DOI:
10.1039/D4NR05046K
(Paper)
Nanoscale, 2025, Advance Article
Enzyme-free optical detection of uric acid using corona phase molecular recognition in near-infrared fluorescent single-walled carbon nanotubes†
Received
1st December 2024
, Accepted 17th March 2025
First published on 7th April 2025
Abstract
Real-time monitoring of uric acid (UA) is essential for preventing and managing chronic diseases. While enzymatic methods are the clinical standard, they are limited by slow reaction times and strict environmental requirements. Enzyme-free sensors offer alternatives but face challenges in analyte selectivity and clear optical signal readouts. In this study, we developed an enzyme-free UA detection method based on corona phase molecular recognition (CoPhMoRe). We developed a near-infrared (NIR) optical nanosensor by functionalizing single-walled carbon nanotubes (SWCNTs) with single-stranded DNA (ssDNA). Through high-throughput screening, we identified the optimal corona of the sensor as (AAT)10/SWCNT and further optimized it with studying the pH effects to account for the natural pH variation in urine. To facilitate point-of-care (POC) application, we integrated the corona nanosensor with an optical paper strip, achieving a rapid and intense turn-on NIR response up to 4 times stronger when exposed to urine samples containing UA concentrations ranging from 5.7 to 500 μM. Moreover, the corona nanosensor-integrated paper strip exhibited reliable performance even under harsh conditions of 70% relative humidity and 40 °C. This study demonstrates the enzyme-free UA detection capability of SWCNT-based CoPhMoRe in complex biofluids, establishing its potential for future application as an optical test strip in POC diagnostics.
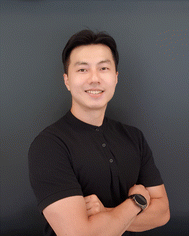 Soo-Yeon Cho | Soo-Yeon Cho is an associate professor of Chemical Engineering at Sungkyunkwan University (SKKU). He received his B.S. and Ph.D. degrees in Chemical Engineering from KAIST in 2019. He was a postdoctoral associate at MIT (2019–2022) and a visiting scholar at UC Berkeley (2016). Prof. Cho received the Innovator Under 35 Asia Pacific (TR35) award from MIT Technology Review in 2023 and the Rising Star in Measurement Science award from ACS in 2024. His research focuses on developing advanced chemical and biological sensor systems for innovative analytical platforms that support smart chemical production and precision medicine. |
Introduction
Uric acid (UA), the product of metabolizing purine-rich foods, has low solubility and readily forms white crystals.1,2 Elevated UA levels can result in hyperuricemia, which is strongly linked to gout. Gout arises when excessive UA in the bloodstream accumulates in joints, forming crystals that incite inflammation.3 This inflammatory response leads to swelling, pain, and immune activation in the affected joints. Moreover, hyperuricemia is associated with crystal deposition in the kidneys, contributing to kidney stone formation, and is linked to a 30% increased risk of hypertension and ischemic stroke, highlighting its significant health implications.4–6 Given these risks, persistently high UA levels can lead to chronic diseases, emphasizing the need for continuous monitoring to aid in disease prevention and effective management of UA levels.
In clinical practice, enzyme-based detection remains the gold standard for UA monitoring (Fig. 1a). This method relies on enzymes such as uricase and peroxidase. Uricase catalyzes the oxidation of UA to allantoin, and then peroxidase produces a measurable color change through chromogenic reactions with the by-product hydrogen peroxide.7,8 Current at-home test kits are commonly composed of test strips based on colorimetry, whereas in hospitals, more accurate diagnoses are achieved by measuring the ultraviolet (UV) absorbance difference during the enzymatic UA conversion.9 Electrochemical sensors employing enzymatic methods have also been developed for point-of-care (POC) application.10 These enzymatic methods provide high sensitivity and broad linear response, facilitating early detection of UA, and remain the primary approach for clinical diagnosis.11 Despite its widespread use, this approach faces notable limitations. The enzymatic process requires a prolonged reaction time, typically around 30 minutes, which hinders its suitability for real-time monitoring.12 Enzyme activity also highly depends on environmental factors such as temperature, ionic strength of the buffer, and enzyme concentration, as it requires incubation at 37 °C for enzyme activity.13 In addition, peroxidase reactions can lack specificity, leading to interference from other substances, and result in a short shelf life due to its incompatibility with preservatives such as sodium azide.14,15
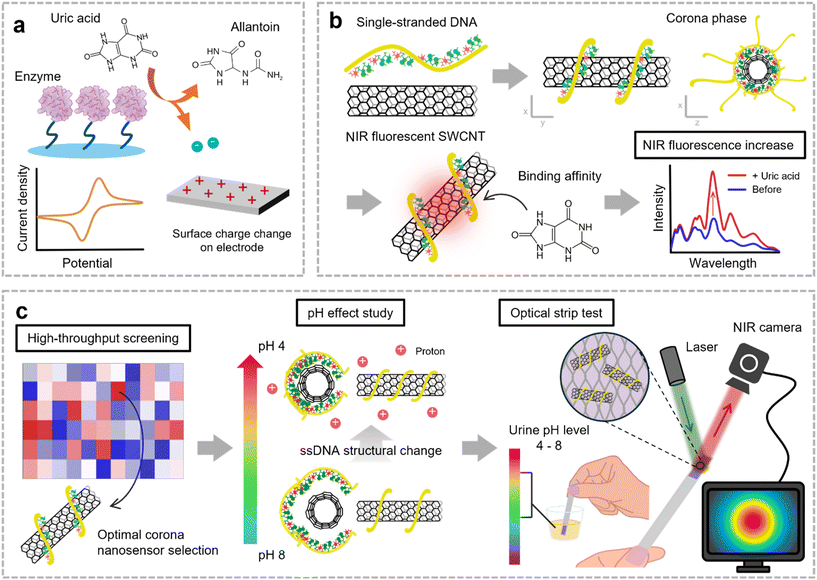 |
| Fig. 1 Schematic illustrations of the enzyme-free UA monitoring through CoPhMoRe of NIR fluorescent SWCNTs. (a) Conventional UA detection method based on the enzymatic reaction converting UA to allantoin. (b) Design of ssDNA/SWCNT nanosensors for enzyme-free UA detection utilizing CoPhMoRe. (c) Overall workflow for optimizing an optical strip integrated with the corona nanosensor for UA detection in human urine with a wide pH range. | |
To overcome these limitations, enzyme-free electrochemical sensing methods have gained attention. Materials such as metal oxides, noble metals, and carbon-based materials are commonly employed for their catalytic properties in UA oxidation, with a porous structure enhancing the surface-to-volume ratio.10,16 However, this approach faces challenges due to signal interference from mixed analytes with similar oxidation–reduction potentials, such as ascorbic acid and tyrosine.17 External factors such as solution pH, ion concentration, and temperature further affect signal reliability. Additionally, electrochemical methods lack imaging capabilities, which highlights the need for POC diagnostic systems incorporating optical transduction for easy and reliable visualization. Therefore, sensor constructs that enable enzyme-free molecular recognition can serve as an effective POC solution for UA monitoring, overcoming the existing limitations.18–20
In this study, we developed an enzyme-free UA detection technique using corona phase molecular recognition (CoPhMoRe).21 The amphiphilic moieties of a synthetic polymer form a three-dimensional (3D) structure resembling a corona phase on the surface of nanomaterials, creating a binding pocket for specific molecules. CoPhMoRe nanosensors corroborate their potential for detecting a wide range of target molecules, from small molecules to oligonucleotides and macromolecules, including even proteins or pathogens.22–25 We designed a nanosensor library by generating synthetic binding sites on near-infrared (NIR) fluorescent single-walled carbon nanotubes (SWCNTs) through functionalization with single-stranded DNA (ssDNA) (Fig. 1b). In particular, when ssDNA is used as the functionalizing agent, its binding affinity and surface coverage on SWCNTs is highly sequence-dependent, resulting in uniquely adaptable 3D molecular recognition sites.26–28 Our CoPhMoRe library was then subjected to high-throughput screening to evaluate each nanosensor's ability to detect UA with selectivity and specificity among six common analytes in urine. Considering the pH fluctuations of human urine, we also investigated the effects of analyte pH on the corona phase of the SWCNT nanosensor by analyzing changes in the surface zeta potential and surface coverage. The optimized nanosensor was then immobilized onto a porous paper membrane, forming a practical optical paper strip (Fig. 1c). Based on the findings in this study, we suggest the potential of corona phase SWCNT nanosensors as a practical and efficient strip test method to detect specific molecules such as UA in complex biological fluids such as urine.
