DOI:
10.1039/D4SC00103F
(Edge Article)
Chem. Sci., 2024,
15, 6095-6105
Can local heating and molecular crowders disintegrate amyloid aggregates?†
Received
6th January 2024
, Accepted 18th March 2024
First published on 19th March 2024
Abstract
The present study employs a blend of molecular dynamics simulations and a theoretical model to explore the potential disintegration mechanism of a matured Aβ octamer, aiming to offer a strategy to combat Alzheimer's disease. We investigate local heating and crowding effects on Aβ disintegration by selectively heating key Aβ segments and varying the concentration of sodium dodecyl sulphate (SDS), respectively. Despite initiation of disruption, Aβ aggregates resist complete disintegration during local heating due to rapid thermal energy distribution to the surrounding water. Conversely, although SDS molecules effectively inhibit Aβ aggregation at higher concentration through micelle formation, they fail to completely disintegrate the aggregate due to the exceedingly high energy barrier. To address the sampling challenge posed by the formidable energy barrier, we have performed well-tempered metadynamics simulations. Simulations reveal a multi-step disintegration mechanism for the Aβ octamer, suggesting a probable sequence: octamer → pentamer/hexamer ⇌ tetramer → monomer, with a rate-determining step constituting 45 kJ mol−1 barrier during the octamer to pentamer/hexamer transition. Additionally, we have proposed a novel two-state mean-field model based on Ising spins that offers an insight into the kinetics of the Aβ growth process and external perturbation effects on disintegration. Thus, the current simulation study, coupled with the newly introduced mean-field model, offers an insight into the detailed mechanisms underlying the Aβ aggregation process, guiding potential strategies for effective disintegration of Aβ aggregates.
1 Introduction
The formation of amyloids due to the aggregation of proteins is associated with various neurodegenerative diseases such as Alzheimer's and Parkinson's. Alzheimer's disease (AD) is the most common form of dementia that affects memory, thinking power, and behavioural patterns, eventually leading to death. The primary cause behind the pathogenesis of AD is the aggregation of the Aβ peptide, a widely accepted hypothesis in the researchers' community.1,2 Therefore, understanding the molecular factors controlling the Aβ aggregation and discovering drug molecules capable of preventing or at least slowing down the Aβ aggregation is a central goal of AD research.
The Aβ peptide forms small soluble oligomers at the early stages of aggregation, which further aggregate into insoluble fibrils and accumulate in the brain.3–6 Hydrophobic amino acids play a central role in the aggregation.7 These residues localize at three distinct regions, namely, (i) the central hydrophobic core, Aβ16–20(KLVFF), (ii) second central region, Aβ29–35(GAIIGLM), and (iii) C-terminal, Aβ36–42(VGGVVIA).8–10 The aggregation process is initiated by forming an aggregation-prone structure that involves the coil-to-β-hairpin structural transition of individual monomers.11–15 This aggregation-prone structure changes the functional properties of the Aβ peptide by forming soluble oligomers that induce intracellular toxicity by crossing the plasma membrane.2 While this information has added significant insight into AD research, a comprehensive picture of the aggregation mechanism is still elusive, and thus further research is needed.
The current understanding of AD research implies that the potential therapeutic strategy for treating Alzheimer's patients is to inhibit the growth of soluble oligomers/fibrils and simultaneously disintegrate the matured oligomers/fibrils. Thus, a more realistic therapeutic approach should be to develop methods to ensure that the Aβ peptide will exist in its non-toxic monomeric form, disintegrating the oligomers/fibrils. Numerous approaches are proposed in this regard, but their practical implementation is a real challenge. For example, various small molecules, including peptides, peptidomimetics, nanoparticles, nano chaperones, macrocycles, etc., have been shown to inhibit Aβ oligomerization.16–21 Recently, Nguyen et al. reviewed a series of experimental and computational findings regarding the monomers, oligomeric intermediates, and fibrils of the amyloid proteins in aqueous and membrane medium and discussed their implications for Alzheimer's disease.2 Many other similar studies using experiments and molecular dynamics simulation studies have been performed to get more insight into the structural aspects of Aβ peptide and AD.22–28 A very recent study reports that external electric or magnetic fields can disintegrate the Aβ oligomers and maybe a potential strategy to treat Alzheimer's patients.21 Similarly, different strategies to disintegrate the amyloid aggregates have been reported, e.g., by ultrasonic waves,29,30 infrared laser,31 laser induced disruption,32 free electron laser irradiation,33etc.34 Despite the discovery of such small inhibitory molecules or the use of external fields for treating Alzheimer's patients, the lack of biocompatibility of the inhibitory molecules primarily renders challenges toward the success of obtaining clinically viable therapeutics. Hence, current AD research focuses on designing biocompatible drug molecules that can inhibit oligomerization and maintain the Aβ peptide in its monomeric form.
Lipopeptide-based nanomaterials derived from naturally occurring biological building blocks have received significant attention in AD research owing to their safety, biodegradability, and biocompatibility.35,36 For instance, Bera et al.,37 in their recent microscopic investigation, illustrated that a myristoyl-KPGPK lipopeptide-based nanovesicle dramatically hinders the random coil to β-sheet transformation of the transmembrane GxxxGxxxGxxxG motif of Aβ-protein and human myelin protein zero, a prerequisite for the oligomerization of the peptide. Förster resonance energy transfer (FRET) assay performed with the synthesized Cy-3 (FRET donor) and Cy-5 (FRET acceptor)-conjugated peptide further confirms that the nanovesicle strongly inhibits the fibril formation of the peptide. Furthermore, this study highlights a suitable balance between the hydrophilic and hydrophobic interaction caused by the nanovesicle, a necessary step for the inhibition. While this could serve as an essential insight for clinically viable drug design, the study failed to address some critical questions necessary for further testing the clinical efficiency of this nanovesicle. For example, they confirmed the nanovesicle's inhibition effect by performing experiments starting from the monomeric form of the peptide. However, this cannot guarantee whether this nanovesicle can disintegrate the already formed oligomer, which is an important criterion to be a clinically viable AD drug. Therefore, it is worthwhile to probe (i) whether such a nanovesicle can disintegrate an already-formed oligomer and (ii) the molecular origin behind the inhibition by the nanovesicle.
