DOI:
10.1039/D4RA04302B
(Paper)
RSC Adv., 2024,
14, 21328-21341
Cefiderocol susceptibility endows hope in treating carbapenem-resistant Pseudomonas aeruginosa: insights from in vitro and in silico evidence†
Received
12th June 2024
, Accepted 28th June 2024
First published on 8th July 2024
Abstract
‘High-risk’ hypermutable clones of Pseudomonas aeruginosa disseminating extensive drug-resistance (XDR) have raised global health concerns with escalating mortality rates in immunocompromised patients. Mutations in conventional drug-targets under antibiotic stress necessitate structural understanding to formulate sustainable therapeutics. In the present study, the major β-lactam antibiotic target, penicillin-binding protein-3 (PBP3) with mutations F533L and T91A, were identified in carbapenemase-positive P. aeruginosa isolates (n = 6) using whole genome sequencing. Antibiotic susceptibility tests showed susceptibility to cefiderocol (MIC ≤ 4 μg ml−1) despite pan-β-lactam resistance in the isolates. Both the mutations reduced local intra-chain interactions in PBP3 that marginally increased the local flexibility (∼1%) in the structures to affect antibiotic-interactions. Molecular dynamics simulations confirmed the overall stability of the PBP3 mutants through root-mean square deviations, radius of gyration, solvent-accessibility and density curves, which favored their selection. Docking studies unveiled that the mutations in PBP3 elicited unfavorable stereochemical clashes with the conventional antibiotics thereby increasing their inhibition constants (IC) up to ∼50 fold. It was deciphered that cefiderocol retained its susceptibility despite mutations in PBP3, due to its higher average binding affinity (ΔG: −8.2 ± 0.4 kcal mol−1) towards multiple PBP-targets and lower average binding affinity (ΔG: −6.7 ± 0.7 kcal mol−1) to β-lactamases than the other β-lactam antibiotics. The molecular dynamics simulations and molecular mechanics Poisson Boltzmann surface area calculations further indicated energetically favorable binding for cefiderocol with PBP3 proteins. The study gave structural insight into emerging non-polar amino acid substitutions in PBP3 causing XDR and recommends prioritizing available antibiotics based on multi-target affinities to overcome challenges imposed by target-protein mutations.
Introduction
Pseudomonas aeruginosa (P. aeruginosa) is a major health threat worldwide constituting almost 20% of all the nosocomial pathogens and causing severe respiratory ailments with high mortality rates, particularly in neutropenic patients. Invasive P. aeruginosa was observed to possess remarkably high levels (15–30% worldwide) of multi-drug resistance (MDR) and extensive drug-resistance (XDR).1–3 The rapid dissemination of ‘high-risk’ clones comprising transferrable XDR elements has raised major concerns around the globe due to its outstanding potential to acquire rapid chromosomal mutations to develop antimicrobial resistance against almost all kinds of antipseudomonal agents.4,5
Aberrant chromosomal mutations as a result of DNA-repair avoidance are being continuously reported in MDR/XDR P. aeruginosa especially isolated from patients with chronic infection. Hypermutable ‘high-risk’ clones showing an accumulation of multiple chromosomal mutations (in conventional targets like penicillin-binding proteins) and expression of various antibiotic evasion mechanisms (expression of efflux pumps, β-lactamase production) have given rise to notable epidemics in the hospital setting and clonal outbreaks of pan-antibiotic-resistant (PDR) strains.5–7 PDR P. aeruginosa has portrayed intrinsic resistome that are independent of antibiotic exposure and/or horizontal gene transfer. Previous studies on the epidemic high-risk clones highlighted the evolutionary dynamics of P. aeruginosa resistome.8–10 However, comprehensive reports on the growing mutational landscape in the pathogen comprising complex regulatory pathways are limited.11,12 Whole-genome sequencing (WGS) and analyses can instrumentally provide leads in the identification, segregation and prioritization of significant acquired mutations besides classical mutations conferring resistance to β-lactams, aminoglycosides, fluoroquinolones, polymixins and carbapenems. The development of new generation cephalosporins however, has shown promising results in addressing the challenges posed by antimicrobial resistance.13 Integrated structural informatics approaches can efficiently decipher underlying molecular signatures contributing to the selection of emerging stable MDR variants.5,14–16 The present study was designed to elucidate the underlying impact of emerging P. aeruginosa mutants from MDR isolates that can potentially cause clonal outbreaks (unpublished data from Christian Medical College & Hospital, Vellore). In vitro antibiotic susceptibilities were amalgamated with in silico structural bioinformatics to prioritize available therapeutics against the pathotype. The information can provide leads for clinical therapeutic research, infection control and antimicrobial stewardship.
Methods
Isolates and antibiotic susceptibility
Clinical strains of P. aeruginosa were identified from routine blood and sputum cultures from 2020 to 2022 at Christian Medical College, Vellore. All the isolates were characterized up to the species level using routine biochemical and automated identification system VITEK®-MS (BioMérieux). The six isolates considered in the present study were reported to show pan beta-lactam resistance. Antimicrobial susceptibility testing (AST) was performed for all the isolates against different antibiotics by the Kirby–Bauer disc-diffusion method and interpreted as per Clinical Laboratory Standards Institute (CLSI) guidelines. The minimum inhibitory concentration (MIC) for antibiotics viz., ceftazidime, piperacillin–tazobactam, meropenem, imipenem, ceftazidime–avibactam, ceftolozane–tazobactam, cefepime–taniborbactam, meropenem–vaborbactam, cefiderocol and imipenem–relebactam were determined for all the studied isolates using broth micro-dilution and interpreted accordingly.
Whole genome sequencing and mutations
The isolates' genomic DNA was extracted using a QIAamp DNA mini kit (Qiagen, Hilden, Germany). Ion Torrent (PGM) sequencer with 400 bp read chemistry (Life Technologies, CA, USA) was used to sequence the whole genome in accordance with the manufacturer's recommendations. Assembler SPAdes v5.0.0.0, which is integrated into Torrent Suite Server version 5.0.3 (Life Technologies), was used to assemble the genomes. The bacterial bioinformatics database and analytic tool PATRIC, as well as the NCBI Prokaryotic Genome Annotation Pipeline (PGAP) (https://www.ncbi.nlm.nih.gov/genomes/static/Pipeline.html), were used to annotate the genomic-sequence. The MLST 2.0 tool (https://cge.cbs.dtu.dk//services/MLST/) was used for sequence-typing. P. aeruginosa ATCC 27853 (NZ_CP015117) was used as a reference to identify mutations in PBPs and other relevant proteins from the studied isolates. The proteins sequences of interest viz., PBP3, PBP1A, PBP1B, PBP2, OXA-10, OXA-486 and KPC-2 were extracted from the whole genome using python script in_silico_PCR.py (https://github.com/simonrharris/in_silico_pcr).
Structure retrieval of target proteins and drugs of interest
The extracted protein sequences were used as reference proteins for BLASTp search in the Protein Data Bank (PDB) and non-redundant databases. The protein structures possessing >99% sequence identity with our WGS data were chosen as reference and retrieved in PDB formats. The 3-D crystal structures of PBP3 [3BPR], PBP2 [7KIS], PBP1B [4OON] were retrieved from PDB whereas PBP1B [AF-Q9X6W0-F1], OXA-10 [AF-P14489-F1], OXA-486 [AF-Q6PL76-F1] and KPC-2 [AF-G8HAR0-F1] were retrieved from AlphaFold 2.0.17,18 The target proteins were screened with Swiss-PDB viewer (SPDBV)19 to induce the specific mutations (reported from WGS data) for docking studies. All the structures were refined and energy-minimized (using GROMOS96 43B1 force-field in vacuo) as per our previous studies.15,20–23 The functional domains of the proteins were determined from the INTERPRO server to interpret protein-ligand interactions.24
The conventional antibiotic molecules against PBP3 viz. imipenem (CID: 104838), meropenem (CID: 441130), cefepime (CID: 5479537), ceftolozane (CID: 53234134), ceftazidime (CID: 5481173) and cefiderocol (CID: 77843966) were obtained from the PubChem database in SDF formats.25 3-D formatting of the antibiotics was achieved using the OpenBabel tool.26 The antibiotics/drugs have been synonymously referred to as 'ligands' in the present study.