Results and discussion
Synthesis and characterization of the corona nanosensor for UA detection
The SWCNT powder produced through the high-pressure carbon monoxide (HiPCO) process was dispersed in a design library of 15 ssDNA to form corona phases on the sidewalls of SWCNTs. Due to the varying degrees of polymer binding affinity and the distinct corona phase structures formed depending on the CNT chirality, we utilized HiPCO SWCNTs, which contain a mixture of multiple chiralities, to ensure comprehensive coverage of binding interactions.29,30 Considering that UA has a molecular mass of 168 Da and a chemical structure similar to that of neurotransmitters, particularly in its heterocyclic structure and hydrogen bonding capacity, we selected ssDNA as the wrapping material with non-covalent functionalization.31–34 The hydrophobic bases of ssDNA engage in strong π–π stacking with the SWCNT surface, while the hydrophilic ssDNA backbone enables supramolecular interactions with target molecules.35 We employed mutational base sequences, fixing the total sequence lengths to either 30 or 32 nucleotides, to generate a structurally diverse library of corona phases. The ssDNA sequences and analytes used in this study are summarized in Tables S1 and S2,† respectively. The resulting dispersions of ssDNA/SWCNT were characterized by ultraviolet-visible-near-infrared (UV-Vis-NIR) absorption spectroscopy (Fig. 2a). The spectra confirmed the successful suspension of SWCNTs, as evidenced by the distinct transition peaks in the short-wave infrared region E11 and the visible wavelength region E22. The chiralities of SWCNTs were identified by referencing previous studies.36–38
 |
| Fig. 2 Design and characterization of the ssDNA/SWCNT corona nanosensor for enzyme-free UA detection. (a) UV-Vis-NIR absorption spectra of the ssDNA/SWCNT nanosensor library featuring over 10 different SWCNT chirality types. (b) High-throughput sensor screening results presented as an NIR fluorescence response heatmap for UA detection, alongside responses of creatine (Cr), glucose (Glu), lactic acid (LA), tyrosine (Tyr), and urea (U). The blue dashed rectangle indicates the optimal nanosensor for UA detection, (AAT)10/SWCNT. (c) NIR fluorescence spectra of the (AAT)10/SWCNT nanosensor in response to the six analytes and buffer. (d) Calibration curve of the (AAT)10/SWCNT nanosensor with the UA concentration ranging from 0.01 to 100 μM in DI water and from 5.7 to 73.7 μM in pooled human urine (n = 3). (e) Comparison of the (AAT)10/SWCNT nanosensor response from pooled human urine spiked with 5.7 and 73.7 μM UA with the expected value from the calibration curve using UA in DI water (n = 3). | |
We conducted high-throughput screening of the constructed ssDNA/SWCNT sensor library (n = 15) against six representative human urinary components—creatine (Cr), glucose (Glu), lactic acid (LA), tyrosine (Tyr), urea (U), and UA—to identify a sensor with selective sensitivity to UA (Fig. 2b).39,40 The sensor response was measured based on the NIR fluorescence emission of the library after 30 min of incubation with each analyte at a concentration of 100 μM. Given the low solubility of UA in water, approximately 360 μM, and its strong dependence on ion composition and temperature, the maximum concentration was set to 100 μM to ensure the use of a stable and fully dissolved solution during the experiments.41,42 The screening results revealed a selective response for UA, showing an average 75% increase in the NIR fluorescence intensity for the (AAT)10/SWCNT nanosensor (sensor ii). UA induces on-responses in all of our libraries, presumably by acting as a reducing agent though electron donation or passivation of defective sites on the SWCNT surface.43 The particularly strong turn-on response was achieved through the uniquely formed corona structure, depending on the DNA sequence, when the surface coverage and hydrodynamic radius of the 3D structure were optimized for UA. All sensor responses were calculated using the formula (I − I0)/I0, where I represents the integrated area of the NIR spectrum with the analyte and I0 represents the area in DI water in the absence of the analyte. The responses were quantified considering all chiralities that show NIR fluorescence between 950 and 1400 nm. For measuring NIR fluorescence, a 721 nm laser was used as the excitation source, which exhibits the strongest and most distinct fluorescence, while simultaneously exciting multiple other chiralities.44 The NIR fluorescence emission spectra with the 721 nm laser demonstrated that the (AAT)10/SWCNT nanosensor is primarily composed of (6,5), (7,6), (8,3), and (9,4) chiral SWCNTs (Fig. 2c).32,36–38
We then evaluated the sensitivity and selectivity of the (AAT)10/SWCNT nanosensor over 0.01–100 μM of UA in DI water and pooled human urine from six different donors (Fig. 2d and Table S3†). The UA concentrations of the actual urine samples were determined using high-performance liquid chromatography (HPLC) (Fig. S1†). We performed a pretreatment process to oxidize ascorbic acid, a major interfering molecule in urine, thereby preventing false negative results.45 Calibration curves were plotted and fitted using Hill's model, and the limit of detection (LOD) was calculated as 69 nM with a dynamic sensing range of 1.66–169 μM in DI water and 163 nM with a dynamic range of 2.85–95.6 μM in human urine.46 The LOD was determined by defining the noise level as the nanosensor response in the absence of UA and calculating three times the signal-to-noise ratio. The dynamic range was defined as the UA concentration range exhibiting a linear response change determined by UA concentrations corresponding to 10% and 90% sensor responses in each fitting model. Despite the potential interference from variations in urine composition among six different donors, the nanosensor exhibited a selective response that consistently increased with increasing UA concentration without significant deviation. Notably, these detection limits are significantly lower than the hyperuricemia diagnostic threshold (approximately 400 μM for males and 350 μM for females in serum, and 600 mg day−1 in urine), demonstrating its potential for clinical monitoring.47–49 Compared to water, UA exhibits higher solubility in urine, reaching approximately 571 μM, due to pH variations and the presence of salts and ions.41,50
The difference in the nanosensor performance between the DI water-based and urine-based systems can be quantitatively assessed by comparing the experimental sensor response from human urine samples with the expected values derived from the calibration curve measured with UA in DI water (Fig. 2e). When comparing the expected values from the calibration curve using UA in DI water with the experimental sensor response using human urine, the difference was 8% at the lowest UA concentration as 5.7 μM but significantly decreased to 0.5% at the highest concentration as 73.7 μM. These marginal differences might be due to the interferences by the reference molecules and pH variation in human urine. As a result, these findings validate the functionality and selectivity of our ssDNA/SWCNT nanosensor even in a complex human urine environment. Unlike conventional methods using uricase that enzymatically degrade UA into specific metabolites such as allantoin for detection, our nanosensor achieved direct recognition of UA without requiring enzymatic processing, demonstrating its robustness and simplicity in real urine samples.