In this work, we aim to address the two points mentioned above by molecular dynamics simulations (details are presented in the ESI†). However, unlike the experimental study mentioned above, we consider sodium dodecyl sulfate (SDS). There are two rationales behind choosing this surfactant molecule over myristoyl-KPGPK lipopeptide. First, SDS is a much simpler molecule than the myristoyl-KPGPK lipopeptide, thus reducing the computational cost. Second, SDS molecules can self-assemble to form micelles due to amphiphilic moieties and hence can mimic a similar environment to myristoyl-KPGPK nanovesicles. Moreover, SDS molecules self-assemble to form tiny clusters at low concentrations and vesicles at high concentrations and modify the solution properties. This property of the SDS molecule has an added advantage as this allows us to modify the strength of intermolecular interactions between the SDS molecules and the Aβ peptide by varying the number of SDS molecules. Since the intermolecular interactions between the monomers primarily guide the aggregation process, the variation of the number of molecules gives us scope to shed light into the aggregation process and, hence, predict what type of interactions must be present in a drug molecule to exhibit the inhibition of aggregation.
We have also investigated whether thermal heating can disrupt the already formed Aβ oligomer. Here we address whether heating can disrupt the β-sheets of the monomers present in the oligomer. If this strategy works for disintegrating the Aβ oligomers, this will be a clinically viable option if the temperature ranges within tolerable physiological conditions. Hence, we apply a moderate temperature only on regions of Aβ peptides primarily known to be responsible for aggregation. Such an application of temperature is simply done by increasing the kinetic energy of the specific atoms of Aβ peptide; hence, we call this “local heating”.
Herein in this study, we tackle the intricacies of the Aβ aggregation process through a unique combination of molecular dynamics simulations and a novel mean-field model. We assess the influence of external perturbations such as a molecular crowder (sodium dodecyl sulphate) and/or “local heating” (selective heating of Aβ segments through increase of the kinetic energy of the respective segments) on disrupting the Aβ octamer using conventional molecular dynamics simulations. While partly disrupted, complete disruption of the Aβ octamer does not happen due to the exceedingly high energy barrier on applying these external perturbations. Well-tempered metadynamics simulations overcome sampling challenges, providing detailed insights into the Aβ disintegration process. Additionally, a two-state mean-field model based on Ising spins introduced for the first time in this study explains the kinetics and external perturbation effects of Aβ disintegration. This integrated approach hence sheds light on the Aβ aggregation mechanism, guiding potential strategies for effective disintegration.
2 Results and discussion
2.1 Equilibrium properties under perturbation
As discussed in the introduction, the most effective approach to treat Alzheimer's disease is to maintain the Aβ peptides in the monomeric non-toxic form by inhibiting the aggregation as well as by disintegrating the preformed Aβ peptide oligomers/fibrils. One recent experiment demonstrated the inhibitory effect of myristoyl-KPGPK lipopeptide-based nanovesicles on Aβ aggregation but did not explore their ability to disintegrate the preformed Aβ oligomer. In this simulation study, we investigate the disintegrating potential of a simpler molecule, sodium dodecyl sulfate (SDS), which possesses similar characteristics to the lipopeptide. We specifically address two points discussed in the following section. (i) Can the SDS molecule disintegrate the Aβ oligomer? (ii) Is there any effect of SDS concentration on Aβ aggregation?
2.1.1 The molecular crowder SDS can be a potent inhibitor but not necessarily be a disruptor of the aggregation in amyloid structures.
Fig. 1A shows a few representative Aβ peptide configurations at each SDS concentration. Results reveal that Aβ peptides form a β-hairpin structure in the presence of SDS molecules, forming a dimer and trimer. This finding is meaningful except for low or high SDS concentration. At low SDS concentrations, we observe the formation of helical conformations in some of the Aβ peptides; however, at high SDS concentrations, the peptides adopt coiled structures. Such a concentration-dependent impact of SDS on Aβ peptide conformation, as observed in our study, aligns with previous research indicating that a high level of crowding inhibits β-hairpin formation.38,39 To assess the disintegrating ability of SDS molecules and the effect of their concentration, we first calculate the root mean square deviation (RMSD) of the Aβ octamer obtained from octamer simulations in the presence of SDS molecules (Fig. 1B) (see also ESI Fig. S1†). Results reveal a decrease in RMSD values with increased SDS molecules. This finding correlates well with favourable hydrophobic interactions between β-sheet regions of Aβ peptide and tail groups of SDS molecules, suggesting stabilization of the Aβ octamer in the presence of SDS molecules. Results presented above suggest that SDS molecules lack the potential to disintegrate the preformed Aβ oligomer within our simulation time scale. Nevertheless, this does not eliminate its potential to inhibit the Aβ aggregation. To explore this possibility, we have conducted simulations of free monomeric Aβ peptides in the presence of the SDS molecules. To ensure that our simulations commence with the monomeric state, we placed eight monomers randomly by maintaining a substantial separation from each other. Furthermore, we have examined the influence of different concentrations of SDS by varying the number of SDS molecules.
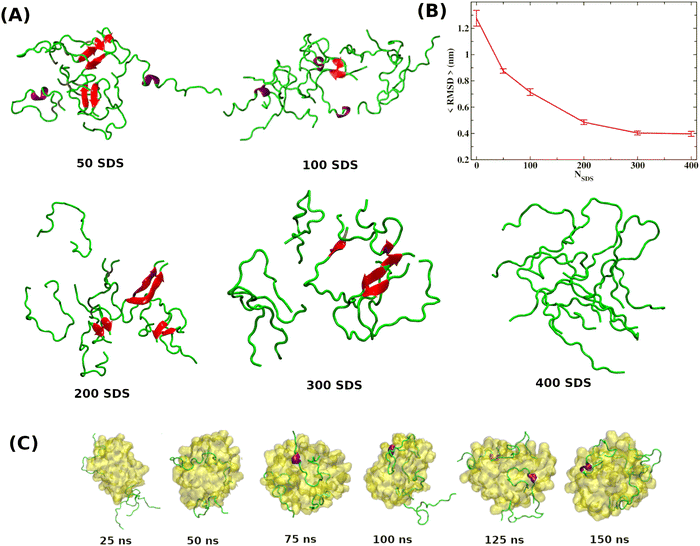 |
| Fig. 1 (A) Few representative Aβ peptide configurations at each SDS concentration. Results reveal that Aβ peptides form a β-hairpin structure in the presence of SDS molecules, forming a dimer and trimer. Formation of secondary structures, i.e., α-helix and β-sheet, are shown in red and purple. (B) The time-averaged root mean square deviation (RMSD) variation of the Aβ octamer obtained from octamer simulations as a function of SDS molecules. (C) Simulations of eight Aβ peptide monomers in the presence of SDS micelles indicate that Aβ monomers get stabilized by wrapping around the SDS micelle, thereby reducing their propensity to associate with others. | |
