DOI:
10.1039/D3RP00348E
(Review Article)
Chem. Educ. Res. Pract., 2024,
25, 594-612
Modeling students’ epistemic cognition in undergraduate chemistry courses: a review†
Received
14th December 2023
, Accepted 4th June 2024
First published on 11th June 2024
Abstract
Thinking about knowledge and knowing (i.e., epistemic cognition) is an important part of student learning and has implications for how they apply their knowledge in future courses, careers, and other aspects of their lives. Three classes of models have emerged from research on epistemic cognition: developmental models, dimensional models, and resources models. These models can be distinguished by how value is assigned to particular epistemic ideas (hierarchy), how consistent epistemic ideas are across time and/or context (stability), and the degree to which people are consciously aware of their own epistemic ideas (explicitness). To determine the extent to which these models inform research on epistemic cognition in chemistry education specifically, we reviewed 54 articles on undergraduate chemistry students’ epistemologies. First, we sought to describe the articles in terms of the courses and unit of study sampled, the methods and study designs implemented, and the means of data collection utilized. We found that most studies focused on the epistemic cognition of individual students enrolled in introductory chemistry courses. The majority were qualitative and employed exploratory or quasi-experimental designs, but a variety of data collection methods were represented. We then coded each article for how it treated epistemic cognition in terms of hierarchy, stability, and explicitness. The overwhelming majority of articles performed a hierarchical analysis of students’ epistemic ideas. An equal number of articles treated epistemic cognition as stable versus unstable across time and/or context. Likewise, about half of the studies asked students directly about their epistemic cognition while approximately half of the studies inferred it from students’ responses, course observations, or written artifacts. These codes were then used to infer the models of epistemic cognition underlying these studies. Eighteen studies were mostly consistent with a developmental or dimensional model, ten were mostly aligned with a resources model, and twenty-six did not provide enough information to reasonably infer a model. We advocate for considering how models of epistemic cognition—and their assumptions about hierarchy, stability, and explicitness—influence the design of studies on students’ epistemic cognition and the conclusions that can be reasonably drawn from them.
Introduction
Ideas about knowledge and knowing underlie many of the behaviors people exhibit and the decisions they make. Whenever people ask a question, they are pursuing some type of knowledge product (e.g., factual information). When they engage in a particular behavior to answer that question, (e.g., typing their question into a search engine), it is likely because they view it as a reliable process for obtaining the desired knowledge product. During the process, they are also relying on ideas regarding appropriate justifications for knowledge (e.g., obtained from a trustworthy source). Importantly, given how often people need to reason with or about knowledge on a daily basis, these considerations tend to be made subconsciously (Hammer and Elby, 2002; Chinn et al., 2011).
Thinking about knowledge and knowing (i.e., epistemic cognition) is also part of student learning in the classroom. Some ways of thinking are independent of subject matter, as exemplified by the common course goal of improving students’ critical thinking skills (Tiruneh et al., 2014). Each discipline also has its own norms regarding what constitutes good knowledge, how it is obtained, what form it should take, etc. (Buehl and Alexander, 2001; Talanquer et al., 2015). These norms may be communicated to students along with specific disciplinary practices and content (Louca et al., 2004; Rosenberg et al., 2006; Russ, 2018). For example, a teacher might respond to a student's answer with a comment like, “That is correct, but how did you arrive at that answer?” Such a comment conveys that reasoning is equally or perhaps more important than a knowledge claim. Like epistemic cognition in daily life, some of what constitutes appropriate use of knowledge in class is communicated tacitly, for example, based on which questions are asked on assessments and which responses to those questions earn points (Snyder, 1973; Entwistle, 1991; Scouller and Prosser, 1994; Scouller, 1998; Momsen et al., 2013).
The epistemic learning that occurs in the classroom has implications for how students understand and apply what they are learning in the course, in future courses, and in other aspects of their lives. Hammer et al. (2005) argue that the conceptual knowledge a person activates in a given context is influenced by the epistemic knowledge they are drawing upon. For example, the role of epistemic knowledge as a “control structure” (Bing and Redish, 2009) was used to understand why a student in an introductory physics class did not see her intuition and everyday experiences as allowed sources of knowledge (Lising and Elby, 2005) and to explain the teacher-initiated shifts in reasoning observed in a group of eighth graders discussing the rock cycle (Rosenberg et al., 2006). These studies suggest that in order to support student learning and transfer of knowledge, which are stated goals of virtually all STEM education reform efforts (American Association for the Advancement of Science, 2011; National Research Council, 2012; Cooper and Klymkowsky, 2013; Talanquer and Pollard, 2017), instructors and researchers need to attend to students’ epistemic cognition.
To support further research on students’ epistemic cognition, we conducted a review of the work that has been done so far in the context of undergraduate chemistry courses. We chose to focus most of our attention on the model of epistemic cognition used, as this informs how data is collected and interpreted. We found, however, that most studies did not include an explicit theory of epistemic cognition. Therefore, we compared three prominent models of epistemic cognition in the literature and identified major differences between them that would likely be evident in a study. We developed a coding scheme based on these differences and used it to infer models of epistemic cognition in the articles we analyzed. We present the results of our analysis, and, drawing on scholarship from the broader field of epistemology research, offer recommendations for future research on epistemic cognition in chemistry education.
Literature background
Models of individual epistemic cognition
Personal epistemology research concerns how individuals think about, construct, and evaluate knowledge. It draws on scholarship across several fields including philosophy, the learning sciences, psychology, and discipline-based education. Several different terms have been used to describe an individual's ideas about knowledge, including “epistemic beliefs” (Hofer and Pintrich, 1997), “epistemic cognition” (Kitchener, 1983; Greene et al., 2008; Sandoval et al., 2016), “epistemic resources” (Hammer and Elby, 2002), and “epistemic games” (Collins and Ferguson, 1993).
Given the variety in terminology, it is perhaps unsurprising that multiple ways of modeling epistemic cognition have been developed. Below we describe the three classes of models that have emerged, along with prominent examples for each (Table 1). For more detailed descriptions and examples, see the reviews published by Hofer and Pintrich (1997) and Sandoval et al. (2016).
Table 1 Models of epistemic cognition
Type of model |
Description |
Literature examples |
Developmental models |
Development of a person's epistemology proceeds through stages of increasing sophistication |
Perry (1970)
|
Kuhn (1999)
|
King and Kitchener (1994)
|
|
Dimensional models |
A person's epistemology consists of multiple aspects (e.g., simplicity of knowledge, certainty of knowledge, sources of knowledge, justifications for knowledge), each of which can vary in sophistication independently of the others. |
Schommer-Aikins (2004)
|
Hofer and Pintrich (1997)
|
|
Resources models |
A person's epistemology is constructed in the moment and the sophistication or utility varies according to the situation. These models typically organize epistemic ideas into categories (e.g., epistemic aim, epistemic form). |
Hammer and Elby (2002)
|
Chinn et al. (2011)
|
Developmental models.
Early models of epistemic cognition reported in the literature can be classified as developmental. A frequently cited example is Perry's scheme of intellectual and ethical development (1970). Through interviews with male college students, he proposed a developmental progression in which students initially view knowledge as objective and unchanging and over time develop an understanding of knowledge as contextual and tentative. Later scholars built upon Perry's work and proposed similar developmental models. Based on her work on reasoning in everyday life, Kuhn (1999) detailed three stages of epistemological development: absolutist, multiplist, and evaluatist. The reflective judgement model developed by King and Kitchener (2004) describes epistemic cognition for thinking about ill-structured problems and contains seven stages grouped into three broad categories: pre-reflective, quasi-reflective, and reflective. Underlying these developmental models is the assumption that different aspects of epistemology (such as the nature of knowledge and sources of knowledge) are correlated and progress in tandem.‡ Furthermore, as a person's epistemology develops, it becomes more sophisticated or expert-like.
Dimensional models.
Later researchers questioned the assumption of correlation and proposed models in which epistemology is characterized along separate, independent dimensions rather than a single developmental sequence. For example, Schommer-Aikins proposed five dimensions: simple knowledge, certain knowledge, source of knowledge, ability to learn, and quick learning (2004).§ In their 1997 review on personal epistemology research, Hofer and Pintrich synthesized ideas from developmental and dimensional models and arrived at a model consisting of four dimensions: simplicity of knowledge, certainty of knowledge, source of knowledge, and justification of knowledge. Although the dimensional models detangle various aspects of epistemology, they still ascribe to the assumption that epistemological beliefs lie on continuums of increasing sophistication.
Resources model.
More recently, Hammer and Elby rejected the idea that some epistemic ideas are inherently more sophisticated than others (2002). In their proposed resources model, a person's epistemology is constructed in the moment from smaller-grained epistemological resources (Hammer and Elby, 2002). Activation of these resources is dynamic and may change in a matter of seconds in response to cues from the environment. While these epistemological resources can be grouped into categories, such as form of knowledge or stance toward knowledge, the resources within a category do not exist on a sophistication continuum. Instead, Hammer and colleagues argue that some epistemological resources may be productive (i.e., useful in progressing toward a goal) in one circumstance while a different set of epistemological resources may be productive in another. Thus, epistemological sophistication, according to Elby and Hammer (2001), “consists of having resources to sort out the complexity of knowledge in different contexts” rather than having “a global, decontextualized opinion about [an] issue.” They point out that while most developmental and dimensional models consider constructing one's own knowledge more sophisticated than receiving knowledge from authority, there are times when this is not necessarily true. For example, they argue that it is probably more worthwhile to accept the biologists’ claim than cows have multiple stomachs rather than go out and dissect one yourself. Likewise, Muis et al. (2006), Chinn et al. (2011), and Sandoval et al. (2016) have also argued for a context-dependent view of epistemic cognition.
Key assumptions in models of epistemic cognition
Developmental and dimensional models can be distinguished from the resources model of epistemic cognition by attending to differences in a few key assumptions (Table 2). These assumptions manifest themselves in the way data is collected and analyzed, as described in the following sections.
Table 2 Comparison of models of epistemic cognition with regards to hierarchy, stability, and explicitness
Model of epistemology |
Hierarchy |
Stability |
Explicitness |
Not inherent to model but consistent with how most studies using this model have been conducted.
|
Developmental |
Hierarchical |
Stable |
Explicita |
Dimensional |
Hierarchical |
Stable |
Explicita |
Resources |
Variable utility |
Unstable |
Implicit |
Hierarchy.
Approaches to evaluating students’ epistemic ideas can vary significantly depending on the model of epistemic cognition. Developmental and dimensional models organize epistemic beliefs in a hierarchical manner. Research conducted according to these models typically seeks to assign students’ epistemic beliefs to levels or stages and evaluate interventions designed to advance students toward more sophisticated or expert-like epistemic beliefs. The resources model, on the other hand, contends that no epistemic idea is universally better than another. Assumptions about hierarchy affect which claims can be reasonably made from analysis of a dataset. For example, if one adopted a resources model of epistemic cognition, it would not make sense to make claims like: “after a 1-week module on the nature of science, students’ post-test scores showed that their understandings of how scientific knowledge is constructed grew more sophisticated”. Such claims rely on the assumption of a developmental hierarchy to be sensible. Since there is no mention of context-dependence in this claim, it may also imply that this hierarchy applies across all contexts. A study employing a resources model might instead focus on understanding the interplay between contextual factors and students’ epistemic cognition.
