DOI:
10.1039/D4RA03816A
(Paper)
RSC Adv., 2024,
14, 29151-29159
Surface-enhanced Raman spectroscopy (SERS) for the diagnosis of acute myocardial infarction (AMI) using blood serum samples†
Received
23rd May 2024
, Accepted 26th July 2024
First published on 13th September 2024
Abstract
Acute myocardial infarction (AMI) is a serious medical condition generally known as heart attack, which is caused by the decreased or completely blocked blood flow to a part of the heart muscle. It is a significant cause of both mortality and morbidity throughout the world. Cardiac troponin-I (cTnI) is an important biomarker at different stages of AMI and is one of the most specific and widely used cardiac skeletal muscle proteins. Delays in medical treatment and inaccurate diagnosis might be the main cause of death of AMI patients. To overcome the death rate of AMI patients, early diagnosis of this disease is crucial. In the current study, surface-enhanced Raman spectroscopy (SERS) is employed for the characterization and diagnosis of this disease using blood serum samples from 49 clinically confirmed acute myocardial infarction (AMI) patients and 17 healthy persons. Silver nanoparticles (AgNPs) are used as the SERS substrate for the recognition of characteristic SERS spectral features, differentiating between healthy and AMI-positive samples. The acute myocardial infarction-positive blood serum samples reveal remarkable differences in spectral intensities at 534, 697, 744, 835, 927, 941, 988, 1221, 1303, 1403, 1481, 1541, 1588 and 1694 cm−1. For the differentiation and quantitative analysis of the SERS spectra, multivariate chemometric tools (including principal component analysis (PCA) and partial least squares regression (PLSR)) are employed. A PLSR model established on the basis of differentiating the SERS spectral features is found to be helpful in the prediction of the levels of cardiac troponin-I (cTnI) in the blood serum samples with the root mean square error of calibration (RMSEC) value of 2.98 ng mL−1 and root mean square errors of prediction (RMSEP) value of 3.98 ng mL−1 for S7.
1 Introduction
Acute myocardial infarction (AMI), also known as heart attack, is a cardiovascular disease (CVD) that is caused by the decreased or completely blocked blood flow to a part of the heart muscle. Acute myocardial infarction is a very common disease, affecting about 3 million people worldwide.1 Annually, more than one million people die in the United States due to this disease. The physical symptoms of myocardial infarction include chest pain, while electrocardiogram (ECG) test results indicate T wave abnormalities and ST segment.2 The enzyme-linked immunosorbent assay (ELISA) is an immunological test that relies on the principle of antigen–antibody binding and is used to detect the target molecule. This method is used for the detection of AMI antigens. Conversely, an electrocardiogram (ECG) is a diagnostic test in which heartbeats produce alterations in the morphology or wave frequency and measure the electrical activity of the heart over a period of time. However, these methods have some limitations, such as false negative and false positive results, limited quantitative accuracy, tediousness and expensive procedures, continuous monitoring, and the requirement of specialized and expensive instrumentation.3–6 Patients with myocardial infarction have high levels of serum cardiac muscle enzyme. However, it has also been observed that there are patients having specifically less ST segment changes and no increment in the cardiac muscle enzyme, but they are still afflicted with myocardial infarction.7 This is due to cardiac troponin-I, making them unique due to the regulatory protein.8 The calcium-mediated interaction between actin and myosin is controlled by cardiac regulatory proteins, including cardiac troponin-T, troponin-I and troponin-C. It has been observed that patients in the early stage of myocardial infarction quickly recover and have a greater chance of returning to their lives, as compared to those who are diagnosed at the later stage.9
Surface-enhanced Raman spectroscopy (SERS) is a powerful analytical technique that combines traditional Raman spectroscopy with the enhanced signals generated by metal nanoparticles such as silver or gold nanoparticles, which act as SERS substrates. The Raman signal of molecules adsorbed on gold or silver nanoparticles (Au or Ag NPs) can be enhanced by 10 (ref. 10–14) times through surface-enhanced Raman spectroscopy (SERS).9–11 SERS allows for the detection of trace amounts of analytes, provides good spectral stability and reproducibility, is inexpensive, requires no sample preparation, and gives accurate and rapid results. Due to these advantages and its highly sensitive and rapid optical detection method, SERS technology has also produced a number of significant achievements in the biomedical diagnostic fields.12–15 Low concentrations of analytes in blood serum samples can be structurally fingerprinted by performing SERS, which has ultra-high sensitivity owing to the plasmon-mediated amplification of electrical fields or chemical enhancement.16 SERS has great potential for the analysis of blood serum samples for diagnosing different diseases, including breast cancer, hepatitis B, hepatitis C, and typhoid.3,17–19 The main advantages of surface-enhanced Raman spectroscopy are its sensitivity and selectivity, which enable the detection of a less-than-monolayer coverage of an analyte on a surface of the serum even in a complex mixture.20,21
Blood serum has the advantage of an easy collection and common usage in the diagnostic testing of myocardial infarction.22 The blood serum comprises DNA, lipids, and proteins, which can be detected with the help of surface-enhanced Raman spectroscopy (SERS).23 Electrocardiograms (ECGs) are currently the primary method used to measure and diagnose AMI. However, not all AMI patients exhibit electrocardiographic irregularities and the sensitivity of the ECG is limited. AMI can be identified using various cardiac biomarkers, such as cardiac troponin I (cTnI), cardiac troponin T (cTnT), creatine kinase-MB, and myoglobin, to obtain information across the problems with the ECG. When compared to other AMI biomarkers, cTnI is considered as the “gold standard” for diagnosing AMI. Muscle contraction is controlled by cTnI, which is a component of the troponin complex. Three different types of molecules make up the troponin complex: troponin C (TnC), cTnI, and troponin T (cTnT). cTnI is a highly unstable protein that is sensitive to proteolytic degradation. Cells suffer from severe ischemia during an infarction, and they will die in 30–60 min if circulation is not restored.24–28
In the current study, surface-enhanced Raman spectroscopy (SERS) is employed for the characterization and diagnosis of acute myocardial infarction (AMI) using blood serum samples of the clinically confirmed 49 patients and 17 healthy ones. The silver nanoparticles (AgNPs) are used as the SERS substrate for recognizing characteristic SERS spectral features and differentiating between healthy and AMI positive samples. Notably, according to a careful literature review, there is no such report published yet.
