Development of an automated platform for monitoring microfluidic reactors through multi-reactor integration and online (chip-)LC/MS-detection†‡
Received
2nd January 2024
, Accepted 20th March 2024
First published on 20th March 2024
Abstract
This work presents a novel microfluidic screening setup with real-time analytics for investigating reactions with immobilised biocatalysts. The setup combines microreactor technology, multi-reactor integration, and online (chip-)LC/MS analysis in a sequential automated workflow. We utilized in-house manufactured fused-silica glass chips as reusable packed-bed microreactors interconnected as individual tube reactors. The potential of this setup was showcased by conducting and optimising a biocatalytic aromatic bromination reaction as the first proof of concept using immobilised vanadium-dependent haloperoxidase from Curvularia inaequalis (CiVHPO). The fusion of a HaloTag™ to CiVHPO was used for efficient and mild covalent linkage of the enzyme onto chloroalkane-functionalized particles. Then, the biotransformation was continuously monitored with automated LC/MS data acquisition in a data-rich manner. By further developing the automation principle, it was possible to sequentially screen multiple different connected packed-bed microreactors for reaction optimization while using only miniature amounts of reactants and biocatalyst. Finally, we present a fast and modular chipHPLC solution for online analysis to reduce the overall solvent consumption by over 80%. We established a modern microfluidic platform for real-time reaction monitoring and evaluation of biocatalytic reactions through automation of the reactant feed integration, flexible microreactor selection, and online LC/MS analysis.
Introduction
The efficient utilisation of biocatalysis plays a pivotal role in achieving the objective of implementing environmentally-friendly chemistry in industrial processes.1,2 Enzymes enable highly selective reactions under mild and economically friendly conditions, leading to their increasing commercial use, including the synthesis of valuable fine chemicals and small-molecule active pharmaceutical ingredients (APIs).3,4
The remarkable surge in enzyme-based processes can be attributed to advances in biotechnology and enzyme engineering,5,6 such as advanced DNA sequencing of genomes, directed evolution, especially in combination with genetic code expansion, and computer-assisted methods.7,8 These developments have facilitated the discovery and development of new, more robust, and powerful enzymes which can also be optimised for non-natural substrates, thus expanding biocatalytic methodologies. Some researchers even consider this period to be the “Golden Age of Biocatalysis”.9
These novel enzymes can be made even more robust by immobilisation,10–12 for which a wide selection of solid support materials and linking methods are available.13,14 Next to considerable cost reduction through increased material stability, a more comprehensive range of reaction conditions can be used in water-immiscible organic solvents.15
Furthermore, enzyme immobilisation not only simplifies the handling of the biocatalysts and the separation of the product from it, but also enables the transition of biocatalytic processes into continuous flow systems as immobilised enzyme reactors (IMERs).16–20 Various conventional continuous flow technologies have already been developed and are commercially available,21–24 offering significant benefits, including process simplification, improved and consistent product quality, easier downstream processing, and the integration of online analytics.
Biocatalysis can benefit significantly from using smaller reaction spaces, particularly in microreactors. The literature shows an increasing number of biotransformations in the microflow regime,25,26 often utilising so-called microfluidic immobilised enzyme reactors (μ-IMERs).26–30 For both IMERs and their microfluidic versions, a range of online analytical methods, including chromatographic and spectroscopic techniques,31–34 were tested in combination with these systems for comprehensive evaluation of the reaction outcome and performance.
Downsizing of chemical flow reactions has, in general, several advantages. This reduces the consumption of reactants such as high-value APIs, immobilised catalysts, and expensive cofactors and improves heat and mass transfer, real-time process control, and mixing behaviour in a shorter time and spatial dimension.35,36 This approach allows processes to be conducted more safely and reduces waste generation. The enhanced flow control in continuous microfluidic systems also facilitates automation and parallelisation,37,38 leading to the development of several low-scale synthesizers.39,40
The field of reactor technology is rapidly advancing, driven by developments in data processing, which encompass the utilisation of recently matured data science disciplines,41–44 machine learning,45,46 and sophisticated artificial intelligence methods.47–49 This inflicts various discussed research areas such as microfluidics,50–52 enzyme development,53–57 or reaction optimisation.58–60 The implementation of these methods underlines the growing necessity for reliable, rapid raw data generation and instrumental hardware control, preferably within continuous flow systems integrated with real-time online analysis.61
For biocatalysis, however, there currently needs to be automated and data-rich methods for rapid and reliable validation of immobilised enzymes and screening of biotransformations.62 Consequently, tedious manual reaction screening is often required. Microfluidic devices are particularly suited for this purpose due to their low consumption, precise fluidic control, and versatility for their integration as either highly integrated or modular lab-on-a-chip devices.63–65
To address this challenge, we propose a biocatalytic automated screening platform in microflow coupled with online LC/MS detection. We recently investigated biotransformations in a digital microfluidic (DMF) approach.66 Building on our previous work with various integrated microfluidic devices for studying immobilised organocatalysts,67–69 we have now transitioned these biotransformations into a microflow platform, leading to improved automation, reactor control, and expanded applications in biocatalytic processes.
We used the vanadium-dependent haloperoxidase (CiVHPO) from the fungus Curvularia inaequalis as a model enzyme for evaluating a biocatalytic bromination reaction.70–72 The enzyme exhibits remarkable properties, including high stability to heat and organic solvents, a broad substrate scope, and the ability to use H2O2 as an easily accessible cosubstrate.73 As a result, numerous papers have been published in recent years utilizing the efficient introduction of halogen atoms by CiVHPO in a wide range of organic compounds.74–80 We decided to covalently attach the CiVHPO to the solid support for better handling. Therefore, we genetically added the HaloTag™ to our protein of interest (CiVHPOHalo) and then linked it via a chloroalkane to ProntoSIL particles. The HaloTag™ method is highly selective and oriented, enabling reproducibility and easy adaptation to other biocatalysts.81–84
After immobilisation, the enzyme was packed into a custom-made microfluidic fused-silica glass chip and was evaluated using the presented system. For screening applications, this setup has been designed to sequentially address multiple parallel packed-bed microreactors with different content or reactants in an automated workflow that allows for high variability. The approach minimises the reactant and catalyst consumption, emphasising the efficiency of this screening platform with minimal laboratory effort. We integrated online analysis to enable continuous, long-term monitoring by coupling the system with LC/MS and implementing automated injections. Additionally, we propose miniaturising the analytical method using chipHPLC, leveraging our previous expertise in this area,85–88 to significantly reduce solvent consumption in the analytical section and accelerate the separation time (Fig. 1).