Assessment of pH impact on CoPhMoRe of the nanosensor
The pH of urine varies from 4 to 8, influenced by diet and hydration, making it essential to consider the effects of ion concentrations and pH on sensor performance.51–54 Enzymatic sensors are constrained by the pH variation of analytes due to their reliance on enzyme activity.13 Similarly, electrical sensors, such as those utilizing electrolyte-gating methods, face challenges from drift and threshold voltage interference caused by ion penetration.55 While enzyme-based and electrical sensors are affected by ion charges, our CoPhMoRe-based sensor also exhibits sensitivity to these factors.56,57 To ensure its reliability in urine across the typical pH range from 4 to 8, we evaluated its sensing behavior under this range.
To isolate the effect of pH on the corona nanosensor, we first measured the NIR fluorescence intensity of the corona nanosensor diluted in two different media, phosphate-buffered saline (PBS) and DI water, following the addition of diverse pH buffers without UA, as a sensor-to-buffer ratio of 9
:
1 (Fig. 3a). The fluorescence intensity was normalized, with the signal standardized at pH 7 for comparison. The results revealed that the unbuffered nanosensor, diluted in DI water, was significantly influenced by the pH of the added buffers, showing a substantial increase of 111% at pH 4. In contrast, the buffered nanosensor, diluted in PBS, was relatively unaffected, exhibiting only a minor change of about 10%. Specifically, the unbuffered nanosensor exhibited increasing fluorescence intensity as the added buffers became more acidic. However, this phenomenon was not observed in the buffered SWCNT sensor, which already possesses a high ionic strength, thereby preventing protons from readily interacting with ssDNA. The slight fluorescence increase at pH 8 might be due to a higher presence of anions compared to pH 7 solution, which suppresses non-radiative recombination of excitons and electron holes in SWCNTs.58,59
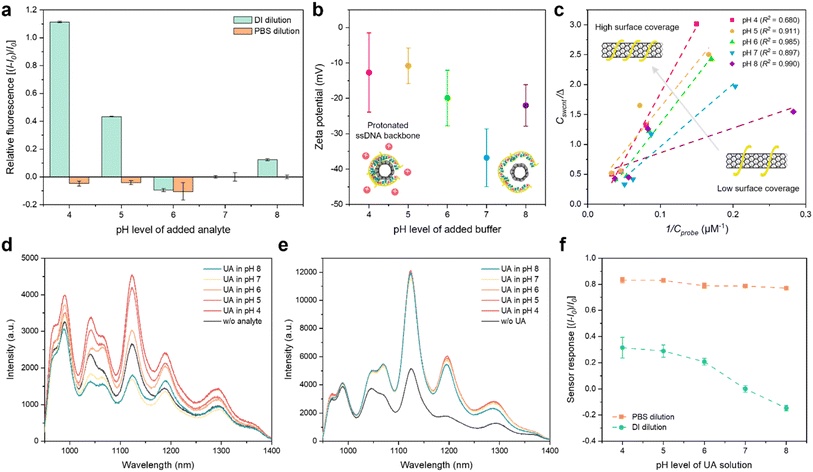 |
| Fig. 3 pH effects on the corona structure and sensing performance of the (AAT)10/SWCNT nanosensor. (a) Normalized NIR intensity variations of the buffered and unbuffered corona nanosensors with the signal standardized at pH 7 after the addition of various pH buffers (n = 3). (b) Zeta potential changes of the unbuffered corona nanosensor after the addition of various pH buffers, showing an increase in the positive direction as the pH decreases. The graph was plotted based on the peak maximum with the standard deviation of the zeta potential distribution. (c) Accessible surface area of the unbuffered corona nanosensor after the addition of various pH buffers revealed by the MPA method, indicating increased coverage as the pH decreases. (d) NIR fluorescence spectra of the unbuffered corona nanosensor recorded with 100 μM UA solution at various pH levels and (e) NIR fluorescence spectra of the buffered corona nanosensor recorded with 100 μM UA solution at various pH levels, showing pH-independent sensor responses. (f) Sensor responses of the buffered and unbuffered corona nanosensors to 100 μM UA solutions at various pH levels (n = 3). | |
We hypothesized that the observed increase in NIR fluorescence intensity of SWCNTs with decreasing pH is due to the protonation of the ssDNA backbone, leading to a compact conformation of the ssDNA on the SWCNT surface. A previous study demonstrated that the increased quantum yield of ssDNA/SWCNT complexes could be attributed to a reduction in quenching sites, resulting from the compact configuration of ssDNA under high ionic strength conditions.56 Similarly, in more acidic solutions, the higher concentration of protons facilitates the protonation of the ssDNA backbone, which reduces the electrostatic repulsion between SWCNTs and ssDNA and promotes the formation of a compact corona structure. To validate this hypothesis, we first measured the zeta potential to assess the surface charge of ssDNA wrapping on SWCNTs. The results indicated an increasing trend in zeta potential with decreasing pH, with the maximum peak of zeta potential distribution shifting from −36.8 mV at pH 7 to −12.7 mV at pH 4 (Fig. 3b). Raw data of the zeta potential are shown in Fig. S2.† This aligns with our expectation that the higher proton concentration under lower pH conditions facilitates the protonation of the ssDNA backbone.