Backbone flexibility and vibrational entropy
DynaMine server was employed to determine the residue-level backbone dynamics of the mutant proteins of interest through backbone N–H S2 order parameter values.27 According to the molecular reference frame, the restricted orientations of atomic bond vectors were represented by the S2 values that depict experimental NMR chemical shifts. S2 values > 0.8 portray considerably rigid conformations.27 Dynamut was used to analyze mutation-based structural changes in a protein.28 Normal mode analysis (NMA) of the parent and mutant protein performed with Dynamut approximated their dynamics and accessible conformations in harmonic motions. Superimposition of normal modes like eigenvectors and eigenvalues validated the vibrational entropies of the proteins. Vibrational Entropy/VE (ΔΔS in kcal mol−1 K−1) explained the occupation probabilities of protein residues in an energy landscape based on average configurational entropies. A considerable decrease in VE increases the rigidity of the proteins.29 The resultant stability assessment of the proteins considered their chemical conformations, pharmacophore vectors, biological/physiological functions and evolutionary impacts resulting from mutations.30
Folding free-energy and residue conservation analysis
SWOTein interface was employed to identify structural integrities due to mutations based on statistical functions pertaining to folding patterns, local interaction cloud, hydrophobic forces and tertiary interactions. The interface accurately assigned free energy values (ΔG) in terms of conformational descriptors like solvent accessibility, torsion-angle and distance (C-α).31
Consurf determined the evolutionary map of amino acids in sequence levels that govern the overall macromolecular integrity and associated functions that determined the selection of the mutants. Based on the phylogenetic correlation between the homologues proteins and the specific sequence dynamics, advanced probabilistic evolutionary models are generated and confidence scores [9 → conserved; 1 → variable] were assigned for interpretation.32
Coarse-grained simulation
CABSflex simulation engine determined the residue-level Root Mean Square Fluctuation (RMSF) trajectories of the proteins of interest by applying default restraints between pair atoms for simulated dynamic orientations within defined spaces.33 The consensus protein fluctuations in an aqueous environment were derived by all-atom molecular dynamics simulation (10 nanosecond timescale with suitable force fields). As default mode, restrain gap = 3.0 was chosen (minimum distance between previous and next amino acid in the chain to be restrained) along with minimum and maximum conformational distances of 3.8 Å and 8.0 Å, respectively.33
Molecular dynamics simulation
Critical understanding of the proteins' (wild and mutant) stability was depicted in a simulated aqueous environment for a favorable timescale. GROMACS 2020.1 package with OPLS-all-atom force-field was used to simulate the protein placed centrally in a cubic box with a uniform edge-distance (1.5 nm) following solvation with a simple point-charge water model and neutralizing the system by adding requisite counter ions (Na+ or Cl−).34 The steepest descent algorithm was adopted for energy minimization with 50
000 steps and 1000 kJ mol−1 nm−1 convergence tolerance. Equilibrations with standard NVT (constant number of particles, volume and temperature) and NPT (constant number of particles, pressure and temperature) ensembles for 150 ps were operated. Particle-mesh Ewald electrostatics summation was used for treating long-range electrostatic interactions with an order of 4.0 and Fourier spacing of 0.16 nm followed by applying Parrinello–Rahman extended coupling ensemble for pressure scaling. The relative overall stability of the wild and mutant proteins was interpreted from the Root Mean Square Deviation (RMSD), radius of gyration (Rg), solvent accessible surface area (SASA) and electron density curves.
Molecular docking
The binding affinities of the simulated proteins (wild and mutants separately) with target antibiotics cefepime, cefiderocol, ceftazidime, ceftolozane, imipenem and meropenem were assessed through molecular docking analysis using AutoDock4.0 and the embedded tools.35 Before the docking analysis, the protein structures were cured by removing crystallographic water molecules and unwanted hetero-atoms. Polar hydrogens were added and non-polar hydrogens were merged. Requisite Kollman charges were finally added to the protein to stabilise its structure in ideal spatial geometry. The torsions were fixed for the ligands and Gasteiger charges were incorporated. The van der Waals well depth of 0.100 kcal mol−1 was assigned for the protein, before saving in PDBQT formats. The grid box of 216000 Å3 was centered at crucial active sites residue (Ser294) to encompass the entire active site domain.36 The binding pockets (in the active site) were further validated from CASTp37 server. Lamarckian genetic algorithm was applied to generate target protein–drug complexes in compatible conformations. The binding energies of the complexes were obtained in 10 different poses. The top-ranked complexes based on the lowest binding energies or ΔG (highest binding affinities) were visualized using Discovery Studio.38 Drug-binding properties were validated from the crucial intermolecular interactions of the complexes.39,40
Protein complex stability assessment using MDS
The stability of the protein complexes with target antibiotics was then analyzed by molecular dynamics simulation for a timescale of 100 ns using the GROMACS suite. The simulation was initiated by generating protein and ligand topology files using CHARMM forcefield 2022 and CGenFF server respectively. The simulation was performed with the TIP3P water model and the system was centered in a dodecahedron box with an edge distance of 1.2 nm. The system was neutralized by adding ions and was subjected to energy minimization using steepest-descent algorithms and convergence-tolerance force. The position restraint topology was generated, which restrained the ligand and the corresponding index file was generated. The system was then subjected to two cycles of equilibrium, namely constant volume (NVT) for 100 ps to attain 300 K temperature, followed by constant pressure (NPT) for 100 ps to attain 1 bar pressure. The system finally proceeded to MD production for 100 ns to obtain the simulated trajectories.
MM/PBSA calculations
The Molecular Mechanics/Poisson–Boltzmann Surface Area (MM/PBSA) was computed to determine the binding free energy for docked complexes. For the present study, we have employed gmxMMPBSA tool that computationally calculates binding free energy with various solvation models. It is based on AMBER's MMPBSA.py, which functions in three different steps, namely preparation which includes the generation of topologies and trajectories, followed by calculation, where the binding free energies are computed, which were analyzed in the final step using the graphical interface gmx_MMPBSA_ana.41,42
Results
Antibiotic susceptibility
AST revealed that all six isolates are pan-drug resistant (PDR), being resistant to tested β-lactam antibiotics and β-lactam–β-lactamase inhibitor combinations as represented in Table 1.
Table 1 Antibiotic susceptibility of KPC-positive P. aeruginosa isolates expressing PBP3 mutants F533L and T91Aa
Accession IDs |
MLST |
β-Lactamases expressed |
PBP3 mutations |
MIC (μg ml−1) |
CAZ |
FDC |
MER |
IMP |
PIP + TAZ |
CAZ + AVI |
TOL + TAZ |
FEP + TAN |
MEM/VAB |
IMP/REL |
CAZ: ceftazidime; FDC: cefiderocol; MER: meropenem; IMP: imipenem; PIP: piperacillin; TOL: ceftolozane; TAZ: tazobactam; AVI: avibactam; FEP: cefepime; TAN: taniborbactam; VAB: vaborbactam; REL: relebactam. |
JAOVDF000000000 |
1801 |
KPC-2, OXA-50/486, OXA-10 |
F533L, T91A |
>128 |
4 |
>128 |
>128 |
>128 |
16 |
64 |
32 |
>128 |
8 |
JAOVEE000000000 |
>128 |
2 |
>128 |
>128 |
>128 |
16 |
>128 |
>128 |
>128 |
64 |
JAOVEH000000000 |
>128 |
2 |
>128 |
>128 |
>128 |
8 |
>128 |
>128 |
>128 |
64 |
JAOVEI0000000 00 |
>128 |
2 |
>128 |
>128 |
>128 |
32 |
>128 |
>128 |
>128 |
128 |
JAOVEJ0000000 00 |
>128 |
1 |
>128 |
>128 |
>128 |
8 |
>128 |
>128 |
>128 |
64 |
JAOVEL000000000 |
>128 |
1 |
>128 |
>128 |
>128 |
16 |
>128 |
>128 |
>128 |
128 |
The isolates showed resistant profile (as per CLSI standards) against ceftazidime, imipenem, meropenem, piperacillin–tazobactam, ceftazidime–avibactam, ceftolozane–tazobactam, cefepime–taniborbactam, meropenem–vaborbactam and imipenem–relebactam. However, all were susceptible to cefiderocol (MIC: ≤ 4 μg ml−1).
Genome characters and mutations
All the six isolates comprised of mutations in PBP3, viz., F533L and T91A. Furthermore, they were found to express β-lactamases blaOXA-10, blaOXA-486 and blaKPC-2 (Table 1). No other target mutations were observed.
