DOI:
10.1039/D3SC05735F
(Edge Article)
Chem. Sci., 2024,
15, 4770-4778
Non-invasive single cell aptasensing in live cells and animals†
Received
27th October 2023
, Accepted 18th February 2024
First published on 19th February 2024
Abstract
We report a genetically encoded aptamer biosensor platform for non-invasive measurement of drug distribution in cells and animals. We combined the high specificity of aptamer molecular recognition with the easy-to-detect properties of fluorescent proteins. We generated six encoded aptasensors, showcasing the platform versatility. The biosensors display high sensitivity and specificity for detecting their specific drug target over related analogs. We show dose dependent response of biosensor performance reaching saturating drug uptake levels in individual live cells. We designed our platform for integration into animal genomes; thus, we incorporated aptamer biosensors into zebrafish, an important model vertebrate. The biosensors enabled non-invasive drug biodistribution imaging in whole animals across different timepoints. To our knowledge, this is the first example of an aptamer biosensor-expressing transgenic vertebrate that is carried through generations. As such, our encoded platform addresses the need for non-invasive whole animal biosensing ideal for pharmacokinetic-pharmacodynamic analyses that can be expanded to other organisms and to detect diverse molecules of interest.
Introduction
Technologies that enable detection of drugs and their metabolites inside individual cells improve drug delivery and optimization, enhancing therapeutic outcomes, and minimizing adverse effects.1,2 Typically, instrument-based analytical methods3–6 are used to measure drugs and their metabolites, but require cell lysis7,8 or embedding and freezing tissues. This precludes real-time cellular measurements9 and non-invasive animal studies,10 thereby limiting studies to bulk cellular populations.11,12 Microscopy, on the other hand, is valuable for non-invasive and real-time monitoring of biomolecules,13 cellular processes,14,15 and tissue patterning.16,17 However, sensing small molecules including drugs and their metabolites remains a challenge due to the lack of probes with specific molecular recognition properties.
The use of fluorescent biosensors, incorporating small molecule probes or genetically encoded fluorescent proteins18–23 has improved real-time detection of molecules inside cells. Indeed, a long list of genetically encoded biosensors18 making use of fluorescent protein approaches, fluorescence resonance energy transfer (FRET),24 or bioluminescence resonance energy transfer (BRET),25 have been developed to sense metal ions,26,27 redox species,28 and essential small molecules.29–32 Recently, a FRET-based encoded biosensor was developed to sense the uptake of sulphonamide diuretic drugs,19 highlighting the utility of monitoring drug uptake in live cells and the promise of genetic biosensors. However, the complexity of modifying promoters and proteins to detect different targets is challenging, preventing their broad application for monitoring a wide range of small molecule drugs.33 Additionally, protein biosensors are often specific to a species, making them unsuitable for use in diverse cell and animal models.34
Aptamers offer versatile solutions for biosensing,35–37 enabling the identification of various small molecules including drugs,38 toxicants,39 toxins,40 metabolites,41 and even ions.42 Aptamers are single-stranded nucleic acids that selectively bind to their targets identified through in vitro selection.43–45 Features that render aptamers preferable to other selective molecules such as antibodies and promoters are their versatility, reproducibility, and programmability.46,47 Indeed, aptamer-coupled nanodevices for tumor imaging can be injected into animals where they accumulate at tumors sites.48 Light-up aptamers have been encoded into cells enabling live-cell imaging of RNA.49,50 However, non-invasive biosensing in single cells and live animals has never been achieved with aptamers.
Here, we developed a new platform to genetically-encode ribozyme-coupled aptamers into mammalian cells at the single-cell level and whole animals (Scheme 1). Aptamers have been coupled to the self-cleaving activity of ribozyme, creating “switches” that control gene expression in yeast and mammalian cells.51–54 RNA aptamers are inserted into the loops of a self-cleaving ribozyme,55–58 such that ribozyme activity is maintained. In this way, when the aptamer-ribozyme construct is encoded in the 3′-UTR of a gene, the mRNA is cleaved and degraded. However, when the aptamer's target binds, it blocks the tertiary interactions necessary for ribozyme activity. As a result, the mRNA is stabilized by the polyA tail and is effectively translated into the fluorescent protein.
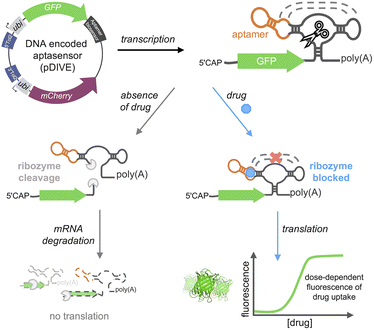 |
| Scheme 1 DIVE platform. The DNA Integrated Versatile Encoded-aptamer biosensor plasmid (pDIVE) is transcribed producing the biosensor composed of an RNA aptamer fused to a ribozyme. In the absence of the drug, the ribozyme's self-cleavage activity facilitates mRNA degradation, resulting in no GFP translation. When the aptamer binds the drug, ribozyme tertiary interactions are blocked thus enabling GFP synthesis in a dose-dependent fashion. | |
We hypothesized that we could leverage aptamer-ribozyme constructs for intracellular biosensing, where any aptamer could theoretically be used to specifically recognize a target-of-interest and a dose-response signal could be generated via a green fluorescent protein (GFP). We therefore generated the first transgenic vertebrate harboring an encoded aptamer biosensor, enabling sensitive and long-term studies of drug cellular uptake throughout the lifespan of a vertebrate. We applied our platform to generate six different aptamer biosensors demonstrating the generalizability of the approach. Furthermore, our biosensors specifically detect drugs of interest with saturable dose-response curves in live cells. In summary, we describe the first aptamer biosensor platform that allows non-invasive and single cell measurements of drug distribution in live cells and whole animals.
