DOI:
10.1039/D4RA03345K
(Paper)
RSC Adv., 2024,
14, 21901-21914
Computational modelling of graphene/aluminum nitride (GP/AlN) hybrid materials for the detection of 2,4 dichlorophenoxyacetic acid (DCP) pollutant
Received
7th May 2024
, Accepted 11th June 2024
First published on 10th July 2024
Abstract
Despite their efficacy in eliminating undesired crops and increasing yield, a range of environmental issues and chronic ailments arise when hazardous chemicals are highly concentrated in wastewater and then deposited into rivers, lakes or the air. Hence, the detection of these chemicals has become a cause of concern for researchers and scientists because they contribute largely to serious health problems. Herein, the potential of newly tailored nanomaterials for the detection of 2,4 dichlorophenoxyacetic acid (DCP) in humans was examined. The theoretical approach adopted in this work is within the framework of density functional theory (DFT) using the DFT/B3LYP-D3/def2SVP computational method. The reduction in the energy gap upon adsorption is indicative of good adsorbing properties. A chemisorption phenomenon was observed for DCP-GP/AlN. However, in most cases, physisorption occurs. Interestingly, the noncovalent nature of the interactions was observed in all the cases, indicating that the material was good. The green colour of the 3D RDG maps implies a significant intermolecular interaction. Sensor mechanisms confirmed that the nanocomposite materials exhibit excellent detection potential for DCP through greater charge transfer, better sensitivity, conductivity, and enhanced adsorption capacity. The potential of nanocomposite materials as stable and promising detectors for DCP pollutants was confirmed in this study. Hence, the studied GP/AlN nanocomposite material can be used in the engineering of future sensor devices for detecting DCP.
1 Introduction
Over the years, various anthropogenic activities have been recorded as nonpoint sources of pollution. In agriculture, the application of pesticides and herbicides poses a risk to human health, and major causes of soil, ground lake and water body contamination can be attributed to the intense consumption of these chemicals, such as 2,4-dichlorophenoxyacetic acid and glyphosate, in the management of poor yields.1–4 Despite the effectiveness of these chemicals in eliminating undesired crops and in turn increasing yield, a range of environmental issues and chronic ailments arise when these chemicals are highly concentrated in wastewater and then deposited into rivers, lakes or the air.5,6 2,4-Dichlorophenol (DCP) is a derivative of phenol with a molecular formula of Cl2C6H3OH. It is crystalline in nature with a melting point of 450 °C and acts as an important precursor in the synthesis of herbicides, which include 2,4-dichlorophenoxyacetic acid (2,4-D) and other chlorinated chemicals.7–9 In research involving zebra fish, DCP tested positive for high toxicity due to its effect on the embryonic developmental process, causing morphological and behavioural defects in the offspring.10 DCP can enter the environment through the decomposition of 2,4-dichlorophenoxyacetic acid in contaminated soil, and DCP can reach water bodies during rainfall.11,12 During volatilization, DCP is introduced into the air and, when inhaled by humans, triggers nasal irritation and other cardiovascular problems.13,14 The effect of phenol-derived herbicides on weeds follows a mechanism of free radical formation, which induces the production of reactive oxygen species (ROS). These free radicals cause structural and cellular deformations of vital plant parts upon interaction with biomolecules such as carbohydrates and proteins.15–17 Phenoloxy chemicals are classified as highly toxic due to their ability to interrupt the cellular and enzymatic processes of various organisms and plants.18,19 Continuous exposure to DCP causes oxidative stress in hepatic tissues, contributing to an increase in kidney and renal problems.20 These observed and documented problems serve as catalysts for modern nanotechnology research, which targets various methods of modifying nanomaterials, such as graphene, for the effective sensing and adsorption of various pollutants, which poses a great risk to plants and humans.