2.1.2 High-concentration micelle formation of SDS as a key driver of aggregation inhibition.
Although the data presented above suggest that high SDS concentrations can inhibit Aβ aggregation, it does not explain its origin; at low or moderate SDS concentrations, SDS molecules cannot inhibit the aggregation process. A high concentration of SDS may promote the formation of SDS micelles, which is essential for inhibiting Aβ aggregation. Simulations of eight Aβ peptide monomers in the presence of SDS micelles indicate that Aβ monomers get stabilized by wrapping around the SDS micelle, thereby reducing their propensity to associate with other Aβ monomers (Fig. 1C). However, SDS micelles cannot disintegrate the preformed Aβ aggregate as confirmed by another simulation of the Aβ octamer in the presence of SDS micelles. Our findings thus suggest that SDS micelles can inhibit the aggregation process; they cannot disintegrate the preformed aggregate, or the energy barrier required for disintegration is prohibitively high at room temperatures (300 K).
2.1.3 SDS-driven phase separation strengthens protein–protein interaction, weakens protein–water interaction, limiting aggregate disintegration.
To scrutinize the molecular origin behind the inability of SDS molecules to disintegrate the Aβ octamer, we calculate the average number of water molecules present within 5 Å of the octamer surface that essentially represents the hydration layer (see the radial distribution function, ESI Fig. S2†). Analyzing this number as a function of SDS molecules reveals a decreasing trend with increasing SDS concentration (Fig. 2A). This observation suggests that the molecular crowder SDS increases the coacervate volume fraction and density but does not partition into the dense phase. Consequently, a higher SDS concentration facilitates dehydration, contributing to an overall gain in entropy. It highlights the significance of entropy gain resulting from water expulsion from the octamer surface upon increasing SDS concentration as a primary driving force behind SDS-assisted Aβ aggregation. We further inspect how the presence of SDS molecules impacts interactions between different system components by computing all possible pairwise interaction energies for the Aβ octamer system as a function of SDS concentration (Fig. 2B). The data demonstrate a substantial alteration in protein–protein (EPP) and protein–water (EPW) interactions during the transition from the monomeric state to the octamer, underscoring the influential role of these interactions in aggregation, particularly in the presence of SDS molecules. The observed changes in these interactions indicate that SDS molecules promote monomer association assisted by the reduced strength of protein–water interaction, resulting in water expulsion. The influence of SDS-assisted promotion of Aβ aggregation intensifies with rising SDS concentration, as depicted by the total net interaction energy plotted against SDS concentration in the inset of Fig. 2B.
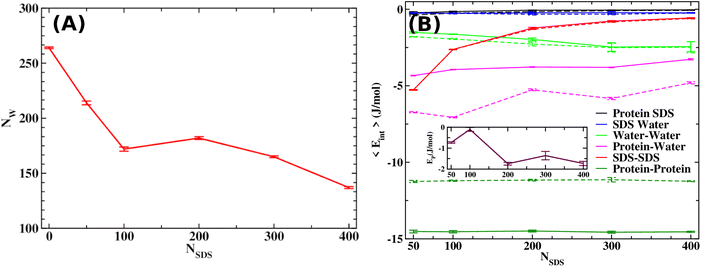 |
| Fig. 2 (A) The average number of water molecules as a function of SDS molecules reveals a decreasing trend upon increasing SDS concentration. (B) All possible pairwise interaction energies for the Aβ octamer system as a function of SDS concentration. The inset figure shows net interaction energy, EP = ΔEPP + ΔEPW + ΔEPS, as a function of SDS. Solid and dashed lines in the figure show the results for the octamer and monomeric peptides. | |
2.1.4 “Local heating” promotes an ordered state over a disordered state, restricting aggregate disruption.
The above discussion shows that SDS molecules cannot disintegrate the Aβ octamer at room temperature (300 K) due to a high energy barrier. An increase in temperature could induce the disintegration. However, this possibility is ruled out by earlier simulation and experimental studies, which show retention or promotion of β-sheet in Aβ peptide with increasing temperature. The primary factor behind the acceleration of the aggregation rate with temperature lies in the favourable entropy gain resulting from the expulsion of more water from the peptide surface. This finding implies that restricting water expulsion while weakening peptide–peptide interaction may lead to the disintegration of the Aβ octamer. To investigate this possibility, we explore the impact of local heating on the Aβ octamer. We term this approach “local heating” since we solely increase the temperature of the peptide segment consisting of residues 25–37 by imparting the necessary kinetic energy while maintaining the rest of the system at an average temperature. We selected this segment due to its crucial role in the aggregation process. Aβ octamer conformations obtained at the end of such simulations shown in Fig. 3C suggest that local heating does not lead to significant disintegration of the preformed aggregate. However, structural modification with increased local heating resembles the global temperature change reported in earlier studies. For instance, we observe a noticeable change in the secondary structure of the Aβ peptides at a temperature of 200 K (see ESI Fig. S3†). However, the peptides retain their β sheet structures at ambient or high temperatures, indicating a shift of equilibrium from a disordered to an ordered state on increasing the effect of local heating.
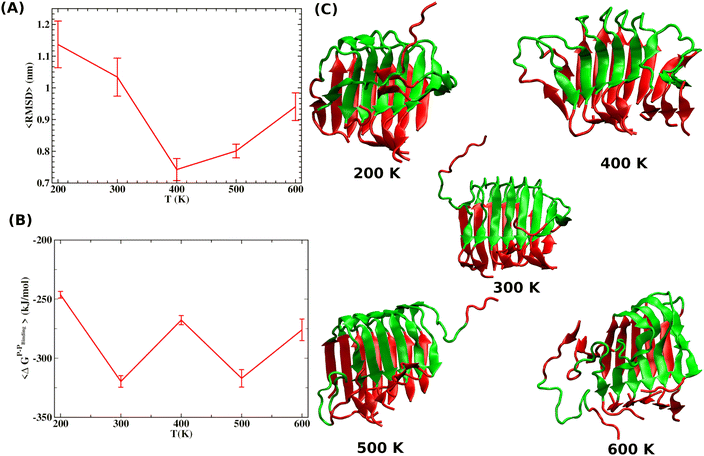 |
| Fig. 3 (A) Time average RMSD of the Aβ octamer upon local heating of the peptide segment as defined in the text. The 〈RMSD〉 values are presented as a function of the T raised in the peptide segment. (B) The average binding free energy (〈ΔGP–Pb〉) of a peptide as obtained from MMPBSA analysis. (C) Configurations for the Aβ octamer obtained at the end of local heating simulations. It clearly shows that the local heating does not lead to significant disintegration of the preformed aggregate. The red color is used to mark the region of the peptide segments consisting of residues 25–37 by imparting the necessary kinetic energy while maintaining the rest of the system at an average temperature. | |
2.1.5 Local heating redistributes peptide thermal energy to the surrounding water, fostering an aggregated state over disruption.
