Exploring diversity: student's (un-)productive use of resonance in organic chemistry tasks through the lens of the coordination class theory
Received
30th October 2023
, Accepted 17th January 2024
First published on 8th February 2024
Abstract
Resonance is a crucial concept in Organic Chemistry that enables both deriving chemical properties from molecular structures and predicting reactions by considering electron density distribution. Despite its importance for problem-solving and learning success, learners encounter various difficulties with this concept. Although prior research suggests that learners struggle to reason about resonance in problem-solving tasks, existing studies are often limited to singular contexts. Given that task approaches and reasoning are context-dependent, little is known about how learners use resonance across task contexts and which characteristics underlie productive concept use. To this end, a qualitative interview study was conducted, in which undergraduate chemistry students (N = 21), all beginners of Organic Chemistry, solved three organic case comparison tasks requiring the consideration of resonance. Through the analytical lens of the coordination class theory, we analysed the extent to which students used their representations of resonance structures, their task approaches, and the variety of resonance-related resource activation and connection in problem-solving across three different contexts. The results show that students’ use of resonance is diverse across the contexts. It can be characterized by a complex interplay of multiple factors reflecting the multifold processes when considering resonance. However, some essential characteristics of productive concept use in problem-solving (e.g., the activation of resources across different granularity levels) could be deduced. Implications for supporting learners’ use of resonance in problem-solving are discussed.
Introduction
Due to its visual nature, external representations play a key role in making sense of chemical phenomena and processes in Organic Chemistry. They carry the explanatory weight of the discipline and make imperceptible entities and processes visible since much implicit and explicit information (e.g., connectivity, polarity, geometry) is embedded within external representations (Hoffmann and Laszlo, 1991; Goodwin, 2008; Cooper et al., 2017; Graulich and Bhattacharyya, 2017). Representations such as molecular structures and mechanistic representations not only fulfil a displaying function but constitute a fundamental means for transmitting and constructing knowledge. They also serve as indispensable problem-solving tools. For instance, inferring molecular properties from representations can help to predict and weigh possible reaction pathways (Goodwin, 2008; Cartrette and Bodner, 2009; Cooper et al., 2017). Proficiency in the sensemaking of representations is crucial for successful reasoning in Organic Chemistry. Successfully engaging with representations, however, is not limited to interpreting given representations. In terms of representational competence, it is also important that learners are able to generate representations and use them in problem-solving (Kozma and Russell, 1997; Kozma and Russell, 2005). Constructing representations (e.g., drawing structural formulas) may provide diagnostic insights into one's mental models by externalizing ideas and may enhance one's chemical thinking (Gilbert, 2005; Van Meter and Garner, 2005; Cooper et al., 2017). Within these sensemaking processes, engaging with representations is always linked to considering and weighing different chemical concepts (Talanquer, 2022). For instance, to assess the acid strength of a compound, one maps and connects the visual features (e.g., functional groups) in a representation to relevant ideas such as pKa values or solvent effects. In turn, these ideas are derived by linking given structural features at a finer grain size (e.g., single atoms) to inductive effects, electronegativity, hybridization, hyperconjugation, or the size of the basic atom. It becomes evident that both the visual features in a representation and the related concepts mutually influence one another in the reasoning process via bottom-up (i.e., infer from the structure) and top-down (i.e., apply knowledge and concepts) processes (Kintsch, 1988; Kriz and Hegarty, 2007).
The resonance concept is closely related to generating and interpreting different representations (e.g., electron potential maps, structural formulas). It describes the delocalization of π electrons between multiple atoms in a conjugated system of a molecule. Usually, this is depicted by several Lewis structures that differ in the spatial arrangement of electrons among overlapping p-orbitals on adjacent atoms (i.e., resonance structures). The hybrid of all possible resonance structures can adequately describe the actual electronic structure of and bonding in a molecule. This is related to energetic considerations as the delocalization of π electrons contributes to the molecule's stabilization (i.e., resonance energy): the more electrons can be reasonably delocalized in a molecule, the lower the potential energy, and the more stable the molecule (Anslyn and Dougherty, 2006; Ogilvie et al., 2018). By constructing resonance structures, the hypothetical electron density distribution in molecules can be visualized, which helps to reason about preferred molecular structures or geometry, to derive chemical properties (e.g., acidity or stability), and to predict or justify reaction pathways (Richardson, 1986; Carle and Flynn, 2020). Moreover, other concepts, such as aromaticity or conjugation, build upon the resonance concept. Given that the resonance concept is pivotal for problem-solving and successful learning in Organic Chemistry, it is critical to understand how students understand and reason about resonance across contexts.
Students’ understanding and use of the resonance concept
Students’ conceptions of resonance.
Although students are expected to have a thorough understanding of resonance after introductory courses in Organic Chemistry and should be able to use this core concept fluently to depict the electronic structure of compounds, research in chemistry education has shown that the resonance concept puts a high cognitive load on learners (Duis, 2011; Brandfonbrener et al., 2021; Tetschner and Nedungadi, 2023). For instance, students have varied perspectives on resonance. Studies show that many students effortlessly recognize resonance structures and explain resonance by describing how to construct resonance structures (Brandfonbrener et al., 2021). Students also sometimes perceive resonance as a rapid exchange of electrons, and interpret resonance structures as equilibrium or as a structural change over time (Taber, 2002; Xue and Stains, 2020; Tetschner and Nedungadi, 2023). Additionally, students may consider resonance structures as distinct species or different perspectives of a molecule through picture analogies (Kim et al., 2019; Brandfonbrener et al., 2021). These findings indicate that learners have varying ways of understanding resonance, i.e., to interpret the static representations on paper as a dynamic electron distribution. Even though some of these ideas represent alternative conceptions, in parts, they touch upon aspects of the scientifically correct explanation of resonance. For instance, although the picture analogy does not reflect the idea that individual resonance structures do not exist as distinct structures, this conception indicates that students understand that single resonance structures do not provide a complete structural representation of a molecule (Brandfonbrener et al., 2021).
Connection with other concepts.
Understanding and using resonance necessitates that learners connect this concept with their prior knowledge (e.g., hybridization, charge separation) and other concepts (Betancourt-Pérez et al., 2010). Studies investigating students’ conceptual understanding of resonance show that students associate resonance with concepts such as stability or reactivity but often cannot appropriately link these concepts. As such, students often use these relationships as general statements or rules (Xue and Stains, 2020; Brandfonbrener et al., 2021). For instance, in a recent study eliciting students’ mental models on acid and base strength in Organic Chemistry (Demirdöğen et al., 2023), it became evident that the relationship between resonance and basicity remained unclear for some students. While they recognized the importance of resonance in assessing the base strength of molecules, their problem-solving often relied on broad, memorized statements (e.g., “the more resonance, the more stable”, p. 1134) (Demirdöğen et al., 2023). Brandfonbrener et al. (2021) furthermore showed that students describe the influence of resonance on reactivity in a more general sense, or establish the relationship between these two concepts by referring to common applications such as acid–base chemistry. While the latter shows that students can establish appropriate conceptual relationships, at least in some contexts, a restricted or overgeneralized view on these intertwined concepts may hinder subsequent problem-solving.
Construction of resonance structures.
Besides the necessary link to other concepts, students’ competence in constructing resonance structures is an indispensable prerequisite to correctly make assumptions about resonance and use it in various situations (Betancourt-Pérez et al., 2010; Carle and Flynn, 2020). A few studies targeting students’ drawing processes of resonance structures point out that students’ main challenges in drawing resonance structures are situated in identifying the correct place to start the movement of electrons, or arise from conceptual difficulties (e.g., adhering to the octet rule) (Betancourt-Pérez et al., 2010; Petterson et al., 2020). Related to these struggles, students with productive resonance structures show a more straightforward drawing process with more direct transitions between the previous and target drawing. In doing so, students with successful drawings adopt an analytical approach by focusing on and carefully assessing relevant, interrelated structural features (Braun et al., 2022).
Use of resonance in problem-solving.
Although studies emphasize resonance as an important concept to determine reaction pathways and to assess properties of molecules (e.g., Ferguson and Bodner, 2008), using this concept in practice (i.e., moving beyond the definition), might be difficult for students (Cartrette and Mayo, 2011). A recent investigation by Carle and colleagues (2020) on students' achievement of resonance-related learning outcomes revealed that only a few students spontaneously consider resonance in mechanistic tasks when they are not explicitly prompted to do so (Carle et al., 2020). Similar insights are given in studies by Shultz and colleagues in the context of addition and acid–base reactions (Finkenstaedt-Quinn et al., 2020; Petterson et al., 2020). They document that students seldom utilized resonance to determine reaction pathways or assess stability. In particular, students in this study seem to have difficulty identifying which entities (i.e., reactants or products) should be considered for determining stabilization through electron delocalization. This indicates the necessity of explicit prompting (Finkenstaedt-Quinn et al., 2020; Petterson et al., 2020).
In summary, students have diverse perspectives on resonance and may face challenges in fully grasping its dynamic nature and interconnectedness with other concepts. Still, it remains unclear how learners use constructed (or given) representations to deduce information to use the resonance concept in problem-solving tasks. Little is known about the various resources (i.e., ideas) related to resonance students activate when engaging with organic chemical tasks. Such insights, however, could inform instructional approaches to support students in learning and considering aspects of resonance in Organic Chemistry.
Theoretical framework
Resource-based model of cognition
According to the resources framework informed by Hammer and colleagues, knowledge cannot be considered as an “intact unit of cognitive structure” (p. 92) (Hammer et al., 2005) acquired in one context and transferred as a whole to another context. Instead, following a fine-grained constructivist view (Elby, 2000), this framework conceives the mind as a dynamic, complex system with a manifold view of the cognitive structure: the activation of fine-grained resources or knowledge elements characterizes one's knowledge and reasoning (Hammer et al., 2005). This framework considers the dynamic nature of students’ reasoning and takes both their in-the-moment thinking and existing knowledge in terms of cognitive structure organization into account (Louca et al., 2004; Hammer et al., 2005; Conlin et al., 2010). As such, resources are cognitive elements at varying grain sizes ranging from small, basic elements like phenomenological primitives, p-prims (i.e., intuitive ideas that constitute one's sense of mechanism without any further justification, such as “closer means stronger” (DiSessa, 1993; DiSessa, 2018)), to more complex structures like coherent theories (Hammer et al., 2005; Richards et al., 2018). The resources are at different states of activation, which can be used across contexts (Hammer et al., 2005; Richards et al., 2018). In this regard, resources can be activated alone or simultaneously within a set with other resources, in which one resource builds onto another, altogether forming chains of successive inferences. A network of resources that is repetitively activated over time may become a cognitive unit, i.e., act as a resource, itself (Hammer et al., 2005). Resources are neither right nor wrong and can only be activated (in-)appropriately in a certain context (Hammer et al., 2005; Richards et al., 2018). In general, resource activation is highly sensitive to the constraints of contexts, as differing contextual features cue the activation of different resources (Hammer et al., 2005; Conlin et al., 2010). Consequently, as resources are not robust in their activation, knowledge cannot be considered stable (Hammer, 2000; Richards et al., 2018). Which resources are activated and to what extent depends on one's framing (i.e., (implicit) expectations) of a given situation, guided by prior knowledge, experiences, and understanding. Framing shapes how a particular situation is perceived, thereby activating a locally coherent set of resources (Tannen, 1993; Hammer et al., 2005; Hutchison and Hammer, 2010). Consequently, task and context variation and prompting impact resource activation and influence one's task approaches and reasoning (Hammer et al., 2005).
Concept understanding through the lens of the coordination class theory
Conceptualization of coordination classes.
In line with the resources framework, the coordination class theory conceptualizes concept understanding (i.e., having a coordination class) as a profound and intertwined knowledge system. It comprises a complex network of fine-grained knowledge elements (i.e., resources in the realm of a concept) activated by perceptual cueing (DiSessa and Wagner, 2005). Consequently, this knowledge system allows us to flexibly “see” a concept in different contexts by reading concept-relevant information from various situations in the world (DiSessa and Sherin, 1998; DiSessa and Wagner, 2005; DiSessa, 2018). Given this core function of coordination classes, not all concepts can be considered coordination classes. DiSessa and Sherin (1998) distinguish coordination classes from category-like concepts. For instance, compare the concept of “cat” and “velocity”. For the first category-like concept, knowing a cat's defining attributes is core, making it possible to decide whether an animal belongs to this species. However, it is impossible to learn how to see these characteristics across varying situations – whether the animal is a cat or not. In contrast, one has to deal with the concept of “velocity” differently to get concept-relevant information. For instance, one might determine the amount of velocity by comparing the movement of two cars in one situation. At the same time, it is necessary to calculate with numbers presented in tables in other situations. As a result, the coordination class theory considers those concepts, which necessitate learning how to see them in a wide range of situations in which they can be applied and recognized (DiSessa and Sherin, 1998; DiSessa and Wagner, 2005). This can be done, for instance, by using different strategies or activating various resources. According to DiSessa and Sherin (1998), coordination classes help to understand how (especially complex, abstract scientific) concepts function in explanation and problem-solving. As such, prior research across the STEM disciplines focusing on learners’ concept understanding has used the coordination class theory to shed light on numerous concepts like energy (Barth-Cohen and Wittmann, 2017), velocity and frequency (Parnafes, 2007; Parnafes and DiSessa, 2013), the law of large numbers (Wagner, 2006), or, in chemistry, reaction rate (Rodriguez et al., 2020a), light- and light-matter interactions (Balabanoff et al., 2020), and the varied population schema (Rodriguez et al., 2020b). In line with the requirements for a coordination class, we argue that the concept of resonance can also be modeled as a coordination class. By reasoning about the electron density distribution in various molecules, thus, touching in its core upon molecules’ properties, resonance can help to describe a molecule's (electronic) state. While this is often done by considering and weighing the stability of resonance structures in a system, one must also be able to perceive resonance in other representations, such as resonance hybrids or electron potential maps. Given that resonance applies in various reaction contexts, one must be able to deal with this wide range of contexts (Carle and Flynn, 2020), thereby using resonance flexibly to reach task-specific conclusions (e.g., assessing molecules’ stabilities or deducing possible reaction pathways).
