DOI:
10.1039/D1SM00047K
(Review Article)
Soft Matter, 2021,
17, 5850-5863
Dynamics in supramolecular nanomaterials
Received
11th January 2021
, Accepted 1st June 2021
First published on 2nd June 2021
Abstract
Self-assembly of amphiphilic small molecules in water leads to nanostructures with customizable structure–property relationships arising from their tunable chemistries. Characterization of these assemblies is generally limited to their static structures – e.g. their geometries and dimensions – but the implementation of tools that provide a deeper understanding of molecular motions has recently emerged. Here, we summarize recent reports showcasing dynamics characterization tools and their application to small molecule assemblies, and we go on to highlight supramolecular systems whose properties are substantially affected by their conformational, exchange, and water dynamics. This review illustrates the importance of considering dynamics in rational amphiphile design.
1. Introduction
Spontaneous self-assembly of amphiphiles occurs when orthogonal interactions with solvent dominate different regions of the same molecule. A typical example of self-assembly in Nature, Fig. 1, illustrates a phospholipid cell membrane in an aqueous environment with embedded transmembrane and surface proteins. In contrast to this static snapshot captured in an image, active molecular components in the cell membrane (e.g., phospholipids, proteins, water, and cholesterol) are in constant motion.1 These intrinsic dynamics mediate all intermolecular interactions, chemical reactions, and physiological responses of the membrane. The importance of dynamics is well elucidated by examining the many components of phospholipid membranes in nature. Eukaryotic membranes exhibit relatively fast dynamics that enable their rapid division, enzyme catalysis, and allosteric regulation,2–4 while the dynamics of archaeal membranes are slower, accounting for their temperature resistance, mechanical stability, and salt tolerance.5–7 From these relationships, it follows that dynamics also play an important role in synthetic supramolecular nanostructures, defined here as nanostructures constructed from amphiphilic small molecules and bound together by noncovalent intermolecular interaction. Similar to natural systems, precise tuning of dynamics within synthetic structures is an important target for achieving control over their properties.
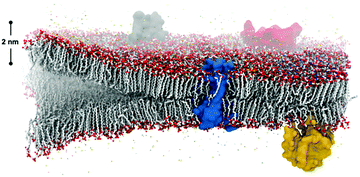 |
| Fig. 1 The co-assembly of biological components in cell membranes gives rise to bilayers with complex functionality and adaptive, controlled dynamics that dictate their function. Image credit: Thomas Lemmin, Laboratory for Molecular Modeling, École Polytechnique Fédérale de Lausanne. | |
Synthetic self-assembling molecules are modeled after the molecular architecture of their biological counterparts. The field of molecular self-assembly has grown dramatically over the past 30 years, as evidenced by the development of theory to predict nanostructure morphologies from the packing parameters of constituent molecules8 and the synthesis of a vast array of new, functional supramolecular nanomaterials.9–11 In addition to the fundamental amphiphilic structure inspired by nature, more and more synthetic self-assembling molecules incorporate strong intermolecular cohesion (e.g., hydrogen bonding,12 π–π stacking,13 charge-transfer interactions14) and stimuli-responsive moieties (e.g., photo-,15 pH-16 and enzyme-responsive17 functionalities). A significant benefit of this bottom-up design strategy is the formation of structures at molecular (<10 nm) length scales with remarkably precise spatial arrangement. Formation of nanoscale supramolecular architectures naturally yield high accessible surface areas, and the surface properties of the structure can be easily tuned by modifying the chemistry of the constituent amphiphiles. Furthermore, other phenomena found in nature like multicomponent co-assembly18,19 and in situ surface functionalization20,21 are implemented in synthetic self-assembly to promote specific intermolecular events. Overall, these advantages of molecular self-assembling materials inspired numerous studies for potential applications during the last 10 years, ranging from biomimetic (e.g., tissue engineering,22 gene and drug delivery,23,24 and as antimicrobial agents25) to optoelectronic (e.g. photoluminescent materials,26 bioimaging,27 biosensing,28 and photovoltaic applications29) to energy-related (e.g., supercapacitance30 and photocatalysis31). Although the historical progress of the field and case studies exceed the scope of this review, we refer readers to a number of comprehensive and specific reviews for further interests.9–11,32–37
Reports describing structure–property relationships of synthetic self-assembling systems are common, however those describing characterization strategies that provide an understanding of dynamics are less prevalent. Static structures of self-assemblies are characterized typically by X-ray or neutron scattering,38–40 circular dichroism,41 transmission electron microscope (TEM),42 and more recently in situ imaging by cryogenic TEM (cryo-TEM).43 While these techniques provide valuable information about the average structure, they do not capture dynamic properties of supramolecular structures.44 Nevertheless, a growing body of literature suggests that dynamics significantly impact the characteristics of amphiphilic assemblies, just as they do in natural self-assembled systems.37,44,45 Therefore, advancing the understanding of dynamics in molecular self-assembly is an important goal that will benefit the next generation of small molecule amphiphiles.
In this review, we first introduce studies that adopt dynamics characterization tools from biochemistry. Based on these studies, we categorize the three types of dynamics as follows: (1) conformational dynamics, i.e. the “jiggling” of molecules; (2) molecular exchange and migration; and (3) hydration water dynamics. Then, we will highlight reports that demonstrate the significance of dynamics in supramolecular design. Computational approaches are critical in driving fundamental understanding of molecular self-assembly in water, especially through all-atom molecular dynamics (MD) simulations that implement increasingly sophisticated force fields.46–49 However the literature describing computational studies will be largely excluded from this review. Similarly, self-assembly of covalent polymers and organic solvent-based liquid crystals fall outside the scope of this review.
2. Experimental tools used to investigate dynamics in supramolecular systems
Intermolecular and intramolecular motions have been experimentally observed among native-state biological macromolecules and superstructures since the 1970s.50,51 Such processes as protein folding/unfolding, ligand binding, cell signaling/regulation and other non-equilibrium events are now understood to be inextricably tied to conformational, exchange, and water dynamics in biological systems.52–54 In addition to computational approaches, various experimental tools have been developed to quantify dynamics over a wide range of time and length scales. Interest in the dynamics of synthetic supramolecular systems has led to the adoption of many biochemical characterization techniques. In this section, we introduce recent studies that experimentally evaluate conformational, exchange, or water dynamics in supramolecular systems using emerging tools.
2.1 Conformational dynamics in supramolecular systems
Conformational dynamics is defined as the time-dependent fluctuation of molecular conformations. This molecular motion is important, but has historically been challenging to measure in supramolecular systems. In contrast, the relationship between conformational dynamics and properties is extensively studied in biochemistry, and its importance is widely accepted.3,4,55,56 A few experimental tools are routinely used in biochemistry including nuclear magnetic resonance (NMR),57,58 vibrational spectroscopy,59 and neutron scattering.60 Adapting these tools to study supramolecular systems directly is challenging due to either the discrepancies in motional time scales or signal sensitivity. Electron paramagnetic resonance (EPR), enabled by site-directed spin labeling (SDSL), has received attention as a suitable technique that overcomes these limitations.61 EPR spectroscopy is highly sensitive, requiring only small concentrations of spin label, and provides a precise measure of local dynamics via the rotational diffusion rate (typically on the order of 106–109 Hz) of the radical nitroxyl spin label.62,63 While the synthesis of stable spin-labeled molecules and technical data analysis previously precluded the implementation of EPR in some cases, recent developments in synthesis64–66 and software67,68 have made EPR more accessible for investigating conformational dynamics in supramolecular systems.
EPR has been used for measuring conformational dynamics on a self-assembled nanofibers composed of peptide amphiphiles (PAs). Ortony et al. positioned radical electron (nitroxyl) moieties into the molecular structure by SDSL, then quantified dynamics by EPR lineshape analysis.69 The PA in this study is composed of three domains: a hydrophobic alkyl tail, a peptide sequence with a high propensity for β-sheet formation, and a charged peptide head group. The driving force for PA self-assembly is the hydrophobic effect, and the formation of β-sheets down the nanofiber long-axis promotes self-assembly into high-aspect-ratio nanofibers (Fig. 2b). While PA 1 with six peptide residues (VVAAEE) was known to form cohesive β-sheet nanofibers, mixtures of PA 1 and PA 2, with an N-methylated valine (VN(me)VAAEE), yielded PA 1/2 nanofibers with weakened β-sheets due to the disruption of hydrogen bonding. EPR spectroscopy for conformational dynamics analysis was performed on both PA 1 and PA 1/2 nanofibers, each co-assembled with spin-labeled PAs, in this study. By placing the spin label at different depths in the nanofiber (Fig. 2a), EPR revealed site-specific dynamics. EPR not only provided qualitative understanding of conformational motion based on the breadth of spectra, but the spectra were also fit to quantify the dynamics parameter, the rotational diffusion rate (kr). Fig. 2c shows that rotational diffusion rates are variable through the cross-section of nanofibers, where both surface and core spin labels exhibit liquid-like (fast) dynamics while β-sheet spin labels move more slowly and are more solid-like. The comparison of dynamics between PA 1 and PA 1/2 nanofibers indicated that dynamics are slowest at the β-sheet region when hydrogen bonding propensity is greatest, as shown in Fig. 2d. This study demonstrates the utility of EPR to evaluate conformational dynamics, and therefore local state of matter, in supramolecular structures quantitatively and precisely, and this tool is foreseen to further contribute to dynamics understanding and design of new supramolecular nanomaterials.