2 Materials and methods
2.1. Preparation of silver nanoparticles (Ag-NPs)
The chemical reduction method has been adopted to prepare silver nanoparticles. This method uses silver nitrate (AgNO3) and trisodium citrate (Na3C6H5O7) as a precursor and reducing agent, respectively. In 500 mL deionized water, 0.085 g of AgNO3 is added and heated at 100 °C, followed by the addition of 0.025 g trisodium citrate. The solution is heated on a hot plate with the use of a magnetic stirrer for an hour until grey-colored silver nanoparticles (Ag NPs) are prepared, which will be employed as the SERS substrate. The characterization of AgNPs has already been discussed in a previously published paper.18
2.2. Isolation of serum from the blood of acute myocardial infarction (AMI) and healthy individuals
As the blood sample forms clot it is removed which leaves behind clear yellowish fluid called serum.29 It contains various proteins, electrolytes, hormones, and other molecules that can provide important information about the health of the patient, including markers of cardiac injury in the case of AMI. All serum samples (n = 66) were obtained from Nishtar Medical University Multan, Pakistan, for this study, including those of 17 healthy patients with diagnosed healthy and 49 acute myocardial infarction patients with confirmed clinical diagnosis.
2.3. SERS spectral acquisition
Using silver nanoparticles as a substrate, a Raman micro-spectrometer (ATR8300BS Optosky, China) equipped with a 785 nm diode laser as an excitation source was used to record all SERS spectra. The sample was held using an aluminum slide with a small groove. Using a micropipette, 40 μL of each sample was incubated with 40 μL of the silver nanoparticles for 30 minutes. From this incubation mixture, 50 μL was transferred to the aluminum slide to acquire the SERS spectra. The sample was focused using a 40× objective, which delivered laser light of 50 mW on the sample. An air-cooled charged coupled detector (CCD) was employed for the reduction of electrical noise. For every sample, 15 SERS spectra were collected at a 15 s integration time in the range of 330 to 1800 cm−1.
2.4. SERS data preprocessing
Using MATLAB R2009a (The Math Works, USA), data preprocessing and analysis were performed. This includes vector normalization, baseline correction, substrate removal, and smoothing of the SERS spectra. The spectra are normalized and noise is removed by the Savitzky–Golay filter algorithm, which makes the peaks more distinct and noticeable. The spectral baseline was adjusted using the rubber band and polynomial methods. First, by using rubber bands, baseline correction was performed, and any remaining baseline correction was performed using polynomials.
