DOI:
10.1039/D1CS00477H
(Review Article)
Chem. Soc. Rev., 2021,
50, 12013-12036
Chemical data intelligence for sustainable chemistry
Received
19th June 2021
First published on 14th September 2021
Abstract
This study highlights new opportunities for optimal reaction route selection from large chemical databases brought about by the rapid digitalisation of chemical data. The chemical industry requires a transformation towards more sustainable practices, eliminating its dependencies on fossil fuels and limiting its impact on the environment. However, identifying more sustainable process alternatives is, at present, a cumbersome, manual, iterative process, based on chemical intuition and modelling. We give a perspective on methods for automated discovery and assessment of competitive sustainable reaction routes based on renewable or waste feedstocks. Three key areas of transition are outlined and reviewed based on their state-of-the-art as well as bottlenecks: (i) data, (ii) evaluation metrics, and (iii) decision-making. We elucidate their synergies and interfaces since only together these areas can bring about the most benefit. The field of chemical data intelligence offers the opportunity to identify the inherently more sustainable reaction pathways and to identify opportunities for a circular chemical economy. Our review shows that at present the field of data brings about most bottlenecks, such as data completion and data linkage, but also offers the principal opportunity for advancement.
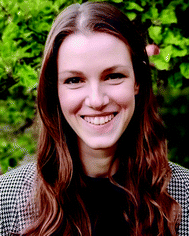
Jana M. Weber
| Jana M. Weber's research focuses on machine learning and complexity science for the development of sustainable process solutions. Jana is currently pursuing her Ph.D. at the Department of Chemical Engineering and Biotechnology at the University of Cambridge (UK). Previously, she studied environmental engineering at RWTH Aachen University (Germany) and Aalto University (Finland). In 2020, Jana was recognized as one of the Top 50 Women in Engineering – Sustainability in the UK. |
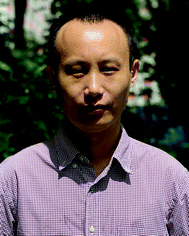
Zhen Guo
| Dr Zhen Guo obtained his Bachelor and Master degree in chemistry in Wuhan University, China, and his PhD in chemical engineering in Nanyang Technological University, Singapore. He focuses on development of in silico solutions for industrial challenges through cheminformatics, data mining, and machine learning. |
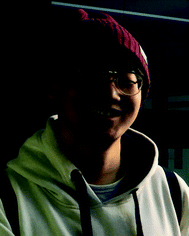
Chonghuan Zhang
| Chonghuan Zhang received his Bachelor's degree in engineering from The University of Sydney (Australia) in 2017 and Master's degree in Chemical Engineering and Biotechnology from University of Cambridge (UK) in 2018. He is currently a PhD candidate at University of Cambridge (UK) and he focuses on applying data informatics to chemistry and synthetic biology. |
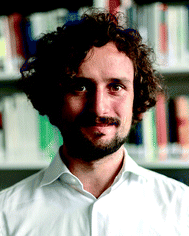
Artur M. Schweidtmann
| Artur M. Schweidtmann is an assistant professor for chemical engineering at Delft University of Technology and co-director of a lab within the TU Delft AI Labs Programme. His research focuses on the combination of artificial intelligence and chemical engineering. He received his Master of Science from RWTH Aachen University in 2017 and defended his Ph.D. from RWTH in 2021, both in Chemical Engineering. During his studies, he spent the academic year 2013/2014 at Carnegie Mellon University as a visiting student via the DAAD ISAP program. He performed his Master thesis at the University of Cambridge. |
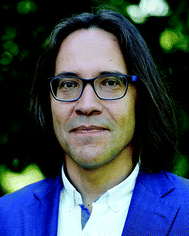
Alexei A. Lapkin
| Alexei Lapkin studied chemistry at Novosibirsk State University and obtained his PhD in Chemical Engineering from the University of Bath. He then held academic positions at Universities of Bath and Warwick, before being elected to his current position of Professor of Sustainable Reaction Engineering @ Cambridge in 2013. His research is focusing on process intensification and developing sustainable chemical technologies supported by artificial intelligence and robotics (lapkingroup.com). He is a co-director of EPSRC Centre for Doctoral Training “Syntech” and a director of ERDF co-funded Innovation Centre in Digital Molecular Technologies “iDMT”. |
1. Introduction
Chemical industries worldwide heavily rely on non-renewable fossil feedstocks, which results in linear economy models, i.e. extract-make-use-dispose schemes.1 This contributes to chemical production becoming the central driver of global oil consumption by the year 2030.2 To tackle the problem, research efforts shifted towards studying the utilization of renewable feedstocks within chemical industries, such as cellulose,3 lignin,4 chitin,5 or bio-wastes.6–8 In recent decades, the scientific community developed new reactions and engineering techniques to produce various value-added chemical compounds from renewable feedstocks.5,9–11 However, one of the main problems when developing sustainable processes is the lack of access to information on all multiple co-existing options, hindering a systematic way to shape early, but key process decisions.12 Novel process routes based on renewable or waste feedstock are in fierce competition with the petrochemical-based market,13–16 where companies operate at economies of scale and have optimised both processes and supply chains for over a century.17,18 Thus, a shift in industrial techniques, if not enforced through strict regulation, can only happen if sustainable alternatives are equally good or even economically superior solutions. Yet, even early-stage schemes of novel reaction routes require process modelling, pre-collected data, and last but not least chemical intuition, making them a long and manual selection process. Thus, there is a need for a systematic and fast tool to identify the most promising reaction routes. The three key aspects to develop such a chemical data intelligence tool are (i) data, (ii) assessment metrics, and (iii) decision-making approaches (see Fig. 1) and will be discussed throughout this study.
 |
| Fig. 1 The three main areas: data, metrics, and decision-making directly influence the search for sustainable reaction pathways in reaction network, but also influence each other. To enable large-scale systematic searches and rankings, automation within and between all areas is key. | |
A systematic picture of the available knowledge on reaction data can be illustrated through a network of chemical reactions, where species are connected with one another through chemical reactions – products and reactants of each reaction are connected. Fig. 2 illustrates how the evolving reaction network can connect feedstock molecules (e.g. from biomass) to target molecules (e.g. drug compounds) over a sequence of reactions involving intermediate molecules (e.g. chemical commodities). The increase in electronic data recordings and thus, data availability, has paved the way for rapid progress on reaction networks mined from large chemical databases, sometimes called the chemical universe or the network of organic chemistry (NOC). Fialkowski et al. first introduced the study of organic synthesis reactions with a network representation based on the Beilstein Database.19 Then, studies on the topology and growth of the network,20–22 synthesis planning through the network,23,24 and applications to One-Pot-Reactions have followed.25 In our previous works, we have highlighted the potential of the NOC for process route selection and for the identification of strategic molecules for sustainable supply chains.26–28 With rapid increases in digitalisation, it is worthwhile to revisit the NOC and identify future avenues for chemical reaction data. Information extraction and information representation play key roles, where tools such as natural language processing (NLP) can lead to more complete datasets and ontological representation, or knowledge graphs, allow machines to better “understand” the data.
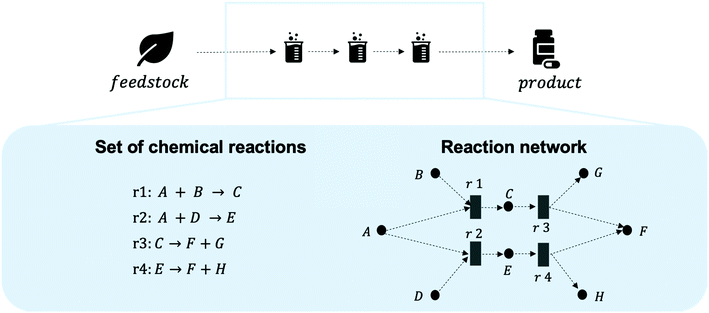 |
| Fig. 2 Reaction networks connect feedstock molecules with target molecules. A sequence of reactions, a reaction route or pathway, is required to connect feedstocks with desired target molecules through intermediate species. Note that an intermediate species is the product of one reaction and the reactant of another in a chemical supply chain, rather than a transient species in a single reaction step. Thus, they are retrievable (as reactant or product) from the database of choice. The best sequence can be identified from large sets of chemical reactions through optimisation of the resulting reaction network. Note that the reaction network is illustrated as a bipartite network here, but multiple other representations are possible. | |
The combination of large sets of chemical reaction data and decision-making algorithms is a prerequisite for fast and systematic assessment of reaction pathways. Reaction Network Flux Analysis (RNFA), which was inspired by the analysis of metabolic networks,29 was first introduced by Voll and Marquardt who identified optimal pathways for bio renewables processing based on a literature review.30 In RNFA, a steady state of the material flows in the system is assumed and the system is optimised for the production of the desired products from available feedstock molecules subject to mass balance constraints.30 The approach was further extended by advanced metrics and supply chain considerations,31,32 to represent process networks,33 and was adopted for the assessment of routes to biopolymers.34 An alternative to the steady state model for reaction network optimisation was presented in ref. 35. Methodologies are evolving quickly, yet their full potential will only be realised if connected to large chemical databases.
To evaluate routes in reaction networks, appropriate criteria must be used. The twelve principles of green chemistry,36 the “productivity scheme”,37,38 and their extension towards green engineering,39 the “improvement scheme”,40 have established a common understanding of environmental considerations in chemical engineering. Metrics, such as the environmental impact factor (E-factor), atom economy (AE), or energy requirements enable us to assess environmental considerations within chemical processes.38 The field is going through a transition from green to sustainable chemistry, which requires the consideration of wider system boundaries. This has been actively discussed and incorporated in life cycle assessment (LCA) literature.41–43 Sustainability criteria should be able to simulate the system boundaries (e.g. demand/supply outside the network) and should be retrievable in an automated manner on early process development stage data sources. Wider chemical reaction systems have previously been analysed based on exergetic efficiencies and sets of chemical heuristics.26
Only together can the areas of data, metrics, and decision-making make the most use of chemical data intelligence and enable practitioners to plan the most sustainable reaction routes. In this work, we explore the potential of semantic data for rich and structured chemical knowledge. Advances in the fields such as NLP and recommendation systems are further reviewed as they promise to tackle the challenge of data scarcity. For sustainability aspects in chemical reactions, we elucidate the importance, as well as challenges, of system thinking. We research a navigation system for chemical space similar to Google Maps, showing us the most sustainable pathways in the entanglement of chemical reactions.
We provide a roadmap with our recommendations for the development of a systematic early-stage sustainability assessment tool in Fig. 3. Within the three research fields, we identify impact opportunities and provide action steps and approximate time frames. The foundation for the recommendations is explained throughout this work in the detailed sections on data, metrics, and decision-making.
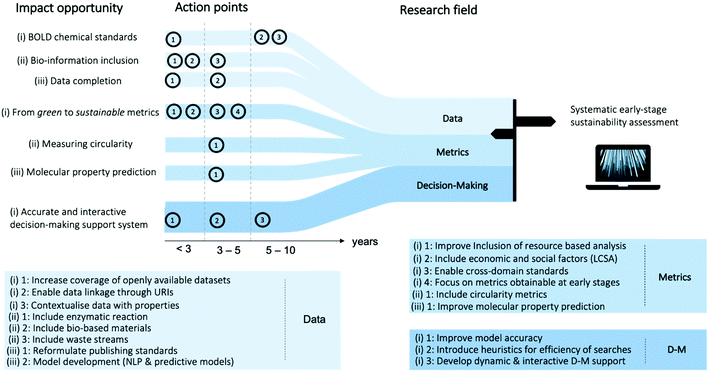 |
| Fig. 3 Roadmap towards systematic early-stage sustainability assessment. Impact opportunities and action points in the three areas data, metrics, and decision-making (d-m) are outlined. Action points are grouped into timeline categories (<3, 3–5, and 5–10 years) and are explained in the boxes below. | |
# 1 Data impact opportunities and action points. The first opportunity is the development of a chemical big open linked data (BOLD) structure. Emphasis lays on the coverage of freely available datasets where single entries are recorded with a uniform resource identifier, enabling linkage to the chemical context and to adjacent fields, such as substance emissions and market values of the molecules. As second impact opportunity, we strongly recommend the inclusion of biological data. This includes molecular transformations from systems biology, purified enzymatic reactions and whole cell transformation, as well as biological feedstocks as primary raw material and as secondary raw material from waste streams. Lastly, we emphasise the importance of complete data structures. While for novel publications, journal standards can enable the recording of stoichiometry, yield, and reaction conditions, the body of already published reactions needs to be revisited in order to withdraw such information in an electronic standard. NLP can gather previously stated information and predictive models can be utilised for the fractions where original sources do not contain the information.
# 2 Metrics impact opportunities and action points. The first opportunity is the transition from green to sustainable metrics. Herein, we recognise the importance to focus on the integration of resource-based assessment, e.g. exergy, which complements emission-based metrics, as well as social and economic assessment. Furthermore, only cross-domain standards, e.g. on allocation and the system boundary, can lead to a successful tool as the chemical sectors spans multiple domains. Last, but not least, we recommend to focus on early-stage metrics, as only early-stage decision-making can lead to inherently sustainable pathways. The second impact opportunity is the measurement of circularity potentials. In order to evaluate the sustainability of a pathway, knowledge on potential uses of waste streams generated throughout the process are indispensable. Considerations on possible upstream treatment and on the stability of molecular properties during multiple cycles of reuse are of interest. The last impact opportunity is the prediction of molecular properties relevant for sustainability assessment, e.g. prediction of chemical exergies from molecular structures.
# 3 Decision-making impact opportunities and action points. Future decision-making systems for early-stage sustainability assessment are required to evolve at two fronts: increasing model accuracy and decreasing model complexity. On the one hand, it is desirable to derive modelling frameworks which take for instance solvents, separations, upstream treatment, material circularity, and sequential manufacturing into account. On the other hand, linear models or heuristic, solving strategies for non-linear models, are essential to facilitate large-scale, and thus, systematic assessment. Last, but not least, sustainability is a dynamic systems problem, which requires a decision-making support able to interact with the dynamic nature of the system. While metrics might be able to map current market prices and data can show the demand for or supply of certain materials at regarded geographic locations, the envisioned decision-making framework should work beyond these static snapshots of the system, e.g. following agent-based modelling approaches.
Enabling the three areas to evolve together will be the key aspect for well-reasoned reaction pathway development, tackling the different aspects of sustainability. We would like to stress the following interfaces between the areas in particular.
# Interface 1: data is the foundation for metrics and decision-making. Accessible and well-documented databases are a prerequisite to further development of metrics. Molecular properties such as chemical exergy, thermodynamic properties or toxicity values are key aspects of sustainability assessment. Further development of computational tools to automatically generate metrics for given systems are needed. Additionally, data on reaction structures, i.e. the stoichiometric relationships, are essential to formulate mass balances as physical constraints of the decision-making formalism. We anticipate that only clear communication of data needs from the metrics and decision-making community will enable sufficiently quick extensions to the current data sources to be developed. Alongside, conversations between the communities should include ontology development to define the characterisation and relationships of data.
# Interface 2: co-development of metrics and decision-making. Throughout this article, we argue that sustainability is a systems science. For a future sustainable chemical supply chain, it is essential to evaluate proposed reactions within their environment, rather than detached from it. Thus, the metrics required to assess the sustainability of novel reaction pathways need to capture wider system interactions considered in the decision-making approach. Here, the foundations of assessing the greenness or the sustainability of reactions are provided by the metrics community, but new requirements for metrics will arise from within the systems modelling community. We anticipate large benefits if both domains come together.