Graphene has been one of the most commonly used carbon materials due to its unique chemical and physical characteristics, with increased hardness and strength compared to those of diamond and steel.21,22 It is made up of a number of carbon atoms arranged in a honeycomb-like shape and is usually single layered.23 One interesting attribute of graphene is its ability to be reinforced in various metal composites when subjected to modifications.24–26 Unlike some carbon nanotubes, graphene has a low density, high thermal conductivity and increased stiffness.27–29 Since the discovery of graphene by Novoselov et al. and the isolation of graphite through the tape stripping method, it has gained attention from various scientists and has been highly considered for application in several fields. Various issues in different fields have sparked the need to improve the features of materials for targeting these problems and developing sustainable solutions. A range of composite materials, such as aluminum–aluminum nitride, graphene–aluminum nitride and many others, have been discovered and are still being explored. Composite materials are composed of two or more constituents, one of which is a reinforcement material and the other of which is a matrix, often called a base material.30,31 The combination of these distinct materials aids in the modification of the behaviours and attributes to cause a dispersion of the material's use in different fields. Due to its outstanding properties of high thermal conductivity, large band gap, electrical sensitivity, shock resistivity, and mechanical strength.32,33 Due to these properties, aluminum nitride has been explored in this research. Over time, AlN has exhibited great potential and is used in the electrical and mechanical industries for the production of semiconductors, mainframes of supercomputers and field emission devices.34–36 It is believed that the potential of AlN has yet to be explored, increasing its potential for various testing and modifications. It has a melting point of 2500 °C or greater and can also exist in solid, liquid or gas states, allowing it to be produced through different nitridation methods.37,38
According to a literature review by Wang et al., cetylpyridium chloride-modified pine sawdust (CPC-PS) exhibited better adsorption of bisphenol A and dichlorophenol (DCP) than PS (pine sawdust). Additionally, kinetic and thermodynamic analyses showed that BPA and DCP adsorption onto CPC-PS fit pseudo-second-order kinetics and the Freundlich model.39 In his work using an activated carbon, pine sawdust pyrolytic carbon (PSPC), to absorb 2,4-DCP and 4-CP, Song et al. reported that the process was spontaneous and that the adsorption capacity of PSAC was significantly reduced during the regeneration process of PSAC with ethanol. Their research introduced a viable method for the adsorption of environmental pollutants.40 Additionally, Lui et al. concluded that a graphene-based hyper crosslinked porous carbon composite (GN/HCPC) showed high adsorption for DCP and for other contaminants, such as tetracycline, phenol and bisphenol A, making the composite a practical and effective adsorbent for the removal of organic contaminants in wastewater.41 In this present research, a composite material was tailored following the combination of graphene and aluminum nitride. Graphene serves as the reinforcement material, and aluminum nitride, the base material, forms a composite material. For novelty, surface modification and exploration of the potential of these materials, the graphene material was doped with the transition metals Fe, Co, and Ni. A total of eight systems, consisting of four surfaces and four complexes, are understudied. Therefore, the objective of this study was to determine the system exhibiting the most suitable sensing potential towards DCP pollutant, in a bid to target the health problems arising from exposure to this pollutant from the inhalation or consumption of contaminated aquatic creatures and water.
2 Computational methodology
For the adsorption of 2,4-dichlorophenoxyacetic acid (DCP) on newly tailored nanocomposite materials, first-principles density functional theory (DFT) was used. The Gaussian 16 program42 and the GaussView 6.0.16 software package43 were used. The DFT/B3LYP-D3/def2SVP computational method was adopted in all calculations. Owing to the presence of heavy metals (Fe, Co, and Ni), the standard Los Alamos National Laboratory, including a double-zeta (LanL2DZ) basis set, was utilized.44 Prior to optimization, all structures were sketched and visualized on Gauss View 6.0.16 software to gain insight into the effects of adsorption on the morphology of the nanocomposite materials. Structural analysis, adsorption energy, quantum descriptor analysis, and topological studies were performed to examine the various adsorption behaviors of the newly tailored materials. The phenomenon of adsorption was observed from the adsorption energy calculated using eqn (1): |
Ead = EComplex − (ESurface + EMolecule)
| (1) |
where the energies of the adsorbate, surface and complex are denoted by EMolecule, ESurface, and EComplex, respectively. The required adsorption energy is denoted by Ead in the equation. The Multiwfn 3.7 program45 was used to calculate the density of states (DOS) and the centre of the d-band, which were then plotted with Origin software.46 The Chemcraft 1.6 program47 was utilized to obtain the HOMO–LUMO plots. The 3D-RDG plot was visualized and generated using the VMD software package.48
3 Results and discussion
3.1 Geometry optimization
The geometry, such as bond lengths, were computed before adsorption to determine the effects of bond length on interactions. A molecule possessing shorter bond lengths is an indication of a strong force of attraction between the bonds, which reduces the reactivity of the systems understudied. On the other hand, weaker bond interactions are understood by longer bonds.49,50 Notably, many properties are determined by the bond length, such as the biological activity, polarity, phase of matter, and reactivity.51 Graphene has a monolayer (single layer) of carbon atoms tightly bonded in a hexagonal honeycomb, and AlN wurzite has a thermodynamically stable crystal structure. The optimized geometries of GP/AlN showed that graphene and wurzite face each other with carbon atoms connected to aluminium (Al) and (N). The bond length of the M–C bond strongly depends on the type of transition metal. Furthermore, there are significant difference in the M–C bond lengths in graphene nanocomposites.
Table 1 shows the computed bond lengths between atoms of different elements (metal–C or Cl–C or N–C or O–C) before and after adsorption. Fig. 1 and 2 show the optimized structures of the studied surfaces and interactions. Fig. 1 shows the optimized structures of the studied surfaces and gases, namely, DCP, GP/AlN, Co@GP/AlN, Fe@GP/AlN, and Ni@GP/AlN. Fig. 2 shows the optimized structures of the studied interactions, namely, DCP-Co@GP/AlN, DCP-GP/AlN, DCP-Fe@GP/AlN, and DCP-Ni@GP/AlN.