Architecture of coordination classes.
Given this perspective on what counts as a coordination class, it is consequently impossible to decide whether one has a concept or not. Instead, following the coordination class theory, concept understanding is complex and context-dependent. For educational purposes, it is important to understand how a learner “sees” these concepts in different situations (DiSessa and Sherin, 1998; DiSessa and Wagner, 2005; Rodriguez et al., 2020b). Consequently, it is crucial to characterize the processes involved within the knowledge system of a coordination class. The architecture of coordination classes is conceptualized as an internal structure characterized by two interdependent structural components: extraction strategies and the inferential net (cf.Fig. 1). In early literature, the coordination class components have been referred to as readout strategies and causal nets. As these terms appeared counterintuitive and caused confusion, DiSessa et al. (2016) suggested a revised terminology by replacing readout strategies with extraction strategies and the causal net with inferential net. These terms also will be used in this publication.
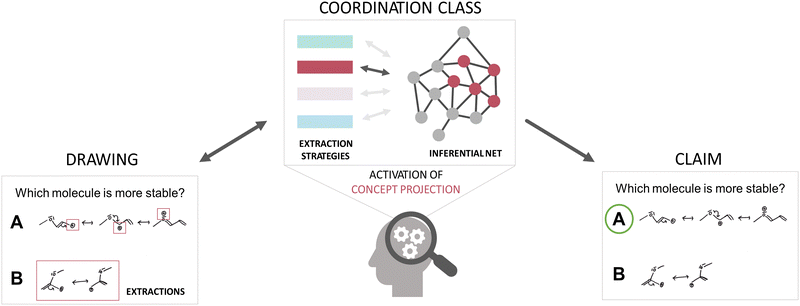 |
| Fig. 1 Illustration of the different components of a coordination class. | |
Extraction strategies capture the individuals’ overarching approaches for obtaining information and organizing sensory information (cf. framing) and thus describe how individuals focus on specific information (i.e., problem-solving approaches) (Wittmann, 2006; Rodriguez et al., 2020b). Extractions are the specific perceptual components attended to by an individual (DiSessa et al., 2016). These might be, for example, structural features of constructed resonance structures (e.g., a conjugated system, the absolute number of resonance structures, charges) (DiSessa and Sherin, 1998; Wittmann, 2002; DiSessa and Wagner, 2005; DiSessa et al., 2016; Rodriguez et al., 2020b). The extractions, in turn, cue the activation and combination of a cluster of concept-related resources, forming an inferential net and helping to reason through a given situation (DiSessa and Sherin, 1998; DiSessa and Wagner, 2005; DiSessa, 2018). As such, resources (often also referred to as knowledge elements) may encode a variety of ideas (e.g., p-prims, if-then statements, heuristics) (Buteler and Coleoni, 2016; Rodriguez et al., 2020b). Therefore, the coordination class of a learner functions as a filter for one's problem-solving (Rodriguez et al., 2020b). To illustrate, consider problem-solving in Organic Chemistry, which is often based on reasoning with drawings of structural formulas. Usually, these drawings contain much chemical information (i.e., connections, involved entities, and their interaction), so the focus of attention can vary depending on the problem. If, for instance, a learner is asked to compare the stability of two carbocations (Fig. 1), they might focus their attention on factors enhancing the stability of the respective structures and employ a task approach driven by stability considerations (extraction strategy). A learner might focus in detail on features in the structure that influence the stability, e.g., hetero atoms, the location of the positive charge, or, if resonance applies, the overall number of valid resonance structures (extractions). These structural features attended to then cue different resources, which result in an inferential net. The activated resources might be, for instance, electronic effects of the hetero atoms (i.e., implicit properties), the overall electron density distribution, or energetic aspects that are combined and lead to the answer to the task. In general, extraction strategies and inferential nets are closely related and influence one another, as new extractions might lead to the activation of new resources. The activation of resources might lead to or hinder the learning and activation of new extraction strategies (DiSessa and Sherin, 1998). As described in the example, given information, such as the prompt to consider stability or the type of representations, might as well cue the activation of specific extraction strategies.
Performance characteristics of coordination class use.
A coordination class allows the perception of certain information using different extraction strategies dependent on the situation. As such, a well-formed coordination class works with a broad set of sophisticated strategies and understandings. They can be applied across contexts and task types with certainty, proving a great extent of span and alignment (DiSessa and Sherin, 1998). Span describes the stability with which knowledge elements can be activated across various contexts and whether a coordination class can be broadly operated across various problem-solving contexts to activate resources and draw conclusions. According to DiSessa and Wagner (2005), this is also the defining feature of robustly transferable knowledge. Alignment, on the other hand, targets the inner consistency of a coordination class. Although different tasks and contexts might necessitate different approaches and might activate various resources, they must yield the same information, i.e., the same conclusion in problem-solving tasks (DiSessa and Wagner, 2005; Buteler and Coleoni, 2016; Rodriguez et al., 2020a). When reasoning about resonance, a high span would mean that students have enough conceptual resources to reason about resonance in a wide range of contexts (e.g., in aromatic and non-aromatic contexts). A high alignment would suggest that students can determine the perceived information reliably by displaying the electron density distribution in a molecule in combination with its thermodynamic consequences (and, for instance, not as distinct structures in certain situations). Otherwise, the coordination class would be ill-formed. Considering these two characteristics, mastery of a coordination class means coordinating resources in various ways depending on the context's requirements (DiSessa and Sherin, 1998). Due to the different relations between these components, it is noteworthy that a learner's coordination class can never be fully captured. One can also show competent task performance even though some relations within the coordination class might be incorrect (Buteler and Coleoni, 2016).
Given that students’ extraction strategies and inferential nets usually remain tacit in problem-solving, using interviews or other qualitative methods helps to shed light on these coordination class components. Singular contexts, however, do not require the activation of the whole coordination class but only a subgroup of activated extraction strategies and resources for that particular context (referred to as concept projections) (DiSessa and Wagner, 2005; DiSessa et al., 2016). Therefore, combining different task contexts offers a more in-depth insight into the nature of one's coordination class and helps to seize the richness of students’ concept understanding and consideration at different scales (Wittmann, 2002; DiSessa, 2018; Rodriguez et al., 2020b).
Research questions
Concept understanding cannot be modeled as an intact cognitive unit being transferred from one context to another. Hence, the coordination class theory can help to explore and grasp the richness of students’ use of resonance in problem-solving by considering it as an interplay of representation-based reasoning and resource activation on different levels across various contexts. It allows us to characterize how students use resonance across different contexts and helps to gain insight into which factors underlie a productive consideration of this concept. Specifically, this qualitative study aims at addressing the following research questions:
(1) How diverse are students’ concept projections of resonance across different problem-solving contexts?
(2) How do the coordination class components contribute to the productivity of students' concept projections of resonance?
Methods
Context and participants
This research study was conducted at a German university in summer 2021. 21 students majoring in chemistry (i.e., chemistry and food chemistry bachelors, and chemistry student teachers) participated in this study. They were recruited on a voluntary basis via e-mail and in-class announcements in the Organic Chemistry 1 (OC1) course. As compensation for their time, the students received 20 euros. The participants’ ages ranged from 19 to 34. Nine of them identified as male, twelve identified as female. Given that the OC1 course is the first lecture in Organic Chemistry at this university, students typically take it in the second or fourth semester of their study programs. The OC1 course has a weekly lecture (3 hours) and tutorial sections (1.5 hours). However, due to the pandemic, during the summer semester 2021, a flipped format was adopted in which students watched tutorial videos and read material (e.g., book chapters), before solving content-related tasks and discussing questions in online tutorial sections. The OC1 course follows a traditional curriculum to convey introductory knowledge of Organic Chemistry by first focusing on structure–property relationships and reactivities of functional groups. It covers typical reaction mechanisms such as electrophilic addition reactions, eliminations, nucleophilic substitutions, and carbonyl reactions. As the study took place near the end of the course, the students were familiar with the reactions addressed in the interview and the resonance concept. The resonance concept and the construction of resonance structures were introduced to the students during the first two weeks of the course and have been applied continuously during the semester with various topics (e.g., to discuss aromaticity).
All participants were informed about their rights and data handling beforehand; informed consent was obtained from all study participants. No further approval by an Institutional Review Board is required at the German university where the study was conducted. Nevertheless, the study followed ethical guidelines, and it was clarified to the students that they could withdraw from the study at any time. All students gave their written consent for the collected data (i.e., their recordings and scans of their worksheets) to be analysed and published by the research team. In this study, participants were given pseudonyms. No identifying information was recorded or scanned to allow participants to re-identify. Since the interviews were conducted in German, students’ interview excerpts were translated into English for this publication.
Data collection
This qualitative study is part of a project that explores students’ reasoning with drawings in problem-solving, and their drawing processes of structural formulas in Organic Chemistry in relation to the resonance concept. Semi-structured interviews have been combined with a paper-pencil setting to investigate students’ use of resonance in problem-solving tasks. They were audio- and video-recorded and lasted between 83 and 140 minutes. Since this study also focused on exploring students’ drawing processes, a mobile eye-tracker (Tobii Pro glasses 3, 50 Hz) was used to capture students’ eye movements during task completion. However, this analysis is not part of this publication. A thorough description of the methodical approach and respective results of this part of the project are reported elsewhere (Braun et al., 2022).
The interviews consisted of three parts. In the first part, the students were asked general questions about resonance (e.g., “When does resonance apply?”, “What is resonance?”, “How do you draw resonance structures?”) as a warm-up. This also served to gain first insights into the participants’ understanding and ability to construct and infer information from their resonance structures. In the second part, students were prompted to solve three organic case comparison tasks that required the application of the resonance concept. First, they were encouraged to solve the tasks freely, i.e., they could write and draw as much as they considered necessary without being disturbed. If they wished, they could also think aloud. After each task, a subsequent retrospective interview focused on their problem-solving. The students were asked to justify their answers, and to explain their problem-solving approach and thought process for the respective tasks. Furthermore, they were asked to describe their drawing strategies and the rationale behind their constructed resonance structures. The interview protocol for the retrospective can be found in Appendix 1. Finally, the last part of the interview comprised an overall reflection, in which general problems concerning the tasks and students’ further needs for these types of tasks were discussed. The study was piloted with five students in spring 2021. The analysis of pilot data led to slight changes of the drawing setting and the interview protocol, with the addition of more follow-up questions to gain deeper insights into students’ problem-solving and drawing processes.
Task design
In the second part of the interview, three case comparison tasks were used to elicit students’ use of and reasoning about resonance in problem-solving. They required the construction of resonance structures to solve the given problems and estimate the differences between the contrasting reactions. Case comparisons support a deeper engagement with a problem. By considering and weighing influential factors when comparing reaction pathways, this task type enhances closely analysing the representations, inferring reactivities and properties, and reflecting on the underlying reaction process (Alfieri et al., 2013; Graulich and Schween, 2018). Therefore, case comparisons represent a suitable instrument for characterizing students’ concept projection of resonance in problem-solving. The utilized case comparisons show varying degrees of familiarity for the students and are situated in aromatic and non-aromatic contexts (Fig. 2).
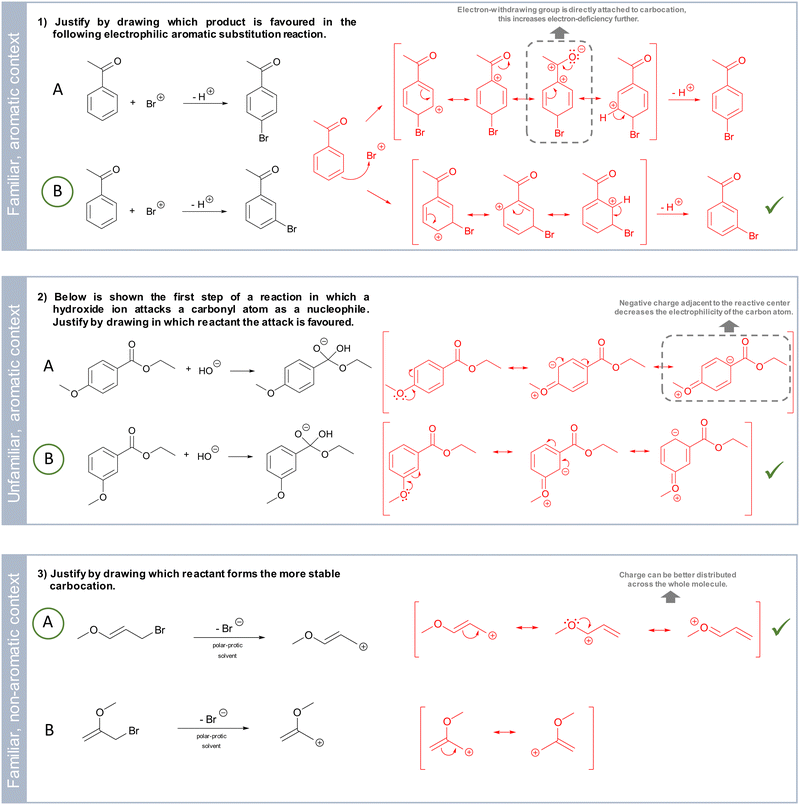 |
| Fig. 2 Task solutions for the utilized case comparisons in the study. | |
The first task addresses an electrophilic aromatic substitution reaction of acetophenone with a bromine ion, either resulting in a para-substituted (A) or meta-substituted product (B) (task 1, Fig. 2). In this task, the two possible reaction pathways need to be contrasted. Given the electron-withdrawing property of the carbonyl substituent of acetophenone, a substitution is favoured in the meta-position. This is due to the instability of the Wheland-intermediate when substituting at the para-position. More precisely, when the bromine is added as a para-substituent, the resulting resonance structures show a low electron density adjacent to the carbonyl substituent. Given its electron-withdrawing property, the electron deficiency would increase even more. This would be energetically unfavourable. In contrast to that, the resulting resonance structures for the attack of the bromine in the meta-position show that a lower electron density can be found at the ortho- and para-positions. This does not lead to energetically unfavourable electronic interactions. Besides this scientifically correct approach to the task, the influence of the electron-withdrawing property of the carbonyl group is often considered to decide on its directing effect as a substituent (Klein, 2012). Similar to the previous approach, here, the corresponding resonance structures of acetophenone illustrate that the electron density is low at the ortho- and para-position. An electrophilic attack necessitates electron density, so the substitution occurs at the meta-position. Since the electrophilic aromatic substitution has been covered in detail during the OC1 course, this task represents a familiar context for the participants.