Structural stability analyses of the PBP3 mutants
The relative backbone dynamics of the parent and PBP3 mutant indicated no major overall change in the average S2-probability score (∼0.810) or rigidity. However, when the specific mutations were considered, F533L reduced the S2-probability score from 0.76 to 0.74, whereas, T91A reduced it from 0.83 to 0.82 [Fig. 1(a) and (b)]. The overall rigidity of the concerned domains was also reduced minimally by 1.0% (ESI File S1†). On the other hand, the average RMSFs of PBP3–F533L (0.93) and PBP3–T91A (0.9) were slightly reduced than the parent PBP3 (0.97) [Fig. 1(c)]. The vibrational entropy of the mutants portrayed a sharp decrease (10–20 times) in the corresponding neighboring residues due to F533L and T91A. The average VE in PBP3_F533L was −0.035 kcal mol−1 whereas that of PBP3_T91A was −0.026 kcal mol−1 [Fig. 1(d)]. Fig. 2(a) depicted the loss of intra-chain H-bond (with T487) and hydrophobic interactions (with V333) due to F533L; and the loss of polar (with K87) hydrophobic interactions (with Q88) due to T91A, which was further correlated with the structural stability patterns. The stability patterns of the overall proteins revealed minimal deviation (by ∼0.1 nm) with respect to their C-α backbone due to F533L, however, the average root mean square deviation (RMSD) of the proteins equilibrated between a narrow range of 0.1 to 0.2 nm [Fig. 2(b)]. Similarly, the radius of gyration (Rg) was slightly enhanced (by ∼0.1 nm) due to F533L, however, the overall trajectories of the parent and mutants were stable between 2.9–3.0 nm [Fig. 2(c)]. The solvent accessible area fluctuated between 235–245 nm2 due to the mutations [Fig. 2(d)]. The mutations altered the local electron density nominally (not shown), however, the overall density remained constant at around 1010 kg m−3 [Fig. 2(e)].
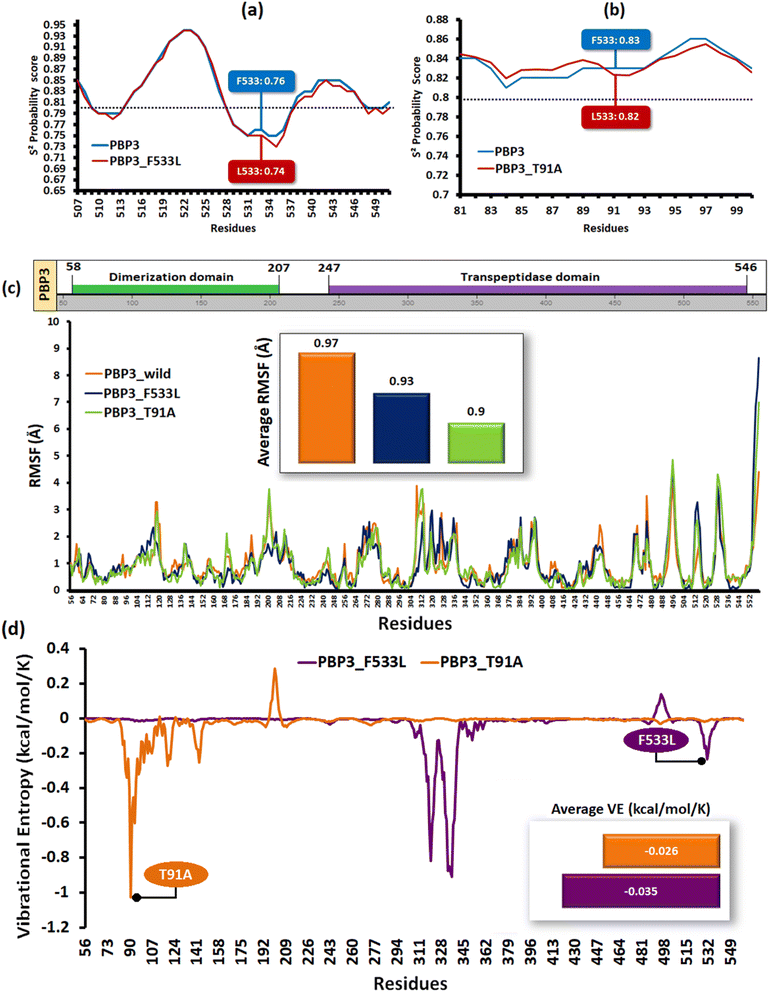 |
| Fig. 1 The structural flexibility of the mutant PBPs (a) relative backbone flexibility in PBP3_F533L (b) relative backbone flexibility in PBP3_T91A (c) the relative RMSF of the parent and mutant PBPs (d) the vibrational entropies of the mutant PBP. | |
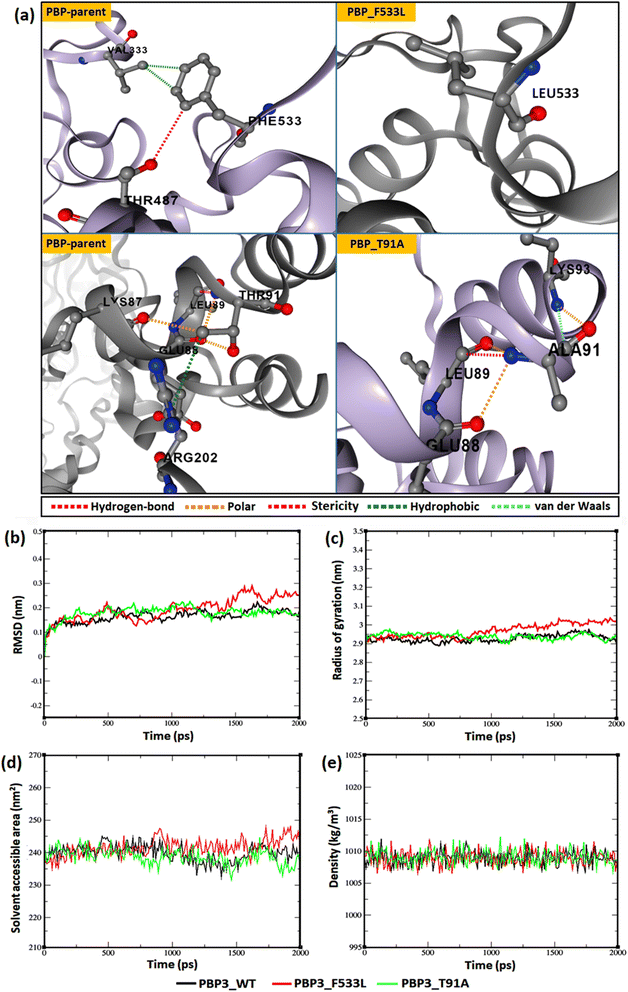 |
| Fig. 2 Relative dynamics of the parent and mutant PBPs (a) loss of intra-chain interactions due to mutations (b) RMSDs (c) radius of gyration (d) solvent accessibility area (e) electron densities. | |
Folding free energy and conservation analysis of the PBP3 mutants
Folding free energy analysis depicted the overall loss or gain in stability due to folding energy alterations. The overall free energies (ΔG in kcal mol−1) of solvent accessibility and torsion were uncompromised with deviations <1.0% [ESI File S2(a) and (b)†]. The conservation analysis revealed that T91A marginally enhanced the conservation confidence of a variable stretch (by 1 unit) of PBP3, whereas F533L located in a highly conserved stretch (scores > 7.0) did not enhance further. However, both the mutant PBP3 possessed an overall average conservation confidence score higher (∼1%) than the parent PBP3 (ESI File S3†).
Relative binding affinity profiles of the antibiotics against P. aeruginosa proteins
The relative binding affinities of the antibiotics indicated that cefiderocol (ΔG: −8.2 ± 0.4 kcal mol−1) possessed the highest average binding affinity against all the PBP-targets in P. aeruginosa viz., PBP3, PBP1A, PBP1B and PBP2 as compared to ceftazidime (ΔG: −7.45 ± 0.5 kcal mol−1), ceftolozane (ΔG: −7.43 ± 0.5 kcal mol−1), cefepime (ΔG: −7.4 ± 0.4 kcal mol−1), imipenem (ΔG: −6.03 ± 0.5 kcal mol−1) and meropenem (ΔG: −6.63 ± 0.7 kcal mol−1) (Table 2).