# Interface 3: defining decision-making environments through regional and dynamic data. Data on the price of molecules illustrates the economic interaction of a system with its environment across the system's boundary. This interaction is further defined by data on the availability and the demand of each molecule, thus mapping the chemical supply chain. However, market prices and supply chains as well as the energy price are temporal and spatially fluctuating. In the long term, modelling novel reaction pathways within a circular chemical supply chain requires dynamic interactive environment descriptions, rather than static snapshots. Here, joint research is needed to develop dynamic environments based on suitable data sources. Future advanced tools may even include regional policy insights, as well as costs associated to infrastructure and personal.
2. Data
Large volumes of open and big data have revolutionised many fields of our modern society.44 While enormous improvements have driven developments in areas such as computer vision, or language recognition, chemical data has yet to overcome urgent challenges, such as establishing openly accessible data with standardised representations and improving quality inconsistencies of existing data.45 FAIR scientific data – findable, accessible, interpretable, and reusable46 – evolving into BOLD concepts47–49 will push chemical discovery and is essential for cross-disciplinary tasks, such as sustainability assessments.
Databases and accessibility
To facilitate large-scale reaction route screening, access to large reaction databases is the stepping stone. When aiming for more sustainable process routes, it is worthwhile to discuss the extraction of reactions from conventional chemical transformation as well as biosynthetic conversion strategies and alternative chemical conversion strategies.
Conventional chemical reaction databases.
There exists a variety of chemical reaction databases with different sizes and accessibility rights as well as distinct coverage of the chemical space. Table 1 outlines a selection of common databases for organic reactions. CASREACT† and ReaxysTM‡ (in the following called Reaxys) are by far the largest databases for chemical reactions. They include scientific literature and a selection of patents, but require users to buy licenses to work with large-scale data. The database called Pistachio§ developed by the company NextMove stores reaction from US patents and has released a publicly available subset CC-Zero¶ of over one million reactions. SPRESI|| is another database for organic reactions, which provides a subset of 500
000 reactions as a free app. The USPTO** database is the smallest, yet it is entirely openly accessible. Open reaction databases are gaining increasing momentum. One recent example is the open reaction database,†† which is a multi-institution initiative to aid machine learning (ML) tasks in chemistry/chemical engineering by providing structured and freely available reaction data. The project sits on GitHub and its launch is planned for early 2021. Regarding data coverage, Thakkar et al. have outlined the differences in data coverage from multiple sources including a dataset based on electronic notebooks from AstraZeneca.50 They studied reaction templates within different databases and found that only 2% of templates were common in all considered data sources.50 Notably, the development of chemical databases is a rapidly developing field. Content breadth and depth are being constantly reviewed and further developed. Reaxys, for instance, now covers supplier information on price, supplier geolocation, packages sizes and much more.
Table 1 Selection of large databases recording chemical reactions
Database |
Size |
Accessibility |
CASREACT |
>128 million reactions |
Proprietary |
Reaxys |
>46 million reactions |
Proprietary |
Pistachio |
>9 million, patent literature based |
Proprietary |
SPRESI |
>4.6 million reactions |
Proprietary |
USPTO |
>3.3 million, patent literature based |
Open |
Biosynthetic reaction databases.
A hybrid system of biosynthetic and conventional chemical synthesis opens up opportunities for efficient (bio)chemical pathways search. Enzymatic reactions can lead to more efficient reaction pathways with reduced operational costs as synthetic biology can enable shortcuts and flexible design of supply chains (see Fig. 4), improving redox efficiency. Moderate temperatures/pressure and the avoidance of metal catalysts or hazardous solvents can ease synthesis and lower operational costs.51 Additionally, enzymatic reactions are well suited to utilise and further functionalise the biological structures in renewable feedstock. Advantages of metabolic alternatives have encouraged synthetic biologists to find biosynthetic routes that produce bulk chemicals and industrial chemicals such as ethanol,52 benzoic acid,53 toluene,54etc., and active pharmaceutical ingredients of pharmaceuticals such as flavonoid55 and tryptophan.56 A metabolic map for the production of bio-based chemicals was summarised by Lee et al.57
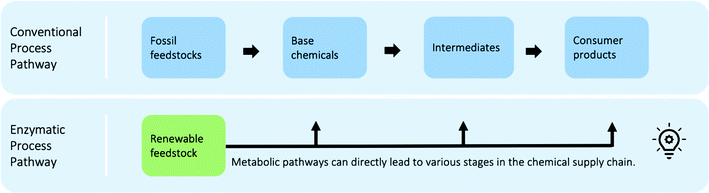 |
| Fig. 4 Illustration of enzymatic process pathways and conventional process pathways based on fossil feedstocks. In conventional processes, feedstocks are first broken into smaller building blocks and then reassembled and functionalised step by step. Renewable feedstock, however, is often already highly functionalised and different enzymatic transformations can make use of this for direct transformation into different stages of the conventional supply chain. | |
Databases such as Kyoto Encyclopaedia of Genes and Genomes (KEGG),58
‡‡ Rhea,59
§§ and the Enzyme Catalytic-mechanism Database60
¶¶ open up opportunities to obtain bio-information for metabolic reaction networks. The most comprehensive biological database is KEGG with currently almost 13
000 recorded reactions.58 However, in comparison to conventional chemical databases, enzymatic databases are relatively small and sparse at present. Synthetic biologists are actively working towards the prediction of metabolic reaction behaviours to populate the databases.61,62
Alternative conversion strategies.
Further opportunities for discovering new reaction pathways include electrochemical or photochemical transformations. Electrocatalytic hydrogenations may hydrogenate molecules in water under ambient conditions and thus replace conventional hydrogenation steps typically requiring elevated pressures.63 Besides, electrochemistry may also unlock entirely new synthetic pathways as novel molecular transformations are observed.64 Harnisch and Urban illustrate the concept of an electrobiorefinery, where they anticipate that the synergies between microbial and electrochemical conversations are likely to impact, amongst others, enlarging product portfolios and exploiting new feedstocks.65 In particular, they outline electrochemistry for decomposition of bio-based feedstocks, e.g. lignin pretreatment, to provide chemical feedstocks, e.g. H2, CH4, or C1- or C2-compounds, but also to electrochemically steer fermentation, e.g. CO2 can be used as carbon source for fermentation cultures.65 Another promising conversion strategy is photochemistry. Research efforts are focusing, for instance, on the utilisation of sunlight to produce CO, ethanol, or methane from CO2 in aqueous solutions, or on solar-driven organic synthesis, where the target is to obtain high-value products.66 He and Janáky state that utilising solar energy and CO2 resources can be expected to yield both fuels and value-added chemicals. They list a range of possible chemical products in their work and compare the performance of different photochemical conversion strategies.67 In large chemical databases, such as Reaxys, one can specify reaction types, also including electro- or photochemical transformations. This allows specific inclusion of alternative conversion strategies and potentially enlarges the toolbox for the development of more sustainable chemical reaction pathways.
Data formats
Linking data sources is often essential for decision-making, but requires rethinking of existing data practices.68 A stepwise approach for data formats, the 5-star plan|||| (cf.Fig. 5), was suggested by Tim Berners-Lee, the inventor of the worldwide web. The plan describes a trajectory for data formats starting by open data in any format and resulting in the semantic web.69 In the semantic web, data is accessible both for humans and machines as data is stored with structure and context, generating meaningful content. The content is made comparable between sources through ontologies.69 Ontologies exploit triple relationships, e.g. Acetone 〈is used as〉 solvent is broken into two concepts “molecule” and “solvent” and their relationship is given by 〈is used as〉. These generate metadata structures, i.e. reusable knowledge representation.70 Additional terminology for data structures is explained in Table 2.
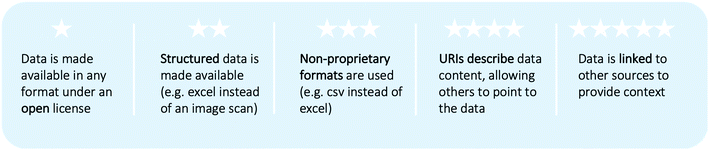 |
| Fig. 5 5-Star plan for open data suggested by Tim Berners-Lee (based on https://5stardata.info/en/). | |
Table 2 Explanation of specific terminology in data representations
Term |
Description |
Knowledge graph |
A knowledge graph uses data in graph structure. Data entities and their semantic types and properties are linked with each other. Knowledge graphs can allow machines and humans to reason from the data. |
Metadata |
Metadata is data about data. One example is structural metadata, which provides schemes and order to data. |
Ontology |
An ontology provides a uniform approach to describe data semantically. It is a specific conceptualization in a format that allows for reasoning and inference.79 |
Semantics |
Semantics provide methods to include meaning to information constructs. Adding meaningful tags to pieces of data brings about better readability as it is an abstraction of what the stored piece of data resembles in the real world. |
Semantic Web |
Tim Berners-Lee, who is the inventor of the World Wide Web, defines the Semantic Web not as a separate one, but as an extension of it. His vision is a web of information which can be processed by computers and which brings structure to the meaningful content of web pages.69 |
Unified resource identifier |
Unified resource identifiers are used to identify any object, e.g. people, books, or places, in web technologies. In semantic web technologies, they help linking objects from different sources. |
Scientific practices to record data openly and in machine-readable formats lack behind. Large chemical databases such as CAS*** are fundamental sources of chemical information, however, they do not fulfil the requirements of the semantic web when it comes to information access and representation.70 This is due to current ways of publishing, e.g. providing PDF files, which support human readability, but are ill-suited for data mining and analysis.68,70 The project open research knowledge graph has made it their mission to change the document-centric information flow in the scientific community into knowledge-based information flows.71–73 The final product is envisioned to be a “structured, interlinked and semantically rich knowledge graph”.73
Chemical data often lacks relational information and is stored in diverse formats. For example, molecule representations range from structural chemical formulas over numeric descriptors to string representations, e.g. SMILES or SMARTS. The chemical mark-up language was introduced to offer semantics for chemical data.74 It allows the integration of various entities, e.g. molecules, spectra, and reactions in mark-up text for electronic use. Adding relationships to the entities, an ontological structure of information emerges. A few early ontologies for chemical engineering have been developed.75,76 OntoCAPE, for example, defines an ontology for chemical processes.77,78 OntoCAPE introduces chemical primitives such as system property, physical dimensions or units to define a system. System properties can have numerous values. The authors illustrate this through the system property “temperature” where different values can be distinguished from each other by the system property “time” both given in their respective units, here degrees Kelvin and time in hours, to record a temperature profile over time.77 The extension of such formalisms to broader domains of chemical applications brings about the potential to gather chemical data in a structured and standardised way even across different subject areas.
Linked data is much needed for sustainability assessment as it allows for holistic and cross-disciplinary assessment.79 The development of knowledge graph technologies potentially enables efficient data handling, including conditional data queries within or between subject areas, and more accurate data inference, due to high contextualising. The group of Prof. Kraft optimises an eco-industrial park, integrating water networks, waste streams, and energy links, to minimise environmental impacts through semantic web technologies.75,80–82 Similar structures of semantic web will be essential to enable sustainability assessment within reaction networks, where temperatures, yields, solvents, and reaction stoichiometry should be recorded and linked with each reaction.83 An entity-based data format would allow us to link further information (e.g. data on waste streams and their compositions, or on regional availabilities of renewable energy) in a modular way, paving the way for more holistic considerations in future reaction planning.
Data completion
While text-mining has enabled gathering large-scale chemical information, such as properties and structures of molecules, connections between reactants and products, to populate electronic chemical databases (cf. Section Databases and accessibility), the methods used in creating the datasets sometimes lack accuracy and miss-classify important pieces of information. Missing stoichiometric data and incorrect recording of multi-step reactions as single-step reactions prevents mass balances within a reaction to be calculated and are major hurdles for decision-making based on automatically generated process options. Furthermore, records of reagents, solvents and catalysts are often inconsistent, e.g. they are sometimes absent or incorrectly recorded as a reactant and no information about required quantities is provided. This is challenging for sustainability assessments because the use of reagents, solvents, and catalysts has a significant influence on environmental impacts.84 Additionally, in some cases, clear identification of specific chemical species within the databases is problematic as mixtures of enantiomers are recorded as pure compounds or entries simply state “mixture out of C3 to C6 hydrocarbons”, without reference to their molecular structures or a database registry numbers. The same problem exists in the identification of complex feedstocks without exact structure and composition, such as lignin, chitin, or cellulose. Inconsistency in temperature and pressure recordings makes an energetic analysis of processes difficult. With rapid developments in the field of NLP and high throughput experiments (HTE), it is expected that data quality will quickly improve and some of these current hurdles for algorithmic use of chemical information will be overcome.
Information extraction from scientific literature.
NLP describes a range of computational techniques to analyse and represent natural text in a human-like manner for a variety of applications.85 One application especially relevant to this review is information extraction, the task of gaining structured knowledge from text. NLP aims (i) to aid human–human communication, e.g. translation tasks, (ii) human–machine communication, e.g. conversational agents such as Apple's Siri, or (iii) to bring about benefit for machines and humans, e.g. through learning from large amounts of data.86 According to Hirschberg and Manning, NLP has seen an immense boost within the last few years due to: an increase in computational power, large availability of linguistic data, successful ML algorithms, e.g. the transformer model,87 and a better understanding of human languages.86 Information extraction from scientific literature brings about great benefits, not only to fill in gaps in data but also to keep track of the ever growing body of literature.86
The last decade has shown immense progress of NLP techniques within chemistry and related fields, making it a promising avenue to overcome data completion tasks. Jessop et al. have developed the Open-Source Chemistry Analysis Routines (OSCAR) software to read entities and information in chemistry publications.88 OSCAR4, a library onto which text-mining tools for chemistry can be built, was released and the authors illustrated that OSCAR may also be applied to other areas of physical sciences as customisation through different dictionaries is possible.88 Such transdisciplinary data systems are of increasing importance as sustainability assessment requires a variety of data. Krallinger et al. present a review on the access of chemical information through text mining techniques and especially value chemical entity recognition as well as the interlinkage to biological data.89 NLP techniques have also gained a foothold in related fields, such as nanotechnology,90 medical/clinical text documents,91 and biomedical texts.92 However, linking data from scientific publications of different chemical subject areas is potentially problematic as definitions and reporting standards may vary. Automated assessment frameworks of data quality for linked open data93,94 may offer the potential to identify pieces of misleading information between communities.
Data acquisition through high-throughput experiments.
In HTE multiple reactions are performed in parallel to quickly answer a specific chemical question.95 High-throughput virtual experiments (HTVE) are often utilised to examine material or drug leads at a large scale, when experimental searching becomes impractical due to high cost or technical issues. They are commonly used in combination; experimental data can be used to calibrate HTVE, while results from HTVE guide further experimental explorations.96–98 This iterative approach has proven to be very powerful, especially when combined with ML guided exploration algorithms.95,99 Eyke et al. employed data produced by HTE to train a ML model, and the ML model predicted reaction outcomes and selected the most informative experimental region for HTE to explore further.96 Chen and Visco built a support vector machine (SVM) model on the basis of experimental data and molecular descriptors, which they trained for the identification of drug candidates98 and Li et al. demonstrated that artificial neural networks (ANNs) trained on density functional theory (DFT) data were able to capture complex absorbate–metal interaction, providing guidance on the design of bimetallic catalysts.100
To further accelerate data collection speed, robotic platforms for chemical experiments have become the focus of many studies.101–104 For instance in 2004, King et al. reported an automatic experimental system called “robot scientist” that was able to independently conduct an entire research cycle, including planning, testing, analysis and re-run if hypothesis and results were inconsistent.101 In the platform assembled by Coley et al., synthetic routes were proposed by a retrosynthesis software and organic synthesis was conducted in flow reactors, automatically configured by a robotic arm.104 In Cronin's group, a robotic platform was constructed along with a standardized architecture for organic synthesis, called the Chemputer.105 By taking advantage of various smart hardware and programming languages, Chemputer system showed the potential to standardize the whole automatic experimental process, from conducting synthesis to generating reports.
