DOI:
10.1039/D3SD00144J
(Critical Review)
Sens. Diagn., 2024,
3, 9-27
Recent progress in digital immunoassay: how to achieve ultrasensitive, multiplex and clinical accessible detection?
Received
10th June 2023
, Accepted 15th November 2023
First published on 21st November 2023
Abstract
The detection of low-abundance proteins in physiological samples is of high importance in clinical diagnostics and pathology research. However, protein biomarkers that can be accurately quantified by the existing detection methods and applied to clinical practice are just the tip of the iceberg. Quite a few proteins with concentrations below pg mL−1, which are closely related to the occurrence and progression of diseases, are not effectively utilized due to the limitations of detection technology. In addition, simultaneously measuring multiple proteins in a single reaction improves the diagnostic accuracy and reliability since most diseases will cause abnormal changes in multiple biomarkers. Therefore, increasing demands to realize ultrasensitive and multiplexed protein detection have promoted the rapid development of digital immunoassay recently. Furthermore, the high-end digital detection is also expected to spark its potential for streamlined and integrated workflows in clinical applications, extending beyond its use in fundamental research within central laboratories. In this review, we focus on elucidating the core strategies and approaches to enhance the performance of digital immunoassay across three key dimensions: sensitivity, multiplexing, and clinical accessibility. Ultimately, we highlight the promising prospects of digital protein detection in the next decade.
1. Introduction
The ultrasensitive and accurate detection of proteins of interest that are related to disease occurrence and progression is of paramount importance throughout the entire cycle of diagnosis and treatment of diseases, such as the diagnosis of diseases at an earlier stage where patients have a better survival rate and faster recovery, prediction of the progression of diseases, and matching specific therapy for patients through precision medicine. At present, the detection sensitivity of the mainstream clinical detection technology is generally pg mL−1–ng mL−1; nevertheless, increasing studies have found that numerous biomarkers at sub pg mL−1 levels in body fluids, especially peripheral blood, are of great clinical value.1–3 For instance, the detection of plasma biomarkers of Alzheimer's disease paves the way for low-cost, non-invasive and low-damage screening of this disease.4 The monitoring of cytokines could detect the early onset of acute inflammation and diseases of the immune system.5,6 Besides, the circulating tumor DNA (ctDNA) in plasma helps detect the primary and recurrent cancer, which has been considered as the most promising biomarker of liquid biopsy for clinical applications.7,8 Due to the disadvantage of insufficient detection sensitivity caused by the ultralow abundance of biomarkers, scarce and inaccessible biological fluids, and the interference of matrix proteins with high concentrations, a large number of potential protein biomarkers with a concentration far below the detection limits of the current laboratory methods have not been well applied so far.9
The demand for detection with ultrahigh sensitivity gave birth to the concept of digital detection and boosted a lot of digital detection technologies, including digital polymerase chain reaction (dPCR),10 nanostring,11,12 beads, emulsions, amplification and magnetics (BEAMing).13,14 The common feature of these technologies is that the signal of a single target molecule can be directly read out through partitioning a single molecule into an isolation chamber or capturing a single target molecule by microspheres. However, the vast majority of digital approaches aim for the detection of nucleic acid molecules. In 2010, Walt group15 pioneered the digital enzyme-linked immunosorbent assay (dELISA) technology for protein ultrasensitive detection via loading the microsphere into the fL-volume microwells. In a precise sequence of steps, initially, microspheres coupled with capture antibodies were incubated with the target antigen and detection antibodies. Subsequently, these microspheres were linked with enzymes and introduced into microwells. This orchestrated process catalysed the substrate, leading to the generation of a discernible fluorescence signal. Only wells containing beads with the single target molecule could emit fluorescence signals, while others with blank microspheres that did not capture the target molecule fail to be lit up, which was regarded as “1” or “0” digital signal respectively. Since the average number of molecules captured on each bead could be controlled to one at most by adjusting the number ratio of target molecules to the beads, the number of target molecules could be converted to the number of “1” signal, which could subsequently be extracted from the microscopic image. Fig. 1 shows the comparison between the conventional ELISA assay (analog mode) and digital ELISA. In digital ELISA, ultrasmall chambers concentrate the reaction and lead to an extremely high localized concentration of fluorescent products, resulting in a high signal-to-background ratio to achieve typically around 10−18 M (fg mL−1) amazing high sensitivity, which improved by approximately 1000 times higher than the analog detection. Since digital ELISA is superior for implementation in the area of ultrasensitive protein biomolecule detection, it has been widely used as gold standard in the diagnostic assessment of viral antigens,16–18 inflammatory biomarkers,19,20 neurological biomarkers21–23 and enzymatic activity.24,25 What is more exciting is that the single molecular array (Simoa) technology based on the principle and technology of Walt group has been successfully commercialized and applied to discover potential biomarkers26 and monitor the course of disease.20
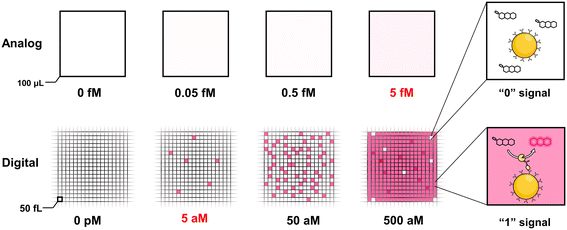 |
| Fig. 1 Difference between the conventional (analog) enzyme-linked immunosorbent assay (ELISA) and digital ELISA. AEB represents the mean number of enzyme molecules per bead at the given concentration of sample. The red number represents the lowest detectable concentration of target protein in analog and digital methods. | |
To break through the limitation of chamber number bringing from physical isolation, other digital immunoassay platforms such as droplet digital ELISA (ddELISA),27,28 single-molecular counting (SMC) technology,29–31 and partition-free digital detection32–34 were exploited to further improve the measurement capacity. Apart from the traditional label modes, numerous inspiring signal-generation approaches have been explored based on either non-fluorescence signal modes including plasmonic signals,35–37 electrical signals,38,39 and the label motion states,40–43 or fluorescence modes including upconversion nanoparticles44–46 and quantum dots.47–49 These works will be described in detail in the main text.
Thus far, it is no doubt that digital bioassay has become a prominent way to realize ultrasensitive detection. Most importantly, after the first generation of dELISA has basically realized the function of high-sensitivity detection (generally, the limit detection has been as low as fg mL−1), new requirements have been put forward to the digital biological detection technology overwhelmingly. For example, on the premise of manufacturing resources as fewer as possible, how should the detection sensitivity be further improved? How should multiplexed and high-throughput detection be achieved in a single reaction to further improve the detection efficiency? How to make digital protein analysis common and affordable for clinical practice applications? These are the main challenges of digital protein detection in the next decade. Collectively, focusing on sensitivity, multiplexity and accessibility for clinical use as Fig. 2 concludes, this paper reviews the insights and schemes of digital immunoassay performance in the recent five years.
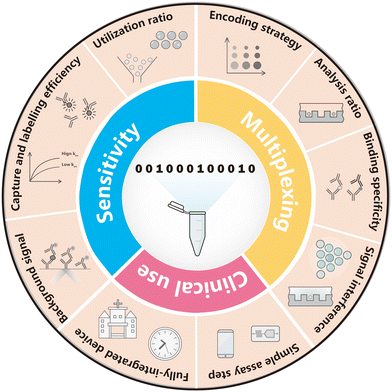 |
| Fig. 2 Schematic summary of the key points of digital immunoassay. | |
2. Sensitivity
Digital immunoassay makes significant improvement in detection sensitivity, which owes to the principle of Poisson distribution. In digital immunoassay, “n” molecules react simultaneously in “m” compartments, and “p” is the probability of “k” molecule in one compartment. “λ” is defined as the average molecule per compartment, and the probability of “k” molecule in one compartment is denoted as X ∼ P(n,λ):
“λ” decreases when the ratio of compartments to molecules increases. When “λ” decreases from 1 to 0.01, P(X = 0) increases from 36.8% to 99.0% theoretically, while P(X = 1) declines from 36.8% to 0.99%. When P(X = 0) is close to 99.0%, only 0 or 1 molecule is entrapped in one compartment, forming the binary signal. Under this circumstance, “1” compartment represents one target molecule that generates a readable signal, while “0” signal represents that there is no target molecule in the compartment. In this case,
P(X = 0) = 1 − P(X = 1) = 1 − fon = e−λ |
“λ” is calculated by the fraction of positive compartments:
λ = −ln P(X = 0) = −ln(1 − fon) |
where fon denotes the fraction of effective compartments that can generate “1” signals.
In general, since the proportional relationship based on Poisson distribution between “λ” and low concentration of target molecule exists in a digital bio-detection system, the absolute concentrations of the target molecules could be accurately calculated without standard curve. Nevertheless, it is worth noting that different from dPCR, dELISA technology exists capture efficiency (such as solid-phase immuno-reaction based on microspheres) or reaction efficiency (such as the recognition of antibodies and antigens), so the digital immunoassay only reflects digital reading on signal retrieve without absolute calculation of target protein in a real sense. Consequently, after digital signal reading, it is still necessary to establish a standard curve to reveal a correlation between the digital signal and the target concentration.
Based on this principle, the digital bioassay is very suitable for measuring low-abundance analytes, and increasing digital detection methods tend to pursuit higher sensitivity to address unresolved clinical pain points. Thus, we organize this section to introduce these ingenious strategies on how to improve the sensitivity of digital immunoassay.
2.1 Increasing analysis efficiency of detection system
2.1.1 Increasing the bead utilization ratio of bead-based digital ELISA.
In the bead-based immunoassay, the capture antibody-modified superparamagnetic microbeads as carriers bind to the molecule of interest, detection antibodies and enzyme labels in sequence. Then the immunocomplexes are isolated into small-size partitions with the fluorescent substrate added, following concentrated fluorescence signals detected by microscopy. Simoa (Fig. 3(A)) and ddELISA (Fig. 3(B)) are two typical methods that microwells and droplets are partitions to physically entrap the single molecule signal. In the digital range, to ensure each bead captures solely one immunocomplex or zero, “λ” should be less than 1, and in this circumstance, the ratio of the resulting protein molecules to beads is typically smaller than 1/10 to follow a Poisson distribution.15 The dynamic range could also be expanded to more than 4
logs by combining the average signal intensity in the analog range, where a single bead carries more than one immunocomplex.
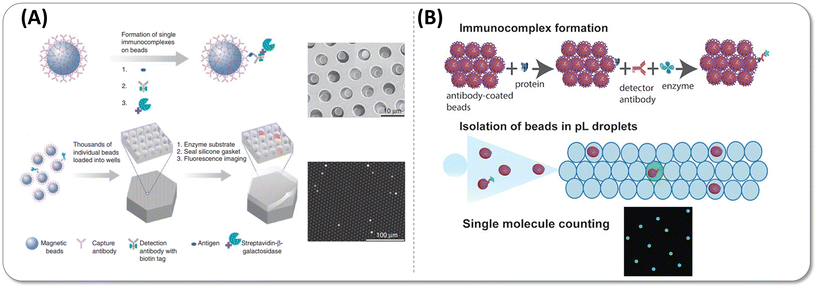 |
| Fig. 3 (A) Digital ELISA based on arrays of femtoliter-sized wells. Reprinted with permission from ref. 15. Copyright 2010 Nature Publishing Group. (B) Single-molecule protein detection using droplet-based digital ELISA. Reprinted with permission from ref. 28. Copyright 2020 American Chemical Society. | |
Increasing the total number of beads analysed, namely, bead utilization ratio, is an effective way to improve the assay sensitivity. The bead utilization ratio refers to the ratio of number of beads analysed to the total number of beads participating in the immune reaction, which is affected by the bead loading mode, loading efficiency, and the beads loss rate during the multi-step reaction process. The number of sampling beads determines the Poisson noise according to the Poisson distribution. Here, Poisson noise
implies the statistical uncertainty caused by insufficient positive signal count, where N represents the number of counted molecules. In practice, Poisson noise is considered to be less than 10% to remain statistically significant. The more beads counted, the lower Poisson error is, so the lower concentrations of molecules could be accurately detected rather than drowned out by Poisson noise. For one thing, when the bead number is fixed, the sample with ultralow concentration (10−15–10−18 M) leads to rare positive beads with increasing Poisson noise, so reducing the Poisson noise during analysis through increasing bead utilization ratio is the key point in the ultrasensitive detection. For another, in digital ELISA, the fewer beads involved in the reaction accounts for the higher average number of enzymes per bead (AEB) under the same target concentration, leading to improved slope of the standard curve, suggesting that the detection sensitivity can be improved if the detection precision remains unchanged.28 Briefly, if the sensitivity is improved by introducing low bead number approach stably and reliably, it is necessary to ensure that as many beads as possible participate in the immune reaction, which are counted and analysed, that is, to improve the utilization ratio of microbeads. Here are two pivotal strategies:
2.1.1.1 Improvement of the loading efficiency to boost the utilization ratio.
Loading efficiency, one of the important factors related to utilization ratio, refers to the number of beads trapped into the wells versus the total number of wells. Since one immunocomplex is isolated in a single partition, the loading efficiency of individual bead-based immunocomplex is limited less than 10% as a result of Poisson distribution during the process of bead encapsulation. To reduce Poisson noise, the most direct way is to increase the number of partitions. Kim et al.50 increased the number of femtoliter partitions to one million to observe more beads and reduce the coefficient of variance (CV) of the background assay determined by the Poisson noise. The sensitivity of prostate specific antigen (PSA) improved by 20 folds higher than the previous dELISA platform with 50
000 wells,51 reaching 2 aM. While this approach undoubtedly enhances detection sensitivity, it increases the fabrication costs and requires higher resolution and larger field-of view of microscopic imaging equipment, which is not amenable for practical use. Due to the convenient and rapid manufacture of plenty of microchambers, droplet microfluidic provides a new way to increase the bead utilization ratio in digital ELISA by encapsulating more beads in the droplets. The generation of droplet through shearing water phase by oil phase leads to high bead utilization. In flow-focusing geometry, the small orifice has the shear-focusing function and thus contributes to uniform droplet generation and low bead loss.52 However, fL droplet generation requires precise manufacturing and dimensional stability of the microchannels, and the narrow channel is likely blocked by microbeads,27 so it gradually fades away from the digital protein detection. The droplets with pL or nL volume (the diameter is about tens to hundreds of microns) could be easily prepared and stably stored in simple chamber. Under the 60% utilization ratio of the beads, the limit of detection (LoD) of IFNγ in ddELISA was ten times lower than Simoa.28 Unfortunately, the Poisson distribution limited the encapsulation efficiency of droplets to beads, resulting in only 10% bead-containing droplets in most ddELISA platforms. Encouragingly, Yue et al.53 proposed a novel bead ordered arrangement droplet (BOAD) system to break through the Poisson distribution and increase the single-bead encapsulation ratio to 86%. Utilizing the principles of Dean flow, hydrodynamic focusing effects, inertial forces, and steric crowding, the BOAD system pre-focused and arranged the beads at set intervals within a channel less than 1 cm in length before droplet generation, which decreased the channel pressure and the manufacturing cost. At a sheath flow ratio of 5, the bead utilization rate (the number of beads in single encapsulation droplets versus the total number of beads) reached 92%. This design has great potential to be applied in ultrahigh multiplexed digital protein detection in the future.
Consequently, the most crucial way to improve the sensitivity is increasing the bead utilization ratio with a high bead loading or encapsulation efficiency. The process of entrapping beads into the partitions (no matter microarray or micro-droplet) greatly affects the bead utilization ratio, with over 60% of beads potentially getting lost.54 The conventional magnetic-mediated encapsulation approach gives rise to only 10% bead loading efficiency in the commercialized digital detection – Simoa, yielding the limitation of accurate measurement in low bead number due to the high Poisson noise. As the loading efficiency increased, the lower AEB could be attained precisely because it can benefit from the reduction of Poisson noise (Fig. 4). In order to increase the number of beads in well, Decrop et al. and Witters et al. explored hydrophilic-in-hydrophobic microwell arrays and electrowetting-on-dielectric-based microfluidic chips to achieve 96% and 98% loading efficiencies respectively, and the sensitivity of β-galactosidase reached 17.4 aM and 10 aM respectively,15,55,56 which was far more sensitive than the LoD of a similar molecular weight target with 10% loading efficiency.24 Besides, Kan et al. designed a magnetic-meniscus sweeping (MMS) approach, which combined the magnetic forces at the bottom of the array with the capillary forces at the receding meniscus of the flow to push as many beads as possible into microwells, achieving a bead loading efficiency of ∼61% and decreasing the bead loss by 34% compared with traditional digital ELISA. It is even more exciting – by employing a low-bead strategy, LoD for IL-17A has been reduced to 0.7 aM, which was an astonishing 437-fold increase in sensitivity compared to standard digital ELISA54 Through the structural design of the sliding chip and the optimization of the sample loading process, Lyu et al.57 invented a sliding microwell array with 70% loading efficiency. With the help of a 2-step sample loading strategy, the duplex quantification of IL-6 and IL-10 showed LoD values of 5.2 and 15.3 fg mL−1, which was around 2–3 times improved compared to a commercial Simoa platform.
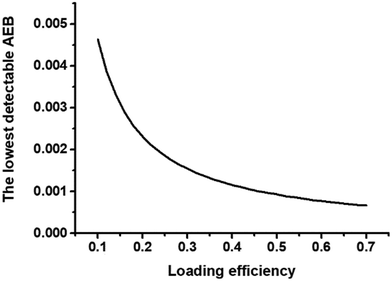 |
| Fig. 4 Theoretical illustration of the relationship of loading efficiency and the lowest detectable AEB in 216 000 wells, the same well number as Simoa disc. | |
2.1.1.2 Reducing bead loss to analyse more beads.
Reducing the loss of beads throughout the whole assay process is another way to improve the bead utilization ratio. As for Simoa, the bead loss could be reduced by optimizing the reaction and detection steps. In conducting reaction steps, bead loss mainly originates from 11 times washing steps. Kan et al. reduced bead loss from 12.1% to zero by a centrifugation washing process. Only less than 1 μL residual volume was left in each washing step.54 However, in the process of detection process, the inherent flaw in microscopic imaging, such as the field of view, the crop and mask processing of the original image, made a large excess of beads fail to be recorded, thus objectively causing a number of beads go undetectable.
To address the loss of beads in loading and imaging, changing the detection mode from physical isolation to non-isolation (partition-free or micro-chamber free) can achieve a significant breakthrough in sensitivity. In traditional digital ELISA, partitions are necessarily used to generate and concentrate the digital detection signal produced from the substrate that is catalysed by enzyme-labeled immunocomplex. As long as the signal generated from labels is anchored on the beads without interference or diffusion and strong enough to be observed, no chamber was needed and the flowing beads could become the individual partitions to liberate the analysis from the space constraints. In the case, all beads involved in immune-reactions could be read out by cytometry and the utilization ratio almost becomes 100% if the loss of beads in the immunoassay is not considered. The dramatical enhancement in the bead utilization ratio demonstrates a significant increase in sensitivity. In 2016, Suzuki's group32 introduced the tyramide signal amplification (TSA) system to realize the compartment-free detection mode with attomolar level sensitivity. Different from the typical horseradish peroxidase (HRP) substrate that display color change, they chose biotinylated tyramide which transformed as a tyramide radical under catalysis to form the covalent bond with aromatic compounds on the surface of a protein. As the fast formation rate of the covalent bond, the deposition of a large number of tyramide radicals was confined to a small area, and then streptavidin-labelled Cy5 was linked to the biotin label on the tyramide radical for visualization. In 2020, Wu et al.26 developed a rolling circle amplification (RCA) strategy to enlarge the single molecule signal on beads in situ and realize digital signal read out with a microscopic image. After the sandwich structure immunocomplex was formed, a streptavidin-labelled circular DNA template combined with the biotinylated detection antibody. In the presence of phi29 DNA polymerase, the primer extended along the ring and produced single-stranded DNA products, following the complementary fluorescently labelled DNA probes hybridized. Thus, the signal of on-beads was localized and enlarged by the nucleic acid amplification. Under a high bead utilization ratio, they used 5-fold fewer beads to measure detectable levels of Brachyury in more chordoma patient samples, which was difficult to achieve in the Simoa system. At the same year, they simultaneously detected eight biomarkers using a flow cytometer with attomolar sensitivity via a low bead strategy.33 In 2021, Zhang et al.58 applied the self-confined principle of T4 polynucleotide kinase phosphatase (T4 PNKP) on single beads to detect T4 PNKP with the concentrations as low as 1.28 × 10−10 U μL−1. This innovative mechanism sparked a new idea to regard T4 PNKP as the enzyme label to fabricate digital sensing systems. In 2022, Chen et al.59 designed a cascade tyramide signal amplification approach to efficiently deposit fluorescence in the bead that bound with target. The LoD for PSA was achieved at 97.2 aM, along with an exceptionally wide dynamic range spanning up to five orders of magnitude.
2.1.2 Increasing the label signal analysis ratio of other digital immunoassay methods.
When the positive events are too rare in digital range, low readout rate and finite readout time prevent analysing total positive events under the real test condition. Only a fraction of these events can be analysed within a given timeframe. Most seriously, the deposition area of positive signal on beads is possible to be hidden on the back of the laser, making them difficult to be fully detected by flow cytometry (FCM) or microscopy.32 Considering that the positive events in traditional digital ELISA are extremely rare and prone to be drowned out by Poisson noise, other digital bioassays emerged to selectively collect nearly 100% label signal rather than stochastically count a small portion of the target signal, which reduced the uncertainty in data statistics.
2.1.2.1 Single-molecule counting (SMC).
The core idea of SMC technology is directly scanning labels on detection antibodies to obtain as many positive events as possible in a shorter time. In capillary flow-based single-molecule detection on plate first proposed by Wu et al.60 in 2006, the fluorescent dye-labelled detection antibodies were eluted and passed one by one through the small detection window (Fig. 5(A)). Under the optical limit scale, the energy of excitation laser in the detection window was improved as much as possible to generate explosive growth in the photons, ensuring that the single molecule signal was adequately identified and distinguished from the background noise. Afterwards, the supermagnetic bead as another target carrier with low non-specific binding (NSB) and high capture efficiency was introduced to make the sensitivity 10 times higher than the plate assay. SMC technology with a LoD value of 10–100 fg mL−1 under 1–2 h incubation has been commercialized and applied in disease diagnosis61,62 and prognosis evaluation.63,64 However, since the non-parallel readout mode restricted assay throughput, the total detection of a 384-well plate lasted for 8 hours, which was obviously too long for clinical practice.29
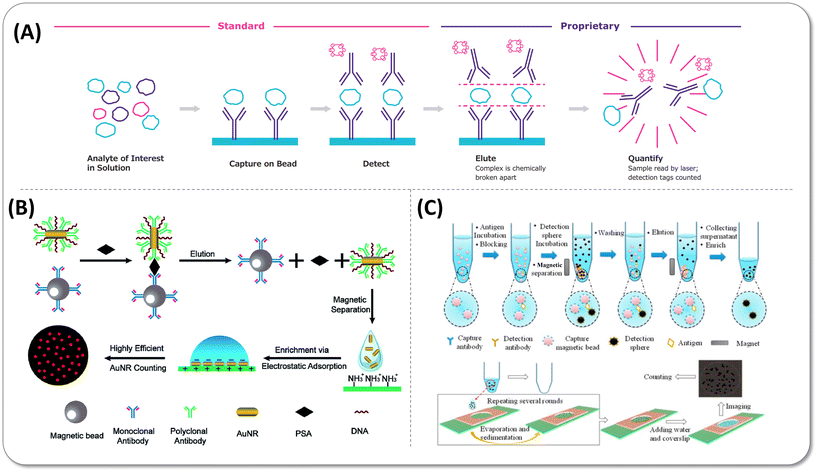 |
| Fig. 5 (A) Single-molecule counting (SMC™) technology. Copyright 2022 Co. Merck KGaA, Darmstadt, Germany (https://www.sigmaaldrich.com). (B) Schematic of elution and enrichment of Au nanorods (AuNRs) label to detect PSA. Reprinted with permission from ref. 65. Copyright 2018 the Royal Society of Chemistry (C) Scheme of elution and concentration detection spheres to count protein number. Reprinted with permission from ref. 66. Copyright 2016 American Chemical Society. | |
2.1.2.2 Elution and enrichment of single-particle-based signal labels.
Apart from fluorescent dye molecule conjugates, nanoparticles have also been explored as labels due to their relatively large size and stable fluorescence property, which is suitable for microscopy imaging. The strategy of eluting and enriching nanoparticle labels saved analysis resources and time by reading the single-particle fluorescence in a small field of view. As early as 2017, Zhu et al.65 eluted Au nanorods (AuNRs) with the strong scattering intensity from immunocomplexes and enriched more than 90% of them on the slide by electrostatic force for the dark-field microscopic image, as shown in Fig. 5(B). The single-molecule detection of PSA was realized in the low concentration range of 10 aM to 100 fM. However, this approach failed in counting all AuNRs that bound with targets primarily due to two reasons. First, AuNRs cannot be 100% eluted. Second, it is difficult to accurately count the number of AuNRs when they aggregate due to electrostatic attraction. Later, Zhang et al.66 employed 3 μm detection antibody-coated polystyrene beads (DPS) to solve the signal overlap originating from aggregation under simple bright field observation, following the three-time evaporation-induced sedimentation to immobilize the DSP on a limited area (Fig. 5(C)). When the positive signals accounted for 0.5‰ with 600
000 capture beads in the assay, the non-eluted DPS was only 20–30 after three-round elution, which could be negligible in the detection range. The successful counting of nearly all target DPS reduced Poisson noise, so the LoD of the carcinoembryonic antigen (CEA) reached 4.9 aM in buffer and 6.1 aM in 10-fold diluted plasma.