2.5. SERS data analysis
Table 1 shows the peak assignments of the SERS spectral features, along with references, which were obtained from the literature for SERS results interpretation. Principal component analysis (PCA) and partial least squares regression (PLSR) analysis were used for data analysis. To obtain the relevant information from spectral data sets, PCA, an unsupervised statistical technique was used; it reduces the dimensionality of the measured Raman spectral data while keeping the sources of variability intact. This results in a number of important principal components (PCs). The PCA helps to differentiate the SERS spectra acquired from healthy and AMI-positive blood serum samples. The PCA scatter plot shows the clusters of SERS spectra of the samples to identify the variability in the samples. The structural and spectral properties of the fingerprint regions in the positive and negative half of the spectrum are principally described by the loading spectra. Note that the loadings are identified as differentiating and significant SERS spectral features.17,44,45
Table 1 Peak assignment comparison of the SERS spectral features in the blood serum samples of healthy and acute myocardial infarction positive samples having low levels and high levels of cardiac troponin-I (cTnI)
Peaks (cm−1) |
Assignment |
Reference |
486 |
DNA |
30 |
534 |
Cholesterol ester |
31 |
591 |
Ascorbic acid, amide IV |
32 |
629 |
δ(C–S)/tyrosine |
30 |
697 |
C–S stretch |
33 |
723 |
C–C stretching protein |
34 |
744 |
T (ring breathing mode of thymine in DNA/RNA) |
34 |
814 |
ν(C–C–O)/L-serine, glutathione |
35 |
835 |
Amino acid of (tyrosine) |
36 |
882 |
Tryptophan and tyrosine residues |
33 |
927 |
COO− wagging and stretching mode |
33 |
941 |
C–C stretching vibration |
37 |
988 |
Amine protein, C–N group |
38 |
1075 |
Stretching vibration (C–N), collagen |
32 |
1095 |
Stretching vibration (C–N), D-mannose |
32 |
1135 |
NH3+ deformation |
33 |
1203 |
Ring vibration, L-tryptophan, phenylalanine/hydroxyproline |
32 |
31 |
1221 |
Nucleic acid |
39 |
1303–1359 |
Tryptophan, α-helix, phospholipids |
40 |
1403 |
N N aromatic stretching |
41 |
1453 |
C–C aromatic ring vibration |
41 |
1481 |
Amide II |
39 |
1541 |
Amide carbonyl group |
42 |
1588 |
C O stretch |
36 |
1694 |
Amide I |
43 |
As a quantitative and a discriminant method, partial least squares regression (PLSR) was employed. The cross-validation method leave-one-out (leave all the SERS spectra of one sample out) was used for evaluating the prediction accuracy of the model. Using the prediction model with respect to the observed variables known as independent variables, the concentration of the analyte (which is also known as dependent variables) was created. The dependent variables are regressed against the independent variables using the regression parameter. The quality of the predictive model and the parameters for model optimization can be evaluated using the yielding residuals or the difference between the variables that are measured and those that are predicted.46,47
3 Results and discussion
To examine the enhancement effects of silver nanoparticles on the human blood serum, SERS spectra were acquired from the healthy samples and myocardial infarction-positive patients having low levels and high levels of cardiac troponin-I (cTnI).
Fig. 1 shows the mean SERS spectra of healthy serum, low disease serum and high disease serum samples. The prominent intensity-based SERS peaks found at 486, 591, 629, 723, 814, 882, 1135, 1203, 1359 and 1453 cm−1 are observed in healthy serum, and in serum with low and high levels of cardiac troponin-I (cTnI). A comparison of the SERS spectra of the healthy versus Acute myocardial infarction (AMI) having low levels and high levels of cardiac troponin-I (cTnI) reveals remarkable differences in spectral intensities at 534, 697, 744, 835, 927, 941, 988, 1075, 1095, 1221, 1303, 1403, 1481, 1541, 1588 and 1694 cm−1. SERS peak assignments are used to obtain a better understanding of the molecular basis of the SERS spectra of human blood serum. Table 1 lists the tentative peak assignments for the observed SERS features in accordance with the literature.
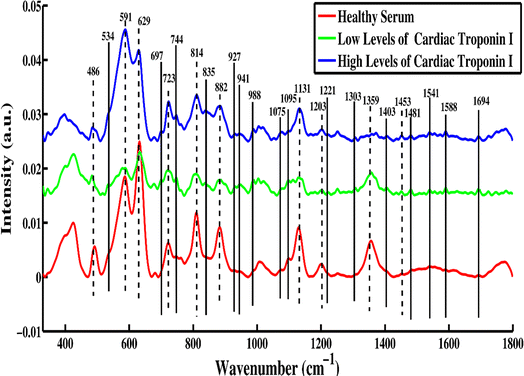 |
| Fig. 1 Mean SERS spectra of blood serum samples of healthy versus two stages of acute myocardial infarction-positive patients having low levels and high levels of cardiac troponin-I (cTnI). | |
To differentiate the SERS features between the disease-positive serum samples and healthy serum, solid lines and dotted lines are used for the SERS bands indication. A solid line represents the peaks that are present only in disease-positive serum samples having low and high levels of cardiac troponin-I, indicating striking differences. The dotted lines represent the peaks that are present in the serum from healthy patients and those with low levels of cardiac troponin-I (cTnI). Peaks are observed at 534 (cholesterol ester), 697 (C–S stretch), 744 (T(ring breathing mode of thymine in DNA/RNA)), 835 (amino acid of (tyrosine)), and 927 cm−1 (C–COO− stretch, phenylalanine). cTnI is a challenging analyte since it contains just eight aromatic amino acids out of its 210 amino acid sequence, and only the aromatic amino acid residues provide a strong SERS signal. Some other observed peaks include the following: 941 (C–C stretching vibration), 988 (amine protein with C–N group), 1075 (stretching vibration (C–N), collagen), 1095 (stretching vibration (C–N), D-mannose), 1221 (nucleic acid), 1303 (tryptophan, α-helix), 1403 (N
N aromatic stretching), 1481 (amide II) and 1541 cm−1 (amide carbonyl group). The SERS peak appearing at 1588 cm−1 (C
O stretch) observed in the low levels of cardiac troponin-I (cTnI) indicates that upon onset of the disease, protein denaturation takes place, which is due to the aromatic amino acid residue. The prominent peak at 1694 cm−1 associated with (amide-I) is more prominent in the serum samples containing low levels and high levels of cardiac troponin-I (cTnI) disease, as compared to the healthy samples.