Table 1 The computed bond lengths before and after adsorption were calculated via the DFT/B3LYP-D3/6-311G(d,p)/def2SVP computational method
Systems |
Bond labels |
Bond length |
Before adsorption |
After adsorption |
DCP-GP/AIN |
Al41–C55 |
4.089 |
4.088 |
C87–Cl114 |
|
3.429 |
N25–C79 |
3.469 |
3.488 |
O116–C79 |
|
3.676 |
DCP-Co@GP/AIN |
Al42–C80 |
3.535 |
3.529 |
N29–C65 |
3.637 |
3.632 |
Co104–O116 |
2.185 |
Cl115–C63 |
|
3.952 |
DCP-Fe@GP/AIN |
Fe104–N25 |
1.971 |
1.971 |
Al42–C60 |
3.111 |
3.104 |
Cl114–C57 |
|
3.402 |
O120–C55 |
|
3.388 |
DCP-Ni@GP/AIN |
Ni104–N25 |
2.042 |
2.043 |
Al42–C60 |
3.002 |
3.382 |
Cl114–C56 |
|
3.492 |
O116–C85 |
|
3.245 |
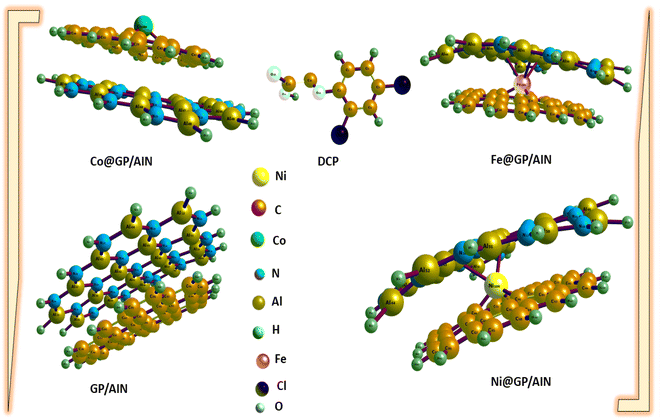 |
| Fig. 1 The optimized structures of the newly tailored nanocomposite materials and the 2,4-dichlorophenoxyacetic acid (DCP). | |
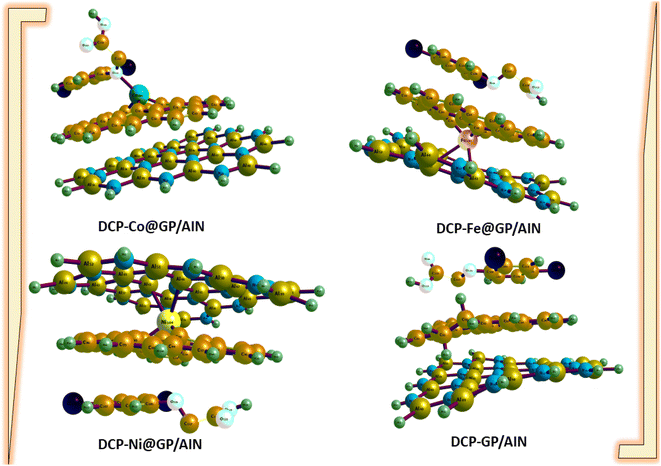 |
| Fig. 2 The optimized structures of the newly tailored nanocomposite materials upon the adsorption of 2,4-dichlorophenoxyacetic acid (DCP). | |
As shown in Table 1, in the DCP-GP/AlN interaction, there was a decrease in the Al41–C55 bond length after adsorption from 4.089 to 4.088 Å and an increase in the N25–C79 bond length from 3.469 to 3.488 Å. In DCP-Co@GP/AlN, there was a decrease in the Al42–C80 bond length from 3.535 to 3.529 Å and in the N29–C65 bond length from 3.637 to 3.632 Å. In DCP-Fe@GP/AlN, there was no change in the Fe104–N25 bond length of 1.971 Å, and there was a decrease in the Al42–C60 bond length from 3.111 to 3.104 Å. In DCP-Ni@GP/AlN, there is an increase in the Ni104–N25 bond length from 2.042 to 2.043 Å and in the Al42–C60 bond length from 3.002 to 3.38 Å.
3.2 Adsorption of 2,4-dichlorophenoxyacetic acid on the tailored nanocomposites
Due to the harmful effect of DCP on humans, the need to detect and adsorb it cannot be resolved. Herein, the molecule was adsorbed on a newly engineered surface tailored by first combining graphene and an aluminum nitride surface. Then, the graphene was modified by adding Fe, Co, and Ni dopants and further combined with the aluminum nitride. Altogether, four different adsorptions were obtained. The best adsorption configurations were ascertained during optimization, and the resulting complexes were confirmed to be in their best conformation. The results obtained from the computations are summarized in Table 2. Upon the adsorption of DCP on the GP/AlN nanocomposite, strong chemisorption occurred, which was attributed to the negative adsorption energy of −16.82 eV in the DCP-GP/AlN complex. However, adsorption on the nanocomposites formed by combining the AlN surface and structurally modified graphene results in weak and physical adsorption, which is best described as physisorption,52 as indicated by the accompanying positive adsorption energies of 15.55, 15.63, and 15.60 eV for DCP-Fe@GP/AlN, DCP-Co@GP/AlN, and DCP-Ni@GP/AlN, respectively (see Table 2). One can infer from the aforementioned results that the GP/AlN nanocomposite strongly adsorbs DCP, which makes it a suitable candidate for use as a sensor for DCP. On the other hand, the weak adsorption associated with the adsorption on the Fe@GP/AlN, Co@GP/AlN, and Ni@GP/AlN surfaces placed these surfaces as detectors for DCP molecules. Since the results obtained in this section are insufficient for determining the suitability of a material as a sensor or detector for 2,4-dichlorophenoxyacetic acid (DCP), further investigations have been carried out to investigate the electronic properties, nature of intermolecular interactions, effect of the d-band center and mechanisms of sensing.
