DOI:
10.1039/D3TA08096J
(Paper)
J. Mater. Chem. A, 2024,
12, 10059-10071
Computational discovery of superior vanadium-niobate-based cathode materials for next-generation all-solid-state lithium-ion battery applications†
Received
28th December 2023
, Accepted 3rd February 2024
First published on 6th February 2024
Abstract
All-solid-state lithium-ion batteries (ASSLIBs) are at the forefront of green and sustainable energy development research. One of the key challenges in the development of ASSLIBs for commercial applications is to find cathode materials that have high capacity, voltage and power density. Using a combination of first-principles calculations and various crystal structure prediction algorithms, we explore the LiVO2–Li3NbO4 pseudobinary tieline to identify novel stoichiometries with improved properties as cathode materials for ASSLIB applications. Based on more than 10
000 Density Functional Theory (DFT) calculations using crystal structures obtained from ab initio random structure searching (AIRSS), genetic algorithm, and configuration enumeration procedures, we predict five novel stoichiometries, Li23Nb7V2O32, Li10Nb3VO14, Li7Nb2VO10, Li11Nb3V2O16, Li4NbVO6, along with an experimentally known stoichiometry, Li5NbV2O8. All the novel stoichiometries are found to have cation-disordered rock-salt crystal structures and fall within 30 meV per atom from the convex hull of the parent compositions. These new phases are predicted to have superior properties compared to the current vanadium-niobate-based electrode materials, including a higher theoretical capacity, lower band gap, higher average Li intercalation voltage, minor volume change upon full Li delithiation, good mechanical and dynamical stability, improved Li-conduction activation barrier and high-temperature stability. Our results are anticipated to inspire further experiments to synthesise and test these specific vanadium-niobate-based materials for their actual performance as Li-ion cathode materials.
1 Introduction
Since the commercialisation of rechargeable lithium-ion batteries (LIBs) in 1991,1 LIBs have come a long way as a solution to clean and effective electrochemical energy storage. Owing to their high energy density, good rate capability, long shelf-life and low maintenance cost, rechargeable LIBs have earned their reputation as the enabling technology for the transportation, aviation, aerospace, and stationary energy storage sectors2–8 including all-electric vehicles (EVs)9 and large scale stationary storage.10 Despite these successes, scaling-up LIBs has been challenging owing to safety reasons primarily attributed to the commonly used organic electrolyte solutions. These can be volatile, flammable and even explosive, potentially causing catastrophic failures, particularly when used in excessive amounts to power energy-intensive applications.
To mitigate the safety risks associated with the conventional liquid electrolytes used in LIBs, all solid-state lithium-ion batteries (ASSLIBs), which utilise a solid electrolyte, have drawn soaring attention in recent years.11,12 Beyond mitigating safety concerns, solid state electrolytes can also provide superior battery performance due to their higher energy density. One of the major remaining roadblocks for the commercialisation of ASSLIBs is the lack of a suitable high-capacity positive electrode material. Thus, to meet the stringent energy demand there is an ever-growing need for developing next-generation battery technologies, in particular, advanced electrode materials that can deliver high power compared to existing ones.
The full potential of ASSLIBs as next-generation batteries can be unlocked by the discovery of new battery materials with superior performance such as higher capacity and energy density, safer operation, better stability, greater longevity, faster charge rates, better component compatibility, and economic viability. Over the years, many oxide- and phosphate-based cathode materials have been studied extensively as promising candidates for cathode materials.11,13 However, some of the commonly used cathode materials suffer from their low theoretical capacity (Qmax), which is a measure of the amount of charge (lithium ions) that can be stored by the material. An extensive (although incomplete) list of such materials is reported by Nitta and co-workers8 where the majority have Qmax values below 300 mA h g−1, except for Li2MnO3 whose Qmax is 458 mA h g−1.
Li2MnO3 and its derivatives have been studied extensively in the past decade as cathode materials14–20 that show anomalously high reversible capacity with relatively good capacity retention. However, Li2MnO3 suffers from capacity and voltage fading during electrochemical cycles20 owing to the layered-to-spinel phase transformation coupled with gradual oxygen loss, which restricts its use for commercial applications. Similarly, LiVO2–Li3NbO4-based systems have also been studied recently by Yabuuchi and co-workers21–23 as a new family of high-capacity cathode materials for rechargeable LIBs. In their work, a particular focus was given to LiVO2–Li3NbO4 solid solutions where they were able to synthesise two specific stoichiometries, Li13Nb3V4O20 (Li1.3Nb0.3V0.4O2)21 and Li5NbV2O8 (Li1.25Nb0.25V0.5O2)23 with reversible capacity of 230 mA h g−1 (Qmax = 390 mA h g−1) and 240 mA h g−1 (Qmax = 375 mA h g−1), respectively. Their work suggests that there is room for further increasing the reversible capacity and energy density, which motivates a more systematic exploration of new LiVO2–Li3NbO4 materials.
In this work, we explored the LiVO2–Li3NbO4 pseudobinary tieline to search for new stoichiometries that could have superior cathode properties for use in ASSLIBs and other conventional LIBs using a combination of first-principles calculations and crystal structure prediction algorithms. To sample the composition space, we generated a database containing more than 10
000 structures optimised using density functional theory (DFT) and obtained from a combination of major databases and our own crystal structure prediction (CSP) calculations. We predicted five novel stoichiometries, Li23Nb7V2O32, Li10Nb3VO14, Li7Nb2VO10, Li11Nb3V2O16, and Li4NbVO6; along with an experimentally known stoichiometry, Li5NbV2O8.23 All the stoichiometries are predicted to have a cation-disordered rock-salt crystal structure which is in good agreement with previous experimental findings.21,23 Interestingly, our newly discovered stoichiometries are predicted to have higher Qmax value, lower band gap, higher average Li intercalation voltage, minor volume changes upon full Li delithiation, good mechanical stability, improved Li+-conduction activation barriers, and high-temperature stability. In particular, we show that some of the new stoichiometries may have improved cathode properties compared to the experimentally known ones. Thus, our work enables the discovery of new lithium vanadium niobate (Li–V–Nb–O) materials and may inspire further experimental work to synthesise and test the actual performance of these new stoichiometries as Li-ion cathode materials.
2 Methods
2.1 Density functional theory calculations
All total energy calculations were performed using plane-wave DFT as implemented in the Vienna Ab Initio Simulation Package (VASP, v.6.2.1).24–26 The Perdew–Burke–Ernzerhof (PBE) exchange–correlation functional27 and projector augmented wave (PAW) pseudopotentials28 were used to represent each element. The Hubbard U (GGA + U) approach29,30 to the V 3d states was applied. The value of U = 3.25 eV was chosen to be consistent with those used for calculations in the Materials Project (MP) database31,32 and other studies.33 All DFT calculations were spin-polarised, except otherwise noted, initialised in a ferromagnetic spin configuration. A plane-wave cutoff energy of 520 eV was used to expand the orbitals, and the Brillouin zone was sampled using the Methfessel–Paxton scheme. Monkhorst–Pack grids34 with a spacing of 0.314 Å−1 were used. The atomic coordinates were fully relaxed during the DFT simulations until the forces on each atom were within 0.05 eV Å−1. An electronic convergence criterion of 10−8 eV and a Gaussian smearing factor of 0.2 eV were adopted, and the VASP default settings for the fast Fourier transform (FFT) grid and optimisation of the projection operators were employed. For the analysis of band structure, density of states, and estimation of band gap values, we used the Pymatgen35 software package. All the database management and the convex hull plotting tasks were done by the MATADOR package.36 All crystal structures were visualised with the VESTA37 software package.
To study Li+ diffusion and high-temperature stability, ab initio molecular dynamics (AIMD) simulations were carried out in the canonical (NVT) ensemble as implemented in VASP.24–26 We performed non-spin-polarised DFT calculations with an energy cutoff of 400 eV for all the AIMD simulations to reduce computational costs. We used supercells with the minimum lattice dimension larger than 10 Å to minimise the finite cell size effect and a Γ-centered 1 × 1 × 1 k-mesh was used for the sampling of the Brillouin zone. The time step was set to 2 fs. For the analysis of Li-ion diffusion and the pair radial distribution function (RDF), we generated at least 50 ps and 10 ps AIMD trajectories, respectively.