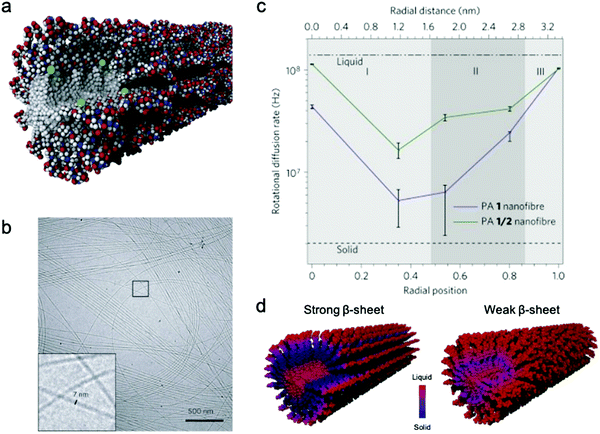 |
| Fig. 2 (a) Representative scheme of a co-assembled supramolecular nanofiber with PA 1 and PA 1c for EPR, where green balls represent spin labels nested in the nanostructure to capture localized conformational dynamics. (b) Cryo-TEM micrograph of PA 1 nanofibers in water. (c) Rotational diffusion rates (kr) extracted from EPR spectral simulations. Horizontal axis shows the distance from nanofiber core to surface. (d) Nanofiber composed of PA 1 (strong β-sheet) and PA 1/2 (weak β-sheet). The comparison of the dynamics between PA 1 and PA 1/2 nanofibers indicates that dynamics are slowest at the β-sheet region when hydrogen bonding propensity is greatest. Adapted from ref. 69, Copyright 2014 Springer Nature. | |
2.2 Exchange dynamics in supramolecular systems
Molecular exchange, migration, rearrangements, and insertions are often encompassed within “membrane dynamics” of biological structures. These motions are important to biological function, and are highly evolved and specialized in natural systems. For example, the introduction of co-assembled species (e.g. cholesterol, fatty acids) mediate membrane dynamics to modify rigidity and deformation, control permeability, and regulate different cellular processes such as neurotransmission.70–73 Similarly, synthetic assemblies exhibit exchange dynamics, defined here as the exchange of amphiphiles in or between self-assembled structures or the solvent environment. In recent reports, a relationship between molecular design, exchange dynamics, and nanostructure is described.74,75 We propose that controlling dynamic exchange in synthetic assemblies will give rise to a new generation of specialized nanostructures, analogous to natural systems. Molecular mixing experiments including Förster resonance energy transfer (FRET)76–78 and hydrogen–deuterium exchange mass spectrometry (HDX-MS)79–81 are routinely used in biochemistry as tools to investigate exchange dynamics, and have recently been adapted for supramolecular nanostructures. In this subsection, we discuss recently reported methodologies for investigating the exchange dynamics in supramolecular nanostructures.
Hydrogen–deuterium exchange mass spectroscopy (HDX-MS).
Lou et al. recently demonstrated the application of HDX-MS for characterizing the nanoscale organization and exchange dynamics of self-assembled supramolecular fibrils in water. By using HDX-MS, these studies were conducted without the use of molecular probes that could perturb the structure.82 Benzene-1,3,5-tricarboxamide (C12BTA) molecules, with three hydroxyl hydrogens at tetra(ethylene glycol) peripheries and three amide hydrogens at the core, were studied in this research (Fig. 3a). C12BTA self-assembles in water via a combination of hydrophobic interactions and directional hydrogen bond formation into long, supramolecular nanofibers. Only two major isotopes corresponding to C12BTA3D and C12BTA6D were observed during HDX. C12BTA3D corresponds to the substitution of three outer hydroxyl groups on C12BTA, and C12BTA6D corresponds to the substitution of three outer hydroxyl and three inner amide groups on C12BTA, resulting from the slower exchange of amide than hydroxyl hydrogens. Time-resolved HDX revealed that the hydrogens of the hydroxyl groups are instantaneously replaced by deuterium during exchange, whereas the transition from C12BTA3D to C12BTA6D presents a kinetic profile as shown in Fig. 3b. This profile was fit to a tri-exponential model with three dissimilar rate constants kinitial = 1.8 × 101 h−1, kfast = 1.4 × 10−1 h−1, and kslow = 0.7 × 10−2 h−1, suggesting that the system exhibits exchange dynamics with different orders of magnitude. Further, the exchange dynamics between BTAs with undecyl and dodecyl alkyl spacers were distinguishable by HDX-MS, but not by FRET83 – all BTA molecules in the system can be probed by HDX-MS, whereas only a subset of dye-labelled molecules are detectable by FRET. HDX-MS has been used to characterize other amphiphile systems,84,85 further demonstrating its value as a technique for probing exchange dynamics in water.
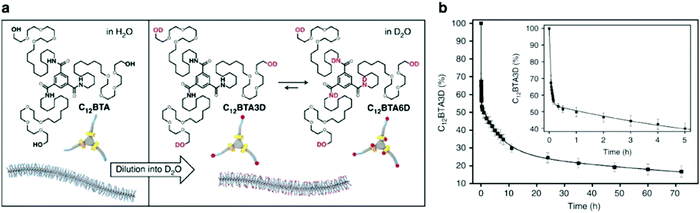 |
| Fig. 3 (a) Chemical structures of C12BTA and its deuterated analogues C12BTA3D and C12BTA6D, schematic representation of the supramolecular polymers formed in water and an illustration of the H/D exchange process. (b) Kinetic profile illustrates hydrogen to deuterium exchange rate of C12BTA3D to C12BTA6D, fit to a triexponential decay. The inset shows an enlargement of the data points and fit for the first 5 h. Adapted from ref. 82, Copyright 2017 Springer Nature. | |
HDX-MS also enables the tracking of exchange of different types of monomers in multicomponent systems.86,87 This capability is achieved by employing mass spectrometry to distinguish between molecular masses. Notably, sample preparation of HDX-MS for self-assembled supramolecular systems is relatively simple and straightforward; HDX-MS in its historical use for protein science relies on protein decomposition to peptide monomers, which are then separated.88 Peptide monomer products obtained by protein decomposition have a propensity to form higher-order structure which may complicate data interpretation. In contrast to peptides, small molecule assemblies investigated well above their critical aggregation concentrations have fewer monomers in solution and the free monomers undergo hydrogen–deuterium exchange on the orders of seconds. A recent study shows that HDX-MS kinetic profiles are insensitive to starting concentration (when 20–200 times higher than the critical assembly concentration) and diluted time for D2O exchange.89 Despite this feature of small molecule assemblies, quantification of the exchange dynamics in some supramolecular systems can be challenging when there are multiple labile hydrogens at different sites of the molecule, and several exchange mechanisms present.89 Although HDX-MS does not inherently provide structural data, the technique provides detailed dynamics information at the molecular level for supramolecular systems without the constraint of introducing molecular labels.
Stochastic optical reconstruction microscopy (STORM).
Most dynamics characterization techniques provide time resolution, but lack spatial information. In contrast, stochastic optical reconstruction microscopy (STORM) can provide molecular exchange dynamics with spatial resolution. STORM, a super-resolution optical microscopy technique, can achieve ∼20 nm resolution by activating random small subsets of fluorophores at a given time and then superimposing each snapshot to obtain an image of the distribution of fluorophores.90 Albertazzi et al. first demonstrated this tool on BTA supramolecular fibers.91 This work successfully tracked the distribution of BTA monomers during exchange by autocorrecting and quantitatively analyzing the localization density profile, or the distribution of fluorophore localizations along the fiber. The results suggest the absence of any local ordering and hence homogeneous exchange along the BTA supramolecular fibers. In contrast to the BTA supramolecular fibers, da Silva et al. observed heterogeneous exchange and spatial variations in dynamics across the length of PA nanofibers by examining the localization density profiles obtained from STORM.92 In this study, two types of PA nanofibers tagged with distinct fluorescent dyes (Cy3-PA and Cy5-PA) were imaged and then combined in solution to probe the mechanism of exchange. After 48 hours, Cy5-PA (red) was found dispersed throughout the entire length of the Cy3-PA (green) nanofiber as shown in the localization maps (Fig. 4a). Tracking the exchange process of Cy3-labelled fiber over a shorter time span revealed that PA nanofibers follow an expulsion–reinclusion mechanism of exchange dynamics. Fig. 4b shows the autocorrelation plots and model fitting from the localization density profiles alone the nanofiber backbones of Fig. 4a. At 1 min, the autocorrelation plots of Cy5-PA fiber can be modeled by a homogeneous distribution, suggesting a random exchange of monomers. As the exchange proceeds, the autocorrelation curves of the Cy5-PA fiber suggest the emergence of heterogeneous exchange behavior and the structural variations along the fiber or between fibers. The increased standard deviation of linear density of Cy5 (Fig. 4c), defined as the number of Cy5 dye localizations per nanometer arc length of initially only Cy3 labelled nanofiber, also indicates the presence of intrinsic structural diversity. The authors concluded that this heterogeneity of PA nanofibers is likely due to the highly cohesive β-sheets within the structure, whereas the supramolecular systems with weak internal cohesion can exchange molecules to produce completely homogeneous structure.