Stability.
A second point of difference between models concerns the stability of students’ epistemic cognition. Researchers employing a developmental model typically treat a person's epistemic beliefs as relatively stable over long periods of time. Dimensional models also treat epistemic beliefs as having trait-like or theory-like characteristics and thus assume they are relatively stable over time. This assumption is often borne out in the form of the research question; for example, asking “Do students gain a more sophisticated understanding of how scientific knowledge is constructed after a lesson on the nature of science?” implies that changes due to the intervention are expected to be much larger than changes due to the dynamics of cognition. An assumption of stability is also evident in the frequent collection of pre- and post-test data, often at the beginning and end of a course. In contrast, resources models assume epistemic cognition is often unstable and can shift rapidly in response to comments from a teacher or peer, for example. Importantly, resources models do not preclude epistemic stability—if one finds a set of resources is frequently useful in a given context, they may consistently activate these resources in contexts that (implicitly) seem similar. Researchers employing a resources model thus tend to collect data over short periods of time, such as one class period.
Assumptions of stability also manifest themselves in the implied generalizability or specificity of epistemic ideas. Some researchers utilizing developmental or dimensional models expect epistemic beliefs to be stable across contexts, as indicated by the domain-general descriptions employed (e.g., absolutists believe in one knowable truth). They would expect students to answer the same way whether a survey is given in a science class, a math class, or an English class. Others have limited their claims to a particular area of knowledge. In fact, Nature of Science (NOS) research has emerged as a somewhat separate field of study (Lederman, 1992). Resources models go even further, contending that researchers should not assume the same epistemological resources are activated in all chemistry classes. Thus, studies that use a resources model typically focus on generating or expanding upon theories rather than obtaining statistically generalizable results.
Explicitness.
One additional assumption worth highlighting regarding the nature of epistemic cognition concerns how it can be studied. A resources model of epistemic cognition regards activation of epistemological resources as a largely subconscious process (Hammer and Elby, 2002). As such, evidence for students’ epistemic cognition is best obtained by observing students’ behavior in the situation of interest (e.g., classroom interactions). Developmental and dimensional models do not discuss whether epistemic cognition is tacit or not, but historically scholars ascribing to these models have probed epistemic beliefs through surveys and interviews in which participants are asked about their beliefs directly. It is assumed, often without strong or clear evidence, that the correlation between self-reported epistemic beliefs and epistemic beliefs inferred from observed behavior is strong.
Social epistemology
Historically, researchers sought to characterize an individual's epistemic beliefs. Like the emergence of social constructivism from constructivism, epistemology researchers began to emphasize in published research the role others, such as the classroom community or society more broadly, play in shaping an individual's epistemic cognition. This has given rise to social epistemology, the study of how people collectively determine how knowledge is created and evaluated (Schmitt, 2017). In the context of education, researchers draw upon social epistemology to understand how classroom communities negotiate epistemic norms. Their emphasis is on the interactions between individuals and between individuals and the wider cultural context in which their education takes place, rather than on the individual's thoughts and behaviors (Sandoval et al., 2016).
Research questions
Our first goal in this review, intended primarily for researchers, is to describe how studies on undergraduate students’ epistemic cognition have been conducted. We were guided by the following questions:
(1) What student populations are the samples drawn from?
(2) Does the study focus on the epistemic ideas of individual students or groups of students?
(3) What methodologies and study designs have been employed?
(4) What means of data collection have been used?
Our second goal is to discuss the models of epistemic cognition that explicitly or implicitly informed studies on undergraduate chemistry students’ epistemic cognition. Since most articles did not report a model of epistemic cognition, we attempted to infer the model by addressing the following questions:
(1) Do chemistry education researchers characterize epistemic ideas as hierarchical in nature or as varying in utility depending on context?
(2) Is epistemic cognition assumed to be stable over time and/or across contexts?
(3) Did researchers infer students’ epistemic ideas from explicit statements about knowledge and knowing or from observations of behavior or interpretation of students’ statements?
We intentionally do not summarize the findings of the studies, largely, because there was so much variation in what it meant to study epistemic cognition. It is difficult to compare, for example, a study that characterized the class consensus on appropriate justifications for arguments with a study that documented changes in individual students’ Likert-scale responses to items describing the simplicity of knowledge. Without more consensus regarding the nature of epistemic cognition and how it should be studied and measured, it seems unproductive, and in some cases impossible, to synthesize results across studies.
Methods
Selection of articles
For this review, we chose to focus on undergraduate chemistry students’ epistemic cognition. Therefore, we started by collecting articles published in the chemistry education journals Journal of Chemical Education and Chemistry Education Research and Practice. We then expanded our search to more general science education journals: Journal of Research in Science and Teaching, Science Education, and International Journal of Science Education. Finally, we searched the ERIC and Taylor & Francis databases.
We used several search terms to find articles that studied epistemology in the context of chemistry courses. We used the search term “epistem*” to find articles containing words related to epistemology, such as “epistemic” and “epistemological.” We also searched “nature of science,” as this research often looks at how people perceive scientific knowledge and how that knowledge is obtained. We also used the more general search term “belief” because we anticipated that many articles would describe students’ beliefs concerning chemistry knowledge and knowing without using the term “epistemic” or “epistemological” to describe these beliefs.
Since some of these searches yielded a large number of hits, we applied a few filters to narrow down the number of results. We decided to limit the scope of this review to papers published between 2000 and 2022. For journals or databases that included multiple types of publications, we restricted the search results to research articles (as opposed to publications describing activities, for example). For the science education journals and databases, “chemistry” was also entered as a search term. The ERIC database also contained the option to restrict results to those tagged as “Higher Education,” which was helpful in eliminating articles focused on K-12 education. Similarly, we used the tag “Education” to narrow the scope of results obtained by searching the Taylor & Francis database.
With these filters in place, the initial searches for “epistem*,” “nature of science,” and “belief” yielded 693 unique articles. From here, we screened the articles manually through the iterative process shown in Fig. 1. Since the scope of this review is limited to undergraduate students’ epistemologies, articles that collected data from K-12 students, graduate students, and teachers or faculty were removed. The first author then performed a keyword search on each article using the terms “epistem,” “nature of science,” and “belief” to determine whether these were the focus of the article or simply mentioned in passing. For many of the articles, these terms were only found in the titles of referenced articles or mentioned in passing. For example, several articles utilized the resources theoretical framework, which encompasses both conceptual and epistemic resources; if the article then went on to characterize only conceptual resources, it was not included in this review.
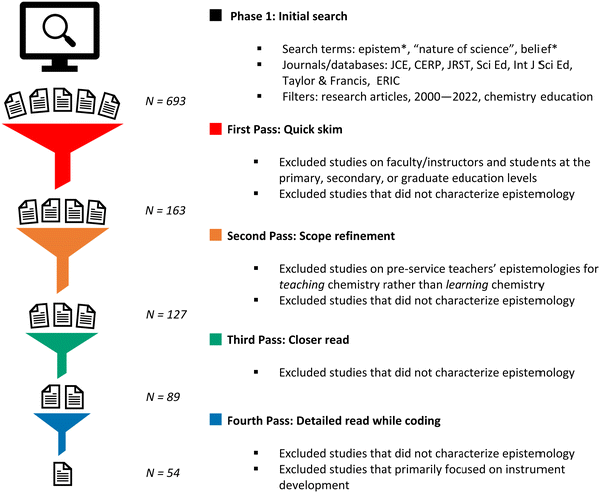 |
| Fig. 1 Article selection process. aBecause epistemic cognition was often intertwined with other aspects of learning (e.g., conceptual learning, affective outcomes), we continuously refined our inclusion criteria, resulting in an iterative selection process. See ESI,† for more details. | |
Following the initial screen of search results, 163 articles remained. The first author skimmed through these to determine if the studies sought to characterize or measure students’ epistemologies. Another 36 articles were removed during this phase, resulting in 127 articles. From here, the first author read through each article in full to determine if it met the criteria described above and reduced the sample down to 85 articles. From there, we started to code the articles, and during this process, we eliminated an additional 31 articles. Some were eliminated because, upon a closer read, they did not focus on characterizing students’ epistemic cognition. A few others were eliminated due to a primary focus on instrument development. The total number of articles included in the analysis for this review is 54. A complete list can be found in the ESI.†
Analysis of articles—descriptive codes
The first part of the analysis involved coding for who was being studied and how they were being studied. Five categories of codes were developed: study population, unit of analysis, methodology, study design, and data collection. The codes within each category are summarized in Table 3 and described in more detail below.
Table 3 List of codes used to describe methodological aspects of the studies
Code |
Description |
Sample |
Intro chem |
Study participants were recruited from a first-year chemistry, introductory chemistry, or general chemistry course for majors or non-majors. |
Intro chem lab |
Study participants were recruited from a first-year chemistry, introductory chemistry, or general chemistry laboratory course for majors or non-majors. |
OChem |
Study participants were recruited from an organic chemistry course for majors or non-majors. |
OChem lab |
Study participants were recruited from an organic chemistry laboratory course for majors or non-majors. |
PChem |
Study participants were recruited from a physical chemistry course or physical chemistry laboratory course. |
Non-course specific |
Study participants were recruited from multiple chemistry courses or were studied as they progressed through multiple chemistry courses. |
Chem for pre-service teachers |
Study participants were recruited from chemistry or science classes designed for pre-service teachers. |
Unit of analysis |
Individual |
Data was collected on individual students. |
Group |
Data was collected on groups of students, ranging from pairs of students to whole classes. |
Individual and group |
Data was collected on both individuals and groups. |
Methodology |
Qualitative |
Non-numerical data, such as words or images, was collected and analyzed for themes, patterns, or features relevant to the research question. |
Quantitative |
Numeric data was collected and analyzed via statistical methods to determine relationships among variables. |
Mixed methods |
A combination of numerical and non-numerical data was collected and analyzed using both quantitative and qualitative techniques. |
Study design |
Exploratory |
A phenomenon was explored on a small sample with no comparison groups or treatments administered. |
Quasi-experimental |
The impact of an intervention was assessed by: |
|
• comparing data collected before and after implementation of the intervention on a treatment group; |
|
• evaluating data collected after implementation of the intervention on a treatment group; |
|
• Comparing data collected on two or more treatment groups before and after implementation of an intervention; |
|
• Comparing data collected on two or more treatment groups after implementation of an intervention. |
Longitudinal |
Data was collected at three or more timepoints over a period of time (semester or longer) to understand and/or measure how an outcome variable changes. |
Correlational |
The quantitative relationship between two or more variables was determined. |
Data collection method |
Interview |
Researchers met with students and asked students to respond to questions orally or complete tasks. Interviews could be conducted with individual students or groups of students. |
Open-ended survey |
Students were asked to respond to a written or electronic set of questions using their own words. |
Written artifact |
Written (or electronic) work that students created as part of their regular coursework. These included laboratory reports, essays, worksheets, and exams. |
Classroom recording |
Video and/or audio recordings of the whole class or small groups of students, typically used to collect classroom dialogue. |
Selected-response survey |
Students were asked to respond to a written or electronic set of questions and/or statements by selecting the response that best aligns with their thoughts. |
Sample.