Results and discussion
A platform for encoding aptamer biosensors into cells and zebrafish
We developed a novel platform for encoding aptamer ribozyme constructs into the zebrafish genome termed “DNA Integrated Versatile Encoded-Aptamer biosensors” (DIVE aptasensors, Scheme 1). We developed a custom DNA plasmid (pDIVE, Fig. S1 and Table S1†) for both zebrafish and mammalian cells whereby upon transcription, multiple copies of the aptasensors are transcribed within each cell serving as a highly sensitive biosensor. GFP acts as the transducer coupled to the aptamer sensor platform; and mCherry acts as an internal positive control for expression and successful DIVE delivery. GFP is normalized to mCherry, accounting for cell death/toxicity, varying concentrations of the transfected biosensor platform, cell–cell variations in gene expression, and whether the plasmid integrated into embryos.59 Everything is controlled by the ubiquitin (ubi) promoter60 that is active throughout zebrafish development and in mammalian cells, enabling constitutive expression and ratiometric analyses in live cells and animals.59,61 Fluorescent proteins were incorporated without codon optimization to minimize differences between mammalian cells and zebrafish providing a single platform for use in both model systems. As such, our DIVE platform can be transfected or injected into cells or integrated into the genome of cells and zebrafish.
We first established and tested negative and positive controls for our DIVE biosensing platform. We cloned controls lacking the aptamer sensing modules, producing an “always OFF” biosensor (DIVE.OFF, negative control) composed of an active self-cleaving ribozyme, and an “always ON” biosensor (DIVE.ON, positive control) composed of an altered ribozyme that lacked self-cleavage activity and therefore did not interfere with GFP expression. We transfected these controls into HEK293T cells and injected them into zebrafish embryos. As expected, DIVE.OFF resulted in very low GFP fluorescence whereas DIVE.ON resulted in high GFP fluorescence, resulting in GFP/mCherry ratios of 0.5 and 3.4, respectively (Fig. 1 and S2†). Notably, DIVE.ON gave the same measured fluorescent ratios as the core plasmid pDIVE containing only GFP and mCherry (ratio = 3.6 in HEK293T cells and 0.8 in zebrafish embryos). Furthermore, DIVE.OFF resulted in higher GFP signal compared to the background fluorescence measured in the cells and embryos (Fig. 1). These controls established the upper and lower biosensing dynamic range and confirmed function in zebrafish.
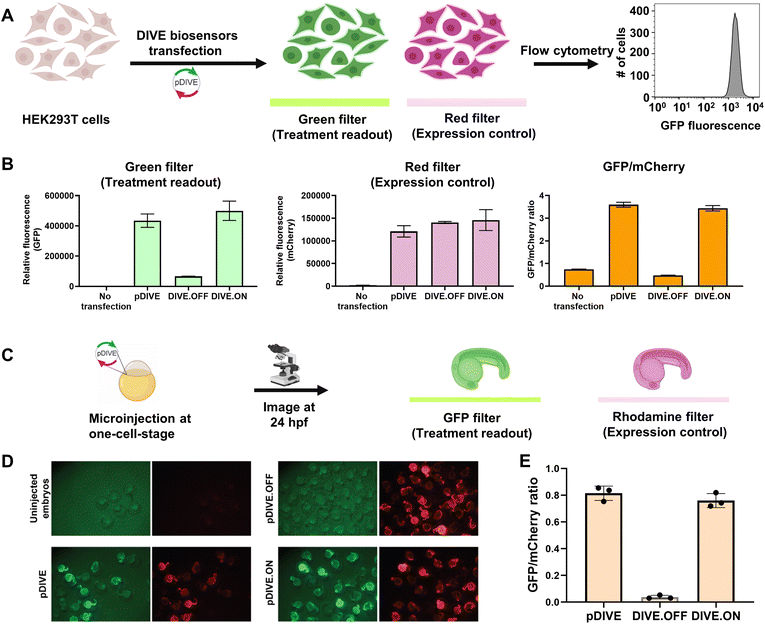 |
| Fig. 1 DIVE platform controls in HEK293T cells and zebrafish embryos. (A) Workflow of the cell assay. (B) Fluorescence measurements for each control: no transfection, the core plasmid pDIVE containing only GFP and mCherry, the DIVE.OFF negative control, and DIVE.ON positive control. GFP and mCherry fluorescence is measured for each cell population. The relative GFP/mCherry ratio is calculated and plotted. Data are the mean and standard deviation of three independent experiments each with technical triplicates. (C) The workflow for testing activity of the DIVE system in zebrafish embryos. WT embryos were injected at the one-cell stage and raised until 1 day post fertilization (dpf). (D) Epifluorescence micrographs from an entire clutch are shown for embryos. (E) Three random embryos from each injection condition were imaged and pixel intensity across the trunk quantified. Embryos lacking the mCherry expression control are not included as this indicates either they were not integrated into the genome or in a region with silent expression. The average GFP/mCherry ratio ± s.d. was plotted. Values for individual fish are shown. | |
DIVE aptasensors for six pharmaceutical drugs
With the appropriate controls in hand, we next compared biosensor activity for six small molecule pharmaceuticals with diverse structures (Fig. S3†) and functions. Theophylline is a bronchodilator used for respiratory disorders; folinic acid is a folate derivative used for decreasing the toxicity of anticancer drugs in healthy cells; gardiquimod is an experimental toll-like receptor (TLR) agonist that modulates the immune system; aciclovir is an antiviral medication and nucleoside analog; and neomycin and tetracyclines are antibiotics. Importantly, protein-based encoded biosensors have not been reported for these molecules with the exception for tetracycline and thus our developed DIVE biosensors dramatically increase the number of drugs that can be monitored within cells.