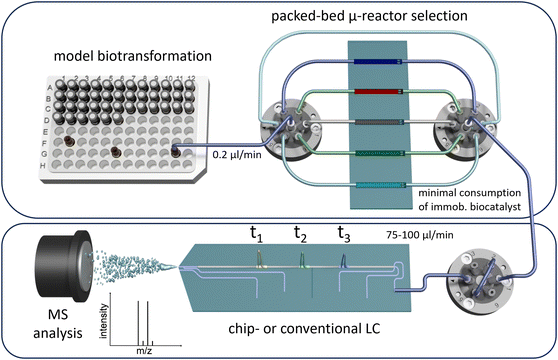 |
| Fig. 1 Schematic sketch of the presented packed-bed multi-microreactor setup with automated reactor selection and on-line (chip)-LC/MS-detection. | |
Results and discussion
Long-term studies for immobilised enzyme evaluation
We first immobilised the CiHVPO enzyme using the HaloTag™ immobilisation technique as a highly selective and oriented one-step covalent linking method under mild conditions. Having sorted out a reproducible covalent linking of our enzyme to silica particles, we packed the material inside a packed-bed microreactor and evaluated it in continuous flow using an aromatic bromination of pyrroles as model biotransformation. Various runs are presented below to illustrate the application of the setup for continuous flow microreactor sequencing. These measurements were conducted to establish the following points: first, to confirm stable enzyme activity over several days; second, to demonstrate that no enzyme leakage is observed with covalent HaloTag™ immobilisation; and third, to validate stable and reproducible reaction conversion for our model biotransformation. To achieve this, the microreactor was monitored for approx. 22 h, chromatograms were measured every 15 min (Fig. 2A), and a constant reactant stream was applied. Two consecutive runs of the same microreactor were then carried out as recycling experiments, with the reactor washed with buffer overnight after each run (Fig. 2B) and the fraction of the product 2 signal visualised (for relative evaluation, only one brominated product isotope considered). These long-term monitoring runs of the immobilised enzyme successfully demonstrated good operational stability with only a slight drop in biocatalytic activity over time. Interestingly, some enzyme activity was regained during the overnight washing step.
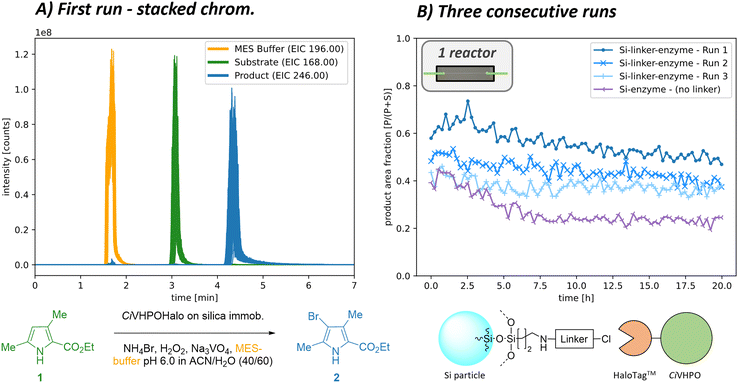 |
| Fig. 2 Monitoring the performance of the immobilised CiVHPOHalo enzyme in a packed-bed microreactor by long-term experiments of the bromination model reaction (scale of 1 10 mM) with constant reactant feed and online HPLC/MS-detection. For these runs, no byproduct formation was visible. A) Stacked view of all acquired chromatograms (run 1: n = 80 chromatograms, approx. 20 h, sampling each 15 min). Three peaks are shown as dominant species, indicating the buffer, reactant 1, and brominated product 2 (EIC only for one brominated product isotope shown). B) Visualisation of the product area fraction in relation to the reactant species over time for three consecutive runs using the same packed-bed microreactor (only one brominated product 2 isotope area considered for visualization). The reactor was flushed overnight with buffer, before introducing a new reaction sample (run 2 & 3: each n = 80; each approx. 22 h). Reactor: packed with CiVHPOHalo on ProntoSil particles (∅ 5 μm, loading f = 10.4 μg mg−1), rct. pump: 0.2 μl min−1 50 mM MES-buffer (residence time approx. 40 s, flushing sample loop with 2 μl min−1 for 3 min at start; dilution: 2 μl min−1 MeCN : H2O, 60 : 40 vol% with 50 mM MES as sample); analysis: Zorbax Eclipse Plus (C18, 4.6 × 100 mm, 3.5 μm, Agilent), 600 μl min−1 MeCN : H2O (70 : 30 vol% with 0.1% FA), 60 bar at pump, 2 μl injection volume. | |
To confirm the selective immobilisation only via the HaloTag™, measurements were conducted with particles without chloroalkane linker. In this case, the enzyme was presumably attached to the ProntoSIL particles through non-covalent interactions during the enzyme immobilisation process. These interactions, however, proved insufficient to withstand the flow conditions, resulting in a significant loss of activity due to clearance of the enzyme from the reactor over time (Fig. 2B). Similarly, blank ProntoSil particles with a linker but without enzyme addition showed no conversion at all. Additional information and further runs at different reaction conditions can be found in the ESI† at section S5.1.
Multi-reactor screening approach
Two multi-selector valves were integrated into the setup to increase control for reaction monitoring or screening purposes, allowing for switching between several different microreactor positions during individual pre-programmed runs. This configuration enables easy integration of multiple packed-bed microreactors in parallel. In addition, a blank capillary was added at the first position, which facilitates acquiring blank measurements of the reactant feed or sole pump feed while bypassing the reactor. Furthermore, by switching off the reactant feed, reactor positions can be flushed between runs, and the efficiency of the washing process can be monitored. An initial validation run using a single connected packed-bed microreactor and a blank capillary is described in the ESI† in section S5.2. A constant reactant feed was provided for that run and then directed to the two reactor positions. Monitoring the signals during reactor switching, the flow behaviour was investigated and validated that no carry-over effects were present.
To further enhance the flexibility of the reactant feed, an autosampler was integrated as an automated tool for reactant feed variation, allowing for sequential screening of various manually prepared samples in an automated workflow. Due to the low reactor flow rate, the autosampler loop provided a constant reactant feed for multiple hours (40 μl, 0.2 μl min−1, approx. 160 min reactant plug plateau). Thus, the platform can be used for testing different reaction conditions, which, combined with the selector valves, can be addressed to multiple different microreactors while minimising enzyme and reactant consumption. This functionality was demonstrated by sequentially testing five adjacent freshly packed reactor channels with different reaction mixtures in a single automated run, as shown in Fig. 3 (all channels packed with CiVHPOHalo immobilised particles; last reactor only half-packed for comparison; sampling each 10 min, with each reactor run approx. 160 min and n = 16 chromatograms; in total n = 258 chromatograms, approx. 43 h).
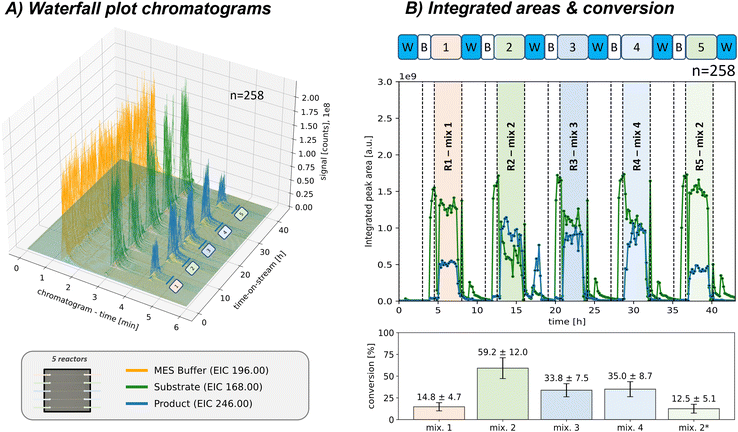 |
| Fig. 3 Automated sequential sample screening approach for monitoring five connected packed-bed microreactors (n = 258 chromatograms, approx. 43 h, sampling each 10 min). Each reactor position selected (pos. 2–6) were sampled by the autosampler with a varying reaction mixture (40 μl sample, each reactor run n = 16, approx. 160 min; all sample compositions in the ESI† in Table S1). For comparison was the last microreactor channel only half-packed (*). Before each reactor run, multiple blank acquisitions were acquired for pump or reactant feed observation and likewise, a washing step was conducted after each reactor run (pos. 1: blank capillary). A) Waterfall diagram of all acquired chromatograms. (EIC only for one brominated product 2 isotope shown) B) integrated areas of the reactant 1 and product 2 (only one brominated product 2 isotope shown here). The reactant 1 conversion is also shown, calculated by comparing the peak area of the reactant 1 bypassing the reactor before the run with the actual run. Detailed description of the sequence, reaction parameters and information on byproduct S2–S5 formation can be found in the ESI† in section S4.3. Reactor: packed with CiVHPOHalo on ProntoSil particles (∅ 5 μm, loading f = 20.6 μg mg−1), rct. pump: 0.2 μl min−1 50 mM MES-buffer (residence time approx. 40 s, no dilution); analysis: Zorbax Eclipse Plus (C18, 4.6 × 100 mm, 3.5 μm, Agilent), 600 μl min−1 MeCN : H2O (70 : 30 vol% with 0.1% FA), 51 bar at pump, 0.2 μl injection volume. “w”/“B”: washing/blank pump, “r”: blank sample, “R1”: reactor run. | |
Blank measurements bypassing the reactor were performed before each separate run to observe the pump or reactant feed background before injecting the sample into the reactor. Those measurements could directly be used to calculate the reactant 1 conversion in the subsequent reactor runs (Fig. 3B). Additionally, washing steps were conducted after each run to flush the respective reactant.