2.2 Enhancing capture and labelling efficiency
Apart from the utilization ratio, it is pivotal for digital bioassays to enhance the capture and labelling efficiencies. Conventionally, the capture efficiency was optimized by selecting the antibody pairs with high affinity. In digital ELISA, the assay performance was particularly determined by the thermodynamic and kinetic features in the interaction of the antibodies and target proteins. The dissociation constant (KD), the ratio of koff to kon, reflects the binding capacity of two species. kon is the binding rate constant, which reflects the binding speed to form the “on” binary state, while koff is the dissociation rate constant related to the dissociation speed. Antibodies with relatively low disassociation constants (KD ∼ 10−11–10−9 M) and high kon (kon > 105 M−1 s−1) are expected to exhibit optimal performance with a higher capture efficiency.67 According to the kinetic profile of protein capture predicted by the Langmuir absorption model, kon of the binding reaction greatly affects the rate of protein capture.67 For example, when kon is 106 M−1 s−1, if KD decreased from 1 nM down to 10 pM, protein capture rate slightly increased from 67% to 89% after 1000 s incubation, regardless of the reactant concentration. However, when kon dropped from 105 M−1 s−1 to 104 M−1 s−1, the capture efficiency declined dramatically from 20% to 2.3% respectively. Furthermore, some works described that the VHH antibody68 and aptamer-modified functional moieties69 with low koff could enhance the analytical sensitivity. Besides, other approaches demonstrated the remarkable elevation of signal-to-noise ratio by means of adjusting the antibody biotinylating state,70 optimizing bead number,33 and poly-enzyme structure design.71
2.3 Decreasing background signal
In the traditional digital detection process, the interference from self-fluorescence background and scattering light increases the difficulty of distinguishing positive from negative signals. Furthermore, the non-specific binding could not be removed totally by several washing steps, which suppresses the detection sensitivity. In the past few years, some new labels and detection strategies have emerged to solve the background signal interference problem.
2.3.1 Diminishing background interference caused by fluorescence.
To avoid the photo bleaching in the readout process of dELISA, a surface-enhanced Raman (SER) probe as an ultrasensitive label with high photo stability was explored in digital assay. Based on the SERS characteristics of noble metal nanoparticles, the Raman scattering signal of molecules adsorbed on the substrate of SERS probes can be enhanced by 105–106 times.72,73 Li et al.74 functionalized capture antibodies on the uniform gold nanopillars to bind the proteins of interest and gold–silver alloy SERS nanotags modified with detection antibodies, as shown in Fig. 6(A). Under the SERS mapping, each nanopillar with one or more nanotags was regarded as a single positive event since the targets on the pillars followed Poisson distribution with the ratio of cytokine molecules to pillar number less than 1/10. The digital SERS platform exhibited the same sensitivity as dELISA with 5.2 aM detectable cytokine concentration in human serum,74 and achieved high specificity due to the strong single-particle SERS signal and less false-positive signals by confocal Raman mapping that only focused on the signal from gold nanopillars.
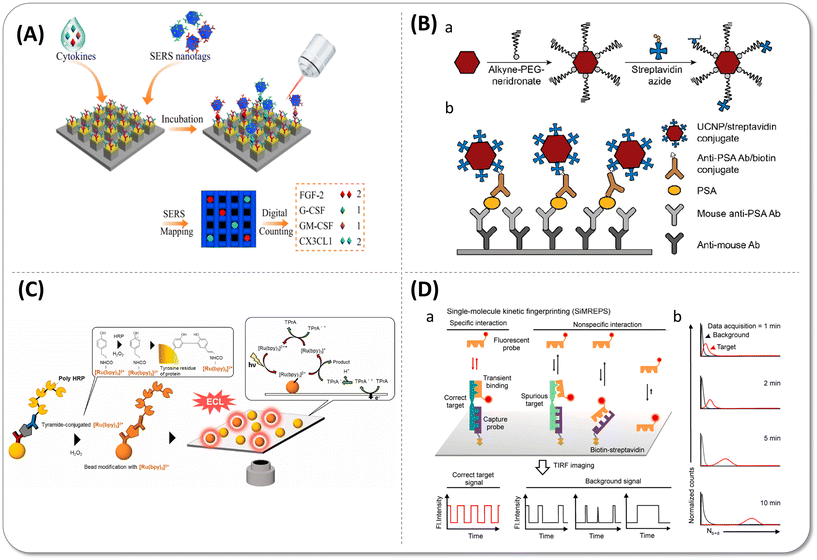 |
| Fig. 6 (A) Single-molecule nanopillar surface-enhanced Raman scattering (SERS) platform for simultaneous detection of four types of cytokines. Reprinted with permission from ref. 74. Copyright 2021 Springer Nature. (B) Schematic representation of upconversion nanoparticle (UCNP) conjugation and a single-molecule upconversion-linked immunosorbent assay (ULISA). (a) Alkyne-polyethylene glycol (PEG)-neridronate coordinates via two phosphonate groups of neridronate to lanthanide ions exposed on the surface of UCNPs. Then streptavidin azide covalently binds to the alkyne group of PEG by a click reaction. (b) Steps of the sandwich ULISA. Reprinted with permission from ref. 45. Copyright 2019 American Chemical Society. (C) Schematic illustration of droplet-free digital ELISA based on electrochemiluminescence (ECL). After the modification reaction of the bead with [Ru(bpy)3]2+-conjugated tyramide using a tyramide signal-amplification system, the beads were dispersed on an indium tin oxide (ITO) electrode for ECL in the presence of the co-reactant tri-n-propylamine (TPrA). Reprinted with permission from ref. 75. Copyright 2023 The authors. Published by Elsevier B.V. (D) Schematic of the strategy of single-molecule kinetic fingerprinting (SiMREPS). (a) Due to distinguishable binding kinetics features as fingerprint, the dynamic binding of low-affinity fluorescent probes (detection antibody or fragment) could differentiate between specific and nonspecific binding by analysis of the fluctuations of localized fluorescent spots over time at single-molecule level. (b) Predicted distribution of the number of binding and dissociation events as a function of time. Reprinted with permission from ref. 76. Copyright 2020 American Chemical Society. | |
The unique anti-Stokes emission property allows upconversion nanoparticles (UCNPs) to be observed easily by the general upconversion microscopy without autofluorescence background interference. In the early stage, Farka et al.44 directly immobilized detection antibodies on the surface of UCNPs to form a sandwich structure with targets and the capture antibodies that were conjugated on the 96-well microtiter plate. Unfortunately, the LoD of PSA was only 1.2 pg mL−1 in blood serum due to the weak capture ability of UCNPs and relatively strong non-specific adsorption although the UCNP labelling system indeed reduced the spontaneous fluorescence interference of the sample itself. Subsequently, this group modified polyethylene glycol (PEG) hydrophilic long chains on the UCNPs to increase the dispersity and reduce the non-specific adsorption (Fig. 6(B)). They also replaced antibody-modified UCNPs by streptavidin (SA)-coated UCNPs with the biotinylated detection antibody binding before. High affinity of biotin-SA and flexible PEG chains promoted the capture efficiency, so further decreased the limit of detection of PSA to 23 fg mL−1.45
Since chemiluminescence does not require an excitation light source, it can greatly reduce the background fluorescence produced by laser irradiation. As shown in Fig. 6(C), Ito et al.75 created chamber-free digital immunoassay based on electrochemiluminescence (ECL). They employed [Ru(bpy)3]2+-conjugated tyramine as the substrate of poly-HRP to facilitate the electric signal amplification of ECL beads, which captured antigens with poly-HRP labelled on the indium tin oxide (ITO) electrode. However, as two poly-HRP molecules on the bead were necessary to activate ECL, the LoD value for goat IgG was only 0.7 pg mL−1.
2.3.2 Distinguishing between specific and non-specific binding.
In single-molecule detection, non-specific binding greatly influences the sensitivity by producing a false-positive signal. Based on the immune response kinetics, Chatterjee et al. established a method called single-molecule recognition through equilibrium Poisson sampling (SiMREPS) to remove the non-specific adsorption from the positive events. Instead of physical isolation as described above, this digital biosensing applied simple glass substrates for immunoreaction and subsequent single-molecule imaging. Owing to significant differences in the affinity of specific binding and non-specific binding, as well as the high resolution of the single-molecule image of total internal reflection microscopy (TIRF), SiMREPS can distinguish false positives from specific binding by using detection antibodies with low koff and exhibit the excellent femtomolar-to-attomolar LoD values (Fig. 6(D)).43,76
3. Multiplexing
From the perspective of diseases, their occurrence and progression accompany the regulation of multiple biomarkers, and hence, the measurement of multi-biomarkers tends to provide more accurate and convincing judgment for disease diagnosis, especially in the early stage of the diseases. Nevertheless, to obtain the concentration of the multiple target molecules of interest, the samples are usually distributed in different reaction tubes and measured one by one, in which only one kind of target was measured in each tube. Under this circumstance, the absolute number of proteins with low abundance decreases, possibly to just a few molecules in one tube. The heterogeneity of molecular distribution per tube increases significantly when the target molecules are rare, exacerbating the difficulty of detecting low-abundance target molecules. Otherwise, the more biomarkers are detected, the larger sample volume is needed, which is not friendly and realistic for patients. Hence, multiplex detection in single tubes that improves detection efficiency with less sample volume and shorter assay time is of great significance in the detection of protein biomarkers with exceptionally low concentrations, paving the promising way for high-throughput disease screening and course of disease monitoring.