A simple coding scheme was developed to summarize the different subsets of undergraduate chemistry students represented in these studies. The code Intro chem describes courses labeled as general, introductory, or first-year chemistry and encompasses variations for chemistry majors, STEM majors, and non-majors. A separate code, Intro chem lab, is used for general or introductory chemistry laboratory courses. The codes OChem and OChem lab describe the organic chemistry lecture and laboratory courses, respectively, that students typically take during their second year of college or university. A few studies recruited students from an upper-level physical chemistry lecture or lab course; these were labeled PChem. No other upper-level chemistry courses were represented in our sample. The code Undergrad chem was applied to studies that recruited students from across different chemistry courses. Finally, the code Chem for pre-service teachers was assigned to studies that sampled students from chemistry classes designed for pre-service teachers.
Unit of analysis.
The unit of analysis code was implemented to distinguish studies on personal epistemology from those on social epistemology. Studies that collected data on each student, consistent with personal epistemology research, were coded as Individual. Studies that collected data on groups of students, consistent with social epistemology research, were coded as Group. A third code, Individual and Group, was included to describe studies that collected data from both individuals and groups of students.
Methodology.
The articles included in this study were also characterized according to their methodologies and study designs. Methodology was described as Qualitative, Quantitative, and Mixed methods. Qualitative studies collect non-numerical data, such as classroom dialogue, that are analyzed by looking for themes, patterns, or other features relevant to the research question (Johnson and Christensen, 2020). Quantitative studies collect numerical data, such as exam scores, that are analyzed via statistical methods (Johnson and Christensen, 2020). Mixed methods studies utilize both types of data and analyses (Johnson and Christensen, 2020).
Study design.
As these methodology categories are quite broad, more specific codes for study designs were employed. Exploratory studies were defined as those that collected data from a single group, absent a treatment, with the goal of understanding some aspect of students’ epistemic cognition. Quasi-experimental studies (Campbell and Stanley, 1963), on the other hand, seek to determine the effects of a treatment on students’ epistemic cognition. We chose not to distinguish between studies that involved a single treatment group versus those that included a control group or multiple treatment groups, nor did we separate studies that used a pre- and post-tests from those that used only post-tests. We reasoned that all of these studies were united by a common goal-assessing the impact of some curricular and/or pedagogical change and the particulars of how they did so were not crucial to this review. Longitudinal studies focus primarily on understanding or documenting change over time. White and Arzi (2005) define a longitudinal study as “one in which two or more measures or observations of a comparable form are made of the same individuals or entities over a period of at least a year.” We modified their criteria slightly when coding. We required that a study collect data at more than two timepoints to distinguish longitudinal studies from the many studies that used pre- and post-tests but were focused on the impact of an intervention rather than the dynamics of epistemic cognition. We also lowered the duration to a semester given that most undergraduate courses operate over a semester rather than a year. Finally, Correlational studies sought to demonstrate a quantitative relationship, or lack thereof, between two or more variables, at least one of which was epistemic.
Data collection.
We expanded upon the codes used by Rodriguez, Hunter, et al. (2020) when describing the different ways in which data on students’ epistemic cognition was collected. Interviews consist of verbal responses to questions posed by the interviewer. This code included interviews conducted with individuals, pairs of students, and focus groups as well as various types of interviews, such as think-aloud (Charters, 2003), stimulated-recall (Dempsey, 2010), and task-based, cognitive clinical interviews (Ginsburg, 1997, Russ et al., 2012). Open-ended surveys included any written or electronic form in which students responded to questions in their own words. Selected-response surveys asked students to select a response from a given list. The code Written artifacts pertains to any work students submitted as part of the course, such as exams or laboratory reports. Finally, Classroom recording describes any audio and/or video recordings of the whole class or small groups of students. Because each study can collect data via multiple avenues, a single study could receive multiple data collection codes.
Analysis—model of cognition codes
Part two of the analysis focused on characterizing each article's treatment of epistemic cognition in terms of hierarchy, stability, and explicitness. Codes belonging to each category are shown in Table 4 and described in detail below. When assigning codes, all sections in an article were considered, but the sections that described data collection and data analysis proved especially useful.
Table 4 List of codes used to describe the assumptions about the nature of epistemic cognition
Code |
Description |
Hierarchy |
Hierarchical |
Certain epistemic ideas are considered more sophisticated, expert-like, or desirable than others. |
Variable utility |
Context determines the value of epistemic ideas. |
Ambiguous |
No indication of value or conflicting statements concerning the relative values of epistemic ideas. |
Stability |
Stable |
Epistemic cognition is treated as stable across contexts and/or relatively long periods of time (e.g., semester, year). |
Unstable |
Epistemic cognition is treated as unstable across context and/or time (on the scale of minutes). |
Unclear |
No indication as to whether epistemic cognition is considered stable or unstable across context and/or time. |
Explicitness |
Explicit |
Participants are asked to respond to statements/questions in which they would need to be consciously aware of their own epistemic ideas. |
Implicit |
Epistemic ideas are inferred from participants’ responses and/or behavior. |
Both |
Data is collected in multiple ways, some of which require participants’ awareness and some of which are inferred. |
Hierarchy.
The first category of codes concerns the hierarchical nature of epistemologies. Some articles characterized students’ responses according to varying levels of sophistication in which some epistemologies were deemed better or more desirable than others. These articles were given the code Hierarchical. Typically, the most sophisticated descriptor was applied to responses that aligned with those an expert chemist or scientist gave. For example, when developing the Colorado Learning Attitudes about Science Survey for chemistry, Adams et al. (2008) administered the survey to chemistry faculty in order to define the expert response for each survey item. Student responses were then scored in terms of how closely they matched the expert responses. In other studies, the relative rank of descriptors seems to have been determined according to the authors’ judgement. Other articles described epistemologies as more or less useful based on the particular circumstance rather than being universally better or worse; these were coded as Variable utility. Viewing epistemologies as of variable utility does not preclude situated hierarchies. In a given context, one may find some ways of knowing and learning more useful than others in advancing a particular aim. For example, one may find accumulating information from public health authorities more useful than building a model of disease spread from experience if one is trying to figure out whether to wear a mask to the supermarket. Variable utility does not mean all epistemologies are equally useful across all contexts. Finally, the code Ambiguous was used for articles that did not discuss the value of particular epistemologies or contained contradictory statements regarding value. For example, an article could invoke a resources model in the theoretical framework section but report a hierarchical coding scheme in the analysis.
Stability.
The stability category of codes was developed to describe how dynamic epistemic cognition was assumed to be, especially as it related to the timescale of each study. The code Stable was used for studies that seemed to view epistemic beliefs as relatively unchanging over long periods of time or in the absence of an intervention (Table 3). None of the articles included in our study directly stated an assumption of stability; rather, we inferred this from the methods used. Many of these studies utilized a pre-post design in which pre- and post-measures were spaced days, weeks, or months apart. Study designs of this sort, we argue, suggest that researchers expected any changes to occur over a long time period (typically a semester). If they anticipated epistemic cognition was dynamic and subject to change over the course of a single class period, they would likely collect data more frequently to distinguish signal from noise.
Views on stability (whether implicit or explicit) could also be inferred from how authors treat the influence of context on epistemic cognition. If the authors described shifts in epistemic ideas in response to different prompts or comments from peers, the articles were coded as Unstable. Finally, if a study made no mention of how stable or unstable they considered epistemologies to be across time and context, it was coded as Unclear. In a few cases, epistemic cognition was treated as stable over time but unstable across contexts; these were also coded as Unclear.
Explicitness.
The third category of codes, explicitness, was used to describe how data on epistemic cognition was obtained and interpreted. Some methods asked the participants to respond to direct questions about their epistemic ideas, which required them to consciously consider their own epistemic beliefs. The prompts often took the form of declarative statements, such as “Knowledge is obtained from authority,” which requires little interpretation on the part of the researcher. Other studies collected data in the form of written artifacts or classroom recordings, from which the researchers needed to infer epistemic cognition indirectly. Although the amount of inference required to make a claim about students’ epistemic ideas varies considerably, for the sake of simplicity, each type of data was simply coded as Explicit or Implicit based on how it was collected and analyzed. Since some articles utilized multiple data strands, the code Both was applied if both explicit and implicit methods of data collection and analysis were used.
Inferred model of cognition.
By considering the hierarchy, stability, and explicitness codes together, we were able to distinguish studies consistent with a developmental or dimensional model from those that were consistent with a resources model. When assigning a model to each study, we required all three codes to be consistent with that model. In addition, we tentatively assigned models to studies whose codes were mostly aligned with that model. For studies in which two codes were consistent with a single model and one was not associated with any model (i.e., Ambiguous, Unclear, or Both), we tentatively assigned the model based on the two matching codes. For example, a study coded as Ambiguous, Unstable, and Implicit was determined to be mostly aligned with a resources model. Studies that were coded as Hierarchical, Stable, and Implicit were considered mostly aligned with a developmental or dimensional model, since the association of these models with the Explicit code was based on literature trends rather than descriptions of the models themselves. For the remaining studies that did not fall into any of the categories described above, we were unable to assign a model as we did not have enough evidence or had contradictory evidence.
Establishing trustworthiness.
Initial coding was carried out by both authors. They individually coded ten articles at a time and then met to compare codes, resolve any discrepancies, and clarify the codebook. This was repeated three times. Subsequently, the first author read each article multiple times over the data analysis period, highlighting and annotating the parts of the text that provided evidence for each code assignment. The second author was brought in to discuss any codes the first author was unsure of.
There is no agreed upon method for establishing trustworthiness in qualitative research (Rolfe, 2006). We chose to establish trustworthiness primarily by recording a detailed decision trail, as recommended by Noble and Smith (2015). This was especially helpful for distinguishing codes based on the presence of evidence (hierarchical, variable utility, stable, unstable, explicit, implicit, both) from those based on lack of evidence (ambiguous, unclear). We chose not to report inter-rater agreement statistics because reaching an acceptable level of inter-rater agreement typically involves several rounds of code refinement on new subsets of data, which was impractical with our limited data set. Furthermore, this is consistent with several other chemistry education review articles (e.g., Bain et al., 2014; Flaherty, 2020; Hunter et al., 2022). The full coded data set with researcher notes may be found in the ESI.†
Findings
Part one
In the first part of our analysis, we sought to describe who was being studied and how they were studied in chemistry education epistemology research. We will report our findings for each category of codes and describe examples of each.