We next investigate why local heating of the crucial Aβ segment promotes an ordered state rather than the expected disordered state. We aim to determine whether the increase in temperature only affects the specified Aβ segment or if it also impacts the surrounding water. To assess this, we calculate the average number of water molecules present within 5 Å of the Aβ octamer that belongs to the essential hydration layer. The data presented in Fig. 4A illustrate a decrease in hydration water on increasing the local heating, indicating depletion of the water layer or, in other words, the expulsion of water from the peptide surface. We further find that the increased water dynamics with rising local heating drives this expulsion, as supported by the shifting of the kinetic energy distribution of the selected water around the specified segment of the Aβ octamer, showing a broadening distribution with increased local heating (Fig. 4B). This finding strongly indicates that the additional energy used to increase the temperature of the peptide segment does not remain confined within the peptide segment but redistributes into the surrounding water molecules. This augmented degree of water expulsion upon increasing local heating contributes to the entropy gain. Such a gain of entropy due to water expulsion is known to drive the aggregation of Aβ peptides as reported in earlier studies.40–44 Hence, this explains why increased local heating resulted in a disordered to ordered transition of the Aβ peptides present in the Aβ octamer.
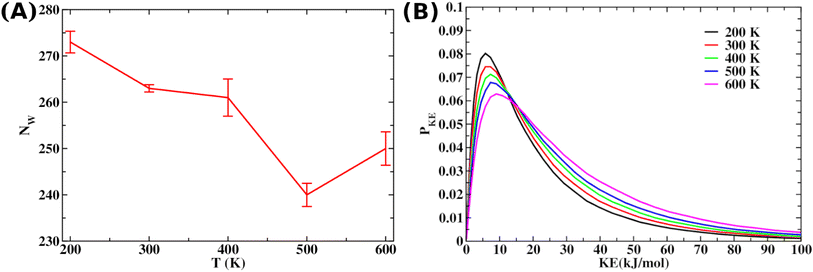 |
| Fig. 4 (A) The average number of water molecules 〈NW〉 present within 5 Å of the Aβ octamer upon local heating. (B) The increased water dynamics with rising local heating drives this expulsion, as supported by the shifting of the kinetic energy distribution of the selected water around the specified segment of the Aβ octamer, showing a broadening distribution with increased local heating as presented in panel B. | |
2.1.6 The defibrillation process encounters an exceptionally high energy barrier.
Subsequently, we investigate the potential of SDS molecules combined with local heating to disintegrate the Aβ octamer. While we have tested a few limited possibilities, the outcome indicates that although the combined effect yields a more significant disintegrating potential than the individual ones, the degree of disintegration is far behind complete disintegration. This observation indicates a considerable energy barrier, preventing complete disruption of the octamer within the simulation time scale. We address the plausible defibrillation/disintegration pathway of the Aβ octamer and the associated barrier through metadynamics simulations, which will be discussed in the following sections.
2.2 Free energy landscape
It is clear from the above discussion that the local heating and the molecular crowders cannot disintegrate the already formed Aβ aggregate due to the exceedingly high energy barrier, restricting the conventional simulation techniques to study a process in detail. To overcome such a problem of conventional MD simulations, we, therefore, investigate the disintegration pathway by performing metadynamics simulations, one of the most commonly used free energy simulation techniques.17 Here we conduct the metadynamics simulations using two collective variables, namely Rg and NCα. The details of the metadynamics simulation are presented in the ESI.†
2.2.1 The transition from the octamer to the pentamer/hexamer emerges as the principal barrier in the defibrillation pathway.
We obtain the free energy landscape from the metadynamics simulations by calculating the free energy as a function of collective variables used for the simulations (see Fig. 5A). The landscape displays several well defined basins of which two important basins correspond to the fully aggregated state (NCα = ∼800 and Rg = ∼1.7 nm) and disaggregated state within the scope of our simulations (NCα = ∼700 and Rg = ∼3.2 nm). Thus, it clearly depicts that the disintegration pathway is a multi-step process since the two above-mentioned basins are enclosed by several well-defined basins. Moreover, there could be multiple possible pathways as any line connecting these two basins potentially represents the disintegration pathway. Nevertheless, all of them are not equally probable. Therefore, we extract the most probable pathway by finding the minimum energy pathway (MEP) using the MEPSA package.45 The so-obtained pathway along with the representative configurations corresponding to the various basins along the pathway are shown in Fig. 5B. It is evident from the figure that the disintegration of the Aβ octamer proceeds through a series of basins, illustrating the complex multi-step pathway. It is crucial to highlight that the observed shift in the energy barrier between different basins, as reflected in this pathway, aligns seamlessly with earlier studies.46,47 This underscores the validity of employing metadynamics simulations and the thoughtful selection of collective variables in our study. We observe that the fully aggregated octamer configuration, denoted as A, must surpass a barrier of ∼45 kJ mol−1 to transform into configuration C, characterized by a smaller aggregate (pentamer/hexamer) with partial disruption. Subsequent disruption of configuration C leads to formation of even smaller aggregates such as monomers or dimers, with a minimal contribution from larger aggregates of a maximum size of 4 (see also ESI Fig. S6†). This continuous disruption process encounters multiple barriers, notably around 20 kJ mol−1, which are approximately two times smaller than the initial barrier during the octamer to pentamer/hexamer transition. Consequently, the octamer to pentamer/hexamer transition emerges as the rate determining step along the defibrillation pathway, constituting the primary defibrillation barrier. The order of magnitude of energy barrier as discussed above is in good agreement with the literature, reaffirming the effectiveness of metadynamics simulations.48 We calculated the probability distribution of the clusters.