Table 2 The relative binding affinities of the antibiotics against target PBPs and β-lactamases
Compounds |
Binding energy (kcal mol−1) |
β-Lactamases |
Target PBPs |
Oxa-488 |
Oxa-10 |
KPC-2 |
PBP-3 |
PBP1A |
PBP1B |
PBP2 |
Cefepime |
−6.4 |
−6.7 |
−8.7 |
−6.9 |
−6.5 |
−8.6 |
−7.6 |
Cefiderocol |
−6.8 |
−6 |
−7.5 |
−8.3 |
−7.8 |
−8.6 |
−8.4 |
Ceftazidime |
−7.4 |
−7.9 |
−7.7 |
−7.3 |
−7 |
−7.5 |
−8.0 |
Ceftolozane |
−7.2 |
−7.2 |
−7.7 |
−7.3 |
−6.7 |
−7.8 |
−7.9 |
Imipenem |
−5.7 |
−5.4 |
−8 |
−6.3 |
−5.4 |
−6.5 |
−5.9 |
Meropenem |
−7.0 |
−9.4 |
−7.5 |
−7.7 |
−6.3 |
−5.5 |
−7.0 |
Furthermore, cefiderocol showed lower average affinity (ΔG: −6.7 ± 0.8 kcal mol−1) to beta-lactamases (KPC-2, OXA-10 and OXA-486) expressed by the studied P. aeruginosa strains as compared to ceftazidime (ΔG: −7.67 ± 0.5 kcal mol−1), ceftolozane (ΔG: −7.37 ± 0.5 kcal mol−1), cefepime (ΔG: −7.4 ± 0.4 kcal mol−1) and meropenem (ΔG: −7.97 ± 0.7 kcal mol−1). Cefepime and imipenem possessed the highest affinity to KPC-2 (ΔG: −8.7 and −8.0 kcal mol−1 respectively) (Table 2).
Binding profiles of the antibiotics affected by PBP3 mutations
The relative inhibition constants (ICs) of the antibiotics with wild (WT) and PBP3 mutants are depicted in Table 3. The mutations F533L and T91A have increased the inhibition constants for all the antibiotics. The maximally affected interaction by F533L was observed for ceftolozane (IC increased from 5.40 μM to 266.5 μM) and imipenem (IC increased from 15.1 μM to 108.7 μM). The ICs for other antibiotics too were increased by ∼2–15 fold due to F533L. Similarly, T91A majorly affected the binding of ceftolozane (IC increased from 5.40 μM to 25.36 μM) and imipenem (IC increased from 15.1 μM to 35.1 μM). The ICs for other antibiotics were elevated by ∼2–10 fold by T91A. The ICs of cefiderocol were consistently low for PBP3_WT as well as PBP3 mutants as compared to other antibiotics.
Table 3 Relative binding energies (BE), inhibition constants and interactions with the antibiotics in wild and mutated PBP3
Antibiotics |
PBP3_WT |
PBP3_F533L |
PBP3_T91A |
IC (μM) |
No. of specific interactionsa |
IC (μM) |
No. of specific interactionsa |
IC (μM) |
No. of specific interactionsa |
Favorable |
Unfavorable |
Favorable |
Unfavorable |
Favorable |
Unfavorable |
Specific interactions comprise of H-bonds and non-canonical interactions. |
Cefepime |
6.69 |
22 |
No |
12.02 |
19 |
Yes |
5.23 |
21 |
No |
Cefiderocol |
0.33 |
32 |
No |
5.40 |
19 |
No |
3.34 |
27 |
Yes |
Ceftazidime |
5.41 |
27 |
No |
6.82 |
24 |
Yes |
12.0 |
25 |
Yes |
Ceftolozane |
5.40 |
28 |
No |
266.5 |
22 |
Yes |
25.36 |
22 |
Yes |
Imipenem |
15.1 |
17 |
No |
108.7 |
18 |
Yes |
35.10 |
14 |
No |
Meropenem |
1.52 |
18 |
No |
15.89 |
17 |
No |
5.91 |
17 |
No |
Intermolecular interactions between the antibiotics and PBP3-mutants
Interactome of individual antibiotics with the targets revealed differences in the interacting residues and loss of intermolecular interactions due to the mutations F533L and T91A (Table 3). Cefepime interacted with PBP3 through a total of 22 connections comprising H-bonds and non-canonical interactions including crucial active site residues like Ser294 [Fig. 3(a)]. For PBP3_F533L, although the interaction with active sites Ser294 and Lys297 was not lost, the introduction of an unfavorable interaction with Tyr409 increased the IC with cefepime [Fig. 3(b)]. PBP3_T91A although established 21 interactions with cefepime, but the interactions with crucial active site residues were lost [Fig. 3(c)]. For cefiderocol, there were 32 interactions combining H-bonds and non-canonical ones with PBP3_WT including active site Ser294 [Fig. 3(d)]. F533L and T91A reduced the number of interactions to 19 and 27 respectively, although interactions with crucial active site residues were retained [Fig. 3(e) and (f)]. Ceftazidime possessed the 27 interactions with PBP3_WT, which was reduced to 24 and 25 by F533L and T91A respectively, besides acquiring unfavorable interactions with Tyr409, Ser485 and Leu481 [Fig. 3(g)–(i)]. When ceftolozane was docked onto the target PBP3 active site, 28 interactions were established; however, due to F533L and T91A, non-canonical interactions were lost alongside the addition of unfavorable interactions with Lys297 and Arg276 [Fig. 4(a)–(c)]. For imipenem, 17 interactions were observed with PBP3_WT. Both T91A and F533L altered the non-canonical interactions-landscape besides introducing unfavorable interaction with active site lysine residues [Fig. 4(d)–(f)]. Finally, meropenem formed 18 interactions with the active site residues including Ser294 [Fig. 4(g)]. Both F533L and T91A resulted in the loss of non-canonical interactions and the H-bonds by compromising the interactions with crucial active site residues like Ser294 [Fig. 4(h) and (i)].