Aptamer-ribozyme constructs51–53,62 were screened for each of our six drugs and the best candidates were incorporated into our pDIVE generating six individual DIVE aptasensors followed by transfection into HEK293T cells (Table S2†). Cells were then incubated with each drug to compare DIVE aptasensor activity following drug uptake via flow cytometry (Fig. S4†). All six DIVE_sensors resulted in an increase in the GFP/mCherry ratio in the presence of the appropriate target drug, confirming biosensing function of the genetically encoded platform in mammalian cells (Fig. 2). Furthermore, our single-cell measurements by flow cytometry were consistent with bulk measurements (Fig. S5†). The DIVE_6 sensor showed the highest response, with a 3.9-fold increase in fluorescence compared to in the absence of the drug. DIVE_3, DIVE_1, and DIVE_2 resulted in up to a 1.9-fold increase in fluorescence as compared to the absence of drug. Importantly, except for the theophylline aptamer, each of the aptamers employed in the biosensors bind to their drug targets with nanomolar dissociation constants under physiological magnesium concentrations (0.5 mM)52 (Fig. S6†). In contrast, the theophylline aptamer, which resulted in the best response (DIVE_6), displays a substantially weaker micromolar binding (KD = 5 ± 2 μM) at 0.5 mM Mg2+ measured using a surface plasmon resonance assay (Fig. S6†).
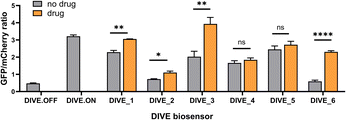 |
| Fig. 2 Comparison of DIVE aptasensors for six drugs. GFP/mCherry ratio for each DIVE aptasensor in HEK293T cells in the presence and absence of its corresponding drug: DIVE_1 with 100 μM aciclovir; DIVE_2 with 1 mM folinic acid; DIVE_3 with 50 μM gardiquimod; DIVE_4 with 2 mM neomycin; DIVE_5 with 500 μM tetracycline; and DIVE_6 with 1 mM theophylline. Data are the mean and standard deviation of three independent experiments each with technical replicates. p values from a two-tailed, unpaired t-test with Welch's correction. Significance summary: p > 0.05 (ns), p ≤ 0.05 (*), p ≤ 0.01 (**), p ≤ 0.0001 (****). | |
Therefore, the difference in responses measured by our DIVE biosensors is not due to aptamer affinity but rather reflects drug uptake into cells. Indeed, it is known that aminoglycosides and other antibiotics have poor cellular uptake into mammalian cells,63 consistent with the low “turn-on” fluorescence measured by DIVE_4 and DIVE_5. Furthermore, folinic acid is a negative charged molecule that requires specific membrane transporters for its transport in and out of the cell. HEK293T cells express these transporters64 but tightly regulate the uptake, corresponding to the small but statistically significant measured DIVE_2 response. Indeed, previous reports demonstrate improved uptake of folinic acid upon overexpressing the human folate transporter SLC46A1.52 Taken together, our results demonstrate that the DIVE aptasensors enable single cell measurement of drug uptake.
DIVE aptasensors show a dose response relationship and distinguish their target drugs from analogs
We next performed a dose-response study using the sensors with the highest response, DIVE_6 and DIVE_3. As the nominal drug concentration was increased, the relative GFP also increased. Data were fit to a saturating dose curve, resulting in half maximal effective concentrations (EC50) of 257 μM (R2 = 0.9928) for DIVE_6 with theophylline and 1.5 μM (R2 = 0.9255) for DIVE_3 with gardiquimod (Fig. 3). Data were also fit using a simple linear regression establishing a linear dynamic range for DIVE_6 between 8 and 320 μM and a limit of detection (LOD) of 7.5 μM. For DIVE_3 the linear dynamic range, based on the nominal dose, extended to 3 μM; however, due to the high background expression of this biosensor, the LOD was approximately 1 μM (Fig. S7†). These results confirm that our DIVE platform enabled the detection of drug uptake into cells in a dose-dependent manner. Dose-response studies are essential in drug development, providing critical information regarding safety and dosing;65 therefore, our biosensors may be useful for monitoring drug uptake in engineered cells or drugs encapsulated into delivery vehicles.
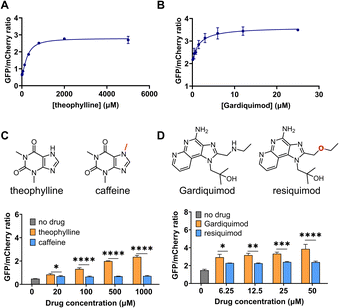 |
| Fig. 3 DIVE aptasensor performance: dose response and specificity. GFP/mCherry ratios after incubating HEK293T cells with increasing concentrations of their corresponding drugs (A) DIVE_6. (B) DIVE_3. (C) DIVE_6 specificity in the presence of theophylline and caffeine. (D) DIVE_3 specificity in the presence of gardiquimod and resiquimod. Data are the mean and standard deviation of three independent biological experiments. p values from a 2-way ANOVA with Šídák's multiple comparisons test indicated. Significance summary: p ≤ 0.05 (*), p ≤ 0.01 (**), p ≤ 0.001 (***), p ≤ 0.0001 (****). | |
Specificity is critical in drug development to avoid negative side effects and toxicity.66 We therefore wanted to ensure that the aptamers maintained their specificity for their targets within the DIVE biosensing platform. We compared biosensor activity in response to analogs of their target drugs. For the DIVE_6 biosensor we incubated cells separately with high concentrations of caffeine which differs from theophylline by a single methyl group. Importantly, the DIVE_6 biosensor showed almost no activity in the presence of caffeine. Even at 1 mM concentrations, the biosensor activity with caffeine was comparable to background levels, highlighting its specificity (Fig. 3C). For the DIVE_3 biosensor, we examined the specificity against the analog resiquimod in which an ether replaces the secondary amine in gardiquimod. Again, our DIVE aptasensor showed almost no activity in the presence of resiquimod, illustrating a high degree of specificity (Fig. 3D).