However, certain intensity-based spectral features are observed in healthy serum, as well as low levels and high levels of cardiac troponin-I (cTnI), including 486 (DNA), 591 (amide IV), 629 δ(C–S), and 723 cm−1 (C–C stretching protein). Peaks at 814 (ν(C–C–O)/L-serine, glutathione) and 882 cm−1 show the tryptophan and tyrosine residues, which indicate the cTn-I protein. The SERS peaks at 1135 (NH3+ deformation), 1203 (hydroxyproline), 1359 (tryptophan, α-helix, phospholipids) and 1453 cm−1 (C–C aromatic ring vibration) are observed, indicating the intensity-based differences in the SERS spectra of healthy and acute myocardial infarction-positive serum samples containing low levels and high levels of cardiac troponin-I (cTnI).
3.1. Principal component analysis (PCA)
Using the principal component analysis (PCA), the Raman spectra of healthy/control serum samples and acute myocardial infarction-positive serum samples having low levels and high levels of cardiac troponin-I (cTnI) were differentiated. It was carried out using the SERS spectra of 17 healthy serum, 25 low levels, and 24 high levels of cardiac troponin-I blood serum samples.
Fig. 2(a) shows the PCA scatter plot of the low and high levels of cardiac troponin-I (cTnI) of disease serum samples versus healthy ones. The green clusters in the SERS spectral data indicate low levels of cardiac troponin-I (cTnI), while the red clusters have been associated with healthy controls. To determine the identified SERS characteristic features and recognition of the PCA diagnostic capacity, a PCA loadings plot of PC-2 was attained. The variability in these groups of SERS spectra is explained by clustering them separately at PC-2. It is easy to distinguish the low levels of cardiac troponin-I (cTnI) serum spectra of AMI patients from those of healthy serum samples. The loadings plot shows the SERS peaks where there are differences between these SERS spectral data sets. Fig. 2(b) shows the peak positions, which show the variations in peak intensity and show striking differences in the disease serum samples.
 |
| Fig. 2 PCA scatter plot (a) and PCA loadings (b) of SERS spectral data of blood serum samples of healthy and acute myocardial infarction positive patients having low levels of cardiac troponin-I (cTnI). | |
The healthy serum samples contain intense peaks at 486 cm−1 (DNA), 591 cm−1 (amide IV), 629 cm−1 (bending of C–S group), 723 cm−1 (proteins), 882 cm−1 (tyrosine), 1135 cm−1 (NH3+ deformation), 1359 cm−1 (phospholipids) and 1453 cm−1 (C–C ring vibration). However, the acute myocardial infarction-positive serum samples contain intense peaks at 534 cm−1 (cholesterol ester), 697 cm−1 (C–S stretch), 744 cm−1 (DNA/RNA), 835 cm−1 (tyrosine), 927 cm−1 (COO− wagging and stretching mode), 988 cm−1 (amine protein), 1075 cm−1 (stretching vibration (C–N), collagen), 1095 cm−1 (stretching vibration (C–N), collagen), 1221 cm−1 (nucleic acid), 1303 cm−1 (tryptophan), 1403 cm−1 (aromatic group), 1481 cm−1 (amide II), 1541 cm−1 (carbonyl group), 1588 cm−1 (C
O stretch) and 1694 cm−1 (amide I). The stretching or bending vibrations of these groups show that the concentration of these biomolecules increases in the serum having acute myocardial infarction.
The PCA scatter plot of the diseased serum samples having high levels of cardiac troponin-I versus healthy ones is shown in Fig. 3(a). The SERS spectral data of blue clusters show the high disease serum, and the healthy/control serum is associated with red clusters. The PCA loadings plot of PC-1 was obtained for the determination of the significant SERS features from healthy and high disease serum. The SERS spectral variability is demonstrated by PC-1 with 39.07% and PC-2 with 22.75%. The loadings plot associated with high disease serum shown in Fig. 3(b) is higher in intensity at 486 cm−1, 591 cm−1, 629 cm−1, 723 cm−1, 814 cm−1, 882 cm−1, 1135 cm−1, 1359 cm−1 and 1453 cm−1. The AMI high disease serum contains peaks at 534 cm−1, 697 cm−1, 835 cm−1, 927 cm−1, 941 cm−1, 988 cm−1, 1075 cm−1, 1095 cm−1, 1221 cm−1, 1303 cm−1, 1403 cm−1, 1481 cm−1, 1541 cm−1, 1588 cm−1 and 1694 cm−1. To understand the development of the acute myocardial infarction, the pairwise PCA of SERS spectra from healthy and acute myocardial infarction-positive blood serum samples was carried out. The SERS spectra of these groups of samples are clustered separately according to PC-1, as shown in the PCA scatter plot in Fig. 3(a). To determine the associated biochemical features, PCA loadings are employed, which highlight the characteristic SERS features of specific biomolecules associated with the progression and development of acute myocardial infarction having high levels of cardiac troponin-I, as indicated in the loadings plot of Fig. 3(b).