Table 2 Summarized adsorption energies of the complexes calculated via the DFT/B3LYP-D3/6-311G(d,p)/def2SVP computational method
Complex |
EComplex |
ESurface |
EGas |
EAds (Ha) |
EAds (eV) |
DCP-GP/AlN |
−8285.659801 |
−6831.814691 |
−1453.226922 |
−0.61819 |
−16.82 |
DCP-Fe@GP/AlN |
−9509.878038 |
−8057.222579 |
−1453.226922 |
0.571463 |
15.55 |
DCP-Co@GP/AlN |
−9628.901013 |
−8176.248285 |
−1453.226922 |
0.574194 |
15.63 |
DCP-Ni@GP/AlN |
−9754.448608 |
−8301.794786 |
−1453.226922 |
0.5731 |
15.60 |
3.2.1 Toxicity of the materials. The potential toxicity for graphene due to their small size and ability to interact with biological systems. However, the specific toxicity depends on factors like surface, shape, size, functionalization, and exposure route. Aluminum nitride is generally considered to have low inherent toxicity. On the other hand, inhalation of AlN dust may lead to respiratory irritation. The metals dopants (Fe, Co, Ni) can be toxic in high concentrations. However, their specific toxicity depends on the metal, its oxidation state, and exposure route. Finally, the toxicity of the nanocomposite can be determined based on the aforementioned components stated in the following ways: size of particles and surface properties of the nanocomposite; the presence and type of metal dopant; and lastly the GP to AlN ratio.
3.3 Electronic properties
3.3.1 HOMO–LUMO analysis. The information obtained from the HOMO−LUMO study pertains to the alteration in the electronic structural characteristics of the examined surfaces. This is mostly as a result of the disparities in energy between the HOMO and LUMO.53 The energy, strength, and stability of the surfaces and the complex following adsorption can be determined using the difference in energy between the two frontier molecular orbitals.54 Given that orbital hybridization corresponds to the bond formation of the GP/AlN nanocomposite and DCP gas molecule, the frontier molecular orbitals (FMOs) were analysed for pure GP/AlN and their equivalent complexes at their superior positions. Table 3 shows that DCP gas has the highest energy gap among all the studied systems. Among the studied surfaces, Ni@GP/AlN has the lowest energy gap value of 1.773 eV, which shows that it is the most reactive and least stable surface, followed by Co@GP/AlN at 2.247 eV, Fe@GP/AlN at 2.447 eV, and GP/AlN at 2.648 eV, which has the highest energy gap value, which shows that it is the least reactive and most stable surface. Among the studied interactions, DCP-Co@GP/AlN has the lowest energy gap value of 1.979 eV, followed by DCP-GP/AlN at 2.282 eV, DCP-Fe@GP/AlN at 2.335 eV, and DCP-Ni@GP/AlN at the highest energy gap value of 10.073 eV, which shows that it is the most stable and least reactive interaction.55 This result shows that upon adsorption, the rate of reactivity decreased. Table 3 shows the ionization potential (IP), electron affinity (EA), chemical softness (S), chemical hardness (η), electronegativity (X), chemical potential (μ), and electrophilicity index (ω) values of the nine studied systems. These descriptors were computed based on Koopmans's approximation and are presented in eqn (2)–(8). |
Ionization energy (I.E.) = −EHOMO
| (2) |
|
Electron affinity (E.A.) = −ELUMO
| (3) |
|
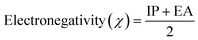 | (4) |
|
 | (5) |
|
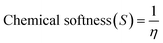 | (6) |
|
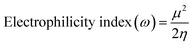 | (7) |
|
Electrochemical potential (μ) = −χ
| (8) |
Table 3 The quantum descriptors were calculated via the DFT/B3LYP-D3/6-311G(d,p)/def2SVP computational method. All units are in electron volts (eV), except for chemical softness (S), which has a unit of eV−1