As proof of principle, the effect of varying reactant 1 concentrations of hydrogen peroxide, sodium vanadate, and ammonium bromide on the examined biocatalytic bromination reaction was screened (detailed reactant compositions in the ESI† at Table S1). A higher hydrogen peroxide proportion (R2: 1.75 eq. H2O2 and 2 eq. NH4Br instead of initial R1: 1.0 eq. and 7.0 eq. mM, respectively) resulted in a higher conversion and product 2 area fraction. However, this led to byproduct formation, which was assigned as an oxygenated species S2–S5 (not shown here; discussion in ESI† section S4.3).
Further increasing the vanadium cofactor (R3: 2 eq. Na3VO4 instead of initial R2: 1 eq.) or the ACN fraction (R4: 70% ACN instead of initial R2: 40%) led to less total reactant conversion, which can be attributed to reduced byproduct S2–S5 formation. At the same time, the product 2 signal remained consistently high. As expected, the last microreactor, containing only a half-packed column (R5), resulted in less total conversion and product 2 formation.
Overall, the automated multi-reactor screening platform demonstrated the capability for efficient and rapid screening of various reaction samples with different compositions, allowing for fast data generation and optimisation of biocatalytic transformations or as validation feedback for enzyme optimization processes (automation and sequencing details, as well as additional experimental details, can be withdrawn from the ESI† at section S5.2).
Integration of chipHPLC analysis
As demonstrated above, our microreactor setup allows for long-time measurements with low consumption of reactants and solvents and the reusability of our biocatalyst, making it an efficient and sustainable method. However, compared to the microreactor, using a commercially available C18-column in the online analytic setup still involves relatively high solvent consumption (600 μl min−1 or approx. 864 ml d−1). To further reduce solvent usage, we integrated a chipHPLC-setup as a modular alternative to the commercially used C18-column (Fig. 4A; the simplified setup is based on a recent joint publication88). Initially, the chipHPLC-setup was integrated, the injection principle visualised, and the duty cycle was automated similarly (detailed description in the ESI† in section S3). The separation was optimized by sampling the reaction performed in batch through a syringe as sample feed. Subsequently, the chipHPLC was coupled to a packed-bed microreactor, as presented above. The achieved separation is presented in Fig. 4B. Herein, the chipHPLC system offered a significantly faster separation time below 2 min, leading to an increased acquisition frequency and, thus, faster real-time monitoring. In addition, such short acquisition times could be used in the future, for instance, in kinetic studies of the initial phase of a catalysed reaction. The system also demonstrated good operational stability with minimal solvent consumption down to 75 μl min−1 or approx. 108 ml d−1, resulting in a reduction of >80%.
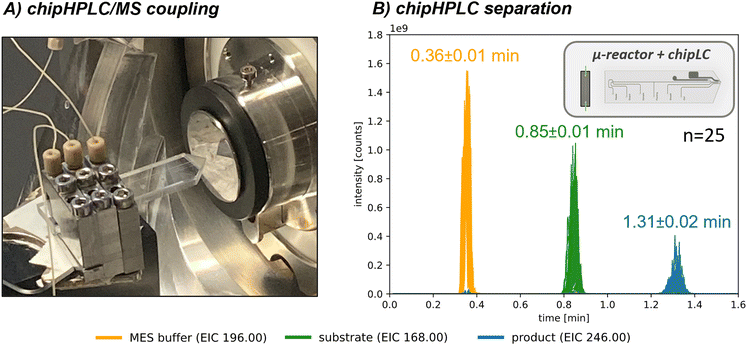 |
| Fig. 4 Integration of chipHPLC as low solvent consumption alternative to conventional LC/MS in the analytical setup. A) Photograph of the chipHPLC positioned in front of the ESI-source. B) Example of the achieved chipHPLC separation for the model reaction coupled to a packed-bed microreactor (n = 25, approx. 2 h, sampling each 5 min; EIC only for one brominated product 2 isotope shown). Reactor: packed with CiVHPOHalo on ProntoSil particles (∅ 5 μm), rct. pump: 0.2 μl min−1 50 mM MES (residence time approx. 40 s, flushing sample loop with 2 μl min−1 for 3 min at start, dilution: 2 μl min−1 MeCN : H2O, 60 : 40 vol% with 50 mM MES as sample); analysis: Xbridge particles 35 mm column length (C18, ∅ 2.5 μm, Agilent), eluent flow: 75 μl min−1 MeCN : H2O (50 : 50 vol% with 0.1% FA), during elution mode: 72 bar at pump, 70 bar at chip, 5 μl injection volume, 4 s injection time. | |
The rapid analysis time with minimal consumption demonstrates this modular approach as optimal for analytical setup integration. Further information on the injection principle and conducted chipHPLC runs, including pressure data, a long-term stability test, and a detailed comparison of the different methods, can be found in the ESI† in section S5.3.
Methods
Microfluidic devices and preparational steps
Microreactor-chip.
The fused-silica glass chips used in this study as packed-bed microreactors were manufactured by an in-house method, using a selective laser-induced etching (SLE) process, wafer-to-wafer alignment, and direct glass–glass bonding, followed by a high-temperature fusion bonding step of two structured wafers with subsequent chip dicing. The chip layout was created using CAD-software Autodesk Inventor Professional 2019 (San Rafael, CA, USA) and converted with CAM-software (Alphacam 2017 R2, Vero Software GmbH, Neu-Isenburg, DE) into a corresponding toolpath for the SLE-device (FEMTOprint f200 aHead P2, Muzzano, CHE). An ultrashort pulsed IR-laser (1030 nm, 400 fs, 230 nJ) was employed to structure the design onto a 4-inch fused silica wafer (thickness 1 mm), followed by a wet chemical-etching step in hot potassium hydroxide solution (8 M, 85 °C, 6 h). A detailed description of the manufacturing process can be found in the ESI† in section S1.1.
As shown in Fig. 5A, the chip design consists of multiple adjacent reactor channels located directly after the chip inlets. Each channel features a semi-circular reactor-bed (measured 76 × 26 × 2 mm; e.g., 15 reactor channels, each with 19 mm length, 150 μm width, and 65 μm depth, resulting in an empty reactor volume of approx. 265 nl and 132 nl packed). Each reactor channel can be separately filled with particulate material and terminates in an integrated weir-structure or μfrit, which retains the packed particles via the keystone effect (similar as we previously presented,89 μfrit shown in Fig. 5C, μfrit channels measuring 150 μm in length, 15–20 μm in width and depth). Beyond the weir-structure, there is an additional short channel (0.9 mm) before reaching the chip outlet. The conical inlet and outlet holes of the channels can be connected by utilising a custom-built high-pressure steel clamp system, allowing for a world-to-chip connection.90 The prepared packed-bed flow reactor can then be directly integrated into the fluidic setup by connection to the reactant feed. For reusability, the weir-structure is positioned on only one side of the chip, enabling reactor operation in a single direction and the possibility of subsequent reactor recycling by flushing the reactor in the opposite direction. This allowed for efficient removal of the particulate material and facilitated the repacking of the reactor with different materials.