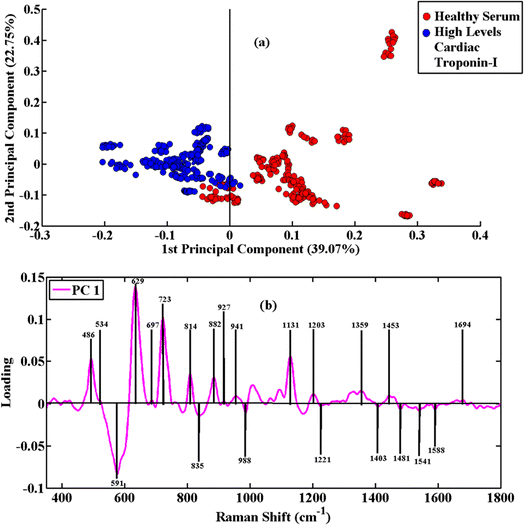 |
| Fig. 3 PCA scatter plot (a) and PCA loadings (b) of SERS spectral data of blood serum samples of healthy and acute myocardial infarction positive patients having low levels of cardiac troponin-I (cTnI). | |
3.2. Partial least squares regression analysis (PLSR)
Using a multivariate calibration method called partial least squares regression (PLSR), the relationship between the levels of the troponin-I and spectral data was established. PLSR maximizes the covariance between the spectral data and reduces the data to a small number of latent variables. The construction of a predictive model is the first step using individual SERS data for the known troponin-I values of 49 acute myocardial infarction serum samples having different levels of cardiac troponin-I (Table S2†). The SERS spectra from these 49 blood serum samples were randomly selected and divided into two sets, including 60% training data and 40% test data sets. Using eight latent variables, the Root Mean Square Error of Cross Validation (RMSECV) is calculated. It is important to avoid over-fitting when the minimal RMSECV is attained. For this reason, just eight latent variables are selected as the optimal number to develop the PLSR prediction model for the levels of troponin-I using SERS spectra of the different blood serum samples of the patients suffering from this disease.
3.2.1. Root mean square error of cross validation (RMSECV). To evaluate the capability of this technique, a PLSR model of the SERS data acquired from different blood serum samples having different troponin-I values in the acute myocardial infarction-positive patients was established, and the troponin-I values were predicted in the blind samples.Fig. 4 shows the calibration vs. validation regression plot, or predicted troponin-I, as a function of the measured troponin-I in this sample, obtained from 8 LVs for the S7 blind sample. The model indicated an RMSEC value of 2.98 ng mL−1 and an RMSEP value of 3.98 ng mL−1 for the provided SERS data set. The (R2) values for the PLSR model, indicating the goodness of the model, were determined to be 0.92 for calibration and 0.87 for the prediction sets. Table 2 shows the predicted troponin-I value for each of the randomly selected five blind samples (S7, S19, S21, and S40), as well as the difference between the predicted and actual troponin-I values. These results indicate the capability of the SERS spectroscopy, along with the chemometric tool, PLSR model, for the diagnosis and quantitative analysis of the troponin-I levels in the acute myocardial infarction-positive patients.
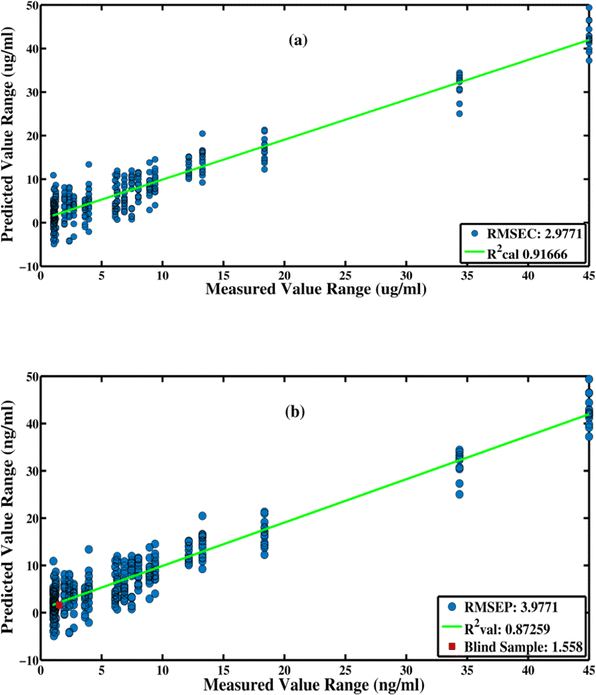 |
| Fig. 4 Regression plots (calibration vs. validation) of the PLSR model for SERS spectral data of different blood serum samples of acute myocardial infarction positive patients having different levels of cardiac troponin-I (cTnI) showing the prediction of the levels of troponin-I in the blind sample (S7). | |
Table 2 Actual and predicted values of troponin-I for blind samples using the PLSR model developed using the SERS spectral data of these blood serum samples
Sample. no. |
Actual value of troponin-I (ng mL−1) |
Predicted value of troponin-I (ng mL−1) |
Deviation from actual value (ng mL−1) |
Goodness of fit (R2) |
S7 |
1.51 |
1.558 |
−0.048 |
0.87259 |
S19 |
9.39 |
9.09 |
0.3 |
0.63123 |
S21 |
13.27 |
13.17 |
0.1 |
0.32442 |
S40 |
0.54 |
0.52 |
0.02 |
0.012221 |
3.2.2. Regression coefficients. PLSR analysis provided regression coefficients, which are displayed in Fig. 5. The features seen here in the positive axis of the Y-axis, which include 534, 744, 835, 927, 1095, and 1541 cm−1, are linked to low and high levels of cardiac troponin-I (cTnI). These features are only seen in the SERS spectra of the low and high levels of cTnI, which are shown in Fig. 1, and have previously been covered in detail. However, there are features found in the negative axis of the Y-axis such as 591, 629, 814, 1131, 1203, 1359, and 1453 cm−1. These features are linked to the SERS features of the healthy/control serum of acute myocardial infarction (AMI), as their intensities gradually increase or decrease from low to high levels of cardiac troponin-I (cTnI) of AMI. PLSR analysis provided the regression coefficients, as shown in Fig. 5. These characteristics, which are found on the positive axis of the Y-axis and include 534, 744, 835, 927, 1095 and 1541 cm−1, are associated with both high and low cardiac troponin-I (cTnI) levels. These characteristics are limited to the low and high levels of cTnI, which are depicted in Fig. 1, and have been thoroughly discussed before in their SERS spectra.