To investigate the mechanical stability of the selected stoichiometries, we carried out elastic constant calculations based on the energy-strain method as implemented in VASPKIT38 where the total energy values were obtained from VASP. We applied a strain of ±0.015 on selected fully relaxed stoichiometries to obtain the full elastic tensor and derived mechanical properties. Using the computed elastic constants, the mechanical stability criteria were evaluated following Mouhat and co-workers39 using the VASPKIT38 code.
The dynamical stability of selected novel structures was verified with phonon calculations using the finite displacement method in conjunction with diagonal supercells40 as implemented in the Phonopy package.41,42 For compounds with larger primitive cells, this approach becomes computationally prohibitive so instead we used the more efficient finite displacement method in conjunction with nondiagonal supercells.43,44
To estimate Li+ vacancy diffusion barriers we carried out climbing image nudged elastic band (CI-NEB) calculations45,46 as implemented in ASE.47 All the calculated NEB paths comprised of five intermediate images. Overall charge neutrality was achieved by adding a positive background charge. All the ionic forces were converged to within 0.05 eV Å−1 with the spring constant set to 0.1 eV Å−1. To estimate the energy at the saddle point, cubic splines were fit through the images along each hop as implemented in ASE.47 Bond valence site energies (BVSEs) were calculated using the softBV program48–50 to rapidly identify the Li+ diffusion pathways and to estimate the Li+ migration barrier in our complex disordered structures. The structural information required for the BVSE calculations was collected from the CIF files generated using VESTA/ASE. The energetics of different Li+ ions, EBVSE, were calculated using grids spanning the structure model with a resolution of 0.1 Å.
2.2 Crystal structure prediction
We employed a variety of crystal structure prediction schemes to sample the composition–structure space spanned by the LiVO2–Li3NbO4 pseudobinary tieline as comprehensively as possible. The databases used in this work are the Materials Project (MP),31,32 the Open Quantum Materials Database (OQMD),51,52 Novel Materials Discovery (NOMAD),53–55 and Automatic FLOW for Materials Discovery (AFLOW);56 and the CSP calculations used ab initio random structure searching (AIRSS),57,58 a genetic algorithm (GA),59–61 and configuration enumeration (ENUM).62 These CSP schemes are discussed next.
2.2.1
Ab initio random structure searching.
At the core of the searching efforts we used the ab initio random structure searching (AIRSS) method57,58 to generate random “sensible” structures constrained by space group symmetry, density, and atomic bond-length. AIRSS has been recognised as a successful tool to efficiently sample the vast composition space in many materials.57,58 Upon generating the structures, we fully optimised them using DFT to locate their local minima. All relaxations were performed individually, that is, without any dependence between calculations. Our initial pool of trial structures was ∼10
000 in the entire LiVO2–Li3NbO4 pseudobinary tieline with no constraints on stoichiometry. However, to reduce the computational cost we imposed a volume constraint Vc based on the total number of Li, Nb, V, and O atoms Natoms in the unit cell such that 5Natoms Å3 ≤ Vc ≤ 15Natoms Å3.
2.2.2 Evolutionary search.
We also used an evolutionary search scheme to identify (meta-)stable structures based on a genetic algorithm (GA) as implemented in the USPEX code featuring local optimisation, real-space representation, and flexible physically motivated variation operators.59–61 For computational efficiency, instead of a full variable-composition evolutionary algorithm to sample the entire quaternary composition space, we instead only used USPEX for selected chemical compositions that were less than 200 meV per atom from the hull as found by the AIRSS searches. Due to the associated computational cost, we could simulate up to a maximum number of 28 atoms per unit cell. The number of structures in the initial generation and each subsequent generation was set to 50. In each generation (except the first generation), 40% of structures were generated using heredity, 40% were generated by mutation (20% by soft mutation, 15% by transmutation, and 5% using spin mutation), and 20% were generated randomly. During the structure search process, the structures in each generation were relaxed through a three-step process with increasing precision. Underlying structure relaxations and energy calculations were done using the VASP package.
2.2.3 Configuration enumeration.
The symmetrically distinct cation ordering in the LiVO2–Li3NbO4 pseudobinary tieline was also evaluated by means of configuration enumeration (ENUM). To do this, we started with a supercell of the I
3m-Li3NbO4 structure (64 atoms), replaced Nb sites with V sequentially while removing Li atoms to maintain charge balance. For each step of the removal/addition process, all configurations were enumerated and subsequently relaxed using VASP. The best candidates, based on a Boltzmann probability distribution and taking into account configurational entropy, were selected as the starting point for the next step where again the removal/addition process was carried out until we reached the R
m-LiVO2 composition. Overall, about 100 cation ordering configurations were produced along the LiVO2–Li3NbO4 pseudobinary tieline.
2.3 Computational workflow
The computational workflow used in this work is schematically presented in Fig. 1. Here we have employed three different CSP techniques ‘independently’ with no shared information with each other at the time of structure generation. We collated all the structures from the different sources only at the stage of analysis. In the very first step, we collected all the structures that contain Li–Nb–V–O including the end-members (LiVO2 and Li3NbO4) from the major databases MP, OQMD, NOMAD, and AFLOW. Then, we started generating our own structures along the LiVO2–Li3NbO4 pseudobinary tieline using AIRSS. After generating a few thousand structures, we got a good estimate on the relevant stoichiometries with low formation energy (usually less than 100 meV per atom) to do more directed searches using USPEX and ENUM. This approach proved to be very efficient for our system, and we collected a total of 11
435 DFT-computed data to qualitatively describe the quaternary Li–Nb–V–O composition–structure space. The thermodynamic phase stability of the candidates in this pool of data was then evaluated by means of plotting a convex hull where we screened the materials whose hull distance was less than 30 meV per atom. Through this screening process, we identified six different stoichiometries, which were then subjected to more detailed analysis (see Results and discussions section). The first quantity that we compute is the theoretical capacity Qmax, and our screening criterion for selecting structures for the next stage was Qmax ≥ 400 mA h g−1. In the next step, we chose candidates having band gap values smaller than 2.0 eV, which was followed by other cathode property calculations, including volume change, average voltage profile, mechanical and dynamical (only selected compositions due to computational cost) stability, Li+ migration pathways, and high-temperature stability. Finally, combining all this information, we propose the best candidates as cathode materials along with a design strategy to search for even better possible cathode materials.
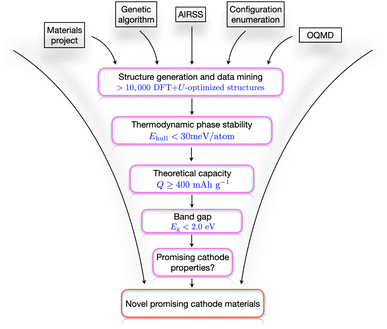 |
| Fig. 1 Computational search workflow. A schematic high-throughput computational workflow is shown that is used in this work to search for novel promising cathode materials. | |
3 Results and discussions
3.1 0 K phase stability and crystal structures
To determine the thermodynamic phase stability at 0 K and to identify novel (meta-)stable structures along the LiVO2–Li3NbO4 pseudobinary tieline, we calculated a convex hull where the end members are Li3NbO4 and LiVO2. The formation energy Ef of any stoichiometry in this tieline are calculated as | Ef[(LiVO2)x(Li3NbO4)(1−x)] = E[(LiVO2)x(Li3NbO4)(1−x)] − xE[LiVO2] − (1 − x)E[Li3NbO4], | (1) |
where E[LiVO2] and E[Li3NbO4] are the DFT computed total energy of the R
m-LiVO2 and I
3m-Li3NbO4 structures, taken from the Materials Project (MP) database31,32 and subsequently optimised with the same DFT settings applied to all other structures. The computed convex hull based on a total of about 10
000 DFT-optimised crystal structures that include both (i) available data in public databases and (ii) our own generated structures based on AIRSS, GAs, and ENUM, is shown in Fig. 2 with points coloured by the provenance of each structure.