The second task shows the first step of the alkaline hydrolysis of substituted ethyl benzoates in which a hydroxide ion is added as a nucleophile to the substituted ethyl benzoates (task 2, Fig. 2). Consequently, students have to compare in which of the two compounds the nucleophilic attack is favoured (Trabert and Schween, 2020). Here, one has to compare the influence of the methoxy substituent on the reactive centre and the electrophilicity of the attacked carbon atom. By doing so, one can infer that in the case of the methoxy substituent in the para-position (A), more electron density can be distributed via electron-donating effects (resonance and inductive effects) to the reactive centre. This lowers the electrophilicity of the carbon atom. Consequently, the nucleophilic attack is favoured at the 3-methoxy ethyl benzoate (B) (Trabert and Schween, 2020). Although this task is seemingly embedded in an aromatic substitution context, the actual mechanism, alkaline hydrolysis, plays a role in this case. This combination is considered an unfamiliar case for the students.
Unlike the first two tasks, the third is situated in a non-aromatic context (task 3, Fig. 2). It addresses the first step of a nucleophilic substitution reaction, in which a bromide ion leaves as a leaving group under the formation of primary carbocations. Here, the students are asked to decide which product is more stable. This can be determined by considering the electron density distribution of the cations reflected by the number of plausible resonance structures of both products. The positive charge can be better distributed in task solution A. Given that during the OC1 course students were regularly asked to assess the stability of carbocations by considering electronic effects, this task is considered familiar to the students.
Data analysis
The interview recordings were transcribed verbatim and implemented into the coding software MAXQDA for subsequent qualitative analysis. Given that a coordination class includes different structural components, the analysis involved several rounds of iterative coding (steps 1–7) to elicit students’ extraction strategies, extractions, and their activated resources (i.e., inferential nets) (Fig. 3).
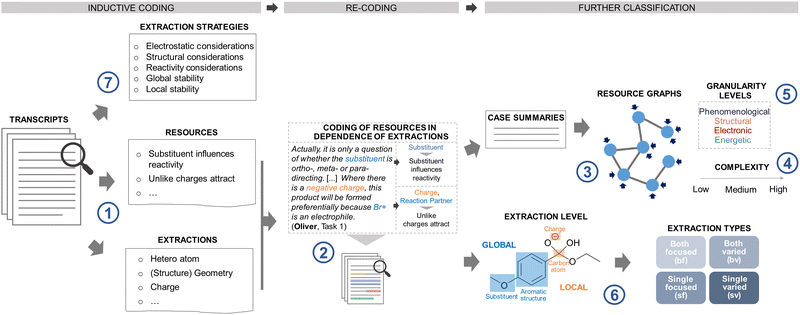 |
| Fig. 3 Illustration of the different steps in data analysis. | |
Initial coding of extractions and resources.
In the first coding cycle, the participants’ interview transcripts were coded in an open process using in-vivo coding (Saldaña, 2016) to derive students’ extractions and every activated resource concerning the resonance concept for each task. The resulting codes were then summarized by creating 31 higher-level codes for activated resources and 17 codes for extractions (i.e., structural features) focused on (Fig. 3, step 1). Examples of student utterances for a resource and the entire lists of inferred extractions and resources can be found in Appendix 2 and 3. In a subsequent coding cycle, students’ transcripts were re-analysed by hierarchically coding their resources in dependence of the extraction, as depicted in Fig. 3 (step 2). Both authors discussed and regularly optimized the coding scheme during this process to represent the data faithfully.
Construction and characterization of resource graphs.
Resource graphs were constructed in a third step to further characterize students’ activated resources within the inferential net (Fig. 3). As developed by Wittmann (2006), resource graphs represent networks of activated, observation-driven resources using a circle and line model. While circles illustrate resources, the lines (or arrows) demonstrate their linkage. This type of diagram sheds light on the activation of resources in a particular setting and emphasizes the students’ task-specific inferential nets. This type of representation has recently been used in chemistry education research to visualize students’ ideas of buffers, varied population schema and reaction rate (Rodriguez et al., 2020a; Rodriguez et al., 2020b; Sheppard and Bauer, 2022). In the following, we refer to inferential nets and resource graphs interchangeably, as both describe the types and combinations of activated resources. To elicit the relationships between the resources, we described students’ problem-solving for each task in case summaries (Kuckartz, 2013) before constructing individual resource graphs for each student. While the first author constructed the resource graphs, both authors engaged in regular discussions and reflection to ensure faithful data representation. Given that resource graphs are individual in nature, we characterized the diagrams further by their complexity to compare the resource graphs across the participants (Fig. 3, step 4). We adapted Yin's et al. (2005) classes of structure complexity in concept maps to differentiate students’ resource graphs as low, medium, and high complexity. If students’ resource graphs mainly demonstrated a loose, linear, or circular structure, they were associated with low complexity. If the resource graphs showed few interconnections illustrated by star and tree structures, they were considered medium complexity. Lastly, a network structure of the activated resource graph demonstrates high complexity and is characterized by many interconnections. Examples of the varying complexity of the resource graphs can be found in the results section and in Appendices 4 and 7. Moreover, we classified the deduced 31 resources by adopting Deng and Flynn's (2021) analytical framework of granularity levels to differentiate them. As such, the resources were either phenomenological, structural, electronic, or energetic in nature (Fig. 3, step 5). A detailed explanation of the granularity levels and an overview of the granularity of the resources can be found in Appendix 3. The first author coded the entire data set. A trained student research assistant independently coded a random sample of 20% of the resource graphs for interrater reliability. A Cohen's kappa coefficient of 0.87 was calculated, which indicates almost perfect reliability and agreement (Landis and Koch, 1977). Any ambiguities were discussed and resolved. In the end, a 100% agreement was reached.
Classification of extractions.
Students’ extractions were further characterized by the level and variety of the respective extractions (Fig. 3, step 6). For this, the extractions were first categorized into local and global extractions. While local extractions are single structural features such as hetero atoms, charges, or mere bonds, global extractions refer to features as a whole (e.g., substituents comprising several atoms, the number of resonance structures). After this, the total number of mentioned extractions within a task and the absolute number of extractions on local and global levels were counted. The degree of local/global extractions in dependence of the total number of extractions was calculated to characterize the level of encountered extractions. If the degree made up less than 1/3 (or, respectively, more than 2/3), students were given the code “single”, as the ratio indicates a predominant use of structural features on one level only, either global or local. If the degree ranged between 1/3 and 2/3, they were ascribed the code “both” as the proportions of both levels were more balanced. For the variety of encountered extractions, the absolute number of different extractions was divided by the total number of mentioned extractions. If more than half of all mentioned extractions differed, students were assigned the code “varied”. Otherwise, they got the code “focused” if they tended to refer to the same extractions when reasoning about the given task. The codes and an exemplary calculation are illustrated in Fig. 4.
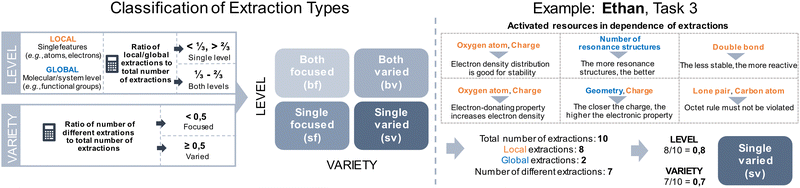 |
| Fig. 4 Classification of the extraction types with a student example to illustrate the calculation. | |
Induction of extraction strategies.
To examine students’ extraction strategies, the interview transcripts were coded inductively for general trends in students’ described problem-solving approaches by constant comparison (Fig. 3, step 7) (Strauss and Corbin, 1990). As such, five general extraction strategies to the tasks could be identified: (1) electrostatic considerations, (2) structural considerations, (3) reactivity considerations, (4) consideration of global stability, and (5) consideration of local stability. Table 1 provides the definitions of the resulting codes and illustrates them by giving student examples for each code.
Table 1 Coding scheme for students' extraction strategies with definitions and student example
Extraction strategy |
Definition |
Student example |
Electrostatic considerations (E) |
The focus is placed on the interaction of charges (i.e., mainly charge attraction/repulsion). |
And then I thought, I'll go back to the topic, resonance. And I just drew, ok, what kind of resonance structures are there for this molecule. I think that this was somehow correct. Later, I drew in the arrows again [referring to electron arrows in a structure] just to see, where a positive charge in the ring is possible, because I know, the substitution will take place at the ring. So, the idea was to dock the Br minus there. (Charlotte, Task 1) |
|
Structural considerations (S) |
The focus is placed on the structure itself, i.e., on the structural composition of the compound and static electron distribution (e.g., steric hindrance, type of substituent, product similarity). |
Tiffany: It would be the geometry. I know that lone pairs take up a lot of space […] They are just closer, it would be logical to say that it reacts here [refers to reaction B], because here [refers to reaction A], the electron density is already very high and when such an addition takes place, it would perhaps be more unrealistic compared to here, where it is a bit further apart. |
I: Is that related to sterics, then? |
Tiffany: I, yes, I would say so, because the lone pairs take up a lot of space. There [refers to oxygen atom in reaction A] are also lone pairs again. I would imagine that might have something to do with that as well. (Tiffany, Task 2) |
|
Reactivity considerations (R) |
The focus is placed on differences in the molecules with regard to the given reaction pathway (e.g., comparison of electrophilicity). This may also involve considerations of local or global stability with regard to the reaction course. |
Yes, there are certain unfavourable positions for positive charges, in which, for example, with electron-withdrawing groups (.) bromine has a minus I-effect, but a plus M-effect, because the plus M-effect actually always predominates. That is, if we now have, for example, a positive charge with bromine, which in this case actually cannot be the case, that would be an unfavourable situation, or if I have a negative charge here [refers to the carbonyl group in a structure of the Wheland-intermediate], that would also be quite unfavourable, because the minus M- and minus I-effect actually pull the electrons out here, that is, this partially positive charge would be increased. […] The reason why it is B, is simply because the positive charge is farthest away from the group, and here, we have it just close to the group [refers to carbonyl group], which would be unfavourable regarding stability. (Jack, Task 1) |
|
Considerations of global stability (G) |
The focus is placed on stability considerations related to the molecule as a whole; stability is the guiding (possibly overgeneralized) principle (e.g., involving considerations of electron density distribution, charge balance, number of resonance structures). |
I think I know what the task is getting at, if I am not mistaken, namely, we can push the negative charge around once in the ring completely and that means it is conjugated. Maybe, who knows. […] That is, we have a stabilization here, yes. So, theoretically, that is actually the idea, that you can push this around here once [refers to reaction A], so that is probably the more stable one and the whole thing will not be the case with the other one [refers to reaction B]. (Jack, Task 2) |
|
Considerations of local stability (L) |
The focus is placed on stability considerations focusing on singular parts of the molecule (e.g., most stable position of a charge, thus, local interpretation of charges). |
I would say that A is more stable, because here, this resonance structure is stabilized [refers to the second resonance structure in A] by the plus I-effect of the alkyl substituent. And here, it is just at the end and there is nothing more [refers to the resonance structures in B], therefore, yes. […] So here, it is obviously about the product, so I just looked how can I delocalize the positive charge, I drew the two/, so I drew the resonance structures of it and then I just briefly thought about it and came to the conclusion that this is the best possible distribution [refers to the second resonance structure in A]. The positive charge can then be best stabilized, because there are actually two resonance structures for each molecule. But up here, this one [second resonance structure in A] is, I don't know, the most valuable, because it can be stabilized best there. […] Um, because then, I also have this positive charge on the O, but that's not necessarily stable and that's why I thought that it makes more sense if I have my positive charge on the C-atom. (Nora, Task 3) |
Some students changed their extraction strategy during problem-solving due to new activation of resources or dead-ends. To capture this, we coded multiple extraction strategies within a task whenever the change led to bigger changes in the problem-solving, e.g., choosing a different answer or constructing new drawings. Again, the first author coded the entire data set, while a trained student research assistant coded a random sample size of 20% using the deduced coding scheme. The calculations permitted a Cohen's kappa value of 0.90, indicating almost perfect agreement (Landis and Koch, 1977). Any ambiguities and differences were resolved, leading to a total agreement of 100%.
The final stage of data analysis involved the comparison of students’ problem-solving to find trends in concept projections across the different tasks, and characteristics of productive concept projections of resonance with respect to the different coordination class components. We considered a concept projection productive if the resulting problem-solving was successful, i.e., if the answer resulting from the problem-solving was correct and if it moved the student closer to the final correct answer (Rodriguez et al., 2019).
Results and discussion
In the following subsections, we present the results of our analysis of students’ resonance consideration in problem-solving organized by our research questions (RQs), (1) the diversity of students’ concept projections across different tasks, and, building on that, outline (2) characteristics underlying productive concept projections of resonance.
RQ 1: How diverse are students’ concept projections of resonance across different problem-solving contexts?
Across the three contexts, all students in our sample showed varying concept projections in terms of the extraction strategies, the types of extractions, the activated resources, and the complexity of the resulting inferential nets. This section outlines general findings on the diversity of students’ concept projections and describes the general use of the coordination class components.
Multiplicity of extraction strategies within and across tasks.