Live-cell imaging of biosensor activity in HEK293T cells
We next determined whether our DIVE aptasensors would be suitable for live-cell imaging. We therefore evaluated the theophylline biosensor in live HEK293T cells. Cells containing DIVE_6 were treated with various concentrations of theophylline and caffeine. Without any fixing or mounting, we directly imaged the cells in the well plates for both green and red fluorescence. As expected, the red filter showed red fluorescence in all cell conditions since mCherry is constitutively expressed. The positive control DIVE.ON displayed bright green fluorescence, whereas the negative control DIVE.OFF showed no green fluorescence (Fig. 4A). Cells harboring DIVE_6 treated with 0.5 and 1 mM theophylline displayed bright green signals (Fig. 4B) and almost no signals were observed in the absence of theophylline or those treated with caffeine (Fig. 4C and S8†). Our live-cell imaging results are comparable to the flow cytometry measurements, thus confirming the robustness of our aptamer biosensors for non-invasive live-cell imaging of drug uptake.
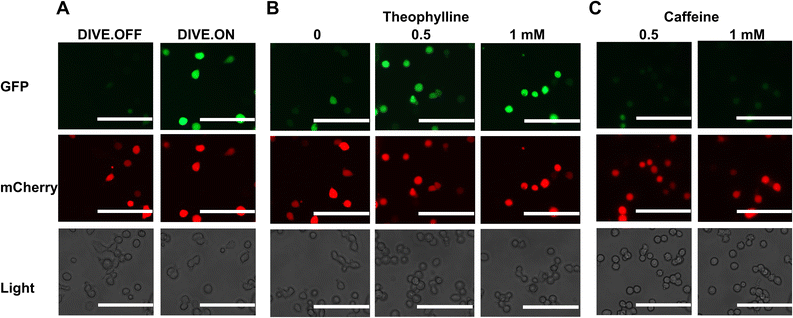 |
| Fig. 4 Live-cell imaging of drug uptake using DIVE aptasensors. (A) HEK293T cells expressing DIVE.OFF and DIVE.ON controls. (B) HEK293T cells expressing DIVE_6 and treated with theophylline. (C) HEK293T cells expressing the DIVE_6 biosensor treated with caffeine. Scale bars: 100 μm. Results are from one experiment; two additional independent experiments were performed (Fig. S8†). | |
Non-invasive cellular biosensing of theophylline in a vertebrate model
Since our aptamer-based biosensors enabled live-cell detection of drug uptake in mammalian cells, we sought to assess their biosensing capabilities in a whole vertebrate animal model. Zebrafish have emerged as a powerful preclinical model for human disease and respond to small molecules and drug treatments at physiologically relevant doses.67 We therefore examined the function of DIVE_6 in zebrafish. The biosensors and controls were integrated into the zebrafish genome using Tol2-mediated transgenesis.68,69 Selected fish expressing the expression control mCherry were raised to adulthood, propagated through F1 and F2 generations. Resulting positive embryos from an F2 outcross expressing the biosensors were used for experiments (Fig. 5A). We performed all experiments using 1 mM theophylline since zebrafish exhibited toxicity effects at higher drug concentrations (Fig. S9†), consistent with theophylline dosing studies in patients.70
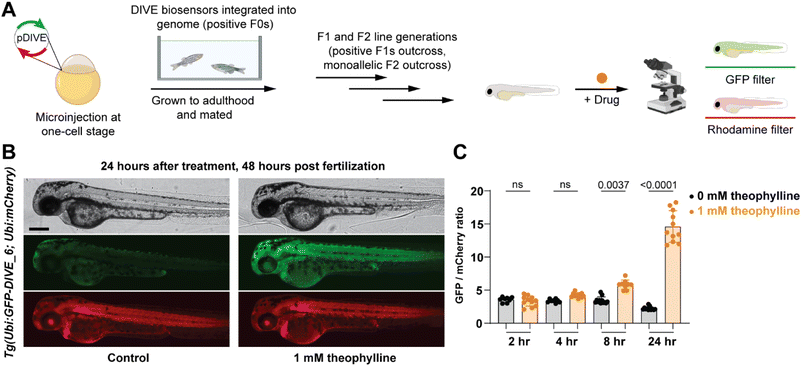 |
| Fig. 5 Non-invasive imaging of drug uptake in new transgenic DIVE expressing zebrafish line. (A) Workflow for generating DIVE aptasensor-expressing transgenic zebrafish Tg(Ubi:GFP-DIVE_6; Ubi:mCherry). Embryos from an F2 outcross were bathed in 1 mM theophylline beginning at 24 hpf. (B) Representative brightfield and fluorescent micrographs from 48 hpf embryos containing the integrated biosensor plasmid (Ubi:GFP-DIVE_6; Ubi:mCherry). Zebrafish orientation is lateral view, anterior left. Scale bar: 250 μm. (C) Quantification of pixel intensity of GFP and mCherry was calculated for the trunk of the zebrafish and the GFP/mCherry ratio plotted. The average GFP/mCherry ratio ± s.d. with values for individual fish shown. p values from a Kruskal–Wallis analysis of variance with post-hoc Dunn's test. | |
Transgenic embryos at 24 hours post fertilization (hpf) expressing DIVE_6 were bathed in media in the absence and presence of theophylline. Imaging of individual embryos revealed a small observable green fluorescence in the treated fish after as little as four hours, with a marked increase in green fluorescence after 24 hours (Fig. 5B). In comparison, there was no change in green fluorescence in the fish that were not bathed in theophylline. In contrast, the DIVE.ON positive control was not impacted by theophylline treatment (Fig. S10†), confirming that the green signal resulted from DIVE_6 detecting theophylline drug uptake. By quantifying the fluorescent signals, the animals treated with 1 mM theophylline for 24 hpf showed a 6.6-fold increase in signal as compared to fish that did not receive the drug (Fig. 5C). This value is comparable to the 3.9-fold signal generated in mammalian cells with 1 mM theophylline. The improved signal measured in zebrafish as compared to mammalian cells likely results from the stable integration of the biosensor into the genome as compared to the transient transfection performed in HEK293T cells. Together these results suggest that HEK293T cells can serve as a biosensing prototyping platform for developing new DIVE transgenic zebrafish lines. Given the short time (days) to test biosensors in mammalians cells compared to zebrafish (months), this would greatly enable the development of new zebrafish biosensing tools.