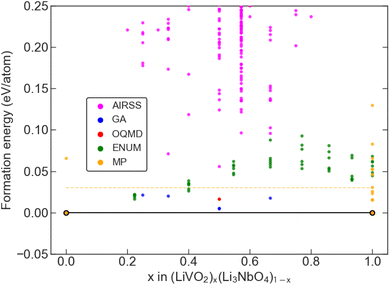 |
| Fig. 2 Convex hull diagram. Convex hull plot along the pseudobinary tieline of LiVO2 and Li3NbO4 showing the formation energy (eV per atom) versus composition along the tieline. The different colours represent the different data sources. The dashed horizontal line is the 30 meV per atom limit on the formation energy, which we take as the 0 K thermodynamic limit. | |
We identified five chemical compositions, along with an experimentally known stoichiometry, which are within 30 meV per atom of the convex hull, as marked by the yellow dashed horizontal line in Fig. 2, which is a reasonable choice of synthesisability of a material in high-throughput studies.33,63–65 Of these six phases, four were identified with the GA and two with ENUM. The predicted ground-state structures for all six compositions, which exhibit a disordered rock-salt crystal structure, are shown in Fig. 3 along with their effective space group. Further details about these structures are provided in Table 1 and 2 and an extended convex hull plot is provided in the ESI (Fig. S1).†
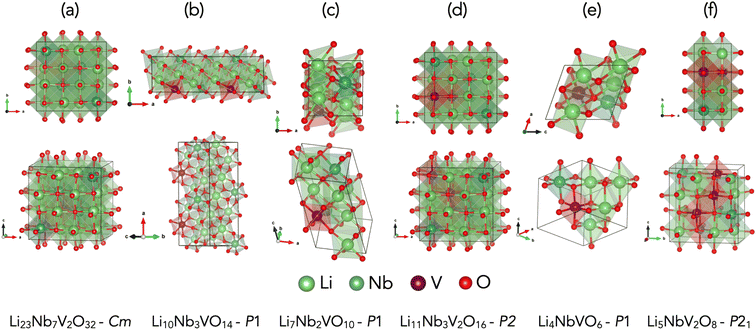 |
| Fig. 3 Predicted ground state crystal structures. Ground state crystal structures found by different crystal structure prediction algorithms whose formation energy value lies within 30 meV per atom from the convex hull of LiVO2–Li3NbO4 pseudobinary tieline for (a) Li23Nb7V2O32 (b) Li10Nb3VO14 (c) Li7Nb2VO10 (d) Li11Nb3V2O16 and (e) Li4NbVO6 (f) Li5NbV2O8. | |
Table 1 Chemical formula, composition, hull distance, space group, and provenance of the six crystal structures identified using our computational framework that are predicted to be synthesisable
Chemical formula |
x in (LiVO2)x (Li3NbO4)(1−x) |
Hull distance (Ehull) (meV per atom) |
Space group |
Provenance (eV) |
Li23Nb7V2O32 |
0.22 |
16.5 |
Cm
|
ENUM |
Li10Nb3VO14 |
0.25 |
21.3 |
P1 |
GA |
Li7Nb2VO10 |
0.33 |
20.2 |
P1 |
GA |
Li11Nb3V2O16 |
0.40 |
26.4 |
P2 |
ENUM |
Li4NbVO6 |
0.5 |
5.0 |
P1 |
GA |
Li5NbV2O8 |
0.67 |
17.5 |
P21 |
GA |
Table 2 Optimised conventional unit cell lattice parameters of different stoichiometries
Chemical formula |
a (Å) |
b (Å) |
c (Å) |
α (deg) |
β (deg) |
γ (deg) |
Li23Nb7V2O32 |
8.506 |
8.378 |
8.506 |
90.0 |
90.0 |
90.0 |
Li10Nb3VO14 |
15.914 |
5.207 |
6.567 |
103.5 |
90.5 |
90.9 |
Li7Nb2VO10 |
5.172 |
5.322 |
7.769 |
70.5 |
108.3 |
95.5 |
Li11Nb3V2O16 |
8.571 |
8.326 |
8.529 |
90.0 |
90.0 |
90.0 |
Li4NbVO6 |
5.116 |
5.145 |
5.183 |
100.5 |
78.3 |
61.9 |
Li5NbV2O8 |
4.079 |
8.297 |
8.830 |
90.0 |
90.0 |
90.0 |
3.2 Theoretical capacity
The theoretical capacity Qmax is an important metric for the design and discovery of novel cathode materials, as it reflects the battery capacity in terms of storing charged ions such as Li+. We calculated Qmax for all our six selected stoichiometries to gain insights into their charging capacity. To estimate Qmax, we used the following equation | 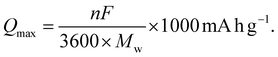 | (2) |
Here, n represents the number of charge carriers, F is the Faraday constant, and Mw is the molecular weight of the material. Using eqn (2), we estimate the value of Qmax for all six stoichiometries in this work. The results are shown in Fig. 4. We observe an almost linear behavior of the Qmax with composition x on the LiVO2–Li3NbO4 pseudobinary tieline. This observation suggests that higher Qmax can be obtained towards the Li3NbO4-rich side of the LiVO2–Li3NbO4 pseudobinary tieline. Comparing the computed Qmax values with those of known cathode materials suggests that the novel stoichiometries are found to outperform others by having higher capacities. However, we note that the experimental capacity of Li5NbV2O8 is lower than the computed Qmax (Fig. 4). There could be many factors behind this, since the experimental measurement of electrochemical performance depends on optimising the device used for the testing, which is highly dependent on selecting the correct mixture of active phase, carbon and polymer, and the protocol used for dispersing these, which are not included in our theoretical calculations.
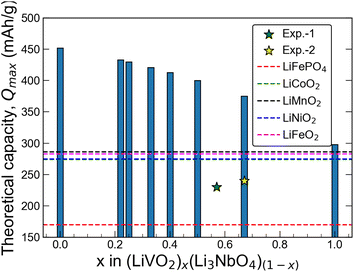 |
| Fig. 4 Theoretical capacity as a function of composition. Theoretical capacity as a function of composition along the LiVO2–Li3NbO4 tieline. Exp. 1 and 2 values are taken from ref. 21 and 23, respectively. | |
3.3 Electronic structure, density of states, and band gap
The electronic band structure provides useful information about the occupancy of the electronic energy levels of an electron within the solid. In Fig. 5 we compile the electronic band structures and the total and element-decomposed density of states (DOS) of all the novel phases discovered in this work. The calculated band structures of the end members (R
m-LiVO2 and I
3m-Li3NbO4) are shown in the SI (Fig. S2†), and the presence of high-energy V-3d states in the valence band makes the band gap of LiVO2 significantly lower than that of Li3NbO4. One may expect that the intermediate stoichiometries that lie along the R
m-LiVO2 and I
3m-Li3NbO4 pseudobinary tieline would also have a reduced band gap compared to that of Li3NbO4. Interestingly, for Li23Nb7V2O32 (Fig. 5(a)) we observe flat bands corresponding to V-3d states (black solid lines) both above and below the Fermi level. We rationalise this observation by noting that the small amount of V atoms per cell act as an impurity in Li23Nb7V2O32, giving rise to the flat bands that significantly reduce the gap of the system compared to the end member materials. As the relative amount of V atoms increases by adding more LiVO2 to Li3NbO4, the flat bands broaden. We also observe that the total DOS near the top of the valence band, arising mostly from V-3d states, increases almost linearly with increasing LiVO2 composition, approaching the DOS of LiVO2. Overall, doping Li3NbO4 with a minimal amount of V atoms leads to a decrease in band gap, and observation that may serve as a design rule for the discovery of novel cathode materials.