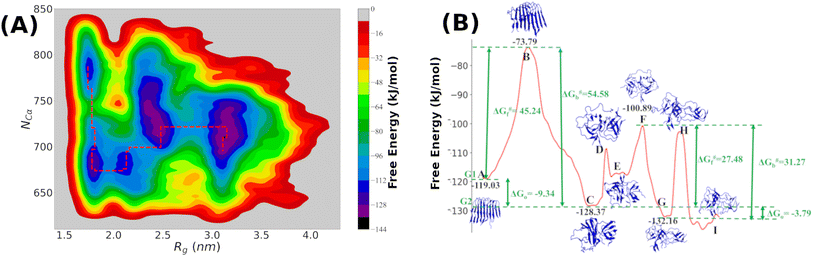 |
| Fig. 5 (A) The contour plot as obtained by projecting the free-energy landscape derived from metadynamics simulation using two collective variables, namely Rg and NCα. The minimum free energy path for the disintegration process is marked by the red lines in the contour plot; (B) one-dimensional view of the minimum energy path and the specific configurations of the Aβ-octamer located on various hills and valleys are shown. The free energy values for each of the hills and valleys are also presented in the figure. | |
2.2.2 Equilibrium between the pentamer/hexamer derived from the octamer and tetramer sets the stage for monomer dispersion.
To obtain a quantitative insight about the defibrillation pathway, we have analyzed the configurations lying on the minimum energy pathway by calculating several properties, such as number of clusters, their population, most probable cluster size, and the secondary structure. The definitions of a cluster and other details are discussed in the ESI.†Fig. 6A and B depict the number of clusters and β-sheet content for the configurations along the pathway, respectively, along with the most probable cluster size and helix content shown in the insets of panels A and B, respectively. The first step in disintegrating the aggregates involves weakening of the octamer. This is associated with reduction in the β-sheet content (from 100% to 60–80%) and number of contacts (800 to 700). Notably, this transition does not result in the complete dissociation of monomers from the aggregate with an occasional appearance of a heptamer with a monomer (no of clusters 2 with a maximum cluster size of 7; see the ESI, Fig. S7†), as indicated by the minimal change of radius of gyration. Following this step, stable pentamer/hexamer configurations form, along with trimers/dimers or a mixture of monomers. This is evidenced by an increase in the radius of gyration and the number of clusters ranging from 3 to 4, with a maximum cluster size of 5 or 6 (see the ESI, Fig. S7†). Subsequently, the so-formed pentamer/hexamer tends to shift to a tetramer (number of clusters ranging from 4 to 5 with a maximum cluster size of 4 as shown in the ESI, Fig. S7†). However, complete transformation into a tetramer is hindered by the frequent reappearance of the hexamer indicated by an increase in the number of contacts and a delicate balance between the β-sheet content and helix content. This process represents a barrier of 20 kJ mol−1, which is two times less than the octamer to pentamer/hexamer transition. Thus, we conclude that there exists an equilibrium between the hexamer and tetramer in this region. Surpassing this barrier results in the formation of dissociated monomers (∼50%) and dimer (∼30%) and tetramer (∼20%). While we did not obtain a completely dispersed monomer with no larger aggregate as the end product, the tetramer configuration obtained at the end is significantly disrupted with a notably low β-sheet content (∼40%). Thus, we propose that this configuration will eventually transform into a completely dispersed monomeric state. Therefore, we suggest octamer → pentamer/hexamer ⇌ tetramer → monomer to be the most probable disintegration pathway of the Aβ octamer.
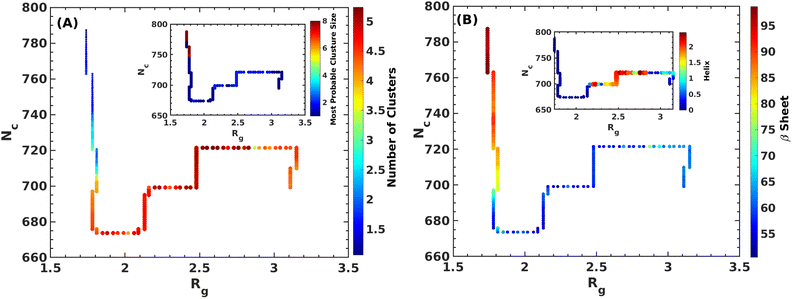 |
| Fig. 6 Various properties of the Aβ-octamer along the MEP. The variation of cluster sizes and the most probable cluster along the MEP are shown in panel (A). In panel (B), we show the variation of secondary structures such as β-sheets and α-helix contents along the MEP. | |
2.3 Mean field model
The complex Aβ aggregation/disintegration process, marked by a sequential structural transition in the free energy landscape obtained from metadynamics simulations has led us to the question: can a simple theoretical model capture the driver for transitioning between free and aggregate states? To address this, we introduce a two-state mean-field model, enabling the determination of critical aggregate size, transition rate, and growth kinetics mechanism. In this minimal model, we consider the peptides as a collection of identical spins whose states are defined by two numbers, −1 and +1, for free and aggregate conditions. We use the average value of the spins as an order parameter to describe this system's degree of aggregation or nucleation kinetics. The evolution of nucleation and growth kinetics can be expressed by representing the order parameter in terms of mole fraction of the aggregate, x | 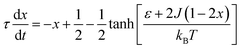 | (1) |
the equilibrium mole fractions (xeq) of the aggregate can be obtained by setting 
| 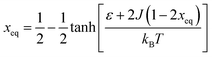 | (2) |
the symbols, ε, J, τ, T, and kB are the energy of an isolated peptide, cooperative strength between the two nearest neighboring peptides, the aggregation time scale, absolute temperature, and Boltzmann's constant, respectively. We obtain the equilibrium points by graphically solving the above non-linear equation. Upon mapping our peptide system with the spin model, we estimate the parameters, ε, J and τ for the first nucleation barrier. The detailed derivation of the model system is presented in the ESI.†
2.3.1 An Ising-based mean field model captures the growth kinetics of Aβ aggregates.
Here, we first extract the parameters from the first barrier of the minimum free energy landscape as shown in panel B of Fig. 5. The estimated barrier heights ΔG† for the forward and backward transitions are 45.24 kJ mol−1 and 54.58 kJ mol−1. The free energy difference between the two states is ΔG0 = −9.34 kJ mol−1. According to our model, the ΔG0 = 2ε estimates the average interaction energy, ε = −4.67 kJ mol−1. We can also obtain the other parameters, such as reorganization energy (λ), which is the energy dissipated on the aggregates' free energy surface if spins change their state from +1 to −1, i.e., aggregate to the free state. Since the primary driving force for forming Aβ aggregates is forming a few lateral hydrogen bonds, we consider the free energy of interaction between peptides and the rest of the system that promotes such hydrogen bonds as the reorganization energy. The explicit form of this energy is given in the ESI.† The obtained λ values for the forward and backward directions are 0.4379 kJ mol−1 and 0.3687 kJ mol−1, respectively. Since τ−1 = τf−1 + τb−1, τf−1 = 1.1957 s, and τb−1 = 1.0929 s the calculated τ value for this transition is 0.5710 s. We performed similar analyses for the other barriers; the results are presented in the ESI (ESI Fig. S4 and S5†).