Advanced data analysis techniques, such as the transformer-based model developed by Schwaller et al., perform well over data from HTE, but are still not feasible for processing historical experimental data, which often suffers from high inconsistencies.106 With the continuous improvements in HTE, HTVE, automation, and data analysis, highly consistent and reliable experimental data may quickly expand, bringing to light more reliable data standards in larger regions of the chemical space.
Data inference
Some data is never reported in primary research publications or patents, but data may be augmented through data inference – a cheap and fast alternative to experimental studies. In this section, we first outline recommender systems as a general way to complete data matrixes and then highlight developments for data inference of the specific contents as illustrated in Fig. 6. Relevant for reaction route search are: firstly, missing reaction conditions, e.g. temperature, pressure, and reagents, secondly, reaction outcomes, e.g. yield is not always available and thirdly, reaction structures; e.g. reactants and/or products and the reaction stoichiometry are missing.
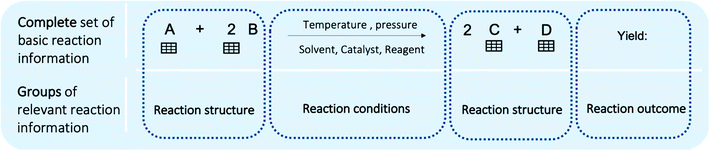 |
| Fig. 6 Illustration of data completion issues for basic chemical reaction information. A set of necessary reaction information for early-stage assessment is shown in the top line. Relevant pieces of information are grouped into reaction structure, reaction conditions, and reaction outcomes in the second line. A, B, C, and D represent molecules and the table below represents structural information of the molecules. Temperatures and pressures can be named either as explicit numbers, as ranges or referred to as ambient. Solvents, catalysts, and reagents are often named; however, their quantity remains unknown. Note that a combination of these issues is most often the case. | |
Recommender systems.
Recommender systems have been an effective approach to deal with information overload and are seen most promising in problems related to “over-choice” of options. A standard recommender system solves a problem of a set of n users and m items, which it generally recommends to the users according to their preference. The relationships between users and items are commonly represented in a n × m matrix, being the core element of the recommender system.107 The matrices are often very sparse as little information is originally available and the aim is to predict what the missing cells will be.107 The entries may range from single bits to unstructured text.108 One prominent example of a recommender system has been the Netflix challenge, where a price of one million US$ was awarded to the team to first model the dataset and predict new ratings to a specified accuracy.109 Matrix completion methods or graph recommender systems have been applied for such problems.107,110,111 For a deeper understanding of recommender systems and their current trends and challenges as well as the underlying deep-learning strategies we refer the reader to these surveys.107,112,113
Within the domain of chemistry, there are early works that recognise the impact of recommender systems for both experimental and computational data. Savage et al. recommend candidate molecules as reactants for the synthesis of desired products. They formulate the problem as link prediction over a graph base, where links represent reactant–product relationships and provide chemical knowledge in the form of molecular fingerprints.114 In 2020, Jirasek et al. have shown an application of a recommender system for the prediction of binary activity coefficients.115 Other examples within chemical engineering include the use of recommender systems to predict drug side effects,116 to estimate the relevance of chemical compounds to form crystals,117 and for material choices in polymerisation experiments.118
Inference of reaction outcomes.
For reaction outcomes, we focus on the inference for yield data. At present, yield records are far from sufficient in most databases. For instance, an exemplary dataset from Reaxys database with 17 million reaction records contains around nine million reactions without any yield information. Additionally, among reaction records with yield information, the yields of only a few products are listed.
Data-driven approaches are promising avenues for yield predictions. Through advances in HTE techniques, detailed and structured experimental data became more easily available. Simultaneously, ML methods evolved and machine-readable representation of molecules and reactions is under constant development. One of the earliest approaches of ML for yield prediction was presented by Kito et al. and predicted the selectivity of catalytic oxidative dehydrogenation reaction products by using an ANN.119
Two trends emerged for yield prediction afterwards. On the one hand, models are based on more accurate, but expensive inputs through descriptors based on DFT and focus on specific reaction types, on the other hand, models aim to identify more generic relationships for multiple reaction types based on cheaper molecular representations. DFT-based descriptors were employed by Yada et al. for tungsten-catalyzed epoxidation of alkenes by using a linear function ensembled in a logistic regression model,120 by Estrada et al. for palladium-catalyzed Buchwald–Hartwig cross-coupling reactions,121 and by Fu et al. to predict yields of Pd-catalyzed Suzuki–Miyaura reactions in the microfluidic system.122 While Yada et al. only trained their model on 14 data points, Estrada et al. worked with a set of 4140 reaction results with the aid of high-throughput screening.
Eyke et al. utilised reaction fingerprints by concatenation of Morgan fingerprints to guide their experimental design for two specific types of reactions through an ANN.96 Sandfort et al. present a broader approach through their structural-based platform for reactivity prediction in organic chemistry.123 The idea was to use molecular fingerprints as the only type of inputs for ML models to solve all kinds of reaction predictions. While no universally applicable fingerprints for all applications were found, only focusing on C–N cross-coupling reactions, a comparable accuracy to the work of Estrada et al. was achieved. Skoraczyński et al. showed reaction examples where subtle changes in molecular structure or reaction conditions led to distinct reaction results, and thus, argued that general descriptors for diverse sets of organic reactions are difficult to set.124 A very recent development in the area is an algorithm based on NLP.106 Their model consists of an encoder and a regression layer to predict yields and is based on reaction SMILES as inputs. On a dataset based on HTE reactions for Buchwald–Hartwig reactions and Suzuki–Miyaura reactions a high prediction accuracy was achieved, while for a generic dataset, the open-source USPTO, poorer accuracy was obtained. While the prediction of accurate yields for diverse reaction types remains a challenge at present, the new methods bring about promising outlooks.
Inference of reaction conditions.
Methods for prediction of reaction conditions, also known as reaction context,125 have evolved from specific methods only valid for certain reaction types and reaction context towards more holistic approaches. The reaction context is made out of discrete decisions, e.g. catalyst and solvents, and continuous decisions, e.g. temperature, pressure, and pH-value, which influence one another. Marcou et al. predicted catalysts and solvents for the Michael reaction through formulating binary classification problems on a set of 198 reactions.126 The prediction of both catalysts and solvents was correct for only eight out of 52 reactions from an external validation set.126 Lin et al. focused on catalyst recommendations for deprotecting reactions and demonstrate their work on catalytic hydrogenation reactions.127 They used the methodology of condensed graph of reactions to reduce a reaction to a single graph, allowing for descriptors and fingerprints, and employed similarity searches to suggest catalysts.127 Gao et al. also recognises such approaches based on chemical similarity, however highlight computational costs in sufficiently large databases.128 Segler and Waller aim for conditions recommendations for different types of reactions utilising a knowledge graph.129 The graph consists of two node types (i.e. reactions and molecules) and a variety of edge types, describing the reaction conditions, e.g. 〈is reactant in〉, 〈is catalyst in〉, 〈is solvent in〉. New conditions are predicted through node and link completion tasks.129 The group by Jensen also aimed for a generic model to predict reaction context, here catalysts, solvents, reagents, and temperature.128 They trained an ANN on a dataset of about 10 million single-step and single-product reactions and allowed for hierarchical ANN structures which take interdependencies between the reaction contexts into account. In 69.6% of the time, a close match to the recorded conditions is found within the top 10 prediction outcomes.128 Also, the optimal selection of reaction conditions can be solved as an inverse design problem by using an optimisation algorithm to change the inputs of an reaction outcome model.
Inference of reaction structures.
To evaluate reaction synthetic routes, masses of products, reactants, and side-products need to be quantified. These can only be computed when all substances and their stoichiometric coefficients are known. However, three main aspects of current data recording hinder this analysis: (i) stoichiometric coefficients are lacking, (ii) reaction co-participants are missing, and (iii) multiple reaction steps are integrated into a single reaction entry.
In the current literature, there exists a limited number of methods for reaction structure completion. Firstly, reaction templates can be utilised. Grzybowski et al. manually curated around 100
000 reaction rules with complete understanding of reaction participants and stoichiometry.24,25 Their templates are now linked with the commercial software SYNTHIA††† and can guide retrosynthesis and analyse carbon efficiency based on mass conservation.130 However, manual curation of reaction rules is far away from exploring the entire chemical space. Secondly, atom mapping, which relies on the rearrangement of atoms in chemical transformations, is promising to tackle this problem. The completion of an exemplary reaction is shown in Fig. 7, where in (a) stoichiometric coefficients are absent and mass balances are not obeyed and in (b) atom mapping describes the exact transformation and reveals the missing species on the product side. The existing atom mapping methods, described in recent reviews,131,132 often convert molecules into graphs and compare the most common subgraphs. However, this results in an NP-hard problem where computational time increases exponentially with the number of atoms in molecules. Jaworski et al. utilise graph-theoretical considerations and chose 20 chemical rules/heuristics to correct mapping of reactions.133 This method attempts to complete the stoichiometry, firstly, by adding small molecules such as acetaldehyde, ammonia, and others to balance the reactions and, secondly, by fitting reactions into popular reaction templates and adding the missing parts. Only if such attempts fail, atom mapping is employed. The work by Schwaller et al. utilised NLP to infer reaction structures.134 A neural network (transformer) was trained on a set of mapped reactions and showed to be able to complete the mapping task quicker and with confidence scores.134 Despite the aforementioned improvements in atom mapping, inferring complete reaction structure remains a challenge at present as a precise prediction of functional transformations is required.
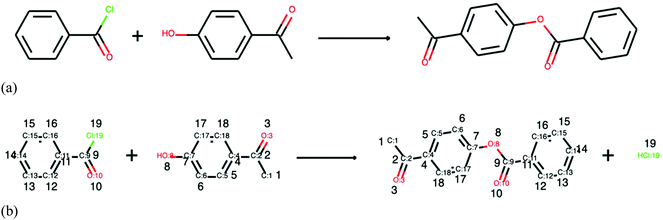 |
| Fig. 7 The principle of atom mapping and how it can aid in the completion of reaction structures is outlined. The sample reaction is retrieved from Reaxys with Reaxys reaction ID: 615722. In (a) participants and stoichiometric coefficients are missing and in (b) the reaction is balanced with help species HCl and stoichiometry coefficients. The recording structure from C:1 to Cl:19 denotes the type of atom and its identifier. | |
Notably, computational capacities have increased immensely over the last few decades, and new computational approaches, such as graphics processing units (GPU) or quantum computing, are promising avenues for complex computational tasks. GPU computing has enormously advanced the field of deep learning in the areas such as computer vision and speech recognition.135 Quantum computing has shown to speed different search algorithms136 and its potential for complex optimisation tasks, such as energy system optimisation, has recently been highlighted.137 However, it has been argued that the power of quantum computing is limited, especially when it comes to NP-hard problems.138 Nevertheless, new computing approaches provide promising avenues for computationally expensive algorithms such as large scale atom mapping challenges.
Besides said data-driven techniques to infer missing species and stoichiometry, it is worthwhile to discuss the automated generation of entire reaction networks based on chemical rules, which also leads to stoichiometric relationships of reaction networks.139,140 For instance, the rule network input generator (RING) can construct complex networks based on a set of input reactants in their SMILES representation and a set of defined reaction rules and constraints.139,140 RING showed to reproduce mechanisms reported in the literature for systems such as dehydration of fructose to produce HMF or acid-catalysed hydrolysis of HMF to levulinic acid.139 Most notably, Marvin et al. combine the network generation method RING with a mixed-integer linear programming (MILP) model for the optimisation of pathways towards biofuel-gasoline blends.141
To generate reaction structures for large-scale networks, encoding all permitted reaction rules and constraints may be tiresome, as theoretically literature and databases can easily provide this information. However, rule-based network generation can be of particular interest in sparse regions of chemical knowledge. Here, methods such as RING can significantly contribute.
With all types of prediction algorithms, it is worthwhile to keep the stochastic nature of the results in mind. While information from the literature often contains measurement uncertainties, introducing predictive algorithms adds model uncertainties. In Section 4 on decision-making, we shortly sketch the influence of uncertainties on finding optimal solutions.
3. Metrics
The assessment of sustainability through metric values is not trivial. As a system challenge, it calls for use of large data sets. At the same time, it is strongly biased by our subjective view of the dynamic concept of sustainability. Metrics to assess sustainability are diverse and difficult to set. Within the United Nations framework of the Sustainable Development Goals (SDGs), there exist 231 unique indicators to measure sustainability in the 17 dimensions of the SDGs. This illustrates the necessary diversity in dimensions and indicators utilised to assess sustainability. Additionally, strong interconnectivity between dimensions has been acknowledged by many,142–146 possibly leading to synergetic effects, but also to trade-offs.143,144
There are limited possibilities to measure the sustainability of reaction routes to such extent as for instance the SDGs would indicate. Yet, the transition from green to sustainable chemistry has identified key aspects which should be considered. While the well-established principles of green chemistry36,38,147,148 and green engineering37–39 lead the way towards more environmental-friendly practices, sustainable chemistry requires the expansion of system boundaries.149–152 On the reaction network level, this means considering entire supply chains as well as moving away from purely environmental concerns towards implicit inclusion of societal and economic ones, their trade-offs, and synergies. The European Technology Platform for Sustainable Chemistry, SusChem,‡‡‡ focuses on projects which combine all three sectors, and the International Sustainable Chemistry Collaborative Centre, ISC3,§§§ highlights inter alia systems thinking, ethical and social responsibility, and circularity as key characteristics for chemists to focus on.153
Systems thinking
In the search for a more sustainable future, systems thinking and systems modelling are powerful tools. A system can be defined as a whole made out of interlinked, possibly nested, subsystems.154 Sustainability is often referred as a system which sustains itself, making the notion of systems thinking even more relevant for the discussion of sustainability assessment. In the following, we will visit the importance of system boundaries with regard to LCA and to circularity.
System boundaries and life cycle assessment.
System boundaries describe the interfaces between the system and its exterior, the environment. The work by Nabavi et al. describes how system boundaries influence the assessment of sustainability aspects in dynamic systems.155 While system dynamic models should have a broad boundary including all variables considered important,156 it is essential to set boundaries somewhere for practical reasons.155 However, the implications of having set these exact boundaries will play an important role throughout the entire modelling process, in particular when dealing with complex sustainability considerations.