With regard to the extraction strategies, in general, the three tasks required different approaches. While for the first and second task (electrophilic aromatic substitution and nucleophilic addition step), the impact of the different substituents and their electron density distribution on the reactive centre needs to be closely examined, the third task (departure step of a leaving group) demands the comparison of the stability of the resulting products. This suggests that students ideally activate a reactivity extraction strategy for the first two tasks and a global stability extraction strategy for the third task. However, students’ extraction strategies differed, as they employed various extraction strategies for the same task as well as inconsistently over all tasks. For instance, in the first task, two students employed a global stability extraction strategy, while 14 chose a reactivity approach. One student used a structural considerations strategy, and four used electrostatic considerations as an extraction strategy. While not all of these extraction strategies led to successful problem-solving in the first task, the same extraction strategies could be productive in other tasks. For instance, Benjamin's extraction strategy, global stability, did not contribute to a correct task solution in the second task as he evaluated the stability of the two given molecules in general (Fig. 5A). Yet, by framing the third task similarly and activating the same extraction strategy, he solved the task successfully (Fig. 5B). Altogether, across all three contexts, various extraction strategies led to productive concept use. This highlights that there are different ways of considering resonance for successful problem-solving, as suggested by the coordination class theory. The inconsistency of students’ extraction strategies over all tasks aligns with this assumption. Students often changed their strategies, for instance, from considering the reactivity in the first task and focusing on global stability in the second task. This indicates that students, perhaps due to the higher structural complexity in the second task, framed the two tasks differently (Tannen, 1993; Hammer et al., 2005; DeFever et al., 2015).
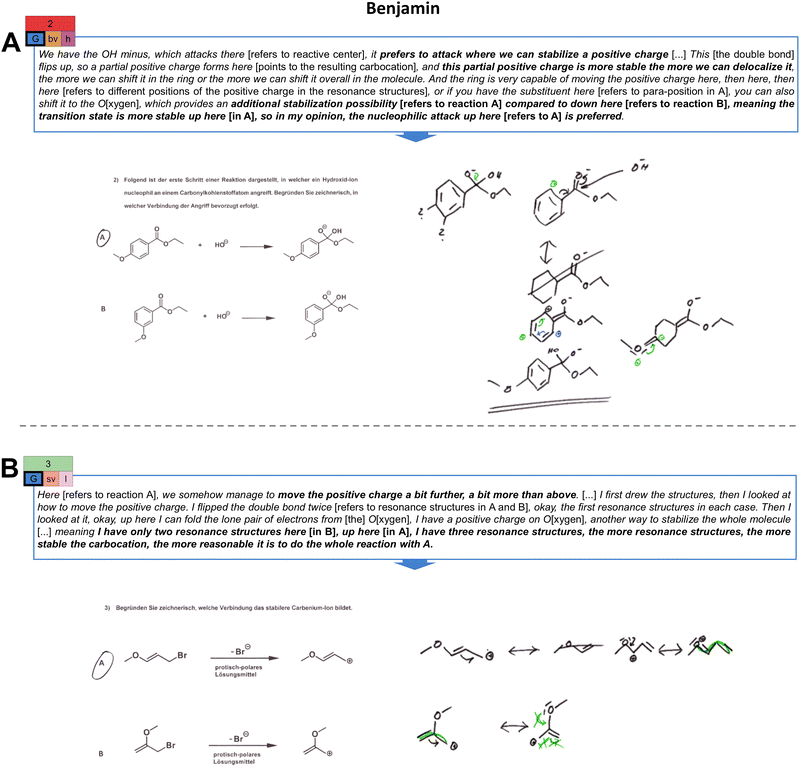 |
| Fig. 5 (A) Benjamin's activation of the global stability (G) extraction strategy in the second (unproductive concept projection) and (B) third task (productive concept projection) along with his constructed drawings as task solution. For the tasks, cf.Fig. 2. Extraction types (bv = both-varied, sv = single-varied) and the complexity of resource graphs (h = high, l = low) are also indicated. The resource graphs can be found in Appendix 7. | |
Even when students applied the same extraction strategy for a task, it did not necessarily lead to the same claim. For instance, despite choosing the same global stability extraction strategy for the third task, Anna's and Liam's success differed (cf.Fig. 6). Given that the extraction strategy is only one component of a coordination class, the interplay between the different coordination class components might have caused these students' varying overall problem-solving success. As depicted in Fig. 6, Liam and Anna attended to different structural features as extractions (single-focused vs. both-focused). Although the subsequent connection of inferred resources results in inferential nets of similar complexity, the resources within the inferential net differ, so that the nature of the inferred resources might have heavily contributed to the overall problem-solving success. This example shows well that even though students seem to approach a task similarly, they can deal with it differently, focusing on other structural features and activating different ideas about resonance.
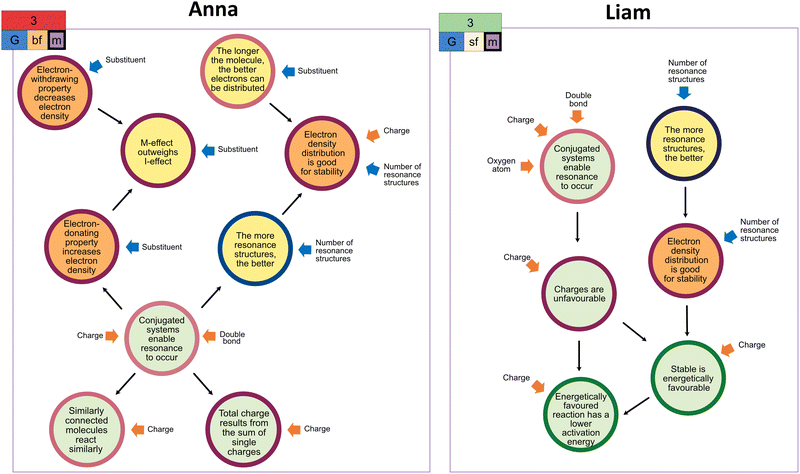 |
| Fig. 6 Anna's and Liam's concept projections for the third task illustrating the respective resource graphs and indicating readout strategy (G = global stability), extraction types (bf = both-focused, sf = single-focused) and complexity of resulting resource graphs (m = medium). For a detailed explanation of the resource graphs, cf. section “Theme 5. The interconnectedness of resources across different levels of granularity enhances productive concept projections”. | |
In many cases, students changed their extraction strategies within a task, sometimes multiple times (5 students in the first and third task, 10 students in the second task). This suggests that in the rather unfamiliar context, students needed more time to orient themselves. This might be possibly due to cognitive overload, e.g., deciding and focusing on relevant aspects (Sweller, 1988). But generally, it shows that the unfamiliar context may have led them to adjust their reasoning, thus, activating more suitable extraction strategies to overcome dead-ends.
Variety of extraction types.
According to the coordination class theory, extraction strategies guide students’ attention to structural information which is extracted during problem-solving (DiSessa and Wagner, 2005). Similar to the extraction strategies, the types of extractions used in the three contexts varied and were not particularly related to unsuccessful or successful problem-solving. Across the contexts, students used a single-focused extraction type in 21% of the concept projections (success rate of 69%). The single-varied extraction type was used in 17% of the concept projections (success rate of 91%). 33% of the concept projections involved a both-focused extraction type (success rate of 57%), while students used a both-varied extraction type in 29% of the concept projections (success rate of 72%). Although the extraction strategies highly influence the extraction of concept-related information, in our cohort, extraction strategies only evoked a certain extraction type in a few cases, underlining the complex interplay of coordination class components. For instance, students with a reactivity extraction strategy took during problem-solving more often structural information at a local and global level into account.
Frequency of activated resources.
As addressed in Anna's and Liam's problem-solving for the third task, the productivity of a concept projection is highly influenced by the interplay and contribution of the different coordination class components, which impact one another. As such, based on the extraction strategies, students’ extractions elicit multiple resources (e.g., p-prims, rules, if-then statements), which are combined to reason and draw meaningful conclusions for the tasks at hand (DiSessa and Wagner, 2005). A closer look at the distribution of resources across the three contexts reveals some tendencies in resource activation. Fig. 7 shows that some resources are activated with a similar frequency across all tasks (e.g., stable is energetically favourable, the closer the charge, the higher the electronic property). Additionally, there exist some context-specific differences in resource activation. For instance, most students activated resources related to reaction processes in the first two tasks. This includes, among others, the activation of resources considering the impact of substituents on the reaction pathway, or the interaction between electron-rich and electron-deficient centres. Interestingly, students in the first task often activated ideas related to electronegativity and the repulsion of similar charges. However, they seldom activated the influence of electron density distribution on the molecule's stability as a resource. In contrast to that, in the third task, students seldom used the resource the more stable, the more reactive even though this task requires comparing the stability of the two compounds. Instead, the students activated the resource the higher substituted, the more stable and charges are unfavourable with higher frequency, indicating that the students activated more resources considering the molecules at a rather local level. Compared to the first two tasks, students more often weighed the resonance structures and used the overall number of resonance structures to determine the molecules’ stability. It is noteworthy that some resources related to electron distribution (e.g., electron density distribution is good for stability, charge distribution according to electronegativity is favoured) are not equally activated across the tasks, although considering electron density is inherent to the resonance concept (Richardson, 1986). Despite differences in students' activation of resources, the overall complexity of their activated inferential nets does not influence per se the productivity of their concept projections. In fact, students’ resource activation and combination can be productive (i.e., contribute to successful problem-solving), regardless of whether the resource graph is of a low or high complexity (cf. examples in Fig. 6, 13, 15, and Appendix 7). This indicates that an inferential net with suitable resources matching the task affordances seems more beneficial than the actual complexity of the resulting inferential net.
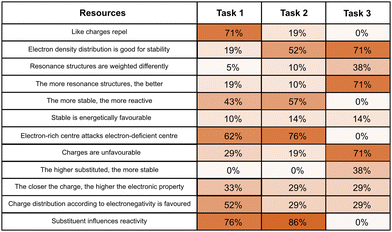 |
| Fig. 7 Relative frequency of activation of selected resources across the different tasks. A full overview of the resource activations can be found in Appendix 5. | |
It can be concluded from these results that students’ concept projections of resonance are diverse and individual across the tasks. They result as a product of the complex interplay of coordination class components, which impact one another and contribute as a whole to the (un-)productivity of a concept projection. As different extraction strategies, extraction types and resources can lead to successful problem-solving, there is not one way of using resonance productively. Given the variety of concept projections and the (un-)productive consideration of resonance in problem-solving, RQ 2 explores what differentiates students’ productive concept projections of resonance from unproductive ones, and what characteristics within the coordination class components underlie the overall productivity of a concept projection.
RQ 2: How do the coordination class components contribute to the productivity of students' concept projections of resonance?
While acknowledging the diversity of concept projections, focusing in more detail on students’ individual problem-solving processes (Hammer et al., 2005) makes it possible to elicit underlying characteristics within the coordination class components and to show their contribution to the overall productivity of the concept projection. Therefore, multiple cases of productive and unproductive concept projections will be qualitatively contrasted to shed light on differences within the coordination class components, their impact on one another, and the aspects differentiating the various concept projections in terms of successful problem-solving.
Theme 1. Inductively and reflectively activated extraction strategies and the task affordances contribute to the productivity of concept projections
Fragmented use of drawings and uncertainty.
Across the three tasks, students used various extraction strategies for dealing with resonance. However, comparing students’ problem-solving revealed some reoccurring trends in their approaches. Irrespective of the concrete extraction strategy, these strategies affected the extractions, the activation of resources, and the overall productivity of the concept projections (i.e., resulting in varying problem-solving success). Consider, for instance, Claire's reasoning in the electrophilic substitution reaction in which she activated electrostatic considerations as an extraction strategy to frame her subsequent problem-solving process (Fig. 8). The excerpt shows that Claire has a strong focus on structural features. Cued by the positive charge (i.e., extraction), she decides to solve the task by forming a negative charge. Although Claire considers the interaction of entities as an important aspect of mechanisms (Russ et al., 2008), her extraction strategy is unproductive. This is due to her focus on salient structural features (i.e., charges) instead of analysing her constructed structures to infer properties enabling the reaction to occur. Consequently, Claire shows a narrowed structural focus when approaching the task. As in many other cases of unproductive concept projections, the extraction strategies were often paired with uncertainty about how to approach the task in general. In Claire's case, this led to the activation of resources, which were rather competing ideas (cf. her resource graph in Appendix 7). More precisely, Claire was unsure how the ketone substituent impacts the electrophilic substitution and assumed that, depending on the situation, the ketone could have different electronic properties. Consequently, she constructed multiple resonance structures with the ketone being both electron-donating and electron-withdrawing (Fig. 8). She then decided on the electron-withdrawing property due to the positive charge of the bromine cation which “need[s] a negative charge”. Claire's case aligns with a huge body of research in chemistry education, demonstrating that if learners struggle to activate relevant conceptual resources (i.e., derive implicit properties), a reliance on explicit structural features occurs, often hindering successful problem-solving (e.g., McClary and Talanquer, 2011; Anzovino and Bretz, 2015; Anzovino and Bretz, 2016; Graulich and Bhattacharyya, 2017; Galloway et al., 2018; Popova and Bretz, 2018b; Graulich et al., 2019). Claire's case exemplifies that students with unproductive extraction strategies do not or only partly use their drawings as a problem-solving tool (Cooper et al., 2017). Instead, they tend to project their ideas into the structures. This approach might be supported by salient structural features or students’ personal views (Hogan and Maglienti, 2001). Claire explored through her drawings the different possibilities for the electronic effect of the carbonyl group. She concluded that the para-position “looked” more plausible to her (Fig. 8). In fact, activating an unproductive extraction strategy might also be related to difficulties in dynamically interpreting electron distribution. As documented by Taber (2002), students’ difficulties in conceptualizing resonance are related to the tendency to interpret structural diagrams literally, with resonance being the re-location of bonds instead of a dynamic delocalization of electrons. Aligning with that, Claire considered the resonance structures as static entities given that she got stuck with the double bond, which, in her opinion, must correspond to the depicted product.
