DOI:
10.1039/D3LC00647F
(Paper)
Lab Chip, 2023,
23, 3961-3977
Artificial intelligence-accelerated high-throughput screening of antibiotic combinations on a microfluidic combinatorial droplet system†
Received
26th July 2023
, Accepted 13th August 2023
First published on 14th August 2023
Abstract
Microfluidic platforms have been employed as an effective tool for drug screening and exhibit the advantages of lower reagent consumption, higher throughput and a higher degree of automation. Despite the great advancement, it remains challenging to screen complex antibiotic combinations in a simple, high-throughput and systematic manner. Meanwhile, the large amounts of datasets generated during the screening process generally outpace the abilities of the conventional manual or semi-automatic data analysis. To address these issues, we propose an artificial intelligence-accelerated high-throughput combinatorial drug evaluation system (AI-HTCDES), which not only allows high-throughput production of antibiotic combinations with varying concentrations, but can also automatically analyze the dynamic growth of bacteria under the action of different antibiotic combinations. Based on this system, several antibiotic combinations displaying an additive effect are discovered, and the dosage regimens of each component in the combinations are determined. This strategy not only provides useful guidance in the clinical use of antibiotic combination therapy and personalized medicine, but also offers a promising tool for the combinatorial screenings of other medicines.
1. Introduction
Antibiotics have greatly lowered the death rate caused by bacterial infections.1,2 However, due to the imprudent and excessive use of antibiotics, many multi-antibiotic resistant bacteria and even superbugs have emerged.3,4 Hence, many known antibiotics, which were highly effective earlier, became obsolete during the past few decades.5
Recently, accumulated evidence revealed that the combination of multiple antibiotics could improve the effectiveness of antibacterial therapy, minimize side effects, and retard the evolution of multi-antibiotic resistant bacterial strains.6,7 For example, the combination of gentamicin and penicillin was found to induce an accelerated death of Group B Streptococcus compared with the use of single antibiotic penicillin.8 To provide timely and targeted combinatorial antibiotic treatments for patients infected with multi-antibiotic resistant bacteria, it is necessary to develop strategies that can rapidly assess therapeutic efficacy of antibiotic combinations. However, due to the numerous types of antibiotics and their combinations, rapid screening of effective and safe antibiotic combinations with optimal dosage remains challenging. The conventional antimicrobial susceptibility assessment (AST), such as agar diffusion,9 broth dilution,10,11 and the Epsilometer test (E-test),12,13 is typically applied for the evaluation of single antibiotics or a few simple antibiotic combinations, which does not comply with the requirements for the rapid screening of complexed antibiotic combinations. Recently, automated instruments for AST have been developed to provide rapid, accurate, and efficient testing. However, they are not accessible for many laboratories due to their high cost.14
Microfluidics is a technique for precise control and manipulation of fluids in micro-sized channels and exhibits the advantages of reduced time, high precision, less consumption of samples and reagents, and low cost. Recent developments in microfluidic technologies have led to their successful application in combinatorial antibiotic screening, which could be classified into two types, platforms based on on-chip concentration gradients and microfluidic droplets.15–17 The former refers to microfluidic chips comprising concentration gradients of antibiotic combinations that are created by the well-designed microchannels.18–20 For example, Ren et al. reported a multiplexed, gradient-based, full-hydrogel microfluidic platform for rapid, high-throughput AST, which adopted a two-layer overlaying channel design that allowed multiple test areas to be integrated on a single device.18–20 Jeon et al. developed a similar microfluidic platform for combinatory antibiotic testing, which generated multiple concentration combinations of an antibiotic pair on a gel-embedded chip in a diffusion-driven manner.20,21 Despite their effectiveness in rapid screening of antibiotic combinations, the strategies based on on-chip concentration gradients require elaborate design and fabrication of the microdevices, and the number of antibiotics for combinations is typically limited. The latter refers to the strategies that utilize active or passive liquid handling techniques (e.g., inject printing, contact printing, T-shaped microchannel method, flow focusing method, coaxial focusing method, etc.) to produce nanoscale to picoscale droplets, in which the target bacteria are encapsulated along with antibiotic combinations.22–29 Since the passive method does not require external energy such as electric field, magnetic field, acoustic field, etc. to generate droplets, it is safer and easier to use than the active approaches and therefore has been extensively applied in combinatorial drug screenings. For instance, Garstecki developed an automated microfluidic droplet platform for screening antibiotic combinations by generating a sequence of droplets with compositions, including reagents and bacterial cell suspensions, which were programmed by the user.25 Merten et al. presented a microdroplet platform for combinatorial drug screening on cancer biopsies, which combined two-phase microfluidics with Braille valves that controlled individual fluid inlets of the microfluidic chip to create droplets containing cells and diverse drug combinations.30 Similarly, Wang et al. proposed a combinatorial nanodroplet platform for screening antibiotic combinations by tuning concentrations of each component in the droplets using independent microchannels with reagent supplies, which were individually opened or closed by pneumatic microvalves in the valve control layer.28 Such systems can generate a large number of droplets in a short period of time, increase the throughput of screening and reduce the reagent consumption. However, the approaches for generating droplets with varying components were either too complicated (e.g., requiring complex microchannels, valve control layers and precise manipulation on multiple microvalves) or limited in the types of combinations.23,25,28,30,31 Moreover, some approaches relied on high voltage for droplet merging, which might cause an unknown effect on bacterial vitality.16,22,32–35
Apart from the abovementioned limitations in the design of microdevices, the large amounts of datasets generated during the screening process generally outpace the abilities of the conventional manual or semi-automatic data analysis, which greatly restricts the throughput of current approaches.
To address these issues, we proposed an artificial intelligence-accelerated high-throughput combinatorial drug evaluation system (AI-HTCDES) for rapid, high-throughput and systematic screening of drug combinations. The system consisted of a microfluidic combinatorial droplet platform and an artificial intelligence (AI)-based data analysis module. The microfluidic combinatorial droplet platform could generate droplets containing the target bacteria and antibiotic combinations of varying types and concentrations in a high-throughput manner. It was independent of complicated microdevices and could conveniently produce droplets of varying components by simply adopting commercialized multiway valves and programming the loading schemes of syringe pumps. Since massive data were produced in the screening processes (>7000 images), an AI-based module was developed to accelerate the data analysis process. As a powerful image analysis tool, AI has faster and more efficient data processing capabilities than many traditional analysis algorithms. It provides a new approach, which is able to identify the features and reveal the implicit relationships from the raw data (e.g., RGB images) by building a multilayer network.36–39 To date, it is widely used in image processing, including image classification,40 region segmentation41 and super-resolution reconstruction.42,43 In our work, the AI-based module was developed to automatically identify and segment the droplets in images, extract the average gray values of the droplets and background, respectively, and calculate the time-dependent density of bacteria in the droplets.
As a proof-of-concept study, four antibiotics and three of their combinations were quantitatively evaluated using the AI-HTCDES, which displayed an additive effect. Meanwhile, the dosage regimens of each component in the combinations were discovered. The AI-HTCDES not only enabled high-throughput screening of antibiotic combinations with effective concentrations, but also helped reveal the dynamic growth of bacteria under the action of different antibiotic combinations. Such a system provided useful guidance in the clinical use of antibiotic combination therapy and personalized medicine and offered a potent tool for many fundamental studies, such as revealing the effect of complex antibacterial drug combinations on bacterial growth, disclosing the interactive patterns between different antibiotics, and investigating the evolution of bacterial resistance under different antibiotic combinations.
2. Materials and methods
2.1. Materials and reagents
Polydimethylsiloxane (PDMS) was purchased from Dow Corning (USA). Rhodamine 6G and rhodamine B (red fluorescent dye) were obtained from Aladdin Chemical Co., Ltd. (Shanghai, China). Fluorescein sodium salt (green fluorescent dye) was supplied by Aladdin Chemical Co., Ltd. (Shanghai, China). Hoechst 33342 (blue fluorescent dye) was acquired from Thermo-Fisher Scientific Co., Ltd. Liquid paraffin was supplied by Zhiyuan Chemical Reagent Co., Ltd. (Tianjin, China). The surfactant ABIL® EM 90 was purchased from Evonik (Germany). Inoculation loops and Luria Broth (LB) liquid medium were acquired from HuanKai Microbiology Technology Co., Ltd. (Guangdong, China). Cefepime was supplied by Macklin Biochemical Technology Co., Ltd. (Shanghai, China). Gentamicin was purchased from TargetMol Chemicals (Shanghai, China). Chloramphenicol and tetracycline were obtained from Macklin Biochemical Technology Co., Ltd. (Shanghai, China).
2.2. Fabrication of the collection chips
The microfluidic devices were fabricated by the replica molding approach. First, an S-shaped channel structure was manufactured by mechanical engraving on an aluminum template. Next, 50 mL of PDMS mixture with a mass ratio of 10
:
1 (base to cross-linker) was poured onto the surface of the aluminum template. After degassing, the PDMS was cured at 80 °C and peeled off from the template. Then, the PDMS replica with S-shaped channels was bonded onto a transparent glass plate for droplet collection and storage (Table S1†). Afterwards, a six-way valve (Table S2†) for droplet generation was connected to the S-shaped collection chip.