To further demonstrate the structural change of the ssDNA corona on SWCNTs, we investigated the surface coverage changes of the nanosensor after the addition of diverse pH buffers utilizing a molecular probe adsorption (MPA) assay. MPA is a method used to measure the accessible nanoparticle surface area using a titration with a quenchable fluorescent molecule.60 The probe can exist in three states: free in solution, adsorbed on the SWCNT surface through π–π stacking interactions, or adsorbed on free ssDNA. The adsorption follows a type 1 Langmuir isotherm. The probe was added to the solution after the pH buffers induced changes in the nanosensor. The equilibrium constant for dissociation between the probe and the nanosensor, KD, is defined as follows.
Here, Cswcnt, Cprobe, and Cad represent the concentrations of the nanosensor, the probe, and the probe adsorbed on the nanosensor, respectively. q denotes the number of vacant sites per nanosensor and Δ represents the difference between the total concentration of the probe and the concentration of the free probe after adsorption.
The surface coverage influenced by the added pH buffers was confirmed using the above equation and a linearly fitted calibration curve describing the relationship between the riboflavin concentration and the fluorescence intensity (Fig. S3†). The KD/q values exhibit an increasing trend with values of 4.13, 10.5, 15.8, 14.9, and 22.3 as the pH decreases from 8 to 4, which implies an increase in surface coverage and a more compact ssDNA structure at lower pH levels (Fig. 3c). Therefore, when targeting dynamic biofluids such as urine, preserving the sensor response through buffer sampling is crucial to mitigate signal interference caused by pH variations in the analyte.
We further affirmed the potential interference caused by urine pH on the corona nanosensor's performance by observing the fluorescence spectra of unbuffered (Fig. 3d) and buffered (Fig. 3e) nanosensors recorded with 100 μM UA solutions at various pH levels. Even at the same UA concentration, the unbuffered sensor exhibited significantly varied on-responses depending on the pH, whereas the buffered sensor demonstrated a highly consistent turn-on response at different pH levels. In addition, the overall fluorescence intensity was stronger in the buffered sensor, attributed to the increased quantum yield resulting from the high ionic strength conditions.56 Fig. 3f summarizes the responses of the nanosensor to 100 μM UA solutions at various pH levels. We also confirmed the preserved sensor performance using UA solutions at pH 4 with various concentrations (Fig. S4†). In the calibration curve for UA under pH 4 conditions, the LOD was calculated as 0.10 nM, even lower than the 69 nM of the limit for UA in DI, as shown in Fig. 2d, which still remains below the diagnostic threshold for hyperuricemia. This demonstrates the stable performance of the ssDNA/SWCNT nanosensor across a wide pH range, corresponding to the pH change of urine, while maintaining high sensitivity and selectivity without the need for enzymes and biological receptors for UA, compared to conventional UA detection sensors (Table S4†).
Nanosensor immobilization in a paper-based optical test strip
To facilitate application in POC diagnostics, we immobilized the optimized corona nanosensor onto a paper substrate, forming an optical UA test strip compatible with existing urine test strip platforms. By employing the porous structure and hydrophilic properties of the poly(ether)sulfone (PES) filter membrane, the ssDNA/SWCNT nanosensor was immobilized onto the substrate through a drop-cast method.61 We selected the PES membrane based on its compatibility and stability under a range of pH conditions. We first diluted the nanosensor in PBS to concentrations of 0.5, 1, 5, and 10 mg L−1 and drop-coated the PES membrane to optimize the sensor performance (Fig. S5†). Among these, the 5 mg L−1 sensor-coated PES membrane exhibited the brightest and broadest NIR fluorescence, which was subsequently used for further experiments. In samples with 10 mg L−1, the effective fluorescence surface area of SWCNTs may have decreased due to sensor aggregation, potentially resulting in a lower NIR signal.62 A photograph of the sensor-coated PES membrane shows uniform embedding of the corona nanosensor across the cm-scale membrane with no visible surface color variations caused by SWCNT aggregation, indicating successful integration with the strip support (Fig. 4a).
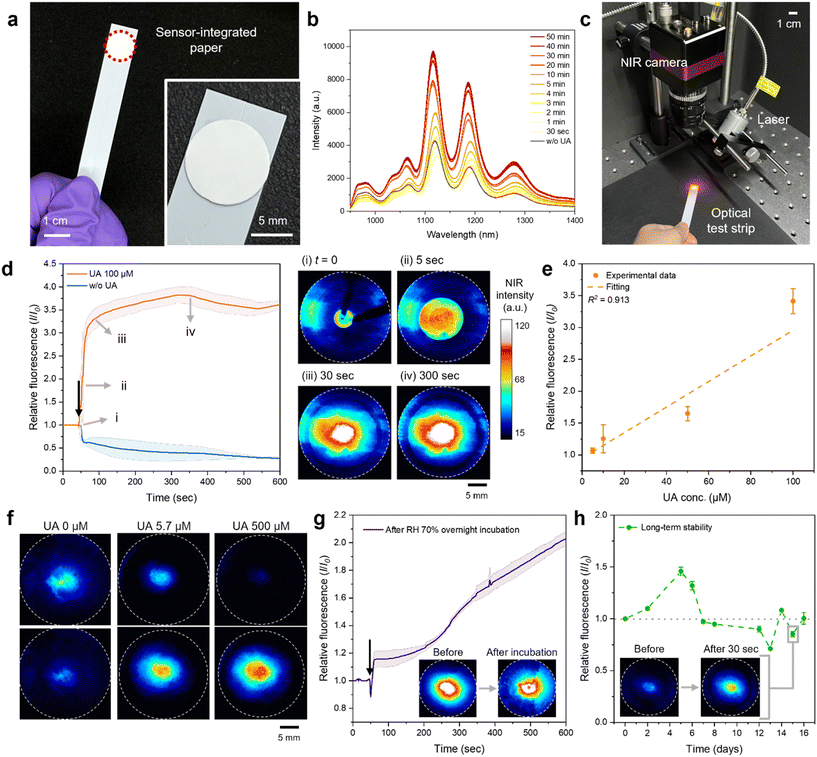 |
| Fig. 4 Immobilization of the (AAT)10/SWCNT nanosensor on a paper substrate for POC UA monitoring. (a) Photograph of the optical test strip with corona nanosensor integration. (b) Real-time fluorescence variations of the corona nanosensor-integrated test strip following the addition of 100 μM UA in DI water. (c) Photograph of the onsite UA detection setup using an NIR camera. (d) Average fluorescence intensity profile of the corona nanosensor-integrated optical test strip following the addition of 100 μM UA in DI water at 50 s indicated by the black arrow, with the solid line representing the mean values and the shaded region indicating the standard deviation (n = 3). The time-series NIR images (i–iv) correspond to the test strip marked in the profile, where the time denotes the elapsed period after the addition of UA solution. The region enclosed by the white dashed line represents the entire test strip area, and all images in this paper are presented using the same color scale. (e) Calibration curve of the corona nanosensor-integrated optical test strip with 5–100 μM UA in DI water (n = 3). The relative fluorescence is defined as the ratio of the test strip's fluorescence intensity 1 minute after UA addition to its intensity before addition. (f) Captured images of the corona nanosensor-integrated test strip (upper) before and (lower) 30 s after the addition of DI water and 5.7 and 500 μM UA in human urine. (g) Average time profile of the fluorescence intensity of the optical test strip after dropping 100 μM UA in DI water at 50 s indicated by the black arrow, following incubation at 40 °C and 70% RH overnight (n = 3). The inset images show the baseline NIR signals before and after incubation. (h) Stably preserved NIR fluorescence intensity of the test strip for 16 days (n = 3). The inset images show test strip response to 100 μM UA in DI water after 15 days of storage. | |
The NIR spectrum of the paper strip confirms that the nanosensor retains its NIR emission properties when embedded within the PES membrane, as evidenced by the similarity in peak positions and intensities compared to the spectrum observed in the liquid phase (Fig. 4b). Immediately after dropping the UA solution, the droplet is not fully absorbed by the paper substrate, leading to light scattering and low NIR intensity. However, as the solution gradually wicks into the paper and achieves uniform distribution, the fluorescence begins to increase. After around 3 min, the sensor showed a stable and consistent on-response to UA. It exhibited a 70% on-response within 10 min of analyte coverage and a 120% on-response after 30 min, demonstrating higher sensor reactivity than the previous setup with the solution-state sensor.