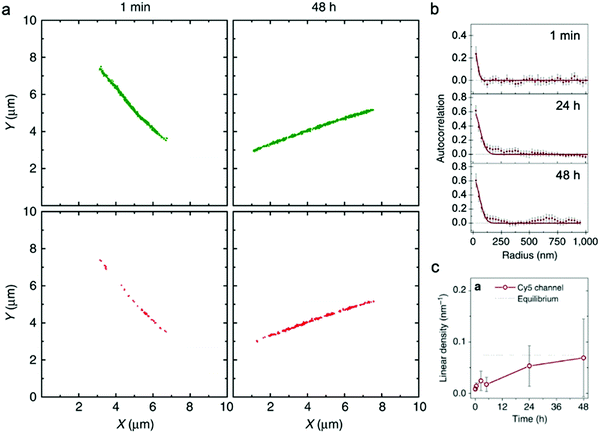 |
| Fig. 4 (a) Localization maps of PA nanofibers with photo-switchable sulfonated cyanine dyes Cy3 (green) and Cy5 (red) reconstructed from STORM. (b) Averaged autocorrelation plots for Cy5-labelled PAs are fit to random exchange models. (c) Time course-averaged linear density of red dye (Cy5) on nanofibers that were initially only labelled with green dyes (Cy3). Adapted from ref. 92, Copyright 2016 Springer Nature. | |
Similar to EPR, and in contrast to HDX-MS, STORM requires labeling the supramolecular nanostructure with molecular probes, which in some cases could disturb self-assembly or surface chemistry.93 Additionally, higher spatial resolution characterization (e.g., cryo-TEM, X-ray scattering) is frequently carried out to complement the spatial resolution (25 nm–1 μm) and time resolution (∼10 minutes) of STORM. In summary, STORM is a powerful technique that allows one to obtain both timescale and spatial information of exchange dynamics, while providing analysis of exchange mechanisms, in supramolecular nanostructures. STORM is also generally applicable to most supramolecular nanomaterials systems and can be used to observe irreversible nucleation and growth mechanisms through the process of self-assembly.94
2.3 Water dynamics in supramolecular systems
The dynamics of water around biological structures is critical to their function and substantially impacts their structural stability and physiological function.95 For example, water at the surface of a protein is likely to mediate binding-efficacy.96 Motions of water in aqueous systems are categorized into three types with distinct dynamics: bulk water, hydration water (located at interface of water and solute), and bound or structural water. The latter two types of water are also expected to play important roles in the structure and properties of supramolecular systems. A few experimental tools allow access to water dynamics information such as dielectric spectroscopy,97,98 NMR,99 neutron scattering techniques,100 and infrared spectroscopy.101 Although these are valuable tools for investigating water dynamics, challenges in spatial resolution and site-specificity pose limitations to their applicability to supramolecular structures. In this subsection, we introduce an emerging EPR-based technique, Overhauser dynamic nuclear polarization relaxometry (ODNP), which can be used to quantify the translational diffusion rate of water molecules over a broad range of water correlation times (200–900 ps) within less than a nanometer of a spin label.102 ODNP has previously been used to investigate water dynamics in polymers, hydrogels, biological macromolecules, and membranes.103–108 Although the technical details of ODNP exceed the scope of this review, we refer interested readers to a number of articles and book chapters, which provide details of theory, data analysis, and experimental protocols.109–111
Ortony et al. mapped water dynamics through the cross-section of a supramolecular PA nanofiber using ODNP.112 For ODNP, nitroxyl spin labels were covalently attached by SDSL at different positions along the peptide amphiphile. Local water dynamics in the supramolecular nanostructure were measured at each spin label site. Water correlation times measured by ODNP (Fig. 5a) reveal that water in the nanofiber's aliphatic core is fast moving, with short correlation times, whereas the water at the surface of nanofibers moves slowly with long correlation times. These results were corroborated by MD simulations (Fig. 5b). The water dynamics profile indicates that water becomes “stuck” in the highly hydrophilic and charged head group domain. The suppressed surface water dynamics are likely to influence the activity of surface-bound chemical moieties, which is relevant to ligand–receptor binding affinities reported in previous studies.113,114
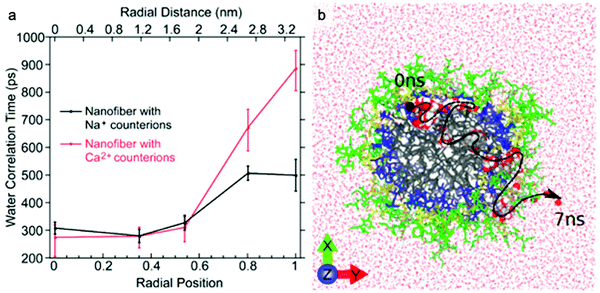 |
| Fig. 5 (a) Water-correlation times measured by ODNP as a function of radial position within the cross section of a supramolecular peptide amphiphile (PA) nanofiber. Translational diffusion rate of water molecules is inversely proportional to water correlation time. (b) Molecular dynamics simulation illustrates the diffusion of a representative water molecule within the nanofiber. Adapted from ref. 112, Copyright 2017 American Chemical Society. | |
ODNP was also used in this report to investigate the effect of modifying counterion on local water dynamics. The nanofiber solution was gelled by adding divalent Ca2+ counterions. Upon addition of Ca2+, the surface water dynamics slow considerably, while no changes to the inner-core water dynamics occur (Fig. 5a). The authors hypothesized that gelation of supramolecular nanofibers occurs via structuring of surface water, rather than the conventional polymer cross-linking model, based on this finding.115 This study demonstrates the ability of ODNP to reveal water dynamics in supramolecular nanostructures, and this site-specific technique is expected to answer questions regarding the effect of water dynamics on structure–property relationships in the future.
3. Harnessing dynamics in molecular design
Structure–property relationships are commonly the focal point of design in molecular self-assembly. With increasing access to, and an improving understanding about, local dynamics in supramolecular structures, reports suggest that dynamics are important in structure–property relationships, namely as structure–dynamics–property relationships, are rising.37,44,45 For example, early studies of peptide amphiphile nanofibers focus on molecular structure-mediated control of mechanical properties,116 biological activity,117 and cell culture,118 differences in properties can now be understood from a dynamics perspective as well. Studies into BTA supramolecular systems have been used to establish relationships between surface dynamics and stimuli-responsiveness,119 but mainly implement computational approaches (which fall beyond the scope of this review). We note that deterministic links between structure, dynamics, and properties of supramolecular nanomaterials remain challenging to investigate experimentally. However, recent studies have started to uncover the significance of dynamics in self-assembled systems, and we summarize those in this section.
Dynamic combinatorial libraries
Dynamic combinatorial libraries, as applied to peptide self-assembly, allow multiple components to reversibly combine and exchange to ultimately favor the structure with the lowest free energy. Pappas et al. demonstrated the use of searchable dynamic peptide libraries to identify self-assembling nanostructures with the specific sequence of the final peptide component dictated by self-assembly propensity.120 Here, unprotected dipeptides were mixed and the nonspecific endoprotease thermolysin, was used to reversibly link dipeptides by covalent bonds. Then, the peptides that self-assembled to form thermodynamically stable structures were effectively removed from solution and amplified via Le Châtelier's principle. This process is schematically represented by the potential energy surface in Fig. 6a. Using this combinatorial method, it is possible to find peptide sequences that self-assemble into stable nanostructures from a mixture of mono- or dipeptides (Fig. 6b).121 Notably in this study, varying the environmental conditions (salts, solvents and combinations) impacted component selection, which is the result of the balance between hydrogen bonding and the hydrophobic effect. Although the dependence on environmental conditions can be explained by energetics (i.e. varying the environment changes the potential energy surface), the rate of molecular exchange should be related to the likelihood reaching of kinetic traps. Therefore, exchange dynamics play a critical role in both the methodology and application of combinatorial library approaches to supramolecular assembly.