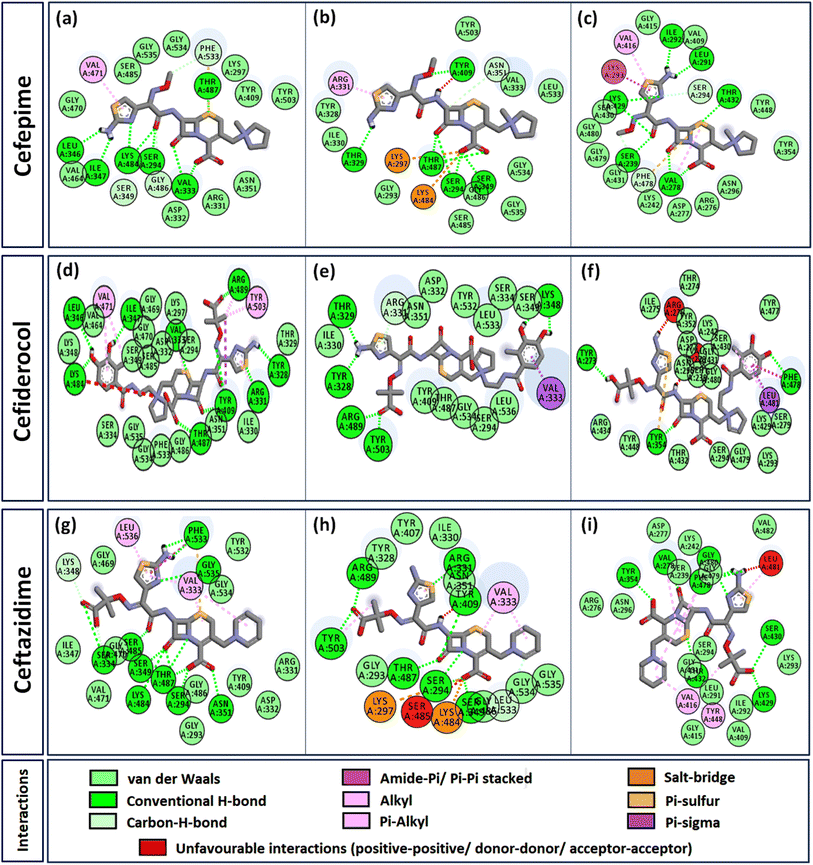 |
| Fig. 3 Interaction profiles of the parent and mutant PBPs with cefepime, cefiderocol and ceftazidime (a) PBP3 + cefepime (b) PBP3_F533L + cefepime (c) PBP3_T91A + cefepime (d) PBP3 + cefiderocol (e) PBP3_F533L + cefiderocol (f) PBP3_T91A + cefiderocol (g) PBP3 + ceftazidime (h) PBP3_F533L + ceftazidime (i) PBP3_T91A + ceftazidime. | |
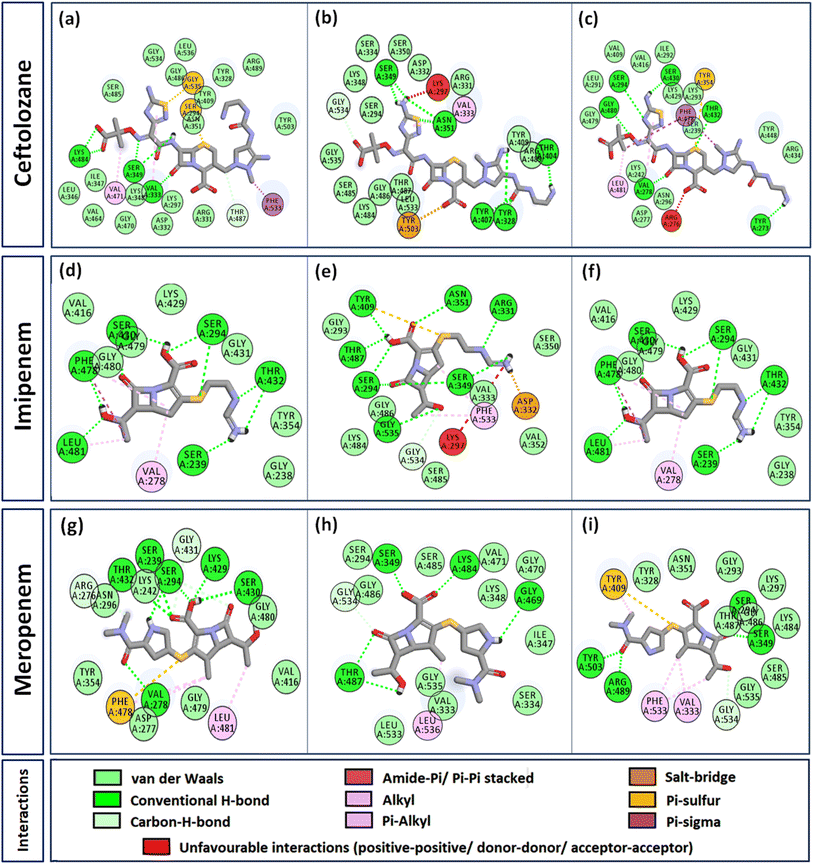 |
| Fig. 4 Interaction profiles of the parent and mutant PBP3s with ceftolozane, imipenem and meropenem (a) PBP3 + ceftolozane (b) PBP3_F533L + ceftolozane (c) PBP3_T91A + ceftolozane (d) PBP3 + imipenem (e) PBP3_F533L + imipenem (f) PBP3_T91A + imipenem (g) PBP3 + meropenem (h) PBP3_F533L + meropenem (i) PBP3_T91A + meropenem. | |
Structural stability assessment of protein complexes using MDS
MDS analyses on the protein complexes identified a stable trajectory for PBPB3 parent and mutants with cefiderocol. The binding of imipenem to PBPB3 wild, PBP3_F533L and PBP3_T91A observed an average RMSD of 0.310 nm, 0.282 nm and 0.319 nm respectively. While with cefiderocol, the RMSD trajectory for the proteins (wild, PBP3_F533L and PBP3_T91A) was observed to be 0.298 nm, 0.381 nm and 0.348 nm respectively [Fig. 5(a)]. The overall backbone fluctuations were identified to be minimal (∼0.02 nm) across the protein complexes, with a slight increase in the fluctuations observed in PBP3_F533L (0.168 nm) and PBP3_T91A (0.165 nm) when bound of cefiderocol [Fig. 5(b)]. The radius of gyration (Rg) was observed to be slightly reduced (∼0.05 nm) depicting an increase in the compactness of the parent (0.294 nm) and mutant proteins (F533L: 2.926 nm; T91A: 2.908 nm) when compared to the protein complexes formed with imipenem [Fig. 5(c)]. The binding affinity between cefiderocol and PBP3 proteins can be attributed to the consistent H-bonds observed in the docked complexes throughout the simulation. Around three H-bonds were consistent with PBP3_cef, while PBP3_F533L_cef and PBP3_T91A_cef showed around two to six bonds. This was evidently higher when compared to the docked complexes of PBP3 proteins and imipenem, where PBP3, PBP3_F533L and PBP3_T91A depicted one to three H-bonds throughout the simulation [Fig. 5(d)]. The interaction energy (IE) plot depicted favorable binding of the parent and mutant proteins with cefiderocol than imipenem. The total IE for the parent protein with cefiderocol was observed to be −207.352 kcal mol−1 while with imipenem, it was observed to be −75.889 kcal mol−1. Similarly the mutant proteins, namely PBP3_F533L_CEF observed an IE of −40.294 kcal mol−1 while PBP3_T91A_CEF observed an IE of −178.414 kcal mol−1. This was evidently lower than the IE observed for PBP3_F533L_IMP (−13.214 kcal mol−1) and PBP3_T91A_IMP (−29.847 kcal mol−1) as represented in Fig. 5(e). A stable potential energy plot was observed for all the protein complexes [Fig. 5(f)]. The compactness of the PBP3 parent resulted in a reduced SASA area (247 nm2) when compared to imipenem (252 nm2). However, there was minimal change in the SASA area for the mutants [Fig. 5(g)]. The solvation free energy plot indicated that cefiderocol bound PBP3 proteins (PBP3: −64.210 kcal mol−1; F533L: −64.252 kcal mol−1; T91A: −61.561 kcal mol−1) were energetically more favorable than imipenem bound PBP3 proteins (PBP3: −62.569 kcal mol−1; F533L: −60.674 kcal mol−1; T91A: −62.298 kcal mol−1) as depicted in Fig. 5(h).
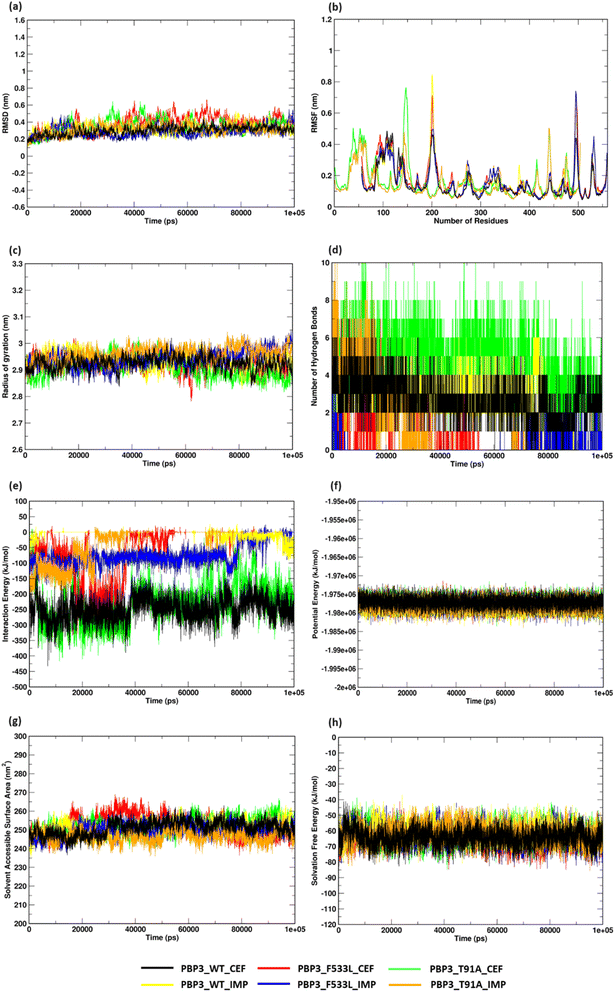 |
| Fig. 5 Structural stability assessment of protein complexes (a) RMSD profile (b) RMSF plot (c) radius of gyration (d) hydrogen bonds (e) interaction energy plot (f) potential energy (g) solvent accessible surface area (h) solvation free energy plot. | |
MM/PBSA calculation to validate energy profiles of docked complexes
After the stabilization of the complexes, the MM/PBSA binding energy for the protein-ligand complexes was computed. We observed the average binding energy of the complexes (PBP3, F533L and T91A) with cefiderocol to be −15.12 kcal mol−1, −6.35 kcal mol−1 and −34.35 kcal mol−1 respectively, while the average binding energy with imipenem was observed to be lower (PBP3: −14.13 kcal mol−1, F533L: −2.34 kcal mol−1 and T91A: −4.09 kcal mol−1) [Fig. 6(a)]. The high binding scores for complexes with cefiderocol can be attributed to the electrostatic energy (EEL) which was −94.76 kcal mol−1 for PBP3, −24.23 kcal mol−1 for F533L and −99.66 kcal mol−1 for T91A. This was evidently lower when compared to the EEL observed for docked complexes with imipenem, which showed −21.58 kcal mol−1 for PBP3, −2.24 kcal mol−1 for F533L and −7.8 kcal mol−1 for T91A [Fig. 6(b)]. The gas-phase molecular mechanics free energy (GGAS) was also markedly lower for docked complex with cefiderocol than imipenem [Fig. 6(c)]. From Fig. 6(d), the van der Waals energy of the docked complexes indicated favorable binding with cefiderocol complexes with PBP3 (−45.63 kcal mol−1), F533L (−8.25 kcal mol−1) and T91A (−34.69 kcal mol−1) than with imipenem (PBP3: −45.63 kcal mol−1; F533L: −5.43 kcal mol−1; T91A: −7.72 kcal mol−1). The average binding energy including, VDWAALS, EEL, GGAS, GSOLV and EEL is tabulated in Table 4. The MM/PBSA binding energy indicated a robust binding of the PBP3 proteins with cefiderocol, which is consistent with the observed intermolecular docking.