3.1 Encoding strategy of multiplex digital immunoassay
3.1.1 Fluorescence barcode strategies.
With little spectral overlap, discrete fluorescence intensity and excellent block process to avoid leakage of fluorescent dyes, superparamagnetic microbeads as coding carriers have been widely used in the multiplexed immunoassay to identify, manipulate, and capture individual target molecules. Microbeads doped with fluorescent dyes or quantum dots (QDs) can be assigned numerous codes by adjusting the fluorescence intensities within the detector's acceptable range. For example, combining the surface topology or internal microstructure of microbeads with fluorescent property, Xu's group has developed several unique encoding strategies, and achieved up to 300 encoding level with FCM as the decoding platform, which supplied sufficient encoding capacity for high-throughput multiplexed detection, as illustrated in Fig. 7(A)–(C).77–79
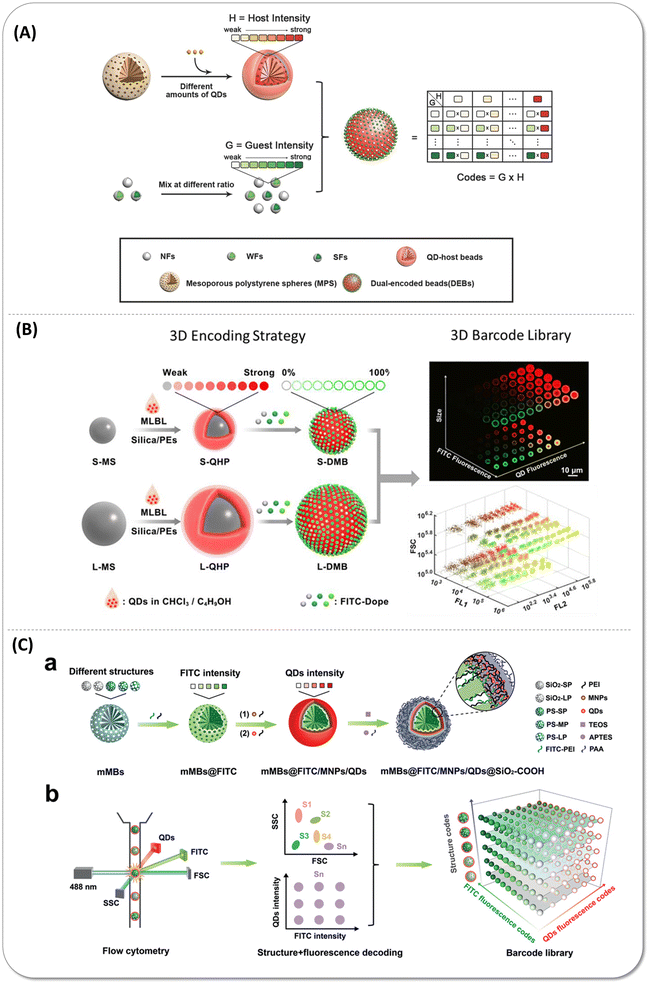 |
| Fig. 7 (A) Schematic illustration of the multiplex encoding strategy of dual-encoded beads (DEBs) through a host–guest structure that fluorescein isothiocyanate isomer I (FITC)-doped nanoparticles (guest) packed onto the quantum dot (QD)-encoded polystyrene microspheres (host). Reproduced with permission from ref. 77. Copyright 2016 American Chemical Society. (B) Schematic showing the three dimensions encoding strategy combined size, fluorescence emission wavelength, and intensity. FITC-doped guest beads immobilized on the small-sized and large-sized QD-encoded host bead surface. Reproduced with permission from ref. 78. Copyright 2017 American Chemical Society. (C) Scheme of the structure-fluorescence combinational encoding strategy. a) Construction process of encoded beads. In various kinds of mesoporous microbeads, FITC encapsulated within mesopores and magnetic nanoparticles as well as QDs immobilized on the outer surface. b) Structure-fluorescence combinational encoding strategy achieved the ultrahigh encoding level of 300. Reproduced with permission from ref. 79. Copyright 2022 Wiley-VCH GmbH. | |
3.1.2 Spatial encoding methods.
Apart from the fluorescence encoding mode, the location-dependent multiplexing was applied to expand multiplicity. Typically, the spatial encoding principle can be realized in two ways: one is to immobilize the capture antibody array on the surface of microwells in different regions, while the other is to conjugate different antibodies onto the surface of barcodes with different fluorescence information and then isolate barcodes into different micro-chambers (Fig. 8). Song et al.80 loaded different antibody-conjugated beads in lateral microarrays and incubated the sample in microarray chips. The lateral array number and bead color allowed the simultaneous detection of 12-plex interleukins in total. Stephens et al.81 printed six different types of capture antibodies on the different zones of the microwell surface to simultaneously measure six serum secretory factors related to glioma tumor progression in a cohort of mice. Since this digital protein microarray method eliminates the use of microbeads from the assay, there is no need to spend an extended amount of time on taking sufficient images of each kind of beads corresponding to each analyte. However, the whole reaction time increased with the multiplicity owing to the poor reaction kinetics of capture antibody on a planar substrate and restricted microarray area.
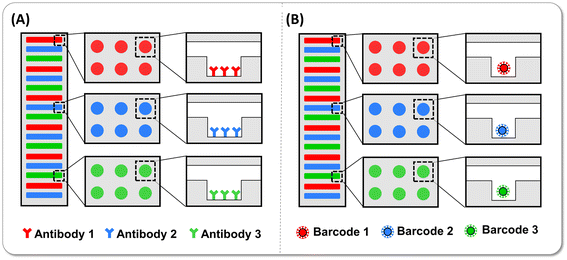 |
| Fig. 8 Scheme of two typical spatial encoding strategies. (A) Print different capture antibodies on the surface of microwell array at different areas. (B) Load various kinds of capture antibody-coupled microspheres into the microwell array at specific location. | |
3.1.3 Other encoding strategies.
Apart from label encoding and space encoding, some new encoding strategies have gained researchers' interest. SERS technology is a good example due to high photo stability and narrow Raman linewidth (∼1–2 nm) in comparison to fluorescence (∼50 nm),82 which breaks through the barrier to coding capacity. Under the stable and universal synthesis, SERS nanotags have great potential to expand the digital multiplex detection ability. Liu et al.83 employed a plasmonic shell on microbeads to increase the SERS signal and built up 25-plexed Raman barcode library by adjusting silent region Raman-coding intensity levels. After the capture antibody functionalized Raman barcode binding with antigens and detection antibodies modified Au nanoflowers, the reporting SER signal was greatly improved due to synergistic SERS effects from the plasmonic shell and the Au nanoflower nanostructure, enabling ultrasensitive measurement of 5-plexed lung cancer markers in the range of fg mL−1 to pg mL−1.
Besides, thanks to the emergence and advances in artificial intelligence (AI), the information of colors and sizes encoding microspheres in the optical microscope image could be directly decoded by the computer vision-based artificial intelligence.84,85
3.2 Key to the performance of multiplex digital immunoassays
During the multiplex digital immunoassay, capture antibody-conjugated barcodes are mixed together with the samples containing all analytes of interest, and each kind of barcode is responsible for binding each sort of target and following labels. Subsequently, multiple barcodes that captured their respective target molecules are loaded into partitions such as microarrays and droplets, even using the encoded barcodes themselves as partitions to complete the digital detection. Each partition is simply analysed by two excitation lights. The first laser detects the coding information of the barcodes to judge the type of molecules measured, and the second laser counts the positive signal of target molecules to reveal the target number. Finally, the concentration of the molecules could be calculated according to the calibration curve. It is worth noting that the accuracy of the analysis closely influences the sensitivity and linear dynamic range of immunoassay. Since data are considered reliable only when the Poisson noise is less than 10%, at least 10
000 such microspheres should be analysed per target to ensure the accuracy of positive signal analysis.
3.2.1 Improving the analysis ratio of beads corresponding to each analyte.
Under the multiplex detection condition, the number of microspheres multiplies and the capacity of microchambers has to be expanded. However, it is not wise and practical to increase the well number due to more difficult fabrication and higher expense. The substituted solution is increasing the loading efficiency of microarrays, but often rises the difficulty of chip design and manufacture. The droplet microfluidic platforms produce millions of partitions in short time, which are ideal candidates for multiplex detection. Yelleswarapu et al.86 integrated and operated a hundred droplet generators in parallel to produce 10 million droplets in 10 min for fast duplex detection via mobile phone imaging in the sub-fM range. Yi et al.87 introduced poly-HRP into pL microdroplets to achieve 5-plex cytokine ddELISA detection within 3 min in the sub-fM range LoD values. Although high-throughput water-in-oil droplets entrap the beads to endow a high bead utilization ratio, digital droplet microfluid is not flawlessly amenable for ultrahigh-multiplexed detection because the encapsulation of beads still obeys the Poisson distribution, which means only 10% droplets at most wrapped beads. Specifically, when microdroplets are used as isolation units for multiple detection, at least 10
000 microspheres for each target molecule are counted to ensure the detection sensitivity. However, counting enough beads will inevitably result in a large number of empty droplets being counted simultaneously, resulting in a huge waste of analytical resources. The more the types of targets, the greater the empty droplets number is, resulting in the time and resource consumption in the imaging and data analysis process. Additionally, the fluorescence background may increase because of the substrate diffusion during the imaging process. Imaging with lower magnification objectives allows a larger area of picture information to be obtained, which promotes sensitivity due to the increase in result precision at a low antigen concentration. However, the lower numerical aperture (NA) increases the background and the fluorescence dispersion of individual microspheres, making it difficult to decode the barcodes with a weaker fluorescence intensity. Taken together, chamber-free detection methods with the on-bead signal are proposed to achieve ultra-multiplexed digital bio-detection. Localized signal amplification methods eliminate bead loss in the process of bead loading originating from Poisson distribution, thus enhancing the speed and precision of data collection. Zhang et al.34 developed a three-plex micro-chamber free digital bio-detection approach based on the tyramide signal amplification strategy. The sensitivity of three tumour markers—CEA, NSE and free PSA (fPSA) were calculated to be 0.4 fM, 9.2 fM and 135.1 fM respectively, 30–15
000 times improvement compared to the conventional multiplexing detection—suspension chip. Wu et al.88 first attempted 8-plexed chamber-free digital ELISA based on RCA. The flow cytometry signal acquisition improved sampling efficiencies and created the conditions for testing with fewer microspheres, giving rise to higher sensitivity than Simoa, reaching the sub-fM level.
3.2.2 Reducing the cross binding among multiple antibodies.
Multiplex digital protein detection puts forward higher requirements for immunoassay specificity. In multiple protein assays, cross-binding interference usually appears between different antibodies or the antibodies and antigens, leading to a false-positive signal. There are some spatial and temporal separation methods proposed to reduce cross-reactivity. Frampton et al.89 immobilized each capture antibody in the specific area on the plate and applied the PEG embossed pattern containing sample and specific detection antibody, which blocked the contact between one capture antibody and another detection antibody. However, the limited motion of capture antibody on the two-dimensional plane reduces the kinetics of reaction with antigens and confines the binding capacity. As a result, a lot of optimizations are often tried to achieve highly sensitive detection. Gilboa et al.90 sequentially input each plex beads to capture each protein, and separated the beads to incubate with its corresponding detection antibody, which decreased the collision probability between the capture antibody and other detection antibodies to a certain extent. However, the cross-reactivity between antigens and capture antibodies, and non-specific binding between antigens and other detection antibodies were not able to be completely removed. Actually, the non-specific bond between detection antibodies and capture antibodies is more difficult to avoid in the multiplex immunoassay, which raises the background signal and reduces the sensitivity. Owing to the ultrasensitive signal recognition method of digital immunoassay, minor non-specific adsorption will have a significant impact on the detection results. Furthermore, the more the multiplicity is, the higher the non-specific binding signal will appear. Therefore, the non-specific binding should be especially considered and minimized in the process of methodology establishment. Antibody pairs originally used for bulk ELISAs are no longer suitable for digital analysis, and so antibody pair selection becomes an important work in the establishment of multiplex digital protein detection methods. Wu et al.91 matched commercially available antibodies to ten common cytokines and screened out the most suitable antibody pairs with low background and high response for each cytokine in the Simoa system, which benefited for those who are devoted to digital immunoassay.
3.2.3 Diminishing signal interference.
How to avoid the signal interference of adjacent chambers that comes from the continuous propagation of light and the connection of liquid during sample loading is another challenge in multiplex detection.
3.2.3.1 Removing optical crosstalk.
Optical crosstalk refers to the fluorescence scattering from one bead into the six neighbouring chambers when beads are loaded in the chambers at a high density, which is related to the reflection of the objective lens and the background noise of the camera sensor as well. Optical crosstalk results in mis-judgment in “1” signal of the chambers containing beads without enzyme labels. In multiplex detection, there are a mass of target proteins of individual different abundance. The beads of low-concentration targets adjacent to the high AEB beads are more likely to be affected by optical crosstalk. This problem enlarged as detection multiplicity increases because the possibility of beads with a slight signal could be more easily lighted up by the others conjugated with proteins of high concentration at a higher bead loading density.
There are some algorithms proposed to reduce optical crosstalk. Song et al.80,92 collected original images with different sorts of false counting to build the data analysis algorithm based on the convolutional neural network. The false-positive signal could apparently be decreased compared with the traditional global thresholding method. This method has a good effect at a low bead loading efficiency. The background of each plex in the dual-plex measurement was similar to the single-plex detection. However, the effectiveness of the method may not be guaranteed at a high bead loading efficiency. Rissin et al.80,92 established a computational method to correct the signal of each well with beads. The baseline was first established based on the empty wells without beads around them in the nearest six directions, and they determined the scattering weight of each plex positive bead to the empty wells. The AEB of other negative beads was decreased from 0.06 to 0.03 when the protein concentration is 100 pg mL−1 in 4-plex measurement. However, there have been few reports on the optimization of optical crosstalk in ultra-multiplexed detection (such as more than 10-plex) because the bead density on the chip significantly increases under such high multiplicity. Under these conditions, the weight subtraction method is hard to work because the beads without neighbours cannot be found. More effective correction algorithms and optical hardware are urgently needed to automatically eliminate this problem especially under the condition of high bead loading efficiency.