We last determined whether GFP expression was reversible with removal of theophylline, thus potentially serving as a useful measurement of drug metabolism and excretion. To test this, embryos were bathed in 1 mM theophylline for 24 hours and then placed in fresh media without drug. GFP expression was decreased at 24 hours after washout with near baseline levels at 48 h (Fig. 6). Our results are consistent with the expected half-life of wild-type GFP (∼26 h).71 Expression of GFP remained high in developing liver and GI tract, suggesting a mechanism by which theophylline may be cleared in the zebrafish.
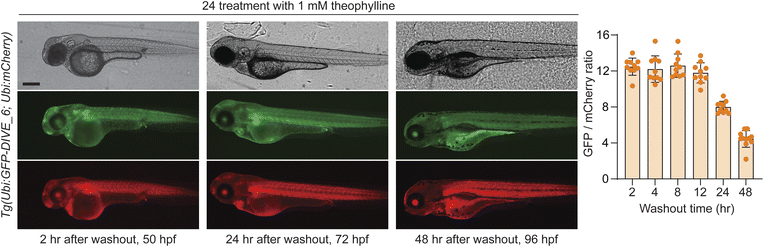 |
| Fig. 6 Washout of theophylline in transgenic DIVE expressing zebrafish line. Representative brightfield and fluorescent micrographs from embryos containing the integrated biosensor plasmid (Ubi:GFP-DIVE_6; Ubi:mCherry) and bathed for 24 hours in 1 mM theophylline. Embryos were measured after removal of theophylline. Zebrafish orientation is lateral view, anterior left. Scale bar: 250 μm. Graph displays quantification of pixel intensity calculated for the trunk of the zebrafish with the GFP/mCherry ratio plotted. The average GFP/mCherry ratio ± s.d. with values for individual fish shown. | |
We anticipate that our encoded aptamer biosensors will be valuable across multiple disciplines that study and monitor drug uptake under different conditions. For example, these biosensors can be used for comparing uptake levels from different drug formulations, or to compare uptake across tissues. Furthermore, drug uptake can be directly compared to animal behavior due to the live and non-invasive nature of these biosensors and therefore will enhance small molecule studies relevant to animal behavior and development. On the other hand, from an environmental perspective, our biosensors can be used to compare the fate, transformations, and uptake of drugs under different environmental conditions.72 Indeed, it has been shown that small changes in water pH alter uptake of common pharmaceuticals in fish.72,73
Our aptamer biosensors are unique in that they can be stably integrated into animal cells and persist through the lifetime and multiple generations of zebrafish. Though aptamers have been developed into numerous biosensing platforms due to their high affinity and ability to undergo conformational changes in response to specific target molecules, we addressed a major challenge in non-invasive live-cell biosensing by coupling the binding properties of RNA aptamers to catalytic RNA. Light-up RNA aptamers including Mango74 and Broccoli75 can also be engineered and encoded into whole animals and may result in faster biosensing response rates, but the light-up aptamer dyes are expected to exhibit irregular distributions across animal tissues, hence complicating precise drug uptake monitoring. One limitation of our platform is the potentially slow biosensing response. For example, theophylline is absorbed quickly.76 While we detect increases in signal in as little as 4 hours, we measure peak concentrations only after 24 hours. As such, future work will explore both faster ribozymes for our DIVE sensors77 as well as use of faster folding proteins78 to improve the rapid real time results. Nevertheless, our biosensors sense uptake, tissue distribution, and metabolism of the drug in a non-invasive manner. Here, we focused on the detection of pharmaceuticals; however, there are thousands of reported aptamers to a wide range of xenobiotics and endogenous molecules including metal ions, toxins, toxicants, lipids, and proteins. As such, our DIVE platform can be theoretically expanded to detect nearly any molecule that accumulates in the cytosol, enabling live-cell imaging or non-invasive whole animal monitoring for a plethora of applications.
Conclusions
In conclusion, we developed a genetically encoded aptamer biosensor platform suitable for transient expression or genetic engineering in mammalian cells and zebrafish and representing the first example of an encoded aptamer sensor in a transgenic vertebrate. We compared different aptamer-based biosensors to a panel of pharmaceuticals, with theophylline and gardiquimod biosensors showing high sensitivity and specificity. Our biosensors enabled single-cell monitoring of drug uptake in live cells in a dose-response manner. We further applied our biosensors to zebrafish, resulting in robust and precise detection of theophylline across various timepoints. We anticipate that these biosensors will be useful for a range of applications, from environmental toxicity monitoring to studying drug pharmacokinetics and formulation.
Ethical statement
The National Institutes of Health Office of Laboratory Animal Welfare (OLAW) is responsible for the administration of the US Public Health Service Policy on Humane Care and Use of Laboratory Animals. Compliance with the standards of the PHS Policy is a term and condition of NIH Grants Policy Statement. East Carolina University's Animal Welfare Assurance number is A3469-01. The IACUC committee at East Carolina University, Greenville, NC, USA approved all animal procedures (AUP#W262).