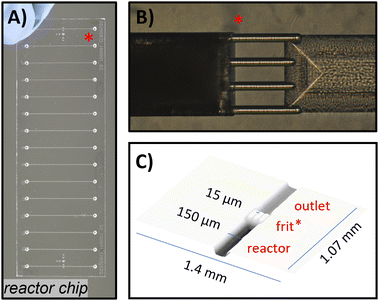 |
| Fig. 5 Fused-silica glass chip used as packed-bed microreactors. A) Photograph of a fully manufactured chip with adjacent 15 microreactor channels. B) Microscopic picture of the integrated μfrit as particle retaining structure, with an already packed channel on the left side. C) Dimensions of the μfrit by laser-scanning microscopic measurements (detailed information can be found in the ESI† section S1.1). | |
LC-chip.
For the final integrated chip-based separations, a bonded borosilicate glass chip was utilised, which was manufactured externally according to an in-house protocol (by iX-factory GmbH, now part of Micronit GmbH, Dortmund, Germany), including conventional photolithography, wet etching, and subsequent high-temperature fusion bonding (a process also described elsewhere,86,90–92 chip dimensions: 45 × 10 × 2.2 mm). In general, the chip design, as shown in Fig. 6, consists of an integrated injection-cross connected to a slurry-packed chromatographic column located in a separation channel with a semicircular cross-section (35 mm length, 90 μm max width, 40 μm depth). At both channel ends, photopolymerised frits were integrated as particle-retaining structures. As stationary phase material, either XBridge (C18, ∅ 2.5 μm, Waters, Milford, USA) or Poroshell particles (C18, ∅ 2.7 μm, Agilent, Santa Clara, USA) were used and packed into the separation column through an additional packing channel. The packing channel was then sealed with a photopolymerised plug, and a monolithic pyramidal electrospray emitter was ground and hydrophobised before use. Similar to the μreactor chip, all fluidic connections were realised by high-pressure clamps as described above.90 More details of the preparation steps can be found in the ESI† in section S1.2.
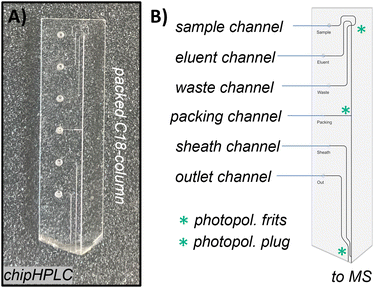 |
| Fig. 6 Borosilicate glass chipHPLC with integrated injection cross, packed separation column, and monolithic electrospray emitter (as we presented before). A) Photograph of a fully manufactured chip. B) Schematic sketch of the chipHPLC describing all inlets and modifications for integration of the separation column (detailed information can be found in the ESI† section S1.2). | |
Slurry-packing.
In both chip variants, the particles were integrated by slurry-packing (either for the biocatalytic material or as a separation column). The chip was positioned in an ultrasonic bath and connected to an HPLC-pump and 6-port-valve with a slurry-filled sample loop (approx. 2–3 mg ml−1 particle slurry). The process could be reversed for the reactor chip by flushing the reactor from the opposite direction.
Fluidic circuit and instrumental setup
The following describes the fluidic instrumental setup, including all components and connections. The setup is shown schematically in Fig. 7A and can be divided into one section for continuous microreactor operation and another section for online LC/MS-analysis, which can be performed by either a conventional or a chipHPLC approach.
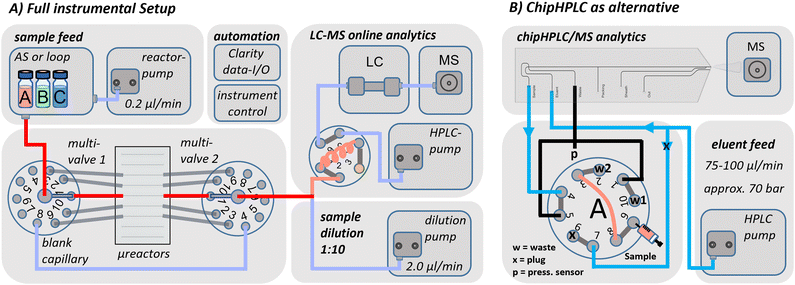 |
| Fig. 7 A) Schematic of the instrumental setup for continuous microreactor operation with LC/MS-detection. The integrated selector valves enabled the selection of up to 10 different microreactor positions of the fused-silica glass chip, whereas the first connection was used mostly for an additional blank capillary. B) Variation of the analytical section of the instrumental setup for low consumption chipHPLC integration. Detailed description of the injection principle and capillary length list can be found in the ESI† in section S2. | |
All system flows were generated using three HPLC pumps in combination. The first pump provided the sample stream through the microreactor at a relatively low flowrate (0.2 μl min−1, 40
:
60 ACN
:
H2O (v/v) + 50 mM MES-buffer pH 6.0; LC-20 AD, Shimadzu, Kyoto, Japan). To provide the respective reactant feed, either a sample loop was connected to an additional valve (e.g., 0.3–1 ml sample reservoir for long-term monitoring experiments; refillable via an external PEEK needle port adapter) or an autosampler to facilitate sample variation during individual runs (40 μl loop, G1377A, Agilent). In certain runs, a second pump was used for sample dilution past the microreactor positions before online analysis (1
:
10 dilution, 1260 Infinity isocratic HPLC pump, Agilent). The final pump was utilised in the analytical section to generate the eluent flow for LC/MS-analysis using a binary pump (600 μl min−1, 70
:
30 ACN
:
H2O (v/v); 1260 Infinity binary HPLC pump, Agilent). The fluidic circuit was realised by combining multiple Nanovolume valves (either two-position or selector valves, with 360 μm connections, Cheminert, VICI AG, Schenkon, Switzerland), which were connected through commercially available PEEK and FS capillary tubing (mostly 360 μm OD, 50–100 μm ID, VICI AG) and high-pressure PEEK fittings on a custom metal stage. The microreactors, connected between the two selector valves, can be selected from ten different positions by switching the fluidic path of the two valves for increased flexibility during automated reaction monitoring.
For online analysis, the reactor stream was guided through an additional 2-position valve (either 0.2 or 2 μl injection loop, depending on whether a reactant sample feed dilution was used), which could be sequentially sampled onto a chromatographic column (Zorbax Eclipse Plus C18, 4.6 × 100 mm, 3.5 μm, Agilent) and detected by an ESI-MS-system (AmaZon SL, Bruker Daltonics GmbH, Bremen, Germany).
Electric actuators controlled all valves, while the operation was automated by a Clarity chromatography data station combined with a Colibrick A/D converter box (DataApex, Prague, Czech Republic). These modules enhanced pump control and pressure monitoring and enabled automated injection sequences of the reactor effluent for LC/MS-detection. This included sampling every 10–15 min and starting acquisitions by connecting to the auxiliary ports of the mass spectrometer. In addition, it was possible to sequentially address multiple microreactor positions in single runs with individual reactant sampling by controlling the selector valves and the connected autosampler. Further information about the instrumental setup and a detailed description of the automation principle, auxiliary connections, and sequencing for multi-reactor operation can be found in the ESI† in section S2 & S3.