The majority of studies focused on students in first-year chemistry courses and pre-service teachers.
Fig. 2 displays the distribution of Sample codes, which describe the chemistry courses from which study participants were drawn. First-year chemistry students were the most studied population among the studies included in this review. Twenty studies sampled students from lecture courses while seven sampled students from the lab component specifically. There was some variation as to the students who were taking these first-year chemistry classes. Some were courses designed for non-majors, some were designed for STEM majors, and others were open to all majors. Pre-service teachers enrolled in chemistry or science courses for pre-service teachers were the second-most common group studied (N = 7). Four studies recruited students who were studying to become chemistry teachers specifically (Ağlarcı et al., 2016; Sendur et al., 2017; Venessa et al., 2019; Çelik, 2020). The other three studies sampled pre-service elementary (or primary) school teachers enrolled in chemistry or science courses specifically designed for them (McDonald, 2010; Çalik and Cobern, 2017; Crujeiras-Pérez and Brocos, 2021). Several studies recruited participants from organic chemistry lecture (N = 6) or laboratory (N = 3) courses. Just three studies involved students from upper-level chemistry courses; interestingly, these were all physical chemistry lecture or laboratory courses. Other studies sought to understand chemistry students’ epistemic cognition outside of the context of a specific class and recruited students from all levels of chemistry (e.g., Li et al., 2013; Sevian and Couture, 2018) or compared students enrolled in introductory and organic chemistry courses (e.g., Hofer, 2004; Mazzarone and Grove, 2013).
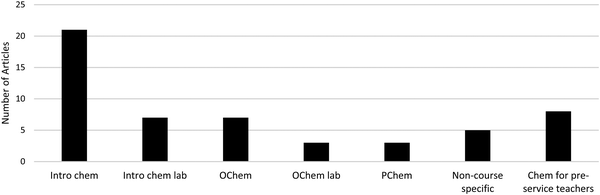 |
| Fig. 2 Distribution of sample codes. | |
The reasons offered for choosing a particular course to study varied. For studies focused on assessing the impact of an intervention (see below), course selection depending on where the intervention was being implemented. Sometimes these interventions were initiated by an individual instructor; other times they were part of a larger department initiative (e.g., Chopra et al., 2017). Some studies on pre-service teachers’ epistemic cognition were in part motivated by the role of teachers in shaping students’ perspectives on chemistry and science more broadly. As Ağlarcı et al. (2016) argue, “science education programs and teachers play a key role in [improving citizens’ images of science], as they are mostly responsible for educating people.”
Most studies examined personal epistemology rather than social epistemology.
As shown in Fig. 3, most studies (N = 38, 72%) collected data from individual students. Individuals responded to surveys, participated in interviews, or wrote their own lab reports or essays. These were used to make inferences about the individual's epistemic cognition. Ten studies characterized aspects of epistemology that belonged to a group of students (Fig. 3). These tended to be studies that focused on group dialogue, especially argumentation. Six studies collected data on individuals as well as groups of students (Fig. 3). For example, Walker et al. (2019) studied argumentation and included in-person argumentation (group dialogue) as well as written arguments (individual lab reports). These results demonstrate that the interplay between students, instructors, and the cultural context in which learning occurs is understudied in chemistry education research on epistemology and may be a productive avenue for future research.
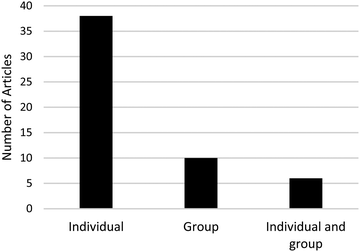 |
| Fig. 3 Distribution of unit of study codes. | |
Exploratory qualitative studies are the most common types of studies on students’ epistemic cognition.
Nearly half (N = 24) of the studies surveyed were classified as exploratory (Fig. 4). Twenty of these were qualitative while the remaining four used a mixed methods approach. The targets of exploration varied considerably. Some focused on specific aspects of epistemic cognition such as students’ epistemic stances when evaluating models (Kelly et al., 2021) or what students considered acceptable justifications for their knowledge products (Becker et al., 2013; Crujeiras-Pérez and Brocos, 2021). Others characterized epistemic cognition more broadly by attending to students’ perspectives on the nature of science (e.g., Venessa et al., 2019; Agustian, 2020).
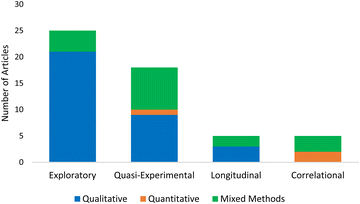 |
| Fig. 4 Distribution of study design codes, separated by Methodology code. Blue corresponds to qualitative studies, yellow corresponds to quantitative studies, and green corresponds to mixed methods studies. | |
A large portion (N = 19) of studies were carried out to assess the impact of a curricular or pedagogical intervention (Fig. 4). Ten of these were qualitative, eight were mixed methods, and one was quantitative. Interventions included using explicit approaches to teaching nature of science (e.g., Çelik, 2020), incorporating inquiry-based laboratory experiments (e.g., Russell and Weaver, 2011), and implementing a new curriculum (e.g., Bowen et al., 2022). Most used a pre-post design in which data was collected from students before and after the intervention. Some of these involved a control group and a treatment group while some only had a treatment group. A few studies only collected data after the intervention.
Five longitudinal studies were present in the sample (Fig. 4). Three were qualitative, and two were mixed methods. Two of these sought to understand how students’ understanding of argumentation, including epistemic aspects, changed as the students participated in an argument-driven inquiry general chemistry laboratory course (Walker and Sampson, 2013; Hosbein et al., 2021). The remaining three examined students’ epistemic cognition more generally, either over two semesters of organic chemistry (Grove and Bretz, 2010; Grove and Bretz, 2012) or over the general chemistry and organic chemistry sequence (Mazzarone and Grove, 2013).
Correlational studies made up the remaining five studies (Fig. 4). In some, the epistemic variable was general. For example, Lee et al. (2022) looked at the relationships among epistemic beliefs, engagement in a flipped chemistry class, and learning outcomes. Other studies examined epistemic variables grounded in chemistry. Li et al. (2013) compared students’ conceptions of learning chemistry to their approaches to learning chemistry and found some correlation. Aguirre-Mendez et al. (2020), measured the relationship between argumentation quality and gains in chemistry content knowledge. All of the correlational studies were quantitative or mixed methods.
Data on students’ epistemic cognition was collected through a variety of methods.
No clear preferences for one method of data collection over others were found in the reviewed articles, as shown in Fig. 5. Interviews (N = 25) and open-ended surveys (N = 21) were the most common approaches to eliciting data on epistemic cognition. Written artifacts (N = 14) and classroom recordings (N = 13) were slightly less common, although both were still used in over a quarter of the studies. Selected-response surveys (N = 6) were the least common means of data collection. Thirty-four studies relied on a single method of data collection while 19 elicited data using more than one method. Written artifacts were mostly used in combination with other data sources while selected-response surveys tended to be used alone. Interviews, open-ended surveys, and classroom recordings were used approximately evenly as the sole data source or in tandem with other sources. Given the complex nature of epistemic cognition, considering the pros and cons of what each data source can offer seems prudent when designing a study.
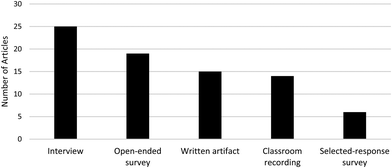 |
| Fig. 5 Methods of data collection. The total number exceeds the number of articles because some studies collected multiple types of data. | |
Part two
In the second phase of our analysis, we coded for assumptions about the hierarchy and stability of epistemic ideas as well as the extent to which epistemic ideas were asked for directly during data collection or inferred during data analysis. We will report our findings for each category of codes and describe examples of each.
Most studies characterized students’ epistemologies in a hierarchical manner.
The vast majority of articles (N = 39, 72%) interpreted students’ epistemologies using non-contextualized hierarchical coding schemes (Fig. 6). The Views on the Nature of Science (VNOS) instrument (Lederman et al., 2002) used in nine of the studies interprets responses as naïve or informed (or somewhere in between, depending on the particular study). Other studies employed Likert scale survey items in which higher (or sometimes lower) scores were indicative of more expert-like responses. For example, Shultz and Gere (2015) asked general chemistry students to respond to the question, “When two different theories arise to explain the same phenomenon, what should scientists do?” The students were given several responses to this question which they were asked to rate on a five-point Likert scale from strongly disagree to strongly agree. Students who strongly agree with the statement, “Scientists should not accept any theory before distinguishing which is best through the scientific method because there is only one truth about phenomenon” were classified as having naïve views on the nature of science as it relates to the certainty of knowledge. Conversely, students who strongly disagreed with the statement were characterized as having sophisticated views related to certainty of knowledge.
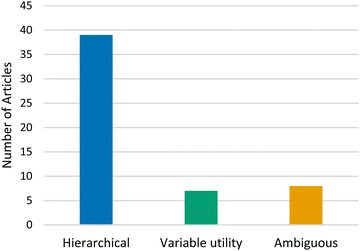 |
| Fig. 6 Distribution of hierarchy codes. Hierarchical (blue) aligns with a developmental or dimensional model. Variable utility (green) aligns with a resources model. | |
Far fewer studies (N = 7, 13%) explicitly stated that different epistemologies were useful in different circumstances. Three of these invoked a resources model of epistemic cognition as part of their theoretical frameworks. For example, Rodriguez, Bain, et al. (2020) used the framework of epistemic games to analyze how students solve kinetics problems during think-aloud interviews. In their discussion of the results, the authors state, “Thus, specific epistemic games are not problematic on their own, but issues arise when students have difficulty switching between games.” One epistemic game is not inherently better than another, but one might be more appropriate for a specific use or type of problem than another. This attention to context and the productive use of knowledge results in quite different implications for teaching. Instead of channeling students toward a single epistemic belief or set of beliefs, the emphasis is on helping students recognize which are appropriate for the problem at hand.
A particularly interesting example of a study coded as Variable utility was offered by Becker et al. (2013). They explored sociochemical norms for reasoning in a physical chemistry course and found that the class tended to value particulate-level explanations for thermodynamic properties of chemical systems. Despite describing what counted as “good” justifications, this study was coded as Variable utility because the participants, rather than the researchers, established what was considered appropriate and inappropriate evidence. Furthermore, what counted as a “good” justification varied somewhat; for some arguments, acceptable justifications were based on mathematical reasoning. Thus, this study demonstrates how epistemic ideas can be evaluated in context and reports on how the classroom community itself, rather than the researchers, characterize their own knowledge.