Fig. 7A shows that the system exhibits bistability where the free and aggregated states correspond with x = 0 and x = 1. We observed a hysteresis loop in our model, as shown in the inset of Fig. 7A. This is a clear signature of phase transitions triggered by the cooperative effect, reflecting the origin of kinetic lag time as often observed in aggregation kinetics. As discussed in the model, the origin of such a cooperative effect can be correlated with the random coupled interaction between peptides and surrounding molecules, which inhibits ordering in the aggregate by loosening lateral hydrogen bond interaction among the β sheet region of the peptides. We find that peptides remain in free states until the value of J reaches a value of 4.8 kJ mol−1, and then sharp transitions occur. The graphical solution of eqn (2) gives us three equilibrium points at xeq = 0.028, 0.152, 0.996 at J = 4.8 kJ mol−1 and ε = −4.67 kJ mol−1. Two of them, 0.028 and 0.996, are stable, corresponding to aggregate and free states, respectively. The unstable solution corresponds to the critical nucleus size and the number is xeq = 0.152, corresponding to the pentamer as a nucleation point for the growth kinetics of Aβ peptides. We obtain a similar number by counting the numbers of the Aβ peptides at the first barrier of the minimum energy path. Therefore, we find a close resemblance between the results obtained from our metadynamics simulation and the proposed mean field model. Therefore, our extracted parameter from the MD simulation analysis provides a quantitative picture behind the Aβ peptide aggregation mechanism.
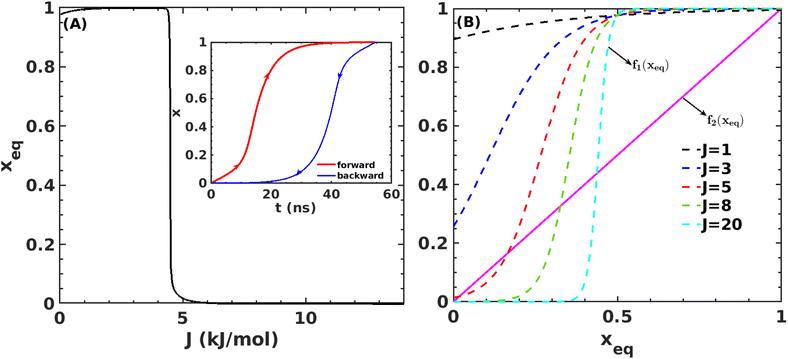 |
| Fig. 7 (A) Variation in xeq with coupling parameter, J. Inset figure represents the formation of a hysteresis loop during the forward process, i.e., aggregate to the free state, and backward process, i.e., free state to aggregated state. (B) Graphical solution of eqn (2) for the first barrier where and f2(xeq) = xeq. The dashed line represents the function f1 at different J values while the solid line represents the f2. | |
2.3.2 Environmental effects alter the growth kinetics of Aβ aggregates.
Furthermore, we consider the effect of the environment that modifies the interactions among peptides. We include those effects through the linear modifications of ε. For example, we assume the effect of temperature and the crowder through the following linear modifications of ε as
ε = ε0 + ΔεT(T − T0) (temperature effect) |
ε = ε0 + ΔεCCc (Crowder effect) |
where ε0 is the unperturbed internal interactions; T and Cc are the temperature and the concentrations of the crowders; and Δε is the gradient of energy. The above model provides a comprehensive view of the nucleation-growth aggregation kinetics and the critical size of a nucleate as a function of cooperative interaction J, T and Crowder's concentration, Cc.
We use gradient Δε = −0.01 kJ mol−1 K−1 and 0.002 kJ mol−1 into our mean-field model to observe the effect of temperature and crowder effects. We set ε = −4.67 kJ mol−1 and J = 4.8 kJ mol−1 throughout our calculation to explore their effects. We chose them because the peptides stay in the free form below those parameter values. Fig. 8A shows the effect of temperature on the kinetics of the aggregated states. It shows that at lower temperatures, T = 200 K, peptides remain in the aggregated form for extended periods. However, the aggregated peptides undergo a transition to form free monomeric states with the increase in temperature, a signature we observe in our MD simulation study. Fig. 8B represents the plot of mole fraction peptide in a free state with increased concentration of SDS surfactant molecules. It suggests that as the concentration of crowders increases, aggregates are stabilized. Therefore, our proposed model captures the nucleation and growth kinetics of Aβ peptide in the presence of heat and crowder from our theoretical analysis.
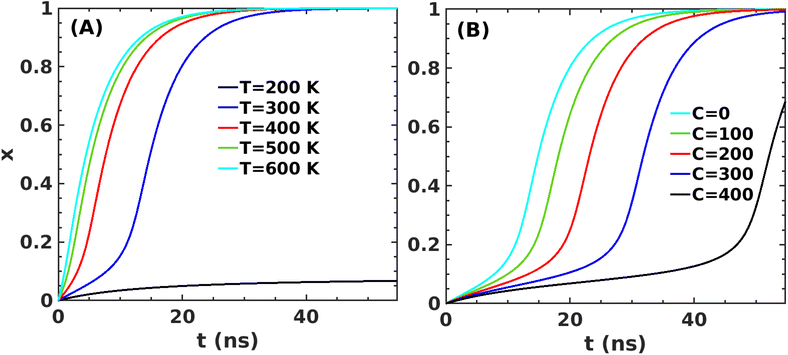 |
| Fig. 8 Variation in x for the first barrier from state A to state C (in Fig. 5B) as a function of time for J = 4.8 kJ mol−1 and ε0 = −4.67 kJ mol−1 (A) at several temperatures and (B) at several concentrations of the crowder. | |
3 Conclusions
Amyloid β (Aβ) peptide self-assembles into larger aggregates, playing a pivotal role in Alzheimer's disease (AD). These aggregates, found in an AD patient's brain, are potentially toxic and can cause serious damage to the brain. Hence, identifying a strategy to prevent the aggregation of Aβ peptide and concurrently promoting the clearance of existing Aβ aggregates are two essential goals in AD research. Successfully achieving these goals involves addressing two key questions: (i) elucidating the underlying molecular details of the growth process of Aβ aggregates and (ii) devising ways to disassemble existing Aβ aggregates or, at the very least, impede their growth process.