Data availability
Supporting data for this manuscript has been uploaded as part of the ESI.†
Author contributions
M. M. and K. M. conceived the idea and designed the research. E. A. O., T. R. and L. W. performed the experiments and analyzed the data. E. A. O. and M. M. wrote the manuscript. E. A. O., K. M. and M. M. revised the manuscript. All the authors approved the final version of the manuscript.
Conflicts of interest
The authors declare no competing financial interest.
Acknowledgements
The research was financially supported by grants from the National Institutes of Health (R21GM143565 to K. M. and M. M.); National Science and Engineering Research Council of Canada (NSERC) (Discovery Grant and Research Tools and Instruments Grant to M. M); the Canada Foundation for Innovation (JELF for equipment to M. M), the Fonds de Recherche Nature et Technologies (Nouveau Researcher grant to M. M). We are grateful to members of the McKeague and Mruk labs for useful discussions. Thanks to Janeva Shahi and Olivia Kovecses for helping set up the assays, Omma Shahara Ayon for help with RNA transcription, and Kaifeng Zhao for help with microscopy. Special thanks to Dr Maira Rivera for the help with figures, elements of each were created with Biorender (with appropriate academic licensing). We thank Prof. Nathan Luedtke for revising the text.
References
- F. Chen, Y. Zhao, Y. Pan, X. Xue, X. Zhang, A. Kumar and X.-J. Liang, Mol. Pharm., 2015, 12, 2237–2244 CrossRef CAS PubMed.
- C. E. McGhee, Z. Yang, W. Guo, Y. Wu, M. Lyu, C. J. DeLong, S. Hong, Y. Ma, M. G. McInnis, K. S. O'Shea and Y. Lu, ACS Cent. Sci., 2021, 7, 1809–1820 CrossRef CAS PubMed.
- X. Lu, B. Lin, N. Xu, H. Huang, Y. Wang and J.-M. Lin, Talanta, 2020, 211, 120732 CrossRef CAS PubMed.
- P. Daumar, R. Dufour, C. Dubois, F. Penault-Llorca, M. Bamdad and E. Mounetou, J. Pharm. Biomed. Anal., 2018, 152, 74–80 CrossRef CAS PubMed.
- Y. Wang and A. B. Hummon, Anal. Chem., 2023, 95, 9227–9236 CrossRef CAS PubMed.
- Y. Yuan, J. Zhang, X. Qi, S. Li, G. Liu, S. Siddhanta, I. Barman, X. Song, M. T. McMahon and J. W. M. Bulte, Nat. Mater., 2019, 18, 1376–1383 CrossRef CAS PubMed.
- X. Zhang, M. Scalf, T. W. Berggren, M. S. Westphall and L. M. Smith, J. Am. Soc. Mass Spectrom., 2006, 17, 490–499 CrossRef CAS PubMed.
- V. Kantae, E. H. J. Krekels, A. Ordas, O. González, R. C. van Wijk, A. C. Harms, P. I. Racz, P. H. van der Graaf, H. P. Spaink and T. Hankemeier, Zebrafish, 2016, 13, 504–510 CrossRef CAS PubMed.
- R. Li, H. Qi, Y. Ma, Y. Deng, S. Liu, Y. Jie, J. Jing, J. He, X. Zhang, L. Wheatley, C. Huang, X. Sheng, M. Zhang and L. Yin, Nat. Commun., 2020, 11, 3207 CrossRef CAS PubMed.
- R. Chen, F. Gore, Q.-A. Nguyen, C. Ramakrishnan, S. Patel, S. H. Kim, M. Raffiee, Y. S. Kim, B. Hsueh, E. Krook-Magnusson, I. Soltesz and K. Deisseroth, Nat. Biotechnol., 2021, 39, 161–164 CrossRef CAS.
- C. Cheng, W. Chen, H. Jin and X. Chen, Cells, 2023, 12 Search PubMed.
- J. Choi, J. Li, S. Ferdous, Q. Liang, J. R. Moffitt and R. Chen, Nat. Commun., 2023, 14, 4929 CrossRef CAS.
- K. Rau and A. Rentmeister, ACS Cent. Sci., 2017, 3, 701–707 CrossRef CAS PubMed.
- A. Chitnis and D. Dalle Nogare, Methods, 2018, 150, 32–41 CrossRef CAS PubMed.
- X. Shu, Curr. Opin. Chem. Biol., 2020, 54, 1–9 CrossRef CAS PubMed.
- F. Amat and P. J. Keller, Dev., Growth Differ., 2013, 55, 563–578 CrossRef PubMed.
- S. Veerapathiran and T. Wohland, J. Biosci., 2018, 43, 541–553 CrossRef PubMed.
- M. Wang, Y. Da and Y. Tian, Chem. Soc. Rev., 2023, 52, 1189–1214 RSC.
- S. Scarabelli, K. T. Tan, R. Griss, R. Hovius, P. L. D'Alessandro, T. Vorherr and K. Johnsson, ACS Sens., 2017, 2, 1191–1197 CrossRef CAS PubMed.
- G. C. H. Mo, B. Ross, F. Hertel, P. Manna, X. Yang, E. Greenwald, C. Booth, A. M. Plummer, B. Tenner, Z. Chen, Y. Wang, E. J. Kennedy, P. A. Cole, K. G. Fleming, A. Palmer, R. Jimenez, J. Xiao, P. Dedecker and J. Zhang, Nat. Methods, 2017, 14, 427–434 CrossRef CAS PubMed.
- M. Marsafari, J. Ma, M. Koffas and P. Xu, Curr. Opin. Biotechnol., 2020, 64, 175–182 CrossRef CAS PubMed.
- D. Paul, S. C. Kales, J. A. Cornwell, M. M. Afifi, G. Rai, A. Zakharov, A. Simeonov and S. D. Cappell, Nat. Commun., 2022, 13, 6364 CrossRef CAS PubMed.