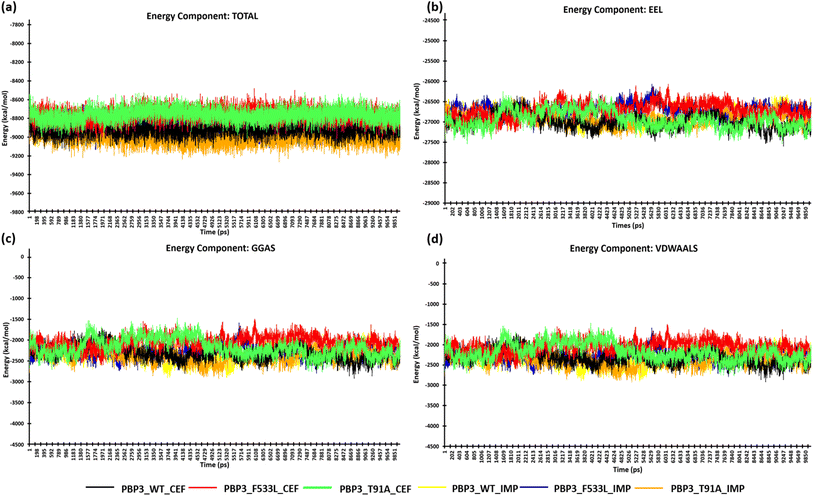 |
| Fig. 6 MM/PBSA calculation of the protein complexes (a) energy component-TOTAL (b) energy component-EEL (c) energy component-GGAS (d) energy component-VDWAALS. | |
Table 4 MM/PBSA calculations for PBP3 proteins complexed with cefiderocol and imipenem
Docked complexes |
VDWAAL (kcal mol−1) |
EEL (kcal mol−1) |
GGAS (kcal mol−1) |
GSOLV (kcal mol−1) |
TOTAL (kcal mol−1) |
PBP3_cef |
−45.63 |
−94.76 |
−140.38 |
125.26 |
−15.12 |
PBP3_imp |
−45.63 |
−21.58 |
−42.76 |
28.63 |
−14.13 |
PBP3_F533L_cef |
−8.25 |
−24.23 |
−32.49 |
26.13 |
−6.35 |
PBP3_F533L_imp |
−5.43 |
−2.24 |
−7.67 |
5.33 |
−2.34 |
PBP3_T91A_cef |
−34.69 |
−99.66 |
−134.35 |
100 |
−34.35 |
PBP3_T91A_imp |
−7.72 |
−7.8 |
−15.52 |
11.43 |
−4.09 |
Discussion
PBP3 is amongst the most extensively exploited drug-targets for β-lactam antibiotics in P. aeruginosa and hence suffers predominant mutational alterations driven by antibiotic stress. Resistance in fast evolving XDR strains of P. aeruginosa is dynamic and may be a result of concerted expression of multiple proteins like drug-efflux pumps (MexEF-OprN), beta-lactamase enzymes (AmpC) and mutations in major drug-targets (PBP3, RNA polymerase).8,9,11,43 However, it is a tedious task to understand the mutational landscape for therapeutic advances, especially by differentiating relevant mutations from natural polymorphisms. Continuous surveillance and in depth structural analysis are essential to understand the underlying impact of mutations, their stability and selection, for sustainable therapeutic designs.10
In the present study, mutations T91A and F533L were reported in carbapenemase-positive P. aeruginosa ST282 (n = 6) in clinical isolates from south India. Extensive resistances to beta-lactams were observed in the isolates. F533L has been earlier reported to reduce the efficacy of ceftazidime, cefepime, piperacillin–tazobactam, meropenem, ceftazidime–avibactam and tazobactam, however, the underlying structural cause was not comprehensively determined.10 F533L is located within the transpeptidase domain [IPR001460] and T91A is located within the dimerization domain [IPR005391] of PBP3. Comparatively, F533L lying in the β-lactam binding domain induced more local alterations (hindering drug-affinity) than T91A lying in the dimerization domain (inducing overall structural stability). The structural profile showed a loss of intra-chain hydrogen bonds and hydrophobic interactions as a result of F533L, which further resulted in its overall fluctuations (higher RMSD) and loss of compactness (greater Rg) in the structure than the parent protein and T91A variant [Fig. 2(a)–(e)].20 The loss of intra-chain interactions also induced a minor increase in local flexibility as observed from the backbone trajectory and overall average vibrational entropies [Fig. 1]. This marginally increased the solvent accessible surface in PBP3_F533L for non-specific (unfavorable) interactions that compromised the conformational specificity of the target drugs to the binding pocket despite the compatibility of the conventionally interacting atoms (Fig. 3 and 4). F533L induced binding pocket volume distortion from 177 Å to 195 Å was observed in CastP results (not shown) but not for T91A may be due to the compensatory polar and van der Waals intra-chain interactions formed by the later [Fig. 2(a)]. The overall low residue level fluctuations (<0.1 Å) and unaltered folding free-energies were reflected upon the increased conservation confidence of the mutants despite minor local adjustments in the structure that facilitated drug-resistance [ESI Files S2(a), (b) and S3†]. The protein complex stability assessment also conferred higher stability to cefiderocol with PBP3 proteins than to imipenem. The minimal fluctuations, increasing compactness, higher interaction energy and higher inter-molecular hydrogen bonding between cefiderocol and PBP3 proteins further support the sustainment of susceptibility of cefiderocol to multi-PBPs in P. aeruginosa. The high binding affinity of the complexes was also validated using MM/PBSA calculations. The calculations involve long-range polar and short-range non-polar interactions to investigate the protein-ligand interactions.44 The binding energies calculated from MM/PBSA were consistent with the intermolecular docking, where an evidently higher binding affinity was observed between cefiderocol and PBP3, especially in mutants F533L and T91A (Table 4).