2.3. Numerical simulations
The process of droplet generation and collection was numerically investigated using the COMSOL Multiphysics 5.6 software. Water (fluid 1) and paraffin oil (fluid 2) were used as the dispersed phase and the continuous phase, respectively. The simulations were carried out in a two-dimensional domain through a six-way droplet generator and an S-shaped channel (Fig. 1) along with the geometric dimensions and the computational mesh. All simulations were fulfilled through the laminar two-phase flow physics module. The boundary condition was set as no slip. The pressure of the outlet border was set as a standard atmospheric pressure to suppress backflow. The droplet length and the distance between the adjacent droplets were measured based on the simulation images with references to the scales using ImageJ. The distribution curves of the droplet size and distance were fitted by the density estimation with the Gaussian kernel function.
 |
| Fig. 1 Fabrication, characterization and simulations of the microfluidic combinatorial droplet platform. (a) A representative photograph of the AI-HTCDES. (b) A representative photograph showing the droplets collected in order by the droplet collection chip. The scale bar is 20 mm. (c) Simulations of the droplet generation and collection. i) No droplets form when the total flow rate of the aqueous phase and the flow rate of the oil phase are 20 μL min−1 and 120 μL min−1, respectively. S = 0. ii) Stable droplets are produced when the total flow rate of the aqueous phase and the flow rate of the oil phase are 80 μL min−1 and 120 μL min−1, respectively. S = 100%. iii) Unstable droplets are generated when the total flow rate of the aqueous phase and the flow rate of the oil phase are 200 μL min−1 and 120 μL min−1, respectively. S = 61.67%. (d) A plot showing the stability of droplets generated at varying flow rates. The gray circular dots indicate the conditions that no droplets form. The red triangular dots represent the conditions that droplets can form with varying stability. | |
2.4. Optimization of the flow rate
The droplet collection chip was modified by cutting part of the outlet into a square shape (∼12 mm × 12 mm, Fig. S1†) to enlarge the outlet, adding a surfactant to the oil phase (3% ABIL® EM 90) and experimentally adjusting the flow rate of total aqueous and oil phases. The flow rates of the aqueous and oil phases were experimentally optimized as follows. Briefly, pure water was introduced as the aqueous phase through inlet I, inlet II and inlet III using a programmable syringe pump (TJP-3A LongerPump, Longer, China). Meanwhile, liquid paraffin (3% ABIL® EM 90) was administered as the continuous phase for emulsification through inlet IV and inlet V using a dual-channel syringe pump (LSP02-2A LongerPump, Longer, China). By adjusting the flow rates of the aqueous and oil phases (Tables S3 and S4†), droplets with varying sizes were generated and then collected on the chip. Besides, a proportion of the droplets was collected in Petri dishes filled with paraffin oil. In this way, the droplets were freely suspended in the oil and maintained a spherical shape, the volume of which could be easily calculated. The bright-field images of the droplets were captured using a computerized charge-coupled device (CCD) camera (Digital Sight DS-Fi2, Nikon, Japan), and the size of the droplets was measured with ImageJ. The droplets in the Petri dish were solely for measuring the droplet volume and not used for image taking and bacteria density processing.
2.5. Validation of the loading schemes
As a demonstration, 3-, 5- and 6-stepped loading schemes were validated (Tables S5–S7†). To better visualize and quantify the drug concentration generated under specified loading schemes, aqueous solutions containing 0.01 mg mL−1 red fluorescent dye (rhodamine 6G), 0.04 mg mL−1 red fluorescent dye (rhodamine B), 0.01 mg mL−1 green fluorescent dye (fluorescein sodium salt) and 0.04 mg mL−1 blue fluorescent dye (Hoechst 33342) were prepared, respectively, as representatives of different drugs. To produce droplets comprising varying concentrations of a single drug, the aqueous solution containing red fluorescent dye (rhodamine 6G) was introduced from inlet II, and pure water was loaded from inlets I and III according to the 3-, 5- and 6-stepped loading schemes. Similarly, to produce droplets comprising varying concentrations of two combinatorial drugs, pure water, the aqueous solution of red fluorescent dye and the aqueous solution of blue fluorescent dye were introduced from inlets I, II and III, respectively, according to the 3- and 5-stepped loading schemes. In addition, to produce droplets comprising varying concentrations of three combinatorial drugs, the aqueous solutions of red fluorescent dye, green fluorescent dye and blue fluorescent dye were selected as representatives of three different drugs using three-stepped loading schemes (Table S8†), respectively. The red, green and blue fluorescence images of the droplets were captured using a CCD camera, and the fluorescence intensity of the droplets was analyzed with ImageJ, respectively. To minimize bias, the droplets were randomly selected at constant intervals, and about 20 droplets produced during the stabilization process were not taken into consideration. The approach was validated by comparing the average fluorescence intensity among all droplets at each step, all droplets except for the first 20 ones at each step, and droplets selected using our approach, which were similar without significant difference (Fig. S2†).
2.6. Calibration of the density of E. coli
A stock solution of E. coli (1.84 × 109 CFU mL−1) was diluted to obtain E. coli suspensions with densities of 9.20 × 108 CFU mL−1, 4.60 × 108 CFU mL−1, 2.30 × 108 CFU mL−1, 1.84 × 108 CFU mL−1, 4.60 × 107 CFU mL−1, 2.30 × 107 CFU mL−1 and 2.30 × 106 CFU mL−1, respectively. The prepared dilutions were loaded as the aqueous phase to generate droplets comprising varying densities of E. coli. The total flow rate of the aqueous phase and oil phase remained at 80 μL min−1 and 200 μL min−1, respectively. The generated droplets were harvested in the S-shaped collection chip, the bright-field images of which were captured using a CCD and the gray value was measured with ImageJ.
2.7. Deep learning-aided image analysis
Deep learning was introduced to analyze the obtained images. Herein, we randomly selected 40 images of droplets containing varying densities of E. coli as the training and validation dataset. A 4-fold cross-validation (CV) experiment was implemented to verify the performance of our model. Briefly, the dataset was divided into four subsets stochastically, three of which were applied for training and the rest for validation. Four different models could be obtained by selecting different subsets for training and validation. The processes of validation were repeated four times by the four models, respectively. Through CV experiments, we obtained four confusion matrices and parameters including the total loss, the classification loss, and the bounding box regression loss. Then the mean value and standard deviation (std) were calculated to evaluate the performance of our neural network models.
The deep learning framework of Pytorch was employed as the backend platform, and the image segmentation network based on the mask region-based convolutional neural network (Mask R-CNN) with ResNet-50 was implemented as the backbone network, which is based on Detectron2, a library that provides image detection and segmentation algorithms. Our model was pre-trained on the image segmentation database of Microsoft COCO to initialize the parameters. Based on the model, we carried out fine-tuning, in which only the weights of the top-down layers in the convolutional backbone network were replaced and the weights of the other layers were frozen, so that the network can combine the information derived from the image during pre-training to better obtain the features of droplets.
Momentum SGD was selected as the optimizer, and the learning rate scheduler was WarmupMultiStepLR. Smooth L1 was employed as the bounding box regression loss function, Cross Entropy was selected as the classification loss function, the learning rate was set to 0.001 and the batch size was set to 3. The fine-tuning was run for 3000 epochs on a hardware platform with Intel Xeon Silver 4210 CPU, 128 GB DDR4 RAM, 1 TB SSD, and Nvidia RTX 2080Ti GPU*4. The precision, recall rate and accuracy were calculated according to the following equations:
|  | (1) |
|  | (2) |
|  | (3) |
where TP represents the number of positive samples (images containing one droplet) that are predicted to be positive samples (images containing one droplet), TN represents the number of negative samples (images containing more than one droplet) that are predicted to be negative samples (images containing more than one droplet), FP represents the number of negative samples (images containing more than one droplet) that are predicted to be positive samples (images containing one droplet), and FN represents the number of positive samples (images containing one droplet) that are predicted to be negative samples (images containing more than one droplet or cannot be recognized).
2.8. Quantitative evaluation of single antibiotic-based monotherapy
Gentamicin, cefepime, chloramphenicol and tetracycline were selected as representative antibiotics. The different antibiotic concentrations for single antibiotic-based monotherapy were set based on the MIC values recorded in the literature.44–49 First, the solutions of gentamicin (4 μg mL−1 and 6 μg mL−1), cefepime (25 ng mL−1 and 50 ng mL−1), chloramphenicol (2 μg mL−1 and 5 μg mL−1) and tetracycline (0.4 μg mL−1 and 0.8 μg mL−1) were prepared by dissolving the weighted powders in LB liquid medium, respectively. Afterwards, the E. coli stock solution (1 × 104 CFU mL−1), the prepared antibiotic solutions, and the LB liquid medium were loaded to the droplet generator through inlet I, inlet II and inlet III, respectively, according to the 3-stepped loading scheme (Table S5†). During the experiment, the flow rates of the total aqueous phase, bacterial solution and oil phase were fixed without adjustment to ensure the consistency of the droplet size, droplet volume and the density of the bacteria in the droplet. The droplets containing E. coli treated by antibiotics of varying types and concentrations were harvested in the collection chips. The collection chips holding droplets were then incubated at 37 °C and taken out for imaging at 0 h, 2 h, 4 h, 6 h, 8 h, 10 h, 12 h, 18 h and 24 h. The bright-field images of the droplets were collected using a CCD camera to evaluate the growth of E. coli. The MICs were calculated based on the growth curves of E. coli. The inhibition curves and half-maximal inhibitory concentration (IC50) were fitted and calculated using the built-in module of log(inhibitor) vs. response – variable slope (four parameters) in GraphPad Prism.