 |
| Fig. 5 Regression coefficients of the PLSR model as compared SERS spectral data of myocardial infarction serum samples with healthy individuals samples. | |
4 Conclusions
The blood serum samples of acute myocardial infarction-positive patients having different levels of cardiac troponin-I (cTnI) and those of healthy serum samples are differentiated by applying the SERS technique with silver nanoparticles as the SERS substrate. By analyzing the differences between the mean spectra of the acute myocardial infarction-positive and healthy blood serum samples, it is found that the composition of the blood serum samples is changed owing to the development of disease. Differences in their biochemical contents are seen in the form of the SERS spectral features, including 697 (C–S stretch), 744 (ring breathing mode of thymine in DNA/RNA), 988 (C–C stretching of protein), 1075 (stretching vibration (C–N), collagen), 1095 (stretching vibration (C–N), D-mannose), 1303 (tryptophan), 1403 (N
N aromatic stretching), 1481 (amide-II), 1541 (amide carbonyl group), 1588 (C
O stretch) and 1694 cm−1 (amide-I). These SERS features clearly differentiate the SERS spectral groups of the acute myocardial infarction-positive patients from healthy ones. PCA and PLSR are statistical tools, which were applied to diagnose and differentiate the SERS spectra of the acute myocardial infarction-positive patients having different levels of cardiac troponin-I from those of the healthy serum samples. Using SERS spectral data from acute myocardial infarction-positive patients having different levels of cardiac troponin-I samples, a PLSR model was established to predict the troponin-I level of unknown/blind samples. The effectiveness of the PLSR model was determined by its goodness of fit (R2) value of 0.87 and root mean square error of cross validation (RMSECV) value of 3.9771. Thus, when combined with PCA and PLSR, the SERS technique offers great potential as a diagnostic and screening tool for acute myocardial infarction-positive patients having different levels of cardiac troponin-I.
Ethical statement
All experiments complied with relevant laws and institutional guidelines and were approved by the Institutional Ethical Review Board (IERB) of Nishtar Medical University Multan, Pakistan, reference number 2230, dated 02-02-24. Informed consent was obtained from all human subjects.
Data availability
All data underlying the results are available as part of the article, and no additional source data are required.
Conflicts of interest
The authors have no known competing financial interests or personal relationships that could have appeared to influence the work reported in this paper.
Acknowledgements
The authors are thankful to the Researcher Supporting Project number (RSPD2024R1035), King Saud University, Riyadh, Saudi Arabia.
References
- K. A. Reimer, R. B. Jennings and A. H. Tatum, Am. J. Cardiol., 1983, 52, 72–81 CrossRef CAS PubMed.
- S. G. Goodman, P. G. Steg, K. A. Eagle, K. A. Fox, J. López-Sendón, G. Montalescot, A. Budaj, B. M. Kennelly, J. M. Gore and J. Allegrone, Am. Heart J., 2006, 151, 654–660 CrossRef PubMed.
- S. Ahmad, M. I. Majeed, H. Nawaz, M. R. Javed, N. Rashid, M. Abubakar, F. Batool, S. Bashir, M. Kashif and S. Ali, Photodiagn. Photodyn. Ther., 2021, 35, 102386 CrossRef CAS PubMed.
- S. Hosseini, P. Vázquez-Villegas, M. Rito-Palomares, S. O. Martinez-Chapa, S. Hosseini, P. Vázquez-Villegas, M. Rito-Palomares and S. O. Martinez-Chapa, Enzyme-Linked Immunosorbent Assay (ELISA) from A to Z, 2018, pp. 67–115 Search PubMed.