ChipHPLC integration to the instrumental setup
A modified analytical section was later employed to enable chip-based separations as a fast but low-consumption alternative to the commercially used C18-column (the general chipHPLC-setup was based on a recent joint publication88). For that purpose, the fluidic connections of the setup were modified according to Fig. 7B, and the custom metal stage was connected directly to the MS source. The chip was mounted on an xyz-linear translation stage (T12XYZ, Thorlabs GmbH, Dachau, Germany), and the chip emitter was precisely positioned in front of the ESI-inlet. Furthermore, the flow rate of the binary eluent pump was lowered accordingly. Initially, the injection principle was evaluated and visualised by a sampling of fluorescent dyes. Then, the duty cycle of the injection principle was similarly automated as before (more details on the setup can be found in the ESI† in section S2.2 and for the injection principle in S5.3).
Immobilisation strategy and model reaction
Enzyme immobilisation.
Typically, 10 mg NH2-coated ProntoSIL was suspended in ethanol (1 ml) followed by addition of the HaloTag™-targeting chloroalkane linker (S1, for structure, see ESI† Fig. S14; 104 μl, 100 mM stock solution in ethanol) and trimethylamine (5.0 μl). After incubation at rt for 16 h at 1200 rpm shaking, the particles were centrifuged and washed with ethanol (3 × 1 ml). Linker loading was determined afterward by Kaiser Test (for detailed information, see ESI† section S4.2).93 For reactor runs using particles without a linker the same strategy was used. However, only ethanol was added instead of the HaloTag™-targeting chloroalkane linker S1 solution. Finally, CiVHPOHalo (15.9 μl, 3.142 μg μl−1 stock solution) and buffer (484 μl, containing 50 mM Tris pH 6.0 and 100 μM Na3VO4) were added to the particles, and the mixture was incubated at rt for 1 h at 1000 rpm shaking. The particles were washed with MES buffer (3 × 1 ml). Interestingly, after immobilisation, a good retention of 68% of the initial activity for CiVHPOHalo on ProntoSIL was observed (for detailed information, see ESI† Fig. S20).
Enzyme quantification.
The enzyme loading on the solid support was determined by quantifying the non-bound enzyme concentrations of CiVHPOHalo in the supernatants using the monochlorodimedon (MCD) Assay (for detailed information, see ESI† Fig. S19) after the immobilisation protocol.94 By measuring the decrease of MCD absorbance (290 nm) over time, enzyme concentrations could be calculated using a calibration slope. With this information, the amount of enzyme on the particles was determined. Usually, 7.47–20.6 μg CiVHPOHalo was immobilised per 1 mg of chloroalkane-modified particle. CiVHPOHalo was also immobilised on the amine functionalised ProntoSIL particles by non-covalent interactions (up to 12.2–18.8 μg of CiVHPOHalo was immobilised per 1 mg of amine particle).
Biocatalytic transformations.
As a model reaction for the presented integrated system, the bromination of ethyl-3,5-dimethyl pyrrole-2-carboxylate (1, 10 mM) to ethyl-4-bromo-3,5-dimethyl pyrrole-2-carboxylate (2) was investigated as shown in Fig. 2A. The standard reaction mixture consisted of MES-buffer (pH 6.0, 50 mM) in 40
:
60 ACN
:
H2O (v/v), with NH4Br as bromination source (70 mM), Na3VO4 as cofactor (1 mM), and H2O2 as oxidation agent (10 mM). A detailed overview of further tested compositions and potential side reactions can be found in the ESI† in section S4.3.
Conclusions
In summary, our presented chip-based microfluidic setup offers a robust platform for monitoring biocatalytic transformations in packed-bed microreactors, featuring an automated injection workflow with almost real-time online LC/MS detection. Furthermore, we integrated a modular chipHPLC-based unit, which serves as a low-consumption alternative to the conventional C18-column used initially and significantly accelerates the separation process, while reducing solvent consumption by over 80%.
Our investigation focused on immobilising a vanadium-dependent haloperoxidase onto silica particles and packing them into microfluidic fused-silica chips. The performance of our newly established system was evaluated by employing a biocatalytic bromination as a model reaction. The covalent immobilisation strategy showed good stability while maintaining high enzyme activity during long-term monitoring experiments using only minute amounts of catalytic material (1–10 mg range).
The setup allows for the sequential monitoring of multiple interconnected microreactors by external valve control through reactor position switching. We showcased this capability by testing various sample compositions of the model biotransformation across multiple continuous microreactors in a single automated run, including blank measurements bypassing the microreactor.
The versatility of this approach allows for easy adaption to a parallel screening of multiple reactions or different immobilised catalytic materials in separate microreactors, making it suitable for various applications, including data feedback of optimised enzymes by directed evolution. Due to its deficient substrate consumption, the system is also well suited for studying APIs and derivatisations. By combining microfluidic approaches with extended setup automation and Python-based data evaluation, such data-rich systems could pave the way for more accessible connections to upcoming data science disciplines for more sustainable and efficient chemical processes.
Author contributions
Conceptualization: H. W., S. S., R. W., S. L., T. G., D. B.; investigation: H. W., S. S., S. L., T. O., R. W.; methodology: H. W., S. S., S. L, M. P., R. W., C. W.; software: H. W.; writing (original draft): H. W., S. S., T. G., D. B.; review & editing: all authors; supervision, funding, project administration: T. G., D. B.
Conflicts of interest
There are no conflicts to declare.
Acknowledgements
We thank Dr. Daniel Splith (Prof. Grundmann group, Leipzig University) for assisting with the laser-scanning microscope measurements. In addition, we would like to thank Monika Hahn (Prof. Grundmann group, Leipzig University) for assisting with the wafer dicing. TG and SS thank the Emmy-Noether program of the German Research Foundation (DFG, GU 1134/3) for generous funding.
Notes and references
- R. A. Sheldon and D. Brady, Green Chemistry, Biocatalysis, and the Chemical Industry of the Future, ChemSusChem, 2022, 15, e202102628 CrossRef CAS PubMed.
- J. M. Woodley, Advances in biological conversion technologies: new opportunities for reaction engineering, React. Chem. Eng., 2020, 5, 632–640 RSC.
- J. Chapman, A. Ismail and C. Dinu, Industrial Applications of Enzymes: Recent Advances, Techniques, and Outlooks, Catalysts, 2018, 8, 238 CrossRef.
- S. Simić, E. Zukić, L. Schmermund, K. Faber, C. K. Winkler and W. Kroutil, Shortening Synthetic Routes to Small Molecule Active Pharmaceutical Ingredients Employing Biocatalytic Methods, Chem. Rev., 2022, 122, 1052–1126 CrossRef PubMed.
- R. A. Sheldon and D. Brady, Broadening the Scope of Biocatalysis in Sustainable Organic Synthesis, ChemSusChem, 2019, 12, 2859–2881 CrossRef CAS PubMed.
- R. A. Sheldon and J. M. Woodley, Role of Biocatalysis in Sustainable Chemistry, Chem. Rev., 2018, 118, 801–838 CrossRef CAS PubMed.
- J. Planas-Iglesias, S. M. Marques, G. P. Pinto, M. Musil, J. Stourac, J. Damborsky and D. Bednar, Computational design of enzymes for biotechnological applications, Biotechnol. Adv., 2021, 47, 107696 CrossRef CAS PubMed.