Systems |
EHOMO |
ELUMO |
Eg |
IP |
EA |
μ |
η |
S |
ω |
EFL |
DCP |
−9.145 |
−0.865 |
8.280 |
9.145 |
0.865 |
5.005 |
4.140 |
0.241 |
3.025 |
−5.005 |
GP/AlN |
−4.887 |
−2.239 |
2.648 |
4.887 |
2.239 |
3.563 |
1.324 |
0.755 |
4.793 |
−3.563 |
Fe@GP/AlN |
−4.900 |
−2.453 |
2.447 |
4.900 |
2.453 |
3.677 |
1.224 |
0.817 |
5.524 |
−3.677 |
Co@GP/AlN |
−5.469 |
−3.222 |
2.247 |
5.469 |
3.222 |
4.345 |
1.124 |
0.889 |
8.402 |
−4.345 |
Ni@GP/AlN |
−4.747 |
−2.974 |
1.773 |
4.747 |
2.974 |
3.861 |
0.886 |
1.129 |
8.406 |
−3.861 |
DCP-GP/AlN |
−4.828 |
−2.546 |
2.282 |
4.828 |
2.546 |
3.687 |
1.141 |
0.876 |
5.957 |
−3.687 |
DCP-Fe@GP/AlN |
−6.253 |
−3.917 |
2.335 |
6.253 |
3.917 |
5.085 |
1.168 |
0.856 |
11.072 |
−5.085 |
DCP-Co@GP/AlN |
−5.216 |
−3.236 |
1.979 |
5.216 |
3.236 |
4.226 |
0.989 |
1.011 |
9.019 |
−4.226 |
DCP-Ni@GP/AlN |
−5.883 |
−4.190 |
1.693 |
5.883 |
−4.190 |
0.846 |
5.036 |
0.198 |
0.071 |
−0.846 |
The ionization potential of the studied surfaces falls within a close range of 4.747–5.469 eV. Also, Co@GP/AlN has the highest ionization potential value of 5.469 eV, whereas the lowest value of 4.747 eV was observed for Ni@GP/AlN. Then, followed by GP/AlN with the value of 4.887 eV, and Fe@GP/AlN with a value of 4.900 eV. Moreover, electron affinity ranges from 2.239 to 3.222 eV, with Co@GP/AlN associated with the highest peak of 3.222 eV, then followed by Ni@GP/AlN with a value of 2.974 eV, and values of 2.453 and 2.239 eV for GP/AlN and Fe@GP/AlN respectively. Fig. 3 and 4 show the HOMO and LUMO plots prior to and upon adsorption, respectively. The plots illustrate the localization of the HOMO and LUMO at sites on the adsorbate and adsorbent.
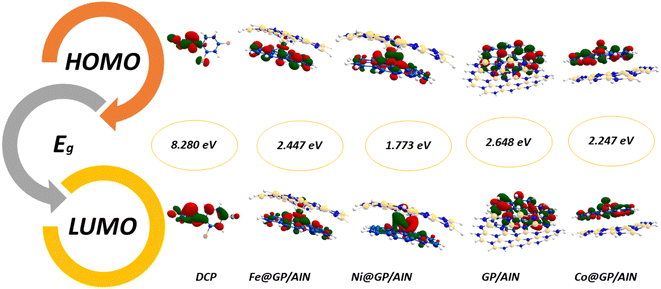 |
| Fig. 3 HOMO and LUMO plots for the nanocomposite materials prior to adsorption. | |
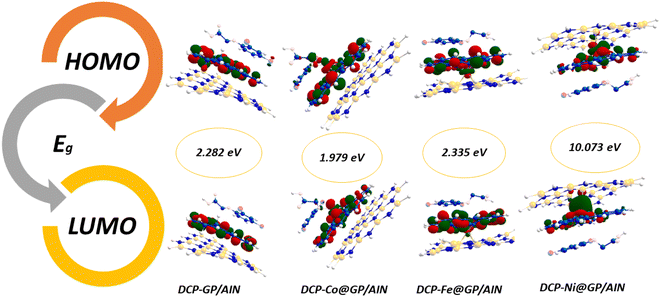 |
| Fig. 4 HOMO and LUMO plots for the nanocomposite materials upon adsorption. | |
3.3.2 NBO analysis. NBO analysis is among the quantum chemical techniques for examining chemical bonds, and the degree of charge transfer between Lewis-type donor orbitals and non-Lewis acceptor orbitals in molecular systems.56 A large value of E2 reflects a stronger inclination from an electron donor to an electron receptor, as a result, a greater degree of conjugation of the entire system.57 They also show a significant connection between the donor orbitals (i) and the receptor orbitals (j). As presented in Table 4, Ni@GP/AlN has the highest stabilization energy of 959.5 kcal mol−1 among the studied surfaces, which shows that it is the most stable surface and can be easily stabilized. This is attributed to the charge transfer resulting from charge delocalization from the antibonding character of the lone pair LP*(1) C70 to the same antibonding character of the lone pair LP*(1) C71. The GP/AlN nanocomposite has the least stabilization energy of 107.6 kcal mol−1, showing that it is the least stable surface among its counterparts. Among the studied interactions, DCP-GP/AlN has the highest stabilization energy of 1284.41 kcal mol−1, which shows that it is the most stable surface upon adsorption, and it is attributed to the charge transfer resulting from charge delocalization from the antibonding character of the lone pair LP*(1) C69 to the same antibonding character of the lone pair LP*(1) C59. Notably, the least stabilization energy was observed in DCP-Ni@GP/AlN upon adsorption. The trend of stabilization energy of the studied interactions follows: DCP-GP/AlN > DCP-Fe@GP/AlN > DCP-Co@GP/AlN > DCP-Ni@GP/AlN.