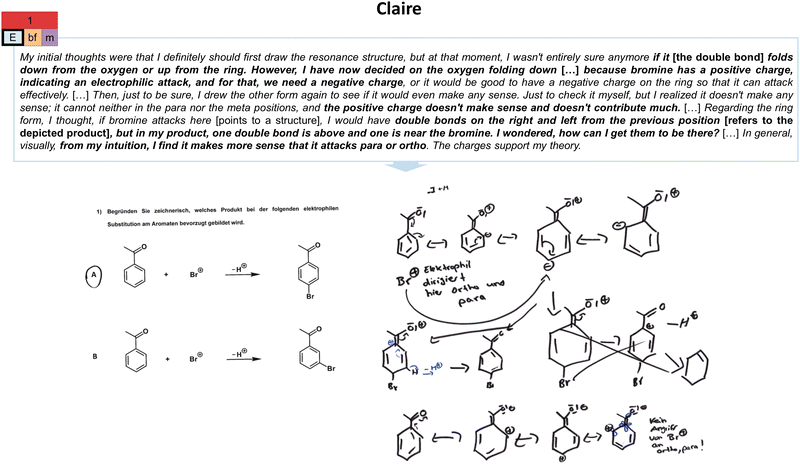 |
| Fig. 8 Illustration of Claire's electrostatic considerations (E) extraction strategy in the first task (cf.Fig. 2) and her constructed drawings. As indicated, her unproductive concept projection also encompasses a both-focused (bf) extraction type and a resource graph of medium complexity (m). The resource graph can be found in Appendix 7. | |
Activation of overgeneralized rules.
Generally, in unproductive concept projections, students’ activation of extraction strategies is in line with many characteristics documented in research on students’ problem-solving in Organic Chemistry (e.g., Ferguson and Bodner, 2008). For instance, with the fact that students’ activation of unproductive extraction strategies is often linked to a narrowed focus on single structural features or the activation of overgeneralized rules. In our study, this often led to problem-solving dead-ends. The excerpt from Jack's initial problem-solving process in the first task, in which he applied a global stability extraction strategy (before changing it later to the reactivity extraction strategy), illustrates that (Fig. 9). Jack struggles with the electron-withdrawing property of the ketone substituent and tries to make the positive charge disappear to gain a more stable system (i.e., the extraction strategy global stability). He does recognize important aspects such as stability in a reaction, and pays attention to charges to consider the impact of varying electron density distribution. But in relation to the activation of ideas targeting the electron-withdrawing effects, the resource electron-withdrawing property removes charges strongly influenced how he used the extraction strategy global stability (cf. his resource graph in Appendix 7). As such, he ended up being puzzled by his approach, as he could not link the electronic effect to resonance, i.e., he got stuck trying to remove the charge. He tried multiple variants to draw his resonance structures, as evident by the number of his drawings (Fig. 9).
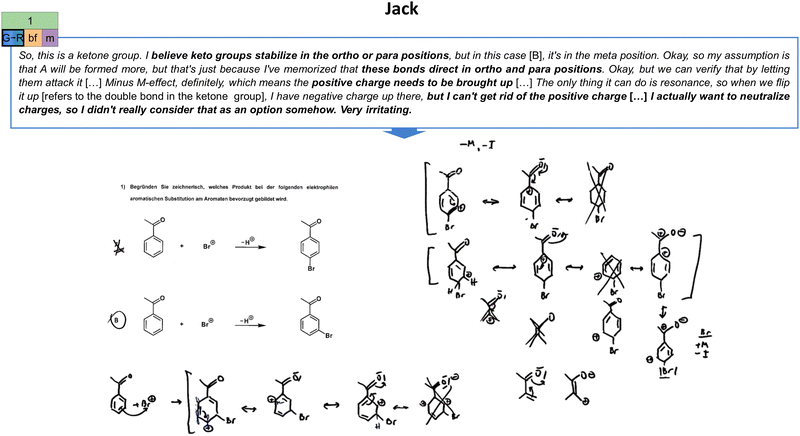 |
| Fig. 9 Illustration of Jack's initial global stability (G) extraction strategy in the first task (cf.Fig. 2) and his constructed drawings. As indicated, his (initially unproductive) concept projection also encompasses a both-focused (bf) extraction type and a resource graph of medium complexity (m). The resource graph can be found in Appendix 7. | |
Inductive and analytical approaches.
Unlike Claire's extraction strategy for the first task, a closer look into her reactivity extraction strategy for the second task reveals various aspects that explain productive concept projections (i.e., contributing to successful problem-solving) (Fig. 10). The excerpt shows that Claire approaches the second task inductively by making herself aware of the problem. Claire reconstructs the reaction step by closely examining the reaction conditions and prerequisites (What is demanded in the task?). She then points out the structural differences and their impact on the reaction (i.e., the impact on the electrophilicity of the reactive centre). Hence, in contrast to the first task (Fig. 8), Claire has a differentiated process orientation and approaches the task more analytically. As a result, Claire interprets and reflects her derived findings regarding resonance (i.e., the extent of charge stabilization) within the given reaction context. She activates resources targeting chemical concepts (i.e., varying electrophilicity) to come to an answer (cf. her resource graph in Appendix 7). Her productive approach is in line with existing research on students’ problem-solving and reasoning, indicating that a more thorough examination of reaction conditions and prerequisites seems beneficial for successful problem-solving (Yuriev et al., 2017; Caspari and Graulich, 2019). As such, Chi et al. (1981) revealed in their early work on experts’ and beginners’ problem-solving that in comparison to beginners who base their approaches on the problems’ literal features, experts approach tasks by initially abstracting underlying scientific principles to solve a problem. Taken together, productive concept projections often occur when extraction strategies match the task affordances (Airey and Linder, 2009), necessitating a more nuanced and analytical approach to a task.
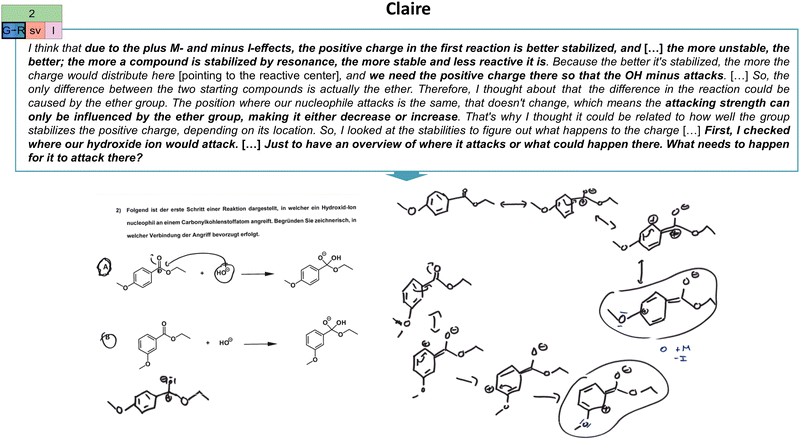 |
| Fig. 10 Illustration of Claire's reactivity (R) extraction strategy in the second task (cf.Fig. 2) and her constructed drawings. As indicated, her productive concept projection also encompasses a single-varied (sv) extraction type and a resource graph of low complexity (l). The resource graph can be found in Appendix 7. | |
Compensation of unsuitable extraction strategies through activation of relevant resources.
Extraction strategies are not always a clear indicator of the productivity of the resulting concept projection. Instead, the different components in the coordination class may compensate for each other. Even if choosing an unsuitable extraction strategy, the components’ interplay gives rise to a productive concept projection, resulting in successful problem-solving (Buteler and Coleoni, 2016). Consider, therefore, Charlotte's problem-solving in the third task as an example, in which she chooses local stability as an extraction strategy (Fig. 11). Here, Charlotte evaluates different factors contributing to the products’ stability. Yet, she does not directly incorporate aspects of resonance concerning electron density distribution. Similar to using a mental rolodex (DeFever et al., 2015), she adeptly navigates through other ideas, such as steric hindrance, inductive effects, and polarity, relative to the structures' geometry, indicating that she tried out several resources to solve the task. Thus, her focus was guided by singular fragments of the molecule. As a reason, she admits that her approach partly stems from her challenges in constructing the structures (“it did not work out, and this somehow works”). The cognitive overload to manage the information (Sweller, 1988) might be a reason why Charlotte incorporated resources targeting other (more familiar) concepts, as they might be easier to activate. For instance, Finkenstaedt-Quinn et al. (2020) showed in their study that students favoured the consideration of substitution over resonance when assessing the stability of molecules, as it allows the mere use of explicit chemical features instead of underlying, implicit electronic properties. Nevertheless, in Charlotte's overall task solution, she somewhat incorporated relevant resources, that enabled a successful task solution. As such, she considered the impact of electronic properties on stabilizing the positive charge (cf. her resource graph in Appendix 7). Even if she constructed valid resonance structures for the reaction in A (Fig. 11), she could not interpret these structures dynamically and did not consider the overall electron density distribution. Instead, due to her local stability approach, she assessed the quality of singular resonance structures by considering the resonance structures as discrete, static entities. Stated differently, she compared in which position the charge is most likely to be stabilized through substituents. Due to that, she struggled to evaluate the positive charge on the oxygen atom (Fig. 11). Although Charlotte's task approach does not match well with the task affordances, it leads to the activation of relevant electronic resources and a correct answer.
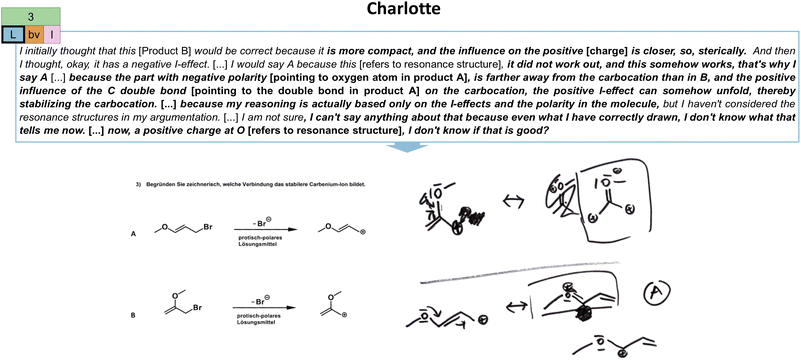 |
| Fig. 11 Illustration of Charlotte's local stability (L) extraction strategy in the third task (cf.Fig. 2) and her constructed drawings. As indicated, her productive concept projection also encompasses a both-varied (bv) extraction type and a resource graph of low complexity (l). The resource graph can be found in Appendix 7. | |
Theme 2. Analytically engaging with one's drawings induces productive changes in extraction strategies
Some students changed their extraction strategies within a task due to new insights during their problem-solving process. Whether the changes in extraction strategies contributed to productive or unproductive concept projections often depended on students’ engagement with their drawings. To illustrate, consider the excerpt from Lucas’ retrospective for the second task (Fig. 12).
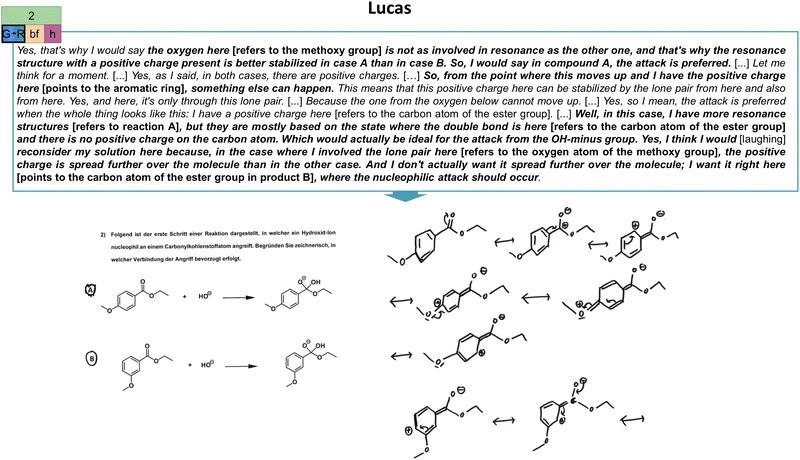 |
| Fig. 12 Illustration of Lucas’ change from the global stability (G) extraction strategy to the reactivity (R) extraction strategy in the second task (cf.Fig. 2) and his constructed drawings. As indicated, his productive concept projection also encompasses a both-focused (bf) extraction type and a resource graph of high (h) complexity. The resource graph can be found in Appendix 7. | |
First, Lucas applied a global stability extraction strategy to assess the preferred reactivity in both reactions, which he changed to a reactivity approach. By doing this, he advanced his ideas. He developed them further by reconsidering and closely analysing his drawings in relation to the task affordances (i.e., comparison of the electrophilicity in the two molecules). He was zooming in and out based on his drawings. Other students also changed their extraction strategies toward successful problem-solving after describing their approach and reasoning. This indicates that (self-)explanations for why one did something help students to refocus, reflect, and engage analytically with the task and their ideas (Chi et al., 1994). Such a meta-level perspective gives rise to productive task approaches with extraction strategies matching the task affordances (Airey and Linder, 2009). In our cohort, students often realized while elaborating their task approach by referring to the respective drawings that something was missing or incongruent with the task requirements. They then started to re-examine their drawings critically. In some cases, the consecutive construction of resonance structures helped the students refocus and gain further insights (e.g., Claire's problem-solving in task 2, cf.Fig. 10). Following that, the analytical engagement with and nuanced analysis of the structures in relation to the task affordances crucially influence the activation of suitable extraction strategies for the tasks. These extraction strategies affect the structural features encountered, as outlined in the following section.
Theme 3. Managing the variety of structural extractions makes the difference
The diversity of concept projections was not paired with clear trends in extraction types regarding productivity (cf. RQ1). Nevertheless, the analysis of students’ problem-solving process indicates that considering structural features in their variety and across the global and local levels contributed to a productive concept projection. Students who encountered different structural features as extractions (sv, bv) were more likely to have a productive concept projection than those who narrowed their extractions to a few structural features (sf, bf) (cf. RQ 1). The reasons for this finding can be exemplified in more detail by contrasting excerpts of Emily's and Alina's problem-solving for the first task.