Interestingly, all of the studies coded as Variable utility were qualitative, and most focused on a single episode of problem-solving, either in a classroom or interview setting. While it is difficult to imagine a quantitative study that would be coded as Variable utility, a mixed methods study could in principle be useful for synthesizing these individual moments over the course of a semester or comparing across classes.
There were also some studies (N = 8, 15%) in which no judgements were made regarding the value of the epistemic ideas elicited. For example, Talanquer (2010) categorized students’ explanations in terms of their structures as non-causal, macrocausal (additive or interactive), and microcausal (additive or static). It was unclear, however, whether particular types of explanation structures were more desirable than others.
An approximately equal number of studies treated epistemology as stable versus unstable.
Stability was the second feature we looked for to aid in inferring the model of epistemic cognition for each paper. We found less evidence on which to make claims about stability than we found for hierarchy, resulting in 16 (30%) coded as Unclear (Fig. 7). Of the remaining studies, 19 (35%) were coded as Stable and 19 (35%) were coded as Unstable (Fig. 7).
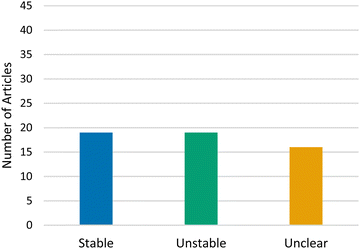 |
| Fig. 7 Distribution of stability codes. Stable (blue) aligns with a developmental or dimensional model. Unstable (green) aligns with a resources model. | |
Studies that utilized a pre-/post-test design made up the majority in the Stable category. Often these kinds of study designs, which were common in our data set, are used to evaluate the effect(s) of an intervention. Examples of interventions related to epistemology or the nature of science include implementation of direct instruction on history of science or nature of science (e.g., Ağlarcı et al., 2016), participation in argument-driven inquiry labs (e.g., Hosbein et al., 2021), and metacognitive interventions (e.g., Saribas et al., 2013).
In the Unstable category, instability was often connected to the context sensitivity observed. For example, Lazenby et al. (2020) conducted a study on students’ epistemic criteria for scientific models and found that “although students’ conceptual resources are potentially productive, they are highly sensitive to context, as evidenced by the variation in themes across domain-general and chemistry-specific tasks.” They noticed that students invoked different criteria depending on the type of model they were thinking about in that moment.
Studies that were coded as Unclear are somewhat difficult to describe as this code was largely based on the absence of evidence rather than the presence of certain features. Typically, these studies involved data collection at a single timepoint and did not discuss how context may have influenced the data. A few studies that were coded as Unclear described epistemic cognition as stable over time but unstable across contexts. For example, McDonald (2010) used a pre-/post-test experimental design to explore students’ views on the nature of science, implying stability over time. However, she observed that asking about nature of science in the context of socioscientific versus scientific contexts elicited different responses from some participants. She interpreted this discrepancy as evidence that students possess multiple epistemologies, some general and some specific to science, implying instability across contexts.
Data on students’ epistemologies was collected using an approximately equal number of explicit and implicit probes.
Finally, we examined how evidence of students’ epistemologies was elicited to provide insight into the model of cognition used. We asked ourselves, when reading about the method of data collection, “Would a student have to think consciously about their own epistemology to provide this data?” If the answer was yes, we coded it as Explicit and if the answer was no, we coded it as Implicit. In doing so, it was helpful to consider the manner in which data was collected. Therefore, we present the results accordingly.
Approximately half of the studies included in our sample collected data using only explicit probes (N = 25, 46%). Most studies that collected data by administering surveys were coded as Explicit (Fig. 8). Many selected-response surveys, like the CHEMX survey used by Mazzarone and Grove (2013), asked students the extent to which they agreed with statements like “Knowledge in chemistry consists of many pieces of information, each of which applies primarily to a specific situation.” Other selected-response surveys asked students to choose the option that most closely aligned with their views. For example, Venessa et al. (2019) used a mostly multiple-choice survey to ask students about purpose of science, the nature of scientific knowledge, and the relationship between science and technology.
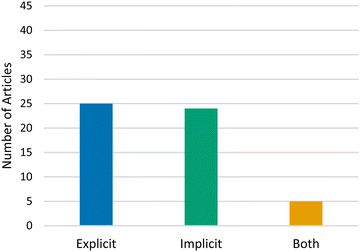 |
| Fig. 8 Distribution of explicitness codes. Explicit (blue) aligns with a developmental or dimensional model. Implicit (green) aligns with a resources model. | |
Explicit items were often found on open-ended surveys and in interview protocols as well. The VNOS survey was used in nine different studies and contained explicit questions like, “What, in your view, is science? What makes science (or scientific discipline such as physics, biology, etc.) different from other disciplines of inquiry (e.g., religion, philosophy)?” Similar kinds of questions were asked in interviews. For example, Havdala and Ashkenazi (2007) asked questions like “How would you define science?” and “Is there any way to find objectivity or certainty in science?” in their interviews with students enrolled in a general chemistry laboratory course. Like the selected-response surveys, these questions require a person to consciously consider the ways in which they think about and/or use knowledge.
Twenty-four studies (44%) were coded as Implicit (Fig. 8). These studies largely collected data in the form of written artifacts or classroom recordings. Written artifacts included reflective essays (e.g., Grove and Bretz, 2012), argumentative writing assignments (e.g., Moon et al., 2019), and lab reports (e.g., Petritis et al., 2021). Classroom recordings typically captured student dialogue as they engaged in problem-solving (e.g., Wickman, 2004) and/or argumentation (e.g., Walker et al., 2019). These sources of data typically provided information about the structure of knowledge or justifications for knowledge. Scientific arguments, written or verbal, were commonly analyzed for the presence of and relationships between claims, evidence, and reasoning, originally derived from Toulmin's model of an argument (1958).
The Implicit code was also applied to some studies that used open-ended surveys and interviews. These typically contained questions that asked students to reflect on their experiences in class or engage in problem-solving. For example, one of the questions Bowen et al. (2022) asked students was “What would you tell [a student thinking about enrolling in organic chemistry] is the most difficult thing about organic chemistry?” Some of the responses to this question were epistemic in nature and revealed challenges related to obtaining or using knowledge. Kelly et al. (2021) conducted interviews in which they first asked students to watch a video on precipitation reactions, then think aloud as they completed a card sort and modeling task to describe the mechanism of precipitation, and finally critique three mechanistic animations for their scientific accuracy. From these interviews, Kelly et al. inferred students’ epistemic activities, such as comparing and modeling, and their epistemic stances, such as doubting or puzzlement.
Twenty-one studies collected multiple strands of data, but only five studies (9%) used a combination of explicit and implicit methods of data collection and received the code Both (Fig. 8). For example, McDonald (2010) surveyed students about their views on the nature of science using the VNOS (Explicit) but also looked at students’ written and verbal scientific arguments surrounding scientific issues (Implicit). Grooms (2020) also combined students’ written scientific arguments (Implicit) with a survey that asked students explicitly about their epistemic ideas related to argumentation (e.g., What is evidence?).
A model of epistemic cognition can be inferred for some studies.
As mentioned previously, most researchers did not frame their studies through the lens of a particular model of epistemic cognition. By considering the set of codes each study received, we acquired some evidence for what model was tacitly informing each study. Fig. 9 depicts the relative number of co-occurrences for each coding combination to help visualize the relationships between codes.
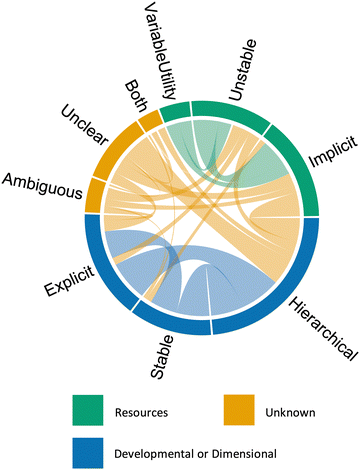 |
| Fig. 9 Chord diagram depicting connections between codes. The width of each link is proportional to the number of studies that received the two codes connected by the link. Blue codes align with a developmental or dimensional model. Green codes align with a resources model. Yellow codes do not align with any model. | |
Developmental and dimensional models were characterized by Hierarchical, Stable, and Explicit codes (Fig. 8). Fifteen studies received these codes, completely aligning with a developmental or dimensional model. An additional eight mostly aligned with these models. Three were coded as Hierarchical, Stable, and Implicit or Both, and five were coded as Hierarchical, Unclear, and Explicit. In total, 23 studies seemed to be informed by a developmental or dimensional model (Fig. 9).
The resources model asserts that epistemic cognition is context-dependent, dynamic, and largely implicit. Thus, an article using a resources model to frame the study should receive codes of Variable utility, Unstable, and Implicit (Fig. 9). Three of the seven studies that reported a resources model were assigned these codes. Of the remaining four studies, two were coded as Ambiguous, Unstable, and Explicit; one was coded as Ambiguous, Unstable, and Both; and one was coded as Hierarchical, Unstable, and Explicit. Four studies that did not report using a model of epistemic cognition received the codes aligned with a resources model (i.e., Variable utility, Unstable, Implicit). An additional three studies were coded as Ambiguous, Unstable, and Implicit, mostly aligning with a resources model. In total, seven studies aligned completely with a resources model and three studies mostly aligned (Fig. 10).
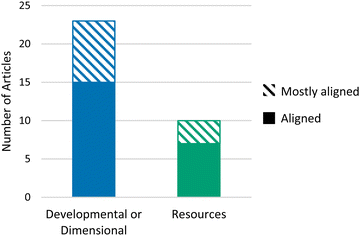 |
| Fig. 10 Models of epistemic cognition assigned based on hierarchy, stability, and explicitness codes. Solid bars represent articles that align with a model in all three codes. Striped bars represent articles that align with a model based on their Hierarchy and Stability codes. | |
The remaining 26 studies received coding combinations that did not clearly align with any model of epistemic cognition. Fifteen of these studies were coded as Hierarchical and Unclear with regards to stability. These were split approximately evenly between Explicit and Implicit codes. Six studies were coded as Hierarchical and Unstable. The remaining studies were either coded as Ambiguous and Unclear (N = 1), Ambiguous and Stable (N = 1), or Ambiguous and Unstable (N = 3). In total, a lack of evidence prevents us from inferring a model of cognition for nearly half of studies on student epistemologies in undergraduate chemistry education research published between 2000 and 2022.
Discussion and implications
Some of the findings reported above are unsurprising. For example, our field tends to study the epistemic cognition of individual students enrolled in introductory chemistry classes within the confines of an academic semester. We suspect this tendency reflects some combination of access to student populations, project timelines, and educational traditions that focus on individual (vs. community) learning. However, just because these sorts of studies have been done in the past does not mean that they represent the only, or best, way to approach exploring epistemic cognition. One might persuasively argue for the importance of longitudinal studies of community epistemic cognition – after all, people reason about scientific questions as members of their social and cultural groups and with other members of those groups across many contexts (Feinstein and Waddington, 2020). Such studies would of course require sustained funding and diverse expertise (e.g., science education, science and technology studies).
