS.
- H. de Beukelaer, M. Hilhorst, Y. Workala, E. Maaskant and W. Post, Polym. Test., 2022, 116, 107803 CrossRef CAS.
- A. Sangroniz, J.-B. Zhu, X. Tang, A. Etxeberria, E. Y. X. Chen and H. Sardon, Nat. Commun., 2019, 10, 3559 CrossRef PubMed.
- X. Tang, A. H. Westlie, L. Caporaso, L. Cavallo, L. Falivene and E. Y.-X. Chen, Angew. Chem., Int. Ed., 2020, 59, 7881–7890 CrossRef CAS PubMed.
- E. C. Quinn, K. M. Knauer, G. T. Beckham and E. Y. X. Chen, One Earth, 2023, 6, 582–586 CrossRef.
- K. W. Meereboer, M. Misra and A. K. Mohanty, Green Chem., 2020, 22, 5519–5558 RSC.
- C. Shi, E. C. Quinn, W. T. Diment and E. Y. X. Chen, Chem. Rev., 2024, 124, 4393–4478 CrossRef CAS PubMed.
- J. Walzberg, S. Sethuraman, T. Ghosh, T. Uekert and A. Carpenter, Energy Res. Soc. Sci., 2023, 100, 103116 CrossRef.
- M. Lemoigne, Bull. Soc. Chim. Biol., 1926, 8, 770–782 CAS.
- L. L. Wallen and W. K. Rohwedder, J. Environ. Sci. Technol., 1974, 8, 576–579 CrossRef CAS.
- R. H. Findlay and D. C. White, Appl. Environ. Microbiol., 1983, 45, 71–78 CrossRef CAS PubMed.
- Z. Li, J. Yang and X. J. Loh, NPG Asia Mater., 2016, 8, e265 CrossRef CAS.
- C. Kourmentza, J. Plácido, N. Venetsaneas, A. Burniol-Figols, C. Varrone, H. N. Gavala and M. A. M. Reis, Bioengineering, 2017, 4, 55 CrossRef PubMed.
- J.-i Choi and S. Y. Lee, Bioprocess Eng., 1997, 17, 335–342 CrossRef CAS.
- H. Salehizadeh and M. C. M. Van Loosdrecht, Biotechnol. Adv., 2004, 22, 261–279 CrossRef CAS PubMed.
- J. Mozejko-Ciesielska, K. Moraczewski, S. Czaplicki and V. Singh, Sci. Rep., 2023, 13, 22289 CrossRef CAS PubMed.
- S. Guleria, H. Singh, V. Sharma, N. Bhardwaj, S. K. Arya, S. Puri and M. Khatri, J. Cleaner Prod., 2022, 340, 130661 CrossRef CAS.
- L. S. Serafim, P. C. Lemos, M. G. E. Albuquerque and M. A. M. Reis, Appl. Microbiol. Biotechnol., 2008, 81, 615–628 CrossRef CAS PubMed.
- S. Chavan, B. Yadav, R. D. Tyagi and P. Drogui, Bioresour. Technol., 2021, 341, 125900 CrossRef CAS PubMed.
- H. Pakalapati, C.-K. Chang, P. L. Show, S. K. Arumugasamy and J. C.-W. Lan, J. Biosci. Bioeng., 2018, 126, 282–292 CrossRef CAS PubMed.
- Q. Chen, Q. Wang, G. Wei, Q. Liang and Q. Qi, Appl. Environ. Microbiol., 2011, 77, 4886–4893 CrossRef CAS PubMed.
- S. Sato, H. Maruyama, T. Fujiki and K. Matsumoto, J. Biosci. Bioeng., 2015, 120, 246–251 CrossRef CAS PubMed.
- G.-Q. Chen, Chem. Soc. Rev., 2009, 38, 2434–2446 RSC.
- R. M. Cywar, N. A. Rorrer, C. B. Hoyt, G. T. Beckham and E. Y. X. Chen, Nat. Rev. Mater., 2022, 7, 83–103 CrossRef CAS.
- M. Winnacker, Eur. J. Lipid Sci. Technol., 2019, 121, 1900101 CrossRef CAS.
- T. Tsuge, Polym. J., 2016, 48, 1051–1057 CrossRef CAS.
- X. Tang, A. H. Westlie, E. M. Watson and E. Y.-X. Chen, Science, 2019, 366, 754–758 CrossRef CAS PubMed.
- A. H. Westlie, E. C. Quinn, C. R. Parker and E. Y. X. Chen, Prog. Polym. Sci., 2022, 134, 101608 CrossRef CAS.
- H.-Y. Huang, W. Xiong, Y.-T. Huang, K. Li, Z. Cai and J.-B. Zhu, Nat. Catal., 2023, 6, 720–728 CrossRef CAS.