In both cases, the inferential nets do not show high complexity and are comparable as each has multiple linear connections (Fig. 13). However, different extraction types are underlying and have influenced the students’ problem-solving success. Consider Emily's focus on single structural features. She predominantly uses extractions at a global level (i.e., the number of resonance structures and the substituent). Even though she refers to the local level, she only focuses on the positive charge (Fig. 13). This indicates that she looked at her drawings with a specific focus, i.e., searching for cues that support stability considerations. As a result, though mentioning the mesomeric effect, she could not specify the carbonyl's electron-donating or -withdrawing property. Her focused attention to structural features in problem-solving is also a common observation in other studies. For instance, Anzovino and Bretz (2015) showed in the context of electrophiles and nucleophiles that students tend to rely primarily on charges when defining and identifying electrophiles and nucleophiles in general, and when dealing with specific chemical reactions. Our own recent findings on students’ drawing processes of resonance structures also show that a focus on singular features such as charges or hetero atoms without a link to other structural features often results in invalid resonance structures (Braun et al., 2022). As a result, Emily struggled to draw missing resonance structures and did not activate charge interactions in her problem-solving. The few extractions encountered in her problem-solving influenced how she approached the task (i.e., she got stuck with the global stability extraction strategy). In fact, across the 19 unsuccessful task solutions, in 12 cases, students used focused extractions. Albeit possibly considering both the local and global levels within their drawings, they generally paid attention to a limited number of structural features and overemphasized certain structural components when activating resources. Consequently, this static focus on singular features might have contributed to activating unproductive resources (e.g., immediately linking the extraction aromatic compounds with the resource aromatic compounds are stable).
In contrast to these focused extractions, a closer look into Alina's excerpt demonstrates that she takes a broad amount of structural information into account (Fig. 13), which underlines a more thorough structural analysis. As seen in both retrospectives (Fig. 13), Alina and Emily approach the task similarly by highlighting the substituents’ function in determining the position of the electrophilic substitution. In doing so, unlike Emily, Alina approaches this differently. She includes more structural information by transitioning between various structural features at the local (e.g., charges, double bond, atom types, lone pair) and global levels (e.g., substituent, reaction partner, reactant, reaction type), and considering a variety of features on each level. As such, she uses an analytic, differentiated approach. She pays attention to the drawings as a coherent system. The interplay of various structural features gives rise to conclusions and resource activations that are relevant to the reaction at hand. This resonates with the reactivity extraction strategy, which is often paired with a more nuanced analysis of the task affordances, as reported in the previous section. Managing the granularity of extractions seems to be beneficial for problem-solving. This involves zooming in and out to analyse in detail the structures in their entirety across both local and global levels (i.e., taking into account the multiplicity of structural features), and combining them to activate resources for problem-solving. Eventually, it allows one to widen the perspective on the given task and the conditions at hand and, consequently, guides one's thinking to activate more productive, analytical resources (e.g., derive electronic effects of the structural composition in a molecule).
Theme 4. Productive concept projections involve more analytical-comparative resources
In comparing the cases of productive and unproductive concept projections, differences in the types of activated resources become evident. Fig. 14 illustrates the activation frequency for a subset of resources used in (un-)productive concept projections.
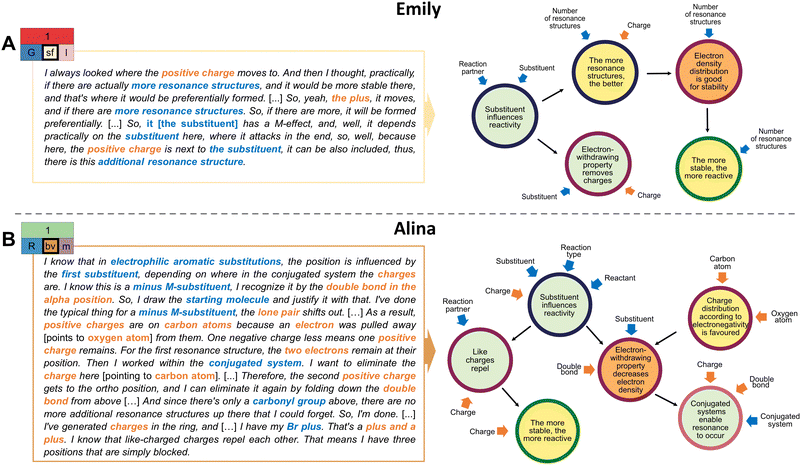 |
| Fig. 13 Comparison of Emily's and Alina's extraction types (single-focused [sf], both-varied [bv]) with an illustration of their overall resulting resource graphs (low [l] and medium [m] complexity) in the first task. While Emily's concept projection is unproductive and exhibits a global stability (G) extraction strategy, Alina's concept projection is productive and involves a reactivity (R) extraction strategy. The orange highlighting in the quotes indicates local extractions, the blue highlighting global extractions. | |
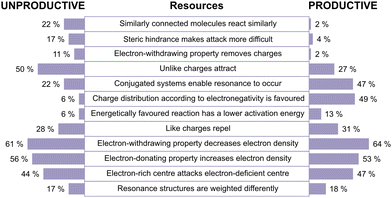 |
| Fig. 14 Relative activation frequency of selected resources in (un-)productive concept projections. | |
When qualitatively contrasting the frequency of resource activation, it becomes evident that students with unproductive concept projections tended to infer more resources that do not touch upon the electron density distribution as the underlying idea of the resonance concept (e.g., the resource steric hindrance makes attack more difficult) (Fig. 14). The students also included more often heuristic-like resources such as similarly connected molecules react similarly or aromatic compounds are stable, and activated other resources like electron-withdrawing property removes charges. The activation of resources that are not related to the actual meaning of resonance (or only consider it in a very simplified way) often does not allow one to make productive concept projections, as in line with former studies (Brandfonbrener et al., 2021; Tetschner and Nedungadi, 2023). Some of these resources are reflections of students’ structural considerations and electrostatic considerations extraction strategies. As such, these resources refer to the structure itself (e.g., steric hindrance makes attack more difficult) and are rather static in nature (e.g., unlike charges attract).
On the contrary, when showing productive concept projections, students more often elicited resources that are (structural-) analytic and weighing-comparative in nature. For instance, they included more often the electronegativity of the atoms in their reasoning and considered the prerequisites for resonance (e.g., conjugated systems enable resonance to occur) during problem-solving. This indicates that students with productive resonance use activate more nuanced ideas. In contrast to unproductive concept projections, students also considered some energetic considerations more frequently (e.g., the relationships between stability and reactivity and energetically favoured reaction has a lower activation energy) (Fig. 14).
Despite these slight differences in the activation of resources, Fig. 14 also shows that students, regardless of the productivity of their concept projection, activated many resources with comparable frequency (cf. also the complete list in Appendix 6). The activation of a specific resource is thus not a sufficient indicator of how resources contribute to the productivity of concept projections. Instead, in accordance with the coordination class theory, it is pivotal to further shed light on how students combine and organize the activated resources within their inferential nets (DiSessa and Sherin, 1998).
Theme 5. The interconnectedness of resources across levels of granularity enhances productive concept projections
When looking at students’ combination of activated resources in their inferential nets, one can contrast Audrey's and Louis’ resource graphs for the first task. Both students used similar types of extractions by referring to the local and global level, but focusing altogether on relatively few features (i.e., both-focused extractions). However, their inferential nets differ in complexity, with a low complexity for Audrey's and a high complexity for Louis’ reasoning (Fig. 15).
Audrey's resource graph reveals that in her reasoning, one resource consecutively activates another one, resulting in a rather linear structure. It depicts two branches of dualistic thoughts and shows different possibilities for reactivities. Similar to Claire (cf.Fig. 8), she assumed that the substituent can have, depending on the situation, an electron-withdrawing or electron-donating effect. Her activated set of resources targets different, singular (static) aspects, such as charge interactions and their influence on the reaction pathway in terms of electron density. Thus, they are analytic and distributive in nature. Her graph further reveals that her resources are mainly situated at the electronic level. She first activates a resource mapping the oxygen atom's reactivity with similar-looking structures from her experience (i.e., a resource at a structural level). Subsequently, she focuses on aspects targeting electron density (i.e., electron-withdrawing or -donating properties, low electron density attracting high electron density, and charge interactions). These are, in fact, crucial aspects when evaluating reaction pathways as they give insight into why a reaction happens (Cooper, 2015). However, Audrey fails to connect her resources with energetic considerations. Similar findings have been recently reported by Pölloth et al. (2023), showing that also high-school chemistry students seldom activate resources attributing energy to structural changes when reasoning about a chemical reaction.
In contrast to that, Fig. 15 shows that Louis’ resource graph is characterized by a higher complexity with resources being (inter-)connected, building a complex net of inferences to solve the task at hand. His reasoning is grounded in considering aspects such as electronegativity and activation energy, which make his problem-solving more differentiated than Audrey's. This is supported by the fact that he activates and combines multiple resources that can be modeled with Deng and Flynn's (2021) analytical framework of granularity levels. As such, he transitions in his problem-solving between different levels. While he first states at a phenomenological level that the substituent influences the position of substitution, he proceeds by activating resources at a structural (e.g., conjugated systems enable resonance to occur), electronic (e.g., electron-withdrawing property decreases electron density), and energetic level (e.g., stable is energetically favourable). Along with the intended learning outcomes of resonance formulated by Carle and Flynn (2020), the transition across granularity levels shows that the student adopts a dynamic, holistic perspective of resonance. By activating resources that target the affordances in this task, Louis activates resources with different characteristics, altogether helping him to draw correct conclusions to the task. His resources are not only analytic and distributive, but also weighing-comparative in nature (i.e., assess the influence and extent of effects, such as electronic effects, by referring to chemical principles). Concretely, he decides on the electron-withdrawing effect of the substituent by combining this resource with electronegativity considerations. Although Audrey also activates the same resource (i.e., electron-withdrawing properties), she does not activate other weighing-comparative resources. In light of the overall comparable frequency of resource activation for all students (cf.Fig. 14), these examples illustrate that the linkage between the different resources crucially decides whether the activated set of resources becomes productive for the concept projection. Irrespective of the overall complexity in their inferential nets, most students activated the electronic effects of substituents across the tasks. However, their joint activation and connection with weighing-comparative resources differentiated a productive from an unproductive resource activation, resulting in a more reflective use of resonance. Results in other disciplines and contexts also leverage this. For instance, Richards and colleagues (2018) found that students tend to activate combinations of different resource types when making conceptual breakthroughs in the context of photovoltaic cells. Similarly, a recent study focusing on students’ conceptualization of buffers points out that emphasizing relations and connections between resources is valuable for conceptual understanding (Sheppard and Bauer, 2022).
Conclusions and implications
This paper aimed at delving into students’ use of resonance in organic-chemical problem-solving across different contexts through the analytical lens of the coordination class theory. It sees a concept in its architecture as an interacting system of extraction strategies and activated resources based on extractions in a given situation. As such, we characterized students’ engagement with constructed drawings of resonance structures, and qualitatively highlighted characteristics that distinguish productive from unproductive concept projections. In line with the assumptions of the resource framework, no apparent, general trend among the concept projections emerged. Instead, students’ consideration of resonance varied for the different contexts (RQ 1). Given the variety of combined coordination class components, it became evident that a different focus in task approaches can lead to successful problem-solving. There is not only one valid way of considering resonance in problem-solving. The diversity is not only caused by different task affordances which lead to situation-specific framing, but also by the complex and individual interplay of extraction strategies, extractions, and activated resources. This detailed analysis also illustrated that a lot is going on when students are asked to consider the resonance concept in problem-solving, i.e., constructing and drawing resonance structures, activating implicit properties of structural features, and linking these together to make a claim about the outcome. However, qualitatively contrasting these diverse concept projections revealed some characteristics underlying productive concept use (RQ 2). We could illustrate how the coordination class components mutually impact one another in a complex interplay. There are multiple ways to come to a correct conclusion. Still, unsurprisingly, a more reflected engagement with the structural drawings favoured a productive concept projection of resonance across all components of a coordination class. This is in line with former research (e.g., Furió et al., 2000; Domin et al., 2008; Cooper et al., 2013; Maeyer and Talanquer, 2013; Popova and Bretz, 2018a; Talanquer, 2022) and stresses the importance of representational competence in problem-solving. In our analysis, it became evident that the structures must be considered holistically for successful problem-solving, i.e., considering and combining structural features across the global and local levels. Often, this was supported by a more inductive and reflective analysis of the task affordances, which resulted in the activation of more appropriate extraction strategies. Since the coordination class components inform one another, a thorough structural analysis of the structures also supports productive changes in extraction strategies during problem-solving.
In unproductive cases, extraction strategies focusing on overgeneralized approaches to the tasks often led to focused extractions, i.e., only paying attention to a few (salient) structural features throughout the problem-solving. Besides being stuck with overgeneralized ideas (e.g., overgeneralized application of global stability), uncertainty and drawing difficulties frequently caused unproductive task approaches. In terms of the resulting inferential nets, students often activated similar resources, whereby students with unproductive concept projections (i.e., unsuccessful problem-solving) slightly tended to infer more often ideas unrelated to the idea of resonance (e.g., steric hindrance), or heuristic-like ideas. What differentiated the activated resources' productivity was their connection and, thus, how students used the resources. As such, a productive concept projection of resonance often involved resources being activated and (inter-)connected across levels of granularity, ranging from phenomenological to energetic considerations. The overall complexity of inferential nets was not inherently related to productivity. Instead, students who successfully solved the problems tended to combine resonance-related ideas by jointly activating analytical and comparative resources. Such an interplay gave rise to a more reflective consideration of the resonance concept.