Regardless of the sample and duration of epistemology-focused studies, researchers will be faced with the choice of collecting data that requires conscious articulation/selection of epistemic ideas or observing behavior to infer the epistemic cognition underlying that behavior. The roughly equal distribution of Explicit and Implicit codes in our data suggests that researchers see advantages and disadvantages to both approaches. Explicit survey or interview questions provide information about the participants’ perceptions of their own epistemic cognition. Data analysis is also relatively straightforward; it requires little interpretation of participants’ responses. This allows researchers to collect data on large numbers of students and perform statistical analyses. Sandoval and Çam (2011) argue, however, that students may experience the context of an interview or survey as substantially different from the context of behaviors researchers are interested in. As such, ideas about knowing and learning activated when responding to a survey or interview question may not map onto epistemologies that underlie behavior in class or in life. This makes it difficult to make reasonable claims about student behavior or suggest classroom interventions based on survey response data alone.
Implicit measurements inherently require the researcher to make more inferences. Rarely does a student say something like, “My epistemic aim at this moment is to avoid obtaining false beliefs.” Instead, they might say something like, “I don’t think that is right. Let's check with the professor,” from which we can perhaps infer an epistemic aim of avoiding false beliefs and the professor as a source of knowledge. As a result, data analysis is more complicated and time-intensive for data collected via implicit measurements. This makes study designs employing these approaches to data collection and analysis less practical for large sample sizes and more difficult for a practitioner to use to evaluate their classes. However, they can offer a more nuanced, context-sensitive picture of students’ epistemologies than explicit measurements because they can capture epistemology in use, i.e., “practical epistemologies” (Sandoval, 2005; Berland et al., 2016).
As with the Explicitness codes, we saw an approximately even distribution of Stability codes. This category of codes was challenging to apply given that few researchers discussed their assumptions of stability or instability, resulting in a large portion of studies coded as Unclear. We argue, however, that this assumption influences the design of the study and the interpretation of data. Administering pre- and post-tests before and after an intervention, for example, would be reasonable if one assumes students’ responses indicate relatively stable epistemic ideas that were expected to persist in the absence of the intervention. But if one assumes students’ responses indicate epistemic ideas invoked in the moment, which may or may not be deeply held, then one would be cautious about attributing any changes in responses to the intervention. Thus, a major takeaway of this review is that future researchers should address assumptions of stability with regard to epistemic cognition.
The most striking finding, we claim, was that more than 70% of studies performed a hierarchical analysis of students’ epistemic cognition data. We hypothesize that Hierarchical studies are so prevalent because creating hierarchies seems intuitive and the results of hierarchical analyses lend themselves to relatively straightforward interpretations. By placing students on a continuum from “naïve” to “expert” epistemic cognition, we can judge how/whether an intervention was successful in supporting hoped-for improvements. We have two major objections to employing context-invariant hierarchies: (1) the assumption that one set of epistemic ideas is best in all circumstances is not reasonable, and (2) assembled hierarchies nearly always position an idealized vision of White, Western norms as most sophisticated and de-value or ignore other powerful and legitimate ways of knowing and learning.
Overemphasis on students advancing toward and achieving the “best” epistemologies may overlook the ways in which other epistemologies could prove useful and act to marginalize whole groups of students. In some articles, students were considered naïve for thinking that there would be a single correct answer. A quick reflection on how we use knowledge, both in chemistry and everyday life, should reveal why equating binary thinking with epistemic immaturity is overly simplistic. Sometimes it is useful to adopt a binary perspective, such as when assessing if you made the desired pharmaceutical compound or its toxic enantiomer. The danger of an inflexible, hierarchical view is that descriptors or measurements of epistemic cognition may be interpreted as value-laden traits of the students themselves, creating difference among groups of students that can be used to justify inequitable treatments of those groups (Kirchgasler, 2017). One might, for example, make claims that students who are identified as “less sophisticated” dualistic thinkers are less capable of engaging in chemistry courses than students who are identified as “more sophisticated” relativist thinkers. This could be used to justify separate tracks whereby “less sophisticated” students are assigned to a “lower level” course. Thus, a rigidly hierarchical viewpoint of epistemology may in fact lead to educational policies that restrict who is allowed to continue studying science.
Furthermore, by defining a universal best epistemology, almost always based on White, Western norms, we ignore or devalue powerful and legitimate systems of knowing that exist in other cultures (Ladson-Billings, 2000). As a consequence, Bang and Medin (2010) assert, “In education, most epistemology research makes the assumption that the epistemologies students come to classrooms with are inferior, or less productive, compared with the one(s) that research and educators (for our purposes, science education) are trying to assist students in learning.” They go on to discuss how such a perspective devalues ways of knowing that Native American communities possess. The prevalent use of hierarchical coding schemes in chemistry education research is consistent with their assertion about science education research generally. Such a view discounts the productive resources that all students possess, but especially those who are already marginalized by our education systems and thus under-represented in science. Adopting a perspective that values multiple ways of constructing and evaluating knowledge is one way that chemistry educators can work toward creating more equitable learning environments.
Saying that “all epistemologies may have utility in some context” should not be read as implying “all epistemologies are equally useful in all contexts”. Most scholars who ascribe to a resources view of epistemic cognition acknowledge the existence of situated epistemological hierarchies, in which some ways of knowing and learning may be particularly useful in advancing toward a particular aim in a given moment. This means that “evaluations of epistemological sophistication must account for the appropriateness and utility of epistemological resources being used in the current context” (Elby and Hammer, 2001; Hammer and Elby, 2002; Berland and Crucet, 2016, p. 10). Theoretical and analytic work of this sort is far from straightforward. One must grapple with questions such as: How should we make arguments that epistemologies are more/less useful without an a priori set of “best epistemologies”? How might we define a “context” for the purposes of this sort of analysis, given that epistemologies can shift over a very short time scale? How should we think about the interplay between instructors’ epistemic learning goals, the design of a learning environment, and ways of knowing and learning students experience as valuable in that environment? Conversations around questions such as these are ongoing in the science education community (e.g., Warren et al., 2020; Pierson et al., 2023), but still fairly rare in the context of college chemistry learning. We are hopeful this article serves to spark more conversations around how and why we define “epistemological sophistication” in the ways that we do.
Limitations
In searching for and selecting articles to include in this review, it was necessary to make decisions to restrict the scope. The search terms “epistem*,” “nature of science,” and “beliefs” were used to find articles, but articles employing other terms to describe students’ thinking about chemistry knowledge may have been missed. Searches were performed in prominent chemistry education and science education journals, as well as a few databases, but nevertheless, some relevant articles may have eluded us. Finally, we chose to restrict our analysis to articles involving students in undergraduate chemistry courses. We do not know if studies on other populations, such as secondary school students, secondary school teachers, graduate students, or college instructors, would exhibit the same trends.
Like all coding schemes, the coding scheme presented in Table 3 is a tool to summarize and interpret the data, albeit at the cost of some resolution. With only three codes per category, much of the variation is obscured. This was especially true for the coding category Explicitness. Among articles coded as Implicit, the degree of inference required ranges depending on the exact nature of the data collection methods. For example, an interview asking students to reflect on their experiences in a course is not the same as observing them as they solve problems in a small group.
Furthermore, in applying our coding scheme, we needed to make decisions based only on what was published in the articles. At the time of this writing, the field has not established agreed-upon guidelines regarding what information should be including in publications on students’ epistemic cognition. For example, assumptions about the stability of epistemic ideas are not usually stated, resulting in a large number of articles coded as Unclear. Thus, much of our coding relied on inferences drawn from the theoretical framework invoked, the data collection and analysis methods used, and the conclusions drawn. It is possible our interpretations do not match the authors’ intended meanings.
In developing our coding scheme, we chose to attend to what we perceive as some of the important assumptions embedded in the various theoretical models of epistemic cognition. Other assumptions were not operationalized in our coding scheme. An example is the extent to which epistemic cognition is domain general or domain specific. (These ideas were incorporated less rigorously into our discussion of stability.)
Conclusions and future directions
The model of epistemic cognition researchers employ informs all aspects of a study, from the research questions that can be asked to the implications and conclusions that can be drawn. We reviewed articles on students’ epistemic cognition in undergraduate chemistry courses and found that very few articles described their model of epistemic cognition, so instead we looked for distinguishing characteristics of developmental or dimensional models versus resources models (i.e., assumptions about hierarchy, stability, and explicitness). From this analysis, we were able to tentatively infer that one third of studies were informed by a developmental or dimensional model, one fifth were informed by a resources model, and the remaining half remained too ambiguous to infer a model. We hope that the coding schemes for hierarchy, stability, and explicitness described here can serve as tools for researchers to support alignment between theory, methods, and implications with regard to epistemic cognition.
Developmental and dimensional models have played an important role in enabling research and discussion regarding the epistemic aspect of students’ education. We argue that a resources model takes into account many of the ideas put forward in these models (e.g., kinds of epistemic knowledge) but incorporates them into a more modern understanding of the dynamic and highly context-dependent nature of cognition (diSessa, 1988; Hammer and Elby, 2002). Furthermore, not only has a resources model been shown to better account for data (Hammer and Elby, 2002), but it does not require the researcher to impose a Eurocentric value system when analyzing the data. Rather, a resources model allows researchers to treat ways of knowing from marginalized communities as valid and valuable. But employing a resources model brings its own set of theoretical questions and methodological challenges. How do we collect and analyze data on large samples in a nuanced way? How does individual resource activation influence collective ideas on knowledge construction and evaluation and vice versa? Who decides (and who should decide) which epistemic resources are useful in a particular context and to whom? Science education researchers have started to grapple with some of these questions, especially those related to collective knowledge construction and epistemic agency in K–12 education (e.g., Stroupe, 2014; Ko and Krist, 2019; González-Howard and McNeill, 2020). We hope that future work on epistemic cognition in chemistry education will explore these questions as well so that we may better understand and support students in constructing, using, and evaluating knowledge in ways that are meaningful in daily life.
Data availability
The list of articles included in the sample, details of the selection process, and coded data can be found at https://osf.io/y94fm/?view_only=89fe46421b894cbba7d5e2cdfbd6b389.
Conflicts of interest
There are no conflicts to declare.
Acknowledgements
The authors would like to thank Brie Bradshaw, Cara Schwarz, Brian Esselman, Nicole Greco, and Lindsay Wells for their helpful conversations and feedback. We gratefully acknowledge support from the U.S. National Science Foundation under Grant No. DUE 2225025. Any opinions, findings, and conclusions or recommendations expressed in this manuscript are those of the authors and do not necessarily reflect the views of the National Science Foundation.
Notes and references
- Adams W. K., Wieman C. E., Perkins K. K. and Barbera J. (2008), Modifying and validating the Colorado Learning Attitudes about Science Survey for use in chemistry, J. Chem. Educ., 85(10), 1435 DOI:10.1021/ed085p1435.