Table 4 NBO analysis results calculated via the DFT/B3LYP-D3/6-311G(d,p)/def2SVP computational method
System |
Donor |
Acceptor |
E2 (kcal mol−1) |
E(j) − E(i) (a.u.) |
F(i,j) (a.u.) |
DCP |
π* (C2–C3) |
π* (C4–C5) |
394.79 |
0.01 |
0.099 |
GP/AlN |
π* (C82–C83) |
σ* (N22–C92) |
107.60 |
0.01 |
0.065 |
Co@GP/AlN |
LP (4) Co104 |
LP (1) C56 |
692.58 |
0.06 |
0.217 |
Fe@GP/AlN |
LP (1) C72 |
LP* (1) C69 |
910.65 |
0.01 |
0.131 |
Ni@GP/AlN |
LP* (1)C70 |
LP* (1) C71 |
959.50 |
0.01 |
0.148 |
DCP-GP/AlN |
LP (1) C69 |
LP* (1)C59 |
1284.41 |
0.01 |
0.146 |
DCP-Co@GP/AlN |
LP* (1) C97 |
LP (1) C94 |
204.69 |
0.02 |
0.084 |
DCP-Fe@GP/AlN |
LP (1) C85 |
LP* (1) C79 |
1033.17 |
0.02 |
0.161 |
DCP-Ni@GP/AlN |
π* (C109–C110) |
π* (C105–C106) |
139.95 |
0.01 |
0.080 |
3.4 Effect of the d-band center
The d-band center is an important analysis used in gaining knowledge regarding the variation in small-molecule chemisorption strength on transitional metals.58 The vertical line in this instance is a common visual showing the highest occupied molecular orbital (HOMO) level. The lowest level of unoccupied molecular orbitals (LUMOs) is correlated with the center of the total density of states (TDOS). The difference between the center of the TDOS and the vertical line corresponding to the HOMO is the d-band center.59,60 As shown in Table 5, DCP-Co@GP/AlN has the highest d-band center value of −2.228 eV, which shows that there is a high rate of adsorption of DCP gas on the Co@GP/AlN surface, while DCP-Ni@GP/AlN possess the lowest d-band center value of −3.637 eV, which shows that there is a low rate of adsorption of the studied gas on the surface. It can be observed that, the d-band center result is in agreement with the adsorption rate, thereby reflecting the existence of the interaction between the surface and gas. Fig. 5 presents the visualization of the systems under the context of the d-band center effect.
Table 5 The d-band center values of the studied systems calculated via the DFT/B3LYP-D3/6-311G(d,p)/def2SVP computational method
System |
Center of TDOS (eV) |
Center of PDOS (eV) |
Vertical dash line (eV) |
d-Band center (eV) |
DCP-GP/AlN |
−10.102 |
−8.674 |
−7.637 |
−2.465 |
DCP-Co@GP/AlN |
−9.936 |
−8.766 |
−7.708 |
−2.228 |
DCP-Fe@GP/AlN |
−9.948 |
−8.654 |
−7.564 |
−2.384 |
DCP-Ni@GP/AlN |
−9.941 |
−8.562 |
−6.304 |
−3.637 |
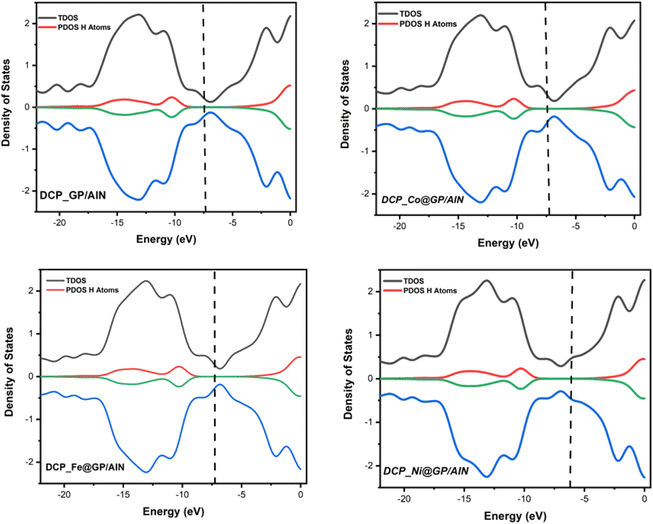 |
| Fig. 5 Visualization of the d-band center plots for the studied systems. | |
3.5 Visual study
3.5.1 QTAIM analysis. As posited by Bader et al., the quantum theory of atoms-in-molecules investigates the critical position of bonds to explore the nature of interactions in the systems under study.61 The QTAIM encompasses the intricacies of interaction nature and, similarly, explains other parameters for an accurate and in-depth study. The structural properties alone may not sufficiently depict the behavioral aspects of the molecules and the distinct features of the interactions. These variations in the bond at different critical bond points contribute to the uniqueness observed in structural and electronic properties, etc.62 Additionally, the charge density extrema can be evaluated through QTAIM analysis. Multiwfn software was utilized to effectively visualize these bonds and determine various parameters in this topological analysis. The calculated electron density, Lagrangian kinetic energy G(r), energy density, H(r), G(r)/V(r), etc., are summarized in Table 6. The nature of interactions can be categorized into covalent, noncovalent and partial covalent bonds and can be observed by the values of the Laplacian of electron density ∇2ρ(r) and H(r). According to previous literature, partial covalent, covalent and noncovalent interactions occur when ∇2ρ(r) > 0 and H(r) < 0, ∇2ρ(r) < 0 and H(r) > 0 and ∇2ρ(r) > 0 and H(r) > 0, respectively.63,64