3.2.3.2 Suppressing substrate diffusion.
Substrate diffusion is another primary challenge that occurs during the substrate catalysis by the enzyme-labelled bead before forming independent reaction chambers via oil sealing. In Simoa, the mixture of bead subpopulations and substrate was pumped into a disc and the beads settled by gravity into the wells of the array for 90 s.93 After that, the fluorocarbon oil was pulled over the array, following the sealing of liquid containing beads and substrate in the wells. It is important to point out that the substrate in liquid-phase is connected before oil sealing, so the substrate molecules catalysed by enzyme can diffuse into the adjacent wells through the connected liquid, resulting in false-positive signals generated on the no-enzyme-labelled beads. Even a few substrate molecules would cause a concentrated signal in a fL volume well, which has a great influence on the background. Most importantly, since high bead loading efficiency should be reached in ultrahigh multiplicity detection, the positive signals on the beads of other plex will also be affected, which obviously reduce the accuracy of measurement.
Hence, in order to reduce the pre-catalysis, Simoa identified “on” beads by fluorescence growth through two images at a 30 s time interval. This method could recognize the real “on” beads from the false-positive beads since only the real “on” beads have an increased fluorescence signal. However, in high-multiplex detection with a high bead loading efficiency, the concentration of each target molecule is different. A high concentration of target molecules leads to several immunocomplexes binding on one bead, resulting in faster growth of fluorescence signal under the same enzyme catalytic time, while the signals of target molecules in digital range increase slowly. When the substrate diffuses before oil sealed, the backgrounds of target molecules with a low concentration increase more at the initial moment, causing the more serious overlap of negative and positive signals. In the same time interval, it is also more difficult to separate positive events from negative beads according to the increase in positive signal. Hence, the Simoa disc platform has not been exploited for ultra-multiplex detection.
Furthermore, an elevated solubility of organic molecules in fluorinated liquids due to specific non-covalent interactions94 boosts the diffusion of substrates from positive droplets into adjacent negative droplets. Several strategies were employed to delay cross-talk including increasing the distance of the droplets and adjusting the formulation of surfactants.95–97 However, the physical isolation complicates chip processing, and new reagents have low universality. Thus far, the substrate diffusion has still hindered the ddELISA multiplex detection. Lyu et al.57 created a bead-based SlipChip (bb-SlipChip) microfluidic device to suppress the background signal of digital ELISA by a parallel 2-step sample loading approach. The process of microsphere loading and substrate catalysis was implemented step by step, reducing the pre-reaction of the immunocomplex and the fluorogenic substrate before imaging. With the approximately 10-fold higher bead loading efficiency than that of the commercialized digital immunoassay system, bb-SlipChip laid the foundations for ultra-multiplexed digital protein analysis.
4. Exploration of digital immunoassay in clinical practice
Ultrasensitive digital detection is expected to detect low-abundance biomarkers effectively, which holds great promise to early discovery of diseases. Nevertheless, commercial digital technologies for ultrasensitive detection such as Simoa and SMC require large device footprints, expensive reagents and equipment, complex assay operation and trained professionals, which are more suitable for central laboratories and research institutes. In addition, the overall sample-to-answer time of these platforms are much longer than the current common detection techniques in clinical use, such as chemiluminescence and immunofluorescence. Accordingly, how to apply the highbrow digital ultrasensitive detection technology to clinical practice becomes the latest goal.
4.1 Simple assay step
Typical bead-based immunoassay requires multiple washing steps to remove non-specific binding, which increases the assay complexity and difficulty of the device integration, so several efforts have been made to simplify the assay process by removing washing steps. In 2019, Akama et al.40 designed a digital homogeneous non-enzymatic immunosorbent assay (HoNon-ELISA), where particles as the labels bound with antigen and formed the sandwich structure with the capture antibody that immobilized on the microwell surface (Fig. 9(A)). The characteristic Brownian motion of tethered particles was unlike free diffusion and nonspecific binding of antigen free particles, so the particles with specific motion features were considered as positive signals without multiple washing steps and signal amplification in the assay process. The LoD of PSA in this approach achieved 0.093 pg mL−1. Duplex detection was explored in the next year, but the whole process regretfully demanded more than one hour for PSA and IL-6 detection with 10−2 aM LoD values.41 In 2021, they reduced the distribution of CV in the fluorescence intensity of the beads due to bead size heterogeneity and reduced the false positives by defining the threshold of root-mean-square displacements (RMSD) and aspect ratio. The sensitivity of PSA was increased by 3.9-fold.98 Cunningham's group designed another wash-free method by identifying the activated Au nanoparticles (AuNPs). In this assay, capture antibody-modified AuNPs were “activated” by binding with target antigens, then the mixture was introduced to the microfluidic cartridge by capillary force of absorption pad containing a detection antibody-functionalized photonic crystal (PC). After activated AuNPs bound to detection antibodies on the surface of PC, AuNPs quenched the reflected light of the PC as a result of the surface plasmon resonance (SPR) effect, which could visualize the single AuNP corresponding to single analytes in the PC surface (Fig. 9(B)).Taking good use of this principle, they can measure HIV-1 capsid protein P24 up to 1 pg mL−1 in a 35 minute detection time99 and 27 pg mL−1 human COVID-19 IgG in a serum sample in a 30 minute detection time.100 Although these approaches somewhat simplify the operation and shorten the assay time, they still require a large fluorescence scanning module and a complex data analysis process, which is a barrier for near-bed operation. In addition, the immune-reaction kinetics of planar substrate was insufficient, and hence, the detection sensitivity was limited at pg mL−1 level.101,102
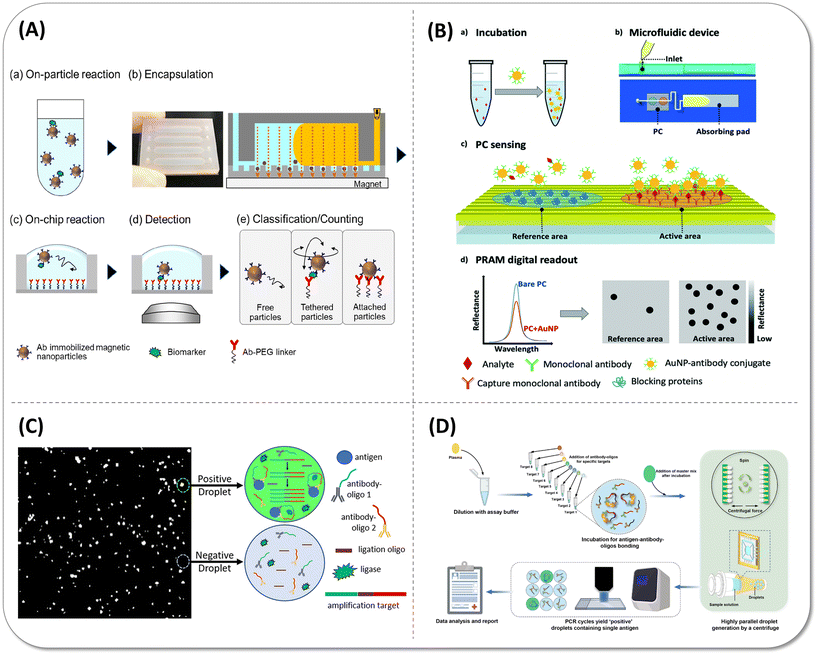 |
| Fig. 9 Wash-free simple digital immunoassay methods. (A) Schematic illustration of digital homogeneous non-enzymatic immunosorbent assay (digital HoNon-ELISA) for protein biomarker detection. (a) Biomarker molecules are captured by antibody-immobilized magnetic nanoparticles. (b) Nanoparticles are magnetically collected and encapsulated into fL-sized reactors, followed by the injection of oil to seal the reactors. (c) Target molecules on the particles react with the antibodies immobilized to the reactor surface through a PEG linker. (d) Bright-field images of the particles were acquired. (e) Particle motion is classified into three patterns, and the tethered particles are counted digitally as target-positive particles. Reproduced with permission from ref. 40. Copyright 2019 American Chemical Society. (B) Workflow of the activate capture and digital counting assay in a microfluidic cartridge. (a) The sample is mixed and incubated with an excess of Au nanoparticle (AuNP)-antibody conjugates. (b) The mixture is introduced into a microfluidic device which comprises a photonic crystal (PC) for sensing and an absorbing pad for pumping. (c) The analyte is sandwiched between the AuNP conjugate and the immobilized capture antibody on the PC surface. (d) High contrast and digital resolution images are obtained by the detection of the reflected intensity drop caused by the local nanoparticle binding. Reproduced with permission from ref. 99. Copyright 2019 The Royal Society of Chemistry. (C) Reaction schematic of the wash-free, single-step polydisperse digital droplet immunoassay (ddIA). After entrapping the antigen, two oligo-conjugated antibodies and reagents for nucleic acid amplification, the polydisperse droplet was subjected to a proximity ligation assay (PLA) and reading was made using a fluorescence microscope. Reproduced with permission from ref. 103. Copyright 2020 American Chemical Society. (D) Overview of the centrifugal droplet digital protein detection (CDPro) workflow. Reproduced with permission from ref. 104. Copyright 2023 The Royal Society of Chemistry. | |
Besides, microdroplet-based biomolecular detection could also be more suitable to point-of-care devices by combining innovate droplet preparation methods and the proximity ligation assay (PLA) to develop wash-free and portable workflow. PLA is a kind of signal amplification strategy based on immune PCR that could transfer the protein measurement to nucleic acid amplification. Byrnes et al.103 vortexed the aqueous reagents and the droplet generation oil simply for 30 s to generate the pL polydisperse droplet without complex instrumentation, in which the targets were detected by one-step PLA (Fig. 9(C)). Tang et al.104 designed the centrifugal droplet generation device to accomplish the detection of sub pg mL−1 targets by digital PLA (Fig. 9(D)). Hundreds of samples could be simultaneously emulsified to droplets with a micron scale diameter within 3 minutes by centrifuge. Surprisingly, the cost can be reduced to $0.5 per device in manufacture. These technologies achieved rapid and stable droplet generation without professional microfluidic chips and air pumps, which have the potential to achieve point-of-care (POC) detection in the future after integration with small PCR instruments.
4.2 Fully integrated and compact detection system
The ultimate goal of the combination of digital biomolecular detection and microfluidic chip is to solve the actual clinical issues, which put forward higher requirements for the equipment integration. Although Quanterix has developed benchtop instrument SR-X to meet the needs of ultrasensitive detection at more application places, it is still a semi-automated workflow that the reaction and imaging processes are not carried out in one container and instrument, so the user intervention exists in the process of transferring sample and the transfer inevitably resulted in losses (Fig. 10(A)). There are some attempts to make real lab-on-a-chip come true by integrating incubation and read-out module.