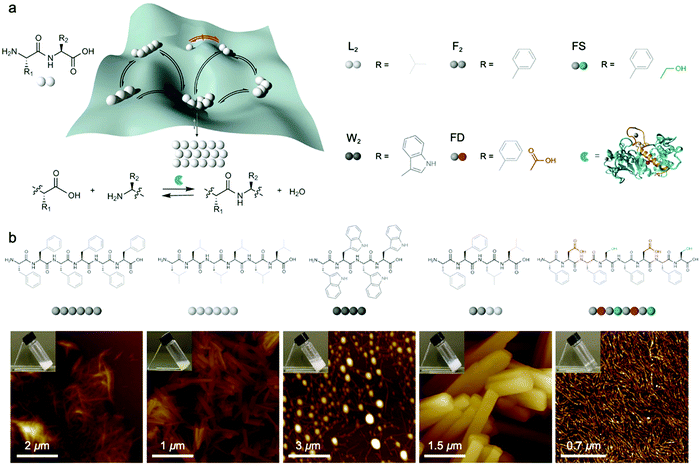 |
| Fig. 6 (a) Potential energy of peptide oligomers (strings of beads) formation and chemical inputs (selected dipeptides from original report and thermolysin). (b) Chemical structure and AFM images of the peptide assemblies that were amplified in the searchable dynamic peptide libraries. Adapted from ref. 120, Copyright 2016 Springer Nature. | |
Dynamics-dictated polymorphism
Matsumoto et al. demonstrated that exchange dynamics dictate nanostructure architecture by studying BTA (benzene-1,3,5-tricarboxamide) amphiphiles.122 In the case shown in Fig. 7, the length of the carboxylic acid-terminated aliphatic arm of amphiphilic BTA molecules was varied. BTA 2 assembles into a diverse assortment of membranes and ribbons due to the formation of bilayers, whereas BTA 3 forms hollow tubes in addition to the morphologies observed in BTA 2. HDX-MS of BTA 3 nanostructures shows slower monomer exchange with an increase in amphiphilicity. In contrast to BTA 2A, BTA 3 shows temperature-dependent monomer exchange rates (Fig. 7b). Also upon increasing temperature, the tubular assemblies of BTA 3 are elongated into well-ordered hollow nanotubes, as shown in Fig. 7c. Based on this knowledge, the authors concluded that polymorphism of BTA 3 originates from the trade-off between slow exchange dynamics and the higher energetic cost of exposing the membrane edges to solvent. Therefore, optimizing exchange dynamics is as critical as molecular structure or solvent condition in molecular engineering of self-assembled architectures. Several recent studies also revealed dynamics-controlled polymorphism in different self-assembly systems by altering temperature,123 pH,124 and ultrasonication conditions.125
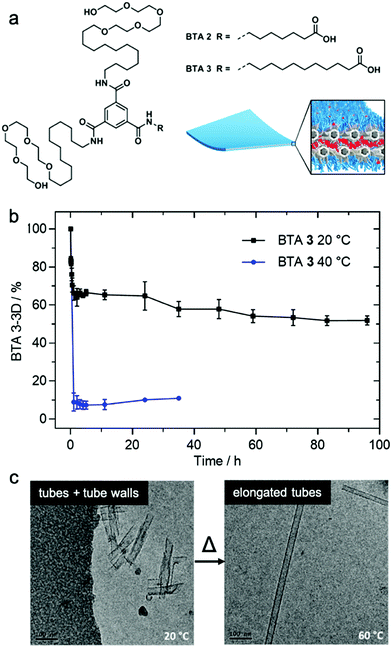 |
| Fig. 7 (a) Chemical structure of BTA 2 and BTA 3, which are schematically illustrated to form a membrane packing morphology. (b) Exchange rates illustrated by HDX-MS reveals the conversion of BTA 3-3D into BTA 3-6D at 20 and 40 °C. (c) Cryo-TEM images of BTA 3 show a transformation from short nanotubes with ill-formed tube walls to well-formed nanotubes after heating from 20 °C to 60 °C. Adapted from ref. 111, Copyright 2018 American Chemical Society. | |
Defect-induced dynamics
Baker et al. reported the first observation of structure–property relationships with regard to equilibrium dynamics in water-soluble supramolecular polymers.93 In this study (1) achiral and (2) chiral BTA molecules were designed. The chiral BTA contains stereogenic methyl groups, whereas the achiral BTA does not (Fig. 8a inset). Upon self-assembly, nearly identical dimensions of BTA nanofibers of (1) and (2) were observed by cryo-TEM, X-ray scattering (SAXS), and STORM. FRET studies reveal that the addition of the stereogenic methyl groups greatly reduces monomer exchange between the fibers, as shown in Fig. 8a. This significant difference in equilibrium dynamics between achiral (1) and chiral (2) was further investigated by MD simulations. Despite average self-assembly energies (ΔE) which are calculated to be similar for (1) and (2), and which suggest that (2) fibers are not particularly more stable than (1) fibers, the internal ordering and uniformity of ΔE along the fibers gives rise to detectable differences. While the ΔE of (1) fibers show a random distribution around an average value, (2) fibers show sequences of stable BTAs for 5–7 monomers interrupted by unstable points (Fig. 8b). Following up on this observation, atomistic, coarse-grained modelling combined with advanced simulation approaches revealed the molecular exchange mechanism in this supramolecular system.126 It is understood from this study that the monomer exchange from the fiber surface is a stepwise process, where monomers diffuse first from the center to structural defects/discontinuities (A to B), and then diffuse into the water (B to C), as shown in Fig. 8c. The theoretical kinetics analysis shows that, although the mechanism of exchange in and out these fibers is globally similar, each exchange step is slowed down by ∼1 to 2 orders of magnitude in chiral nanofibers (2) compared to achiral nanofibers (1), consistent with the experimental observation. Since exchanging monomers directly from the nanofiber core to the solvent is energetically unfavorable, controlling the structural defects along the nanofiber is key for their dynamics. The presence of defects in self-assembling nanostructures is currently a topic of interest,127,128 and the relationship between local dynamics near defects and the “average” dynamics that we can observe experimentally is still an open question for the field. Understanding molecular-level exchange dynamics may allow us to rationally design bioinspired materials with controllable dynamic properties.
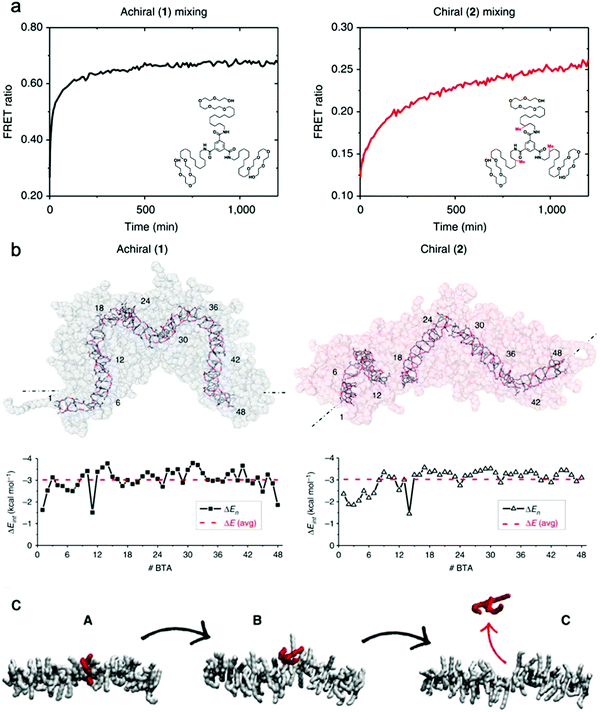 |
| Fig. 8 (a) Kinetic profiles of achiral (1) and chiral (2) fibers measured by FRET with their chemical structures inset. (b) Last snapshots of MD simulations of achiral (1) and chiral (2) fibers with self-assembly interaction energies (ΔE) of individual BTA molecules as a function of their positions. ΔE is defined as the energy gain for one BTA to stay incorporated with the fiber rather than being molecularly dissolved in solution. Adapted from ref. 93, Copyright 2015 Springer Nature. (c) A stepwise mechanism of monomer exchange shows that a defect/breakage must be first created along the stack (A and B transition) from which the monomer can jump out from the fiber (B and C transition). Adapted from ref. 126, Copyright 2017 Springer Nature. | |
Optimized molecular exchange drives the formation of hierarchical structure
Freeman et al. recently reported a hierarchical superstructure self-assembled from PA–DNA conjugates and PAs, which only occured within a limited range of monomer exchange dynamics.129 Mixing the aqueous solution containing self-assembled PA nanofibers with complementary oligonucleotides (Fig. 9a) not only yielded a cross-linked gel, as expected, but also a superstructure in which large micrometer-sized bundles of fibers segregated within a network of individual fibers. MD simulations highlighted that only homogeneous hydrogels were formed when the molecular exchange of DNA monomers between PA nanofibers was prohibited, whereas bundles of nanofibers were formed when molecular exchange is allowed between PA nanofibers (Fig. 9b). Monomer exchange was also confirmed experimentally by FRET in this report. These observations indicate that exchange dynamics can impact and modify the macroscopic and hierarchical structure in supramolecular self-assembly. Furthermore, MD simulation also showed that the bundle growth rate is sensitive to the balance between the monomer–monomer association energy and the energy associated with conjugated interaction across the fiber. Following this concept, Wester et al. experimentally proved that the formation of bundled hierarchical filaments can be controlled by altering the intermolecular cohesion of self-assembled monomers.130 PAs with oppositely charged surface groups and with or without β-sheet domains were synthesized as shown in Fig. 9c. Thorough structural investigation via TEM, SAXS and FTIR revealed that that assemblies without β-sheet domains – that is, with weak interactions and fast exchange dynamics – readily form fibrous superstructures of bundled filaments as molecules redistribute upon mixing. In contrast, assemblies with β-sheet domains – strong, cohesive interactions and slow exchange dynamics – suppress superstructure formation as shown in Fig. 9d. Additionally, the viscosity of the former mixture increased drastically after mixing and bundled filaments are formed, indicating a new method in processing of supramolecular materials to create 3D structures. This finding once again demonstrates the dynamics–property relationships in supramolecular self-assembly, and suggests that controlling dynamics can be important for future applications of molecular self-assembly.