Modelling chemical reactions from a systems perspective also strongly relies on the chosen boundaries. LCA is a common systems-based method to quantify environmental impacts on life cycle inventories.41–43 In LCA, a functional unit, e.g. one kg of the desired product, is taken as reference, and boundaries are drawn, e.g. cradle–gate–grave, often as wide as possible to follow the materials and energy flows. Associated with these are the environmental burdens which can be summed up for impact categories, such as the cumulative energy demand (CED), the global warming potential (GWP), human toxicity, or land use. Social or socio-economic life cycle assessment (S-LCA) incorporates social indicators and describes impacts such as working hours and local employment.157,158 LCA requires the specification of a system boundary on various levels, the ultimate one being the one between nature and the technical system.159 The scope of investigation requires further boundaries as the temporal and the geographic dimensions. Additionally, boundaries are chosen when deciding for metrics of interest (will impacts on aquatic life be within or outside the system boundary?). The tool named “strategic life cycle management” utilises sustainability principles as system boundaries and aims to provide an even wider overview.160
A reaction network is a subsystem, which is in exchange with its environment over a system boundary, see Fig. 8. Decision-making requires the assignment of assessment aspects to flows that are in exchange with the environment, e.g. what are the monetary values of mass flows, or what is the availability or the demand of mass flows in the geographic context. The choice of system boundary therewith strongly frames the problem and assessment aspects at the system boundary, e.g. how useful, toxic, expensive streams crossing the boundary are, strongly impact the results of any study. As of now, it is difficult to associate these aspects to large quantities of molecules as necessary for large-scale network data. However, semantic web and knowledge graph technologies are envisioned for scientific and chemical data. They can lead the community towards a future where assessment may be easily associated with a large diversity of chemical species.
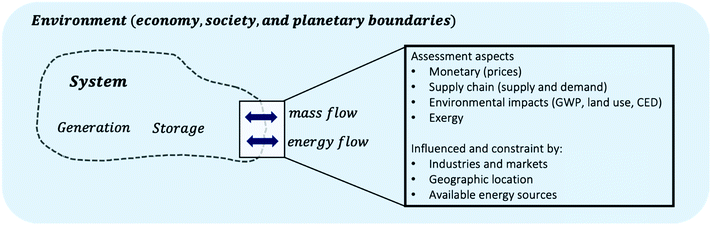 |
| Fig. 8 Exchange of mass and energy at the system boundary from reaction networks (systems) and the economy, society and planetary boundaries. Mass and energy exchange with the environment can be assessed in multiple dimensions, e.g. the value of a mass flow leaving the system can be determined based on its monetary value, the demand for it or its environmental impact. Assessment criteria are influenced by the wider environment, e.g. energy markets and availability of renewable sources vary at different geographic locations and in time. | |
At present, a chemical reaction network is commonly built based on: (i) one or more feedstock molecules of interest, and (ii) one or more product molecules of interest. Reactions connecting feedstock(s) and product(s) are then introduced manually from literature review or automatically from electronic databases or reaction generators, sometimes constrained by a maximum size of reaction steps. Some queries are open-ended on the product or the feedstock side, allowing queries such as, which is the best feedstock to produce product X, or which valuable products can be produced from feedstock Y. The system boundary can now be further specified, e.g. which species can be exchanged with the environment. Assessment metrics describe the exchange at the system boundary.
System boundaries and circular economy.
Nowadays, many industries strive for different levels of circularity of their supply chains.161,162 By industrial symbiosis companies can exchange material flows, allowing by-products from one industrial process to become the feedstock of another and thereby closing material loops.163 In contrast to closing the loop through technology, biodegradable products close the biological loop.163
Including circularity in system modelling requires careful evaluation of system boundaries. Fig. 9 outlines that the aspects of circularity may lay within the system boundary, e.g. recycle streams within multi-step reactions, or may lay outside of current system boundary, e.g. similarly to BASF's Verbund system¶¶¶ companies or geographical regions can exchange material flows. While internal system circularity, see Fig. 9 (left), influences the necessary exchange of flow quantities, external circularity, see Fig. 9 (right), influences the assessment metrics. Utilising a material as system input, which is an output from another system can contribute to the overall reduction of waste and minimisation of raw material use, e.g. substituting for either fossil or renewable feedstocks. This should be taken into account when evaluation reaction pathways.
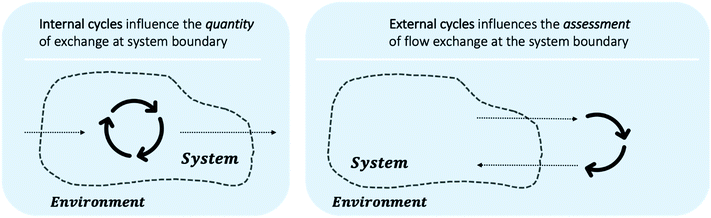 |
| Fig. 9 Circularity within one system (left) and between multiple systems (right). Circularity within one system affects the flow quantity exchanged at the system boundary, e.g. less solvent is needed as input and generated as output if solvent recovery takes place. Circularity between multiple systems should affect the assessment of exchanged flow quantity, e.g. output flows that can be used at input flows for other processes should be preferred over waste output flows. | |
Molecular circularity indicators are required to inform on alternative utilisation possibilities. The Ellen McArthur Foundation has shaped the discussion on circularity indicators, introducing the material circularity indicator to aid assessing material flows both at product and at company level164 and its tool Circulytics,|||||| which measures circularity for businesses. Additionally, the World Business Council for Sustainable Development has introduced the circular transition indicators as quantitative framework to measure sustainability for businesses. With respect to material circularity in chemical engineering, Razza et al. provide metrics for biobased and biodegradable products, emphasising the biological cycle (extraction of renewable feedstock – use – composting or biodegradation in soil) in contrast to technological recycle steps.165 Most notable, Lokesh et al. have extended common green chemistry metrics towards capturing circularity aspects.166 We note the need of a waste stream database, which records large waste streams over various industries and maps functional molecules and pretreatment to the streams, allowing circularity assessment of chemical loops.
The knowledge on the demand and supply (the usefulness) of material streams across the boundary will allow for the design of circular chemical solutions. For example, in view of a circular chemical supply chain, it is impossible to measure and compare the circularity of two competing reaction pathway options without considering their respective environments. One reaction pathway may seem less competitive due to a high amount of waste material generated throughout the processes. If, however, such material is in demand across the system boundary, the reaction pathway becomes increasingly competitive and the entire system more circular. Note that quantifying the circularity is not a molecular property and hardly a reaction property, but rather a system property, solvable only by data-based descriptions of the environment of a system.
Sustainability metrics for reaction networks from large databases
Ideally, a detailed analysis of social and environmental impacts in an (S-)LCA should be performed to evaluate reaction routes, yet, early-stage reaction data is at present not sufficient for such scopes of analysis. Sustainability assessment is ideally performed early on, as it allows for cheaper and faster implementations of process improvements167–169 and for designing inherently sustainable pathways.12 However, detailed process knowledge is often only available at the end of the process development pipeline.170,171 Streamlining methods to estimate LCA impacts of molecules in early stages try to circumvent this problem.169,170,172–175 Yet, only a few take differences in process conditions into account167,168,176 and the possibility of completely different reaction routes within the reaction network are not discussed at present.
The literature on reaction network optimisation has employed various metrics – much simpler than a full LCA – to assess the sustainability of reaction routes. The metrics utilised by Voll and Marquardt cover mass balances, energy, and cost criteria.30 Zhang et al. utilised the enthalpy of reaction as energy criteria, however conclude that it is not sensitive enough; they recommend the inclusion of separation processes for better performance.34 In later works, some considerations of environmental impacts were included through energy consumption, resource consumption, emission impact, and toxicity potential or the CED and the GWP in the objective function.31,32 While the previous metrics of assessment were built on manually curated data, large-scale reaction network optimisation can only work with metrics obtainable for millions of molecules and reactions in an automated manner.
Mass-based evaluation.
Most early metrics within the field of green chemistry are mass-based evaluations of the reactions. The most influential ones are the AE,177 the E factor,178 and the reaction mass efficiency (RME).38 |  | (1) |
| 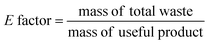 | (2) |
|  | (3) |
Eqn (1)–(3) require knowledge on the following factors: all participating species and their molecular weight must be known, the reaction stoichiometry is required, and differentiation between products and waste needs to be enabled. If circularity is a premise for sustainable processes, we will need to reassess the binary classification into waste and product for such metrics. Eqn (3) also requires information about product yield. More detailed mass-based metrics, e.g. the mass intensity (MI) or process mass intensity (PMI), include the use of solvents, catalysts, and other substances, leading to a more holistic assessment of the reactions.150 |  | (4) |
|  | (5) |
As discussed in the section on Inference of reaction structures, stoichiometry and participating species are often not known and require computationally expensive atom mapping for completion. The section on Inference of reaction outcomes explains that predictions of yield are at present impossible for generic sets of reaction. Furthermore, the differentiation between waste and product is complicated in the context of larger system boundaries and circular economy, where waste streams are seen as potential feedstocks. Improvements in information extraction and data inference can make much more data available in the future. Evolving towards a linked data structure will make evaluations of molecules at system boundaries much easier in reaction networks. Eqn (4) and (5) require additional information on the masses of all involved materials, which also necessitates advanced information extraction techniques for chemical entity recognition.
Assuming reaction data is available, some automated tools can be used to determine mass-based metrics in reaction synthesis plans, e.g. the environmental assessment tool for organic synthesis (EATOS),**** the American Chemical Society PMI (prediction) calculator,†††† or the Andraos’ algorithm.179,180 The EATOS and Andraos’ method were found most rigorous for material efficiency metrics180 and the Green star181,182 and University of Toronto green chemistry initiative method183 were recommended for environmental and hazard impacts in introductory analysis.184 Key challenges for any of the given algorithms at present are: firstly, approximations if data is missing, e.g. general scaling factors for the required masses of organic solvents and aqueous washes,180 secondly, the comparability to biotransformation synthesis,180 thirdly, the evaluation of only linear synthesis trees or synthesis networks,185,186 and last but not least, the integration of recycles for solvents and catalysts.179 Simplified algorithms for linear and tree cases were introduced179,186 and applied in a reaction network.26
Exergy-based evaluation.
Exergy is the maximum amount of work, which can be extracted from a system when the system is brought to thermodynamic equilibrium with components of the natural environment through reversible processes.187 It is a measure of energy quality as it quantifies the ability of a form of energy to do physical work. Exergy destruction is proportional to the entropy generated due to irreversible processes.188 Thus, exergy destruction is a measure of degradation of both energy and material in a system.189
Exergetic analysis has been linked to both, the environmental and the economic aspects of sustainability. From the environmental perspective, the concept of exergy has been positively highlighted as it takes the natural environment into account as a reference state.190 Ao et al. however stress that before widely accepting exergy as an environmental impact indicator, more work needs to be done.191 From the economical perspective, it has been noted that exergy can be strongly linked to costs through exergoeconomics.192 Labour and capital costs for processes can be included in exergy evaluation193 and it may be the most useful function for solving cost-optimisation problems.194 For more information on exergy as a process and/or sustainability indicator, we refer the reader to the reviews by Dewulf et al. and Romero and Linares.190,193
In the context of reaction network optimisation, in our previous work, we utilised an exergy assessment for ranking reaction routes.26 To describe a reaction we included both the physical and chemical exergy of participating species and evaluated further the exergy requirements for process heating and separation.26 Exergetic analysis was applied to rank 15 reaction route options after a priori removal of reactions with insufficient data.
Exergy-based analysis at large scales requires automated retrieval of thermodynamic data. Physical exergies can be computed based on specific heat capacities retrieved from the software COSMOtherm RS, while the computation of chemical exergies pose a larger challenge. Approaches utilising linear regression models for specific types of molecules, e.g. solid or liquid fuels,195–198 more advanced ML models,199–202 and group contribution techniques203 have been proposed. Promising for large-scale data, an atomic contribution model was shown to provide a generic framework to provide simple, yet relatively accurate estimations of the standard molar chemical exergies.204 An alternative is a prediction of Gibb's free energy of formation for compounds, e.g. through the Joback method as in ref. 26, from which the chemical exergy can be calculated based on the tabulated exergies of elements.187,205 In the future, we expect graph convolutional neural networks to predict necessary properties to a high accuracy.206,207
Early-stage assessment.
To a certain extent, simple chemical rules can substitute the computation of data-intensive metrics at present. Especially for large scale datasets, manual data curation from simulations and/or experiments is not an option. In our previous work, we have hence introduced a few simple chemical heuristics, which can be utilised to provide a rough filtration of reaction routes.26 For instance, datasets may be screened for reactions that have a minimum number of records, making them more reliable, or which report a yield value above a certain threshold, making them more efficient. Further heuristics utilise the chemical structure of the materials and may be applied for example to prevent aromatics or certain heteroatoms. We outline an extended list of example heuristics in Table 3. While efficiency potentials may contribute to the environmental and economic dimensions, toxicity potentials shed light on social and environmental issues, and the reliability of the data can bring advantages in social and economic perspectives through faster and safer process development. Note that one heuristic can also cover multiple potentials.
Table 3 Outline of possible heuristics for large-scale screening. Note that all heuristics are independent from stoichiometry and need to be adjusted based on the problem formulation. The list is by no means complete and the functions and potentials listed are exemplary
Heuristic |
Function |
Potential |
Carbon counts |
Remove reactions with large variation of carbon counts |
Efficiency |
Catalysts |
Remove reactions using undesired catalysts |
Efficiency, toxicity |
Fragments |
Preserve/prevent selected fragments throughout the route |
Efficiency, toxicity |
Number of records |
Remove reactions with few records |
Reliability |
Publication year |
Remove old reactions |
Reliability |
Reaction type |
Remove all reactions which are not of desired reaction type |
Reliability |
Reagents |
Remove reactions using undesired reagents |
Toxicity |
Similarity |
Guarantee smooth structural transition along a reaction route |
Efficiency, reliability |
Solvents |
Remove reactions using undesired solvents |
Efficiency, toxicity |
Yield |
Remove reactions with yields lower than threshold |
Efficiency |
4. Decision making
Optimisation algorithms have proven to be a reliable tool for optimal decision-making in complex problems, in particular, in complex network structures. Within reaction network optimisation, decisions on the sequence of reactions from feedstock molecules to target species are required. There commonly exists a variety of reaction sequence possibilities to connect different molecules, see Fig. 10, and appropriate algorithms can make decisions based on metrics discussed in the previous section. Strategies to solve the optimisation formalism depend on the underlying network structure of the problem. The characteristic for the problem of reaction network optimisation however is the number of products and reactants which connect to one reaction, cf.Fig. 10, and which can lead to complex and cyclic network structures.
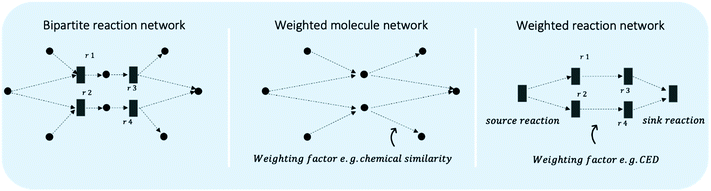 |
| Fig. 10 Illustration of reaction routes in chemical reaction networks. The bipartite reaction network represents reactions as bar nodes (r1 to r4) and molecules as circular nodes. Decision-making is required to decide between two alternatives reaction sequences (r1 and r3 vs. r2 and r4), which connect the same feedstock molecule to the same target molecule. However, different co-reactants are required and different co-products/waste are generated. The directed and weighted molecule network and reaction network are projections of the bipartite network and can function as simplification for shortest path search algorithms. The quantities on the edges illustrate possible weighting schemes. | |
Decision-making in network structures has been broadly explored in many different fields. Examples are vehicle routing or navigation systems,208,209 in tree searches (chemical application of retrosynthesis),210–212 and in supply chain optimisation and task scheduling (chemical application of batch plants in process industries).213–215 In the following, we will shortly review a selection of related fields and highlight their similarities and differences to reaction network optimisation. We will then visit recent literature on reaction network optimisation, which takes the fully connected structure of chemical reaction networks into account. In Table 4 we explain domain-specific terminology.