Furthermore, the strains expressed KPC-2 and OXA-10, which might have potentially hydrolyzed their substrates imipenem [DB01598] and meropenem [DB00760] respectively resulting in their high MICs. However, the susceptibility of cefiderocol was sustained due to its multi-PBP specificity and lower affinity towards β-lactamases (Table 2). Hence it can still be the drug of choice against similar P. aeruginosa pathotypes (especially PBP3-variants) and can also be tested in combination with suitable β-lactamase inhibitors.45 The MIC patterns were supported by a change in inhibition constant (ki) calculated as ΔG = RT
ln(ki) [where T is the absolute temperature and R is the gas constant]. Greater propensity for protein-ligand interaction is indicated by low ki.46,47 In the present study, it was also observed that avibactam and relebactam can reduce the MIC values by hindering the efficiency of the β-lactamases (Table 1). Hence, new BL–BLI formulations using avibactam and relebactam can show promising results against XDR P. aeruginosa. Notably, cefiderocol, a siderophore cephalosporin, has emerged as a promising addition to the antibiotic arsenal. Cefiderocol demonstrates a unique mechanism of action, utilizing the bacterial iron transport system to penetrate the outer membrane of Gram-negative bacteria, including multidrug-resistant strains, such as Pseudomonas aeruginosa, Acinetobacter baumannii and Enterobacterales. Cefiderocol contains a chlorocatechol group at the end of the C-3 side chain, which, further increases its stability against β-lactamases.48 Moreover, cefiderocol has shown potential for treating infections caused by carbapenem-resistant organisms, filling a critical gap in the antibiotic armamentarium. With a broad spectrum of action, cefiderocol has shown stability against β-lactamases including serine-carbapenemases (class A such as KPC and class D such as OXA-48) and metallo-β-lactamases (class B such as VIM, NDM and IMP), however the underlying mechanisms were not elucidated explicitly.49 In a recent study, cefiderocol showed clinical efficacy against carbapenem-resistant Acinetobacter baumannii expressing blaOXA-58, blaOXA-51, blaOXA-23, blaOXA-24.50 Clinical studies have demonstrated its efficacy and safety profile in the management of difficult-to-treat infections. However, genomic surveillance and stewardship efforts are essential to understand the susceptibility underpinnings and mitigate the risk of emerging resistant strains.48,51,52 Therefore, the present study holds significance in elucidating the potential underlying mechanism for the efficiency of cefiderocol against carbapenem-resistant Pseudomonas, particularly expressing carbapenemases as well as harbouring drug-target mutations. The same will definitely encourage the clinical application of cefiderocol against similar MDR strains and enhance our general understanding for new-antibiotic designs.
Our group has recently reported the application of novel β-lactam–β-lactamase inhibitors against similar targets from XDR Acinetobacter baumannii.53 However, mutational resistome against specific antibiotics in XDR species has evolved by continuous exposure to the antibiotic themselves as well as other concurrently used antibiotics or earlier exposures to related antibiotics.11 A recent report demonstrated the concurrent resistance to cefotaxime and meropenem exploiting identical PBP-targets in S. pneumoniae.14 Therefore, the selection of stable mutants can be reduced by minimizing antibiotic stress on specific P. aeruginosa targets and focusing on antibiotics having multiple-targets, like cefiderocol.
Conclusion
PBP3 mutations T91A and F533L in carbapenemase-positive P. aeruginosa rendered β-lactam antibiotics ineffective thereby challenging β-lactam–β-lactamase inhibitor (BL–BLI) combinations. The structural dynamics simulations and docking studies revealed stability of both the mutants by local flexibility adjustments that favored their selection to hinder the drug-interaction with active-site through unfavorable clashes. However, cefiderocol was found effective due to its higher affinities towards multiple-PBPs and lower affinity towards β-lactamases as compared to the other antibiotics. The study portrayed the impact of uncharged non-polar amino-acid substitutions in PBP-targets that hindered drug-affinity without affecting protein-stability. Hence, antibiotics like cefiderocol with multi-target specificity can be used to overcome the challenge imposed by mutations in conventional protein-targets.
Data availability
The data supporting this article have been included as part of the ESI.†
Author contributions
AA: supervision, fund acquisition and manuscript review. BV: conceptualization, supervision and manuscript review. SR: supervision and manuscript review. SG: in silico analysis, writing original draft. GA: in silico analysis, writing original draft. SB: in silico study design, experimentation and analysis, writing original draft.
Conflicts of interest
The authors declare that there is no conflict of interest.
Acknowledgements
The authors would like to thank the Indian Council of Medical Research (ICMR), Govt. of India, for the research grant IRIS ID: 2021-11889; AMR/Adhoc/290/2022-ECD-II. The authors would like to acknowledge Dr Yamuna Devi Bakthavatchalam (Scientist-C, CMC-Vellore) for her invaluable inputs in framing the study. The authors thank Wockhardt Limited, Aurangabad for their generous help regarding antibiotics availability. The authors do thank the administration of VIT, Vellore.
References
- Q. Shi, C. Huang, T. Xiao, Z. Wu and Y. Xiao, Antimicrob. Resist. Infect. Control, 2019, 8, 68 CrossRef.
- J. P. Horcajada, M. Montero, A. Oliver, L. Sorlí, S. Luque, S. Gómez-Zorrilla, N. Benito and S. Grau, Clin. Microbiol. Rev., 2019, 32(4), e00031 CrossRef CAS PubMed.
- Centers for Disease Control and Prevention, Pseudomonas aeruginosa in Healthcare Settings, 2024, Available at: https://www.cdc.gov/healthcare-associated-infections/ Search PubMed.
- M. Bassetti, L. Taramasso, D. R. Giacobbe and P. Pelosi, Expert Rev. Anti-Infect. Ther., 2012, 10, 585–596 CrossRef CAS PubMed.
- C. López-Causapé, L. M. Sommer, G. Cabot, R. Rubio, A. A. Ocampo-Sosa, H. K. Johansen, J. Figuerola, R. Cantón, T. J. Kidd, S. Molin and A. Oliver, Sci. Rep., 2017, 7, 5555 CrossRef PubMed.
- N. Woodford, J. F. Turton and D. M. Livermore, FEMS Microbiol. Rev., 2011, 35, 736–755 CrossRef CAS PubMed.
- A. Oliver, X. Mulet, C. López-Causapé and C. Juan, Drug Resistance Updates, 2015, 21–22, 41–59 CrossRef.
- G. Cabot, C. López-Causapé, A. A. Ocampo-Sosa, L. M. Sommer, M. Á. Domínguez, L. Zamorano, C. Juan, F. Tubau, C. Rodríguez, B. Moyà, C. Peña, L. Martínez-Martínez, P. Plesiat and A. Oliver, Antimicrob. Agents Chemother., 2016, 60, 7415–7423 CrossRef CAS.
- E. del Barrio-Tofiño, C. López-Causapé, G. Cabot, A. Rivera, N. Benito, C. Segura, M. M. Montero, L. Sorlí, F. Tubau, S. Gómez-Zorrilla, N. Tormo, R. Durá-Navarro, E. Viedma, E. Resino-Foz, M. Fernández-Martínez, C. González-Rico, I. Alejo-Cancho, J. A. Martínez, C. Labayru-Echverria, C. Dueñas, I. Ayestarán, L. Zamorano, L. Martinez-Martinez, J. P. Horcajada and A. Oliver, Antimicrob. Agents Chemother., 2017, 61(11), e01589 CrossRef PubMed.
- C. López-Causapé, G. Cabot, E. del Barrio-Tofiño and A. Oliver, Front. Microbiol., 2018, 9, 685 CrossRef.
- C. Muller, P. Plésiat and K. Jeannot, Antimicrob. Agents Chemother., 2011, 55, 1211–1221 CrossRef CAS PubMed.
- C. Juan, G. Torrens, M. González-Nicolau and A. Oliver, FEMS Microbiol. Rev., 2017, 41, 781–815 CrossRef CAS PubMed.
- A. J. Kunz Coyne, A. El Ghali, D. Holger, N. Rebold and M. J. Rybak, Infect. Dis. Ther., 2022, 11, 661–682 CrossRef PubMed.
- R. Varghese, S. Basu, A. Neeravi, A. Pragasam, V. Aravind, R. Gupta, A. Miraclin, S. Ramaiah, A. Anbarasu and B. Veeraraghavan, Front. Microbiol., 2022, 12, 1–13 Search PubMed.
- C. Shankar, S. Basu, B. Lal, S. Shanmugam, K. Vasudevan, P. Mathur, S. Ramaiah, A. Anbarasu and B. Veeraraghavan, Front. Cell. Infect. Microbiol., 2021, 11, 1–18 Search PubMed.
- P. Priyamvada, R. Debroy, A. Anbarasu and S. Ramaiah, World J. Microbiol. Biotechnol., 2022, 38(9), 153 CrossRef CAS PubMed.
- J. L. Sussman, D. Lin, J. Jiang, N. O. Manning, J. Prilusky, O. Ritter and E. E. Abola, Acta Crystallogr., Sect. D: Biol. Crystallogr., 1998, 54, 1078–1084 CrossRef CAS.