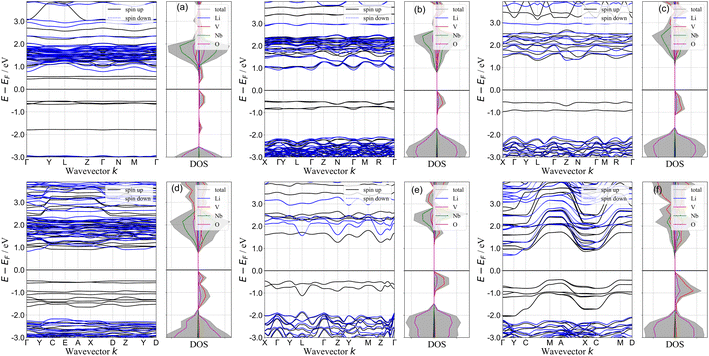 |
| Fig. 5 Band structure and density of states plots. Electronic band structure and element-decomposed density of states of (a) Li23Nb7V2O32 (b) Li10Nb3VO14 (c) Li7Nb2VO10 (d) Li11Nb3V2O16 (e) Li4NbVO6 and (f) Li5NbV2O8 structures. | |
The band gap of a material in part determines its electronic conductivity, and the electronic resistance of electrode materials can be analyzed using DOS plots. For an ideal cathode material, the band gap should be as small as possible, and ideally metallic. In this work, we computed the band gap with DFT+U for all the novel stoichiometries from their difference between CBM and VBM in the band structures shown in Fig. 5. We show the computed band gaps in Fig. 6(a). For all structures, the band gap is below 2.0 eV, which is significantly lower compared to that of R
m-LiVO2 and I
3m-Li3NbO4, and also comparable to the gap of other well-known cathode materials.66–68 Note that the band gap values that we report here correspond to the fully-lithiated structures and thus represent the theoretical upper limit, which may nonetheless be difficult to achieve in reality. For completeness, we also computed the band gap of the fully-delithiated structures (theoretical lower limit) where band gaps vanished due to their metallic nature. Therefore, we expect that the newly proposed stoichiometries will have lower band gaps for practical applications compared to the values reported here. Overall, the lower band gaps found in all the stoichiometries, especially at low LiVO2 content (Li23Nb7V2O32), further endorses the potential of these compositions as cathode materials.
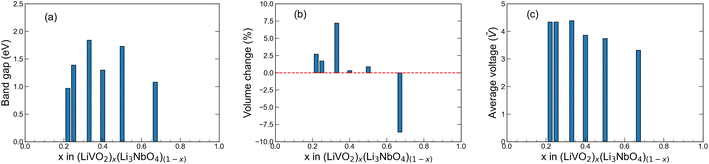 |
| Fig. 6 Band gap, volume change and average voltage plots. (a) Band gap, (b) volume change upon full delithiation and (c) average voltage upon full delithiation for the predicted stoichiometries in this work. | |
3.4 Volumetric change
Low volumetric changes of the electrode materials are desired to minimise the risk of dewetting and cracking of an ASSB battery during cycling. To estimate the change in volume upon full lithiation/delithiation in the new promising stoichiometries, we used the following expression:33 | 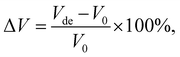 | (3) |
where Vde and V0 represent the volume of the cell upon full delithiation and lithiation (the predicted original structure in this work), respectively. Consequently, ΔV represents the maximum theoretical limit of volume change of the cathode materials during actual battery application. Using this equation, we compute the ΔV value for all six stoichiometries (along with the end members for comparison) and the results are shown in Fig. 6(b). We see that, except for the experimentally known stoichiometry of Li5NbV2O8, all other five novel stoichiometries have ΔV values smaller than 7.5%, which indicates their notable structural stability upon full lithiation/delithiation as compared to many other known cathode materials.8,69 The overall small volume change observed in our calculations for all compositions studied here is consistent with the work of Yabuuchi and co-workers,23 where they attributed the small volume change to the migration of V atoms into small and narrow tetrahedral sites. This process leads to an increase in the electrostatic interaction and as a result, the crystal lattice expands upon Li extraction, a feature we also observe in our calculations that results in a positive ΔV in most cases.
3.5 Average voltage
Electrochemical average voltages for the new structures were calculated from the available DFT total energies. The average voltage
for a given stoichiometry, Lix1NbVO, can be computed by considering the individual changes in voltage upon the step-wise removal of Li, e.g., Lix2NbVO with x1 > x2 as:70 | 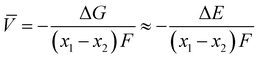 | (4) |
|  | (5) |
|  | (6) |
E(Lix1NbVO) and E(NbVO) are the DFT-computed total energies of the fully-lithiated and delithiated phases, respectively. In eqn (5), we set x2 = 0 assuming all the Li ions are removed from the structure in one step, yielding a simple approximation to
compared to a complete piece-wise approximation to the voltage curve.33,69,71 The computed value of
for all six stoichiometries is shown in Fig. 6(c). We find that stoichiometries with a smaller LiVO2 content have a higher
. The experimentally-known stoichiometry, Li5NbV2O8, has the lowest value of
. The calculated achievable values of
for the stoichiometries on the Li3NbO4-rich side compare well to other well-known cathode materials,8,69 encouraging further work to find even higher
values and suggesting a design rule for the discovery of improved cathode materials.
Here it is to be noted that to calculate a typical piece-wise voltage profile for every novel stoichiometry, the ground-state structure needs to be identified for each of the new composition emerged upon removal of Li+ from the host stoichiometry. However, this is a challenging task as the solid-solution phases are metastable at 0 K and more sophisticated computational techniques such as DFT-based cluster expansion together with grand canonical Monte Carlo simulations70 are necessary to identify such phases, which are not included in this work. Therefore, we provided a rather simple, but insightful criterion, average voltage values to compare our candidate materials, which has also been used successfully in other studies.33,69,71 Full open-circuit voltage profiles can be computed for comparing with the measured ones when the candidate materials are synthesised, but they are not crucial at this point to evaluate the potential of new stoichiometries.
3.6 Mechanical stability
One of the primary reasons for the degradation of cathode materials of LIBs is the phase transformation that may take place during the charge–discharge process. A phase transformation can happen if the structure is mechanically unstable, while a mechanically-stable structure can go through many charge–discharge cycles improving the lifetime of the material. In order to estimate the mechanical stability of the newly-identified stoichiometries, we calculated their elastic constants Cij and derive relevant mechanical properties that are useful for the design of cathode materials and that can be compared directly with experiments. The computed values of Cij for all stoichiometries are listed in the ESI Sec. III.† All our structures turn out to be mechanically stable according to the stability criteria laid down by Mouhat and co-workers, suggesting that these structures may go through many charge–discharge cycles as required for promising cathode materials. Moreover, we found that the values of C11, C22, and C33 are significantly higher than the values of C44, C55, and C66 for all six stoichiometries, suggesting strong resistance to uniaxial stress implying that shear deformations are more likely to occur,72 as observed in other Li-ion cathode materials.73,74
From the values of Cij, we further calculated the bulk modulus B, the shear modulus G, Pugh's Ratio B/G, Young's modulus E, and Poisson's ratio ν. These parameters play a critical role in determining the mechanical stability against an external force change and ductility of materials. In this work, we use the Voigt–Reuss–Hill (VRH) approximation75,76 to calculate the polycrystalline properties, which is the average of the Voigt and Reuss equations representing the upper and lower limits of the polycrystalline constants, respectively. The values of E and ν are then deduced using the following relationships:75,76
|  | (7) |
|  | (8) |
All the values of these polycrystalline properties are listed in Table 3. The B and G moduli are a measure of resistance to volume change by an applied pressure and a measure of resistance to reversible deformations upon shear stress, respectively. E represents the ratio of stress and strain and can reflect the hardness of materials. For all our stoichiometries, we note that the values of B are much larger than the corresponding values of G, suggesting that they have a higher resistance to volume deformations under hydrostatic pressure, which is also observed in other Li-ion cathode materials.73,74 Another important empirical metric used to characterise the brittle-ductility behavior of materials is Pugh's ratio B/G.77 A high B/G ratio is associated with ductility, whereas a low value generally represents brittleness. The critical value distinguishing the two behaviors was found by Ravindran and co-workers78 to be about 1.75. The Poisson ratio ν indicates the covalent nature of materials79 and higher values of ν are associated with better elastic properties.74
Table 3 Average mechanical properties: bulk modulus B (in GPa), shear modulus G (in GPa), Pugh's ratio B/G, Young's modulus E (in GPa), and Poisson's ratio ν for different stoichiometries
Chemical formula |
B
|
G
|
B/G |
E
|
ν
|
Li23Nb7V2O32 |
97.4 |
58.0 |
1.68 |
145.3 |
0.25 |
Li10Nb3VO14 |
97.6 |
63.2 |
1.54 |
156.0 |
0.23 |
Li7Nb2VO10 |
52.3 |
36.8 |
1.42 |
89.5 |
0.21 |
Li11Nb3V2O16 |
98.5 |
60.8 |
1.62 |
151.4 |
0.24 |
Li4NbVO6 |
106.5 |
70.1 |
1.52 |
172.4 |
0.23 |
Li5NbV2O8 |
109.7 |
81.6 |
1.34 |
196.1 |
0.20 |
Interestingly, we find that moving towards the Li3NbO4-rich side, B, G, and E decrease but B/G and ν increase. The relatively lower values of B, G, and E for Li3NbO4-rich compositions indicate that they possess improved mechanical properties, whereas the higher values of B/G and ν indicate that they exhibit more ductile character helping reduce Li dendrite formation role, a key advantage for ASSB applications. This suggests that, the newly discovered stoichiometries towards the Li3NbO4-rich side of the LiVO2–Li3NbO4 pseudobinary tieline will have better mechanical properties than the experimentally known stoichiometries of Li1.3Nb0.3V0.4O2 (ref. 21) and Li1.25Nb0.25V0.5O2 (ref. 23) with Li23Nb7V2O32 having the best ductile properties. Our results further suggest that it may be possible to search for even more ductile materials by considering more Li3NbO4-rich compositions for which B/G can reach values up to 1.75 and higher and thus may serve as a design rule for finding cathode materials with improved mechanical properties.