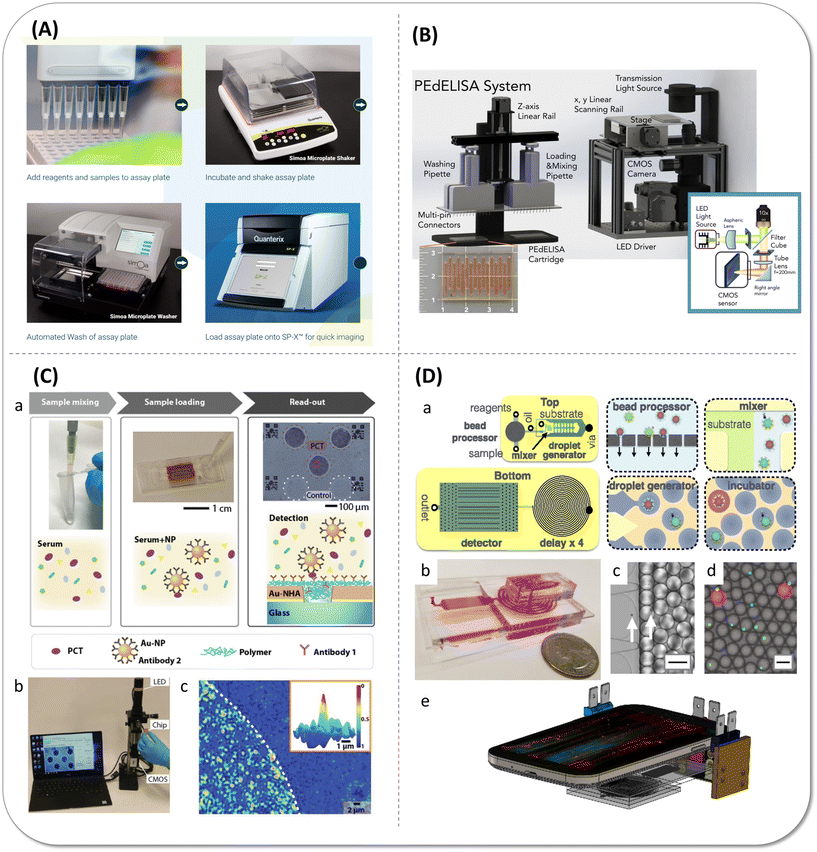 |
| Fig. 10 Integrated digital protein detection devices. (A) Schematic illustration of Simoa SP-X™ workflow. Copyright 2023 Co. Quanterix, Billerica, MA, USA (https://www.quanterix.com). (B) Schematic and photo image of the pre-equilibrium digital enzyme-linked immunosorbent assay (PEdELISA) system, which comprises a disposable microfluidic chip (inset), an automated fluidic dispensing and mixing module (left), and a 2-dimensional inverted fluorescence scanning module (right). Reproduced with permission from ref. 20. Copyright 2021 The American Society of Hematology. (C) Portable digital nanoparticle-enhanced plasmonic imager for biomarkers detection. (a) Reaction schematic of the digital nanoparticle-enhanced plasmonic imager. (b) The prototype reader uses a CMOS camera and a narrow-band LED source to record the transmitted images from a nanoplasmonic chip. (c) Plasmonic image of gold nanohole arrays (Au-NHA) area bound with nanoparticles. Reproduced with permission from ref. 105. Copyright 2019 WILEY-VCH GmbH. (D) Integrated microdroplet megascale detector (μMD) workflow. (a) A schematic of the μMD chip, showing both a top view and a bottom view. Each cartoon shows a schematic of the modules that are incorporated onto the μMD. (b) A photograph of the disposable μMD chip. (c) A micrograph showing the droplet generator encapsulate microbeads into droplets. The arrows highlight the microbeads (scale bar = 50 μm). (d) A fluorescence micrograph of the droplets after the delay line (scale bar = 50 μm). (e) A schematic of the μMD platform, consisting of a mobile phone, three light sources, and the disposable μMD chip. Reproduced with permission from ref. 86. Copyright 2019 National Academy of Sciences of the United States of America. | |
Song et al.20 combined a microarray cartridge, a compact fluidic dispensing and mixing module and a low-cost fluorescence scanning module to successfully accomplish large-scale parallel and rapid detection of ten cytokines from cytokine release syndrome (CRS) patients within 30 minutes, as can be seen in Fig. 10(B). They preloaded the capture antibody-coated beads into the well and incubated them later with the samples and the detection antibodies to exactly realize digital signal reading through a low target-to-bead ratio in a very short incubation time. However, the preloading strategy restricted the antigen recognition ability of antibodies, and pre-equilibrium stage quenching that stopped the reaction at 63.2% asymptotic value scarified LoD (sub pg mL−1 level) for fast sample-to-answer time. Belushkin et al.105 built a novel digital biomarker detection system based on the heat maps of gold nanohole array (Au-NHA) transmission intensity. The surface of Au-NHA was functionalized with the C-reactive protein (CRP) capture antibody. When CPR captured on Au-NHA following the detection antibody tethered AuNP binding, the transmission reduced by AuNPs captured on nanoholes on the basis of the Au-NHA extraordinary optical transmission (EOT) effect. CRP at 27 pg mL−1 could be detected within 2 hours, which is at least 4 orders of magnitude lower than clinically relevant concentrations. Later, they also optimized experimental workflow to measure another septic related biomarker, procalcitonin (PCT), in 20 microliter blood serum with high correlation to chemiluminescence analysis (CLIA) (Fig. 10(C)). Owing to the high sensitivity, they can identify sepsis from noninfectious systemic inflammatory response syndrome (SIRS) and healthy subjects within 15 minutes, which is dramatically faster than most laboratory immunoassays.106 Most importantly, the sample bound in the Au-NHA chip was measured by the compact and portable bright-field plasmonic imager containing a CMOS camera and a narrow-band LED source, which is extremely suitable for ambulances and emergency departments to get quick results at the early stage. However, the precise photolithography of nanohole array brought inconvenience for manufacture, and AuNPs outside the nanohole and false-positive signal inhibited the sensitivity. What is more, although both of the above-mentioned research involved immune reactions and in situ detection within the microfluidic chip, the reaction module and the detection module were not fully integrated. Yelleswarapu et al.86 designed a microdroplet megascale detector (μMD) to operate 107 pL droplets in parallel by hundreds of droplet generators and conducted the whole detection process in the highly integrated microfluidic chip, including the incubation process of immunoreaction. Interestingly, the acquisition and demonstration of signal was achieved using a mobile phone rather than a huge fluorescence microscope, as illustrated in Fig. 10(D). The high-throughput droplet generation gave rise to high bead sampling, dramatically rocketing the sensitivity of the low multiplicity detection. The duplex GM-CSF and IL-6 assay detected as low as 0.004 pg mL−1 (300 aM), which was similar to Simoa. Unfortunately, the mass beads took more than two hours to sample, which is not suitable for fast clinical detection.
To the best of our knowledge, integration and miniaturization constitute the tendency of digital assays for clinical practice. It has been witnessed that the microfluidic devices served as reactors and high signal-to-ratio images were acquired using an accessible reader with small size. However, the requirement of short incubation time and slow planar reaction kinetics tend to pose a risk to the sensitivity, which lose the advantage of digital detection. Additionally, the accuracy and stability of device should be verified. Chamber-free detection is convenient for clinical laboratory, but requires multiple reactions for signal amplification, which actually increases the assay complexity. Therefore, it is still a challenge on how to develop the compact digital bio-detection instrument for clinical accessible detection with similar LoD values to traditional digital ELISA.
5. Summary and outlook
Digital bioassay counts the number of “0” and “1” signals of each individual reactor rather than the bulk information value of the entire reaction system, which inhibits the background noise interference and brings a leap in detection performance. Thanks to advances in microfluidic technology, optical instruments and image processing algorithms, the access to single molecular events can be achieved in a higher throughput and simpler manner. The crossing and combination of various detection approaches also blossomed into diverse digital methods. Overall, the development of the digital immunoassay over the past few years mainly focused on the enhancement of sensitivity, multiplexing and clinical accessibility.
In terms of improving sensitivity, utilization ratio, reaction efficiency and background interference are the three perspectives of great concern. First, the bead utilization ratio has been improved in bead-based digital ELISA on the basis of raising the bead loading ratio and inhibiting the bead loss. In addition, label elution and enrichment strategy made nearly 100% signal of labelled-biomarkers analysed come true. Moreover, the creative design of microfluidic chip structures that break through the Poisson distribution and improve the single-bead encapsulation is expected to solve the issue of low utilization ratio especially when the target category increases. Second, the affinity of antibody pairs and the ligand–receptor pairs greatly influence the capture and label efficiency. Selecting good antibody pairs and keeping the antibody beneficial orientation would improve the antigen binding rate and capacity. High-affinity ligand–receptor combinations also facilitate rapid binding of label materials. Third, other tags such as nanoparticles with photon-upconversion and LSPR properties are beneficial to reduce interference from auto-fluorescence background signals. The distinctive binding features of antibody and antigen binding become a potential tool to distinguish the true positive signal from the non-specific binding without a washing step.
It is stated that the limited encoding capacity, low bead analysis rate and signal interference hold the barriers to multiplexing. The limitation of droplet encapsulation rate in ddELISA and bead loading efficiency in Simoa seriously affects the detection accuracy and resources as the multiplicity increases. Advances in imaging in the future may revolutionize the design of droplet microfluidic devices and the arrangement of droplets, so more droplets are able to be analysed in less time with the help of fast scanning and image reconstruction. As for the reaction, the non-specific crossing reactions much more serious than the single-plex assay are supposed to be focused on, so screening antibody pairs to get the highest affinity ones and exclude those with cross-reaction as well as non-specific adsorption becomes the pivotal step in the establishment of multiplex detection methodology. Increasingly, the innovative algorithm, chip design, and label type will be developed to reduce the signal interference to some extent.
To sum up, chamber-free strategies reveal that microbeads as both solid-phase carriers and isolation blocks display the most potential blueprint in all the exploration of digital immunoassay. With localized signal amplification, the digital signal on microbeads was easily counted using a flow cytometer, which has a great advantage in both digital detection sensitivity and throughput. To ensure the detection performance, here are some basic considerations on the construction of the partition-free detection method. First, signal generation should be specific and localized. The diffusion and deposition of signal molecules without specific binding site may contribute to the poor monodisperse positive signal distribution, which cause the overlap with a background signal and even generate false-positive signals. Second, improving the capture efficiency is the most effective and universal means to improve digital inspection performance. Furthermore, the large-size modules could produce a higher signal intensity, but probably decline the labelling efficiency. Multi-step signal amplification processes also diminish the final label efficiency. Hence, the continuous development of more ingenious signal amplification strategies is still highly expected. With the development of the binding and labelling approach, chamber-free digital biomolecular detection will attain excellent performance and the highest return on investment.
From the perspective of clinical accessibility, although there are plenty of approaches for the ultrasensitive quantification of biomarkers in the laboratory setting, the on-side operations have rarely taken advantage of digital detection performance up till now, owing to the problematic balance of the detection speed, sensitivity and device size. The pursuit of fast detection speed is bound to incomplete binding and low capture efficiency, while the ultrahigh sensitivity cannot be realized without sufficient assay time and marvellous signal readout equipment. In this regard, it is critical to generate and manipulate the reactors through a microfluidic chip, where the low assay volume can increase the diffusion kinetics and amplify the single-molecule signal. In the future, the clinical practical digital detection must have integrated operation and portable readout, which liberates the assay from the microtube. Accordingly, in order to satisfy the precise identification of the digital signals using a common reader, simple signal generation and amplification approaches with a high signal-to-noise ratio, and new data analysis algorithms to extract correct information from complex images are essential in the further research.
In the next decade, digital immunoassay is expected to primarily develop in two directions. One focuses on the improvement of sensitivity and multiplexing in a laboratory setting, denoting the discovery of new biomarkers for early screening and early diagnosis of diseases. Based on the advantage of digital bioassay, in the other aspect, it places emphasis on developing simpler devices that are suitable for clinical service as daily test tools. On the basis of the characteristics of microfluidic chips, the promising modules could be effectively integrated as low-cost detection platforms suitable for primary hospitals and community hospitals. We believe that the improvement of scientific and functional performance of digital protein detection will make a great contribution to the field of in vitro diagnosis and open up more possibilities for researchers to fight with diseases.
Author contributions
The manuscript was written through contributions of all authors. All authors have approved the final version of the manuscript. Yutong Zhang: conceptualization, investigation, writing – original draft, writing – review & editing. Hongchen Gu: funding acquisition. Hong Xu: supervision, conceptualization, writing – review & editing, funding acquisition.
Conflicts of interest
There are no conflicts of interest to declare.
Acknowledgements
This work is supported by the National Natural Science Foundation of China (Grant No. 31927803 and 82272122) and Interdisciplinary Program of Shanghai Jiao Tong University (Grant No. YG2022ZD028).
References
- J. Xu, Z. Sun, W. Li, L. Liu, F. Gao and D. Pan, J. Med. Virol., 2022, 94, 2645–2652 CrossRef CAS PubMed
.
- K. Sun, Y. W. Deng, J. Chen, G. H. Du, C. Song, J. Chen, L. Pan, X. Han, Y. F. Wang and G. Y. Tang, J. Dent. Sci., 2022, 17, 256–263 CrossRef PubMed
.