Table 6 The topological parameters calculated via the DFT/B3LYP-D3/6-311G(d,p)/def2SVP computational methoda
System |
Bonds |
CPs |
P(r) |
∇2ρ(r) |
G(r) |
V(r) |
H(r) |
ELF |
LOL |
G(r)/V(r) |
λ1 |
λ2 |
λ3 |
λ1/λ3 |
E |
The units of the parameters in Table 5 are as follows: P(r), e/Ang3; ∇2ρ(r), e/Ang3; λ1, λ2, and λ3 have a unit of e/Ang5 respectively; G(r) and V(r) have units of eV; H(r) has a unit of eV Ang3/e; ellipticity of electron density (ε), electron localization function (ELF), G(r)/V(r) and λ1/λ3 are dimensionless. |
DCP@GP/AlN |
C82–N28 |
177 |
0.369 |
0.111 |
0.203 |
−0.130 |
0.739 |
0.150 |
0.110 |
−1.562 |
−0.197 |
−0.519 |
0.136 |
−1.449 |
2.797 |
C72–Al36 |
341 |
0.628 |
0.149 |
0.306 |
−0.240 |
0.656 |
0.385 |
0.167 |
−1.275 |
−0.230 |
−0.882 |
0.180 |
−1.278 |
1.607 |
Cl115–H123 |
131 |
0.477 |
0.171 |
0.326 |
−0.226 |
0.101 |
0.138 |
0.106 |
−1.442 |
−0.271 |
−0.374 |
0.236 |
−1.148 |
0.379 |
DCP-Fe@GP/AlN |
H76–N28 |
158 |
0.417 |
0.135 |
0.250 |
−0.162 |
0.882 |
0.150 |
0.110 |
−1.543 |
−0.953 |
−0.182 |
0.163 |
−5.847 |
0.911 |
O116–C60 |
210 |
0.531 |
0.222 |
0.409 |
−0.262 |
0.147 |
0.127 |
0.102 |
−1.561 |
−0.182 |
−0.371 |
0.278 |
−0.655 |
1.036 |
Cl115–C 105 |
192 |
0.571 |
0.178 |
0.369 |
−0.293 |
0.764 |
0.196 |
0.124 |
−1.259 |
−0.243 |
−0.324 |
0.235 |
−1.034 |
0.335 |
DCP-Co@GP/AlN |
N118–H102 |
218 |
0.336 |
0.116 |
0.204 |
−0.117 |
0.872 |
0.110 |
0.960 |
−1.743 |
0.141 |
−0.178 |
−0.703 |
−0.201 |
1.526 |
N119–C95 |
247 |
0.453 |
0.154 |
0.289 |
−0.193 |
0.961 |
0.149 |
0.110 |
−1.497 |
−0.134 |
−0.208 |
0.188 |
−0.713 |
0.561 |
Co104–Cl114 |
280 |
0.154 |
0.227 |
0.819 |
−0.107 |
−0.252 |
0.993 |
0.249 |
−7.654 |
−0.928 |
0.376 |
−0.563 |
1.648 |
0.648 |
DCP-Ni@GP/AlN |
C81–Al44 |
211 |
0.625 |
0.141 |
0.307 |
−0.262 |
0.453 |
0.374 |
0.165 |
−1.172 |
−0.176 |
−0.233 |
0.182 |
−0.967 |
0.325 |
N22–N18 |
139 |
0.497 |
0.151 |
0.290 |
−0.201 |
0.884 |
0.199 |
0.125 |
−1.443 |
−0.149 |
−0.274 |
0.194 |
−0.768 |
0.842 |
Cl115–H113 |
248 |
0.646 |
0.194 |
0.411 |
−0.336 |
0.749 |
0.238 |
0.135 |
−1.223 |
−0.284 |
−0.390 |
0.262 |
−1.084 |
0.374 |
In this study, all the complexes exhibited noncovalent interactions except for the Co104–Cl114 bond in the DCP-Co@GP/AlN complex, which is partially covalent. The P(r) and ∇2ρ(r) values confirm the existence of a noncovalent nature of the interaction since all the values are greater than zero (>0). Consequently, the λ1/λ3 values for the complexes DCP@GP/AlN, DCP-Fe@GP/AlN, DCP-Co@GP/AlN and DCP-Ni@GP/AlN, as reported in Table 6, are all less than that of the H76–N28 bond in DCP-Fe@GP/AlN, which has a smaller negative value of −5.847 a.u. and indicates the strong presence of intermolecular interactions across all the complexes. Furthermore, a great degree of stability in the interaction and charge distribution is confirmed by the domination of the small values of bond ellipticity. Additionally, a previous literature review established that when the G(r)/V(r) values are greater than one, a covalent interaction occurs, and partial covalent and noncovalent interactions occur when the values are between 1 and 0.5 and less than 0.5, respectively.65,66 The G(r)/V(r) further confirms the presence of noncovalent interactions since all values are less than one. According to the analysed parameters, all the complexes exhibit a degree of stability and a noncovalent nature of interaction, which is required for outstanding sensor performance.