Table 4 Explanation of specific terminology in decision-making
Term |
Description |
Big O notation |
The big O notation refers to the time or memory needed to run an algorithm. It is a theoretical measure of the asymptotic behaviour of an algorithm. |
Constraints |
Constraints determine the feasible space in which variables can lay. They impose limitations, e.g. that material flows cannot be less than zero. |
Deterministic optimisation |
Deterministic optimisation means all algorithms based on a rigorous mathematical approach, which will lead to the same solution space when run multiple times with the same system parameters. |
Heuristic function |
A heuristic function approximates certain parts of a problem in order to solve a problem more quickly. Precision is traded for speed. |
Linear programming |
Linear programming (LP) problems consist out of a linear objective function and linear constraints. |
Mixed-integer programming |
In mixed-integer programming discrete variables are added to the continuous variables used within the objective function and the constraints. |
Objective function |
The objective function describes the value to be optimised. It is a real-value function, by general convention to be minimised over alternative system variables. |
Relaxation |
A relaxation is an underestimation of a more complicated system to a simpler system. In optimisation, relaxations can transform hard problems into approximated, yet solvable ones. |
Decision-making in network structures
Many decision-making problems can be represented by different network structures where fluxes or connections are optimised. The bipartite reaction network may be approximated by graph projections to reactions or molecules, see Fig. 10, which in turn allow for certain search strategies.
Navigation systems, such as Google Maps, explore the shortest path between two endpoints in a weighted network, where the weighting is the distance or the time required to travel between the points. The algorithm behind navigation systems is often based on the Dijkstra algorithm invented by Edsger Dijkstra in 1959. The algorithm works on a weighted graph, visits each node of the graph, and updates a table on the shortest distances to all others from a selected starting node. Its time complexity is O(|E| + |V|log|V|) where E is the number of edges and V is the number of vertices.208 An extension of the Dijkstra algorithm is the A* (A-star) algorithm, which changes the way the algorithm selects the next node to visit. While in the Dijkstra algorithm, this has been done based on the cost between the start node and the next node, the A* algorithm adds a heuristic function to this process, which estimates the cost from the node to be chosen to the target node.209
While these routing algorithms bring about benefit in implementation and scale-up, they are not directly applicable to reaction networks. Fig. 10 illustrates simplifications of an illustrative reaction network to a directed and weighted network either focusing on molecules or on reactions. The shortest pathway search may be able to regard parts of sustainability considerations in the weights, however, lacks the systems perspective, where co-products/waste and the source of all co-reactants are regarded as inherently connected to the chosen route. Furthermore, the meaning of weights in reaction networks depends on the case study, as they could be the emissions produced, the costs generated, chemical similarity, or any valid combination of our understanding of sustainability.
Tree-based network searches are utilised in the field of automated retrosynthesis planning. The retrosynthetic analysis describes the task of transforming the structure of a synthetic target molecule into known and simpler starting molecules by constructing a sequence of molecule deconstructions.216 Traditionally, iterative cycles of logical analysis and perception were applied by chemists to the target compound and the available data space.217 In automated, or computer-aided, retrosynthesis, an algorithm proposes the most suitable synthesis route. The search space resembles a tree with molecules as nodes and reactions as edges, is intractable large, and the decision-making task is the identification of the most suitable branches within the tree. Solving retrosynthetic trees has been largely inspired by tree problems in games such as Chess or Go, however, retrosynthetic trees are considerably different as they are usually shallower (∼10–20 steps) but the branching factor is higher (around 200 options at each node).210
ML-based techniques have significantly impacted tree-based retrosynthetic analysis.218–220 Segler et al. demonstrated a Monte Carlo Tree Search (MCTS) with three deep neural networks; two ANNs for reaction rule extraction and one as a reinforcement framework.210 Kishimoto et al. investigate two common search techniques within the domain; MCTS and depth-first proof-number (DFPN) search. They find that the enhanced MCTS by Segler et al. outperforms common DFPN, however propose a new DFPN with heuristic rules for edge initialisation, which outperforms Segler's algorithm regarding time complexity and delivers equivalent success rates.211 Coley et al. utilise molecular similarity to inform on edge choices221 and demonstrate how a learned synthetic complexity metric can assist to scan the exponentially increasing search space.212 After further development, Segler et al. present their algorithm based on ANNs and symbolic artificial intelligence, which showed to produce routes that chemists found on average equivalent to the literature reported routes.222 Their algorithm has recently been commercialised by Elsevier and Pending.AI as Reaxys Predictive Retrosynthesis.
Despite differences in network structure, quick searching strategies from the field of computer-assisted retrosynthesis will become immensely valuable when data and metric hurdles are overcome. For sustainability consideration, the task in reaction networks is truly the optimisation of the entire system, including co-products and co-reactants, rather than one synthetic pathway. While retrosynthetic analysis aims towards any known, simple, and cheap starting molecules, starting molecules fulfilling sustainability considerations largely constrain the search space for sustainable pathway identification. Thus, the network topology resembles two branching trees, which meet in the middle, see the description of the “forward-backward” network built by ref. 223 and the overall aim is to optimise the entire system. Nevertheless, techniques from the field of ML-based retrosynthesis will inspire the development of new methods to handle large reaction networks. To take advantage of the full potential of ML-based techniques for decision-making in the chemical domain, the need for explainable artificial intelligence has been emphasised in a recent review article on drug discovery.224 They identify the current lack of an open-community platform but highlight the potential of explainable artificial intelligence for the discovery of novel bioactive compounds.224 Similarly, for computational tools to identify novel reaction pathways, we would expect a faster uptake within the community if solution strategies are comprehensible by chemists and chemical engineers.
Other network systems, from which algorithms can be explored, are batch/job scheduling problems. Here multiple inputs and multiple outputs are taken into account per batch and the sequential manner of performed reactions is regarded. In process industries, consumer products are produced by sequential processing of chemical and physical tasks (in our case a chemical reaction, but generally any kind of task). Tasks require different process units and different storage facilities for in and outputs, which constrain the solution space.213 In single machine batching and scheduling problems, a set of jobs need to be processed by one machine, where jobs of similar type can be processed together and jobs from different families separately.215 The capacity of the machine as well as processing time and heating or cooling requirements strongly constrain the feasible region.215 If working with multiple pieces of equipment, problems are further constrained by sequential requirements, e.g. some pieces of equipment are always used before others, and by equipment interference problems, e.g. certain tasks cannot be performed simultaneously.214
While reaction networks and batch scheduling jobs exhibit similarities in their network structure, it is worthwhile to note their divergence in problem specifics, such as interference constraints, a large variety of different types of tasks, and task-specific constraints such as cooling/heating. However, algorithms from the mature field of batch/task scheduling will come in beneficial when automated large-scale reaction network optimisation develops from the conceptional early-stage design towards different implementation levels, considering supply chains and production planning. Very promising concepts for this are integrated decision-making strategies.225
Pathway optimisation in integrated biorefineries
Identifying the most promising pathway alternatives for the production of chemicals from renewable feedstock has been the focus of superstructure optimisation for integrated biorefineries. A superstructure describes a network of technologies, in particular, a process diagram with all hypothetically useful units and connections.226,227 The advantage of optimising the superstructure e.g. of processes and streams in a biorefinery is that complex interactions between different design choices are considered. However, a rich structure is necessary requiring much data and often leading to large-scale, non-convex, mixed-integer, nonlinear programming models.226
Superstructure problems can be formulated by distinct programming models (i.e. disjunctive programming).228 One approach is a formulation as mixed-integer-nonlinear programming (MINLP) problem.226,227 Giuliano et al. optimise a superstructure for levulinic acid, succinic acid, and ethanol product from lignocellulosic biomass.227 In their approach, rigorous process models account for significant nonlinearities leading to the MINLP formulation. Their problem is linearised to a MILP problem through variable discretisation methods. Kong et al. optimise a superstructure including heat integration and utility plant design by an MINLP problem to which they propose a set of solution methods to speed up the computation.226 Nonlinearities are introduced by processing unit models, where outlet material flows and outlet temperature are nonlinear functions as well as heat and electricity requirements. Alternatively, Garcia and You describe their product and process network by an NLP.229 Nonconvex terms are caused through economic considerations such as capital expenditures. They utilise a piecewise linear approximation, leading to an easier solvable MILP problem.229 Some works formulate the interdependencies through linear models.223,230
Additionally, most works handle contradicting objective functions through a multi-objective framework. Andiappan et al. formulate a multi-objective optimisation of the superstructure for an integrated biorefinery, addressing possible trade-offs between economic and environmental objectives through two approaches. A bi-level formulation maximises the gross profit on the upper level, subject to the minimisation of the environmental burden and the reaction heat on the lower level. Alternatively, fuzzy optimisation is extended by introducing upper and lower bounds for the factor lambda accounting for the satisfaction of all three objectives.230 Garcia and You utilise the epsilon constraint method to allow for multiple objectives.229
Early-stage pathways optimisation in reaction networks
In contrast to rich superstructures with rigorous unit operation models, technologies, and utility integration, stand early-stage evaluation methods. Most promising reaction pathways are estimated at an early-stage without rigorous process models of different technologies. One example of such an early stage approach is Bao et al.'s short-cut method for the preliminary synthesis of process technology pathways.231 They propose a chemical species/conversion operator diagram which they optimise through an NLP model. Nonlinearities are introduced through entering and leaving species flowrates in conversion operators and through annualised costs of conversion. Instead of rigorous models, they assess various conversion technologies through characteristics such as yield and cost.231 Further early-stage methods will be discussed in the following three sections.
Reaction network flux analysis.
Optimal reaction pathways for the conversion of renewable feedstocks are often examined by the approximate method RNFA.30,232 The RNFA is inspired by earlier works on metabolic networks29,233 and models mass flows and reactions through linear balance equations for all components. Hereby, sink and source terms represent supply and demand. To model the reactions, all participating species and the stoichiometry of all reactions need to be known. The RNFA does not account for mixing and separation.
While the core of the problem formulation lays in an LP formulation for mass balances that can be efficiently solved in polynomial time (e.g., using state-of-the-art solvers like CPLEX),234,235 integers have been introduced to account for the activity of fluxes, resulting in a MILP problem.232 Also, alternative optima were identified through the integer constraints,30,232,236 while at present CPLEX can already account for alternative solutions in LPs without manual extension to MILP formulations. In the work of Besler et al. knowledge on active fluxes has also been used to describe non-flux-related costs for reaction pathways, e.g. toxicity. In the work by Dahmen and Marquardt the RNFA is combined with a model for computer-aided molecular design for mixtures, which resulted in an NLP model. Nonlinearities were introduced through the consideration of mixtures, which requires the solver to recompute properties of compositions at each step.237
Notably, the RNFA can lead to degenerated solutions when components are consumed and generated in cycles (e.g., equilibrium reactions or protecting groups). Similarly, huge recycle streams can occur as separation is simplified. The RNFA has been successfully applied to identify optimal reaction pathways for biofuel and biopolymer synthesis.31,34
Process network flux analysis.
An extension of the RNFA is the process network flux analysis (PNFA)33 where pseudo-components and -reactions are introduced to resemble mixing and separation fluxes. For this, all possible mixtures and potential separation tasks are identified a priori, modelled through short-cut methods, and included as pseudo-components and -reactions. The PNFA resembles the superstructure optimisation problems as it aims to include more detailed process knowledge. Operating cost or energy demand of separations are considered through pre-computed energy demands. Besides, binary variables are introduced for all equipment using big-M formulations that are active when the respective flux is greater than zero, allowing the estimation of the number of process units. The investment costs are considered through binary variables and nonlinear cost correlations. Multiple objectives are taken into consideration by the epsilon constraint method. The overall problem results in an MINLP problem that can be solved using deterministic global solvers like BARON238,239 or MAiNGO.240 However, solving nonlinear programs is often NP-hard and thus limited to small problem instances. The PNFA, formulated using GAMS241 and solved by BARON, has been successfully used for biofuels production33 also including the biomass supply chain32 and for pathway considerations for biofuel product design.242
Petri net optimisation.
An alternative modelling approach for the optimisation of pathways in reaction networks is a Petri net. A Petri net explicitly takes the reaction sequence into account which can be an important factor during optimisation. Petri nets were first introduced by Carl Adam Petri243 and are a type of directed bipartite network. In bipartite networks, two node types exist and links can only connect nodes of different types. In Petri nets, the node types are places (resembling molecules) and transitions (resembling reactions). Their input and output relations are shown by links, called arcs and an incidence matrix, which records the stoichiometry. A flow between places via transitions is given by a marking of places with tokens. Such a marking describes a state of a Petri net. Tokens change from one place to another through the firing of transitions, leading to a change in state.244,245
The Petri net optimisation (PNO) problem determines an optimal sequence of firing certain transitions and a formulation for reaction route optimisation was presented by ref. 35 after an extension of the formulation from ref. 246. Petri nets have been used to model chemical and biological reaction networks,243,245,247,248 while the use of PNO in chemical engineering has to date mostly focused on batch scheduling.246,249 The MILP problem has higher model complexity than the LP core of the RNFA. Working with a PNO formulation allows to have a more detailed analysis of the solution space, e.g. the reaction sequence is considered, degenerated solutions are prevented, the maximum size of reaction steps is controlled, and non-flux dependent costs can be introduced.35 The number of continuous variables is higher by a factor of the number of reaction steps and the MILP formulation introduces binary variables per reaction and reaction step. Additionally, the number of constraints is higher, due to constraints on the firing of the transitions in sequence.
Uncertainty in decision-making
Data underlying decision-making algorithms often bring about uncertainties, e.g. through experiments and measurements, through data inference to build complete datasets, through real-life scenarios of market prices, and through dynamic changes in supply and demand. To account for uncertainties in key parameters, deterministic models, which describe parameter uncertainties by bounds of anticipated derivations, or stochastic programming, which takes probability distribution functions for parameters into account, are applied.250
The field of optimisation under uncertainty already contains well-established methods, e.g. stochastic programming, robust optimisation, or fuzzy programming, which can be applied on reaction networks with uncertain data.250,251 While stochastic programming approaches generate comprehensive solutions based on probabilities, they are often computationally expensive. Robust programming defines uncertainties as inequality constraints and is often a good alternative if probability distributions are not known.251 For pathways selection in integrated biorefineries, some works have integrated uncertainties. Morales-Rodriguez et al. have applied stochastic process optimisation for lignocellulosic ethanol production and Kasaš et al. outline a strategy based on stochastic programming merging four distinct solution techniques for a bioethanol product case study.252,253 Tay et al. and Tang et al. solve MINLP problems for integrated biorefineries using robust optimisation.251,254 Uncertainties in the aforementioned studies cover amongst others the market price, supply of biomass, and demand for products as well as technological constraints.
Including uncertainties during decision-making brings about benefits as it avoids non-optimal or infeasible solutions, but requires models that inform on uncertainties within the prediction task.250 For reaction networks, this means that uncertainties for key parameters, e.g. stoichiometry and helper species, or reaction conditions need to be collected during data inference stages.
5. Conclusions and perspective
The identification of sustainable reactions is a highly complex and interdisciplinary challenge. In this review we present the first multidisciplinary perspective, integrating the fields of data, metrics, and decision-making to guide and accelerate further developments. We highlight synergies between the fields and potential for future developments.