- D. Duffy, E. Nemes, A. Llibre, V. Rouilly, M. Musvosvi, N. Smith, E. Filander, H. Africa, S. Mabwe, L. Jaxa, B. Charbit, H. Mulenga, M. Tameris, G. Walzl, S. Malherbe, S. Thomas, M. Hatherill, N. Bilek, T. J. Scriba, M. L. Albert and C. Milieu Interieur, Clin. Infect. Dis., 2021, 73, e3398–e3408 CrossRef CAS PubMed
.
- X. Wu, Z. X. Xiao, J. W. Yi, S. N. Ding, H. C. Gu, W. Q. Wu, J. F. Luo, X. N. Liang, L. Zheng, H. Xu, Q. H. Zhao and D. Ding, Clin. Chem., 2021, 67, 1628–1639 CrossRef PubMed
.
- I. B. McInnes, C. D. Buckley and J. D. Isaacs, Nat. Rev. Rheumatol., 2016, 12, 63–68 CrossRef CAS PubMed
.
- D. T. Teachey, S. F. Lacey, P. A. Shaw, J. J. Melenhorst, S. L. Maude, N. Frey, E. Pequignot, V. E. Gonzalez, F. Chen, J. Finklestein, D. M. Barrett, S. L. Weiss, J. C. Fitzgerald, R. A. Berg, R. Aplenc, C. Callahan, S. R. Rheingold, Z. Zheng, S. Rose-John, J. C. White, F. Nazimuddin, G. Wertheim, B. L. Levine, C. H. June, D. L. Porter and S. A. Grupp, Cancer Discovery, 2016, 6, 664–679 CrossRef CAS PubMed
.
- J. C. M. Wan, C. Massie, J. Garcia-Corbacho, F. Mouliere, J. D. Brenton, C. Caldas, S. Pacey, R. Baird and N. Rosenfeld, Nat. Rev. Cancer, 2017, 17, 223–238 CrossRef CAS PubMed
.
- J. D. Merker, G. R. Oxnard, C. Compton, M. Diehn, P. Hurley, A. J. Lazar, N. Lindeman, C. M. Lockwood, A. J. Rai, R. L. Schilsky, A. M. Tsimberidou, P. Vasalos, B. L. Billman, T. K. Oliver, S. S. Bruinooge, D. F. Hayes and N. C. Turner, J. Clin. Oncol., 2018, 36, 1631–1641 CrossRef CAS PubMed
.
- L. Cohen and D. R. Walt, Chem. Rev., 2019, 119, 293–321 CrossRef CAS PubMed
.
- K. R. Sreejith, C. H. Ooi, J. Jin, D. V. Dao and N. T. Nguyen, Lab Chip, 2018, 18, 3717–3732 RSC
.
- H. F. Tsang, V. W. Xue, S. P. Koh, Y. M. Chiu, L. P. Ng and S. C. Wong, Expert Rev. Mol. Diagn., 2017, 17, 95–103 CrossRef CAS PubMed
.
- G. K. Geiss, R. E. Bumgarner, B. Birditt, T. Dahl, N. Dowidar, D. L. Dunaway, H. P. Fell, S. Ferree, R. D. George, T. Grogan, J. J. James, M. Maysuria, J. D. Mitton, P. Oliveri, J. L. Osborn, T. Peng, A. L. Ratcliffe, P. J. Webster, E. H. Davidson, L. Hood and K. Dimitrov, Nat. Biotechnol., 2008, 26, 317–325 CrossRef CAS PubMed
.
- J. A. Denis, E. Guillerm, F. Coulet, A. K. Larsen and J. M. Lacorte, Mol. Diagn. Ther., 2017, 21, 587–600 CrossRef CAS PubMed
.
- F. Diehl, M. Li, Y. He, K. W. Kinzler, B. Vogelstein and D. Dressman, Nat. Methods, 2006, 3, 551–559 CrossRef CAS PubMed
.
- D. M. Rissin, C. W. Kan, T. G. Campbell, S. C. Howes, D. R. Fournier, L. Song, T. Piech, P. P. Patel, L. Chang, A. J. Rivnak, E. P. Ferrell, J. D. Randall, G. K. Provuncher, D. R. Walt and D. C. Duffy, Nat. Biotechnol., 2010, 28, 595–599 CrossRef CAS PubMed
.
- K. Leirs, P. Tewari Kumar, D. Decrop, E. Perez-Ruiz, P. Leblebici, B. Van Kelst, G. Compernolle, H. Meeuws, L. Van Wesenbeeck, O. Lagatie, L. Stuyver, A. Gils, J. Lammertyn and D. Spasic, Anal. Chem., 2016, 88, 8450–8458 CrossRef CAS PubMed
.
- D. A. Olsen, C. L. Brasen, S. Kahns, J. B. Madsen, H. Kierkegaard, H. Christensen, A. Jensen, T. V. Sydenham, J. K. Moller, J. S. Madsen and I. Brandslund, Sci. Rep., 2021, 11, 20323 CrossRef CAS PubMed
.
- D. Shan, J. M. Johnson, S. C. Fernandes, H. Suib, S. Hwang, D. Wuelfing, M. Mendes, M. Holdridge, E. M. Burke, K. Beauregard, Y. Zhang, M. Cleary, S. Xu, X. Yao, P. P. Patel, T. Plavina, D. H. Wilson, L. Chang, K. M. Kaiser, J. Nattermann, S. V. Schmidt, E. Latz, K. Hrusovsky, D. Mattoon and A. J. Ball, Nat. Commun., 2021, 12, 1931 CrossRef PubMed
.
- H. Patel, N. J. Ashton, R. J. B. Dobson, L. M. Andersson, A. Yilmaz, K. Blennow, M. Gisslen and H. Zetterberg, Sci. Rep., 2021, 11, 6357 CrossRef CAS PubMed
.
- Y. Song, E. Sandford, Y. Tian, Q. Yin, A. G. Kozminski, S. H. Su, T. Cai, Y. Ye, M. T. Chung, R. Lindstrom, A. Goicochea, J. Barabas, M. Olesnavich, M. Rozwadowski, Y. Li, H. B. Alam, B. H. Singer, M. Ghosh, S. W. Choi, M. Tewari and K. Kurabayashi, Blood, 2021, 137, 1591–1602 CrossRef CAS PubMed
.
- E. Perez-Ruiz, D. Decrop, K. Ven, L. Tripodi, K. Leirs, J. Rosseels, M. van de Wouwer, N. Geukens, A. De Vos, E. Vanmechelen, J. Winderickx, J. Lammertyn and D. Spasic, Anal. Chim. Acta, 2018, 1015, 74–81 CrossRef CAS PubMed
.
- L. Song, D. R. Lachno, D. Hanlon, A. Shepro, A. Jeromin, D. Gemani, J. A. Talbot, M. M. Racke, J. L. Dage and R. A. Dean, Alzheimer's Res. Ther., 2016, 8, 58 CrossRef PubMed
.
- X. Wu, Z. Xiao, J. Yi, S. Ding, H. Gu, W. Wu, J. Luo, X. Liang, L. Zheng, H. Xu, Q. Zhao and D. Ding, Clin. Chem., 2021, 67, 1628–1639 CrossRef PubMed
.
- B. Thangavelu and A. M. Boutte, ACS Omega, 2021, 6, 9609–9616 CrossRef CAS PubMed
.
- X. Wang, A. F. Ogata and D. R. Walt, J. Am. Chem. Soc., 2020, 142, 15098–15106 CrossRef CAS PubMed
.
- C. Wu, P. M. Garden and D. R. Walt, J. Am. Chem. Soc., 2020, 142, 12314–12323 CrossRef CAS PubMed
.
- J. U. Shim, R. T. Ranasinghe, C. A. Smith, S. M. Ibrahim, F. Hollfelder, W. T. Huck, D. Klenerman and C. Abell, ACS Nano, 2013, 7, 5955–5964 CrossRef CAS PubMed
.
- L. Cohen, N. Cui, Y. Cai, P. M. Garden, X. Li, D. A. Weitz and D. R. Walt, ACS Nano, 2020, 14, 9491–9501 CrossRef CAS PubMed
.
- J. Todd, B. Freese, A. Lu, D. Held, J. Morey, R. Livingston and P. Goix, Clin. Chem., 2007, 53, 1990–1995 CrossRef CAS PubMed
.
- T. E. Kaier, R. Twerenbold, B. Alaour, P. Badertscher, C. Puelacher, J. Marjot, J. Boeddinghaus, T. Nestelberger, K. Wildi, D. Wussler, M. Rubini-Gimenez, T. Reichlin, M. Marber and C. Mueller, Eur. Heart J., 2018, 39, 218 CrossRef
.
- M. P. Bonaca, R. G. O'Malley, S. A. Murphy, P. Jarolim, M. J. Conrad, E. Braunwald, M. S. Sabatine and D. A. Morrow, Eur. Heart J. Acute Cardiovasc. Care, 2015, 4, 431–440 CrossRef PubMed
.
- K. Akama, K. Shirai and S. Suzuki, Anal. Chem., 2016, 88, 7123–7129 CrossRef CAS PubMed
.
- C. Wu, T. J. Dougan and D. R. Walt, ACS Nano, 2022, 16, 1025–1035 CrossRef CAS PubMed
.
- J. Zhang, J. Wu, C. Chen, G. He, W. Liu, S. Xu, H. Gu, Y. Wang and H. Xu, Talanta, 2023, 262, 124685 CrossRef CAS PubMed
.
- W. Jing, Y. Wang, Y. Yang, Y. Wang, G. Ma, S. Wang and N. Tao, ACS Nano, 2019, 13, 8609–8617 CrossRef CAS PubMed
.
- D. Sevenler, G. G. Daaboul, F. Ekiz Kanik, N. L. Unlu and M. S. Unlu, ACS Nano, 2018, 12, 5880–5887 CrossRef CAS PubMed
.
- W. Zhang, T. Dang, Y. Li, J. Liang, H. Xu, G. L. Liu and W. Hu, Sens. Actuators, B, 2021, 348, 130711 CrossRef CAS
.
- L. He, D. R. Tessier, K. Briggs, M. Tsangaris, M. Charron, E. M. McConnell, D. Lomovtsev and V. Tabard-Cossa, Nat. Commun., 2021, 12, 5348 CrossRef CAS PubMed
.
- Z. Wu, C. H. Zhou, L. J. Pan, T. Zeng, L. Zhu, D. W. Pang and Z. L. Zhang, Anal. Chem., 2016, 88, 9166–9172 CrossRef CAS PubMed
.
- K. Akama, N. Iwanaga, K. Yamawaki, M. Okuda, K. Jain, H. Ueno, N. Soga, Y. Minagawa and H. Noji, ACS Nano, 2019, 13, 13116–13126 CrossRef CAS PubMed
.
- K. Akama and H. Noji, Lab Chip, 2020, 20, 2113–2121 RSC
.
- E. W. A. Visser, J. Yan, L. J. van IJzendoorn and M. W. J. Prins, Nat. Commun., 2018, 9, 2541 CrossRef PubMed
.
- T. Chatterjee, A. Knappik, E. Sandford, M. Tewari, S. W. Choi, W. B. Strong, E. P. Thrush, K. J. Oh, N. Liu, N. G. Walter and A. Johnson-Buck, Proc. Natl. Acad. Sci. U. S. A., 2020, 117, 22815–22822 CrossRef CAS PubMed
.
- Z. Farka, M. J. Mickert, A. Hlavacek, P. Skladal and H. H. Gorris, Anal. Chem., 2017, 89, 11825–11830 CrossRef CAS PubMed
.
- M. J. Mickert, Z. Farka, U. Kostiv, A. Hlavacek, D. Horak, P. Skladal and H. H. Gorris, Anal. Chem., 2019, 91, 9435–9441 CrossRef CAS PubMed
.
- M. Haase and H. Schafer, Angew. Chem., Int. Ed., 2011, 50, 5808–5829 CrossRef CAS PubMed
.
- X. Liu, C. Huang, X. Dong, A. Liang, Y. Zhang, Q. Zhang, Q. Wang and H. Gai, Chem. Commun., 2018, 54, 13103–13106 RSC
.
- X. Liu, C. Huang, C. Zong, A. Liang, Z. Wu, Y. Zhang, Q. Zhang, W. Zhao and H. Gai, ACS Sens., 2018, 3, 2644–2650 CrossRef CAS PubMed
.
- X. Liu, Y. Sun, X. Lin, X. Pan, Z. Wu and H. Gai, Anal. Chem., 2021, 93, 3089–3095 CrossRef CAS PubMed
.