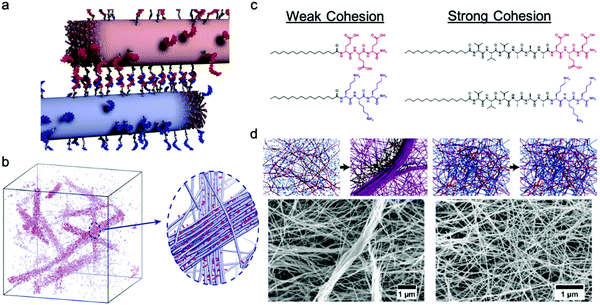 |
| Fig. 9 (a) Illustration of PA fibers cross-linked by DNA hybridization; fibers are shown in their initial state prior to monomer exchange. (b) Simulation snapshots showing the emergence of bundles of fibers when molecular exchange is allowed. Magnified view shows bundle of fibers (blue) enriched with DNA (pink) in a matrix of individual fibers depleted of DNA monomers. Adapted from ref. 129, Copyright 2018 The American Association for the Advancement of Science. (c) Molecular structures of weak and strong cohesive interaction PAs. (d) Representation of formation process and SEM images of self-assembled superstructures of weak and strong cohesive interaction PAs. Adapted from ref. 130, Copyright 2020 American Chemical Society. | |
Stabilizing the nanostructure slows down dynamics
Although the studies described above highlight the importance of optimizing dynamics in more fluid assemblies such as those for biomaterials applications, a different approach by Christoff-Tempesta et al. presents small-molecule amphiphiles which form ultra-stable nanostructures in water by completely suppressing molecular exchange.131 Non-covalent intermolecular interactions in supramolecular systems are usually relatively weak, and as a result, they often lead to fast dynamics and are unstable in air, where they lack the hydrophobic driving force required to maintain their nanostructure. In this study, a Kevlar-inspired domain was introduced into the amphiphile molecular structure in order to suppress conformational and exchange dynamics (Fig. 10a). In this case, aromatic amides (aramids) were positioned between a hydrophilic head group and an aliphatic tail to yield “aramid amphiphiles” (AAs). AAs spontaneously self-assemble into nanoribbons upon addition of water, with collective hydrogen bonding propagating down the long-axis, and interplane π–π stacking dominating across the nanoribbon width. Statistical topographical analysis of atomic force micrographs revealed that individual nanoribbons exhibit a Young's modulus of 1.7 GPa and tensile strength of 1.9 GPa. Similar to PAs,132 AA nanoribbons’ high surface charge densities allow them to be shear-aligned in divalent salt solution to form a 1-dimensional gels of arbitrary length (Fig. 10c). The mechanical stability of the individual nanoribbons, combined with the strong interfacial interactions, allow AAs to maintain their structural stability upon drying, forming flexible, solid-state threads of aligned nanoribbons that support 200 times their weight (Fig. 10c). In this study, the AA motif was intentionally designed to minimize the dynamics in this system, where undetectably slow exchange was observed by FRET (Fig. 10d). This study provides a route to nanostructured, air-stable molecular materials by slowing exchange dynamics to overcome the instabilities pervasive in small molecule assemblies.
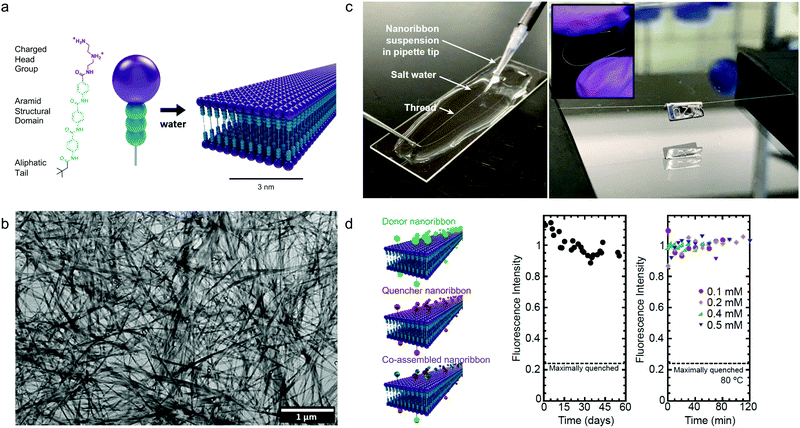 |
| Fig. 10 Self-assembly of aramid amphiphiles (AAs). (a) A representative molecular structure of an aramid amphiphile and illustration showing its self-assembly into mechanically robust nanoribbons with suppressed molecular exchange. (b) Dried AA nanoribbons of are observed in a representative transmission electron micrograph (TEM). (c) Left: A nanoribbon suspension is extruded into a sodium sulfate solution to form a 1-dimensional gel. Right: A 5 cm-long nanoribbon thread, dried from the gel, whose mass totals 0.1 mg is suspended over a trough and supports a 20 mg weight. Inset: The thread composed of aligned nanoribbons that can be bent and handled easily. (d) Nanoribbons labeled with the FRET donor EDANS is quenched by 76% when co-assembled with FRET quencher DABCYL (left). A nearly constant fluorescence intensity is observed after mixing donor and quencher ribbons over 55 days (middle) and after heating to 80 °C (right), showing that molecular exchange is undetectable. Adapted from ref. 131, Copyright 2021 Springer Nature. | |
4. Conclusions and outlook
In this review, we introduced recently established experimental tools for dynamics investigation of small molecule assemblies, and we highlighted several reports that reveal the importance of these dynamics. While structure–property relationships have long been a prevailing design concept in synthetic self-assembling systems, attention to dynamic behavior and structure–dynamics–property relationships has recently come into focus. Techniques including EPR, HDX-MS, STORM and ODNP, have begun addressing questions related to conformational, exchange, and water dynamics in supramolecular systems. EPR spectroscopy requires incorporation of nitroxyl radical spin labels, provides quantitative information about molecular motion with sub-nanometer resolution, and is now more accessible due to advances in synthetic techniques and EPR lineshape analysis software. HDX-MS can be used to characterize monomer exchange in supramolecular systems without disturbing the structure, and is broadly applicable to constituent amphiphiles with discrete molecular weights and labile hydrogens, even in multicomponent systems. STORM is a powerful technique to obtain both timescale and spatial information of exchange dynamics in supramolecular structures, and can provide insight into exchange mechanisms. ODNP requires spin labeling, and is useful for investigating the dynamics of water molecules with site specificity. The use of these experimental tools in supramolecular assemblies has shown the value in studying their dynamics. Additionally, it is important to continue adopting new biophysical characterization tools such as state-of-the-art fluorescence spectroscopy,133 magnetic resonance spectroscopy,134in situ X-ray techniques,135 and others. This improved understanding of dynamics will allow us to think of self-assembled nanostructures not only in 3-dimensional space, but also propagating in time.
Author contributions
J. H. O. conceived the topic of this review. Y. C. designed the structure of this manuscript and reviewed the literature. Y. C. and J. H. O. wrote the manuscript. Y. C., T. C.-T. and S. J. K. edited the manuscript, with direction from J. H. O.
Conflicts of interest
The authors declare no competing interests.
Acknowledgements
The authors thank Thomas Lemmin for supplying the graphic in Fig. 1. This work was supported in part by the National Science Foundation under Grant No. CHE-1945500. This work was supported in part by the Professor Amar G. Bose Research Grant Program and the Abdul Latif Jameel Water and Food Systems Lab. T. C.-T. acknowledges the support of the National Science Foundation Graduate Research Fellowship Program under Grant No. 1122374 and the support of the Martin Family Society of Fellows for Sustainability.
References
- S. J. Singer and G. L. Nicolson, Science, 1972, 175, 720–731 CrossRef CAS PubMed.