Implications for teaching and learning
Many intertwined aspects impact the consideration of resonance in problem-solving. This comprises constructing valid resonance structures, matching the task affordances, unpacking the representations (e.g., recognizing and using relevant structural information), and interpreting and connecting inferred conceptual ideas (Talanquer, 2022). As a result, there is not just one way to use resonance in problem-solving, as shown in the complex and diverse interplay of extraction strategies, extractions, and resource activation. Concerning the coordination class components, it became apparent that students experience conceptual and procedural challenges when reasoning with their resonance structures, eventually hampering productive concept projections. Therefore, teaching should consider these different conceptual and procedural aspects of concept use more. Stated differently, we should encourage students to pay more attention to their drawings, as they are crucial for the dynamic conceptualization and comprehensive use of resonance in problem-solving. Since in our interviews, simple metacognitive follow-up questions (e.g., “How did you get to your solution?”) often helped the students to focus and refine their reasoning, the need for slowing down students’ problem-solving process became apparent. To foster analytic thinking and a more reflective approach to the tasks, a suitable intervention could be scaffolding the use of resonance by sequencing the complex, multistep problem-solving process (e.g., Wood et al., 1976; Benson, 1997; Graulich and Caspari, 2020). One possibility could be the use of checking lists as well as metacognitive questions (e.g., Yuriev et al., 2017). They explicitly guide students to focus their attention on structural prerequisites for resonance, to incorporate those structural features, and to elicit and connect diverse ideas across levels of granularity. For instance, in our study, students activated many resources with a similar frequency, irrespective of the productivity of the concept projection. But they did not always connect their ideas. For example, they referred to aspects on a phenomenological level but did not always link the activated resources to the structural, electronic, or energetic level. Consequently, metacognitive questions could target more holistic reasoning across the levels of granularity. Fig. 16 depicts a possible scaffold by sequencing the problem-solving process into the steps “Analyse” (i.e., become aware of the affordances of the task, but also the given structures and structural properties), “Draw” (i.e., help students to assess and reflect on their drawings), and “Conclude” (i.e., connect drawing-based inferences to the initially stated requirements of the tasks), altogether guiding the students through the steps in problem-solving and facilitating a more differentiated task approach.
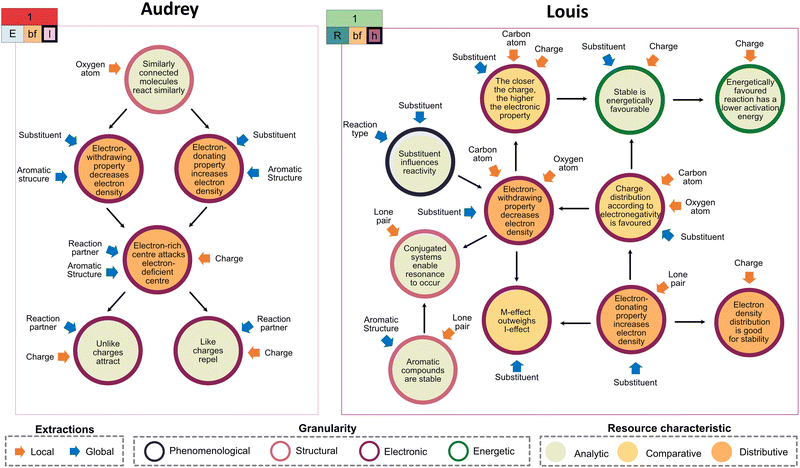 |
| Fig. 15 Resource graphs for Audrey's and Louis' problem-solving in the first task with an indication of the resource granularity (color of outer circles) and resource characteristic (color of the inner circles). Louis exhibits a productive concept projection with a reactivity (R) extraction strategy, a both-focused (bf) extraction type and a resource graph of high (h) complexity. Audrey's concept projection is unproductive and encompasses an electrostatic considerations (E) extraction strategy, a both-focused (bf) extraction type and a resource graph of low (l) complexity. | |
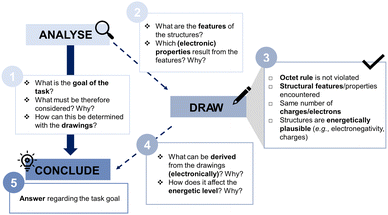 |
| Fig. 16 Possible metacognitive scaffold for supporting students' concept use in problem-solving. | |
Taking different structural features into account is beneficial for successful problem-solving. However, being able to construct valid resonance structures is a crucial prerequisite for that. Our findings revealed that students who (partially) struggled with constructing resonance structures or were uncertain about their drawings' plausibility, often avoided the resonance concept or applied it arbitrarily. Consequently, this emphasizes that drawing valid resonance structures must be sufficiently trained before it can be used in problem-solving. Besides scaffolding reflective drawing by using, for instance, checking lists, the externalization of drawing processes could help students construct resonance structures by focusing learners’ attention on relevant features (Braun et al., 2022).
There is more to the successful use of resonance besides supporting students’ reflective engagement with their constructed representations. To build a more integrative coordination class (DiSessa and Wagner, 2005), it is also crucial to use the concept in a variety of situations with differing task affordances to match resonance-related learning outcomes (e.g., assess stability, acidity, predict reaction pathways) (Carle and Flynn, 2020). The exposure to multiple contexts is necessary for building a variety of extraction strategies, extending concept projections, and, thus, coordinating a sufficient range of resources (DiSessa and Wagner, 2005). This could support a more flexible and proficient use of resonance when approaching problem-solving tasks. Unproductive extraction strategies often resulted when students relied on a fixed sequence of steps, applied overgeneralized rules, or, irrespective of the task affordances, used static approaches equalizing resonance with global stability. This heavily affected the extractions and the subsequent activation of resources. To overcome students’ static reference to stability whenever reasoning about resonance (e.g., Brandfonbrener et al., 2021), teaching should highlight more explicitly the link to the idea of electron density distribution and the energetic account. In doing so, the actual meaning of resonance would be taken more into account (Xue and Stains, 2020). Expanding tasks to the consideration of resonance beyond stability assessments can also help to overcome students’ overreliance on stability, and to deal successfully with resonance in various contexts.
Implications for research
This paper focused on the qualitative characterization of students' use of resonance in problem-solving in three contexts at one time point during the semester. Therefore, future studies could consider how students’ concept projections develop throughout the semester, what differentiates the conceptual use of experts in comparison to novices, or how effective interventions targeting the differentiated use of resonance in problem-solving are. Given some students’ difficulties in constructing resonance structures and recognizing if resonance applies (for instance, in the non-aromatic context), more research is needed to elicit the underlying reasons for students’ difficulties (e.g., how the variation of structural features contributes to the activation of resonance related resources). Our study also showed that students, in line with the resonance concept, often focus on electronic effects in their reasoning. Yet, they sometimes express alternative conceptions and use electronic effects in an overgeneralized way. Thus, students’ conceptualization of electronic effects necessitates more research.
Limitations
This qualitative study is explorative; thus, several limitations must be considered. We characterized students’ concept use in a study setting that differed from their usual instructional environment. This might have influenced their overall concept projections as well. Furthermore, given the duration of the interview, the sophistication of the explanations might have also varied due to fatigue, students’ motivation, or the prompts of the retrospective. Given that we relied in our analysis on students’ utterances, students may have paid attention to other structural features as extractions and activated other resources, which were not verbalized. As such, the result is also an artifact of the interview process, in which follow-up questions might have cued students to different aspects of the task, albeit similar for all participants. Lastly, although we iteratively and carefully characterized students’ resource activation, the constructed resource graphs represent a summary of the reasoning process, which heavily depended on students’ verbal explaining and, thus, could not display the process in detail.
Author contributions
Irina Braun: conceptualization, investigation, methodology, formal analysis, visualization, writing – original draft. Nicole Graulich: conceptualization, supervision, methodology, visualization, writing – review and editing.
Conflicts of interest
There are no conflicts to declare.
Appendices
Appendix #1: interview protocol for the retrospective
1. Please justify your answer and explain in detail your thought process for solving this task.
a. How did you know what to do? How did you get to your solution?
b. Can you please describe chronologically your created drawings (i.e., how do they develop over time?)
c. If started anew, why?
d. If problems were encountered, what were the difficulties?
2. Why did you construct the drawings, i.e., how did they help you to solve the problem?
a. What (and how) did you deduce from your single drawings?
b. What do the different components of the drawings mean (e.g., charges) with regard to the problem?
c. Why did you skip/ignore certain drawings?
d. If no drawings: Why did you solve the tasks mentally?
3. How did you construct your drawings (resonance structures)?
a. What is the rationale behind your drawings? Based on what criteria did you move from one structure to another? How did you get to your structures?
b. How do you determine that your drawings are valid?
4. On a scale of 1–5 (1 = easy, 5 = difficult), how difficult was the task completion for you?
a. Why?
b. Why was the task simpler/harder than the other tasks?
Appendix #2: codes for extractions and resources
The codes for the extractions and resources were inductively derived from the interview transcripts using in-vivo coding in a first coding circle. Subsequently, broader categories for grasping underlying ideas concerning the extractions and resources were inferred. The following examples illustrate the coding process:
Example I:
Substituent influences reactivity, exemplary student utterances:
– “Substituent influences positive charge”
– “Keto group directs meta”
– “First substituent stabilizes or destabilizes the resulting charge”
– “The substituent has a different impact, depending on its position”
Example II:
Unlike charges attract, exemplary student utterances:
– “Adjacent positive and negative charges make no sense”
– “Positive and negative charges neutralize each other”
– “Different charges attract”
– “The plus attracts electrons”
Across the different interviews, 31 resources could be found (cf. Appendix #3), as well as 17 extractions. These are listed as follows as local/global extractions:
Local |
Global |
Charge |
Number of resonance structures |
Double bond |
Reaction partner |
Single bond |
Reactant |
Oxygen atom (hetero atom) |
Product |
Lone pair/electrons |
Alkyl group |
Carbon atom |
Reaction type |
|
Aromatic structure |
|
Substituent (functional group) |
|
Geometry |
|
Conjugated system |
|
Number of bonds |
Appendix #3: overview of the different resources and their level of granularity
According to Deng and Flynn (2021), the phenomenological granularity level is describing chemical phenomena arising from molecules and atoms' interactions because of their properties. Thus, the ideas arise as a result of the granularity levels below. For example, by activating the resource The more resonance structures, the better, one states a common rule grounded in electronic and energetic considerations, i.e., the consideration of electron density distribution and its linkage to energetics. The structural level describes resources that target structural features of molecules or atoms (e.g., steric bulk, which refers to the composition of a molecule). The granularity level below, the electronic level, captures ideas referring to electronic features of molecules and atoms (e.g., electron-donating effect, the description of electron density distribution, or the reference to charge interaction). Lastly, the energetic level captures ideas targeting thermodynamic and kinetic considerations, thus, referring to the energetics of a reaction (e.g., the evaluation of varying stabilities in terms of the reaction pathway). In our data, some resources are a mixture of different granularity levels, which we classified in dependence of which level is in the foreground. For instance, the resource Aromatic compounds are stable targets both the structural and energetic levels. However, here, the structural consideration outweighs the energetic one because the conjugated ring system gives rise to higher stability.
Appendix #4: classification of students’ resource graphs
To classify students’ resulting resource graphs, we adapted Yin et al.'s (2005) coding system for the structure complexity in concept maps. As such, students’ resource graphs were classified as a whole, i.e., in dependence of their overall structure with regard to (inter-)connectedness of the different activated resources.
Appendix #5: frequency of resource activation across the different contexts
Appendix #6: relative frequency of resource activation in dependence of the productivity of the concept projection
Appendix #7: exemplary students’ resource graphs for different tasks
Acknowledgements
This publication represents a component of the first author's doctoral (Dr rer. nat.) thesis in the Faculty of Biology and Chemistry at the Justus Liebig University Giessen, Germany. We wish to thank Helena Rist for her help in data analysis. We thank all students who participated in the study as well as the members of the Graulich Research Group for their support and fruitful discussions.
References
- Airey J. and Linder C., (2009), A disciplinary discourse perspective on university science learning: Achieving fluency in a critical constellation of modes, J. Res. Sci. Teach., 46, 27–49.
- Alfieri L., Nokes-Malach T. J. and Schunn C. D., (2013), Learning through case comparisons: A meta-analytic review, Educ. Psychol., 48, 87–113.
- Anslyn E. V. and Dougherty D. A., (2006), Modern physical organic chemistry, Sausalito, California: University Science Books.
- Anzovino M. E. and Bretz S. L., (2015), Organic chemistry students' ideas about nucleophiles and electrophiles: the role of charges and mechanisms, Chem. Educ. Res. Pract., 16, 797–810.
- Anzovino M. E. and Bretz S. L., (2016), Organic chemistry students' fragmented ideas about the structure and function of nucleophiles and electrophiles: a concept map analysis, Chem. Educ. Res. Pract., 17, 1019–1029.
- Balabanoff M. E., Al Fulaiti H., Bhusal S., Harrold A. and Moon A. C., (2020), An exploration of chemistry students’ conceptions of light and light-matter interactions in the context of the photoelectric effect, Int. J. Sci. Educ., 42, 861–881.
- Barth-Cohen L. A. and Wittmann M. C., (2017), Aligning Coordination Class Theory With a New Context: Applying a Theory of Individual Learning to Group Learning, Sci. Educ., 101, 333–363.
- Benson B. K., (1997), Scaffolding, Engl. J., 86, 126–127.
- Betancourt-Pérez R., Olivera L. J. and Rodríguez J. E., (2010), Assessment of organic chemistry students’ knowledge of resonance-related structures, J. Chem. Educ., 87, 547–551.
- Brandfonbrener P. B., Watts F. M. and Shultz G. V., (2021), Organic Chemistry Students’ Written Descriptions and Explanations of Resonance and Its Influence on Reactivity, J. Chem. Educ., 98, 3431–3441.
- Braun I., Langner A. and Graulich N., (2022), Let's draw molecules: Students’ sequential drawing processes of resonance structures in organic chemistry, Front. Educ., 7, 1055280.
- Buteler L. and Coleoni E., (2016), Solving problems to learn concepts, how does it happen? A case for buoyancy, Phys. Rev. Phys. Educ. Res., 12, 020144.