The nanosensor-integrated test strips were then monitored using a compact NIR camera under laser excitation, addressing the challenge of incorporating an InGaAs detector in POC diagnostics (Fig. 4c). The average NIR intensity profile, along with the corresponding captured NIR images, showed a consistent and predictable increase in the NIR fluorescence across the paper substrate upon the addition of a few drops of 100 μM UA solution, compared to that without UA (Fig. 4d). A corresponding video is provided in the ESI.† The test strip emits a strong NIR signal right after the solution contacts the paper substrate, showing an average intensity 4 times higher than that of the strip without UA. Then, we confirmed the performance of our optical test strip with 5–100 μM UA solutions based on the NIR intensity 1 min after UA addition, which is the corresponding time to a 90% response in the time profile (Fig. 4e). The linear calibration curve shows an LOD of 8.5 μM and a dynamic range of 5–100 μM, demonstrating the potential for concentration-specific sensing using the optical test strip. This detection limit is slightly higher than the LOD of the pristine nanosensor dispersion (69 nM), which may be attributed to the reduced interfacial area available for analyte interaction after paper integration. Previous fluorescence-based UA sensors have predominantly been designed to operate within the visible light range.63 However, when applied to paper substrates, their signals often suffer from scattering and absorption, complicating integration into optical strips.64,65 In contrast, our corona nanosensor leverages NIR fluorescence, providing a strong signal clarity and effective detection even on paper substrates.66,67 We also tested the test strips with pooled human urine samples with 5.7 and 500 μM UA (Fig. 4f). The increasing NIR signal with increasing UA concentration was maintained after contact with actual urine and the fluorescence intensity with 500 μM UA was approximately twice as strong as that of 5.7 μM UA, measured 3 min after analyte addition (Fig. S6†). In addition, the absorption behavior of urine on the paper substrate was also observed, providing additional diagnostic information beyond fluorescence signals (Fig. S7†). By observing how urine spreads and wicks into the substrate, properties such as viscosity and surface tension can be inferred.68–71 For instance, higher viscosity may slow down the absorption rate, resulting in distinct patterns or delayed fluorescence stabilization. These insights could be leveraged to complement traditional diagnostic methods, offering a more comprehensive analysis of the urine sample's physical and chemical characteristics in the future.
Since CoPhMoRe and the paper substrate can be sensitive to environmental moisture, we assessed the sensing stability of the nanosensor-coated strip under conditions of elevated relative humidity (RH) and temperature. Even after exposure to 70% RH and 40 °C overnight, the baseline NIR signal remained stable (inset, bottom-right) and the sensor response showed consistent turn-on behavior without significant changes (Fig. 4g). The slightly delayed response time observed may be attributed to hindered UA diffusion caused by retained water molecules. We also evaluated the long-term stability of the paper strip over 15 days, confirming that the baseline NIR signal was maintained within 20% (Fig. 4h). Even when stored in a dried state, the corona nanosensor reliably maintained its intrinsic NIR signal over an extended period. Additional experiments assessed the sensor's long-term performance after 15 days of storage (Fig. S8†). The fluorescence response remained stable, with no noticeable decrease in sensitivity or signal intensity. The robust performance of the CoPhMoRe nanosensor, even when integrated with a paper substrate under harsh conditions, demonstrates its potential for practical UA testing applications. This approach provides a simple and cost-effective process, as the substrate itself is inexpensive and requires no additional equipment for storage and transport, making it highly suitable for practical applications compared to previous UA detection sensors (Table S4†). The detector also has the potential to be deployed as a future POC device, as it can effectively capture the NIR signals of the corona nanosensor using even low specification NIR cameras such as Raspberry Pi.72,73 Moreover, when manufacturing the optical strips, even slight differences in the concentration and dropping of SWCNT dispersions can affect the resulting NIR signals. The introduction of automated synthesis processes and printing technologies can overcome this challenge by ensuring uniform volume and concentration in the bulk dispersions, thus enabling applications with consistent and reproducible performance across multiple SWCNT batches.
Conclusions
In conclusion, we developed a CoPhMoRe nanosensor capable of selectively detecting UA in urine without the use of enzymes. We utilized the non-covalent functionalization of NIR fluorescent SWCNTs with ssDNA arrays. Based on high-throughput screening of the nanosensor library, the (AAT)10/SWCNT nanosensor exhibited selectivity and sensitivity even in actual human urine. We investigated the pH effect on the surface corona structure and sensing performance of (AAT)10/SWCNT by examining the zeta potential and MPA. Finally, we immobilized the optimized SWCNT nanosensor on the PES membrane to fabricate a paper-based optical UA test strip. We demonstrated a rapid and strong turn-on NIR response of the optical test strip, up to 4 times stronger when exposed to UA solutions at concentrations ranging from 5.7 to 500 μM in actual urine. Considering the effects of humidity and temperature, which are potential weaknesses of the nanosensor-integrated paper, our optical test demonstrated a stable response to UA even after long-term storage for up to 15 days. The enzyme-free SWCNT nanosensor represents a highly practical and transformative solution for future UA POC diagnostics.
Experimental section
Materials
All chemical materials were purchased from Sigma-Aldrich unless stated otherwise. SWCNT powders produced by the HiPCO process were purchased from NanoIntegris (Batch# HR27-149A) and used without further processing. ssDNA sequences were purchased from Integrated DNA Technologies.