3.7 Dynamical stability
Fig. 7 shows the phonon dispersion and corresponding density of states (DOS) calculated under the harmonic approximation for two of our most promising stoichiometries: (a) Li23Nb7V2O32 and (b) Li4NbVO6. The absence of imaginary modes indicates that these two structures are dynamically stable, with no predicted phase transitions. We note that there is an imaginary mode with small frequency near Γ approaching from the (0, 0, 0.5) point for Li23Nb7V2O32. This can be attributed to an artifact of the Fourier interpolation which could be removed by calculating explicit dynamical matrices on a finer grid in this direction. However, this is computationally very expensive given the size of our disordered supercell and should not impact our conclusion that both structures are dynamically stable.
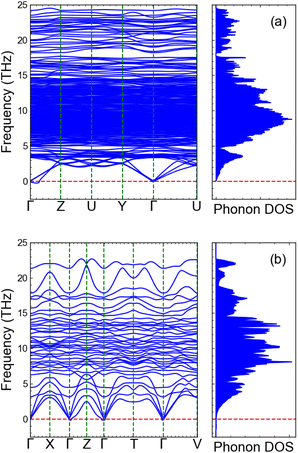 |
| Fig. 7 Phonon dispersion plots. Phonon dispersion under the harmonic approximation and the corresponding density of states for (a) Li23Nb7V2O32 and (b) Li4NbVO6. The absence of imaginary frequencies confirms the dynamical stability of the structures. | |
3.8 Kinetics of Li+ transport
Understanding the kinetics of Li+ transport is crucial for the design of any battery component. Atomistic mechanisms of Li+ transport, such as diffusion pathways and corresponding activation barriers, can provide useful information that is otherwise difficult to obtain from experiments. Over the past few decades, the kinetic transport of several Li-excess transition-metal oxide cathodes and anodes has been well studied based on percolation theory.80–85 In this work, we have utilised three common methodologies to obtain a qualitative understanding of atomistic diffusion processes in these materials: (i) AIMD (ii) CINEB, and (iii) BVSE. We initially ran a 40 ps computationally-expensive AIMD simulations on the various stoichiometries with and without Li+ vacancies at low (600 K), medium (1000 K) and high (1400 K) temperatures (Fig. S9†). In all cases, we did not find significant Li+ movement during the 40 ps run, revealing the sluggish nature of Li+ diffusion in these materials, likely owing to the rock-salt crystal framework. This is in good agreement with previous reports on Li3NbO4
86 and LiVO2.87 Next, we used another computationally-expensive technique, CI-NEB, on the simplest stoichiometry, Li4NbVO6, containing only 12 atoms in the unit cell with 4 unique Li sites. We chose this stoichiometry for the convenience of CI-NEB calculations where identifying predefined diffusion pathways is a requirement. However, a few of our stoichiometries contain large supercells where identifying unique Li sites and enumerating all possible corresponding unique diffusion pathways is a challenging task. Therefore, in the last step we used a computationally inexpensive BVSE approach to qualitatively estimate diffusion barriers and reaction pathways in these complex disordered materials.
In Fig. 8, we compile our CI-NEB and BVSE findings for Li4NbVO6 to benchmark these two methods. The results for the remaining stoichiometries are shown in Fig. S3–S7 in the ESI† (BVSE-computed full diffusion pathways and corresponding diffusion barriers). Fig. 8(a) represents the unit cell of the Li4NbVO6 crystal structure with four symmetrically unique Li sites marked on it. The various possible elementary Li+ jumps are marked with different colours. In Fig. 8(b), CI-NEB computed Li+ migration barriers are shown with the same colour coding that represents the different elementary Li+ hops in Fig. 8(a). The lowest CI-NEB barrier is found for the Li1 → Li4 → Li1 → Li4 pathway along the (001) direction, with an activation barrier of 0.56 eV (path-3). The full Li1 → Li4 → Li1 → Li4 pathway can be easily visualised in Fig. 8(c) in a larger supercell, marked by black arrows.
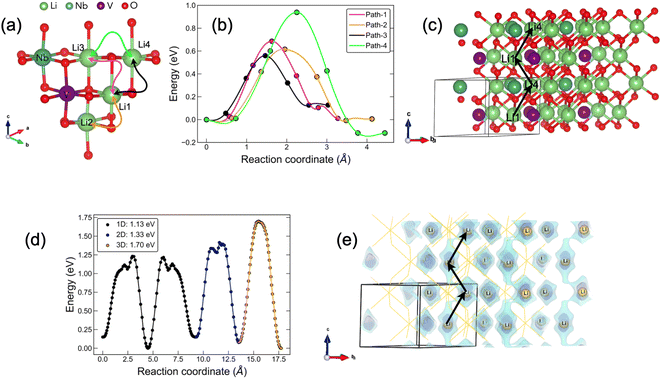 |
| Fig. 8 Schematic overview of the Li+ diffusion in Li4NbVO6. (a) Unit cell of Li4NbVO6 showing all possible elementary Li+ hops coloured according to their diffusion barriers. (b) DFT–CI–NEB computed energy barriers where the different colours correspond to the elementary jumps shown in (a). (c) The long-range minimum energy pathway for Li+ (Li1 ↔ Li4) is shown in a 2 × 2 × 2 supercell identified to be along the (001) direction marked by black arrows. (d) BVSE computed Li+ migration pathway for 1D, 2D, and 3D percolation network with corresponding activation barriers. (e) 3D network of Li+ pathways (blue isosurface) calculated by BVSE. The minimum energy pathway for Li+ migration is identified to be along the (001) direction (marked by black arrows) and matches exactly with the CI-NEB results shown in (c). | |
For the other stoichiometries, as mentioned earlier, we have a more complex spatial distribution of Li, Nb, and V ions in a rather large unit cell, making the CI-NEB approach unfeasible. Therefore, we use the BVSE method which is computationally fast and provides useful information for complex disordered materials. Since BVSE is rather less accurate in estimating activation barriers than CI-NEB, we first compare the BVSE-computed activation barrier for 1D, 2D, and 3D percolation networks and also the minimum energy pathway (MEP) with that of the CI-NEB computed results. In Fig. 8(d) we show the BVSE-predicted Li+ pathway and the corresponding activation barriers for 1D, 2D, and 3D percolation networks, which are 1.13 eV, 1.33 eV, and 1.70 eV, respectively. These values are significantly overestimated as compared to the CI-NEB computed value of 0.559 eV, likely due to the use of rigid structures in BVSE as opposed to CI-NEB. This suggests that BVSE computed diffusion barriers may act as an upper limit; however, the BVSE method does correctly identify the MEP for Li+ along the (001) direction (marked by black arrows in Fig. 8(e)) in agreement with the CI-NEB method. This endorses the suitability of the BVSE method for a qualitative comparison of the activation barriers corresponding to 1D, 2D, and 3D Li+ percolation networks for our other complex stoichiometries. The resulting BVSE activation barriers of Li+ migration are compiled in Table 4.