For comparison, the evaluation of single antibiotics based on a 96-well plate was conducted as well. Briefly, 1 mg mL−1 gentamicin, cefepime, tetracycline and chloramphenicol stock solutions were diluted to the designated concentration, and the E. coli suspension was diluted to 2800 CFU mL−1. Afterwards, 180 μL of E. coli stock solution and 20 μL of antibiotic solution were mixed in each well on the plate. The plates were then incubated at 37 °C and the absorbance of each well was measured at 0 h, 2 h, 4 h, 6 h, 8 h, 10 h, 12 h, 18 h and 24 h, respectively.
2.9. Screenings of antibiotic combinations
To quantitatively evaluate the antibiotic combinations, the stock solutions of gentamicin (2.00 μg mL−1, 3.00 μg mL−1 and 4.00 μg mL−1), chloramphenicol (1.66 μg mL−1 and 3.33 μg mL−1), tetracycline (0.27 μg mL−1 and 0.40 μg mL−1) and cefepime (25.00 ng mL−1 and 33.00 ng mL−1) were prepared first. Then the following combinations for stock solutions were selected for the proof-of-concept study, gentamicin/chloramphenicol (2.00 μg mL−1/1.66 μg mL−1, 3.00 μg mL−1/3.33 μg mL−1), gentamicin/cefepime (3.00 μg mL−1/25.00 ng mL−1, 4.00 μg mL−1/33.00 ng mL−1) and gentamicin/tetracycline (2.00 μg mL−1/0.27 μg mL−1, 3.00 μg mL−1/0.40 μg mL−1). The different antibiotic concentrations for antibiotic combinations were set based on the MIC values of single antibiotics, which were determined by the single antibiotic-based monotherapy experiment. Briefly, the E. coli suspension (1 × 104 CFU mL−1) and the two types of antibiotic solutions for combination were loaded through inlet I, inlet II and inlet III, respectively, to generate combinations with varying concentrations. The droplets containing E. coli treated by antibiotic combinations of varying types and concentrations were harvested in the collection chips. The collection chips holding droplets were then incubated at 37 °C and taken out for imaging at 0 h, 2 h, 4 h, 6 h, 8 h, 10 h, 12 h, 18 h and 24 h. The bright-field images of the droplets were collected using a CCD camera to evaluate the growth of E. coli. The MICs were calculated based on the growth curves of E. coli.
For each combination, the fractional inhibitory concentration (FIC) index was calculated (the FIC index = MIC of drug A in combination/MIC of drug A alone + MIC of drug B in combination/MIC of drug B alone). A FIC index ≤ 0.5 means synergism, 0.5 < FIC index ≤ 1 indicates additive, 1 < FIC index ≤ 2 suggests irrelevance, and a FIC index > 2 implies antagonism.50
2.10. Significant difference analysis
Experimental data were presented as mean ± std. A simple linear model was adopted for fitting using GraphPad Prism 8. Statistical analysis was performed using two-way ANOVA. A value of P < 0.05 was considered as statistically significant, and P < 0.01, P < 0.001 and P < 0.0001 were considered highly statistically significant.
3. Results and discussion
3.1. Working principles of the AI-HTCDES
The AI-HTCDES consists of two parts, a microfluidic combinatorial droplet platform and an AI-based data analysis module. The microfluidic combinatorial droplet platform was composed of programmable syringe pumps, a microfluidic droplet generator and a droplet collection chip. The working principle of the platform is demonstrated in Scheme 1a. Firstly, droplets containing the target bacteria and antibiotic combinations of varying types and concentrations could be steadily produced in a high-throughput manner by programming the loading schemes of the syringe pumps. Then the droplets were harvested and aligned in order in the S-shaped channel of the collection chip. Afterwards, the bright-field images of the droplets were obtained by microscopy imaging and the gray values of the droplets were measured to determine the density of bacteria.
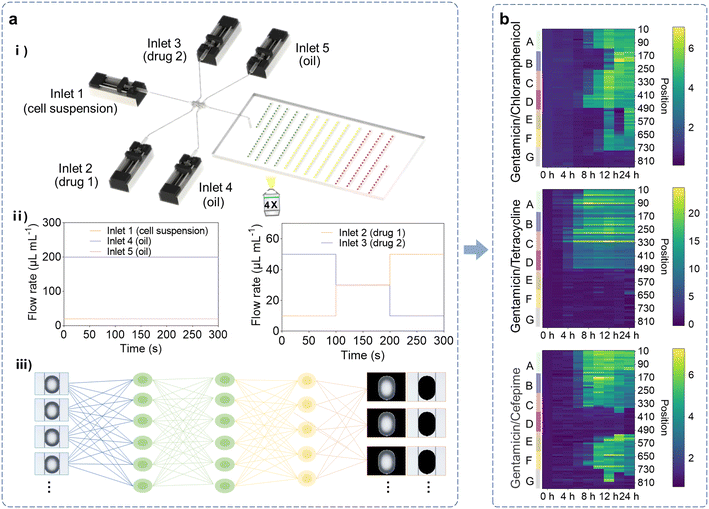 |
| Scheme 1 Schematics showing the working principles of the AI-HTCDES. (a) i) A schematic diagram of the microfluidic combinatorial droplet platform. ii) The loading schemes of different solutions. iii) The architecture of the AI-based data analysis module. (b) Heat maps showing bacteria growth under antibiotic combinations of varying types and concentrations at the time points of 0 h, 2 h, 4 h, 6 h, 8 h, 10 h, 12 h, 18 h and 24 h. | |
Since massive images were produced in our experiment (more than 7000 images), traditional manual analysis would be labor-intensive, time-consuming and error-prone. Therefore, we developed an AI-based module to accelerate the data analysis process, which could automatically identify and segment the droplets in images, and then extract the average gray values of the droplets and background, respectively. The quantitative evaluation results for the antibiotic combinations of interest were ultimately summarized as heat maps, which provided useful guidance in the clinical use of antibiotic combination therapy and personalized medicine (Scheme 1b).
3.2. Fabrication, characterization and simulations of the microfluidic combinatorial droplet platform
The microfluidic combinatorial droplet platform comprising programmable syringe pumps, a microfluidic droplet generator and a droplet collection chip was set up according to the working principles of the AI-HTCDES (Fig. 1a). A commercial six-way valve was adopted as the droplet generator (Table S2†). The oil phase was loaded to the droplet generator at a constant flow rate to exert uniform shear stress on the aqueous phases. The droplets were generated and then collected in order by the droplet collection chip (Fig. 1b). The actual dimensions of the droplet collection chip were measured, which exhibited high consistency with the designed values (Table S1†).
To demonstrate the feasibility of the microfluidic combinatorial droplet platform for droplet generation and collection, numerical simulations were carried out. To quantify the ability of droplets to maintain the original size, the parameter of stability (S) was proposed, which is defined according to the following equation:
where
L1 represents the channel length from the inlet to the position where droplets start to fuse, and
L0 represents the total channel length from the inlet to the outlet. The value of
S = 0 indicates that no droplets form. The value of
S = 100% implies that the droplets are steadily generated and stably reside in the S-shaped channel of the droplet collection chip. The value of 0 <
S < 100% suggests that the droplets unstably reside in the S-shaped channel and a proportion of them will merge. The distance between the adjacent droplets and the droplet size characterized by the droplet length parallel to the axis of the channel were characterized as well. According to the simulation results, when the total flow rate of the aqueous phase was fixed at 80 μL min
−1 and the flow rate of the oil phase was higher than 200 μL min
−1, or the flow rate of the oil phase was fixed at 120 μL min
−1 and the total flow rate of the aqueous phase varied between 20 μL min
−1 and 60 μL min
−1, the droplets could not form (
Fig. 1c-i and d). In contrast, when the total flow rate of the aqueous phase was fixed at 80 μL min
−1 and the flow rate of the oil phase ranged between 60 μL min
−1 and 120 μL min
−1, or the flow rate of the oil phase was fixed at 120 μL min
−1 and the total flow rate of the aqueous phase ranged between 80 μL min
−1 and 140 μL min
−1, the droplets could be steadily generated and stably reside in the S-shaped channel of the droplet collection chip (
Fig. 1c-ii and d and S3a
†). Moreover, the droplets were uniform in size and the distances between two adjacent droplets remained nearly constant (Fig. S4
†). It was worth noting that under certain conditions, as the droplets moved towards the outlet, the distance between them was reduced and the neighboring droplets started to merge to generate larger droplets due to the accumulated pressure (
Fig. 1c-iii and d, S3b and S4
†). It was observed that when the total flow rate of the aqueous phase was fixed at 80 μL min
−1 and the flow rate of the oil phase ranged between 140 μL min
−1 and 180 μL min
−1, or the flow rate of the oil phase was fixed at 120 μL min
−1 and the total flow rate of the aqueous phase was higher than 160 μL min
−1, the stability of the droplets varied between ∼21% and ∼97% (
Fig. 1d). To avoid the merging issue and ensure an appropriate droplet size as much as possible, we modified the droplet collection chip by enlarging the outlet to release pressure (Fig. S1
†), adding a surfactant to the oil phase (see the Materials and methods section for details) and experimentally adjusting the flow rate of total aqueous and oil phases. In this way, droplets with appropriate size could be successfully produced and remain stable in the collection chip within a wide range of practical flow rates (
Fig. 1b and S5
†).