Preparation and characterization of the ssDNA/SWCNT nanosensor
The SWCNT dispersions were prepared by combining 1 mg of SWCNTs and 1 mg of ssDNA in 1 mL of DNase-free water. This mixture was bath sonicated (Daihan Scientific, WUC-D03H) for 10 min and tip sonicated (Qsonica, Q125) in an ice bath with a 0.125 in. probe for 30 min at 40% amplitude. The crude SWCNT dispersions were centrifuged (Labogene, 1730R) twice at 17
000g for 1 h to remove undispered SWCNT bundles. The top 80% of the supernatant was collected after each round of centrifugation. Absorption spectra of the SWCNT dispersions with 1/60 dilution were recorded using a UV-Vis-NIR spectrometer (Shimadzu, UV-2600i) to approximate the concentration of the stock solutions, utilizing an extinction coefficient of ε632 = 0.036 L (mg cm)−1.
NIR fluorescence measurements of the nanosensors
Screening of the ssDNA/SWCNT sensor library against UA and significant competitors was performed using a customized setup, which consists of an inverted microscope (Olympus, IX73) with a 20× objective and a 900 nm long-pass filter, coupled to a monochromator/spectrograph (Princeton Instruments, HRS 300 S) and a liquid nitrogen-cooled InGaAs detector (Princeton Instruments, PyLoN IR 1024). The samples were excited with a 300 mW 721 nm photodiode laser (CNI Optics, PSU-H-LED). The exposure time was held constant across a given experiment and varied between 2 and 5 s. Fluorescence spectra were background corrected using SWCNT-free solution in an equivalent volume and collected from 950 to 1400 nm. The SWCNT stock solutions were diluted to 0.5 and 1 mg L−1 in DI water or PBS. Analytes were prepared as stock solutions at 100 μM in DI water. Among them, UA was prepared as a 300 μM solution by overnight heating at 60 °C with stirring due to its poor solubility, then diluted to 100 μM and stored in aliquots at room temperature.42,74 ssDNA/SWCNT and analytes were mixed in a ratio of 9
:
1 to achieve a final volume of 200 μL and incubated for 30 min before collecting measurements. The additional nanosensor performance measurements involving various pooled human urine samples were performed in accordance with the guidelines of the Bioethics and Safety Act of Korea and approved by the Institutional Review Board (IRB) at Sungkyunkwan University (IRB approval no. SKKU 2025-02-025). Informed consent was obtained from human participants of this study. The human urine samples were treated with 1 IU ml−1 ascorbate oxidase for 10 min at 37 °C to exclude the effect of ascorbic acid on the nanosensors.
pH effect on UA detection of the nanosensor
The pH level of the analytes was determined using a pH meter (Trans Instruments, BP3001). The buffers for observing the pH effect on the ssDNA/SWCNT sensors were adjusted to the desired pH level using 1 M HCl or 1 M NaOH in 1× PBS solution (pH 7.2). 100 μM UA solutions at various pH levels were prepared using the same adjustment method. The zeta potentials of the ssDNA/SWCNT dispersions were measured using Dynamic Light Scattering (DLS) (Malvern, Zetasizer Nano ZS90). The accessible surface area of the ssDNA/SWCNT nanosensor according to the pH level of the analytes was compared using the molecular probe adsorption technique performed using a spectrofluorometer (Horiba, Fluoromax Plus C) and a microwell-plate reader (Horiba, MicroMax 384).60 Riboflavin was excited at 460 nm and its emission spectra were recorded from 480 to 600 nm with 1 s integration time and 2 nm step size. The wavelength at the maximum fluorescence peak was 515–520 nm. The SWCNT stock solution was dialyzed in DNase-free water overnight to remove free DNA using dialysis tubes with a molecular weight cut-off of 12 kDa and diluted to 10 mg L−1. Riboflavin was dissolved in DI water and prepared as the stock solution at 2 mM. ssDNA/SWCNT, pH buffer, and riboflavin were combined in a ratio of 9
:
1
:
1. ssDNA/SWCNT and pH buffer were initially mixed and incubated for 30 min. Then, riboflavin was added to reach final concentrations of 0–50 μM, followed by an additional 30 min of incubation.
Paper immobilization of the nanosensor for POC applications
The SWCNT stock solutions were diluted to a concentration of 0.5–10 mg L−1 in PBS and deposited onto a PES membrane for 1 h using a drop casting method. After surface drying, light agitation around 30 revolutions per minute (rpm) in water was applied on the sensor-integrated papers for 1 h to remove unbound SWCNTs, thereby improving the sensor signal and overall performance. They were later dried and stored in a dried state before collecting measurements. The NIR spectra were recorded using the same customized setup as the screening measurements. All spectra were background corrected using an uncoated paper sample acquired with 2 s exposure time. Spatiotemporal detection with NIR imaging was performed using an NIR camera (Raptor Photonics, Owl 640 T) with an H1-SW 25 mm SWIR lens (RPL-VS-2514) and an 850 nm long-pass filter under excitation with a 300 mW 721 nm laser (CNI Optics, PSU-H-LED). Sequential NIR images were captured starting 10 min after addition of 10–20 μL of DI water, followed by the introduction of UA solution 50 s later. The calibration curve was predicted using 5–100 μM UA solutions based on the NIR intensity 1 min after UA addition, which is the corresponding time to a 90% response in the time profile. To evaluate device stability, the sensor-integrated papers were exposed to conditions of 40 °C and 70% relative humidity overnight. For long-term measurements, the sensor-integrated papers were stored at room temperature and ambient humidity.
Author contributions
M.Y. and S.-Y.C. conceived the idea and designed the project. M.Y. conducted the experiments and analysis with the assistance of S.S. and S.L. M.Y. and S.-Y.C. wrote the manuscript with input from all the authors. All authors contributed to discussions of the research.
Data availability
The data supporting the plots presented in this paper and other findings of this study are available from the corresponding author upon request.
Conflicts of interest
There are no conflicts to declare.
Acknowledgements
This research was supported by the National Research Foundation (NRF) grant funded by the Korean government (MSIT) (No. RS-2023-00211580 and RS-2023-00237308). This research was also supported by a grant of the Korea Health Technology R&D Project through the Korea Health Industry Development Institute (KHIDI), funded by the Ministry of Health & Welfare, Republic of Korea (RS-2023-KH140761).
References
- R. L. Wortmann, Curr. Opin. Rheumatol., 2002, 14, 281–286 Search PubMed.
- E. Kedar and P. A. Simkin, Adv. Chronic Kidney Dis., 2012, 19, 392–397 Search PubMed.
- K. T. Clebak, A. Morrison and J. R. Croad, Am. Fam. Physician, 2020, 102, 533–538 Search PubMed.
- J. Padda, K. Khalid, S. Padda, N. L. Boddeti, B. S. Malhi, R. Nepal, A. C. Cooper and G. Jean-Charles, Cureus, 2021, 13, e18172 Search PubMed.