In this study, we used a combination of state-of-the-art molecular simulations and a theoretical model to answer the two above-mentioned questions. Our investigation especially delved into the efficacy of disintegrating the Aβ octamer, a crucial nucleus that could subsequently aggregate to form even larger aggregates.49,50 We employed detailed conventional molecular dynamics simulations to assess the impact of both local heating and the molecular crowder sodium dodecyl sulfate (SDS) on this process. Employing well-tempered metadynamics simulations, we further explored the disintegration pathway of Aβ octamer and the associated barrier. Finally, we introduced a novel two-state mean-field model based on Ising spins, offering an explanation on the growth kinetics and the prediction of the critical nucleus size on considering the cooperative effect of Aβ monomer association.
We elevated the temperature of crucial Aβ segments (25 to 37 residues) by appropriately scaling the kinetic energy to explore the influence of local heating, while the concentration of the SDS was varied to examine the crowding effect. Results revealed that Aβ aggregates resist complete disintegration during local heating despite initiation of disruption. We found that rapid distribution of thermal energy from the peptide segments to the surrounding water impedes complete breakdown of the aggregates. Conversely, although SDS molecules effectively inhibit Aβ aggregation by inducing destabilization, they fail to completely disintegrate the aggregate due to an exceedingly high energy barrier.
We addressed the exceedingly high energy barrier for the defibrillation process observed in the conventional simulations by employing metadynamics simulations. Exploration of the free energy landscape constructed from such metadynamics simulations enabled us to deduce the disintegration pathway of the Aβ octamer. Results indicated a primary energy barrier of 45 kJ mol−1, followed by several barriers of approximately half the primary barrier. Cluster analysis and structural characterization of the clusters showed that the octamer to pentamer/hexamer transition constitutes the rate-determining step with a barrier of 45 kJ mol−1, followed by several shallow barriers of 20 kJ mol−1, resulting in the equilibrium between the tetramer and hexamer. Such a tetramer–hexamer equilibrium sets the ultimate fate of the aggregate into the dispersed monomeric state with a negligible population of the Aβ octamer. We thus conjecture that octamer → pentamer/hexamer ⇌ tetramer → monomer is the most probable disintegration pathway of the Aβ octamer.
Finally, we incorporated the free energy data (free energy barrier and the free energy values) into our proposed two-state mean-field model based on Ising spins. This simple yet potent model not only allowed us to capture the kinetics of the growth process but also offered an explanation for the influence of local heating and crowder on the disintegration process of the Aβ aggregates. Importantly, incorporation of the free energy data corresponding to the primary barrier into the model revealed a pentamer to be the critical size. The observed consistency of the pentamer as the critical size, as deduced from metadynamics simulations, underscores the effectiveness of our proposed mean-field model. Thus, the current simulation study, coupled with the newly introduced mean-field model, offers an insight into the detailed mechanisms underlying the Aβ aggregation process.
Data availability
Yes, we have provided all the required data related to this paper in the ESI.†
Author contributions
S. K. S., N. K., and P. K. designed the research, performed the research, and wrote the paper. N. K. analyzed the data.
Conflicts of interest
There are no conflicts to declare.
Acknowledgements
S. K. S. is supported by SERB, the Department of Science and Technology, and the Government of India (CRG/2022/000345).
Notes and references
- J. Hardy, Has the amyloid cascade hypothesis for Alzheimer’s disease been proved?, Curr. Alzheimer Res., 2006, 3, 71–73 CrossRef CAS PubMed.
- P. H. Nguyen, A. Ramamoorthy, B. R. Sahoo, J. Zheng, P. Faller, J. E. Straub, L. Dominguez, J.-E. Shea, N. V. Dokholyan and A. De Simone,
et al.
, Chem. Rev., 2021, 121, 2545–2647 CrossRef CAS PubMed.
- D. J. Selkoe, Physiol. Rev., 2001, 81, 740–766 CrossRef PubMed.
- M. A. Findeis, Pharmacol. Ther., 2007, 116, 266–286 CrossRef CAS PubMed.
- J. T. Jerrett and P. T. Lansbury, Cell, 1993, 73, 1055–1058 CrossRef PubMed.
- D. Chakraborty, J. E. Straub and D. Thirumalai, Proc. Natl. Acad. Sci. U. S. A., 2020, 117, 19926–19937 CrossRef CAS PubMed.
- X. Han and H. Gefei, ACS Chem. Neurosci., 2018, 9, 198–210 CrossRef CAS PubMed.
- L. Triguero, R. Singh and R. Prabhakar, J. Phys. Chem. B, 2008, 112, 2159–2167 CrossRef CAS PubMed.
- A. D. Williams, E. Portelius, I. Kheterpal, J.-t. Guo, K. D. Cook, Y. Xu and R. Wetzel, J. Mol. Biol., 2004, 335, 833–842 CrossRef CAS PubMed.
- W. M. Berhanu and U. H. Hansmann, PLoS One, 2012, 7, e41479 CrossRef CAS PubMed.
- A. Abelein, J. P. Abrahams, J. Danielsson, A. Gräslund, J. Jarvet, J. Luo, A. Tiiman and S. K. Wärmländer, JBIC, J. Biol. Inorg. Chem., 2014, 19, 623–634 CrossRef CAS PubMed.
- S. G. Itoh and H. Okumura, J. Phys. Chem. B, 2014, 118, 11428–11436 CrossRef CAS PubMed.
- S. Maity, M. Hashemi and Y. L. Lyubchenko, Sci. Rep., 2017, 7, 2344 CrossRef PubMed.
- S. G. Itoh, M. Yagi-Utsumi, K. Kato and H. Okumura, ACS Chem. Neurosci., 2022, 13, 3139–3151 CrossRef CAS PubMed.
- M. Khaled, I. Ronnback, L. L. Ilag, A. Graslund, B. Strodel and N. Osterlund, J. Am. Chem. Soc., 2023, 145, 18340–18354 CrossRef CAS PubMed.