Currently, the field of data brings about most bottlenecks, and therewith greatest potential for advancement. Data is, at present, incomplete, lacking information necessary to perform mass balances over large numbers of reactions. Furthermore, enabling linkages of various data sources, e.g. regional waste stream compositions, pretreatment options, or end-of-life use, is essential when dealing with questions of sustainability. For the field of sustainability metrics, we envision, that molecular property prediction, e.g. by graph convolutional networks, will allow more accurate evaluations of different environmental metrics and that linked and accessible data sources will allow assessments across the system boundary. In the area of decision-making, we highlight the importance of the structure of reaction networks (multiple in- and outputs, circular interactions) and the scalability of the previously suggested algorithms as main factors of importance. Methods in the field are well-established and most likely to evolve further through smarter heuristics or ML-guided approximations, enabling solution of system-level problems.
Our findings elucidate the interface between the three areas. This allows scientists to take into account possible improvements within other fields so that we will jointly work towards more sustainable use of present resources. This also highlights the need for targeted interdisciplinary funding across the three domains. Through such targeted interventions society will achieve a faster transition towards developing truly sustainable solutions. The contribution of this work, while conceptual, provides a roadmap towards systematic reaction pathway planning based on rapid digitalisation of chemical data.
Conflicts of interest
Jana Weber, Zhen Guo and Alexei Lapkin are founders of Chemical Data Intelligence (CDI) Pte Ltd. The company is exploiting know-how and developed codes on analysis of large reaction networks.
Acknowledgements
J. M. W. gratefully acknowledges the Department of Chemical Engineering and Biotechnology at the University of Cambridge for funding her PhD scholarship. AMS is supported by the TU Delft AI Labs Programme. C. Z. acknowledges funding of his PhD scholarship by Cambridge Trust and Chinese Scholarship Council. This work was supported by National Research Foundation (NRF), Prime Minister's Office, Singapore under its Campus for Research Excellence and Technological Enterprise (CREATE) program: Cambridge Centre for Advanced Research and Education in Singapore Ltd (CARES, C4T project) (AAL & ZG). CDI Pte Ltd is a spin off company of CARES Ltd.
References
- J. H. Clark, Curr. Opin. Green Sustainable Chem., 2017, 8, 10–13 CrossRef.
- A. Kätelhön, R. Meys, S. Deutz, S. Suh and A. Bardow, Proc. Natl. Acad. Sci. U. S. A., 2019, 166, 11187–11194 CrossRef PubMed.
- S. Wang, G. Dai, H. Yang and Z. Luo, Prog. Energy Combust. Sci., 2017, 62, 33–86 CrossRef.
- Z. Sun, B. Fridrich, A. De Santi, S. Elangovan and K. Barta, Chem. Rev., 2018, 118, 614–678 CrossRef CAS PubMed.
- A. D. Sadiq, X. Chen, N. Yan and J. Sperry, ChemSusChem, 2018, 11, 532–535 CrossRef CAS PubMed.
- Z. Guo, N. Yan and A. A. Lapkin, Curr. Opin. Chem. Eng., 2019, 26, 148–156 CrossRef.
- M. Alexandri, R. Schneider, H. Papapostolou, D. Ladakis, A. Koutinas and J. Venus, ACS Sustainable Chem. Eng., 2019, 7, 6569–6579 CrossRef CAS.
- M. Arshadi, T. M. Attard, R. M. Lukasik, M. Brncic, A. M. Da Costa Lopes, M. Finell, P. Geladi, L. N. Gerschenson, F. Gogus, M. Herrero, A. J. Hunt, E. Ibáñez, B. Kamm, I. Mateos-Aparicio, A. Matias, N. E. Mavroudis, E. Montoneri, A. R. C. Morais, C. Nilsson, E. H. Papaioannou, A. Richel, P. Rupérez, B. Škrbić, M. B. Solarov, J. Švarc-Gajić, K. W. Waldron and F. J. Yuste-Córdoba, Green Chem., 2016, 18, 6160–6204 RSC.
- H. Yang, G. Gözaydln, R. R. Nasaruddin, J. R. G. Har, X. Chen, X. Wang and N. Yan, ACS Sustainable Chem. Eng., 2019, 7, 5532–5542 CrossRef CAS.
- L. Y. Jia, M. Raad, S. Hamieh, J. Toufaily, T. Hamieh, M. M. Bettahar, G. Mauviel, M. Tarrighi, L. Pinard and A. Dufour, Green Chem., 2017, 19, 5442–5459 RSC.
- A. V. Bridgwater, Biomass Bioenergy, 2012, 38, 68–94 CrossRef CAS.
- A. D. Patel, K. Meesters, H. Den Uil, E. De Jong, K. Blok and M. K. Patel, Energy Environ. Sci., 2012, 5, 8430–8444 RSC.
- B. E. Dale, J. Chem. Technol. Biotechnol., 2003, 78, 1093–1103 CrossRef CAS.
- P. F. H. Harmsen, M. M. Hackmann and H. L. Bos, Biofuels, Bioprod. Biorefin., 2014, 8, 306–324 CrossRef CAS.
- H. Storz and K. Vorlop, Appl. Agric. For. Res., 2013, 63, 321–332 Search PubMed.
- J. B. McKinlay, C. Vieille and J. G. Zeikus, Appl. Microbiol. Biotechnol., 2007, 76, 727–740 CrossRef CAS PubMed.
- L. G. Papageorgiou, Comput. Chem. Eng., 2009, 33, 1931–1938 CrossRef CAS.
- T. Verbeek and A. Mah, Econ. Geogr., 2020, 96, 363–387 CrossRef.
- M. Fialkowski, K. J. M. Bishop, V. A. Chubukov, C. J. Campbell and B. A. Grzybowski, Angew. Chem., 2005, 117, 7429–7435 CrossRef.
- K. J. M. Bishop, R. Klajn and B. A. Grzybowski, Angew. Chem., Int. Ed., 2006, 45, 5348–5354 CrossRef CAS PubMed.
- P. M. Jacob and A. Lapkin, React. Chem. Eng., 2018, 3, 102–118 RSC.
- E. J. Llanos, W. Leal, D. H. Luu, J. Jost, P. F. Stadler and G. Restrepo, Proc. Natl. Acad. Sci. U. S. A., 2019, 116, 12660–12665 CrossRef CAS PubMed.
- B. A. Grzybowski, K. J. M. Bishop, B. Kowalczyk and C. E. Wilmer, Nat. Chem., 2009, 1, 31–36 CrossRef CAS PubMed.
- S. Szymkuć, E. P. Gajewska, T. Klucznik, K. Molga, P. Dittwald, M. Startek, M. Bajczyk and B. A. Grzybowski, Angew. Chem., Int. Ed., 2016, 55, 5904–5937 CrossRef PubMed.
- C. M. Gothard, S. Soh, N. A. Gothard, B. Kowalczyk, Y. Wei, B. Baytekin and B. A. Grzybowski, Angew. Chem., Int. Ed., 2012, 51, 7922–7927 CrossRef CAS PubMed.
- P. M. Jacob, P. Yamin, C. Perez-Storey, M. Hopgood and A. A. Lapkin, Green Chem., 2017, 19, 140–152 RSC.
- A. A. Lapkin, P. K. Heer, P. M. Jacob, M. Hutchby, W. Cunningham, S. D. Bull and M. G. Davidson, Faraday Discuss., 2017, 202, 483–496 RSC.
- J. M. Weber, P. Lió and A. A. Lapkin, React. Chem. Eng., 2019, 4, 1969–1981 RSC.
- J. D. Orth, I. Thiele and B. O. Palsson, Nat. Biotechnol., 2010, 28, 245–248 CrossRef CAS PubMed.
- A. Voll and W. Marquardt, AIChE J., 2012, 58, 1788–1801 CrossRef CAS.
- K. Ulonska, A. Voll and W. Marquardt, Energy Fuels, 2016, 30, 445–456 CrossRef CAS.
- K. Ulonska, A. König, M. Klatt, A. Mitsos and J. Viell, Ind. Eng. Chem. Res., 2018, 57, 6980–6991 CrossRef CAS.
- K. Ulonska, M. Skiborowski, A. Mitsos and J. Viell, AIChE J., 2016, 62, 3096–3108 CrossRef CAS.
- D. Zhang, E. A. Del Rio-Chanona and N. Shah, ACS Sustainable Chem. Eng., 2017, 5, 4388–4398 CrossRef CAS.
- J. M. Weber, A. M. Schweidtmann, E. Nolasco and A. A. Lapkin, Eur. Symp. Comput. Aided Process Eng., 2020, 48, 1843–1848 Search PubMed.
-
P. T. Anastas and J. C. Warner, Green Chemistry: Theory and Practice, Oxford University Press, Oxford, 1998, pp. 29–56 Search PubMed.
- S. L. Y. Tang, R. L. Smith and M. Poliakoff, Green Chem., 2005, 7, 761–762 RSC.
- F. G. Calvo-Flores, ChemSusChem, 2009, 2, 905–919 CrossRef CAS PubMed.
- P. T. Anastas and J. B. Zimmermann, Environ. Sci. Technol., 2003, 94–101 CrossRef PubMed.
- S. Y. Tang, R. A. Bourne, R. L. Smith and M. Poliakoff, Green Chem., 2008, 10, 268–269 RSC.
- ISO 14044, 2006, Environmental management — Life cycle assessment — Requirements and guidelines, International Organization for Standardization, available from https://www.iso.org/standard/38498.html.
- L. Jacquemin, P. Y. Pontalier and C. Sablayrolles, Int. J. Life Cycle Assess., 2012, 17, 1028–1041 CrossRef CAS.
- M. A. Curran, Curr. Opin. Chem. Eng., 2013, 2, 273–277 CrossRef.
-
V. Kapur, Hydrocarbon Processing, 2015.
- C. W. Coley, N. S. Eyke and K. F. Jensen, Angew. Chem., Int. Ed., 2020, 59, 23414–23436 CrossRef CAS PubMed.
- M. D. Wilkinson, M. Dumontier, Ij. J. Aalbersberg, G. Appleton, M. Axton, A. Baak, N. Blomberg, J. W. Boiten, L. B. da Silva Santos, P. E. Bourne, J. Bouwman, A. J. Brookes, T. Clark, M. Crosas, I. Dillo, O. Dumon, S. Edmunds, C. T. Evelo, R. Finkers, A. Gonzalez-Beltran, A. J. G. Gray, P. Groth, C. Goble, J. S. Grethe, J. Heringa, P. A. C. t Hoen, R. Hooft, T. Kuhn, R. Kok, J. Kok, S. J. Lusher, M. E. Martone, A. Mons, A. L. Packer, B. Persson, P. Rocca-Serra, M. Roos, R. van Schaik, S. A. Sansone, E. Schultes, T. Sengstag, T. Slater, G. Strawn, M. A. Swertz, M. Thompson, J. Van Der Lei, E. Van Mulligen, J. Velterop, A. Waagmeester, P. Wittenburg, K. Wolstencroft, J. Zhao and B. Mons, Sci. Data, 2016, 3, 160018 CrossRef PubMed.
- M. Lnenicka and J. Komarkova, Gov. Inf. Q, 2019, 36, 129–144 CrossRef.
- M. Janssen and G. Kuk, J. Organ. Comput. Electron. Commer, 2016, 26, 3–13 CrossRef.
-
M. Janssen, R. Matheus and A. Zuiderwijk, International Conference on Electronic Government, 2015, vol. 9248, pp. 79–90.
- A. Thakkar, T. Kogej, J. L. Reymond, O. Engkvist and E. J. Bjerrum, Chem. Sci., 2020, 11, 154–168 RSC.
- Y.-S. Ko, J. W. Kim, J. A. Lee, T. Han, G. B. Kim, J. E. Park and S. Y. Lee, Chem. Soc. Rev., 2020, 49, 4615–4636 RSC.
- K. C. Thomas and W. M. Ingledew, J. Ind. Microbiol., 1992, 10, 61–68 CrossRef CAS.
- N. J. H. Averesch and J. O. Krömer, Front. Bioeng. Biotechnol., 2018, 6, 32 CrossRef PubMed.
- C. Fischer-Romero, B. J. Tindall and F. Jüttner, Int. J. Syst. Bacteriol., 1996, 46, 183–188 CrossRef CAS PubMed.
- T. Fehér, A. G. Planson, P. Carbonell, A. Fernández-Castané, I. Grigoras, E. Dariy, A. Perret and J. L. Faulon, Biotechnol. J., 2014, 9, 1446–1457 CrossRef PubMed.
- V. E. Balderas-Hernández, A. Sabido-Ramos, P. Silva, N. Cabrera-Valladares, G. Hernández-Chávez, J. L. Báez-Viveros, A. Martínez, F. Bolívar and G. Gosset, Microb. Cell Fact., 2009, 8, 1–12 CrossRef PubMed.
- S. Y. Lee, H. U. Kim, T. U. Chae, J. S. Cho, J. W. Kim, J. H. Shin, D. I. Kim, Y. S. Ko, W. D. Jang and Y. S. Jang, Nat. Catal., 2019, 2, 18–33 CrossRef CAS.
- M. Kanehisa and S. Goto, Nucleic Acids Res., 2000, 28, 27–30 CrossRef CAS PubMed.
- A. Morgat, T. Lombardot, K. B. Axelsen, L. Aimo, A. Niknejad, N. Hyka-Nouspikel, E. Coudert, M. Pozzato, M. Pagni, S. Moretti, S. Rosanoff, J. Onwubiko, L. Bougueleret, I. Xenarios, N. Redaschi and A. Bridge, Nucleic Acids Res., 2017, 45, 415–418 CrossRef PubMed.
- N. Nagano, Nucleic Acids Res., 2005, 33, 407–412 CrossRef PubMed.
- M. Oh, T. Yamada, M. Hattori, S. Goto and M. Kanehisa, J. Chem. Inf. Model., 2007, 47, 1702–1712 CrossRef CAS PubMed.
- Y. Moriya, D. Shigemizu, M. Hattori, T. Tokimatsu, M. Kotera, S. Goto and M. Kanehisa, Nucleic Acids Res., 2010, 38, 138–143 CrossRef PubMed.
- J. E. Matthiesen, J. M. Carraher, M. Vasiliu, D. A. Dixon and J. P. Tessonnier, ACS Sustainable Chem. Eng., 2016, 4, 3575–3585 CrossRef CAS.
- M. J. Orella, Y. Román-Leshkov and F. R. Brushett, Curr. Opin. Chem. Eng., 2018, 20, 159–167 CrossRef.
- F. Harnisch and C. Urban, Angew. Chem., Int. Ed., 2018, 57, 10016–10023 CrossRef CAS PubMed.
- V. Balzani, G. Pacchioni, M. Prato and A. Zecchina, Rend. Lincei, 2019, 30, 443–452 CrossRef.
- J. He and C. Janáky, ACS Energy Lett., 2020, 5, 1996–2014 CrossRef CAS PubMed.
- S. J. Lusher, R. McGuire, R. C. Van Schaik, C. D. Nicholson and J. De Vlieg, Drug Discovery Today, 2014, 19, 859–868 CrossRef CAS PubMed.
- T. Berners-Lee, J. Hendler and O. Lassila, Sci. Am., 2001, 284, 34–43 CrossRef.
- P. Murray-Rust, Nature, 2008, 451, 648–651 CrossRef CAS PubMed.
- S. Auer and S. Mann, Ser. Libr., 2019, 76, 35–41 CrossRef.
-
M. Y. Jaradeh, A. Oelen, K. E. Farfar, M. Prinz, J. D’Souza, G. Kismihok, M. Stocker and S. Auer, Proceedings of the 10th International Conference on Knowledge Capture, 2019, pp. 243–246 Search PubMed.