- E. J. d. S. Luz, W. R. Schwartz, G. Cámara-Chávez and D. Menotti, Comput. Methods Programs Biomed., 2016, 127, 144–164 CrossRef PubMed.
- P. Xiong, S. M.-Y. Lee and G. Chan, Front. Cardiovasc. Med., 2022, 9, 860032 CrossRef PubMed.
- S. Yusuf, S. Hawken, S. Ôunpuu, T. Dans, A. Avezum, F. Lanas, M. McQueen, A. Budaj, P. Pais and J. Varigos, Lancet, 2004, 364, 937–952 CrossRef PubMed.
- S. S. Anand, S. Islam, A. Rosengren, M. G. Franzosi, K. Steyn, A. H. Yusufali, M. Keltai, R. Diaz, S. Rangarajan and S. Yusuf, Eur. Heart J., 2008, 29, 932–940 CrossRef PubMed.
- M. J. Stampfer, M. R. Malinow, W. C. Willett, L. M. Newcomer, B. Upson, D. Ullmann, P. V. Tishler and C. H. Hennekens, Jama, 1992, 268, 877–881 CrossRef CAS PubMed.
- M. Nielsen, C. Andersson, T. A. Gerds, P. K. Andersen, T. B. Jensen, L. Køber, G. Gislason and C. Torp-Pedersen, Eur. Heart J., 2013, 34, 1198–1203 CrossRef PubMed.
- B. F. H. S. R. Group, Am. J. Hum. Genet., 2005, 77, 1011–1020 CrossRef PubMed.
- S. A. Everson-Rose, T. T. Lewis, K. Karavolos, K. A. Matthews, K. Sutton-Tyrrell and L. H. Powell, Am. Heart J., 2006, 152, 982 CrossRef PubMed.
- E. J. Benjamin, S. S. Virani, C. W. Callaway, A. M. Chamberlain, A. R. Chang, S. Cheng, S. E. Chiuve, M. Cushman, F. N. Delling and R. Deo, Circulation, 2018, 137, e67–e492 CrossRef PubMed.
- N. G. Frangogiannis, Compr. Physiol., 2011, 5, 1841–1875 Search PubMed.
- R. Ullah, S. Khan, Z. Ali, H. Ali, A. Ahmad and I. Ahmed, Photodiagn. Photodyn. Ther., 2022, 39, 102924 CrossRef CAS PubMed.
- X. X. Han, R. S. Rodriguez, C. L. Haynes, Y. Ozaki and B. Zhao, Nat. Rev. Methods Primers, 2021, 1, 87 CrossRef CAS.
- S. Akbar, M. I. Majeed, H. Nawaz, N. Rashid, A. Tariq, W. Hameed, S. Shakeel, G. Dastgir, R. Z. A. Bari and M. Iqbal, Anal. Lett., 2022, 55, 1588–1604 CrossRef CAS.
- S. Nasir, M. I. Majeed, H. Nawaz, N. Rashid, S. Ali, S. Farooq, M. Kashif, S. Rafiq, S. Bano and M. N. Ashraf, Photodiagn. Photodyn. Ther., 2021, 33, 102152 CrossRef CAS PubMed.
- M. Akram, M. I. Majeed, H. Nawaz, N. Rashid, M. R. Javed, M. Z. Ali, A. Raza, M. Shakeel, H. M. ul Hasan and Z. Ali, Photodiagn. Photodyn. Ther., 2022, 40, 103199 CrossRef CAS PubMed.
- K. Thygesen, J. S. Alpert, A. S. Jaffe, B. R. Chaitman, J. J. Bax, D. A. Morrow and H. D. White, Circulation, 2018, 138, e618–e651 CrossRef PubMed.
- S. Mendis, K. Thygesen, K. Kuulasmaa, S. Giampaoli, M. Mähönen, K. Ngu Blackett and L. Lisheng, Int. J. Epidemiol., 2011, 40, 139–146 CrossRef PubMed.
- K. Thygesen, J. S. Alpert, A. S. Jaffe, M. L. Simoons, B. R. Chaitman and H. D. White, Circulation, 2012, 126, 2020–2035 CrossRef PubMed.
- B. Ibanez, S. Halvorsen, M. Roffi, H. Bueno, H. Thiele, P. Vranckx, F.-J. Neumann, S. Windecker and S. James, Eur. Heart J., 2018, 39, 4239–4242 CrossRef PubMed.
- A. Katrukha, A. Bereznikova, V. Filatov and T. Esakova, Clin. Chem. Lab. Med., 1999, 37, 11–12 CrossRef PubMed.
- R. S. Alves, F. A. Sigoli and I. O. Mazali, Nanotechnology, 2020, 31, 505505 CrossRef CAS PubMed.
- X. Fu, Y. Wang, Y. Liu, H. Liu, L. Fu, J. Wen, J. Li, P. Wei and L. Chen, Analyst, 2019, 144, 1582–1589 RSC.
- C. Lin, L. Li, J. Feng, Y. Zhang, X. Lin, H. Guo and R. Li, Microchim. Acta, 2022, 189, 1–8 CrossRef PubMed.