Table 4 Overall activation energy barrier for the 1D, 2D, and 3D percolation network of Li+ migration in the different novel stoichiometries estimated by BVSE
Chemical formula |
1D (eV) |
2D (eV) |
3D (eV) |
Li23Nb7V2O32 |
0.61 |
0.62 |
0.63 |
Li10Nb3VO14 |
0.76 |
0.98 |
1.41 |
Li7Nb2VO10 |
1.01 |
1.09 |
1.33 |
Li11Nb3V2O16 |
0.75 |
0.77 |
0.91 |
Li4NbVO6 |
1.13 |
1.33 |
1.70 |
Li5NbV2O8 |
1.18 |
1.34 |
3.40 |
We highlight that the end-member materials of the LiVO2–Li3NbO4 tieline, that is, LiVO2 and Li3NbO4, have a rather high Li+ activation energy barrier86,87 as compared to some of our stoichiometries discovered in this work. In particular, the lowest activation energy path for Li3NbO4 is found to be 1.13 eV86 and Panchmatia and co-workers reported that stoichiometric LiVO2 does not have mobile Li+.87 They were, however, able to increase Li+ mobility in lithiated non-stoichiometric LiVO2 compounds. In this regard, our Li3NbO4-rich compounds, such as Li23Nb7V2O32, appear more promising as evidenced by BVSE computed low 1D, 2D, and 3D percolation network Li+ migration barriers, likely due to the presence of a small amount of V doping. However, further study is required to get a complete picture of atomic diffusion processes and how to enhance Li+ movement in these promising stoichiometries.
3.9 High-temperature stability
For practical applications, cathode materials may need to operate at high temperatures, particularly in an ASSLIB setting, which can cause severe damage if the underlying crystal framework is not stable at that temperature. We used AIMD simulations at 600 K to investigate the impact of temperature on our newly discovered materials. From the AIMD trajectories, we compute the radial distribution function (RDF) of Nb–Nb and Nb–V at 0 ps and at 10 ps. We focus on these two pairs of atoms as they provide more insights into the overall structural stability during charging/discharging cycles at high temperatures compared to other pairings. Our results are shown in Fig. 9. We observe sharp peaks for both Nb–Nb and Nb–V pairs for all the stoichiometries after running for 10 ps at 600 K indicating the crystalline structure framework is preserved. This finding is further supported by the insignificant total energy variation with AIMD steps (Fig. S8 in ESI†). These results suggest that all our newly discovered structures are expected to have good thermal stability, ensuring the safety aspect for commercial battery applications.
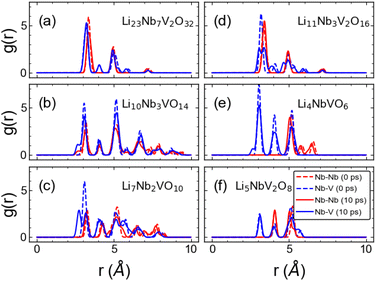 |
| Fig. 9 Radial distribution function plots. Radial distribution function between Nb–Nb (red) and Nb–V (blue) of (a) Li23Nb7V2O32 (b) Li10Nb3VO14 (c) Li7Nb2VO10 (d) Li11Nb3V2O16 (e) Li4NbVO6 and (f) Li5NbV2O8 structures at 0 ps (dashed line) and 10 ps (solid line) obtained from AIMD simulations at 600 K. | |
In Table 5, we summarise our key findings. For all six promising stoichiometries, we report their corresponding property values with an asterisk (*) mark representing the best value among the six stoichiometries. Overall, we find that the Li23Nb7V2O32 composition gets the highest number of * marks, excluding the mechanical/dynamical/high-temperature stability which is common to all the six stoichiometries. This indicates that Li23Nb7V2O32 is likely to be the best candidate as a cathode material for all-solid-state Li-ion battery applications.
Table 5 The computed properties of the novel stoichiometries discovered in this work. The asterisks (*) represents the best value for a given property. The band gap value without parenthesis and with parenthesis represent the indirect and direct band gap, respectively
Chemical formula |
E
hull (meV per atom) |
Q
max (mA h g−1) |
(V) |
Band gap (eV) |
ΔV (%) |
Mechanically stable? |
Dynamically stable? |
High-T stable? |
Activation barrier (eV) |
Li23Nb7V2O32 |
16.5 |
433* |
4.34* |
0.97 (0.98)* |
+2.7 |
✓ |
✓ |
✓ |
0.61* |
Li10Nb3VO14 |
21.3 |
430 |
4.34* |
1.39 (1.50) |
+1.7 |
✓ |
N/A |
✓ |
0.76 |
Li7Nb2VO10 |
20.2 |
421 |
4.39 |
1.84 (1.88) |
+7.2 |
✓ |
N/A |
✓ |
1.01 |
Li11Nb3V2O16 |
26.4 |
413 |
3.86 |
1.30 (1.43) |
+0.3* |
✓ |
N/A |
✓ |
0.75 |
Li4NbVO6 |
5.0* |
400 |
3.74 |
1.73 (1.76) |
+0.9 |
✓ |
✓ |
✓ |
1.13 |
Li5NbV2O8 |
17.5 |
375 |
3.32 |
1.08 (1.55) |
−8.6 |
✓ |
N/A |
✓ |
1.18 |
4 Conclusions
We present a comprehensive and systematic study of first-principles crystal structure prediction calculations on the LiVO2–Li3NbO4 tieline to identify novel stoichiometries with improved properties that can be used as cathode materials for ASSLIB applications. It is to be, however, noted that the applications of these materials are not limited to ASSLIBs and can be used for other conventional rechargeable Li-ion batteries which makes them even more attractive materials for wider battery applications. Based on the thermodynamic stability criterion applied over more than 10
000 DFT-computed structures, we identify five novel stoichiometries, Li23Nb7V2O32, Li10Nb3VO14, Li7Nb2VO10, Li11Nb3V2O16, Li4NbVO6, along with an experimentally known stoichiometry, Li5NbV2O8, all having a rock-salt crystal structure. From our calculations, we predict that all the novel stoichiometries have promising cathode properties, and highlight the largely unexplored Li3NbO4-rich side as having improved properties compared to the LiVO2-rich side. Our analysis suggests that Li23Nb7V2O32 will have the best cathode properties among all the stoichiometries considered in this work, having higher capacity, higher average voltage, lower band gap and lower activation barrier (see Table 5). Our work illustrates how theoretical calculations for the prediction of novel vanadium-niobate-based cathode materials can guide experimental synthesis, and paves the way for the discovery of other disordered ASSLIB cathode materials.
Code availability
All the major codes, except for VASP (see https://www.vasp.at/) which requires a license, used in this work are publicly-available such as AIRSS (see https://airss-docs.github.io/) and USPEX (see https://uspex-team.org/en) for crystal structure prediction, MATADOR for high-throughput work, database management and convex hull plot (see https://matador-db.readthedocs.io/en/latest/), Pymatgen for electronic structure and radial distribution function analysis (see https://pymatgen.org/), Phonopy for phonon analysis (see https://phonopy.github.io/phonopy/), Vaspkit for mechanical property calculations (see https://vaspkit.com/), Atomic Simulation Environment (ASE) for CI-NEB calculations and general purpose use (see https://wiki.fysik.dtu.dk/ase/) and softBV for diffusion pathway analysis (see https://www.dmse.nus.edu.sg/asn/softBV-GUI.html). The in-house configuration enumeration code (config_enum) used for CSP can be made available upon request from the corresponding authors.
Data availability
The main data supporting the findings of this study are available within the paper and its ESI.† Further numerical data are available from the authors upon reasonable request. All the DFT-optimised structure files are available in the GitHub repository: https://github.com/bkarasulu/Li-V-Nb-O_structures.
Author contributions
T. C. and B. K. contributed to the conception and design of this study, and T. C. was responsible for performing the calculations and detailed analysis. B. M. performed one of the phonon calculations and provided critical feedback regarding phonon analysis. A. T. and R. I. W contributed to the initial discussion. T. C. drafted the original manuscript, which was then reviewed and revised by all the co-authors. All authors reviewed and approved the manuscript prior to submission.
Conflicts of interest
The authors declare no competing interests.
Acknowledgements
T. C. and B. K. acknowledge support from the UK Engineering and Physical Sciences Research Council (EPSRC) through the Early Career Fellowship grant (EP/T026138/1). T. C. further acknowledges fruitful discussions with Benedict Saunders and Huseyin Sener Sen. B. M. acknowledges support from a UKRI Future Leaders Fellowship (MR/V023926/1), from the Gianna Angelopoulos Programme for Science, Technology, and Innovation, and from the Winton Programme for the Physics of Sustainability. A. T. and R. I. W. thank Companhia Brasileira de Metalurgia e Mineração (CBMM) for funding. Computing facilities were provided by the Scientific Computing Research Technology Platform (SCRTP) at the University of Warwick. DFT calculations were performed using the Avon, Sulis and Orac HPC platforms, and Sulis is funded by the EPSRC Grant (EP/T022108/1) and the HPC Midlands+ consortium. Part of the calculations were performed using resources provided by the Cambridge Tier-2 system (operated by the University of Cambridge Research Computing Service and funded by EPSRC [EP/P020259/1]).