- W. Xiong, B. Liu, Y. Shen, K. Jing and T. R. Savage, Protein engineering design from directed evolution to de novo synthesis, Biochem. Eng. J., 2021, 174, 108096 CrossRef CAS.
- N. J. Turner and R. Kumar, Editorial overview: Biocatalysis and biotransformation: The golden age of biocatalysis, Curr. Opin. Chem. Biol., 2018, 43, A1–A3 CrossRef CAS PubMed.
- F. L. C. Almeida, A. S. Prata and M. B. S. Forte, Enzyme immobilization: what have we learned in the past five years?, Biofuels, Bioprod. Biorefin., 2022, 16, 587–608 CrossRef CAS.
- H.-J. Federsel, T. S. Moody and S. J. C. Taylor, Recent Trends in Enzyme Immobilization—Concepts for Expanding the Biocatalysis Toolbox, Molecules, 2021, 26, 2822 CrossRef CAS PubMed.
- J. M. Bolivar, J. M. Woodley and R. Fernandez-Lafuente, Is enzyme immobilization a mature discipline? Some critical considerations to capitalize on the benefits of immobilization, Chem. Soc. Rev., 2022, 51, 6251–6290 RSC.
- J. Zdarta, A. Meyer, T. Jesionowski and M. Pinelo, A General Overview of Support Materials for Enzyme Immobilization: Characteristics, Properties, Practical Utility, Catalysts, 2018, 8, 92 CrossRef.
- F. T. T. Cavalcante, A. L. G. Cavalcante, I. G. de Sousa, F. S. Neto and J. C. S. dos Santos, Current Status and Future Perspectives of Supports and Protocols for Enzyme Immobilization, Catalysts, 2021, 11, 1222 CrossRef CAS.
- R. A. Sheldon, A. Basso and D. Brady, New frontiers in enzyme immobilisation: robust biocatalysts for a circular bio-based economy, Chem. Soc. Rev., 2021, 50, 5850–5862 RSC.
- P. Žnidaršič-Plazl, The Promises and the Challenges of Biotransformations in Microflow, Biotechnol. J., 2019, 14, 1800580 CrossRef PubMed.
- A. I. Benítez-Mateos, M. L. Contente, D. Roura Padrosa and F. Paradisi, Flow biocatalysis 101: design, development and applications, React. Chem. Eng., 2021, 6, 599–611 RSC.
- P. De Santis, L.-E. Meyer and S. Kara, The rise of continuous flow biocatalysis – fundamentals, very recent developments and future perspectives, React. Chem. Eng., 2020, 5, 2155–2184 RSC.
- F. Paradisi, Flow Biocatalysis, Catalysts, 2020, 10, 645 CrossRef CAS.
- M. Santi, L. Sancineto, V. Nascimento, J. Braun Azeredo, E. V. M. Orozco, L. H. Andrade, H. Gröger and C. Santi, Flow Biocatalysis: A Challenging Alternative for the Synthesis of APIs and Natural Compounds, Int. J. Mol. Sci., 2021, 22, 990 CrossRef CAS PubMed.
- M. B. Plutschack, B. Pieber, K. Gilmore and P. H. Seeberger, The Hitchhiker's Guide to Flow Chemistry, Chem. Rev., 2017, 117, 11796–11893 CrossRef CAS PubMed.
- M. Guidi, P. H. Seeberger and K. Gilmore, How to approach flow chemistry, Chem. Soc. Rev., 2020, 49, 8910–8932 RSC.
- L. Capaldo, Z. Wen and T. Noël, A field guide to flow chemistry for synthetic organic chemists, Chem. Sci., 2023, 14, 4230–4247 RSC.
- L. Rogers and K. F. Jensen, Continuous manufacturing – the Green Chemistry promise?, Green Chem., 2019, 21, 3481–3498 RSC.
- L. Yang, Y. Sun and L. Zhang, Microreactor Technology: Identifying Focus Fields and Emerging Trends by Using CiteSpace II, ChemPlusChem, 2023, 88, e202200349 CrossRef CAS PubMed.
- A. Šalić and B. Zelić, Synergy of Microtechnology and Biotechnology: Microreactors as an Effective Tool for Biotransformation Processes, Food Technol. Biotechnol., 2018, 56, 464–479 Search PubMed.
- E. J. S. Brás, V. Chu, J. P. Conde and P. Fernandes, Recent developments in microreactor technology for biocatalysis applications, React. Chem. Eng., 2021, 6, 815–827 RSC.
- F. W. M. Ling, H. A. Abdulbari and S. Y. Chin, Heterogeneous Microfluidic Reactors: A Review and an Insight of Enzymatic Reactions, ChemBioEng Rev., 2022, 9, 265–285 CrossRef.
- E. Laurenti and A. dos Santos Vianna Jr, Enzymatic microreactors in biocatalysis: history, features, and future perspectives, Biocatalysis, 2016, 1, 148–165 Search PubMed.
- Y. Zhu, Q. Chen, L. Shao, Y. Jia and X. Zhang, Microfluidic immobilized enzyme reactors for continuous biocatalysis, React. Chem. Eng., 2020, 5, 9–32 RSC.
- B. Wouters, S. A. Currivan, N. Abdulhussain, T. Hankemeier and P. J. Schoenmakers, Immobilized-enzyme reactors integrated into analytical platforms: Recent advances and challenges, TrAC, Trends Anal. Chem., 2021, 144, 116419 CrossRef CAS.
- E. Calleri, C. Temporini, R. Colombo, S. Tengattini, F. Rinaldi, G. Brusotti, S. Furlanetto and G. Massolini, Analytical settings for in-flow biocatalytic reaction monitoring, TrAC, Trends Anal. Chem., 2021, 143, 116348 CrossRef CAS.
- T. Jurina, T. Sokač Cvetnić, A. Šalić, M. Benković, D. Valinger, J. Gajdoš Kljusurić, B. Zelić and A. Jurinjak Tušek, Application of Spectroscopy Techniques for Monitoring (Bio)Catalytic Processes in Continuously Operated Microreactor Systems, Catalysts, 2023, 13, 690 CrossRef CAS.
- T. Kampe, A. König, H. Schroeder, J. G. Hengstler and C. M. Niemeyer, Modular Microfluidic System for Emulation of Human Phase I/Phase II Metabolism, Anal. Chem., 2014, 86, 3068–3074 CrossRef CAS PubMed.
- Y. Liu and X. Jiang, Why microfluidics? Merits and trends in chemical synthesis, Lab Chip, 2017, 17, 3960–3978 RSC.
- J.-C. M. Monbaliu and J. Legros, Will the next generation of chemical plants be in miniaturized flow reactors?, Lab Chip, 2023, 23, 1349–1357 RSC.
- C. P. Breen, A. M. K. Nambiar, T. F. Jamison and K. F. Jensen, Ready, Set, Flow! Automated Continuous Synthesis and Optimization, Trends Chem., 2021, 3, 373–386 CrossRef.
- S. V. Ley, D. E. Fitzpatrick, R. J. Ingham and R. M. Myers, Organic Synthesis: March of the Machines, Angew. Chem., Int. Ed., 2015, 54, 3449–3464 CrossRef CAS PubMed.
- D. Perera, J. W. Tucker, S. Brahmbhatt, C. J. Helal, A. Chong, W. Farrell, P. Richardson and N. W. Sach, A platform for automated nanomole-scale reaction screening and micromole-scale synthesis in flow, Science, 2018, 359, 429–434 CrossRef CAS PubMed.
- S. Chatterjee, M. Guidi, P. H. Seeberger and K. Gilmore, Automated radial synthesis of organic molecules, Nature, 2020, 579, 379–384 CrossRef CAS PubMed.