- B. Ibanez, S. James, S. Agewall, M. J. Antunes, C. Bucciarelli-Ducci, H. Bueno, A. L. Caforio, F. Crea, J. A. Goudevenos and S. Halvorsen, Eur. Heart J., 2018, 39, 119–177 CrossRef PubMed.
- M. K. Tuck, D. W. Chan, D. Chia, A. K. Godwin, W. E. Grizzle, K. E. Krueger, W. Rom, M. Sanda, L. Sorbara and S. Stass, J. Proteome Res., 2009, 8, 113–117 CrossRef CAS PubMed.
- H. Li, Q. Wang, J. Tang, N. Gao, X. Yue, F. Zhong, X. Lv, J. Fu, T. Wang and C. Ma, Biosens. Bioelectron., 2021, 189, 113315 CrossRef CAS PubMed.
- M. Tahira, H. Nawaz, M. I. Majeed, N. Rashid, S. Tabbasum, M. Abubakar, S. Ahmad, S. Akbar, S. Bashir and M. Kashif, Photodiagn. Photodyn. Ther., 2021, 34, 102329 CrossRef CAS PubMed.
- Y. Chen, M. Chen, J. Lin, W. Lai, W. Huang, H. Chen and G. Weng, J. Appl. Spectrosc., 2016, 83, 798–804 CrossRef CAS.
- M. E. Benford, M. Wang, J. Kameoka and G. L. Coté, Plasmonics in Biology and Medicine, 2009, vol. 7192 Search PubMed.
- S. Shakeel, H. Nawaz, M. I. Majeed, N. Rashid, M. R. Javed, A. Tariq, B. Majeed, A. Sehar, S. Murtaza and N. Sadaf, Photodiagn. Photodyn. Ther., 2022, 39, 102949 CrossRef CAS PubMed.
- S. Feng, R. Chen, J. Lin, J. Pan, G. Chen, Y. Li, M. Cheng, Z. Huang, J. Chen and H. Zeng, Biosens. Bioelectron., 2010, 25, 2414–2419 CrossRef CAS PubMed.
- S. M. Julien, Org. Geochem., 2022, 174, 104516 CrossRef.
- A. I. Saviñon-Flores, F. Saviñon-Flores, G. Trejo, E. Méndez, Ş. Ţălu, M. A. González-Fuentes and A. Méndez-Albores, Front. Chem., 2022, 10, 1017305 CrossRef PubMed.
- A. L. Ferreira, L. F. de Lima, A. S. Moraes, R. J. Rubira, C. J. Constantino, F. L. Leite, A. O. Delgado-Silva and M. Ferreira, Appl. Surf. Sci., 2021, 554, 149565 CrossRef CAS.
- B. Li, H. Ding, Z. Wang, Z. Liu, X. Cai and H. Yang, Spectrochim. Acta, Part A, 2022, 272, 120997 CrossRef CAS PubMed.
- S. Sánchez-Rojo, B. Martínez-Zérega, E. Velázquez-Pedroza, J. Martínez-Espinosa, L. Torres-González, A. Aguilar-Lemarroy, L. Jave-Suárez, P. Palomares-Anda and J. González-Solís, Rev. Mex. Fis., 2016, 62, 213–218 Search PubMed.
- W. Y. Lim, C.-H. Goh, T. M. Thevarajah, B. T. Goh and S. M. Khor, Biosens. Bioelectron., 2020, 147, 111792 CrossRef CAS PubMed.
- A. Mahadevan-Jansen and R. Richards-Kortum, Annu. Int. Conf. IEEE Eng. Med. Biol. Soc., IEMBS, 1997, vol. 6, DOI:10.1109/IEMBS.1997.756895.
- A. Subaihi, L. Almanqur, H. Muhamadali, N. AlMasoud, D. I. Ellis, D. K. Trivedi, K. A. Hollywood, Y. Xu and R. Goodacre, Anal. Chem., 2016, 88, 10884–10892 CrossRef CAS PubMed.
- G. Cao, M. Chen, Y. Chen, Z. Huang, J. Lin, J. Lin, Z. Xu, S. Wu, W. Huang and G. Weng, Laser Phys. Lett., 2015, 12, 125702 CrossRef.
- A. A. Derda, C. C. Woo, T. Wongsurawat, M. Richards, C. N. Lee, T. Kofidis, V. A. Kuznetsov and V. A. Sorokin, Physiol. Genomics, 2018, 50, 648–657 CrossRef CAS PubMed.
- G. Soufi, E. Dumont, Y. Göksel, R. Slipets, R. A. Raja, K. Schmiegelow, H. Bagheri, A. Boisen and K. Zor, Biosens. Bioelectron.: X, 2023, 14, 100382 CAS.
- M. A. Bakkar, H. Nawaz, M. I. Majeed, A. Naseem, A. Ditta, N. Rashid, S. Ali, J. Bajwa, S. Bashir and S. Ahmad, Spectrochim. Acta, Part A, 2021, 245, 118900 CrossRef CAS PubMed.
|
This journal is © The Royal Society of Chemistry 2024 |