References
- X. Zeng, M. Li, D. Abd El-Hady, W. Alshitari, A. S. Al-Bogami, J. Lu and K. Amine, Adv. Energy Mater., 2019, 9, 1900161 CrossRef.
- J. W. Choi and D. Aurbach, Nat. Rev. Mater., 2016, 1, 16013 CrossRef CAS.
- M. Li, J. Lu, Z. Chen and K. Amine, Adv. Mater., 2018, 30, 1800561 CrossRef PubMed.
- X. Zeng, C. Zhan, J. Lu and K. Amine, Chem, 2018, 4, 690–704 CAS.
- K. Turcheniuk, D. Bondarev, V. Singhal and G. Yushin, Nature, 2018, 559, 467 CrossRef CAS PubMed.
- Y. Ding, Z. P. Cano, A. Yu, J. Lu and Z. Chen, Electrochem. Energy Rev., 2019, 2, 28 Search PubMed.
- K. Bourzac, Nature, 2015, 526, S105 CrossRef CAS PubMed.
- N. Nitta, F. Wu, J. T. Lee and G. Yushin, Mater. Today, 2015, 18, 252–264 CrossRef CAS.
- J. B. Goodenough and Y. Kim, Chem. Mater., 2010, 22, 587–603 CrossRef CAS.
- P. Kubiak, Z. Cen, C. M. López and I. Belharouak, J. Power Sources, 2017, 372, 16–23 CrossRef CAS.
- L. M. Morgan, M. P. Mercer, A. Bhandari, C. Peng, M. M. Islam, H. Yang, J. Holland, S. W. Coles, R. Sharpe, A. Walsh, B. J. Morgan, D. Kramer, M. S. Islam, H. E. Hoster, J. S. Edge and C.-K. Skylaris, Prog. Energy, 2021, 4, 012002 CrossRef.
- T. Famprikis, P. Canepa, J. A. Dawson, M. S. Islam and C. Masquelier, Nat. Mater., 2019, 18, 1278–1291 CrossRef CAS PubMed.
- M. S. Whittingham, Chem. Rev., 2014, 114, 11414–11443 CrossRef CAS PubMed.
- Z. Lu and J. R. Dahn, J. Electrochem. Soc., 2002, 149, A815 CrossRef CAS.
- A. D. Robertson and P. G. Bruce, Chem. Commun., 2002, 2790–2791 RSC.
- N. Yabuuchi, K. Yoshii, S.-T. Myung, I. Nakai and S. Komaba, J. Am. Chem. Soc., 2011, 133, 4404–4419 CrossRef CAS PubMed.
- N. Yabuuchi, R. Hara, M. Kajiyama, K. Kubota, T. Ishigaki, A. Hoshikawa and S. Komaba, Adv. Energy Mater., 2014, 4, 1301453 CrossRef.
- C. Johnson, J.-S. Kim, C. Lefief, N. Li, J. Vaughey and M. Thackeray, Electrochem. Commun., 2004, 6, 1085–1091 CrossRef CAS.
- J. Bréger, M. Jiang, N. Dupré, Y. S. Meng, Y. Shao-Horn, G. Ceder and C. P. Grey, J. Solid State Chem., 2005, 178, 2575–2585 CrossRef.
- K. Shimoda, M. Oishi, T. Matsunaga, M. Murakami, K. Yamanaka, H. Arai, Y. Ukyo, Y. Uchimoto, T. Ohta, E. Matsubara and Z. Ogumi, J. Mater. Chem. A, 2017, 5, 6695–6707 RSC.
- N. Yabuuchi, M. Takeuchi, S. Komaba, S. Ichikawa, T. Ozaki and T. Inamasu, Chem. Commun., 2016, 52, 2051–2054 RSC.
- N. Yabuuchi, M. Nakayama, M. Takeuchi, S. Komaba, Y. Hashimoto, T. Mukai, H. Shiiba, K. Sato, Y. Kobayashi, A. Nakao, M. Yonemura, K. Yamanaka, K. Mitsuhara and T. Ohta, Nat. Commun., 2016, 7, 13814 CrossRef CAS PubMed.
- M. Nakajima and N. Yabuuchi, Chem. Mater., 2017, 29, 6927–6935 CrossRef CAS.
- G. Kresse and J. Furthmüller, Comput. Mater. Sci., 1996, 6, 15–50 CrossRef CAS.
- G. Kresse and J. Furthmüller, Phys. Rev. B: Condens. Matter Mater. Phys., 1996, 54, 11169–11186 CrossRef CAS PubMed.
- G. Kresse and D. Joubert, Phys. Rev. B: Condens. Matter Mater. Phys., 1999, 59, 1758–1775 CrossRef CAS.
- J. P. Perdew, K. Burke and M. Ernzerhof, Phys. Rev. Lett., 1996, 77, 3865–3868 CrossRef CAS PubMed.
- P. E. Blöchl, Phys. Rev. B: Condens. Matter Mater. Phys., 1994, 50, 17953–17979 CrossRef PubMed.
- J. Hubbard, Proc. R. Soc. London, Ser. A, 1963, 276, 238–257 Search PubMed.
- V. I. Anisimov, J. Zaanen and O. K. Andersen, Phys. Rev. B: Condens. Matter Mater. Phys., 1991, 44, 943–954 CrossRef CAS PubMed.
- A. Jain, S. P. Ong, G. Hautier, W. Chen, W. D. Richards, S. Dacek, S. Cholia, D. Gunter, D. Skinner, G. Ceder and K. A. Persson, APL Mater., 2013, 1, 011002 CrossRef.
- S. P. Ong, S. Cholia, A. Jain, M. Brafman, D. Gunter, G. Ceder and K. A. Persson, Comput. Mater. Sci., 2015, 97, 209–215 CrossRef.
- Z. Zhang, X. Zhang, X. Zhao, S. Yao, A. Chen and Z. Zhou, ACS Omega, 2019, 4, 7822–7828 CrossRef CAS PubMed.
- H. J. Monkhorst and J. D. Pack, Phys. Rev. B: Solid State, 1976, 13, 5188–5192 CrossRef.
- S. P. Ong, W. D. Richards, A. Jain, G. Hautier, M. Kocher, S. Cholia, D. Gunter, V. L. Chevrier, K. A. Persson and G. Ceder, Comput. Mater. Sci., 2013, 68, 314–319 CrossRef CAS.
- M. L. Evans and A. J. Morris, J. Open Source Softw., 2020, 5, 2563 CrossRef.
- K. Momma and F. Izumi, J. Appl. Crystallogr., 2011, 44, 1272–1276 CrossRef CAS.
- V. Wang, N. Xu, J.-C. Liu, G. Tang and W.-T. Geng, Comput. Phys. Commun., 2021, 267, 108033 CrossRef CAS.
- F. Mouhat and F.-X. Coudert, Phys. Rev. B: Condens. Matter Mater. Phys., 2014, 90, 224104 CrossRef.
- K. Parlinski, Z. Q. Li and Y. Kawazoe, Phys. Rev. Lett., 1997, 78, 4063–4066 CrossRef CAS.
- A. Togo, L. Chaput, T. Tadano and I. Tanaka, J. Phys.: Condens.Matter, 2023, 35, 353001 CrossRef PubMed.
- A. Togo, J. Phys. Soc. Jpn., 2023, 92, 012001 CrossRef.
- J. H. Lloyd-Williams and B. Monserrat, Phys. Rev. B: Condens. Matter Mater. Phys., 2015, 92, 184301 CrossRef.
- B. Monserrat, J. Phys.: Condens.Matter, 2018, 30, 083001 CrossRef PubMed.
- G. Henkelman, B. P. Uberuaga and H. Jónsson, J. Chem. Phys., 2000, 113, 9901–9904 CrossRef CAS.
- G. Henkelman and H. Jónsson, J. Chem. Phys., 2000, 113, 9978–9985 CrossRef CAS.