-
S. Auer, A. Kasprzik, V. Kovtun, M. Stocker, M. Prinz and M. E. Vidal, Proceedings of the 8th International Conference on Web Intelligence, Mining and Semantics, 2018, pp. 1–6.
- P. Murray-Rust and H. S. Rzepa, J. Chem. Inf. Comput. Sci., 1999, 39, 928–942 CrossRef CAS.
- F. Farazi, J. Akroyd, S. Mosbach, P. Buerger, D. Nurkowski, M. Salamanca and M. Kraft, J. Chem. Inf. Model., 2019, 60, 108–120 CrossRef PubMed.
- A. Menon, N. B. Krdzavac and M. Kraft, Curr. Opin. Chem. Eng., 2019, 26, 33–37 CrossRef.
- J. Morbach, A. Yang and W. Marquardt, Eng. Appl. Artif. Intell., 2007, 20, 147–161 CrossRef.
- J. Morbach, A. Wiesner and W. Marquardt, Comput. Chem. Eng., 2009, 33, 1546–1556 CrossRef CAS.
- A. Eibeck, M. Q. Lim and M. Kraft, Comput. Chem. Eng., 2019, 131, 106586 CrossRef CAS.
- M. Pan, J. Sikorski, C. A. Kastner, J. Akroyd, S. Mosbach, R. Lau and M. Kraft, Energy Procedia, 2015, 75, 1536–1541 CrossRef.
- M. Kraft and A. Eibeck, Chem. Ing. Tech., 2020, 92, 967–977 CrossRef CAS.
- L. Zhou, C. Zhang, I. A. Karimi and M. Kraft, Energy Procedia, 2017, 142, 2953–2958 CrossRef.
- P. M. Jacob, T. Lan, J. M. Goodman and A. A. Lapkin, J. Cheminform., 2017, 9, 1–12 CrossRef PubMed.
- P. Yaseneva, P. Hodgson, J. Zakrzewski, S. Falß, R. E. Meadows and A. A. Lapkin, React. Chem. Eng., 2016, 1, 229–238 RSC.
-
E. D. Liddy, Encyclopedia of Library and Information Science, Marcel Decker, Inc, NY, USA, 2nd edn, 2001 Search PubMed.
- J. Hirschberg and C. D. Manning, Science, 2015, 349, 261–266 CrossRef CAS PubMed.
-
A. Vaswani, N. Shazeer, N. Parmar, J. Uszkoreit, L. Jones, A. N. Gomez, Ł. Kaiser and I. Polosukhin, 2017, arXiv:1706.03762.
- D. M. Jessop, S. E. Adams, E. L. Willighagen, L. Hawizy and P. Murray-Rust, J. Cheminform., 2011, 3, 1–12 CrossRef PubMed.
- M. Krallinger, O. Rabal, A. Lourenço, J. Oyarzabal and A. Valencia, Chem. Rev., 2017, 117, 7673–7761 CrossRef CAS PubMed.
- N. A. Lewinski and B. T. McInnes, Beilstein J. Nanotechnol., 2015, 6, 1439–1449 CrossRef CAS PubMed.
- P. M. Nadkarni, L. Ohno-Machado and W. W. Chapman, J. Am. Med. Inform. Assoc., 2011, 18, 544–551 CrossRef PubMed.
-
M. Neumann, D. King, I. Beltagy and W. Ammar, 2019, arXiv:1902.07669.
- A. Zaveri, A. Rula, A. Maurino, R. Pietrobon, J. Lehmann and S. Auer, Semant. Web, 2016, 7, 63–93 Search PubMed.
- J. Debattista, S. Auer and C. Lange, J. Data Inf. Qual., 2016, 8, 1–32 CrossRef.
- S. M. Mennen, C. Alhambra, C. L. Allen, M. Barberis, S. Berritt, T. A. Brandt, A. D. Campbell, J. Castañón, A. H. Cherney, M. Christensen, D. B. Damon, J. Eugenio De Diego, S. García-Cerrada, P. García-Losada, R. Haro, J. Janey, D. C. Leitch, L. Li, F. Liu, P. C. Lobben, D. W. C. Macmillan, J. Magano, E. McInturff, S. Monfette, R. J. Post, D. Schultz, B. J. Sitter, J. M. Stevens, I. I. Strambeanu, J. Twilton, K. Wang and M. A. Zajac, Org. Process Res. Dev., 2019, 23, 1213–1242 CrossRef CAS.
- N. S. Eyke, W. H. Green and K. F. Jensen, React. Chem. Eng., 2020, 5, 1963–1972 RSC.
- E. O. Pyzer-Knapp, G. N. Simm and A. A. Guzik, Mater. Horiz., 2016, 3, 226–233 RSC.
- J. J. F. Chen and D. P. Visco, Chem. Eng. Sci., 2017, 159, 31–42 CrossRef CAS.
- E. Danielson, J. H. Golden, E. W. McFarland, C. M. Reaves, W. H. Weinberg and X. Di Wu, Nature, 1997, 389, 944–948 CrossRef CAS.
- Z. Li, S. Wang, W. S. Chin, L. E. Achenie and H. Xin, J. Mater. Chem. A, 2017, 5, 24131–24138 RSC.
- R. D. King, K. E. Whelan, F. M. Jones, P. G. K. Reiser, C. H. Bryant, S. H. Muggleton, D. B. Kell and S. G. Oliver, Nature, 2004, 427, 247–252 CrossRef CAS PubMed.
- A. M. Schweidtmann, A. D. Clayton, N. Holmes, E. Bradford, R. A. Bourne and A. A. Lapkin, Chem. Eng. J., 2018, 352, 277–282 CrossRef CAS.
- C. Houben and A. A. Lapkin, Curr. Opin. Chem. Eng., 2015, 9, 1–7 CrossRef.
- C. W. Coley, D. A. Thomas, J. A. M. Lummiss, J. N. Jaworski, C. P. Breen, V. Schultz, T. Hart, J. S. Fishman, L. Rogers, H. Gao, R. W. Hicklin, P. P. Plehiers, J. Byington, J. S. Piotti, W. H. Green, A. John Hart, T. F. Jamison and K. F. Jensen, Science, 2019, 365, 1–9 CrossRef PubMed.
- S. Steiner, J. Wolf, S. Glatzel, A. Andreou, J. M. Granda, G. Keenan, T. Hinkley, G. Aragon-Camarasa, P. J. Kitson, D. Angelone and L. Cronin, Science, 2019, 363, 1–8 CrossRef PubMed.
- P. Schwaller, A. C. Vaucher, T. Laino and J.-L. Reymond, Mach. Learn. Sci. Technol., 2021, 2, 015016 CrossRef.
- D. Jannach, P. Resnick, A. Tuzhilin and M. Zanker, Commun. ACM, 2016, 59, 94–102 CrossRef.
- P. Resnick and H. R. Varian, Commun. ACM, 1997, 40, 56–58 CrossRef.
- A. Feuerverger, Y. He and S. Khatri, State Sci., 2012, 27, 202–231 Search PubMed.
- E. J. Candès and B. Recht, Found. Comput. Math., 2009, 9, 717–772 CrossRef.
-
V. Kalofolias, X. Bresson, M. Bronstein and P. Vandergheynst, 2014, arXiv1408.1717.2014.
- G. Adomavicius and A. Tuzhilin, IEEE Trans. Knowl. Data Eng., 2005, 17, 734–749 Search PubMed.
- S. Zhang, L. Yao, A. Sun and Y. Tay, ACM Comput. Surv., 2019, 52, 1–38 Search PubMed.
-
J. Savage, A. Kishimoto, B. Buesser, E. DIaz-Aviles and C. Alzate, Proc. 11th ACM Conf. Recomm. Syst., 2017, pp. 210–214.
- F. Jirasek, R. A. S. Alves, J. Damay, R. A. Vandermeulen, R. Bamler, M. Bortz, S. Mandt, M. Kloft and H. Hasse, J. Phys. Chem. Lett., 2020, 11, 981–985 CrossRef CAS PubMed.
- W. Zhang, H. Zou, L. Luo, Q. Liu, W. Wu and W. Xiao, Neurocomputing, 2016, 173, 979–987 CrossRef.
- A. Seko, H. Hayashi and I. Tanaka, J. Chem. Phys., 2018, 148, 241719 CrossRef PubMed.
- N. H. Park, D. Y. Zubarev, J. L. Hedrick, V. Kiyek, C. Corbet and S. Lottier, Macromolecules, 2020, 53, 10847–10854 CrossRef CAS.
- S. Kite, T. Hattori and Y. Murakami, Appl. Catal., A, 1994, 114, 173–178 CrossRef.
- A. Yada, K. Nagata, Y. Ando, T. Matsumura, S. Ichinoseki and K. Sato, Chem. Lett., 2018, 47, 284–287 CrossRef CAS.
- J. G. Estrada, D. T. Ahneman, R. P. Sheridan, S. D. Dreher and A. G. Doyle, Science, 2018, 362, 6416 CrossRef PubMed.
- Z. Fu, X. Li, Z. Wang, Z. Li, X. Liu, X. Wu, J. Zhao, X. Ding, X. Wan, F. Zhong, D. Wang, X. Luo, K. Chen, H. Liu, J. Wang, H. Jiang and M. Zheng, Org. Chem. Front., 2020, 7, 2269–2277 RSC.
- F. Sandfort, F. Strieth-Kalthoff, M. Kühnemund, C. Beecks and F. Glorius, Chem, 2020, 6, 1379–1390 CAS.
- G. Skoraczyñski, P. DIttwald, B. Miasojedow, S. Szymkuc, E. P. Gajewska, B. A. Grzybowski and A. Gambin, Sci. Rep., 2017, 7, 1–9 CrossRef PubMed.
- A. A. Lapkin, A. Voutchkova and P. Anastas, Chem. Eng. Process. Process Intensif., 2011, 50, 1027–1034 CrossRef CAS.
- G. Marcou, J. Aires De Sousa, D. A. R. S. Latino, A. De Luca, D. Horvath, V. Rietsch and A. Varnek, J. Chem. Inf. Model., 2015, 55, 239–250 CrossRef CAS PubMed.
- A. I. Lin, T. I. Madzhidov, O. Klimchuk, R. I. Nugmanov, I. S. Antipin and A. Varnek, J. Chem. Inf. Model., 2016, 56, 2140–2148 CrossRef CAS PubMed.
- H. Gao, T. J. Struble, C. W. Coley, Y. Wang, W. H. Green and K. F. Jensen, ACS Cent. Sci., 2018, 4, 1465–1476 CrossRef CAS PubMed.
- M. H. S. Segler and M. P. Waller, Chem. – Eur. J., 2017, 23, 6118–6128 CrossRef CAS PubMed.
- B. A. Grzybowski, S. Szymkuć, E. P. Gajewska, K. Molga, P. Dittwald, A. Wołos and T. Klucznik, Chem, 2018, 4, 390–398 CAS.
- W. L. Chen, D. Z. Chen and K. T. Taylor, Wiley Interdiscip. Rev.: Comput. Mol. Sci., 2013, 3, 560–593 CAS.
- G. A. Preciat Gonzalez, L. R. P. El Assal, A. Noronha, I. Thiele, H. S. Haraldsdóttir and R. M. T. Fleming, J. Cheminform., 2017, 9, 1–15 CrossRef PubMed.
- W. Jaworski, S. Szymkuć, B. Mikulak-Klucznik, K. Piecuch, T. Klucznik, M. Kaźmierowski, J. Rydzewski, A. Gambin and B. A. Grzybowski, Nat. Commun., 2019, 10, 1–11 CrossRef CAS PubMed.
- P. Schwaller, B. Hoover, J.-L. Reymond, H. Strobelt and T. Laino, Sci. Adv., 2021, 7, eabe4166 CrossRef PubMed.
- Y. Lecun, Y. Bengio and G. Hinton, Nature, 2015, 521, 436–444 CrossRef CAS PubMed.
- E. Rieffel and W. Polak, ACM Comput. Surv., 2000, 32, 300–335 CrossRef.
- A. Ajagekar and F. You, Energy, 2019, 179, 76–89 CrossRef.
- J. Preskill, Quantum, 2018, 2, 79 CrossRef.
- S. Rangarajan, A. Bhan and P. Daoutidis, Ind. Eng. Chem. Res., 2010, 49, 10459–10470 CrossRef CAS.
- S. Rangarajan, A. Bhan and P. Daoutidis, Comput. Chem. Eng., 2012, 45, 114–123 CrossRef CAS.
- W. A. Marvin, S. Rangarajan and P. Daoutidis, Energy Fuels, 2013, 27, 3585–3594 CrossRef CAS.
- J. M. Weber, C. P. Lindenmeyer, P. Liò and A. A. Lapkin, Int. J. Sustainable High. Educ., 2021, 22, 25–41 CrossRef.
- K. Vladimirova and D. Le Blanc, Sustainable Dev., 2016, 24, 254–271 CrossRef.
-
K. Vladimirova and D. Le Blanc, How well are the links between education and other sustainable development goals covered in UN flagship reports? A contribution to the study of the science-policy interface on education in the UN system, New York, USA, 2015.
- R. Costanza, L. Daly, L. Fioramonti, E. Giovannini, I. Kubiszewski, L. F. Mortensen, K. E. Pickett, K. V. Ragnarsdottir, R. De Vogli and R. Wilkinson, Ecol. Econ., 2016, 130, 350–355 CrossRef.
- D. Le Blanc, Sustainable Dev., 2015, 23, 176–187 CrossRef.
-
R. A. Sheldon, I. Arends and U. Hanefeld, Green chemistry and catalysis, John Wiley & Sons, 2007 Search PubMed.
- R. A. Sheldon, Green Chem., 2014, 16, 950–963 RSC.
- M. A. Gonzalez and R. L. Smith, Environ. Prog., 2003, 22, 269–276 CrossRef CAS.
- C. Jiménez-González, D. J. C. Constable and C. S. Ponder, Chem. Soc. Rev., 2012, 41, 1485–1498 RSC.
- P. Marion, B. Bernela, A. Piccirilli, B. Estrine, N. Patouillard, J. Guilbot and F. Jérôme, Green Chem., 2017, 19, 4973–4989 RSC.
-
A. A. Lapkin, Handbook of Green Chemistry, 2010, pp. 1–16 Search PubMed.
-
K. Kümmerer, A.-K. Amsel, D. Bartkowiak, A. Bazzanella, C. Blum and C. Cinquemani, Key Characteristics of Sustainable Chemistry, 2021.
-
Y. Merali and P. Allen, SAGE Handbook of Complexity and Management, 2011, pp. 31–52 Search PubMed.
- E. Nabavi, K. A. Daniell and H. Najafi, J. Clean. Prod., 2017, 140, 312–323 CrossRef.
-
J. D. Sternam, System Dynamics: Systems Thinking and Modeling for a Complex World, 2002 Search PubMed.
- C. Benoît, G. A. Norris, S. Valdivia, A. Ciroth, A. Moberg, U. Bos, S. Prakash, C. Ugaya and T. Beck, Int. J. Life Cycle Assess, 2010, 15, 156–163 CrossRef.
- A. Jørgensen, A. Le Bocq, L. Nazarkina and M. Hauschild, Int. J. Life Cycle Assess, 2008, 13, 96–103 CrossRef.
- A.-M. Tillman, T. Ekvall, H. Baumann and T. Rydberg, J. Clean. Prod., 1994, 2, 21–29 CrossRef.
- H. Ny, J. P. MacDonald, G. Broman, R. Yamamoto and K. H. Robèrt, J. Ind. Ecol., 2006, 10, 61–77 CrossRef.