3.3. Tuning the size and composition of droplets in a programmable way
Since the practical parameters of the system were more complicated than the simplified simulation model, the simulation data and the droplet microfluidic results might not be exactly the same. Therefore, we need to further validate and optimize the system by experiment. The size of the droplets could be controlled by adjusting the flow rates of the aqueous and oil phases.39 Firstly, the flow rate of the oil phase was fixed at 200 μL min−1 and the total flow rate of the total aqueous phase was programmatically adjusted to increase from 20 μL min−1 to 170 μL min−1 (Table S3†). As shown in Fig. 2a and S6,† droplets with varying sizes were produced. To calculate the volume of the droplets, the frequency of droplet generation was measured, which ranged from 67 min−1 to 201 min−1, as shown in Fig. 2b-i. Therefore, the theoretical volume of droplets can be calculated according to the following equation:where Vt represents the theoretical volume of the droplet, Qaq is the total flow rate of the aqueous phase (μL min−1), and v represents the frequency of droplet generation (min−1). Since the droplets were deformed in the S-shaped channels, their volumes could not be precisely determined. Hence, the droplets were then gently pushed out of the chip and collected in Petri dishes filled with paraffin oil. In this way, the droplets were freely suspended in the oil and maintained a spherical shape, the Vm of which can be easily calculated by a simple ball volume formula:where R represents the radius of the droplet, which could be determined by measuring the images of the droplets collected in Petri dishes (Fig. 2b-ii). As shown in Fig. 2b-iii, when the total flow rate of the aqueous phase increased, Vt augmented linearly from 0.30 ± 0.01 μL to 0.85 ± 0.02 μL, which was consistent with the Vm that increased linearly from 0.29 ± 0.04 μL to 0.98 ± 0.02 μL. However, the results indicated that Vm was slightly larger than Vt. This might be attributed to the fact that the droplet was in contact with the bottom of the Petri dish due to gravity, resulting in a larger bias of the radius.
 |
| Fig. 2 Tuning the size and composition of droplets in a programmable way. (a) Bright-field images of droplets at varying flow rates of the aqueous phase (20 μL min−1, 50 μL min−1, 80 μL min−1, 110 μL min−1, 140 μL min−1, 170 μL min−1, respectively) with a constant oil phase flow rate of 200 μL min−1. The scale bar is 300 μm. (b) i) Generation frequency, ii) radius and iii) volume of droplets at varying total flow rates of the aqueous phase. Simple linear regressions were fitted to the data, respectively (frequency: y = 0.876x + 60.70, R2 = 0.9719, n = 13; radius: y = 1.345x + 409.70, R2 = 0.8718, n = 13; theoretical volume: y = 0.004x + 0.27, R2 = 0.9809, n = 13; measured volume: y = 0.005x + 0.24, R2 = 0.8502, n = 13). (c) i) Generation frequency, ii) radius and iii) volume of droplets at varying flow rates of the oil phase. Simple linear regressions were fitted to the data, respectively (frequency: y = 0.534x + 26.17, R2 = 0.9940, n = 13; radius: y = −0.913x + 726.50, R2 = 0.9357, n = 13; theoretical volume: y = −0.003x + 1.26, R2 = 0.9079, n = 13; measured volume: y = −0.004x + 1.44, R2 = 0.9043, n = 13). (d) The average fluorescence intensity and representative fluorescence images of droplets under a 5-stepped loading scheme for a single drug. A simple linear regression was fitted to the data (y = 12.490x + 25.03, R2 = 0.8894, n = 13). (e) The average fluorescence intensity and representative fluorescence images of droplets under a 3-stepped loading scheme for drug combinations. Simple linear regressions were fitted to the data (blue fluorescence: y = −15.850x + 66.36, R2 = 0.9792, n = 13; red fluorescence: y = 17.500x − 1.05, R2 = 0.9928, n = 13). (f) The average fluorescence intensity and representative fluorescence images of droplets under a 5-stepped loading scheme for drug combinations. Simple linear regressions were fitted to the data (blue fluorescence: y = −8.732x + 63.42, R2 = 0.9768, n = 13; red fluorescence: y = 8.656x + 3.42, R2 = 0.9619, n = 13). The scale bar is 300 μm. | |
Secondly, the total flow rate of the aqueous phase was fixed at 80 μL min−1 and the flow rate of the oil phase was programmatically adjusted to increase from 100 μL min−1 to 260 μL min−1 (Table S4†). As demonstrated in Fig. S7,† droplets with varying sizes were produced as well. The results showed that as the flow rate of the oil phase increased, the frequency of droplet generation increased from 76 min−1 to 166 min−1, and the radius of the droplet was reduced accordingly (Fig. 2c). Meanwhile, the Vt decreased linearly from 1.04 ± 0.01 μL to 0.48 ± 0.01 μL, and the Vm decreased linearly from 1.15 ± 0.06 μL to 0.51 ± 0.08 μL (Fig. 2c-iii). These results suggested that the size of droplets could be tuned in a programmable way. Based on the results, flow rates of 200 μL min−1 for the oil phase and 80 μL min−1 for the total aqueous phase were selected to generate droplets with suitable size and stability. It is worth noting that compared with the simulation results, the droplets could be produced and remain stable in the collection chip in the unstable range indicated by the simulation results, which should be attributed to the modification of the droplet collection chip and the surfactant added to the oil phase that increased the stability of the droplets.
To realize the drug screenings, the loading schemes of the aqueous phases should be programmable to produce droplets containing drug combinations with varying concentrations. Based on the given loading scheme of aqueous solutions, the formulations of the droplets could be determined by counting the order of the droplet in the channels of the S-shaped collection chip.
To verify the programmability of loading schemes, solutions containing different fluorescent dyes were adopted for quantification. As a demonstration, the 3-stepped loading scheme for a single drug was firstly validated (Table S5†). Briefly, the solution containing the red fluorescent dye was loaded from inlet II, and the flow rate increased from 10 μL min−1 to 50 μL min−1 at a rate of 20 μL min−1 in every 90 s. To maintain a constant total flow rate of the aqueous phase, pure water was loaded from inlet III and the flow rate was reduced from 50 μL min−1 to 10 μL min−1 at a rate of 20 μL min−1 in every 90 s. Besides, another pure water sample representing the bacteria suspension was introduced from inlet I, the flow rate of which remained constant at 20 μL min−1. In this way, droplets containing red fluorescent dye with varying concentrations could be generated. The results indicated that the fluorescence intensity of the droplets was linearly correlated with the generation step, verifying the feasibility of the 3-stepped loading scheme (Fig. S8a†). Secondly, a 5-stepped loading scheme for a single drug was validated to further verify the programmability of loading schemes (Table S6†). The results demonstrated that the fluorescence intensity of the droplets increased linearly with the generation step as well, which was consistent with the pattern of the loading scheme (Fig. 2d). Moreover, it was found that about 133 and 80 droplets containing the same concentration combination were produced at each step for parallel measurement in the 3- and 5-stepped loading schemes, respectively. The concentration variety of antibiotics could be further increased by adding steps in the loading schemes. For example, 6 concentrations per chip could be obtained by adopting a 6-stepped loading scheme (Table S7 and Fig. S8b†).
Afterwards, the 3- and 5-stepped loading schemes for drug combinations were also verified, where the solutions containing the red and blue fluorescent dyes were loaded from inlet II and inlet III, respectively, to emulate the different types of drug solutions. It was shown that droplets exhibiting red and blue fluorescence of different intensities were obtained according to the loading schemes. It was revealed that the red and blue fluorescence intensities of droplets were linearly correlated with the generation step, respectively, verifying the feasibility of the 3- and 5-stepped loading schemes for drug combinations (Fig. 2e and f). These results suggested that the composition of droplets could be tuned in a programmable way as well. Combinations containing more types of drugs could be achieved by replacing the six-way valves by valves with more inlets or the assembling of different valves. For example, combinations of 3 types of antibiotics that were represented and visualized by three types of fluorescent dyes (red, green and blue fluorescence) could be obtained by using seven-way valves assembled from Y-shaped and six-way valves, as shown in Fig. S8c and S9.†
3.4. Calibration of E. coli concentration and AI-aided image analysis
E. coli has been widely used in the laboratory for assessment of antibiotics.51,52 Herein, as a proof-of-concept study, it was selected as a model to verify the feasibility of the AI-HTCDES. In previous work, it has been validated that the gray value of the microscopy images of the bacteria colony is quantitatively correlated with the bacterial density.20 Hence, the gray value of the images for droplets containing bacteria was measured to calibrate the bacterial density in our work. Firstly, to determine quantitative relationships between the bacterial density and the gray value, droplets containing given bacterial densities were generated and sequentially collected in the S-shaped chip (Fig. 3a). The microscopy images of the droplets were taken and then manually segmented into droplets and background area without droplets. Subsequently, the gray value of the droplets at the time point of n hour (Gdn) and the gray value of the background area without droplets at the corresponding time point of n hour (Gbn) were determined to obtain the normalized gray value (Gn). | Gn = (Gbn − Gdn)/(Gb0 − Gd0) | (7) |
A linear relationship between the bacterial density and the gray value within the range of 2.3 × 106 to 1.84 × 109 CFU mL−1 was revealed (Fig. 3b). It complied with the fact that when the bacterial density increased, the transmittance of droplets decreased, and therefore the normalized gray value increased. To better quantify the bacterial density in the lower density range (2.3 × 106–4.6 × 107 CFU mL−1), the results in that window were fitted to a new linear regression, as shown in Fig. 3b. These results indicated that it was feasible to apply the gray value of the microscopy images of droplets to quantify the bacterial density.