- A. J. Krmpot, S. N. Nikolić, S. Oasa, D. K. Papadopoulos, M. Vitali, M. Oura, S. Mikuni, P. Thyberg, S. Tisa, M. Kinjo, L. Nilsson, L. Terenius, R. Rigler and V. Vukojević, Anal. Chem., 2019, 91, 11129–11137 CrossRef CAS PubMed.
- G. Bhabha, J. Lee, D. C. Ekiert, J. Gam, I. A. Wilson, H. J. Dyson, S. J. Benkovic and P. E. Wright, Science, 2011, 332, 234–238 CrossRef CAS PubMed.
- S.-R. Tzeng and C. G. Kalodimos, Nature, 2009, 462, 368–372 CrossRef CAS PubMed.
- J. L. C. M. van de Vossenberg, A. J. M. Driessen and W. N. Konings, Extremophiles, 1998, 2, 163–170 CrossRef CAS PubMed.
- W. Shinoda, M. Mikami, T. Baba and M. Hato, Chem. Phys. Lett., 2004, 390, 35–40 CrossRef CAS.
- A. O. Chugunov, P. E. Volynsky, N. A. Krylov, I. A. Boldyrev and R. G. Efremov, Sci. Rep., 2014, 4, 7462 CrossRef CAS PubMed.
-
J. N. Israelachvili, Intermolecular and Surface Forces, Elsevier Academic Press Inc, San Diego, 3rd edn, 2011 Search PubMed.
- S. Zhang, Nat. Biotechnol., 2003, 21, 1171–1178 CrossRef CAS PubMed.
- T. Aida, E. W. Meijer and S. I. Stupp, Science, 2012, 335, 813–817 CrossRef CAS PubMed.
- M. J. Webber, E. A. Appel, E. W. Meijer and R. Langer, Nat. Mater., 2016, 15, 13–26 CrossRef CAS PubMed.
- S. E. Paramonov, H.-W. Jun and J. D. Hartgerink, J. Am. Chem. Soc., 2006, 128, 7291–7298 CrossRef CAS PubMed.
- S. S. Babu, V. K. Praveen and A. Ajayaghosh, Chem. Rev., 2014, 114, 1973–2129 CrossRef CAS PubMed.
- A. Das and S. Ghosh, Angew. Chem., Int. Ed., 2014, 53, 2038–2054 CrossRef CAS PubMed.
- C. Wang, Q. Chen, H. Xu, Z. Wang and X. Zhang, Adv. Mater., 2010, 22, 2553–2555 CrossRef CAS PubMed.
- D. S. Kim, J. Chang, S. Leem, J. S. Park, P. Thordarson and J. L. Sessler, J. Am. Chem. Soc., 2015, 137, 16038–16042 CrossRef CAS PubMed.
- M. Kumar, P. Brocorens, C. Tonnelé, D. Beljonne, M. Surin and S. J. George, Nat. Commun., 2014, 5, 5793 CrossRef CAS PubMed.
- S. Fleming and R. V. Ulijn, Chem. Soc. Rev., 2014, 43, 8150–8177 RSC.
- B. O. Okesola and A. Mata, Chem. Soc. Rev., 2018, 47, 3721–3736 RSC.
- M. Zelzer and R. V. Ulijn, Chem. Soc. Rev., 2010, 39, 3351–3357 RSC.
- J. B. Matson, C. J. Newcomb, R. Bitton and S. I. Stupp, Soft Matter, 2012, 8, 3586–3595 RSC.
- E. Beniash, J. D. Hartgerink, H. Storrie, J. C. Stendahl and S. I. Stupp, Acta Biomater., 2005, 1, 387–397 CrossRef PubMed.
- H. Kim and C. Leal, ACS Nano, 2015, 9, 10214–10226 CrossRef CAS PubMed.
- A. G. Cheetham, R. W. Chakroun, W. Ma and H. Cui, Chem. Soc. Rev., 2017, 46, 6638–6663 RSC.
- L. Lombardi, Y. Shi, A. Falanga, E. Galdiero, E. de Alteriis, G. Franci, I. Chourpa, H. S. Azevedo and S. Galdiero, Biomacromolecules, 2019, 20, 1362–1374 CrossRef CAS PubMed.
- D.-Y. Kim, J. Koo, S.-I. Lim and K.-U. Jeong, Adv. Funct. Mater., 2018, 28, 1707075 CrossRef.
- M. Ni, S. Zhuo, C. Iliescu, P. T. C. So, J. S. Mehta, H. Yu and C. A. E. Hauser, J. Biophotonics, 2019, 12, e201900065 CrossRef CAS PubMed.
- M. Yemini, M. Reches, E. Gazit and J. Rishpon, Anal. Chem., 2005, 77, 5155–5159 CrossRef CAS PubMed.
- Y. Yamamoto, G. Zhang, W. Jin, T. Fukushima, N. Ishii, A. Saeki, S. Seki, S. Tagawa, T. Minari, K. Tsukagoshi and T. Aida, Proc. Natl. Acad. Sci. U. S. A., 2009, 106, 21051–21056 CrossRef CAS PubMed.
- P. Beker, I. Koren, N. Amdursky, E. Gazit and G. Rosenman, J. Mater. Sci., 2010, 45, 6374–6378 CrossRef CAS.
- A. S. Weingarten, R. V. Kazantsev, L. C. Palmer, D. J. Fairfield, A. R. Koltonow and S. I. Stupp, J. Am. Chem. Soc., 2015, 137, 15241–15246 CrossRef CAS PubMed.
- A. Levin, T. A. Hakala, L. Schnaider, G. J. L. Bernardes, E. Gazit and T. P. J. Knowles, Nat. Rev. Chem., 2020, 4, 615–634 CrossRef CAS.
- R. V. Ulijn and A. M. Smith, Chem. Soc. Rev., 2008, 37, 664–675 RSC.
- L. Guyon, E. Lepeltier and C. Passirani, Nano Res., 2018, 11, 2315–2335 CrossRef CAS.
- O. Dumele, J. Chen, J. V. Passarelli and S. I. Stupp, Adv. Mater., 2020, 32, 1907247 CrossRef CAS.
- K. Tao, P. Makam, R. Aizen and E. Gazit, Science, 2017, 358, eaam9756 CrossRef PubMed.
- T. D. Clemons and S. I. Stupp, Prog. Polym. Sci., 2020, 111, 101310 CrossRef CAS PubMed.
-
G. Ochbaum and R. Bitton, in Self-assembling Biomaterials, ed. H. S. Azevedo and R. M. P. da Silva, Woodhead Publishing, 2018, vol. 14, pp. 291–304 Search PubMed.
- C. L. Pizzey, W. C. Pomerantz, B.-J. Sung, V. M. Yuwono, S. H. Gellman, J. D. Hartgerink, A. Yethiraj and N. L. Abbott, J. Chem. Phys., 2008, 129, 095103 CrossRef PubMed.
- M. Wang, J. Wang, P. Zhou, J. Deng, Y. Zhao, Y. Sun, W. Yang, D. Wang, Z. Li, X. Hu, S. M. King, S. E. Rogers, H. Cox, T. A. Waigh, J. Yang, J. R. Lu and H. Xu, Nat. Commun., 2018, 9, 5118 CrossRef.
- G. Gottarelli, S. Lena, S. Masiero, S. Pieraccini and G. P. Spada, Chirality, 2008, 20, 471–485 CrossRef CAS.
- H. Jeffery, D. E. Beniash and S. I. Stupp, Science, 2001, 294, 1684–1688 CrossRef PubMed.
- C. J. Newcomb, T. J. Moyer, S. S. Lee and S. I. Stupp, Curr. Opin. Colloid Interface Sci., 2012, 17, 350–359 CrossRef CAS PubMed.
- T. Aida and E. W. Meijer, Isr. J. Chem., 2020, 60, 33–47 CrossRef CAS.
- C. Perego and G. M. Pavan, CHIMIA Int. J. Chem., 2020, 74, 734 CrossRef CAS PubMed.
- O.-S. Lee, S. I. Stupp and G. C. Schatz, J. Am. Chem. Soc., 2011, 133, 3677–3683 CrossRef CAS PubMed.
- O.-S. Lee, V. Cho and G. C. Schatz, Nano Lett., 2012, 12, 4907–4913 CrossRef CAS PubMed.
- A. Manandhar, M. Kang, K. Chakraborty, P. K. Tang and S. M. Loverde, Org. Biomol. Chem., 2017, 15, 7993–8005 RSC.
- D. Bochicchio and G. M. Pavan, Adv. Phys.: X, 2018, 3, 1436408 Search PubMed.
- J. A. McCammon, B. R. Gelin and M. Karplus, Nature, 1977, 267, 585–590 CrossRef CAS.
- A. Seelig and J. Seelig, Biochemistry, 1974, 13, 4839–4845 CrossRef CAS PubMed.