- W. R. Stahel, Nature, 2016, 531, 435–438 CrossRef CAS PubMed.
- J. Kirchherr, D. Reike and M. Hekkert, Resour., Conserv. Recycl., 2017, 127, 221–232 CrossRef.
-
B. Dittrich-Krämer, C. Bunte, A. Kircherer and T. Schaffrannek, Global Goals Yearbook, 2018 Search PubMed.
-
Ellen MacArthur Foundation, Circularity Indicators An approach to measuring circularity, 2019.
- F. Razza, C. Briani, T. Breton and D. Marazza, Resour., Conserv. Recycl., 2020, 159, 104753 CrossRef.
- K. Lokesh, A. S. Matharu, I. K. Kookos, D. Ladakis, A. Koutinas, P. Morone and J. Clark, Green Chem., 2020, 22, 803–813 RSC.
- P. Karka, S. Papadokonstantakis and A. Kokossis, Int. J. Life Cycle Assess, 2019, 24, 1675–1700 CrossRef.
- P. Karka, S. Papadokonstantakis, K. Hungerbühler and A. Kokossis, Comput. - Aided Chem. Eng., 2014, 34, 543–548 CAS.
- G. Wernet, S. Papadokonstantakis, S. Hellweg and K. Hungerbühler, Green Chem., 2009, 11, 1826–1831 RSC.
- R. Calvo-Serrano, M. González-Miquel, S. Papadokonstantakis and G. Guillén-Gosálbez, Comput. Chem. Eng., 2018, 108, 179–193 CrossRef CAS.
- P. Karka, S. Papadokonstantakis and A. Kokossis, Comput. - Aided Chem. Eng., 2019, 46, 97–102 CAS.
- R. G. Hunt, T. K. Boguski, K. Weitz and A. Sharma, Int. J. Life Cycle Assess., 1998, 3, 36–42 CrossRef.
- A. Marvuglia, M. Kanevski and E. Benetto, Environ. Int., 2015, 83, 72–85 CrossRef CAS PubMed.
- G. Wernet, S. Hellweg, U. Fischer, S. Papadokonstantakis and K. Hungerbühler, Environ. Sci. Technol., 2008, 42, 6717–6722 CrossRef CAS PubMed.
- R. Song, A. A. Keller and S. Suh, Environ. Sci. Technol., 2017, 51, 10777–10785 CrossRef CAS PubMed.
- J. Kleinekorte, L. Kröger, K. Leonhard and A. Bardow, Comput. - Aided Chem. Eng., 2019, 46, 1447–1452 Search PubMed.
- B. M. Trost, Science, 1991, 254, 1471–1477 CrossRef CAS PubMed.
- R. A. Sheldon, Green Chem., 2007, 9, 1273–1283 RSC.
- J. Andraos, Org. Process Res. Dev., 2005, 9, 149–163 CrossRef CAS.
- J. Andraos, ACS Sustainable Chem. Eng., 2016, 4, 1917–1933 CrossRef CAS.
- M. G. T. C. Ribeiro, D. A. Costa and A. A. S. C. Machado, Green Chem. Lett. Rev., 2010, 3, 149–159 CrossRef CAS.
- R. C. C. Duarte, M. G. T. C. Ribeiro and A. A. S. C. Machado, J. Chem. Educ., 2015, 92, 1024–1034 CrossRef CAS.
- S. M. Mercer, J. Andraos and P. G. Jessop, J. Chem. Educ., 2012, 89, 215–220 CrossRef CAS.
- J. Andraos, M. L. Mastronardi, L. B. Hoch and A. Hent, ACS Sustainable Chem. Eng., 2016, 4, 1934–1945 CrossRef CAS.
- J. Andraos and A. Hent, J. Chem. Educ., 2015, 92, 1820–1830 CrossRef CAS.
- J. Andraos, Org. Process Res. Dev., 2006, 10, 212–240 CrossRef CAS.
-
J. Szargut, D. Morris and F. Steward, Energy analysis of thermal chemical, and metallurgical processes, Hemisphere Publishing, New York, USA, 1988 Search PubMed.
-
A. Bejan, G. Tsatsaronis and M. J. Moran, Thermal design and optimization, John Wiley & Sons, New York, 1995 Search PubMed.
- K. Kaygusuz and S. Bilgen, Energy Sources, Part A, 2009, 31, 287–298 CrossRef.
- J. C. Romero and P. Linares, Renewable Sustainable. Energy Rev., 2014, 33, 427–442 CrossRef.
- Y. Ao, L. Gunnewiek and M. A. Rosen, Int. J. Green Energy, 2008, 5, 87–104 CrossRef CAS.
- G. Tsatsaronis, Chem. Eng. Technol., 1996, 19, 163–169 CrossRef CAS.
- J. Dewulf, H. Van Langenhove, B. Muys, S. Bruers, B. R. Bakshi, G. F. Grubb, D. M. Paulus and E. Sciubba, Environ. Sci. Technol., 2008, 42, 2221–2232 CrossRef CAS PubMed.
- S. Bilgen, S. Keleş and K. Kaygusuz, Energy, 2012, 41, 380–385 CrossRef CAS.
- G. Song, L. Shen and J. Xiao, Ind. Eng. Chem. Res., 2011, 50, 9758–9766 CrossRef CAS.
- Y. Zhang, W. Zhao, B. Li, H. Zhang, B. Jiang and C. Ke, Energy, 2016, 106, 400–407 CrossRef.
- G. Song, J. Xiao, H. Zhao and L. Shen, Energy, 2012, 40, 164–173 CrossRef CAS.
- J. H. Shieh and L. T. Fan, Energy Sources, 1982, 6, 1–46 CrossRef CAS.
- S. Sharifian, M. Madadkhani, M. Rahimi, M. Mir and A. Baghban, Pet. Sci. Technol., 2019, 37, 2174–2181 CrossRef CAS.
- F. Gharagheizi and M. Mehrpooya, Energy Convers. Manage., 2007, 48, 2453–2460 CrossRef CAS.
- Y. W. Huang, M. Q. Chen, Y. Li and J. Guo, Energy, 2016, 114, 1164–1175 CrossRef CAS.
- M. Mir, M. Kamyab, M. Janghorban Lariche, R. Razavi and A. Baghban, Pet. Sci. Technol., 2018, 36, 1022–1029 CrossRef CAS.
- F. Gharagheizi, P. Ilani-Kashkouli and R. C. Hedden, Energy, 2018, 158, 924–935 CrossRef CAS.
- R. Haghbakhsh and S. Raeissi, Fluid Phase Equilib., 2020, 507, 112397 CrossRef CAS.
- R. Rivero and M. Garfias, Energy, 2006, 31, 3310–3326 CrossRef CAS.
- A. M. Schweidtmann, J. G. Rittig, A. König, M. Grohe, A. Mitsos and M. Dahmen, Energy Fuels, 2020, 34, 11395–11407 CrossRef CAS.
- C. W. Coley, W. Jin, L. Rogers, T. F. Jamison, T. S. Jaakkola, W. H. Green, R. Barzilay and K. F. Jensen, Chem. Sci., 2019, 10, 370–377 RSC.
-
D. R. Lanning, G. K. Harrell and J. Wang, Proc. 2014 ACM Southeast Reg. Conf., 2014, pp. 1–3.
- H. Mehta, P. Kanani and P. Lande, Int. J. Comput. Appl., 2019, 178, 41–46 Search PubMed.
-
M. Segler, M. Preuß and M. P. Waller, 2017, arXiv:1702.00020.
-
A. Kishimoto, B. Buesser, B. Chen and A. Botea, 33rd Conference on Neural Information Processing Systems, 2019 Search PubMed.
- C. W. Coley, W. H. Green and K. F. Jensen, Acc. Chem. Res., 2018, 51, 1281–1289 CrossRef CAS PubMed.
- K. Neumann, C. Schwindt and N. Trautmann, Or Spectr., 2002, 24, 251–279 CrossRef.
- A. S. Manne, Oper. Res., 1959, 8, 219–223 CrossRef.
- G. Dobson and R. S. Nambimadom, Oper. Res., 2001, 49, 52–65 CrossRef.
- E. J. Corey, Angew. Chem., Int. Ed. Engl., 1991, 30, 455–465 CrossRef.
-
E. J. Corey, The logic of chemical synthesis, 1991 Search PubMed.
- M. Koch, T. Duigou and J. L. Faulon, ACS Synth. Biol., 2020, 9, 157–168 CrossRef CAS PubMed.
- J. S. Schreck, C. W. Coley and K. J. M. Bishop, ACS Cent. Sci., 2019, 5, 970–981 CrossRef CAS PubMed.
- X. Wang, Y. Qian, H. Gao, C. W. Coley, Y. Mo, R. Barzilay and K. F. Jensen, Chem. Sci., 2020, 11, 10959–10972 RSC.
- C. W. Coley, L. Rogers, W. H. Green and K. F. Jensen, ACS Cent. Sci., 2017, 3, 1237–1245 CrossRef CAS PubMed.
- M. H. S. Segler, M. Preuss and M. P. Waller, Nature, 2018, 555, 604–610 CrossRef CAS PubMed.
- V. Pham and M. El-Halwagi, AIChE J., 2012, 58, 1212–1221 CrossRef CAS.
- J. Jiménez-Luna, F. Grisoni and G. Schneider, Nat. Mach. Intell., 2020, 2, 573–584 CrossRef.
- L. S. Dias and M. G. Ierapetritou, Comput. Chem. Eng., 2017, 106, 826–835 CrossRef.
- L. Kong, S. M. Sen, C. A. Henao, J. A. Dumesic and C. T. Maravelias, Comput. Chem. Eng., 2016, 91, 68–84 CrossRef CAS.
- A. Giuliano, R. Cerulli, M. Poletto, G. Raiconi and D. Barletta, Ind. Eng. Chem. Res., 2016, 55, 10699–10717 CrossRef CAS.
- I. Grossmann, Optim. Eng., 2002, 3, 227–252 CrossRef.
- D. J. Garcia and F. You, AIChE J., 2015, 61, 530–554 CrossRef CAS.
- V. Andiappan, A. S. Y. Ko, V. W. S. Lau, L. Y. Ng, R. T. L. Ng, N. G. Chemmangattuvalappil and D. K. S. Ng, AIChE J., 2015, 61, 132–146 CrossRef CAS.
- B. Bao, D. K. S. Ng, D. H. S. Tay, A. Jiménez-Gutiérrez and M. M. El-Halwagi, Comput. Chem. Eng., 2011, 35, 1374–1383 CrossRef CAS.
- A. Besler, A. Harwardt and W. Marquardt, Comput. - Aided Chem. Eng., 2009, 26, 243–248 CAS.
- C. H. Schilling and B. O. Palsson, Proc. Natl. Acad. Sci. U. S. A., 1998, 95, 4193–4198 CrossRef CAS PubMed.
-
N. Karmarkar, Proc. sizteenth Annu. ACM Symp. Theory Comput., 1984, pp. 302–311.
-
P. Gács and L. Lovász, Mathematical Programming at Oberwolfach, Springer Berlin, Heidelberg, 1981, pp. 61–68 Search PubMed.
- M. Hechinger, A. Voll and W. Marquardt, Comput. Chem. Eng., 2010, 34, 1909–1918 CrossRef CAS.
- M. Dahmen and W. Marquardt, Energy Fuels, 2017, 31, 4096–4121 CrossRef CAS.
- M. Tawarmalani and N. V. Sahinidis, Math. Program., Ser. B, 2005, 103, 225–249 CrossRef.
- M. R. Kılınç and N. V. Sahinidis, Optim. Methods Software, 2018, 33, 540–562 CrossRef.
-
D. Bongartz, J. Najman, S. Sass and A. Mitsos, MAiNGO: McCormick based algorithm for mixes integer nonlinear global optimization, Technical report, 2018.
-
M. R. Bussieck and A. Meeraus, Modeling languages in mathematical optimization, Springer, Boston, MA, 2004, pp. 137–157 Search PubMed.
- A. König, L. Neidhardt, J. Viell, A. Mitsos and M. Dahmen, Comput. Chem. Eng., 2020, 134, 106712 CrossRef.
-
C. A. Petri, PhD thesis, Technische Hochschule Darmstadt, 1962.
- M. Ghaeli, P. A. Bahri, P. Lee and T. Gu, Comput. Chem. Eng., 2005, 29, 249–259 CrossRef CAS.
- J. L. Peterson, ACM Comput. Surv., 1977, 9, 223–252 CrossRef.
- E. C. Yamalidou and J. C. Kantor, Comput. Chem. Eng., 1991, 15, 503–519 CrossRef.
- I. Koch, Mol. Inform., 2010, 29, 838–843 CrossRef CAS PubMed.
- C. Chaouiya, Brief. Bioinform., 2007, 8, 210–219 CrossRef CAS PubMed.
- T. Gu, P. A. Bahri and G. Cai, Int. J. Appl. Math. Comput. Sci., 2003, 13, 527–536 Search PubMed.
- L. T. Biegler and I. E. Grossmann, Comput. Chem. Eng., 2004, 28, 1169–1192 CrossRef CAS.
- D. H. S. Tay, D. K. S. Ng and R. R. Tan, Environ. Prog. Sustainable Energy, 2013, 32, 384–389 CrossRef CAS.
- R. Morales-Rodriguez, A. S. Meyer, K. V. Gernaey and G. Sin, Comput. Chem. Eng., 2012, 42, 115–129 CrossRef CAS.
- M. Kasaŝ, Z. Kravanja and Z. N. Pintariĉ, Comput. - Aided Chem. Eng., 2011, 29, 407–411 Search PubMed.
- M. C. Tang, M. W. S. Chin, K. M. Lim, Y. S. Mun, R. T. L. Ng, D. H. S. Tay and D. K. S. Ng, Clean Technol. Environ. Policy, 2013, 15, 783–799 CrossRef CAS.
Footnotes |
† https://www.cas.org/support/documentation/reactions. |
‡ https://supportcontent.elsevier.com/RightNow%20Next%20Gen/Reaxys/New_RX_FactSheet_Jul_2018.pdf. |
§ https://www.nextmovesoftware.com/pistachio.html. |
¶ https://nextmovesoftware.com/blog/2014/02/27/unleashing-over-a-million-reactions-into-the-wild/. |
|| https://www.deepmatter.io/spresi/. |
** https://figshare.com/articles/dataset/Chemical_reactions_from_US_patents_1976-Sep2016_/5104873. |
†† https://github.com/open-reaction-database. |
‡‡ https://www.kegg.jp/kegg/kegg1a.html. |
§§ https://www.rhea-db.org/statistics. |
¶¶ http://ezcatdb.cbrc.jp/EzCatDB/. |
|||| https://5stardata.info/en/. |
*** https://www.cas.org/support/documentation/cas-databases. |
††† https://www.sigmaaldrich.com/chemistry/chemical-synthesis/synthesis-software.html. |
‡‡‡ http://www.suschem.org/about. |
§§§ https://www.isc3.org/en/about-isc3.html. |
¶¶¶ https://www.basf.com/global/en/who-we-are/strategy/verbund.html. |
|||||| https://www.ellenmacarthurfoundation.org/resources/apply/circulytics-measuring-circularity. |
**** http://www.metzger.chemie.uni-oldenburg.de/eatos/english.html (accessed 25.02.2021). |
†††† https://www.acs.org/content/acs/en/greenchemistry/research-innovation/tools-for-green-chemistry.html (accessed 25.02.2021). |
|
This journal is © The Royal Society of Chemistry 2021 |