- S. H. Kim, S. Iwai, S. Araki, S. Sakakihara, R. Iino and H. Noji, Lab Chip, 2012, 12, 4986–4991 RSC
.
- D. H. Wilson, D. W. Hanlon, G. K. Provuncher, L. Chang, L. Song, P. P. Patel, E. P. Ferrell, H. Lepor, A. W. Partin, D. W. Chan, L. J. Sokoll, C. D. Cheli, R. P. Thiel, D. R. Fournier and D. C. Duffy, Clin. Chem., 2011, 57, 1712–1721 CrossRef CAS PubMed
.
- L. Shang, Y. Cheng and Y. Zhao, Chem. Rev., 2017, 117, 7964–8040 CrossRef CAS PubMed
.
- X. Yue, X. Fang, T. Sun, J. Yi, X. Kuang, Q. Guo, Y. Wang, H. Gu and H. Xu, Biosens. Bioelectron., 2022, 211, 114384 CrossRef CAS PubMed
.
- C. W. Kan, C. I. Tobos, D. M. Rissin, A. D. Wiener, R. E. Meyer, D. M. Svancara, A. Comperchio, C. Warwick, R. Millington, N. Collier and D. C. Duffy, Lab Chip, 2020, 20, 2122–2135 RSC
.
- D. Decrop, G. Pardon, L. Brancato, D. Kil, R. Zandi Shafagh, T. Kokalj, T. Haraldsson, R. Puers, W. van der Wijngaart and J. Lammertyn, ACS Appl. Mater. Interfaces, 2017, 9, 10418–10426 CrossRef CAS PubMed
.
- D. Witters, K. Knez, F. Ceyssens, R. Puers and J. Lammertyn, Lab Chip, 2013, 13, 2047–2054 RSC
.
- W. Lyu, J. Yi, Q. Guo, Y. Wang, J. Zhang, T. Sun, H. Ma, T. Tang, X. Quan, H. Xu, F. Shen and H. Gu, ACS Nano, 2023, 17, 12641–12651 CrossRef CAS PubMed
.
- L. Zhang, W. Fan, D. Jia, Q. Feng, W. Ren and C. Liu, Anal. Chem., 2021, 93, 14828–14836 CrossRef CAS PubMed
.
- D. Chen, X. Zhang, L. Zhu, C. Liu and Z. Li, Chem. Sci., 2022, 13, 3501–3506 RSC
.
- A. H. Wu, N. Fukushima, R. Puskas, J. Todd and P. Goix, Clin. Chem., 2006, 52, 2157–2159 CrossRef CAS PubMed
.
- A. Bhumkar, C. Magnan, D. Lau, E. S. W. Jun, N. Dzamko, Y. Gambin and E. Sierecki, Angew. Chem., Int. Ed., 2021, 60, 11874–11883 CrossRef CAS PubMed
.
- J. Todd, P. Simpson, J. Estis, V. Torres and A. H. Wub, Cytokine+, 2013, 64, 660–665 CrossRef CAS PubMed
.
- K. B. Gordon, A. W. Armstrong, P. Foley, M. Song, Y. K. Shen, S. Li, E. J. Munoz-Elias, P. Branigan, X. Liu and K. Reich, J. Invest. Dermatol., 2019, 139, 2437–2446 e2431 CrossRef CAS PubMed
.
- B. Strober, A. Alikhan, B. Lockshin, R. Shi, J. Cirulli and P. Schafer, J. Dermatol. Sci., 2019, 96, 126–133 CrossRef CAS PubMed
.
- L. Zhu, G. Li, S. Sun, H. Tan and Y. He, RSC Adv., 2017, 7, 27595–27602 RSC
.
- Q. Zhang, X. Zhang, J. Li and H. Gai, Anal. Chem., 2020, 92, 654–658 CrossRef CAS PubMed
.
- L. Chang, D. M. Rissin, D. R. Fournier, T. Piech, P. P. Patel, D. H. Wilson and D. C. Duffy, J. Immunol. Methods, 2012, 378, 102–115 CrossRef CAS PubMed
.
- T. L. Dinh, K. C. Ngan, C. B. Shoemaker and D. R. Walt, Anal. Chem., 2016, 88, 11335–11339 CrossRef CAS PubMed
.
- D. Wu, E. Katilius, E. Olivas, M. Dumont Milutinovic and D. R. Walt, Anal. Chem., 2016, 88, 8385–8389 CrossRef CAS PubMed
.
- L. Cohen and D. R. Walt, Bioconjugate Chem., 2018, 29, 3452–3458 CrossRef CAS PubMed
.
- Y. Wang, Z. Gao, J. Yi, H. Zhou, X. Fang, H. Xu, J. Zhao and H. Gu, RSC Adv., 2019, 9, 23658–23665 RSC
.
- D. L. Jeanmaire and R. P. Van Duyne, J. Electroanal. Chem. Interfacial Electrochem., 1977, 84, 1–20 CrossRef CAS
.
- M. G. Albrecht and J. A. Creighton, J. Am. Chem. Soc., 2002, 99, 5215–5217 CrossRef
.
- J. Li, A. Wuethrich, A. A. I. Sina, H. H. Cheng, Y. Wang, A. Behren, P. N. Mainwaring and M. Trau, Nat. Commun., 2021, 12, 1087 CrossRef CAS PubMed
.
- K. Ito, K. Y. Inoue, K. Ino and H. Shiku, Biosens. Bioelectron.: X, 2023, 13, 100312 CAS
.
- S. Mandal, Z. Li, T. Chatterjee, K. Khanna, K. Montoya, L. Dai, C. Petersen, L. Li, M. Tewari, A. Johnson-Buck and N. G. Walter, Acc. Chem. Res., 2021, 54, 388–402 CrossRef CAS PubMed
.
- D. S.-z. Zhang, Y. Jiang, H. Yang, Y. Zhu, S. Zhang, Y. Zhu, D. Wei, Y. Lin, P. Wang, Q. Fu, H. Xu and H. Gu, Adv. Funct. Mater., 2016, 26, 6146–6157 CrossRef CAS
.
- S. Lu, D. S. Zhang, D. Wei, Y. Lin, S. Zhang, H. He, X. Wei, H. Gu and H. Xu, Chem. Mater., 2017, 29, 10398–10408 CrossRef CAS
.
- Y. Wang, C. Chen, J. He, Y. Cao, X. Fang, X. Chi, J. Yi, J. Wu, Q. Guo, H. Masoomi, C. Wu, J. Ye, H. Gu and H. Xu, Small, 2021, 17, e2100315 CrossRef PubMed
.
- Y. Song, J. Zhao, T. Cai, A. Stephens, S. H. Su, E. Sandford, C. Flora, B. H. Singer, M. Ghosh, S. W. Choi, M. Tewari and K. Kurabayashi, Biosens. Bioelectron., 2021, 180, 113088 CrossRef CAS PubMed
.
- A. D. Stephens, Y. Song, B. L. McClellan, S.-H. Su, S. Xu, K. Chen, M. G. Castro, B. H. Singer and K. Kurabayashi, Biosens. Bioelectron., 2023, 237, 115536 CrossRef CAS PubMed
.
- S. C. Tsao, J. Wang, Y. Wang, A. Behren, J. Cebon and M. Trau, Nat. Commun., 2018, 9, 1482 CrossRef PubMed
.
- X. Liu, M. Li, X. Yu, L. Shen and W. Li, Biosens. Bioelectron., 2023, 219, 114804 CrossRef CAS PubMed
.
- W. Zhao, Y. Zhou, Y.-Z. Feng, X. Niu, Y. Zhao, J. Zhao, Y. Dong, M. Tan, Y. Xianyu and Y. Chen, ACS Nano, 2023, 17, 13700–13714 CrossRef CAS PubMed
.
- Y. Zhou, W. Zhao, Y. Feng, X. Niu, Y. Dong and Y. Chen, Anal. Chem., 2023, 95, 1589–1598 CAS
.
- V. Yelleswarapu, J. R. Buser, M. Haber, J. Baron, E. Inapuri and D. Issadore, Proc. Natl. Acad. Sci. U. S. A., 2019, 116, 4489–4495 CrossRef CAS PubMed
.
- J. Yi, Z. Gao, Q. Guo, Y. Wu, T. Sun, Y. Wang, H. Zhou, H. Gu, J. Zhao and H. Xu, Sens. Actuators, B, 2022, 369, 132214 CrossRef CAS
.
- C. Wu, T. J. Dougan and D. R. Walt, ACS Nano, 2022, 16, 1025–1035 CrossRef CAS PubMed
.
- J. P. Frampton, J. B. White, A. B. Simon, M. Tsuei, S. Paczesny and S. Takayama, Sci. Rep., 2014, 4, 4878 CrossRef CAS PubMed
.
- T. Gilboa, A. M. Maley, A. F. Ogata, C. Wu and D. R. Walt, Adv. Healthcare Mater., 2021, 10, e2001111 CrossRef PubMed
.
- D. Wu, M. D. Milutinovic and D. R. Walt, Analyst, 2015, 140, 6277–6282 RSC
.
- D. M. Rissin, C. W. Kan, L. Song, A. J. Rivnak, M. W. Fishburn, Q. Shao, T. Piech, E. P. Ferrell, R. E. Meyer, T. G. Campbell, D. R. Fournier and D. C. Duffy, Lab Chip, 2013, 13, 2902–2911 RSC
.
- A. J. Rivnak, D. M. Rissin, C. W. Kan, L. Song, M. W. Fishburn, T. Piech, T. G. Campbell, D. R. DuPont, M. Gardel, S. Sullivan, B. A. Pink, C. G. Cabrera, D. R. Fournier and D. C. Duffy, J. Immunol. Methods, 2015, 424, 20–27 CrossRef CAS PubMed
.
- J. M. Vincent, J. Fluorine Chem., 2008, 129, 903–909 CrossRef CAS
.
- P. Gruner, B. Riechers, L. A. C. Orellana, Q. Brosseau, F. Maes, T. Beneyton, D. Pekin and J. C. Baret, Curr. Opin. Colloid Interface Sci., 2015, 20, 183–191 CrossRef CAS
.
- Z. Gao, J. Yi, J. Zhao, H. Gu, H. Zhou and H. Xu, Sens. Actuators, B, 2020, 324, 128716 CrossRef CAS
.
- P. Gruner, B. Riechers, B. Semin, J. Lim, A. Johnston, K. Short and J. C. Baret, Nat. Commun., 2016, 7, 10392 CrossRef CAS PubMed
.
- K. Akama and H. Noji, Analyst, 2021, 146, 1303–1310 RSC
.
- C. Che, N. Li, K. D. Long, M. A. Aguirre, T. D. Canady, Q. Huang, U. Demirci and B. T. Cunningham, Lab Chip, 2019, 19, 3943–3953 RSC
.
- B. Zhao, C. Che, W. Wang, N. Li and B. T. Cunningham, Talanta, 2021, 225, 122004 CrossRef CAS PubMed
.
- W. Jing, Y. Wang, C. Chen, F. Zhang, Y. Yang, G. Ma, E. H. Yang, C. L. N. Snozek, N. Tao and S. Wang, ACS Sens., 2021, 6, 399–407 CrossRef PubMed
.
- Y. Wang, Y. Yang, C. Chen, S. Wang, H. Wang, W. Jing and N. Tao, ACS Sens., 2020, 5, 1126–1131 CrossRef CAS PubMed
.
- S. A. Byrnes, T. Huynh, T. C. Chang, C. E. Anderson, J. J. McDermott, C. I. Oncina, B. H. Weigl and K. P. Nichols, Anal. Chem., 2020, 92, 3535–3543 CrossRef CAS PubMed
.
- Z. Tang, F. Lv, D. E. Reynolds, S. Zhang, S. Zheng, J. Ko, Y. Chen and Y. Wang, Lab Chip, 2023, 23, 2758–2765 RSC
.
- A. Belushkin, F. Yesilkoy and H. Altug, ACS Nano, 2018, 12, 4453–4461 CrossRef CAS PubMed
.
- A. Belushkin, F. Yesilkoy, J. J. Gonzalez-Lopez, J. C. Ruiz-Rodriguez, R. Ferrer, A. Fabrega and H. Altug, Small, 2020, 16, e1906108 CrossRef PubMed
.
|
This journal is © The Royal Society of Chemistry 2024 |