- M. Varadi, S. Anyango, M. Deshpande, S. Nair, C. Natassia, G. Yordanova, D. Yuan, O. Stroe, G. Wood, A. Laydon, A. Žídek, T. Green, K. Tunyasuvunakool, S. Petersen, J. Jumper, E. Clancy, R. Green, A. Vora, M. Lutfi, M. Figurnov, A. Cowie, N. Hobbs, P. Kohli, G. Kleywegt, E. Birney, D. Hassabis and S. Velankar, Nucleic Acids Res., 2022, 50, D439–D444 CrossRef CAS PubMed.
- W. Kaplan and T. G. Littlejohn, Briefings Bioinf., 2001, 2, 195–197 CrossRef CAS PubMed.
- S. Basu, A. Naha, B. Veeraraghavan, S. Ramaiah and A. Anbarasu, J. Cell. Biochem., 2022, 123, 115–127 CrossRef CAS.
- R. Debroy and S. Ramaiah, Int. J. Biochem. Cell Biol., 2022, 151, 106279 CrossRef CAS PubMed.
- A. Naha and S. Ramaiah, 3 Biotech, 2022, 12, 1–15 CrossRef PubMed.
- S. Basu, R. Varghese, R. Debroy, S. Ramaiah, B. Veeraraghavan and A. Anbarasu, Microb. Pathog., 2022, 170, 105694 CrossRef CAS PubMed.
- S. Hunter, R. Apweiler, T. K. Attwood, A. Bairoch, A. Bateman, D. Binns, P. Bork, U. Das, L. Daugherty, L. Duquenne, R. D. Finn, J. Gough, D. Haft, N. Hulo, D. Kahn, E. Kelly, A. Laugraud, I. Letunic, D. Lonsdale, R. Lopez, M. Madera, J. Maslen, C. McAnulla, J. McDowall, J. Mistry, A. Mitchell, N. Mulder, D. Natale, C. Orengo, A. F. Quinn, J. D. Selengut, C. J. A. Sigrist, M. Thimma, P. D. Thomas, F. Valentin, D. Wilson, C. H. Wu and C. Yeats, Nucleic Acids Res., 2009, 37, D211–D215 CrossRef CAS.
- S. Kim, P. A. Thiessen, E. E. Bolton, J. Chen, G. Fu, A. Gindulyte, L. Han, J. He, S. He, B. A. Shoemaker, J. Wang, B. Yu, J. Zhang and S. H. Bryant, Nucleic Acids Res., 2016, 44, D1202–D1213 CrossRef CAS PubMed.
- N. M. O'Boyle, M. Banck, C. A. James, C. Morley, T. Vandermeersch and G. R. Hutchison, J. Cheminf., 2011, 3, 1–14 Search PubMed.
- E. Cilia, R. Pancsa, P. Tompa, T. Lenaerts and W. F. Vranken, Nucleic Acids Res., 2014, 42, 264–270 CrossRef.
- C. H. M. Rodrigues, D. E. V. Pires and D. B. Ascher, Nucleic Acids Res., 2018, 46, W350–W355 CrossRef CAS PubMed.
- M. Goethe, I. Fita and J. M. Rubi, J. Chem. Theory Comput., 2015, 11, 351–359 CrossRef CAS PubMed.
- D. E. V. Pires, D. B. Ascher and T. L. Blundell, Nucleic Acids Res., 2014, 42, 314–319 CrossRef PubMed.
- Q. Hou, F. Pucci, F. Ancien, J. M. Kwasigroch, R. Bourgeas and M. Rooman, Bioinformatics, 2021, 37, 1963–1971 CrossRef CAS.
- H. Ashkenazy, S. Abadi, E. Martz, O. Chay, I. Mayrose, T. Pupko and N. Ben-Tal, Nucleic Acids Res., 2016, 44, W344–W350 CrossRef CAS.
- M. Jamroz, A. Kolinski and S. Kmiecik, Nucleic Acids Res., 2013, 41, 427–431 CrossRef.
- D. Van Der Spoel, E. Lindahl, B. Hess, G. Groenhof, A. E. Mark and H. J. C. Berendsen, J. Comput. Chem., 2005, 26, 1701–1718 CrossRef CAS.
- G. M. Morris, D. S. Goodsell, M. E. Pique, W. L. Lindstrom, R. Huey, W. E. Hart, S. Halliday, R. Belew and A. J. Olson, J. Comput. Chem., 2009, 30, 2785–2791 CrossRef CAS PubMed.
- S. Sainsbury, L. Bird, V. Rao, S. M. Shepherd, D. I. Stuart, W. N. Hunter, R. J. Owens and J. Ren, J. Mol. Biol., 2011, 405, 173–184 CrossRef CAS PubMed.
- W. Tian, C. Chen, X. Lei, J. Zhao and J. Liang, Nucleic Acids Res., 2018, 46, W363–W367 CrossRef CAS PubMed.
- B. L. Jejurikar and S. H. Rohane, Asian J. Res. Chem., 2021, 14, 135–138 Search PubMed.
- S. K. Miryala, S. Basu, A. Naha, R. Debroy, S. Ramaiah, A. Anbarasu and S. Natarajan, J. Mol. Liq., 2021, 341, 117340 CrossRef CAS.
- K. Vasudevan, S. Basu, A. Arumugam, A. Naha, S. Ramaiah, A. Anbarasu and B. Veeraraghavan, bioRxiv, preprint, 2021, DOI:10.1101/2021.07.05.451101.
- M. S. Valdés-Tresanco, M. E. Valdés-Tresanco, P. A. Valiente and E. Moreno, J. Chem. Theory Comput., 2021, 17, 6281–6291 CrossRef PubMed.
- B. R. Miller, T. D. McGee, J. M. Swails, N. Homeyer, H. Gohlke and A. E. Roitberg, J. Chem. Theory Comput., 2012, 8, 3314–3321 CrossRef CAS.
- P. Juarez, K. Jeannot, P. Plésiat and C. Llanes, Antimicrob. Agents Chemother., 2017, 61(8), e00585 CrossRef CAS PubMed.
- C. Wang, D. Greene, L. Xiao, R. Qi and R. Luo, Front. Mol. Biosci., 2018, 4, 87 CrossRef PubMed.
- M. Rajavel, V. Kumar, H. Nguyen, J. Wyatt, S. H. Marshall, K. M. Papp-Wallace, P. Deshpande, S. Bhavsar, R. Yeole, S. Bhagwat, M. Patel, R. A. Bonomo and F. van den Akker, mBio, 2021, 12(1), e03058 CrossRef CAS PubMed.
- N. M. Tam, M. Q. Pham, H. T. Nguyen, N. D. Hong, N. K. Hien, D. T. Quang, H. T. Thu Phung and S. T. Ngo, RSC Adv., 2021, 11, 22206–22213 RSC.
- C. L. D. Ortiz, G. C. Completo, R. C. Nacario and R. B. Nellas, Sci. Rep., 2019, 9, 17096 CrossRef PubMed.
- T. Aoki, H. Yoshizawa, K. Yamawaki, K. Yokoo, J. Sato, S. Hisakawa, Y. Hasegawa, H. Kusano, M. Sano, H. Sugimoto, Y. Nishitani, T. Sato, M. Tsuji, R. Nakamura, T. Nishikawa and Y. Yamano, Eur. J. Med. Chem., 2018, 155, 847–868 CrossRef CAS PubMed.
- K. S. Kaye, T. Naas, J. M. Pogue and G. M. Rossolini, Infect. Dis. Ther., 2023, 12, 777–806 CrossRef PubMed.
- A. Uskudar-Guclu, S. Danyildiz, H. C. Mirza, M. Akcil Ok and A. Basustaoglu, Eur. J. Clin. Microbiol. Infect. Dis., 2024, 43(6), 1171–1179 CrossRef CAS PubMed.
- M. Bassetti, R. Echols, Y. Matsunaga, M. Ariyasu, Y. Doi, R. Ferrer, T. P. Lodise, T. Naas, Y. Niki, D. L. Paterson, S. Portsmouth, J. Torre-Cisneros, K. Toyoizumi, R. G. Wunderink and T. D. Nagata, Lancet Infect. Dis., 2021, 21, 226–240 CrossRef CAS PubMed.
- F. M. E. Wagenlehner and K. G. Naber, Lancet Infect. Dis., 2019, 19, 22–23 CrossRef PubMed.
- A. Naha, S. Vijayakumar, B. Lal and B. A. Shankar, J. Cell. Biochem., 2021, 1–25 Search PubMed.
|
This journal is © The Royal Society of Chemistry 2024 |