 |
| Fig. 3 Calibration of E. coli concentration and AI-aided image analysis. (a) The bright field images of droplets with different bacterial densities (i–viii: 2.30 × 106 CFU mL−1, 2.30 × 107 CFU mL−1, 4.60 × 107 CFU mL−1, 1.84 × 108 CFU mL−1, 2.30 × 108 CFU mL−1, 4.60 × 108 CFU mL−1, 9.20 × 108 CFU mL−1, 1.84 × 109 CFU mL−1). The scale bar is 300 μm. (b) The quantitative relationship between the bacterial density and the gray value (y = 2.857x + 4.98, R2 = 0.9535, n = 13). The inset showing the quantitative relationship between the bacterial density and the gray value in the lower range as indicated by the dashed square (2.3 × 106 to 4.6 × 107 CFU mL−1, y = 3.777x + 3.46, R2 = 0.1318, n = 13). (c) Main architecture of the mask region-based convolutional neural network (Mask R-CNN). The network consists of a convolutional network (Conv. layers), a region proposal network (RPN), a RoIAlign layer, a predictor and a segmentation module. (d) Representative micrographs of the droplets containing E. coli at different time points, and the corresponding prediction result by the Mask R-CNN. The scale bar is 300 μm. (e) The classification loss curves for the training and validation data, respectively. (f) The total loss curves for the training and validation data, respectively. (g) The combined confusion matrix of the 4-fold cross-validation (CV) experiment to evaluate the effectiveness of the model. | |
The measurement based on conventional image analysis tools (e.g., ImageJ, Image-Pro, etc.) requires manual assistance, which is extremely time-consuming when the number of images is large. Since thousands of images were produced in our experiment (more than 7000 images), it would be time-consuming and error-prone to quantify each of them. Therefore, an AI-based module was developed to accelerate the image analysis process, which could automatically identify and segment the droplets in images, and then extract the average gray values of the droplets and background, respectively.
Deep learning, as an emerging area of research in the field of AI, was introduced to analyze the obtained images. Herein, 40 images of droplets containing varying densities of E. coli were randomly selected for training and validation. A 4-fold cross-validation (CV) experiment was implemented to verify the performance of our model. The Mask R-CNN model was adopted to identify and segment the droplets. Compared with the commonly used deep-learning-based image analysis algorithms, such as R-CNN, Fast R-CNN and Faster R-CNN, it could generate not only just the bounding boxes and the classifications of the detected objects, but also the masks of the objects, which improves the accuracy of segmentation. The main architecture of the Mask R-CNN is shown in Fig. 3c.53 It consisted of a convolutional network (Conv. layers), a region proposal network (RPN), a RoIAlign layer, a predictor and a segmentation module. The convolutional network, which comprised several ResNet-50 convolutional layers, was used to extract the feature maps of droplets. The RPN revealed the proposed areas that contained droplets. Then the RoIAlign layer combined the proposed areas with the feature maps and sent them to the predictor. Eventually, the segmentation module divided the droplets from the background according to the recognition and classification results generated by preceding processes.
These steps were repeated several times to optimize and adjust the size of the bounding box that precisely enclosed the droplets. As shown in Fig. 3d, the droplets could be effectively identified and segmented. The percentages in Fig. 3d represented the possibility that the bounding box contained a droplet, which was credible enough to be accepted when it is greater than 70%. Based on the identification results, a segmentation module was carried out for dividing the droplets from the background and calculating the gray values respectively.
The classification loss, which represents the precision of the classification for each bounding box, decreased to 3.50 × 10−3 after 3000 epochs (Fig. 3e). The total loss of the model, which includes the loss of the RPN and the loss of the Mask R-CNN predictor, indicates the total deviation of the model. In the experiment, it decreased rapidly to 2.30 × 10−2 after 3000 epochs (Fig. 3f). The bounding box regression loss, which evaluates the consistency between the bounding box and the shapes of droplets, decreased to 5.71 × 10−3 in 15 minutes (Fig. S10†). Therefore, these results indicated the viability and robustness of the framework of the Mask R-CNN for the identification and the segmentation of the droplets.
According to the results of the model recognition performance obtained by identification and segmentation of the images, the confusion matrix was obtained (Fig. 3g). The accuracy based on the validation dataset reached 95.0 ± 5.8%, implying that the Mask R-CNN fine-tuned on the droplet image dataset could identify the droplets precisely. Furthermore, by adjusting the structure of the neural network and training the neural network with images containing diverse droplets, such as images containing a distorted-shaped droplet or multiple droplets, the accuracy of identification and the versatility could be further improved.54,55
3.5. Quantitative evaluation of single antibiotic-based monotherapy
Prior to the screening of antibiotic combinations, several representative antibiotics including gentamicin (one of the aminoglycosides), chloramphenicol (one of the amphenicols), tetracycline (one of the tetracyclines) and cefepime (one of the β-lactams) were selected for quantitative evaluation, respectively (Fig. 4a). Herein, droplets containing E. coli and each antibiotic of six concentration gradients were generated and collected using the AI-HTCDES. Then the growth of E. coli was monitored and recorded by bright-field imaging at multiple time points (Fig. 4b and S11–S13†). The obtained images were then analyzed in a high-throughput manner by the AI-based data analysis module. It was found that all four antibiotics with high concentrations exhibited a prominent inhibitory effect on E. coli growth (Fig. 4c and d), validating their antibiotic efficacies. They generally displayed dose-independent killing effects, but the effects were insignificant for some antibiotics when the concentrations were low (Fig. 4c and d). The results implied that the dose of antibiotics should be at a high enough concentration to be effective. Based on the growth curves of E. coli under varying conditions, the MICs were then calculated. The MICs of gentamicin, chloramphenicol, tetracycline and cefepime were determined to be 3.75 μg mL−1, 3.13 μg mL−1, 0.50 μg mL−1 and 31.25 ng mL−1, respectively (Fig. 4d), implying their diverse inhibitory effects. Among them, cefepime, which directly inhibits bacterial cell wall synthesis to exert its antibacterial effects, leading to bacteria swelling, rupture and autophagy,56 demonstrated the lowest MIC. Gentamicin, chloramphenicol and tetracycline, which exert bactericidal effects by disturbing mRNA translation, preventing bacterial protein synthesis, which leads to the defect of the cell membrane,57–59 showed distinctly higher MICs. These results indicated that antibiotics that directly disrupted the normal structure of bacteria had a greater impact on bacterial growth.
 |
| Fig. 4 Quantitative evaluation of single antibiotic-based monotherapy. (a) The schematics for loading the antibiotics (gentamicin, chloramphenicol, tetracycline or cefepime), E. coli suspension, LB solution and oil phase to form the droplets. (b) Bright field images of the droplets containing E. coli exposed to gentamicin at multiple time points. The scale bar is 300 μm. (c) Heat maps showing the time-lapse change of normalized E. coli density under treatment of different single antibiotics. i) Gentamicin (A–G: 0.00 μg mL−1, 0.50 μg mL−1, 0.75 μg mL−1, 1.50 μg mL−1, 2.25 μg mL−1, 2.50 μg mL−1, 3.75 μg mL−1); ii) chloramphenicol (A–G: 0.00 μg mL−1, 0.25 μg mL−1, 0.63 μg mL−1, 0.75 μg mL−1, 1.25 μg mL−1, 1.88 μg mL−1, 3.13 μg mL−1); iii) tetracycline (A–G: 0.00 μg mL−1, 0.05 μg mL−1, 0.10 μg mL−1, 0.15 μg mL−1, 0.25 μg mL−1, 0.30 μg mL−1, 0.50 μg mL−1); and iv) cefepime (A–G: 0.00 ng mL−1, 3.13 ng mL−1, 6.25 ng mL−1, 9.38 ng mL−1, 15.63 ng mL−1, 18.75 ng mL−1, 31.25 ng mL−1). (d) Plots showing the time-lapse change of normalized gray value of droplets under treatment of different single antibiotics. i) Gentamicin, ii) chloramphenicol, iii) tetracycline and iv) cefepime. n = 13, *P < 0.05, **P < 0.01, ***P < 0.001 and ****P < 0.0001. (e) IC50 of the four antibiotics. i) Gentamicin, ii) chloramphenicol, iii) tetracycline and iv) cefepime. | |
To further validate the results, the quantitative evaluation of these single antibiotics was carried out in the conventional well plates as well. The results indicated that the MICs of gentamicin, chloramphenicol, tetracycline and cefepime were 4.40 μg mL−1, 3.80 μg mL−1, 0.36 μg mL−1, and 3.80 ng mL−1, respectively (Fig. S14†), which showed high consistency with the results obtained by the AI-HTCDES, suggesting the effectiveness of our system in quantitative evaluation of single antibiotics.