- H. Frauenfelder, S. Sligar and P. Wolynes, Science, 1991, 254, 1598–1603 CrossRef CAS PubMed.
- G. Di Paolo and P. De Camilli, Nature, 2006, 443, 651–657 CrossRef CAS PubMed.
- P. Ball, Chem. Rev., 2008, 108, 74–108 CrossRef CAS PubMed.
- G. Zaccai, Science, 2000, 288, 1604–1607 CrossRef CAS PubMed.
- J. S. Fraser, M. W. Clarkson, S. C. Degnan, R. Erion, D. Kern and T. Alber, Nature, 2009, 462, 669–673 CrossRef CAS PubMed.
- A. G. P. III, Annu. Rev. Biophys. Biomol. Struct., 2001, 30, 129–155 CrossRef PubMed.
-
A. G. Palmer, C. D. Kroenke and J. Patrick Loria, in Methods in Enzymology, ed. T. L. James, V. Dötsch and U. Schmitz, Academic Press, 2001, vol. 10, pp. 204–238 Search PubMed.
- S. Woutersen, Y. Mu, G. Stock and P. Hamm, Proc. Natl. Acad. Sci. U. S. A., 2001, 98, 11254–11258 CrossRef CAS.
- F. Gabel, D. Bicout, U. Lehnert, M. Tehei, M. Weik and G. Zaccai, Q. Rev. Biophys., 2002, 35, 327–367 CrossRef CAS PubMed.
-
W. R. Lindemann and J. H. Ortony in Self-assembling Biomaterials, ed. H. S. Azevedo and R. M. P. da Silva, Woodhead Publishing, 2018, 13, pp. 275–289 Search PubMed.
- D. E. Budil, S. Lee, S. Saxena and J. H. Freed, J. Magn. Reson., Ser. A, 1996, 120, 155–189 CrossRef CAS.
- W. L. Hubbell, D. S. Cafiso and C. Altenbach, Nat. Struct. Biol., 2000, 7, 735–739 CrossRef CAS.
- C. Toniolo, M. Crisma and F. Formaggio, Pept. Sci., 1998, 47, 153–158 CrossRef CAS.
- W. R. Lindemann, E. D. Evans, A. J. Mijalis, O. M. Saouaf, B. L. Pentelute and J. H. Ortony, Sci. Rep., 2020, 10, 2597 CrossRef CAS PubMed.
- W. R. Lindemann, A. J. Mijalis, J. L. Alonso, P. P. Borbat, J. H. Freed, M. A. Arnaout, B. L. Pentelute and J. H. Ortony, Biomacromolecules, 2020, 21, 2786–2794 CrossRef CAS PubMed.
- S. Stoll and A. Schweiger, J. Magn. Reson., 2006, 178, 42–55 CrossRef CAS PubMed.
- W. R. Lindemann, T. Christoff-Tempesta and J. H. Ortony, Biophys. J., 2020, 119, 1937–1945 CrossRef CAS PubMed.
- J. H. Ortony, C. J. Newcomb, J. B. Matson, L. C. Palmer, P. E. Doan, B. M. Hoffman and S. I. Stupp, Nat. Mater., 2014, 13, 812–816 CrossRef CAS PubMed.
- T. Harayama and H. Riezman, Nat. Rev. Mol. Cell Biol., 2018, 19, 281–296 CrossRef CAS PubMed.
- O. G. Mouritsen and M. J. Zuckermann, Lipids, 2004, 39, 1101–1113 CrossRef CAS.
- K. Simons and E. Ikonen, Science, 2000, 290, 1721–1726 CrossRef CAS PubMed.
- Z. Korade and A. K. Kenworthy, Neuropharmacology, 2008, 55, 1265–1273 CrossRef CAS PubMed.
- A. Sarkar, R. Sasmal, C. Empereur-mot, D. Bochicchio, S. V. K. Kompella, K. Sharma, S. Dhiman, B. Sundaram, S. S. Agasti, G. M. Pavan and S. J. George, J. Am. Chem. Soc., 2020, 142, 7606–7617 CrossRef CAS PubMed.
- A. Sarkar, T. Behera, R. Sasmal, R. Capelli, C. Empereur-mot, J. Mahato, S. S. Agasti, G. M. Pavan, A. Chowdhury and S. J. George, J. Am. Chem. Soc., 2020, 142, 11528–11539 CrossRef CAS PubMed.
- P. G. Wu and L. Brand, Anal. Biochem., 1994, 218, 1–13 CrossRef CAS PubMed.
- A. Nolles, E. Hooiveld, A. H. Westphal, W. J. H. van Berkel, J. M. Kleijn and J. W. Borst, Langmuir, 2018, 34, 12083–12092 CrossRef CAS PubMed.
- P. Rajdev and S. Ghosh, J. Phys. Chem. B, 2019, 123, 327–342 CrossRef CAS PubMed.
- I. R. Kleckner and M. P. Foster, Biochim. Biophys. Acta, 2011, 1814, 942–968 CrossRef CAS PubMed.
- L. Konermann, J. Pan and Y.-H. Liu, Chem. Soc. Rev., 2011, 40, 1224–1234 RSC.
- S. Maric, T. K. Lind, M. R. Raida, E. Bengtsson, G. N. Fredrikson, S. Rogers, M. Moulin, M. Haertlein, V. T. Forsyth, M. R. Wenk, T. G. Pomorski, T. Arnebrant, R. Lund and M. Cárdenas, Sci. Rep., 2019, 9, 7591 CrossRef PubMed.
- X. Lou, R. P. M. Lafleur, C. M. A. Leenders, S. M. C. Schoenmakers, N. M. Matsumoto, M. B. Baker, J. L. J. van Dongen, A. R. A. Palmans and E. W. Meijer, Nat. Commun., 2017, 8, 15420 CrossRef PubMed.
- C. M. A. Leenders, M. B. Baker, I. A. B. Pijpers, R. P. M. Lafleur, L. Albertazzi, A. R. A. Palmans and E. W. Meijer, Soft Matter, 2016, 12, 2887–2893 RSC.
- M. Fernández-Castaño Romera, X. Lou, J. Schill, G. ter Huurne, P.-P. K. H. Fransen, I. K. Voets, C. Storm and R. P. Sijbesma, J. Am. Chem. Soc., 2018, 140, 17547–17555 CrossRef PubMed.
- J. Liu, M. J. G. Schotman, M. M. R. M. Hendrix, X. Lou, P. P. Marín San Román, I. K. Voets and R. P. Sijbesma, J. Polym. Sci., 2021 DOI:10.1002/pol.20200888.
- B. N. S. Thota, X. Lou, D. Bochicchio, T. F. E. Paffen, R. P. M. Lafleur, J. L. J. van Dongen, S. Ehrmann, R. Haag, G. M. Pavan, A. R. A. Palmans and E. W. Meijer, Angew. Chem., Int. Ed., 2018, 57, 6843–6847 CrossRef CAS.
- R. P. M. Lafleur, S. M. C. Schoenmakers, P. Madhikar, D. Bochicchio, B. Baumeier, A. R. A. Palmans, G. M. Pavan and E. W. Meijer, Macromolecules, 2019, 52, 3049–3055 CrossRef CAS.
- G. R. Masson, J. E. Burke, N. G. Ahn, G. S. Anand, C. Borchers, S. Brier, G. M. Bou-Assaf, J. R. Engen, S. W. Englander, J. Faber, R. Garlish, P. R. Griffin, M. L. Gross, M. Guttman, Y. Hamuro, A. J. R. Heck, D. Houde, R. E. Iacob, T. J. D. Jørgensen, I. A. Kaltashov, J. P. Klinman, L. Konermann, P. Man, L. Mayne, B. D. Pascal, D. Reichmann, M. Skehel, J. Snijder, T. S. Strutzenberg, E. S. Underbakke, C. Wagner, T. E. Wales, B. T. Walters, D. D. Weis, D. J. Wilson, P. L. Wintrode, Z. Zhang, J. Zheng, D. C. Schriemer and K. D. Rand, Nat. Methods, 2019, 16, 595–602 CrossRef CAS PubMed.
- X. Lou, S. M. C. Schoenmakers, J. L. J. van Dongen, M. Garcia-Iglesias, N. M. Casellas, M. Fernández-Castaño Romera, R. P. Sijbesma, E. W. Meijer and A. R. A. Palmans, J. Polym. Sci., 2021, 1–11 CAS.
- B. Huang, M. Bates and X. Zhuang, Annu. Rev. Biochem., 2009, 78, 993–1016 CrossRef CAS PubMed.
- L. Albertazzi, D. van der Zwaag, C. M. A. Leenders, R. Fitzner, R. W. van der Hofstad and E. W. Meijer, Science, 2014, 344, 491–495 CrossRef CAS PubMed.
- R. M. P. da Silva, D. van der Zwaag, L. Albertazzi, S. S. Lee, E. W. Meijer and S. I. Stupp, Nat. Commun., 2016, 7, 11561 CrossRef CAS PubMed.