- S. Burgstaller, H. Bischof, T. Rauter, T. Schmidt, R. Schindl, S. Patz, B. Groschup, S. Filser, L. van den Boom, P. Sasse, R. Lukowski, N. Plesnila, W. F. Graier and R. Malli, ACS Sens., 2021, 6, 3994–4000 CrossRef CAS PubMed.
- A. Miyawaki and Y. Niino, Mol. Cell, 2015, 58, 632–643 CrossRef CAS PubMed.
- L. Mi, Q. Yu, A. Karunanayake Mudiyanselage, R. Wu, Z. Sun, R. Zheng, K. Ren and M. You, ACS Sens., 2023, 8, 308–316 CrossRef CAS PubMed.
- K. Mishra, J. P. Fuenzalida-Werner, F. Pennacchietti, R. Janowski, A. Chmyrov, Y. Huang, C. Zakian, U. Klemm, I. Testa, D. Niessing, V. Ntziachristos and A. C. Stiel, Nat. Biotechnol., 2022, 40, 598–605 CrossRef CAS PubMed.
- H. Bischof, M. Rehberg, S. Stryeck, K. Artinger, E. Eroglu, M. Waldeck-Weiermair, B. Gottschalk, R. Rost, A. T. Deak, T. Niedrist, N. Vujic, H. Lindermuth, R. Prassl, B. Pelzmann, K. Groschner, D. Kratky, K. Eller, A. R. Rosenkranz, T. Madl, N. Plesnila, W. F. Graier and R. Malli, Nat. Commun., 2017, 8, 1422 CrossRef PubMed.
- Z. Chen, S. Zhang, X. Li and H.-w. Ai, Cell Chem. Biol., 2021, 28, 1542–1553 CrossRef CAS PubMed.
- J. P. Keller, J. S. Marvin, H. Lacin, W. C. Lemon, J. Shea, S. Kim, R. T. Lee, M. Koyama, P. J. Keller and L. L. Looger, Cell Rep., 2021, 35, 109284 CrossRef CAS PubMed.
- F. Sun, J. Zeng, M. Jing, J. Zhou, J. Feng, S. F. Owen, Y. Luo, F. Li, H. Wang, T. Yamaguchi, Z. Yong, Y. Gao, W. Peng, L. Wang, S. Zhang, J. Du, D. Lin, M. Xu, A. C. Kreitzer, G. Cui and Y. Li, Cell, 2018, 174, 481–496 CrossRef CAS PubMed.
- T. Yoshida, H. Nakajima, S. Takahashi, A. Kakizuka and H. Imamura, ACS Sens., 2019, 4, 3333–3342 CrossRef CAS PubMed.
- X. A. Cambronne, M. L. Stewart, D. Kim, A. M. Jones-Brunette, R. K. Morgan, D. L. Farrens, M. S. Cohen and R. H. Goodman, Science, 2016, 352, 1474–1477 CrossRef CAS PubMed.
- A. Alsaafin and M. McKeague, Biosens. Bioelectron., 2017, 94, 94–106 CrossRef CAS PubMed.
- Y. H. Wang, K. Y. Wei and C. D. Smolke, Annu. Rev. Chem. Biomol. Eng., 2013, 4, 69–102 CrossRef CAS PubMed.
- D. B. Karloff and J. M. Heemstra, Cell Chem. Biol., 2021, 28, 1539–1541 CrossRef CAS PubMed.
- A. Chamorro-Garcia, J. Gerson, C. Flatebo, L. Fetter, A. M. Downs, N. Emmons, H. L. Ennis, N. Milosavic, K. Yang, M. Stojanovic, F. Ricci, T. E. Kippin and K. W. Plaxco, ACS Sens., 2023, 8, 150–157 CrossRef CAS PubMed.
- L. F. Yang, M. Ling, N. Kacherovsky and S. H. Pun, Chem. Sci., 2023, 14, 4961–4978 RSC.
- O. Alkhamis, J. Canoura, C. Willis, L. Wang, J. Perry and Y. Xiao, J. Am. Chem. Soc., 2023, 145, 12407–12422 CrossRef CAS PubMed.
- Y. Su, H. Xu, Y. Chen, J. Qi, X. Zhou, R. Ge and Z. Lin, New J. Chem., 2018, 42, 2850–2856 RSC.
- J. Canoura, H. Yu, O. Alkhamis, D. Roncancio, R. Farhana and Y. Xiao, J. Am. Chem. Soc., 2021, 143, 805–816 CrossRef CAS PubMed.
- Y. Ding and J. Liu, J. Am. Chem. Soc., 2023, 145, 7540–7547 CrossRef CAS PubMed.
- Y. Ouyang, Y. Biniuri, M. Fadeev, P. Zhang, R. Carmieli, M. Vazquez-Gonzalez and I. Willner, J. Am. Chem. Soc., 2021, 143, 11510–11519 CrossRef CAS PubMed.
- A. D. Ellington and J. W. Szostak, Nature, 1990, 346, 818–822 CrossRef CAS PubMed.
- F. Ricci, A. Vallée-Bélisle, A. J. Simon, A. Porchetta and K. W. Plaxco, Acc. Chem. Res., 2016, 49, 1884–1892 CrossRef CAS PubMed.
- C. Tuerk and L. Gold, Science, 1990, 249, 505–510 CrossRef CAS PubMed.
- M. C. DeRosa, A. Lin, P. Mallikaratchy, E. M. McConnell, M. McKeague, R. Patel and S. Shigdar, Nat. Rev. Methods Primers, 2023, 3, 54 CrossRef CAS.
- G. A. Wang, X. Wu, F. Chen, C. Shen, Q. Yang and F. Li, J. Am. Chem. Soc., 2023, 145, 2750–2753 CrossRef CAS PubMed.