IC50, a measure of the effectiveness of a compound in inhibiting biochemical activity, was calculated as well (Fig. 4e). The results demonstrated that cefepime showed the lowest IC50 (19.81 ng mL−1), gentamicin and chloramphenicol had comparable IC50 (2.97 μg mL−1 and 1.37 μg mL−1, respectively), and tetracycline exhibited an IC50 (0.17 μg mL−1) in between those of cefepime and chloramphenicol, which were consistent with the pattern of MICs.
3.6. Screenings of antibiotic combinations
Afterwards, the screening of drug combinations using the AI-HTCDES was explored. Three representative antibiotic combinations were selected for investigation, including gentamicin/chloramphenicol, gentamicin/tetracycline, and gentamicin/cefepime (Fig. 5a). Herein, droplets containing E. coli and each antibiotic combination of seven concentration gradients were generated and collected using the AI-HTCDES. Then the growth of E. coli was monitored and recorded by bright-field imaging at multiple time points (Fig. 5b and S15 and S16†). The obtained images were then analyzed in a high-throughput manner by the AI-based data analysis module. The results showed that the antibiotic combinations with varying concentrations exhibited diverse inhibitory effects (Fig. 5c). For example, all of the concentration combinations of gentamicin/chloramphenicol did not completely inhibit the growth of E. coli except for the concentration combination of 1.88 μg mL−1/0.42 μg mL−1, while gentamicin/tetracycline and gentamicin/cefepime could completely inhibit the growth of E. coli under multiple concentration combinations (gentamicin/tetracycline: 0.38 μg mL−1/0.25 μg mL−1, 1.13 μg mL−1/0.15 μg mL−1, 1.88 μg mL−1/0.05 μg mL−1; gentamicin/cefepime: 1.88 μg mL−1/3.13 ng mL−1, 2.50 μg mL−1/4.13 ng mL−1). Such results implied that the AI-HTCDES provided an effective approach for searching effective antibiotic combinations and their dosage regimens without relying on the prior knowledge and experience of operators.
 |
| Fig. 5 Screenings of antibiotic combinations. (a) The schematics for loading the antibiotic combinations (gentamicin/chloramphenicol, gentamicin/tetracycline, or gentamicin/cefepime), E. coli suspension and oil phase to form the droplets. (b) Bright field images of the droplets containing E. coli exposed to gentamicin/chloramphenicol at multiple time points (A–E represent concentrations of 0 μg mL−1/0 μg mL−1, 0.75 μg mL−1/0.62 μg mL−1, 0.38 μg mL−1/2.08 μg mL−1, 1.13 μg mL−1/1.25 μg mL−1 and 1.88 μg mL−1/0.42 μg mL−1, respectively). The scale bar is 300 μm. (c) Heat maps showing the time-lapse change of normalized E. coli density under treatment of different antibiotic combinations. i) Gentamicin/chloramphenicol (A–G: 0.00 μg mL−1/0.00 μg mL−1, 0.25 μg mL−1/1.04 μg mL−1, 0.75 μg mL−1/0.62 μg mL−1, 1.25 μg mL−1/0.21 μg mL−1, 0.38 μg mL−1/2.08 μg mL−1, 1.13 μg mL−1/1.25 μg mL−1 and 1.88 μg mL−1/0.42 μg mL−1); ii) gentamicin/tetracycline (A–G: 0.00 μg mL−1/0.00 μg mL−1, 0.25 μg mL−1/0.17 μg mL−1, 0.75 μg mL−1/0.10 μg mL−1, 1.25 μg mL−1/0.03 μg mL−1, 0.38 μg mL−1/0.25 μg mL−1, 1.13 μg mL−1/0.15 μg mL−1 and 1.88 μg mL−1/0.05 μg mL−1); iii) gentamicin/cefepime (A–G: 0.00 μg mL−1/0.00 ng mL−1, 0.38 μg mL−1/15.63 ng mL−1, 1.13 μg mL−1/9.38 ng mL−1, 1.88 μg mL−1/3.13 ng mL−1, 0.50 μg mL−1/20.63 ng mL−1, 1.50 μg mL−1/12.38 ng mL−1 and 2.50 μg mL−1/4.13 ng mL−1). (d) Plots showing the time-lapse change of normalized gray value of droplets under treatment of different antibiotic combinations. i) Gentamicin/chloramphenicol, ii) gentamicin/tetracycline and iii) gentamicin/cefepime. n = 13. The concentrations of A–G for each drug combination are the same as those in the heat maps of (c). | |
To further quantify their inhibitory effect, the FIC indices (see the Materials and methods section for details) were calculated according to the growth curves of E. coli under varying antibiotic combinations (Fig. 5d). The FIC indices for the combination of gentamicin/tetracycline, gentamicin/chloramphenicol and gentamicin/cefepime were determined to be 0.60, 0.63 and 0.60, respectively, suggesting the additive effect of the pairwise antibiotics. In most cases, clinical combination therapy exploits synergistic alliances to achieve higher therapeutic efficacy than either monotherapy,60 but additive combinations may also be selected for other reasons, like to decrease rates of resistance.61 The above findings suggested that the AI-HTCDES could provide useful guidance in the clinical use of antibiotic combination therapy.
4. Conclusions
In our work, an AI-HTCDES was developed for rapid, high-throughput and systematic screening of drug combinations. With this system, droplets containing the target bacteria and antibiotic combinations of varying types and concentrations were generated in a programmable and high-throughput manner. We acquired more than 7000 bright-field micrographs to record the growth state of bacteria and the gray value was demonstrated as an effective index to quantify the bacteria density. A Mask R-CNN model was constructed for image analysis to accelerate the screening process, which demonstrated a high accuracy of 95.0 ± 5.8%. As a proof-of-concept study, four antibiotics (i.e., gentamicin, cefepime, chloramphenicol and tetracycline) were firstly evaluated using the AI-HTCDES, respectively, by determining their IC50 and MIC. Furthermore, three antibiotic combinations (i.e., gentamicin/chloramphenicol, gentamicin/tetracycline, and gentamicin/cefepime) were quantitatively assessed using the AI-HTCDES, respectively. It was found that the combinations of gentamicin/chloramphenicol, gentamicin/cefepime and gentamicin/tetracycline displayed an additive effect. Meanwhile, the effective dosage regimens of each component in the combinations were discovered. This strategy not only provides useful guidance in the clinical use of antibiotic combination therapy and personalized medicine, but also offers a promising tool for the combinatorial screenings of other medicines. It also helps reveal the effect of complex antibacterial drug combinations on bacterial growth, disclose the interactive patterns between different antibiotics, and investigate the evolution of bacterial resistance under different antibiotic combinations. Currently, the assay time for the AI-HTCDES was 24 hours, which can be further shortened by reducing the time interval for imaging and using a CCD of higher sensitivity to distinguish the subtle change of the droplet gray values in the early stage of bacteria growth. In order to further scale up the screening process, multiple groups of channels can be integrated into one single chip and the channel length can be extended, which also benefits the screening of a large antibiotic library. To further increase the throughput, more advanced hardware and algorithms should be developed. For example, a large field-of-view camera with high resolution can be applied to capture images comprising a whole chip, so that the time for imaging will be greatly reduced and the deep-learning algorithms for droplet segmentation can be fully exploited. Also, algorithms that can intelligently recognize the position of droplets and then initiate microscopy imaging can be developed to automate and accelerate the process of image acquisition.
Author contributions
Deyu Yang: investigation, validation, data curation, formal analysis, visualization, writing – original draft. Ziming Yu: investigation, validation, data curation, formal analysis, visualization, writing – original draft. Mengxin Zheng: investigation. Wei Yang: investigation. Zhangcai Liu: investigation. Jianhua Zhou: methodology, writing – review & editing, resources, funding acquisition. Lu Huang: conceptualization, methodology, supervision, writing – review & editing, resources, funding acquisition. All authors discussed the results and contributed to the final manuscript.
Conflicts of interest
The authors declare that they have no known competing financial interests or personal relationships that could have appeared to influence the work reported in this paper.
Acknowledgements
This work was financially supported by the National Natural Science Foundation of China (No. 22004135), Guangdong Basic and Applied Basic Research (Grant No. 2023A1515010647) and the Shenzhen Science and Technology Program (Grant No. RCBS20210706092409020, JCYJ20190807160401657 and JCYJ20190807160415074).
References
- J. M. Stokes, A. J. Lopatkin, M. A. Lobritz and J. J. Collins, Bacterial metabolism and antibiotic efficacy, Cell Metab., 2019, 30, 251–259, DOI:10.1016/j.cmet.2019.06.009
.
- Y. X. Li, X. Y. Xia, X. H. Li, K. Xiao and X. W. Zhuang, Correlation between the use of antibiotics and development of a resistant bacterial infection in patients in the ICU, BioSci. Trends, 2018, 12, 517–519, DOI:10.5582/bst.2018.01130
.
- S. Kaur, S. Hariharan and S. Dharmaraj, Superbugs: the powerful warriors, Int. J. Pharma Sci. Res., 2020, 11, 1506–1526, DOI:10.13040/ijpsr.0975-8232.11(4).1506-26
.