- A. H. Westlie and E. Y. X. Chen, Macromolecules, 2020, 53, 9906–9915 CrossRef CAS.
- Z. Zhou, A. M. LaPointe, T. D. Shaffer and G. W. Coates, Nat. Chem., 2023, 15, 856–861 CrossRef CAS PubMed.
- F. D. Kopinke, M. Remmler and K. Mackenzie, Polym. Degrad. Stab., 1996, 52, 25–38 CrossRef CAS.
- C. Kim, A. Chandrasekaran, T. D. Huan, D. Das and R. Ramprasad, J. Phys. Chem. C, 2018, 122, 17575–17585 CrossRef CAS.
- E. R. Antoniuk, P. Li, B. Kailkhura and A. M. Hiszpanski, J. Chem. Inf. Model., 2022, 62, 5435–5445 CrossRef CAS PubMed.
- G. Bradford, J. Lopez, J. Ruza, M. A. Stolberg, R. Osterude, J. A. Johnson, R. Gomez-Bombarelli and Y. Shao-Horn, ACS Cent. Sci., 2023, 9, 206–216 CrossRef CAS PubMed.
- A. N. Wilson, P. C. St John, D. H. Marin, C. B. Hoyt, E. G. Rognerud, M. R. Nimlos, R. M. Cywar, N. A. Rorrer, K. M. Shebek, L. J. Broadbelt, G. T. Beckham and M. F. Crowley, Macromolecules, 2023, 56, 8547–8557 CrossRef CAS PubMed.
- J. C. Worch, H. Prydderch, S. Jimaja, P. Bexis, M. L. Becker and A. P. Dove, Nat. Rev. Chem., 2019, 3, 514–535 CrossRef CAS.
- C. S. Biswas, K. Mitra, S. Singh, K. Ramesh, N. Misra, B. Maiti, A. K. Panda, P. Maiti, M. Kamigaito, Y. Okamoto and B. Ray, Colloid Polym. Sci., 2015, 293, 1749–1757 CrossRef CAS.
- D. J. A. Cameron and M. P. Shaver, J. Polym. Sci., Part A: Polym. Chem., 2012, 50, 1477–1484 CrossRef CAS.
- K. K. Bejagam, J. Lalonde, C. N. Iverson, B. L. Marrone and G. Pilania, J. Phys. Chem. B, 2022, 126, 934–945 CrossRef CAS PubMed.
- G. Pilania, C. N. Iverson, T. Lookman and B. L. Marrone, J. Chem. Inf. Model., 2019, 59, 5013–5025 CrossRef CAS PubMed.
- A. Boublia, T. Lemaoui, J. AlYammahi, A. S. Darwish, A. Ahmad, M. Alam, F. Banat, Y. Benguerba and I. M. AlNashef, ACS Sustainable Chem. Eng., 2023, 11, 208–227 CrossRef CAS.
- T.-S. Lin, C. W. Coley, H. Mochigase, H. K. Beech, W. Wang, Z. Wang, E. Woods, S. L. Craig, J. A. Johnson, J. A. Kalow, K. F. Jensen and B. D. Olsen, ACS Cent. Sci., 2019, 5, 1523–1531 CrossRef CAS PubMed.
- Y. Hayashi, J. Shiomi, J. Morikawa and R. Yoshida, npj Comput. Mater., 2022, 8, 222 CrossRef CAS.
- A. J. Gormley and M. A. Webb, Nat. Rev. Mater., 2021, 6, 642–644 CrossRef CAS PubMed.
- K. Min, J. D. Cuiffi and R. T. Mathers, Nat. Commun., 2020, 11, 727 CrossRef CAS PubMed.
- H. Yamada, C. Liu, S. Wu, Y. Koyama, S. Ju, J. Shiomi, J. Morikawa and R. Yoshida, ACS Cent. Sci., 2019, 5, 1717–1730 CrossRef CAS PubMed.
- G. Liu and M. Jiang, Presented in part at the ICLR, 2023.
- C.-K. Lee, C. Lu, Y. Yu, Q. Sun, C.-Y. Hsieh, S. Zhang, Q. Liu and L. Shi, J. Chem. Phys., 2021, 154, 024906 CrossRef CAS PubMed.
- M. R. Yates and C. Y. Barlow, Resour., Conserv. Recycl., 2013, 78, 54–66 CrossRef.
- M. Saavedra del Oso, R. Nair, M. Mauricio-Iglesias and A. Hospido, Resour., Conserv. Recycl., 2023, 199, 107242 CrossRef.
- M. Koch, S. Spierling, V. Venkatachalam, H.-J. Endres, M. Owsianiak, E. B. Vea, C. Daffert, M. Neureiter and I. Fritz, Sci. Total Environ., 2023, 863, 160991 CrossRef CAS PubMed.
- I. D. Posen, P. Jaramillo and W. M. Griffin, Environ. Sci. Tech., 2016, 50, 2846–2858 CrossRef CAS PubMed.