- J. Zhao, J. Gao, W. Xue, Z. Di, H. Xing, Y. Lu and L. Li, J. Am. Chem. Soc., 2018, 140, 578–581 CrossRef CAS PubMed.
- A. D. Cawte, P. J. Unrau and D. S. Rueda, Nat. Commun., 2020, 11, 1283 CrossRef CAS PubMed.
- Y. Peng, L. Shu, X. Deng, X. Huang, X. Mo, F. Du and Z. Tang, Anal. Chem., 2023, 95, 13762–13768 CrossRef CAS PubMed.
- M. McKeague, Y. H. Wang and C. D. Smolke, ACS Chem. Biol., 2015, 10, 2463–2467 CrossRef CAS PubMed.
- J. S. Xiang, M. Kaplan, P. Dykstra, M. Hinks, M. McKeague and C. D. Smolke, Nat. Commun., 2019, 10, 4327 CrossRef PubMed.
- B. Townshend, A. B. Kennedy, J. S. Xiang and C. D. Smolke, Nat. Methods, 2015, 12, 989–994 CrossRef CAS PubMed.
- Y. H. Wang, M. McKeague, T. M. Hsu and C. D. Smolke, Cell Syst., 2016, 3, 549–562 CrossRef CAS PubMed.
- P. Marschall, J. B. Thomson and F. Eckstein, Cell. Mol. Neurobiol., 1994, 14, 523–538 CrossRef CAS PubMed.
- C. Hammann, A. Luptak, J. Perreault and M. de la Peña, Rna, 2012, 18, 871–885 CrossRef CAS PubMed.
- M. N. Win and C. D. Smolke, Proc. Natl. Acad. Sci. U. S. A., 2007, 104, 14283–14288 Search PubMed.
- J. A. Nelson, I. Shepotinovskaya and O. C. Uhlenbeck, Biochemistry, 2005, 44, 14577–14585 CrossRef CAS PubMed.
- M. B. Elowitz, A. J. Levine, E. D. Siggia and P. S. Swain, Science, 2002, 297, 1183–1186 CrossRef CAS PubMed.
- C. Mosimann, C. K. Kaufman, P. Li, E. K. Pugach, O. J. Tamplin and L. I. Zon, Development, 2011, 138, 169–177 CrossRef CAS PubMed.
- J. M. Raser and E. K. O'Shea, Science, 2004, 304, 1811–1814 CrossRef CAS PubMed.
- B. Townshend, J. S. Xiang, G. Manzanarez, E. J. Hayden and C. D. Smolke, Nat. Commun., 2021, 12, 1437 CrossRef CAS PubMed.
- N. W. Luedtke, P. Carmichael and Y. Tor, J. Am. Chem. Soc., 2003, 125, 12374–12375 CrossRef CAS PubMed.
- S. Thakur, D. More, B. Rahat, K. L. Khanduja and J. Kaur, Mol. Cell. Biochem., 2016, 411, 151–160 CrossRef CAS PubMed.
- G. Emilien, W. van Meurs and J. M. Maloteaux, Pharmacol. Ther., 2000, 88, 33–58 CrossRef CAS PubMed.
- T. Kijima, T. Shimizu, S. Nonen, M. Furukawa, Y. Otani, T. Minami, R. Takahashi, H. Hirata, I. Nagatomo, Y. Takeda, H. Kida, S. Goya, Y. Fujio, J. Azuma, I. Tachibana and I. Kawase, J. Clin. Oncol., 2011, 29, e588–e590 CrossRef PubMed.
- E. E. Patton, L. I. Zon and D. M. Langenau, Nat. Rev. Drug Discovery, 2021, 20, 611–628 CrossRef CAS PubMed.
- G. Abe, M. L. Suster and K. Kawakami, Methods Cell Biol., 2011, 104, 23–49 CAS.
- M. L. Suster, H. Kikuta, A. Urasaki, K. Asakawa and K. Kawakami, Methods Mol. Biol., 2009, 561, 41–63 CrossRef CAS PubMed.
- M. Shannon, Arch. Intern. Med., 1999, 159, 989–994 CrossRef CAS PubMed.
- N. Kitsera, A. Khobta and B. Epe, Biotechniques, 2007, 43, 222–227 CrossRef CAS PubMed.
- A. Bechu, J. Liao, C. Huang, C. Ahn, M. McKeague, S. Ghoshal and A. Moores, ACS Appl. Nano Mater., 2021, 4, 8417–8428 CrossRef CAS.
- D. Alsop and J. Y. Wilson, Aquat. Toxicol., 2019, 210, 11–18 CrossRef CAS PubMed.
- R. J. Trachman 3rd, A. Autour, S. C. Y. Jeng, A. Abdolahzadeh, A. Andreoni, R. Cojocaru, R. Garipov, E. V. Dolgosheina, J. R. Knutson, M. Ryckelynck, P. J. Unrau and A. R. Ferre-D'Amare, Nat. Chem. Biol., 2019, 15, 472–479 CrossRef PubMed.
- G. S. Filonov, J. D. Moon, N. Svensen and S. R. Jaffrey, J. Am. Chem. Soc., 2014, 136, 16299–16308 CrossRef CAS PubMed.
- D. J. Rowe, I. D. Watson, J. Williams and D. J. Berry, Ann. Clin. Biochem., 1988, 25(Pt 1), 4–26 CrossRef CAS PubMed.
- A. B. Kennedy, J. C. Liang and C. D. Smolke, Nucleic Acids Res., 2012, 41, e41 CrossRef PubMed.
- D. J. Rosenman, Y. M. Huang, K. Xia, K. Fraser, V. E. Jones, C. M. Lamberson, P. Van Roey, W. Colón and C. Bystroff, Protein Sci., 2014, 23, 400–410 CrossRef CAS PubMed.
|
This journal is © The Royal Society of Chemistry 2024 |