- Ağlarcı O., Sarıçayır H. and Şahin M., (2016), Nature of science instruction to Turkish prospective chemistry teachers: the effect of explicit-reflective approach, Cogent Educ., 3(1), 1213350 DOI:10.1080/2331186X.2016.1213350.
- Aguirre-Mendez C., Chen Y.-C., Terada T. and Techawitthayachinda R., (2020), Predicting components of argumentative writing and achievement gains in a general chemistry course for nonmajor college students, J. Chem. Educ., 97(8), 2045–2056 DOI:10.1021/acs.jchemed.0c00042.
- Agustian H. Y., (2020), Students’ understanding of the nature of science in the context of an undergraduate chemistry laboratory, Electronic J. Res. Sci. Math. Educ., 24(2), 56–85.
- American Association for the Advancement of Science, (2011), Vision and change in undergraduate biology education: A call to action.
- Bain K., Moon A., Mack M. R. and Towns M. H., (2014), A review of research on the teaching and learning of thermodynamics at the university level, Chem. Educ. Res. Pract., 15(3), 320–335 10.1039/C4RP00011K.
- Bang M. and Medin D., (2010), Cultural processes in science education: supporting the navigation of multiple epistemologies, Sci. Educ., 94(6), 1008–1026 DOI:10.1002/sce.20392.
- Becker N., Rasmussen C., Sweeney G., Wawro M., Towns M. and Cole R., (2013), Reasoning using particulate nature of matter: an example of a sociochemical norm in a university-level physical chemistry class, Chem. Educ. Res. Pract., 14(1), 81–94 10.1039/C2RP20085F.
- Berland L. K. and Crucet K., (2016), Epistemological trade-offs: accounting for context when evaluating epistemological sophistication of student engagement in scientific practices, Sci. Educ., 100(1), 5–29 DOI:10.1002/sce.21196.
- Berland L. K., Schwarz C. V., Krist C., Kenyon L., Lo A. S. and Reiser B. J., (2016), Epistemologies in practice: making scientific practices meaningful for students, J. Res. Sci. Teach., 53(7), 1082–1112 DOI:10.1002/tea.21257.
- Bing T. J. and Redish E. F., (2009), Analyzing problem solving using math in physics: epistemological framing via warrants, Phys. Rev. Spec. Top.--Accel. Beams, 5(2), 020108 DOI:10.1103/PhysRevSTPER.5.020108.
- Bowen R. S., Flaherty A. A. and Cooper M. M., (2022), Investigating student perceptions of transformational intent and classroom culture in organic chemistry courses, Chem. Educ. Res. Pract., 23(3), 560–581 10.1039/D2RP00010E.
- Buehl M. M. and Alexander P. A., (2001), Beliefs about academic knowledge, Educ. Psychol. Rev., 13(4), 385–418 DOI:10.1023/A:1011917914756.
- Çalik M. and Cobern W. W., (2017), A cross-cultural study of CKCM efficacy in an undergraduate chemistry classroom, Chem. Educ. Res. Pract., 18(4), 691–709 10.1039/C7RP00016B.
- Campbell D. T. and Stanley J. C., (1963), Experimental and quasi-experimental designs for research, Houghton Mifflin Company.
- Çelik S., (2020), Changes in nature of science understandings of preservice chemistry teachers in an explicit, reflective, and contextual nature of science teaching, Int. J. Res. Educ. Sci., 6(2), 315–326 DOI:10.46328/ijres.v6i2.892.
- Charters E., (2003), The use of think aloud methods in qualitative research an introduction to think aloud methods, Brock Educ. J., 12(2), 68–82.
- Chinn C. A., Buckland L. A. and Samarapungavan A., (2011), Expanding the dimensions of epistemic cognition: arguments from philosophy and psychology, Educ. Psychol., 46(3), 141–167 DOI:10.1080/00461520.2011.587722.
- Chopra I., O’Connor J., Pancho R., Chrzanowski M. and Sandi-Urena S., (2017), Reform in a general chemistry laboratory: How do students experience change in the instructional approach? Chem. Educ. Res. Pract., 18(1), 113–126 10.1039/C6RP00082G.
- Collins A. and Ferguson W., (1993), Epistemic forms and epistemic games: structures and strategies to guide inquiry, Educ. Psychol., 28(1), 25–42 DOI:10.1207/s15326985ep2801_3.
- Cooper M. and Klymkowsky M., (2013), Chemistry, life, the universe, and everything: a new approach to general chemistry, and a model for curriculum reform, J. Chem. Educ., 90(9), 1116–1122 DOI:10.1021/ed300456y.
- Crujeiras-Pérez B. and Brocos P., (2021), Pre-service teachers’ use of epistemic criteria in the assessment of scientific procedures for identifying microplastics in beach sand, Chem. Educ. Res. Pract., 22(2), 237–246 10.1039/D0RP00176G.
- Dempsey N. P., (2010), Stimulated recall interviews in ethnography, Qualitative Soc., 33(3), 349–367 DOI:10.1007/s11133-010-9157-x.
- diSessa A. A., (1988), Knowledge in pieces, in Constructivism in the computer age, G. Forman and P. B. Pufall (ed.) Lawrence Erlbaum Associates, Inc., pp. 49–70.
- Elby A. and Hammer D., (2001), On the substance of a sophisticated epistemology, Sci. Educ., 85(5), 554–567 DOI:10.1002/sce.1023.
- Entwistle N. J., (1991), Approaches to learning and perceptions of the learning environment, Higher Educ., 22(3), 201–204 DOI:10.1007/BF00132287.
- Feinstein N. W. and Waddington D. I., (2020), Individual truth judgments or purposeful, collective sensemaking? Rethinking science education's response to the post-truth era, Educ. Psychol., 55(3), 155–166 DOI:10.1080/00461520.2020.1780130.
- Flaherty A. A., (2020), A review of affective chemistry education research and its implications for future research, Chem. Educ. Res. Pract., 21(3), 698–713 10.1039/C9RP00200F.
- Ginsburg H. P., (1997), Entering the child's mind: The clinical interview in psychological research and practice, Cambridge University Press DOI:10.1017/CBO9780511527777.
- González-Howard M. and McNeill K. L., (2020), Acting with epistemic agency: characterizing student critique during argumentation discussions, Sci. Educ., 104(6), 953–982 DOI:10.1002/sce.21592.
- Greene J. A., Azevedo R. and Torney-Purta J., (2008), Modeling epistemic and ontological cognition: philosophical perspectives and methodological directions, Educ. Psychol., 43(3), 142–160 DOI:10.1080/00461520802178458.
- Grooms J., (2020), A comparison of argument quality and students’ conceptions of data and evidence for undergraduates experiencing two types of laboratory instruction, J. Chem. Educ., 97(8), 2057–2064 DOI:10.1021/acs.jchemed.0c00026.
- Grove N. P. and Bretz S. L., (2010), Perry's Scheme of Intellectual and Epistemological Development as a framework for describing student difficulties in learning organic chemistry, Chem. Educ. Res. Pract., 11(3), 207–211 10.1039/C005469K.
- Grove N. P. and Bretz S. L., (2012), A continuum of learning: from rote memorization to meaningful learning in organic chemistry, Chem. Educ. Res. Practice, 13(3), 201–208 10.1039/C1RP90069B.
- Hammer D. and Elby A., (2002), On the form of a personal epistemology, in Personal epistemology: The psychology of beliefs about knowledge and knowing, B. K. Hofer and P. R. Pintrich (ed.), pp. 169–190.
- Hammer D., Elby A., Scherr R. E. and Redish E. F., (2005), Resources, framing, and transfer, in Transfer of learning from a modern multidisciplinary perspective, J. P. Mestre (ed.) pp. 89–119.
- Havdala R. and Ashkenazi G., (2007), Coordination of theory and evidence: effect of epistemological theories on students’ laboratory practice, J. Res. Sci. Teach., 44(8), 1134–1159.
- Hofer B. K., (2004), Exploring the dimensions of personal epistemology in differing classroom contexts: student interpretations during the first year of college, Contemp. Educ. Psychol., 29(2), 129–163 DOI:10.1016/j.cedpsych.2004.01.002.
- Hofer B. K. and Pintrich P. R., (1997), The development of epistemological theories: beliefs about knowledge and knowing and their relation to learning, Rev. Educ. Res., 67(1), 88–140 DOI:10.3102/00346543067001088.
- Hosbein K. N., Lower M. A. and Walker J. P., (2021), Tracking student argumentation skills across general chemistry through Argument-Driven Inquiry using the Assessment of Scientific Argumentation in the Classroom observation protocol, J. Chem. Educ., 98(6), 1875–1887 DOI:10.1021/acs.jchemed.0c01225.
- Hunter K. H., Rodriguez J.-M. G. and Becker N. M., (2022), A review of research on the teaching and learning of chemical bonding, J. Chem. Educ., 2022, 99(7), 2451–2464 DOI:10.1021/acs.jchemed.2c00034.
- Johnson R. B. and Christensen L., (2020), Educational research: Quantitative, qualitative, and mixed approaches, 7th edn, SAGE Publications, Inc.
- Kelly R. M., Akaygun S., Hansen S. J. R., Villalta-Cerdas A. and Adam J., (2021), Examining learning of atomic level ideas about precipitation reactions with a resources framework, Chem. Educ. Res. Pract., 22, 886–904 10.1039/D0RP00071J.
- King P. M. and Kitchener K. S., (1994), Developing reflective judgement: Understanding and promoting intellectual growth and critical thinking in adolescents and adults, Jossey-Bass.
- King P. M. and Kitchener K. S., (2004), Reflective judgment: theory and research on the development of epistemic assumptions through adulthood, Educ. Psychol., 39(1), 5–18 DOI:10.1207/s15326985ep3901_2.
- Kirchgasler K. L., (2017), Scientific Americans: Historicizing the making of difference in early 20th century U.S. science education, in T. A. Popkewitz, J. Diaz and C. Kirchgasler (ed.), A political sociology of educational knowledge, 1st edn, Routledge, pp. 87–102.
- Kitchener K. S., (1983), Cognition, metacognition, and epistemic cognition: a three-level model of cognitive processing, Human Dev., 26(4), 222–232 DOI:10.1159/000272885.
- Ko M. M. and Krist C., (2019), Opening up curricula to redistribute epistemic agency: a framework for supporting science teaching, Sci. Educ., 103(4), 979–1010 DOI:10.1002/sce.21511.
- Kuhn D., (1999), A developmental model of critical thinking, Educ. Res., 28(2), 16–46 DOI:10.3102/0013189X028002016.
- Ladson-Billings G., (2000), Racialized discourses and ethnic epistemologies, Handbook of qualitative research, 2nd edn, SAGE Publications, Inc., pp. 257–277.