- G. Meral, Global Plastics Outlook, OECD, 2022.
- EPA, Composting in WARM, (https://19january2017snapshot.epa.gov/sites/production/files/2016-03/documents/cmpstng_ovrview.pdf).
- S. Kaza, L. C. Yao, P. Bhada Tata, F. Van Woerden, T. M. R. Martin, K. R. B. Serrona, R. Thakur, F. Pop, S. Hayashi, G. Solorzano, N. S. Alencastro Larios, R. A. Poveda and A. Ismail, What a Waste 2.0: A Global Snapshot of Solid Waste Management to 2050 (English), Urban Development Series, Washington, D.C., World Bank Group Search PubMed.
- E. Corella-Puertas, C. Hajjar, J. Lavoie and A.-M. Boulay, J. Cleaner Prod., 2023, 418, 138197 CrossRef CAS.
- K. L. Law, N. Starr, T. R. Siegler, J. R. Jambeck, N. J. Mallos and G. H. Leonard, Sci. Adv., 2020, 6, eabd0288 CrossRef PubMed.
- R. Geyer, J. R. Jambeck and K. L. Law, Sci. Adv., 2017, 3, e1700782 CrossRef PubMed.
- A. Milbrandt, K. Coney, A. Badgett and G. T. Beckham, Resour., Conserv. Recycl., 2022, 183, 106363 CrossRef.
- S. Wang, K. A. Lydon, E. M. White, J. B. Grubbs, III, E. K. Lipp, J. Locklin and J. R. Jambeck, Environ. Sci. Technol., 2018, 52, 5700–5709 CrossRef CAS PubMed.
- J. D. Maasakkers, D. J. Varon, A. Elfarsdóttir, J. McKeever, D. Jervis, G. Mahapatra, S. Pandey, A. Lorente, T. Borsdorff, L. R. Foorthuis, B. J. Schuit, P. Tol, T. A. van Kempen, R. van Hees and I. Aben, Sci. Adv., 2022, 8, eabn9683 CrossRef CAS PubMed.
- L. F. Rivas, S. A. Casarin, N. C. Nepomuceno, M. I. Alencar, J. A. M. Agnelli, E. S. D. Medeiros, A. D. O. Wanderley, M. P. D. Oliveira, A. M. D. Medeiros and A. S. F. E. Santos, Polimeros, 2017, 27, 122–128 CrossRef.
- A. Soroudi and I. Jakubowicz, Eur. Polym. J., 2013, 49, 2839–2858 CrossRef CAS.
- I. Zembouai, S. Bruzaud, M. Kaci, A. Benhamida, Y.-M. Corre and Y. Grohens, J. Polym. Environ., 2014, 22, 449–459 CrossRef CAS.
- C. Tang and C. Y. Ryu, Sustainable polymers from biomass, John Wiley & Sons, 2017 Search PubMed.
- H. Ariffin, H. Nishida, M. A. Hassan and Y. Shirai, Biotechnol. J., 2010, 5, 484–492 CrossRef CAS PubMed.
- A. Dhaini, V. Hardouin-Duparc, A. Alaaeddine, J.-F. Carpentier and S. M. Guillaume, Prog. Polym. Sci., 2024, 149, 101781 CrossRef CAS.
- J. Yu, D. Plackett and L. X. L. Chen, Polym. Degrad. Stab., 2005, 89, 289–299 CrossRef CAS.
- X. Song, F. Liu, H. Wang, C. Wang, S. Yu and S. Liu, Polym. Degrad. Stab., 2018, 147, 215–221 CrossRef CAS.
- X. Song, H. Wang, C. Wang, F. Liu, S. Yu, S. Liu and Z. Song, J. Polym. Environ., 2019, 27, 862–870 CrossRef CAS.
- S. Leow, A. J. Koehler, L. E. Cronmiller, X. Huo, G. D. Lahti, Y. Li, G. R. Hafenstine, D. R. Vardon and T. J. Strathmann, Catal. Sci. Technol., 2021, 11, 6866–6876 RSC.
- K. P. Sullivan, A. Z. Werner, K. J. Ramirez, L. D. Ellis, J. R. Bussard, B. A. Black, D. G. Brandner, F. Bratti, B. L. Buss, X. Dong, S. J. Haugen, M. A. Ingraham, M. O. Konev, W. E. Michener, J. Miscall, I. Pardo, S. P. Woodworth, A. M. Guss, Y. Román-Leshkov, S. S. Stahl and G. T. Beckham, Science, 2022, 378, 207–211 CrossRef CAS PubMed.
- W. Partenheimer, Catal. Today, 2003, 81, 117–135 CrossRef CAS.
- E. Bäckström, K. Odelius and M. Hakkarainen, Ind. Eng. Chem. Res., 2017, 56, 14814–14821 CrossRef.