- A. H. Larsen, J. J. Mortensen, J. Blomqvist, I. E. Castelli, R. Christensen, M. Dułak, J. Friis, M. N. Groves, B. Hammer, C. Hargus, E. D. Hermes, P. C. Jennings, P. B. Jensen, J. Kermode, J. R. Kitchin, E. L. Kolsbjerg, J. Kubal, K. Kaasbjerg, S. Lysgaard, J. B. Maronsson, T. Maxson, T. Olsen, L. Pastewka, A. Peterson, C. Rostgaard, J. Schiøtz, O. Schütt, M. Strange, K. S. Thygesen, T. Vegge, L. Vilhelmsen, M. Walter, Z. Zeng and K. W. Jacobsen, J. Phys.: Condens. Matter, 2017, 29, 273002 CrossRef PubMed.
- H. Chen, L. L. Wong and S. Adams, Acta Crystallogr., Sect. B, 2019, 75, 18–33 CrossRef CAS PubMed.
- H. Chen and S. Adams, IUCrJ, 2017, 4, 614–625 CrossRef CAS PubMed.
- L. L. Wong, K. C. Phuah, R. Dai, H. Chen, W. S. Chew and S. Adams, Chem. Mater., 2021, 33, 625–641 CrossRef CAS.
- S. Kirklin, J. E. Saal, B. Meredig, A. Thompson, J. W. Doak, M. Aykol, S. Rühl and C. Wolverton, npj Comput. Mater., 2015, 1, 15010 CrossRef CAS.
- J. E. Saal, S. Kirklin, M. Aykol, B. Meredig and C. Wolverton, JOM, 2013, 65, 1501–1509 CrossRef CAS.
- C. Draxl and M. Scheffler, J. Phys.: Mater., 2019, 2, 036001 CAS.
- C. Draxl and M. Scheffler, MRS Bull., 2018, 43, 676–682 CrossRef.
- M. Scheffler, M. Aeschlimann, M. Albrecht, T. Bereau, H.-J. Bungartz, C. Felser, M. Greiner, A. Groß, C. T. Koch, K. Kremer, W. E. Nagel, M. Scheidgen, C. Wöll and C. Draxl, Nature, 2022, 604, 635–642 CrossRef CAS PubMed.
- S. Curtarolo, W. Setyawan, G. L. Hart, M. Jahnatek, R. V. Chepulskii, R. H. Taylor, S. Wang, J. Xue, K. Yang, O. Levy, M. J. Mehl, H. T. Stokes, D. O. Demchenko and D. Morgan, Comput. Mater. Sci., 2012, 58, 218–226 CrossRef CAS.
- C. J. Pickard and R. J. Needs, Phys. Rev. Lett., 2006, 97, 045504 CrossRef PubMed.
- C. J. Pickard and R. J. Needs, J. Phys.: Condens. Matter, 2011, 23, 053201 CrossRef PubMed.
- A. R. Oganov and C. W. Glass, J. Chem. Phys., 2006, 124, 244704 CrossRef PubMed.
- A. R. Oganov, A. O. Lyakhov and M. Valle, Acc. Chem. Res., 2011, 44, 227–237 CrossRef CAS PubMed.
- A. O. Lyakhov, A. R. Oganov, H. T. Stokes and Q. Zhu, Comput. Phys. Commun., 2013, 184, 1172–1182 CrossRef CAS.
- G. L. W. Hart and R. W. Forcade, Phys. Rev. B: Condens. Matter Mater. Phys., 2008, 77, 224115 CrossRef.
- Z. Zhu, I.-H. Chu and S. P. Ong, Chem. Mater., 2017, 29, 2474–2484 CrossRef CAS.
- G. Hautier, A. Jain, S. P. Ong, B. Kang, C. Moore, R. Doe and G. Ceder, Chem. Mater., 2011, 23, 3495–3508 CrossRef CAS.
- Z. Wang, I.-H. Chu, F. Zhou and S. P. Ong, Chem. Mater., 2016, 28, 4024–4031 CrossRef CAS.
- A. Chakraborty, M. Dixit, D. Aurbach and D. T. Major, npj Comput. Mater., 2018, 4, 60 CrossRef.
- M. Momeni, H. Yousefi Mashhour and M. M. Kalantarian, J. Alloys Compd., 2019, 787, 738–743 CrossRef CAS.
- N. Tsebesebe, K. Kgatwane, R. Ledwaba and P. Ngoepe, J. Phys.: Conf. Ser., 2022, 2298, 012010 CrossRef.
- T. Mueller, G. Hautier, A. Jain and G. Ceder, Chem. Mater., 2011, 23, 3854–3862 CrossRef CAS.
- A. Urban, D.-H. Seo and G. Ceder, npj Comput. Mater., 2016, 2, 16002 CrossRef CAS.
- F. Zhou, M. Cococcioni, C. A. Marianetti, D. Morgan and G. Ceder, Phys. Rev. B: Condens. Matter Mater. Phys., 2004, 70, 235121 CrossRef.
- H. Mohapatra and C. J. Eckhardt, J. Phys. Chem. B, 2008, 112, 2293–2298 CrossRef CAS PubMed.
- Y. Xie, H.-T. Yu, T.-F. Yi and Y.-R. Zhu, ACS Appl. Mater. Interfaces, 2014, 6, 4033–4042 CrossRef CAS PubMed.
- X. Hou, J. Liang, T. Zhang, Y. Li, S. Tang, H. Sun, J. Zhang and H. Xie, J. Phys. Chem. C, 2017, 121, 22656–22664 CrossRef CAS.
- A. Reuss, Z. Angew. Math. Mech., 1929, 9, 49–58 CrossRef CAS.
- R. Hill, Proc. Phys. Soc., 1952, 65, 349 CrossRef.
- S. Pugh, London, Edinburgh Dublin Phil. Mag. J. Sci., 1954, 45, 823–843 CrossRef CAS.
- P. Ravindran, L. Fast, P. A. Korzhavyi, B. Johansson, J. Wills and O. Eriksson, J. Appl. Phys., 1998, 84, 4891–4904 CrossRef CAS.
- V. V. Bannikov, I. R. Shein and A. L. Ivanovskii, Phys. Status Solidi RRL, 2007, 1, 89–91 CrossRef CAS.
- R. J. Clément, Z. Lun and G. Ceder, Energy Environ. Sci., 2020, 13, 345–373 RSC.
- H. Liu, Z. Zhu, Q. Yan, S. Yu, X. He, Y. Chen, R. Zhang, L. Ma, T. Liu, M. Li, R. Lin, Y. Chen, Y. Li, X. Xing, Y. Choi, L. Gao, H. S.-y. Cho, K. An, J. Feng, R. Kostecki, K. Amine, T. Wu, J. Lu, H. L. Xin, S. P. Ong and P. Liu, Nature, 2020, 585, 63–67 CrossRef CAS PubMed.
- P. Barnes, Y. Zuo, K. Dixon, D. Hou, S. Lee, Z. Ma, J. G. Connell, H. Zhou, C. Deng, K. Smith, E. Gabriel, Y. Liu, O. O. Maryon, P. H. Davis, H. Zhu, Y. Du, J. Qi, Z. Zhu, C. Chen, Z. Zhu, Y. Zhou, P. J. Simmonds, A. E. Briggs, D. Schwartz, S. P. Ong and H. Xiong, Nat. Mater., 2022, 21, 795–803 CrossRef CAS PubMed.
- A. Urban, J. Lee and G. Ceder, Adv. Energy Mater., 2014, 4, 1400478 CrossRef.
- Y. Zeng, B. Ouyang, J. Liu, Y.-W. Byeon, Z. Cai, L. J. Miara, Y. Wang and G. Ceder, Science, 2022, 378, 1320–1324 CrossRef CAS PubMed.
- N. Twu, X. Li, A. Urban, M. Balasubramanian, J. Lee, L. Liu and G. Ceder, Nano Lett., 2015, 15, 596–602 CrossRef CAS PubMed.
- N. Kuganathan, A. Kordatos, N. Kelaidis and A. Chroneos, Sci. Rep., 2019, 9, 2192 CrossRef PubMed.
- P. M. Panchmatia, A. R. Armstrong, P. G. Bruce and M. S. Islam, Phys. Chem. Chem. Phys., 2014, 16, 21114–21118 RSC.
|
This journal is © The Royal Society of Chemistry 2024 |