- A. K. Tausche, T. L. Jansen, H. E. Schröder, S. R. Bornstein, M. Aringer and U. Müller-Ladner, Dtsch. Ärztebl. Int., 2009, 106, 549–555 Search PubMed.
- D. J. Stewart, V. Langlois and D. Noone, Integr. Blood Pressure Control, 2019, 43–62 CAS.
- G. Sanders, A. Pasman and F. Hoek, Clin. Chim. Acta, 1980, 101, 299–303 CAS.
- E. Akyilmaz, M. K. Sezgintürk and E. Dinçkaya, Talanta, 2003, 61, 73–79 Search PubMed.
- Y. Zhao, X. Yang, W. Lu, H. Liao and F. Liao, Microchim. Acta, 2009, 164, 1–6 CrossRef CAS.
- Q. Wang, X. Wen and J. Kong, Crit. Rev. Anal. Chem., 2020, 50, 359–375 CrossRef CAS.
- F. Liao, Y. S. Zhao, L. N. Zhao, J. Tao, X. Y. Zhu and L. Liu, J. Zhejiang Univ., Sci., B, 2006, 7, 497–502 Search PubMed.
- A. L. Levy and J. Keitge, Standard Methods of Clinical Chemistry: By the American Association of Clinical Chemists, 2013, pp. 1 Search PubMed.
- H. Bisswanger, Perspect. Sci, 2014, 1, 41–55 Search PubMed.
- G. Domagk and H. Schlicke, Anal. Biochem., 1968, 22, 219–224 CrossRef CAS.
- M. Soukup, I. Biesiada, A. Henderson, B. Idowu, D. Rodeback, L. Ridpath, E. G. Bridges, A. M. Nazar and K. G. Bridges, Diabetol. Metab. Syndr., 2012, 4, 14 CrossRef CAS.
- Y. Yang, Y. Song, X. Bo, J. Min, O. S. Pak, L. Zhu, M. Wang, J. Tu, A. Kogan, H. Zhang, T. K. Hsiai, Z. Li and W. Gao, Nat. Biotechnol., 2020, 38, 217–224 CrossRef CAS PubMed.
- D. W. Kimmel, G. LeBlanc, M. E. Meschievitz and D. E. Cliffel, Anal. Chem., 2012, 84, 685–707 Search PubMed.
- M. Yoon, Y. Lee, S. Lee, Y. Cho, D. Koh, S. Shin, C. Tian, Y. Song, J. Kang and S.-Y. Cho, Sens. Diagn., 2024, 3, 203–217 RSC.
- Y. Lee, W. Kim, Y. Cho, M. Yoon, S. Lee, J. Lee, S. Oh, Y. Song, B. J. Lee and Y. Kim, ACS Nano, 2024, 18, 13214–13225 CrossRef CAS.
- S. Lee, G. Ryu, S. Shin, W. Kim, M. Yoon, Y. Kim, S. Park, Y. Kim and S.-Y. Cho, ACS Nano, 2025, 19, 950–962 CrossRef CAS.
- J. Zhang, M. P. Landry, P. W. Barone, J.-H. Kim, S. Lin, Z. W. Ulissi, D. Lin, B. Mu, A. A. Boghossian, A. J. Hilmer, A. Rwei, A. C. Hinckley, S. Kruss, M. A. Shandell, N. Nair, S. Blake, F. Şen, S. Şen, R. G. Croy, D. Li, K. Yum, J.-H. Ahn, H. Jin, D. A. Heller, J. M. Essigmann, D. Blankschtein and M. S. Strano, Nat. Nanotechnol., 2013, 8, 959–968 Search PubMed.
- J. P. Giraldo, M. P. Landry, S. Y. Kwak, R. M. Jain, M. H. Wong, N. M. Iverson, M. Ben-Naim and M. S. Strano, Small, 2015, 11, 3973–3984 Search PubMed.
- J. D. Harvey, P. V. Jena, H. A. Baker, G. H. Zerze, R. M. Williams, T. V. Galassi, D. Roxbury, J. Mittal and D. A. Heller, Nat. Biomed. Eng., 2017, 1, 0041 CrossRef CAS PubMed.
- G. Bisker, J. Dong, H. D. Park, N. M. Iverson, J. Ahn, J. T. Nelson, M. P. Landry, S. Kruss and M. S. Strano, Nat. Commun., 2016, 7, 10241 CAS.
- N. M. Bardhan, D. Ghosh and A. M. Belcher, Nat. Commun., 2014, 5, 4918 Search PubMed.
- M. Zheng, A. Jagota, M. S. Strano, A. P. Santos, P. Barone, S. G. Chou, B. A. Diner, M. S. Dresselhaus, R. S. Mclean and G. B. Onoa, Science, 2003, 302, 1545–1548 CAS.
- A. A. Alizadehmojarad, X. Zhou, A. G. Beyene, K. E. Chacon, Y. Sung, R. L. Pinals, M. P. Landry and L. Vuković, Adv. Mater. Interfaces, 2020, 7, 2000353 Search PubMed.
- P. V. Jena, M. M. Safaee, D. A. Heller and D. Roxbury, ACS Appl. Mater. Interfaces, 2017, 9, 21397–21405 CAS.
- D. P. Salem, M. P. Landry, G. Bisker, J. Ahn, S. Kruss and M. S. Strano, Carbon, 2016, 97, 147–153 Search PubMed.
- A. Shankar, J. Mittal and A. Jagota, Langmuir, 2014, 30, 3176–3183 CAS.
- R. El Ridi and H. Tallima, J. Adv. Res., 2017, 8, 487–493 Search PubMed.
- S. Kruss, M. P. Landry, E. Vander Ende, B. M. A. Lima, N. F. Reuel, J. Zhang, J. Nelson, B. Mu, A. Hilmer and M. Strano, J. Am. Chem. Soc., 2014, 136, 713–724 CAS.
- J. Ackermann, J. T. Metternich, S. Herbertz and S. Kruss, Angew. Chem., Int. Ed., 2022, 61, e202112372 CAS.
- S. Jeong, D. Yang, A. G. Beyene, J. T. Del Bonis-O'Donnell, A. M. Gest, N. Navarro, X. Sun and M. P. Landry, Sci. Adv., 2019, 5, eaay3771 Search PubMed.
- X. Tu, S. Manohar, A. Jagota and M. Zheng, Nature, 2009, 460, 250–253 Search PubMed.
- X. Wei, T. Tanaka, Y. Yomogida, N. Sato, R. Saito and H. Kataura, Nat. Commun., 2016, 7, 12899 Search PubMed.
- B. Podlesny, T. Shiraki and D. Janas, Sci. Rep., 2020, 10, 9250 CrossRef CAS PubMed.
- B. Podlesny, B. Olszewska, Z. Yaari, P. V. Jena, G. Ghahramani, R. Feiner, D. A. Heller and D. Janas, Sci. Rep., 2021, 11, 10618 CrossRef CAS PubMed.