- M. B. Baker, L. Albertazzi, I. K. Voets, C. M. A. Leenders, A. R. A. Palmans, G. M. Pavan and E. W. Meijer, Nat. Commun., 2015, 6, 6234 CrossRef PubMed.
- L. H. Beun, L. Albertazzi, D. van der Zwaag, R. de Vries and M. A. Cohen Stuart, ACS Nano, 2016, 10, 4973–4980 CrossRef CAS PubMed.
- S. K. Pal and A. H. Zewail, Chem. Rev., 2004, 2099–2124 CrossRef CAS PubMed.
- J. Schiebel, R. Gaspari, T. Wulsdorf, K. Ngo, C. Sohn, T. E. Schrader, A. Cavalli, A. Ostermann, A. Heine and G. Klebe, Nat. Commun., 2018, 9, 3559 CrossRef PubMed.
- N. Nandi, K. Bhattacharyya and B. Bagchi, Chem. Rev., 2000, 100, 2013–2046 CrossRef CAS PubMed.
- S. K. Pal, J. Peon, B. Bagchi and A. H. Zewail, J. Phys. Chem. B, 2002, 106, 12376–12395 CrossRef CAS.
- N. V. Nucci, M. S. Pometun and A. J. Wand, Nat. Struct. Mol. Biol., 2011, 18, 245–249 CrossRef CAS PubMed.
- D. Russo, R. K. Murarka, J. R. D. Copley and T. Head-Gordon, J. Phys. Chem. B, 2005, 109, 12966–12975 CrossRef CAS.
- Y. S. Kim, L. Liu, P. H. Axelsen and R. M. Hochstrasser, Proc. Natl. Acad. Sci. U. S. A., 2009, 106, 17751–17756 CrossRef CAS.
- J. H. Ortony, C.-Y. Cheng, J. M. Franck, R. Kausik, A. Pavlova, J. Hunt and S. Han, New J. Phys., 2011, 13, 015006 CrossRef.
- P. J. M. Stals, C.-Y. Cheng, L. van Beek, A. C. Wauters, A. R. A. Palmans, S. Han and E. W. Meijer, Chem. Sci., 2016, 7, 2011–2015 RSC.
- J. H. Ortony, S.-H. Choi, J. M. Spruell, J. N. Hunt, N. A. Lynd, D. V. Krogstad, V. S. Urban, C. J. Hawker, E. J. Kramer and S. Han, Chem. Sci., 2014, 5, 58–67 RSC.
- S. Hussain, J. M. Franck and S. Han, Angew. Chem., Int. Ed., 2013, 52, 1953–1958 CrossRef CAS PubMed.
- B. D. Armstrong, J. Choi, C. López, D. A. Wesener, W. Hubbell, S. Cavagnero and S. Han, J. Am. Chem. Soc., 2011, 133, 5987–5995 CrossRef CAS PubMed.
- J. M. Franck, Y. Ding, K. Stone, P. Z. Qin and S. Han, J. Am. Chem. Soc., 2015, 137, 12013–12023 CrossRef CAS.
- J. H. Ortony, D. S. Hwang, J. M. Franck, J. H. Waite and S. Han, Biomacromolecules, 2013, 14, 1395–1402 CrossRef CAS PubMed.
-
I. Kaminker, R. Barnes and S. Han, in Methods in Enzymology, ed. P. Z. Qin and K. Warncke, Academic Press, 2015, vol. 16, pp. 457–483 Search PubMed.
- J. R. Biller, R. Barnes and S. Han, Curr. Opin. Colloid Interface Sci., 2018, 33, 72–85 CrossRef CAS.
-
J. M. Franck and S. Han, in Methods in Enzymology, ed. A. J. Wand, Academic Press, 2019, vol. 5, pp. 131–175 Search PubMed.
- J. H. Ortony, B. Qiao, C. J. Newcomb, T. J. Keller, L. C. Palmer, E. Deiss-Yehiely, M. Olvera de la Cruz, S. Han and S. I. Stupp, J. Am. Chem. Soc., 2017, 139, 8915–8921 CrossRef CAS PubMed.
- G. A. Silva, C. Czeisler, K. L. Niece, E. Beniash, D. A. Harrington, J. A. Kessler and S. I. Stupp, Science, 2004, 303, 1352–1355 CrossRef CAS PubMed.
- S. Sur, F. Tantakitti, J. B. Matson and S. I. Stupp, Biomater. Sci., 2015, 3, 530–532 RSC.
- T. Christoff-Tempesta, A. J. Lew and J. H. Ortony, Gels, 2018, 4, 40 CrossRef PubMed.
- E. T. Pashuck, H. Cui and S. I. Stupp, J. Am. Chem. Soc., 2010, 132, 6041–6046 CrossRef CAS.
- S. Sur, C. J. Newcomb, M. J. Webber and S. I. Stupp, Biomaterials, 2013, 34, 4749–4757 CrossRef CAS PubMed.
- C. J. Newcomb, S. Sur, J. H. Ortony, O.-S. Lee, J. B. Matson, J. Boekhoven, J. M. Yu, G. C. Schatz and S. I. Stupp, Nat. Commun., 2014, 5, 3321 CrossRef PubMed.
- A. Torchi, D. Bochicchio and G. M. Pavan, J. Phys. Chem. B, 2018, 122, 4169–4178 CrossRef CAS PubMed.
- C. G. Pappas, R. Shafi, I. R. Sasselli, H. Siccardi, T. Wang, V. Narang, R. Abzalimov, N. Wijerathne and R. V. Ulijn, Nat. Nanotechnol., 2016, 11, 960–967 CrossRef CAS PubMed.
- R. J. Williams, A. M. Smith, R. Collins, N. Hodson, A. K. Das and R. V. Ulijn, Nat. Nanotechnol., 2009, 4, 19–24 CrossRef CAS PubMed.
- N. M. Matsumoto, R. P. M. Lafleur, X. Lou, K.-C. Shih, S. P. W. Wijnands, C. Guibert, J. W. A. M. van Rosendaal, I. K. Voets, A. R. A. Palmans, Y. Lin and E. W. Meijer, J. Am. Chem. Soc., 2018, 140, 13308–13316 CrossRef CAS PubMed.
- Y. Dorca, J. Cerdá, J. Aragó, E. Ortí and L. Sánchez, Chem. Mater., 2019, 31, 7024–7032 CrossRef CAS.
- M. A. VandenBerg, J. K. Sahoo, L. Zou, W. McCarthy and M. J. Webber, ACS Nano, 2020, 14, 5491–5505 CrossRef CAS.
- M. Wehner, M. I. S. Röhr, M. Bühler, V. Stepanenko, W. Wagner and F. Würthner, J. Am. Chem. Soc., 2019, 141, 6092–6107 CrossRef CAS PubMed.
- D. Bochicchio, M. Salvalaglio and G. M. Pavan, Nat. Commun., 2017, 8, 147 CrossRef PubMed.
- D. Bochicchio, S. Kwangmettatam, T. Kudernac and G. M. Pavan, ACS Nano, 2019, 13, 4322–4334 CrossRef CAS PubMed.
- P. Gasparotto, D. Bochicchio, M. Ceriotti and G. M. Pavan, J. Phys. Chem. B, 2020, 124, 589–599 CrossRef CAS PubMed.
- R. Freeman, M. Han, Z. Álvarez, J. A. Lewis, J. R. Wester, N. Stephanopoulos, M. T. McClendon, C. Lynsky, J. M. Godbe, H. Sangji, E. Luijten and S. I. Stupp, Science, 2018, 362, 808–813 CrossRef CAS PubMed.
- J. R. Wester, J. A. Lewis, R. Freeman, H. Sai, L. C. Palmer, S. E. Henrich and S. I. Stupp, J. Am. Chem. Soc., 2020, 142, 12216–12225 CrossRef CAS PubMed.
- T. Christoff-Tempesta, Y. Cho, D.-Y. Kim, M. Geri, G. Lamour, A. J. Lew, X. Zuo, W. R. Lindemann and J. H. Ortony, Nat. Nanotechnol., 2021, 16, 447–454 CrossRef CAS.
- S. Zhang, M. A. Greenfield, A. Mata, L. C. Palmer, R. Bitton, J. R. Mantei, C. Aparicio, M. O. de la Cruz and S. I. Stupp, Nat. Mater., 2010, 9, 594–601 CrossRef CAS.
- J. Sankaran and T. Wohland, APL Bioeng., 2020, 4, 020901 CrossRef CAS.
- M. R. Hansen, R. Graf and H. W. Spiess, Chem. Rev., 2016, 116, 1272–1308 CrossRef CAS.
- J. Standfuss, Curr. Opin. Struct. Biol., 2019, 57, 63–71 CrossRef CAS.
|
This journal is © The Royal Society of Chemistry 2021 |
Click here to see how this site uses Cookies. View our privacy policy here.