- S. Begum, A. Pramanik, K. Gates, Y. Gao and P. C. Ray, Antimicrobial peptide-conjugated MoS2-based nanoplatform for multimodal synergistic inactivation of superbugs, ACS Appl. Bio Mater., 2019, 2, 769–776, DOI:10.1021/acsabm.8b00632
.
- P. J. Stogios and A. Savchenko, Molecular mechanisms of vancomycin resistance, Protein Sci., 2020, 29, 654–669, DOI:10.1002/pro.3819
.
- J. Dawan and J. Ahn, Effectiveness of antibiotic combination treatments to control heteroresistant salmonella typhimurium, Microb. Drug Resist., 2021, 27, 441–449, DOI:10.1089/mdr.2020.0027
.
- E. J. Zheng, J. M. Stokes and J. J. Collins, Eradicating bacterial persisters with combinations of strongly and weakly metabolism-dependent antibiotics, Cell Chem. Biol., 2020, 27, 1544–1552, DOI:10.1016/j.chembiol.2020.08.015
.
- C. Ruppen, A. Lupo, L. Decosterd and P. Sendi, Is penicillin plus gentamicin synergistic against clinical group B streptococcus isolates?: an in vitro study, Front. Microbiol., 2016, 7, 1680, DOI:10.3389/fmicb.2016.01680
.
- J. A. Karlowsky, P. R. S. Lagace-Wiens, N. M. Laing, M. R. Baxter, H. J. Adam and G. G. Zhanel, Susceptibility of clinical isolates of Escherichia coli to fosfomycin as measured by four in vitro testing methods, J. Clin. Microbiol., 2020, 58, e01306–e01320, DOI:10.1128/JCM.01306-20
.
- X. Q. Tang, Y. L. Shen, X. N. Song, M. Benghezal, B. J. Marshall, H. Tang and H. Li, Reassessment of the broth microdilution method for susceptibility testing of Helicobacter pylori, J. Infect. Dis., 2020, 226, S486–S492, DOI:10.1093/infdis/jiac389
.
- A. Turlej-Rogacka, B. B. Xavier, L. Janssens, C. Lammens, O. Zarkotou, S. Pournaras, H. Goossens and S. Malhotra-Kumar, Evaluation of colistin stability in agar and comparison of four methods for MIC testing of colistin, Eur. J. Clin. Microbiol. Infect. Dis., 2018, 37, 345–353, DOI:10.1007/s10096-017-3140-3
.
- S. Sheffer-Levi, A. Rimon, V. Lerer, T. Shlomov, S. Coppenhagen-Glazer, C. Rakov, T. Zeiter, R. Nir-Paz, R. Hazan and V. Molcho-Pessach, Antibiotic susceptibility of cutibacterium acnes strains isolated from israeli acne patients, Acta Derm.-Venereol., 2020, 100, 1–8, DOI:10.2340/00015555-3654
.
- M. Miftahussurur, K. A. Fauzia, I. A. Nusi, P. B. Setiawan, A. F. Syam, L. A. Waskito, D. Doohan, N. Ratnasari, A. Khomsan, I. K. Adnyana, J. Akada and Y. Yamaoka, E-test versus agar dilution for antibiotic susceptibility testing of Helicobacter pylori: a comparison study, BMC Res. Notes, 2020, 13, 22, DOI:10.1186/s13104-019-4877-9
.
- J. Chen, M. Tomasek, A. Cruz, M. L. Faron, D. K. Liu, W. H. Rodgers and V. Gau, Feasibility and potential significance of rapid in vitro qualitative phenotypic antimicrobial susceptibility testing of gram-negative bacilli with the ProMax system, PLoS One, 2021, 16, e0249203, DOI:10.1371/journal.pone.0249203
.
- J. Zhai, C. Li, H. Li, S. Yi, N. Yang, K. Miao, C. Deng, Y. Jia, P. I. Mak and R. P. Martins, Cancer drug screening with an on-chip multi-drug dispenser in digital microfluidics, Lab Chip, 2021, 21, 4749–4759, 10.1039/d1lc00895a
.
- H. Zhang, A. R. Guzman, J. A. Wippold, Y. Li, J. Dai, C. Huang and A. Han, An ultra high-efficiency droplet microfluidics platform using automatically synchronized droplet pairing and merging, Lab Chip, 2020, 20, 3948–3959, 10.1039/d0lc00757a
.
- G. Du, Q. Fang and J. M. den Toonder, Microfluidics for cell-based high throughput screening platforms - a review, Anal. Chim. Acta, 2016, 903, 36–50, DOI:10.1016/j.aca.2015.11.023
.
- Z. Liu, H. Sun and K. Ren, A multiplexed, gradient-gased, full-hydrogel microfluidic platform for rapid, high-throughput antimicrobial susceptibility testing, ChemPlusChem, 2017, 82, 792–801, DOI:10.1002/cplu.201600654
.
- H. Sun, Z. Liu, C. Hu and K. Ren, Cell-on-hydrogel platform made of agar and alginate for rapid, low-cost, multidimensional test of antimicrobial susceptibility, Lab Chip, 2016, 16, 3130–3138, 10.1039/c6lc00417b
.
- S. Kim, F. Masum, J. K. Kim, H. J. Chung and J. S. Jeon, On-chip phenotypic investigation of combinatory antibiotic effects by generating orthogonal concentration gradients, Lab Chip, 2019, 19, 959–973, 10.1039/c8lc01406j
.
- Z. Wang, M. C. Kim, M. Marquez and T. Thorsen, High-density microfluidic arrays for cell cytotoxicity analysis, Lab Chip, 2007, 7, 740–745, 10.1039/b618734j
.
- A. Kulesa, J. Kehe, J. E. Hurtado, P. Tawde and P. C. Blainey, Combinatorial drug discovery in nanoliter droplets, Proc. Natl. Acad. Sci. U. S. A., 2018, 115, 6685–6690, DOI:10.1073/pnas.1802233115
.
- A. M. Kaushik, K. Hsieh, L. Chen, D. J. Shin, J. C. Liao and T. H. Wang, Accelerating bacterial growth detection and antimicrobial susceptibility assessment in integrated picoliter droplet platform, Biosens. Bioelectron., 2017, 97, 260–266, DOI:10.1016/j.bios.2017.06.006
.
- S. Jakiela, T. S. Kaminski, O. Cybulski, D. B. Weibel and P. Garstecki, Bacterial growth and adaptation in microdroplet chemostats, Angew. Chem., Int. Ed., 2013, 52, 8908–8911, DOI:10.1002/anie.201301524
.
- K. Churski, T. S. Kaminski, S. Jakiela, W. Kamysz, W. Baranska-Rybak, D. B. Weibel and P. Garstecki, Rapid screening of antibiotic toxicity in an automated microdroplet system, Lab Chip, 2012, 12, 1629–1637, 10.1039/c2lc21284f
.
- W. Han and X. Chen, A review on microdroplet generation in microfluidics, J. Braz. Soc. Mech. Sci. Eng., 2021, 43, 247, DOI:10.1007/s40430-021-02971-0
.
- Y. Wei, Y. Zhu and Q. Fang, Nanoliter quantitative high-throughput screening with large-scale tunable gradients based on a microfluidic droplet robot under unilateral dispersion mode, Anal. Chem., 2019, 91, 4995–5003, DOI:10.1021/acs.analchem.8b04564
.
- H. Li, P. Zhang, K. Hsieh and T.-H. Wang, Combinatorial nanodroplet platform for screening antibiotic combinations, Lab Chip, 2022, 22, 621–631, 10.1039/d1lc00865j
.
- J. Kehe, A. Kulesa, A. Ortiz, C. M. Ackerman, S. G. Thakku, D. Sellers, S. Kuehn, J. Gore, J. Friedman and P. C. Blainey, Massively parallel screening of synthetic microbial communities, Proc. Natl. Acad. Sci. U. S. A., 2019, 116, 12804–12809, DOI:10.1073/pnas.1900102116
.
- F. Eduati, R. Utharala, D. Madhavan, U. P. Neumann, T. Longerich, T. Cramer, J. Saez-Rodriguez and C. A. Merten, A microfluidics platform for combinatorial drug screening on cancer biopsies, Nat. Commun., 2018, 9, 2434, DOI:10.1038/s41467-018-04919-w
.
- S. Jakiela, T. S. Kaminski, O. Cybulski, D. B. Weibel and P. Garstecki, Bacterial growth and adaptation in microdroplet chemostats, Angew. Chem., Int. Ed., 2013, 52, 8908–8911, DOI:10.1002/anie.201301524
.
- C. Li, Q. Ke, C. Yao, C. Yao, Y. Mi, M. Wu and L. Ge, Comparison of bipolar and unipolar pulses in cell electrofusion: simulation and experimental research, IEEE Trans. Biomed. Eng., 2019, 66, 1353–1360, DOI:10.1109/TBME.2018.2872909
.
- S. B. Jin, G. Y. Ye, N. Cao, X. L. Liu, L. G. Dai, P. P. Wang, T. Wang and X. Y. Wei, Acoustics-controlled microdroplet and microbubble fusion and its application in the synthesis of hydrogel microspheres, Langmuir, 2022, 38, 12602–12609, DOI:10.1021/acs.langmuir.2c02080
.