- Lazenby K., Stricker A., Brandriet A., Rupp C. A. and Becker N. M., (2020), Undergraduate chemistry students’ epistemic criteria for scientific models, J. Chem. Educ., 97(1), 16–26 DOI:10.1021/acs.jchemed.9b00505.
- Lederman N. G., (1992), Students’ and teachers’ conceptions of the nature of science: a review of the research, J. Res. Sci. Teach., 29(4), 331–359 DOI:10.1002/tea.3660290404.
- Lederman N. G., Abd-El-Khalick F., Bell R. L. and Schwartz R. S., (2002), Views of nature of science questionnaire: toward valid and meaningful assessment of learners’ conceptions of nature of science, J. Res. Sci. Teach., 39(6), 497–521 DOI:10.1002/tea.10034.
- Lee J., Park T. and Davis R. O., (2022), What affects learner engagement in flipped learning and what predicts its outcomes? British J. Educ. Technol., 53(2), 211–228 DOI:10.1111/bjet.12717.
- Li W.-T., Liang J.-C. and Tsai C.-C., (2013), Relational analysis of college chemistry-major students’ conceptions of and approaches to learning chemistry, Chem. Educ. Res. Practice, 14(4), 555–565 10.1039/C3RP00034F.
- Lising L. and Elby A., (2005), The impact of epistemology on learning: a case study from introductory physics, Am. J. Phys., 73(4), 372 DOI:10.1119/1.1848115.
- Louca L., Elby A., Hammer D. and Kagey T., (2004), Epistemological resources: applying a new epistemological framework to science instruction, Educ. Psychol., 39(1), 57–68 DOI:10.1207/s15326985ep3901_6.
- Mazzarone K. M. and Grove N. P., (2013), Understanding epistemological development in first- and second-year chemistry students, J. Chem. Educ., 90(8), 968–975 DOI:10.1021/ed300655s.
- McDonald C. V., (2010), The influence of explicit nature of science and argumentation instruction on preservice primary teachers’ views of nature of science, J. Res. Sci. Teach., 47(9), 1137–1164 DOI:10.1002/tea.20377.
- Momsen J., Offerdahl E., Kryjevskaia M., Montplaisir L., Anderson E. and Grosz N., (2013), Using assessments to investigate and compare the nature of learning in undergraduate science courses, CBE—Life Sci. Educ., 12(2), 239–249 DOI:10.1187/cbe.12-08-0130.
- Moon A., Moeller R., Gere A. R. and Shultz G. V., (2019), Application and testing of a framework for characterizing the quality of scientific reasoning in chemistry students’ writing on ocean acidification, Chem. Educ. Res. Pract., 20(3), 484–494 10.1039/C9RP00005D.
- Muis K. R., Bendixen L. D. and Haerle F. C., (2006), Domain-generality and domain-specificity in personal epistemology research: philosophical and empirical reflections in the development of a theoretical framework, Educ. Psychol. Rev., 18(1), 3–54 DOI:10.1007/s10648-006-9003-6.
- National Research Council, (2012), A framework for K-12 science education: Practices, crosscutting concepts, and core ideas, National Academies Press, p. 13165 DOI:10.17226/13165.
- Noble H. and Smith J., (2015), Issues of validity and reliability in qualitative research, Evidence Based Nursing, 18(2), 34–35 DOI:10.1136/eb-2015-102054.
- Perry, Jr. W. J., (1970), Forms of ethical and intellectual development in the college years: A scheme, Holt, Rinehart and Winston.
- Petritis S. J., Kelley C. and Talanquer V., (2021), Exploring the impact of the framing of a laboratory
experiment on the nature of student argumentation, Chem. Educ. Res. Pract., 22(1), 105–121 10.1039/D0RP00268B.
- Pierson A. E., Brady C. E., Clark D. B. and Sengupta P., (2023), Students’ epistemic commitments in a heterogeneity-seeking modeling curriculum, Cognition Instruction, 41(2), 125–157.
- Rodriguez J.-M. G., Bain K. and Towns M. H., (2020), The role of epistemology and epistemic games in mediating the use of mathematics in chemistry: implications for mathematics instruction and research on undergraduate mathematics education, Int. J. Res. Undergraduate Math. Educ., 6(2), 279–301 DOI:10.1007/s40753-019-00110-8.
- Rodriguez J.-M. G., Hunter K. H., Scharlott L. J. and Becker N. M., (2020), A review of research on process oriented guided inquiry learning: implications for research and practice, J. Chem. Educ., 97(10), 3506–3520 DOI:10.1021/acs.jchemed.0c00355.
- Rolfe G., (2006), Validity, trustworthiness and rigour: Quality and the idea of qualitative research, J. Adv. Nursing, 53(3), 304–310 DOI:10.1111/j.1365-2648.2006.03727.x.
- Rosenberg S., Hammer D. and Phelan J., (2006), Multiple epistemological coherences in an eighth-grade discussion of the rock cycle, J. Learn. Sci., 15(2), 261–292.
- Russ R. S., (2018), Characterizing teacher attention to student thinking: a role for epistemological messages, J. Res. Sci. Teach., 55(1), 94–120 DOI:10.1002/tea.21414.
- Russ R. S., Lee V. R. and Sherin B. L., (2012), Framing in cognitive clinical interviews about intuitive science knowledge: dynamic student understandings of the discourse interaction, Sci. Educ., 96(4), 573–599 DOI:10.1002/sce.21014.
- Russell C. B. and Weaver G. C., (2011), A comparative study of traditional, inquiry-based, and research-based laboratory curricula: impacts on understanding of the nature of science, Chem. Educ. Res. Practice, 12(1), 57–67 10.1039/C1RP90008K.
- Sandoval W. A., (2005), Understanding students’ practical epistemologies and their influence on learning through inquiry, Sci. Educ., 89, 634–656.
- Sandoval W. A. and Çam A., (2011), Elementary children's judgments of the epistemic status of sources of justification, Sci. Educ., 95(3), 383–408 DOI:10.1002/sce.20426.
- Sandoval W. A., Greene J. A. and Bråten I., (2016), Understanding and promoting thinking about knowledge: origins, issues, and future directions of research on epistemic cognition, Rev. Res. Educ., 40, 457–496.
- Saribas D., Mugaloglu E. Z. and Bayram H., (2013), Creating metacognitive awareness in the lab: outcomes for preservice science teachers, Eurasia J. Math., Sci. Technol. Educ., 9(1), 83–88 DOI:10.12973/eurasia.2013.918a.
- Schmitt F., (2017), Social Epistemology, in J. Greco and E. Sosa (ed.), The Blackwell guide to epistemology, John Wiley and Sons, Ltd, pp. 354–382 DOI:10.1002/9781405164863.ch15.
- Schommer-Aikins M., (2004), Explaining the epistemological belief system: introducing the embedded systemic model and coordinated research approach, Educ. Psychol., 39(1), 19–29 DOI:10.1207/s15326985ep3901_3.
- Scouller K. M., (1998), The influence of assessment method on students’ learning approaches: multiple choice question examination versus assignment essay, Higher Educ., 35(4), 453–472 DOI:10.1023/A:1003196224280.
- Scouller K. M. and Prosser M., (1994), Students’ experiences in studying for multiple choice question examinations, Studies Higher Educ., 19(3), 267–279 DOI:10.1080/03075079412331381870.
- Sendur G., Polat M. and Kazancı C., (2017), Does a course on the history and philosophy of chemistry have any effect on prospective chemistry teachers’ perceptions? The case of chemistry and the chemist, Chem. Educ. Res. Pract., 18(4), 601–629 10.1039/C7RP00054E.
- Sevian H. and Couture S., (2018), Epistemic games in substance characterization, Chem. Educ. Res. Pract., 19(4), 1029–1054 10.1039/C8RP00047F.
- Shultz G. V. and Gere A. R., (2015), Writing-to-learn the Nature of Science in the context of the Lewis dot structure model, J. Chem. Educ., 92(8), 1325–1329 DOI:10.1021/acs.jchemed.5b00064.
- Snyder B., (1973), The hidden curriculum, Cambridge, MA: The MIT Press.
- Stroupe D., (2014), Examining classroom science practice communities: How teachers and students negotiate epistemic agency and learn science-as-practice, Sci. Educ., 98(3), 487–516 DOI:10.1002/sce.21112.
- Talanquer V., (2010), Exploring dominant types of explanations built by general chemistry students, Int. J. Sci. Educ., 32(18), 2393–2412 DOI:10.1080/09500690903369662.
- Talanquer V., Bolger M. and Tomanek D., (2015), Exploring prospective teachers’ assessment practices: noticing and interpreting student understanding in the assessment of written work, J. Res. Sci. Teach., 52(5), 585–609 DOI:10.1002/tea.21209.
- Talanquer V. and Pollard J., (2017), Reforming a large foundational course: successes and challenges, J. Chem. Educ., 94(12), 1844–1851 DOI:10.1021/acs.jchemed.7b00397.
- Tiruneh D. T., Verburgh A. and Elen J., (2014), Effectiveness of critical thinking instruction in higher education: a systematic review of intervention studies, Higher Educ. Studies, 4(1), p1 DOI:10.5539/hes.v4n1p1.
- Toulmin S. E., (1958), The uses of argument, Cambridge University Press.
- Venessa D. M., Hernani H. and Halimatul H. S., (2019), Exploring view of nature of science and technology pre-service chemistry teachers, J. Sci. Learn., 3(1), 19–28 DOI:10.17509/jsl.v3i1.17757.
- Walker J. P. and Sampson V., (2013), Learning to argue and arguing to learn: argument-Driven Inquiry as a way to help undergraduate chemistry students learn how to construct arguments and engage in argumentation during a laboratory course, J. Res. Sci. Teach., 50(5), 561–596 DOI:10.1002/tea.21082.
- Walker J. P., Van Duzor A. G. and Lower M. A., (2019), Facilitating argumentation in the laboratory: the challenges of claim change and justification by theory, J. Chem. Educ., 96(3), 435–444 DOI:10.1021/acs.jchemed.8b00745.
- Warren B., Vossoughi S., Rosebery A. S., Bang M. and Taylor E. V., (2020), Multiple ways of knowing*: Re-imagining disciplinary learning, Handbook of the cultural foundations of learning, Routledge, pp. 277–294.
- White R. T. and Arzi H. J., (2005), Longitudinal studies: designs, validity, practicality, and value, Res. Sci. Educ., 35(1), 137–149 DOI:10.1007/s11165-004-3437-y.
- Wickman P.-O., (2004), The practical epistemologies of the classroom: a study of laboratory work, Sci. Educ., 88(3), 325–344 DOI:10.1002/sce.10129.
Footnotes |
† Electronic supplementary information (ESI) available. See DOI: https://doi.org/10.1039/d3rp00348e |
‡ King and Kitchener (2004) caution that participants’ views can fall into multiple categories, and that each stage is best understood as where the majority of their views lie. |
§ Note some scholars question whether the last two dimensions are actually epistemic. |
|
This journal is © The Royal Society of Chemistry 2024 |