- Y. Shi, P. L. Prieto, T. Zepel, S. Grunert and J. E. Hein, Automated Experimentation Powers Data Science in Chemistry, Acc. Chem. Res., 2021, 54, 546–555 CrossRef CAS PubMed.
- M. Mowbray, M. Vallerio, C. Perez-Galvan, D. Zhang, A. Del Rio Chanona and F. J. Navarro-Brull, Industrial data science – a review of machine learning applications for chemical and process industries, React. Chem. Eng., 2022, 7, 1471–1509 RSC.
- B. Mahjour, Y. Shen and T. Cernak, Ultrahigh-Throughput Experimentation for Information-Rich Chemical Synthesis, Acc. Chem. Res., 2021, 54, 2337–2346 CrossRef CAS PubMed.
- S. D. Dreher and S. W. Krska, Chemistry Informer Libraries: Conception, Early Experience, and Role in the Future of Cheminformatics, Acc. Chem. Res., 2021, 54, 1586–1596 CrossRef CAS PubMed.
- F. Strieth-Kalthoff, F. Sandfort, M. H. S. Segler and F. Glorius, Machine learning the ropes: principles, applications and directions in synthetic chemistry, Chem. Soc. Rev., 2020, 49, 6154–6168 RSC.
- J. M. Cole, How the Shape of Chemical Data Can Enable Data-Driven Materials Discovery, Trends Chem., 2021, 3, 111–119 CrossRef CAS.
- A. F. De Almeida, R. Moreira and T. Rodrigues, Synthetic organic chemistry driven by artificial intelligence, Nat. Rev. Chem., 2019, 3, 589–604 CrossRef.
- M. H. S. Segler, M. Preuss and M. P. Waller, Planning chemical syntheses with deep neural networks and symbolic AI, Nature, 2018, 555, 604–610 CrossRef CAS PubMed.
- W. Wang, Y. Liu, Z. Wang, G. Hao and B. Song, The way to AI-controlled synthesis: how far do we need to go?, Chem. Sci., 2022, 13, 12604–12615 RSC.
- D. McIntyre, A. Lashkaripour, P. Fordyce and D. Densmore, Machine learning for microfluidic design and control, Lab Chip, 2022, 22, 2925–2937 RSC.
- A. Isozaki, J. Harmon, Y. Zhou, S. Li, Y. Nakagawa, M. Hayashi, H. Mikami, C. Lei and K. Goda, AI on a chip, Lab Chip, 2020, 20, 3074–3090 RSC.
- F. Grisoni, Combining generative artificial intelligence and on-chip synthesis for de novo drug design, Sci. Adv., 2021, 7, eabg3338 CrossRef CAS PubMed.
- D. Heckmann, C. J. Lloyd, N. Mih, Y. Ha, D. C. Zielinski, Z. B. Haiman, A. A. Desouki, M. J. Lercher and B. O. Palsson, Machine learning applied to enzyme turnover numbers reveals protein structural correlates and improves metabolic models, Nat. Commun., 2018, 9, 5252 CrossRef CAS PubMed.
- N. M. Ralbovsky and J. P. Smith, Machine Learning and Chemical Imaging to Elucidate Enzyme Immobilization for Biocatalysis, Anal. Chem., 2021, 93, 11973–11981 CrossRef CAS PubMed.
- S. Mazurenko, Z. Prokop and J. Damborsky, Machine Learning in Enzyme Engineering, ACS Catal., 2020, 10, 1210–1223 CrossRef CAS.
- K. K. Yang, Z. Wu and F. H. Arnold, Machine-learning-guided directed evolution for protein engineering, Nat. Methods, 2019, 16, 687–694 CrossRef CAS PubMed.
- Z. Wu, S. B. J. Kan, R. D. Lewis, B. J. Wittmann and F. H. Arnold, Machine learning-assisted directed protein evolution with combinatorial libraries, Proc. Natl. Acad. Sci. U. S. A., 2019, 116, 8852–8858 CrossRef CAS PubMed.
- M. Meuwly, Machine Learning for Chemical Reactions, Chem. Rev., 2021, 121, 10218–10239 CrossRef CAS PubMed.
- B. J. Shields, J. Stevens, J. Li, M. Parasram, F. Damani, J. I. M. Alvarado, J. M. Janey, R. P. Adams and A. G. Doyle, Bayesian reaction optimization as a tool for chemical synthesis, Nature, 2021, 590, 89–96 CrossRef CAS PubMed.
- J. Guo, B. Ranković and P. Schwaller, Bayesian Optimization for Chemical Reactions, Chimia, 2023, 77, 31 CrossRef CAS PubMed.
- P. Sagmeister, J. D. Williams and C. O. Kappe, The Rocky Road to a Digital Lab, Chimia, 2023, 77, 300 CrossRef CAS PubMed.
- D. S. Mattes, N. Jung, L. K. Weber, S. Bräse and F. Breitling, Miniaturized and Automated Synthesis of Biomolecules—Overview and Perspectives, Adv. Mater., 2019, 31, 1806656 CrossRef PubMed.
- S. Battat, D. A. Weitz and G. M. Whitesides, An outlook on microfluidics: the promise and the challenge, Lab Chip, 2022, 22, 530–536 RSC.
- V. C. Romao, S. A. M. Martins, J. Germano, F. A. Cardoso, S. Cardoso and P. P. Freitas, Lab-on-Chip Devices: Gaining Ground Losing Size, ACS Nano, 2017, 11, 10659–10664 CrossRef CAS PubMed.
- X. Lai, M. Yang, H. Wu and D. Li, Modular Microfluidics: Current Status and Future Prospects, Micromachines, 2022, 13, 1363 CrossRef PubMed.
- A. Das, C. Weise, M. Polack, R. D. Urban, B. Krafft, S. Hasan, H. Westphal, R. Warias, S. Schmidt, T. Gulder and D. Belder, On-the-Fly Mass Spectrometry in Digital Microfluidics Enabled by a Microspray Hole: Toward Multidimensional Reaction Monitoring in Automated Synthesis Platforms, J. Am. Chem. Soc., 2022, 144, 10353–10360 CrossRef CAS PubMed.
- R. Warias, A. Zaghi, J. J. Heiland, S. K. Piendl, K. Gilmore, P. H. Seeberger, A. Massi and D. Belder, An Integrated Lab-on-a-chip Approach to Study Heterogeneous Enantioselective Catalysts at the Microscale, ChemCatChem, 2018, 10, 5382–5385 CrossRef CAS.
- H. Westphal, R. Warias, H. Becker, M. Spanka, D. Ragno, R. Gläser, C. Schneider, A. Massi and D. Belder, Unveiling Organocatalysts Action – Investigating Immobilized Catalysts at Steady-State Operation via Lab-on-a-Chip Technology, ChemCatChem, 2021, 13, 5089–5096 CrossRef CAS.
- H. Westphal, R. Warias, C. Weise, D. Ragno, H. Becker, M. Spanka, A. Massi, R. Gläser, C. Schneider and D. Belder, An integrated resource-efficient microfluidic device for parallelised studies of immobilised chiral catalysts in continuous flow via miniaturized LC/MS-analysis, React. Chem. Eng., 2022, 7, 1936–1944 RSC.
- J. W. P. M. Schijndel, P. Barnett, J. Roelse, E. G. M. Vollenbroek and R. Wever, The Stability and Steady-State Kinetics of Vanadium Chloroperoxidase from the Fungus Curvularia Inaequalis, Eur. J. Biochem., 1994, 225, 151–157 CrossRef PubMed.