- L. Marang, Y. Jiang, M. C. M. van Loosdrecht and R. Kleerebezem, Bioresour. Technol., 2013, 142, 232–239 CrossRef CAS PubMed.
- C. M. Vermeer, L. J. Bons and R. Kleerebezem, Appl. Microbiol. Biotechnol., 2022, 106, 605–618 CrossRef CAS PubMed.
- L. Zhou, Z. Zhang, C. Shi, M. Scoti, D. K. Barange, R. R. Gowda and E. Y.-X. Chen, Science, 2023, 380, 64–69 CrossRef CAS PubMed.
- J. Blumenstein, J. Albert, R. P. Schulz and C. Kohlpaintner, Ullmann’s Encycl. Ind. Chem., 2000, 1–20 Search PubMed.
- V. Elhami, M. A. Hempenius and B. Schuur, Ind. Eng. Chem. Res., 2023, 62, 916–923 CrossRef CAS.
- C.-C. Chen, L. Dai, L. Ma and R.-T. Guo, Nat. Rev. Chem., 2020, 4, 114–126 CrossRef PubMed.
- R.-J. Müller, H. Schrader, J. Profe, K. Dresler and W.-D. Deckwer, Macromol. Rapid Commun., 2005, 26, 1400–1405 CrossRef.
- S. Yoshida, K. Hiraga, T. Takehana, I. Taniguchi, H. Yamaji, Y. Maeda, K. Toyohara, K. Miyamoto, Y. Kimura and K. Oda, Science, 2016, 351, 1196–1199 CrossRef CAS PubMed.
- A. Carniel, V. d A. Waldow and A. M. d Castro, Biotechnol. Adv., 2021, 52, 107811 CrossRef CAS PubMed.
- V. Tournier, C. M. Topham, A. Gilles, B. David, C. Folgoas, E. Moya-Leclair, E. Kamionka, M. L. Desrousseaux, H. Texier, S. Gavalda, M. Cot, E. Guémard, M. Dalibey, J. Nomme, G. Cioci, S. Barbe, M. Chateau, I. André, S. Duquesne and A. Marty, Nature, 2020, 580, 216–219 CrossRef CAS PubMed.
- Å. M. Ronkvist, W. Xie, W. Lu and R. A. Gross, Macromolecules, 2009, 42, 5128–5138 CrossRef.
- Y. Cui, Y. Chen, X. Liu, S. Dong, Y. E. Tian, Y. Qiao, R. Mitra, J. Han, C. Li, X. Han, W. Liu, Q. Chen, W. Wei, X. Wang, W. Du, S. Tang, H. Xiang, H. Liu, Y. Liang, K. N. Houk and B. Wu, ACS Catal., 2021, 11, 1340–1350 CrossRef CAS.
- B. C. Knott, E. Erickson, M. D. Allen, J. E. Gado, R. Graham, F. L. Kearns, I. Pardo, E. Topuzlu, J. J. Anderson, H. P. Austin, G. Dominick, C. W. Johnson, N. A. Rorrer, C. J. Szostkiewicz, V. Copié, C. M. Payne, H. L. Woodcock, B. S. Donohoe, G. T. Beckham and J. E. McGeehan, Proc. Natl. Acad. Sci. U. S. A., 2020, 117, 25476–25485 CrossRef CAS PubMed.
- H. Lu, D. J. Diaz, N. J. Czarnecki, C. Zhu, W. Kim, R. Shroff, D. J. Acosta, B. R. Alexander, H. O. Cole, Y. Zhang, N. A. Lynd, A. D. Ellington and H. S. Alper, Nature, 2022, 604, 662–667 CrossRef CAS PubMed.
- R. Wei, D. Breite, C. Song, D. Gräsing, T. Ploss, P. Hille, R. Schwerdtfeger, J. Matysik, A. Schulze and W. Zimmermann, Adv. Sci., 2019, 6, 1900491 CrossRef PubMed.
- W. Zhou, S. Bergsma, D. I. Colpa, G.-J. W. Euverink and J. Krooneman, J. Environ. Manage., 2023, 341, 118033 CrossRef CAS PubMed.
- M. Knoll, T. M. Hamm, F. Wagner, V. Martinez and J. Pleiss, BMC Bioinf., 2009, 10, 89 CrossRef PubMed.
- K. Numata, H. Abe and Y. Doi, Can. J. Chem., 2008, 86, 471–483 CrossRef CAS.
- V. R. Viljakainen and L. A. Hug, Environ. Microbiol., 2021, 23, 1717–1731 CrossRef CAS PubMed.
- H. Dong, X. Yang, J. Shi, C. Xiao and Y. Zhang, Polymers, 2023, 15, 2333 CrossRef CAS PubMed.
|
This journal is © The Royal Society of Chemistry 2024 |