- S. Giorgetti, C. Greco, P. Tortora and F. A. Aprile, Int. J. Mol. Sci., 2018, 19, 2677 CrossRef PubMed.
- J. A. Lemkul and D. R. Bevan, Biochemistry, 2010, 49, 3935–3946 CrossRef CAS PubMed.
- Y. Qiao, M. Zhang, J. Zheng and G. Liang,
et al.
, Phys. Chem. Chem. Phys., 2017, 19, 155–166 RSC.
- J. Lu, Q. Cao, C. Wang, J. Zheng, F. Luo, J. Xie, Y. Li, X. Ma, L. He and D. Eisenberg,
et al.
, Front. Mol. Neurosci., 2019, 12, 54 CrossRef CAS PubMed.
- P. Velander, L. Wu, F. Henderson, S. Zhang, D. R. Bevan and B. Xu, Biochem. Pharmacol., 2017, 139, 40–55 CrossRef CAS PubMed.
- S. Kalita, H. Bergman, K. D. Dubey and S. Shaik, J. Am. Chem. Soc., 2023, 145(6), 3543–3553 CrossRef CAS PubMed.
- F. S. Ruggeri, J. Adamcik, J. S. Jeong, H. A. Lashuel, R. Mezzenga and G. Dietler, Angew. Chem., 2015, 127, 2492–2496 CrossRef.
- B. Caughey and P. T. Lansbury Jr, Annu. Rev. Neurosci., 2003, 26, 267–298 CrossRef CAS PubMed.
- J. T. Jarrett and P. T. Lansbury Jr, Cell, 1993, 73, 1055–1058 CrossRef CAS PubMed.
- J. Meinhardt, G. G. Tartaglia, A. Pawar, T. Christopeit, P. Hortschansky, V. Schroeckh, C. M. Dobson, M. Vendruscolo and M. Fändrich, Protein Sci., 2007, 16, 1214–1222 CrossRef CAS PubMed.
- A. K. Paravastu, I. Qahwash, R. D. Leapman, S. C. Meredith and R. Tycko, Proc. Natl. Acad. Sci. U. S. A., 2009, 106, 7443–7448 CrossRef CAS PubMed.
- B. Strodel, Curr. Opin. Struct. Biol., 2021, 67, 145–152 CrossRef CAS PubMed.
- H. Okumura, J. Phys. Chem. B, 2023, 127, 10931–10940 CrossRef CAS PubMed.
- H. Okumura and S. G. Itoh, J. Am. Chem. Soc., 2014, 136, 10549–10552 CrossRef CAS PubMed.
- M. Hoang Viet, P. Derreumaux and P. H. Nguyen, J. Chem. Phys., 2016, 145, 174113 CrossRef PubMed.
- M. Hoang Viet, P. Derreumaux, M. S. Li, C. Roland, C. Sagui and P. H. Nguyen, J. Chem. Phys., 2015, 143, 155101 CrossRef PubMed.
- H. Okumura, S. G. Itoh, K. Nakamura and T. Kawasaki, J. Phys. Chem. B, 2021, 125, 4964–4976 CrossRef CAS PubMed.
- H. Okumura, S. G. Itoh, H. Zen and K. Nakamura, PLoS One, 2023, 18, e0291093 CrossRef CAS PubMed.
- A. B. Poma, T. T. M. Thu, L. T. M. Tri, H. L. Nguyen and M. S. Li, J. Phys. Chem. B, 2021, 125, 7628–7637 CrossRef CAS PubMed.
- A. Levin, T. A. Hakala, L. Schnaider, G. J. Bernardes, E. Gazit and T. P. Knowles, Nat. Rev. Chem, 2020, 4, 615–634 CrossRef CAS.
- G. Wei, Z. Su, N. P. Reynolds, P. Arosio, I. W. Hamley, E. Gazit and R. Mezzenga, Chem. Soc. Rev., 2017, 46, 4661–4708 RSC.
- T. Bera, P. C. Saha, T. Chatterjee, S. Kar and S. Guha, Bioconjugate Chem., 2022, 33, 1201–1209 CrossRef CAS PubMed.
- S. Menon and N. Sengupta, Biochim. Biophys. Acta, Proteins Proteomics, 2019, 1867, 941–953 CrossRef CAS PubMed.
- D. C. Latshaw, M. Cheon and C. K. Hall, J. Phys. Chem. B, 2014, 18, 13513–13526 CrossRef PubMed.
- J. D. Camino, P. Gracia and N. Cremades, Biophys. Chem., 2021, 269, 106520 CrossRef CAS PubMed.
- P. Khatua, J. C. Jose, N. Sengupta and S. Bandyopadhyay, Phys. Chem. Chem. Phys., 2016, 18, 30144–30159 RSC.
- J. D. Camino, P. Gracia and N. Cremades, Biophys. Chem., 2021, 269, 106520 CrossRef CAS PubMed.
- D. Thirumalai, G. Reddy and J. E. Straub, Acc. Chem. Res., 2012, 45, 83–92 CrossRef CAS PubMed.
- S.-H. Chong and S. Ham, Acc. Chem. Res., 2015, 48, 956–965 CrossRef CAS PubMed.
- I. Marcos-Alcalde, J. Setoain, J. I. Mendieta-Moreno, J. Mendieta and P. Gomez-Puertas, Bioinformatics, 2015, 31, 3853–3855 CrossRef CAS PubMed.
- A. Mukherjee and B. Bagchi, J. Chem. Phys., 2004, 120, 1602–1612 CrossRef CAS PubMed.
- T. Pal, S. Sahoo, K. P. Ghanta and S. Bandyopadhyay, J. Mol. Liq., 2022, 368, 120779 CrossRef CAS.
- R. Nelson, M. R. Sawaya, M. Balbirnie, A. Ø. Madsen, C. Riekel, R. Grothe and D. Eisenberg, Nature, 2005, 435, 773–778 CrossRef CAS PubMed.
- G. Bitan, M. D. Kirkitadze, A. Lomakin, S. S. Vollers, G. B. Benedek and D. B. Teplow, Proc. Natl. Acad. Sci. U. S. A., 2003, 100, 330–335 CrossRef CAS PubMed.
- C.-T. Lee and E. M. Terentjev, J. Chem. Phys., 2017, 147, 105103 CrossRef PubMed.
|
This journal is © The Royal Society of Chemistry 2024 |
Click here to see how this site uses Cookies. View our privacy policy here.