- Carle M. S., El Issa R. J., Pilote N. and Flynn A. B., (2020), Ten Essential Delocalization Learning Outcomes: How Well Are They Achieved? ChemRxiv, preprint DOI:10.26434/chemrxiv-2021-c12b9-v2.
- Carle M. S. and Flynn A. B., (2020), Essential learning outcomes for delocalization (resonance) concepts: How are they taught, practiced, and assessed in organic chemistry? Chem. Educ. Res. Pract., 21, 622–637.
- Cartrette D. P. and Bodner G. M., (2009), Non-mathematical problem solving in organic chemistry, J. Res. Sci. Teach., 47, 643–660.
- Cartrette D. P. and Mayo P. M., (2011), Students' understanding of acids/bases in organic chemistry contexts, Chem. Educ. Res. Pract., 12, 29–39.
- Caspari I. and Graulich N., (2019), Scaffolding the structure of organic chemistry students’ multivariate comparative mechanistic reasoning, Int. J. Phys. Chem. Educ., 11, 31–43.
- Chi M. T., De Leeuw N., Chiu M.-H. and LaVancher C., (1994), Eliciting self-explanations improves understanding, Cogn. Sci., 18, 439–477.
- Chi M. T. H., Feltovich P. J. and Glaser R., (1981), Categorization and Representation of Physics Problems by Experts and Novices, Cogn. Sci., 5, 121–152.
- Conlin L. D., Gupta A. and Hammer D., (2010), in Ohlsson S. and Catrambone R. (ed.), Proceedings of the 32nd Annual Meeting of the Cognitive Science Society, Austin, TX: Cognitive Science Society, pp. 19–24.
- Cooper M. M., (2015), Why Ask Why? J. Chem. Educ., 92, 1273–1279.
- Cooper M. M., Corley L. M. and Underwood S. M., (2013), An investigation of college chemistry students' understanding of structure-property relationships, J. Res. Sci. Teach., 50, 699–721.
- Cooper M. M., Stieff M. and DeSutter D., (2017), Sketching the Invisible to Predict the Visible: From Drawing to Modeling in Chemistry, Top. Cogn. Sci., 9, 902–920.
- DeFever R. S., Bruce H. and Bhattacharyya G., (2015), Mental rolodexing: Senior chemistry majors’ understanding of chemical and physical properties, J. Chem. Educ., 92, 415–426.
- Demirdöğen B., Nelsen I. and Lewis S. E., (2023), Organic chemistry students’ use of stability in mental models on acid and base strength, Chem. Educ. Res. Pract., 24, 1127–1141.
- Deng J. M. and Flynn A. B., (2021), Reasoning, granularity, and comparisons in students’ arguments on two organic chemistry items, Chem. Educ. Res. Pract., 22, 749–771.
- DiSessa A. A., (1993), Toward an epistemology of physics, Cogn. Instr., 10, 105–225.
- DiSessa A. A., (2018), in Kaiser G., Forgasz H., Graven M., Kuzniak A., Simmt E. and Xu B. (ed.), Invited Lectures from the 13th International Congress on Mathematical Education, Springer, ch. 5, pp. 65–84.
- DiSessa A. A. and Sherin B. L., (1998), What changes in conceptual change? Int. J. Sci. Educ., 20, 1155–1191.
- DiSessa A. A. and Wagner J. F., (2005), in Mestre J. P. (ed.), Transfer of learning from a modern multi-disciplinary perspective, Greenwich, CT: Information Age Publishing, vol. 89, pp. 121–154.
- DiSessa A. A., Sherin B. L. and Levin M., (2016), in Levin M. and Brown N. J. (ed.), Knowledge and interaction: A synthetic agenda for the learning sciences, New York, London: Routledge, pp. 30–71.
- Domin D. S., Al-Masum M. and Mensah J., (2008), Students’ categorizations of organic compounds, Chem. Educ. Res. Pract., 9, 114–121.
- Duis J. M., (2011), Organic chemistry educators’ perspectives on fundamental concepts and misconceptions: An exploratory study, J. Chem. Educ., 88, 346–350.
- Elby A., (2000), What students' learning of representations tells us about constructivism, J. Math. Behav., 19, 481–502.
- Ferguson R. and Bodner G. M., (2008), Making sense of the arrow-pushing formalism among chemistry majors enrolled in organic chemistry, Chem. Educ. Res. Pract., 9, 102–113.
- Finkenstaedt-Quinn S. A., Watts F. M., Petterson M. N., Archer S. R., Snyder-White E. P. and Shultz G. V., (2020), Exploring Student Thinking about Addition Reactions, J. Chem. Educ., 97, 1852–1862.
- Furió C., Calatayud M. L., Bárcenas S. L. and Padilla O. M., (2000), Functional fixedness and functional reduction as common sense reasonings in chemical equilibrium and in geometry and polarity of molecules, Sci. Educ., 84, 545–565.
- Galloway K. R., Leung M. W. and Flynn A. B., (2018), A comparison of how undergraduates, graduate students, and professors organize organic chemistry reactions, J. Chem. Educ., 95, 355–365.
- Gilbert J. K., (2005), in Gilbert J. K. (ed.), Visualization in science education, Springer, pp. 9–27.
- Goodwin W., (2008), Structural formulas and explanation in organic chemistry, Found. Chem., 10, 117–127.
- Graulich N. and Bhattacharyya G., (2017), Investigating students' similarity judgments in organic chemistry, Chem. Educ. Res. Pract., 18, 774–784.
- Graulich N. and Caspari I., (2020), Designing a scaffold for mechanistic reasoning in organic chemistry, CTI, 1, 19–30.
- Graulich N. and Schween M., (2018), Concept-Oriented Task Design: Making Purposeful Case Comparisons in Organic Chemistry, J. Chem. Educ., 95, 376–383.
- Graulich N., Hedtrich S. and Harzenetter R., (2019), Explicit versus implicit similarity–exploring relational conceptual understanding in organic chemistry, Chem. Educ. Res. Pract., 20, 924–936.
- Hammer D., (2000), Student resources for learning introductory physics, Am. J. Phys., 68, S52–S59.
- Hammer D., Elby A., Scherr R. E. and Redish E. F., (2005), in Mestre J. P. (ed.), Transfer of learning from a modern multidisciplinary perspective, Greenwich, CT: Information Age Publishing, vol. 89, pp. 89–120.
- Hoffmann R. and Laszlo P., (1991), Representation in chemistry, Angew. Chem., Int. Ed. Engl., 30, 1–16.
- Hogan K. and Maglienti M., (2001), Comparing the epistemological underpinnings of students' and scientists' reasoning about conclusions, J. Res. Sci. Teach., 38, 663–687.
- Hutchison P. and Hammer D., (2010), Attending to student epistemological framing in a science classroom, Sci. Educ., 94, 506–524.
- Kim T., Wright L. K. and Miller K., (2019), An examination of students' perceptions of the Kekulé resonance representation using a perceptual learning theory lens, Chem. Educ. Res. Pract., 20, 659–666.
- Kintsch W., (1988), The role of knowledge in discourse comprehension: a construction-integration model, Psychol. Rev., 95, 163–182.
- Klein D., (2012), Organic chemistry, John Wiley & Sons, Inc.
- Kozma R. B. and Russell J., (1997), Multimedia and understanding: Expert and novice responses to different representations of chemical phenomena, J. Res. Sci. Teach., 34, 949–968.
- Kozma R. and Russell J., (2005), in Gilbert J. K. (ed.), Visualization in science education, Dordrecht: Springer, pp. 121–145.
- Kriz S. and Hegarty M., (2007), Top-down and bottom-up influences on learning from animations, Int. J. Hum. Comput. Stud., 65, 911–930.
- Kuckartz U., (2013), Qualitative text analysis: A guide to methods, practice and using software, Los Angeles: SAGE Publications.
- Landis J. R. and Koch G. G., (1977), The Measurement of Observer Agreement for Categorical Data, Biometrics, 33, 159–174.
- Louca L., Elby A., Hammer D. and Kagey T., (2004), Epistemological resources: Applying a new epistemological framework to science instruction, Educ. Psychol., 39, 57–68.
- Maeyer J. and Talanquer V., (2013), Making predictions about chemical reactivity: Assumptions and heuristics, J. Res. Sci. Teach., 50, 748–767.
- McClary L. and Talanquer V., (2011), Heuristic reasoning in chemistry: Making decisions about acid strength, Int. J. Sci. Educ., 33, 1433–1454.
- Ogilvie W., Ackroyd N., Browning C. S., Deslongchamps G. and Sauer E., (2018), Organic Chemistry: Mechanistic Patterns, Nelson.
- Parnafes O., (2007), What Does “Fast” Mean? Understanding the Physical World Through Computational Representations, J. Learn. Sci., 16, 415–450.
- Parnafes O. and DiSessa A. A., (2013), Microgenetic learning analysis: A methodology for studying knowledge in transition, Hum. Dev., 56, 5–37.
- Petterson M. N., Watts F. M., Snyder-White E. P., Archer S. R., Shultz G. V. and Finkenstaedt-Quinn S. A., (2020), Eliciting student thinking about acid–base reactions via app and paper–pencil based problem solving, Chem. Educ. Res. Pract., 21, 878–892.
- Pölloth B., Diekemper D. and Schwarzer S., (2023), What resources do high school students activate to link energetic and structural changes in chemical reactions? – A qualitative study, Chem. Educ. Res. Pract., 24, 1153–1173.
- Popova M. and Bretz S. L., (2018a), “It's Only the Major Product That We Care About in Organic Chemistry”: An Analysis of Students’ Annotations of Reaction Coordinate Diagrams, J. Chem. Educ., 95, 1086–1093.
- Popova M. and Bretz S. L., (2018b), Organic chemistry students’ interpretations of the surface features of reaction coordinate diagrams, Chem. Educ. Res. Pract., 19, 919–931.
- Richards A. J., Jones D. C. and Etkina E., (2018), How Students Combine Resources to Make Conceptual Breakthroughs, Res. Sci. Educ., 50, 1119–1141.
- Richardson W., (1986), Teaching the concept of resonance with transparent overlays, J. Chem. Educ., 63, 518–519.
- Rodriguez J.-M. G., Bain K., Hux N. P. and Towns M. H., (2019), Productive features of problem solving in chemical kinetics: more than just algorithmic manipulation of variables, Chem. Educ. Res. Pract., 20, 175–186.
- Rodriguez J.-M. G., Stricker A. R. and Becker N. M., (2020a), Exploring the Productive Use of Metonymy: Applying Coordination Class Theory to Investigate Student Conceptions of Rate in Relation to Reaction Coordinate Diagrams, J. Chem. Educ., 97, 2065–2077.
- Rodriguez J.-M. G., Stricker A. R. and Becker N. M., (2020b), Students’ interpretation and use of graphical representations: insights afforded by modeling the varied population schema as a coordination class, Chem. Educ. Res. Pract., 21, 536–560.
- Russ R. S., Scherr R. E., Hammer D. and Mikeska J., (2008), Recognizing mechanistic reasoning in student scientific inquiry: A framework for discourse analysis developed from philosophy of science, Sci. Educ., 92, 499–525.
- Saldaña J., (2016), The coding manual for qualitative researchers, Los Angeles: Sage Publications.
- Sheppard M. A. W. and Bauer C. F., (2022), Student Conceptions of pH Buffers Using a Resource Framework: Layered Resource Graphs and Levels of Resource Activation, J. Chem. Educ., 100, 22–33.
- Strauss A. and Corbin J., (1990), Basics of Qualitative Research: Grounded Theory Procedures and Techniques, Newbury Park, CA: Sage publications.
- Sweller J., (1988), Cognitive load during problem solving: Effects on learning, Cogn. Sci., 12, 257–285.
- Taber K. S., (2002), Compounding quanta: Probing the frontiers of student understanding of molecular orbitals, Chem. Educ. Res. Pract., 3, 159–173.
- Talanquer V., (2022), The Complexity of Reasoning about and with Chemical Representations, JACS Au, 2, 2658–2669.
- Tannen D., (1993), Framing in discourse, New York: Oxford University Press.
- Tetschner G. C. and Nedungadi S., (2023), Obtaining Validity Evidence During the Design and Development of a Resonance Concept Inventory, J. Chem. Educ., 100, 3795–3805.
- Trabert A. and Schween M., (2020), How do electronic substituent effects work?–Additional contrasting cases for a differentiated inquiry illustrated by the example of alkaline ester hydrolysis, CHEMKON, 27, 22–33.
- Van Meter P. and Garner J., (2005), The Promise and Practice of Learner-Generated Drawing: Literature Review and Synthesis, Educ. Psychol. Rev., 17, 285–325.
- Wagner J. F., (2006), Transfer in pieces, Cogn. Instr., 24, 1–71.
- Wittmann M. C., (2002), The object coordination class applied to wave pulses: Analysing student reasoning in wave physics, Int. J. Sci. Educ., 24, 97–118.
- Wittmann M. C., (2006), Using resource graphs to represent conceptual change, Phys. Rev. Spec. Top. Phys. Educ. Res., 2, 020105.
- Wood D., Bruner J. S. and Ross G., (1976), The role of tutoring in problem solving, J. Child Psychol. Psychiatry, 17, 89–100.
- Xue D. and Stains M., (2020), Exploring students’ understanding of resonance and its relationship to instruction, J. Chem. Educ., 97, 894–902.
- Yin Y., Vanides J., Ruiz-Primo M. A., Ayala C. C. and Shavelson R. J., (2005), Comparison of two concept-mapping techniques: Implications for scoring, interpretation, and use, J. Res. Sci. Teach., 42, 166–184.
- Yuriev E., Naidu S., Schembri L. S. and Short J. L., (2017), Scaffolding the development of problem-solving skills in chemistry: guiding novice students out of dead ends and false starts, Chem. Educ. Res. Pract., 18, 486–504.
|
This journal is © The Royal Society of Chemistry 2024 |