- R. Gupta, G. Hou, R. Renirie, R. Wever and T. Polenova, 51V NMR Crystallography of Vanadium Chloroperoxidase and Its Directed Evolution P395D/L241V/T343A Mutant: Protonation Environments of the Active Site, J. Am. Chem. Soc., 2015, 137, 5618–5628 CrossRef CAS PubMed.
- E. F. Gérard, T. Mokkawes, L. O. Johannissen, J. Warwicker, R. R. Spiess, C. F. Blanford, S. Hay, D. J. Heyes and S. P. de Visser, How Is Substrate Halogenation Triggered by the Vanadium Haloperoxidase from Curvularia inaequalis?, ACS Catal., 2023, 13, 8247–8261 CrossRef PubMed.
- V. Agarwal, Z. D. Miles, J. M. Winter, A. S. Eustáquio, A. A. El Gamal and B. S. Moore, Enzymatic Halogenation and Dehalogenation Reactions: Pervasive and Mechanistically Diverse, Chem. Rev., 2017, 117, 5619–5674 CrossRef CAS PubMed.
- G. T. Höfler, A. But, S. H. H. Younes, R. Wever, C. E. Paul, I. W. C. E. Arends and F. Hollmann, Chemoenzymatic Halocyclization of 4-Pentenoic Acid at Preparative Scale, ACS Sustainable Chem. Eng., 2020, 8, 2602–2607 CrossRef PubMed.
- E. Fernández-Fueyo, M. van Wingerden, R. Renirie, R. Wever, Y. Ni, D. Holtmann and F. Hollmann, Chemoenzymatic Halogenation of Phenols by using the Haloperoxidase from Curvularia inaequalis, ChemCatChem, 2015, 7, 4035–4038 CrossRef.
- C. J. Seel, A. Králík, M. Hacker, A. Frank, B. König and T. Gulder, Atom-Economic Electron Donors for Photobiocatalytic Halogenations, ChemCatChem, 2018, 10, 3960–3963 CrossRef CAS.
- H. Li, S. H. H. Younes, S. Chen, P. Duan, C. Cui, R. Wever, W. Zhang and F. Hollmann, Chemoenzymatic Hunsdiecker-Type Decarboxylative Bromination of Cinnamic Acids, ACS Catal., 2022, 12, 4554–4559 CrossRef CAS PubMed.
- C. E. Wells, L. P. T. Ramos, L. J. Harstad, L. Z. Hessefort, H. J. Lee, M. Sharma and K. F. Biegasiewicz, Decarboxylative Bromooxidation of Indoles by a Vanadium Haloperoxidase, ACS Catal., 2023, 13, 4622–4628 CrossRef CAS.
- L. J. Harstad, C. E. Wells, H. J. Lee, L. P. T. Ramos, M. Sharma, C. A. Pascoe and K. F. Biegasiewicz, Decarboxylative halogenation of indoles by vanadium haloperoxidases, Chem. Commun., 2023, 59, 14289–14292 RSC.
- E. Fernández-Fueyo, S. H. H. Younes, S. van Rootselaar, R. W. M. Aben, R. Renirie, R. Wever, D. Holtmann, F. P. J. T. Rutjes and F. Hollmann, A Biocatalytic Aza-Achmatowicz Reaction, ACS Catal., 2016, 6, 5904–5907 CrossRef.
- C. G. England, H. Luo and W. Cai, HaloTag Technology: A Versatile Platform for Biomedical Applications, Bioconjugate Chem., 2015, 26, 975–986 CrossRef CAS PubMed.
- J. Döbber and M. Pohl, HaloTagTM: Evaluation of a covalent one-step immobilization for biocatalysis, J. Biotechnol., 2017, 241, 170–174 CrossRef PubMed.
- M. P. Thompson, I. Peñafiel, S. C. Cosgrove and N. J. Turner, Biocatalysis Using Immobilized Enzymes in Continuous Flow for the Synthesis of Fine Chemicals, Org. Process Res. Dev., 2019, 23, 9–18 CrossRef CAS.
- G. V. Los, L. P. Encell, M. G. McDougall, D. D. Hartzell, N. Karassina, C. Zimprich, M. G. Wood, R. Learish, R. F. Ohana, M. Urh, D. Simpson, J. Mendez, K. Zimmerman, P. Otto, G. Vidugiris, J. Zhu, A. Darzins, D. H. Klaubert, R. F. Bulleit and K. V. Wood, HaloTag: A Novel Protein Labeling Technology for Cell Imaging and Protein Analysis, ACS Chem. Biol., 2008, 3, 373–382 CrossRef CAS PubMed.
- C. Lotter, J. J. Heiland, V. Stein, M. Klimkait, M. Queisser and D. Belder, Evaluation of Pressure Stable Chip-to-Tube Fittings Enabling High-Speed Chip-HPLC with Mass Spectrometric Detection, Anal. Chem., 2016, 88, 7481–7486 CrossRef CAS PubMed.
- S. K. Piendl, C.-R. Raddatz, N. T. Hartner, C. Thoben, R. Warias, S. Zimmermann and D. Belder, 2D in Seconds: Coupling of Chip-HPLC with Ion Mobility Spectrometry, Anal. Chem., 2019, 91, 7613–7620 CrossRef CAS PubMed.
- J. J. Heiland, D. Geissler, S. K. Piendl, R. Warias and D. Belder, Supercritical-Fluid Chromatography On-Chip with Two-Photon-Excited-Fluorescence Detection for High-Speed Chiral Separations, Anal. Chem., 2019, 91, 6134–6140 CrossRef CAS PubMed.
- K. Svensson, C. Weise, H. Westphal, S. Södergren, D. Belder and K. Hjort, Coupling microchip pressure regulators with chipHPLC as a step toward fully portable analysis system, Sens. Actuators, B, 2023, 385, 133732 CrossRef CAS.
- S. K. Piendl, T. Schönfelder, M. Polack, L. Weigelt, T. van der Zwaag, T. Teutenberg, E. Beckert and D. Belder, Integration of segmented microflow chemistry and online HPLC/MS analysis on a microfluidic chip system enabling enantioselective analyses at the nanoliter scale, Lab Chip, 2021, 21, 2614–2624 RSC.
- C. Lotter, J. J. Heiland, V. Stein, M. Klimkait, M. Queisser and D. Belder, Evaluation of Pressure Stable Chip-to-Tube Fittings Enabling High-Speed Chip-HPLC with Mass Spectrometric Detection, Anal. Chem., 2016, 88, 7481–7486 CrossRef CAS PubMed.
- S. Thurmann, L. Mauritz, C. Heck and D. Belder, High-performance liquid chromatography on glass chips using precisely defined porous polymer monoliths as particle retaining elements, J. Chromatogr. A, 2014, 1370, 33–39 CrossRef CAS PubMed.
- J. J. Heiland, C. Lotter, V. Stein, L. Mauritz and D. Belder, Temperature Gradient Elution and Superheated Eluents in Chip-HPLC, Anal. Chem., 2017, 89, 3266–3271 CrossRef CAS PubMed.
- Y. Sun, F. Kunc, V. Balhara, B. Coleman, O. Kodra, M. Raza, M. Chen, A. Brinkmann, G. P. Lopinski and L. J. Johnston, Quantification of amine functional groups on silica nanoparticles: a multi-method approach, Nanoscale Adv., 2019, 1, 1598–1607 RSC.
- D. R. Morris and L. P. Hager, Chloroperoxidase, J. Biol. Chem., 1966, 241, 1763–1768 CrossRef CAS PubMed.
|
This journal is © The Royal Society of Chemistry 2024 |
Click here to see how this site uses Cookies. View our privacy policy here.