- N. Sarigul, F. Korkmaz and İ. Kurultak, Sci. Rep., 2019, 9, 20159 CrossRef CAS.
- R. S. Lord and J. A. Bralley, Altern. Med. Rev., 2008, 13, 292–306 Search PubMed.
- H. Iwata, S. Nishio, M. Yokoyama, A. Matsumoto and M. Takeuchi, J. Urol., 1989, 142, 1095–1098 CrossRef CAS PubMed.
- J. N. Loeb, Arthritis Rheum., 1972, 15, 189–192 CAS.
- A. J. Lee, X. Wang, L. J. Carlson, J. A. Smyder, B. Loesch, X. Tu, M. Zheng and T. D. Krauss, Nano Lett., 2011, 11, 1636–1640 CAS.
- S. M. Bachilo, M. S. Strano, C. Kittrell, R. H. Hauge, R. E. Smalley and R. B. Weisman, Science, 2002, 298, 2361–2366 Search PubMed.
- W. Lee, Y. Kim, S. Chang, A.-j. Lee and C.-H. Jeon, J. Clin. Lab. Anal., 2017, 31, e22080 Search PubMed.
- S.-Y. Cho, X. Jin, X. Gong, S. Yang, J. Cui and M. S. Strano, Anal. Chem., 2021, 93, 14685–14693 Search PubMed.
- P. H. F. Gois and E. R. M. Souza, Cochrane Database Syst. Rev., 2020, 9, Cd008652 Search PubMed.
- C. George, S. W. Leslie and D. A. Minter, Hyperuricemia, StatPearls Publishing, Treasure Island (FL), 2024 Search PubMed.
- S. T. Choi, J. S. Song, S. J. Kim, C. H. Kim and S. J. Moon, J. Korean Med. Sci., 2020, 35, e95 Search PubMed.
- K. Sakhaee and N. M. Maalouf, Semin. Nephrol., 2008, 28, 174–180 CAS.
- N. Karki and S. W. Leslie, Struvite and Triple Phosphate Renal Calculi, StatPearls Publishing, Treasure Island (FL), 2024 Search PubMed.
- T. Remer and F. Manz, J. Am. Diet. Assoc., 1995, 95, 791–797 CAS.
- L. Bankir, J. Perucca, P. Norsk, N. Bouby and M. Damgaard, Ann. Nutr. Metab., 2017, 70(Suppl 1), 51–61 Search PubMed.
- J. G. Wolfsberger, E. C. Hunt, S. S. Bobba, S. Love-Rutledge and B. Vogler, Metabolomics, 2022, 18, 80 Search PubMed.
- S. Cao, P. Sun, G. Xiao, Q. Tang, X. Sun, H. Zhao, S. Zhao, H. Lu and Z. Yue, Electrochem. Sci. Adv., 2023, 3, e2100207 Search PubMed.
- D. P. Salem, X. Gong, A. T. Liu, V. B. Koman, J. Dong and M. S. Strano, J. Am. Chem. Soc., 2017, 139, 16791–16802 Search PubMed.
- N. Sultana, H. Dewey and J. Budhathoki-Uprety, Sens. Diagn., 2022, 1, 1189–1197 Search PubMed.
- F. Wang, G. Dukovic, E. Knoesel, L. E. Brus and T. F. Heinz, Phys. Rev. B:Condens. Matter Mater. Phys., 2004, 70, 241403 CrossRef.
- L. Cognet, D. A. Tsyboulski, J.-D. R. Rocha, C. D. Doyle, J. M. Tour and R. B. Weisman, Science, 2007, 316, 1465–1468 CrossRef CAS PubMed.
- M. Park, D. P. Salem, D. Parviz, X. Gong, K. S. Silmore, T. T. S. Lew, D. T. Khong, M. C.-Y. Ang, S.-Y. Kwak, M. B. Chan-Park and M. S. Strano, Nano Lett., 2019, 19, 7712–7724 CrossRef CAS PubMed.
- D. P. Salem, X. Gong, A. T. Liu, K. Akombi and M. S. Strano, Anal. Chem., 2020, 92, 916–923 CrossRef CAS.
- H. Pathangi, P. M. Vereecken, A. Klekachev, G. Groeseneken and A. Witvrouw, J. Nanosci., 2014, 2014, 328627 Search PubMed.
- C. Ma, N. Jiang, X. Sun, L. Kong, T. Liang, X. Wei and P. Wang, Biosens. Bioelectron., 2023, 237, 115495 CrossRef CAS PubMed.
- T. H. Ulep and J. Y. Yoon, Nano Convergence, 2018, 5, 14 Search PubMed.
- A. Luongo, A. R. von Stockert, F. D. Scherag, T. Brandstetter, M. Biesalski and J. Rühe, ACS Biomater. Sci. Eng., 2023, 9, 6379–6389 CAS.
- C. Tian, Y. Lee, Y. Song, M. R. Elmasry, M. Yoon, D.-H. Kim and S.-Y. Cho, ACS Appl. Nano Mater., 2024, 7, 5576–5586 CAS.
- Y. Song, C. Tian, Y. Lee, M. Yoon, S. E. Yoon and S.-Y. Cho, ACS Meas. Sci. Au, 2023, 3, 393–403 CAS.
- A. Fathi-Azarbayjani and A. Jouyban, BioImpacts, 2015, 5, 29–44 Search PubMed.
- D. V. Trukhin, O. V. Sinyachenko, V. N. Kazakov, S. V. Lylyk, A. M. Belokon and U. Pison, Colloids Surf., B, 2001, 21, 231–238 Search PubMed.
- B. A. Inman, W. Etienne, R. Rubin, R. A. Owusu, T. R. Oliveira, D. B. Rodriques, P. F. Maccarini, P. R. Stauffer, A. Mashal and M. W. Dewhirst, Int. J. Hyperthermia, 2013, 29, 206–210 Search PubMed.
- M. Pradella, R. M. Dorizzi, F. Rigolin and B. E. Statland, Crit. Rev. Clin. Lab. Sci., 1988, 26, 195–242 CAS.
- M. H. Wong, J. P. Giraldo, S.-Y. Kwak, V. B. Koman, R. Sinclair, T. T. S. Lew, G. Bisker, P. Liu and M. S. Strano, Nat. Mater., 2017, 16, 264–272 CrossRef CAS PubMed.
- M. Yoon, S. Shin, S. Lee, J. Kang, X. Gong and S.-Y. Cho, ACS Sens., 2024, 9, 6311–6319 CrossRef CAS PubMed.
- N. Norazmi, Z. A. Rasad, M. Mohamad and H. Manap, IOP Conf. Ser.: Mater. Sci. Eng., 2017, 257, 012031 Search PubMed.
|
This journal is © The Royal Society of Chemistry 2025 |
Click here to see how this site uses Cookies. View our privacy policy here.