- S. K. Fan, P. W. Huang, T. T. Wang and Y. H. Peng, Cross-scale electric manipulations of cells and droplets by frequency-modulated dielectrophoresis and electrowetting, Lab Chip, 2008, 8, 1325–1331, 10.1039/b803204a
.
- H. Z. Sun, Y. K. Ren, Y. Tao, T. Y. Jiang and H. Y. Jiang, Flexible online in-droplet cell/synthetic particle concentration utilizing alternating current electrothermal-flow field-effect transistor, Lab Chip, 2021, 21, 1987–1997, 10.1039/d0lc01328e
.
- B. Chen, J. Dong, M. Ruelas, X. Ye, J. He, R. Yao, Y. Fu, Y. Liu, J. Hu, T. Wu, C. Zhou, Y. Li, L. Huang, Y. S. Zhang and J. Zhou, Artificial intelligence-assisted high-throughput screening of printing conditions of hydrogel architectures for accelerated diabetic wound healing, Adv. Funct. Mater., 2022, 32, 2201843, DOI:10.1002/adfm.202201843
.
- J. He, J. Zhou, J. Dong, Z. Su and L. Huang, Revealing the effects of microwell sizes on the crystal growth kinetics of active pharmaceutical ingredients by deep learning, Chem. Eng. J., 2022, 428, 131986, DOI:10.1016/j.cej.2021.131986
.
- L. Huang, Z. Liu, J. He, J. Li, Z. Wang, J. Zhou and Y. Chen, AI-aided high-throughput profiling of single-cell migration and proliferation on addressable dual-nested microwell arrays, Cell Rep. Phys. Sci., 2023, 4, 101276, DOI:10.1016/j.xcrp.2023.101276
.
- L. Huang, D. Yang, Z. Yu, J. He, Y. Chen and J. Zhou, Deep learning-aided high-throughput screening of time-resolved protein crystallization on programmable microliter-droplet systems, Chem. Eng. J., 2022, 450, 138267, DOI:10.1016/j.cej.2022.138267
.
- A. M. Patil, M. D. Patil and G. K. Birajdar, White blood cells image classification using deep learning with canonical correlation analysis, IRBM, 2021, 42, 378–389, DOI:10.1016/j.irbm.2020.08.005
.
- R. M. Roy and P. M. Ameer, Segmentation of leukocyte by semantic segmentation model: a deep learning approach, Biomed. Signal Process. Control, 2021, 65, 102385, DOI:10.1016/j.bspc.2020.102385
.
- C. Belthangady and L. A. Royer, Applications, promises, and pitfalls of deep learning for fluorescence image reconstruction, Nat. Methods, 2019, 16, 1215–1225, DOI:10.1038/s41592-019-0458-z
.
- H. Wang, Y. Rivenson, Y. Jin, Z. Wei, R. Gao, H. Gunaydin, L. A. Bentolila, C. Kural and A. Ozcan, Deep learning enables cross-modality super-resolution in fluorescence microscopy, Nat. Methods, 2019, 16, 103–110, DOI:10.1038/s41592-018-0239-0
.
- L. Jakobsen, D. Sandvang, V. F. Jensen, A. M. Seyfarth, N. Frimodt-Møller and A. M. Hammerum, Gentamicin susceptibility in Escherichia coli related to the genetic background: problems with breakpoints, Clin. Microbiol. Infect., 2007, 13, 830–832, DOI:10.1111/j.1469-0691.2007.01751.x
.
- M. A. S. Moreira, J. A. Oliveira, L. M. Teixeira and C. A. Moraes, Detection of a chloramphenicol efflux system in Escherichia coli isolated from poultry carcass, Vet. Microbiol., 2005, 109, 75–81, DOI:10.1016/j.vetmic.2005.04.012
.
- A. K. Kidsley, S. Abraham, J. M. Bell, M. O'Dea, T. J. Laird, D. Jordan, P. Mitchell, C. A. McDevitt and D. J. Trott, Antimicrobial susceptibility of Escherichia coli and Salmonella spp. Isolates from healthy pigs in Australia: results of a pilot national survey, Front. Microbiol., 2018, 9, 1207, DOI:10.3389/fmicb.2018.01207
.
- Y. Hamada, C. A. Sutherland and D. P. Nicolau, Impact of revised cefepime CLSI breakpoints on Escherichia coli and Klebsiella pneumoniae susceptibility and potential impact if applied to Pseudomonas aeruginosa, J. Clin. Microbiol., 2015, 53, 1712–1714, DOI:10.1128/JCM.03652-14
.
- C. G. Rivera, P. P. Narayanan, R. Patel and L. L. Estes, Impact of cefepime susceptible-dose-dependent MIC for Enterobacteriaceae on reporting and prescribing, Antimicrob. Agents Chemother., 2016, 60, 3854–3855, DOI:10.1128/aac.00442-16
.
- B. Rasmussen, H. F. Noller, G. Daubresse, B. Oliva, Z. Misulovin, D. M. Rothstein, G. A. Ellestad, Y. Gluzman, F. P. Tally and I. Chopra, Molecular basis of tetracycline action: identification of analogs whose primary target is not the bacterial ribosome, Antimicrob. Agents Chemother., 1991, 35, 2306–2611, DOI:10.1128/aac.35.11.2306
.
- J. Parada, M. Diaz, E. Hermosilla, J. Vera, G. Tortella, A. B. Seabra, A. Quiroz, E. Hormazabal and O. Rubilar, Synthesis and antibacterial activity of manganese-ferrite/silver nanocomposite combined with two essential oils, Nanomaterials, 2022, 12, 2137, DOI:10.3390/nano12132137
.
- N. Bongaerts, Z. Edoo, A. A. Abukar, X. Song, S. Sosa-Carrillo, S. Haggenmueller, J. Savigny, S. Gontier, A. B. Lindner and E. H. Wintermute, Low-cost anti-mycobacterial drug discovery using engineered E. coli, Nat. Commun., 2022, 13, 3905, DOI:10.1038/s41467-022-31570-3
.
- R. C. I. Azucena, C. L. J. Roberto, Z. R. Martin, C. Z. Rafael, H. H. Leonardo, T. P. Gabriela and C. R. Araceli, Drug susceptibility testing and synergistic antibacterial activity of curcumin with antibiotics against enterotoxigenic Escherichia coli, Antibiotics, 2019, 8, 43, DOI:10.3390/antibiotics8020043
.
- W. K. Jia, Y. Y. Tian, R. Luo, Z. H. Zhang, J. Lian and Y. J. Zheng, Detection and segmentation of overlapped fruits based on optimized mask R-CNN application in apple harvesting robot, Comput. Electron. Agric., 2020, 172, 105380, DOI:10.1016/j.compag.2020.105380
.
- Z. Cai and N. Vasconcelos, Cascade R-CNN: high quality object detection and instance segmentation, IEEE Trans. Pattern Anal. Mach. Intell., 2021, 43, 1483–1498, DOI:10.1109/TPAMI.2019.2956516
.
- Z. Zhou, M. M. R. Siddiquee, N. Tajbakhsh and J. Liang, UNet++: redesigning skip connections to exploit multiscale features in image segmentation, IEEE Trans. Med. Imaging, 2020, 39, 1856–1867, DOI:10.1109/TMI.2019.2959609
.
- L. B. Barradell and H. M. Bryson, Cefepime: a review of its antibacterial activity, pharmacokinetics properties and therapeutic use, Drugs, 1994, 47, 471–505, DOI:10.2165/00003495-199447030-00007
.
- N. Izghirean, C. Waidacher, C. Kittinger, M. Chyba, G. Koraimann, B. Pertschy and G. Zarfel, Effects of ribosomal protein S10 flexible loop mutations on tetracycline and tigecycline susceptibility of escherichia coli, Front. Microbiol., 2021, 12, 663835, DOI:10.3389/fmicb.2021.663835
.
- M. E. O'Sullivan, Y. Song, R. Greenhouse, R. Lin, A. Perez, P. J. Atkinson, J. P. MacDonald, Z. Siddiqui, D. Lagasca, K. Comstock, M. E. Huth, A. G. Cheng and A. J. Ricci, Dissociating antibacterial from ototoxic effects of gentamicin C-subtypes, Proc. Natl. Acad. Sci. U. S. A., 2020, 117, 32423–32432, DOI:10.1073/pnas.2013065117
.
- C. Kirschmann and B. D. Davis, Phenotypic suppression in Escherichia coli by chloramphenicol and other reversible inhibitors of the ribosome, J. Bacteriol., 1969, 98, 152–159, DOI:10.1128/jb.98.1.152-159.1969
.
- F. T. Fadare, E. A. E. Elsheikh and A. I. Okoh, In vitro assessment of the combination of antibiotics against some integron-harbouring enterobacteriaceae from environmental sources, Antibiotics, 2022, 11, 1090, DOI:10.3390/antibiotics11081090
.
- G. J. Sullivan, N. N. Delgado, R. Maharjan and A. K. Cain, How antibiotics work together: molecular mechanisms behind combination therapy, Curr. Opin. Microbiol., 2020, 57, 31–40, DOI:10.1016/j.mib.2020.05.012
.
|
This journal is © The Royal Society of Chemistry 2023 |
Click here to see how this site uses Cookies. View our privacy policy here.