3.5.2 3D RDG plots. Understanding the concept surrounding the 3D isosurface of systems is made possible by studying noncovalent interactions, which is often referred to as reduced density gradient (RDG) analysis. This analysis explores the interaction within and between the adsorbate and the adsorbent in a study. Its application spans various fields, such as medicinal chemistry and computational studies.67 These interactions do not promote the transfer of electrons but follow a colouration pattern. In previous studies, various forces are represented in a colour range, which are shown as scattered patches or lobes in some cases around the isosurface.68 NCI analysis focuses on the study of weak interactions, the van der Waals force of attraction, which is confirmed when green-colored lobes or patches are found around the interaction area of a complex. The blue-colored region depicts the strong presence of hydrogen bonding, and the red areas indicate the existence of steric interactions. These interactions affect the behaviors of the individual complexes, causing variation across them upon interaction.69,70 Fig. 6 shows all the computationally visualized isosurfaces of the studied complexes. As represented in the figure, all complexes are observed to have a large green isosurface scattered around the composite material area and the pollutant area, implying significant intermolecular interactions. The DCP@GP/AlN complex exhibited only van der Waals forces of attraction, and the DCP-Fe@GP/AlN, DCP-Co@GP/AlN and DCP-Ni@GP/AlN complexes were dominated by green iso-surfaces and a slight red colour around some regions, indicating the presence of strong van der Waals forces between the molecules of the adsorbate and adsorbent and slight steric forces within the composite material. The observed trends in the isosurface indicate that the DCP@GP/AlN complex is most suitable and possesses a very weak interaction, although other complexes show great potential in the sensing and adsorption of the 2,4-dichlorophenoxyacetic acid (DCP) pollutant, which is a precursor in the formation of herbicides and pesticides.
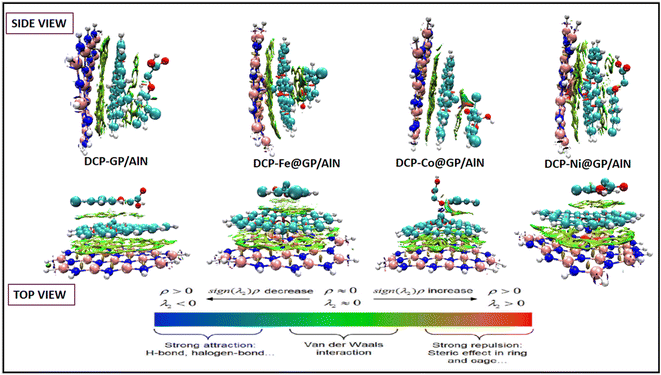 |
| Fig. 6 3D-RDG plots of the complexes in two views, namely, the side view and top view. | |
3.6 Sensing mechanisms
3.6.1 Work function and electrical conductivity. According to the Kevin probe,71 when the work function of a semiconductor changes, it affects the electron density of that material; as a result of the instability of the emission properties, an electrical signal is produced. The greatest changes in the work functions of −0.7808 and 0.3831 were observed for the Ni@GP/AlN and Fe@GP/AlN materials, respectively, indicating greater changes in the electron density of the materials and greater electrical signals, as provided by the Kevin probe. The high work functions of 5.09, 4.23, and 3.69 eV are attributed to DCP-Fe@GP/AlN, DCP-Co@GP/AlN, and DCP-GP/AlN, respectively (see Table 7). It is noteworthy to mention that greater work function indicates high performance of a material comparatively. Hence, this attribute is an indicator of a material suitable for designing detector and sensor devices.
Table 7 Sensor mechanisms calculated by DFT/B3LYP-D3/6-311G(d,p)/def2SVP
Systems |
ϕ (eV) |
Δϕ (eV) |
Qt (e) |
ΔN |
ΔE-back-donation (eV) |
% ΔEg |
DCP-GP/AlN |
3.69 |
0.0349 |
0.220 |
0.011387 |
−0.28528 |
−13.83 |
DCP-Fe@GP/AlN |
5.09 |
0.3831 |
1.149 |
0.039285 |
−0.29194 |
−4.56 |
DCP-Co@GP/AlN |
4.23 |
−0.0276 |
0.995 |
−0.00802 |
−0.24749 |
−11.90 |
DCP-Ni@GP/AlN |
0.85 |
−0.7808 |
1.125 |
6.254571 |
−1.25911 |
−4.52 |
The conduction of electricity is an attribute of a good sensor material. The energy gap can be related to the electrical conductivity of a material via eqn (9) in such a way that a higher energy gap results in a higher conductivity and vice versa.72–74 Theoretically, electrical conductivity is calculated using eqn (9), where the constant, temperature, energy gap, and Boltzmann constant are denoted by K, T, Eg, and B, respectively.
The percentage changes in the energy gap, % ΔEg, computed are summarized in Table 7, wherein a decrease in the energy gap was observed upon adsorption (indicator of increased reactivity). The greatest percentage change in the Eg of DCP-GP/AlN and DCP-Co@GP/AlN were −13.83 and −11.90%, respectively. Similarly, DCP-Fe@GP/AlN and DCP-Ni@GP/AlN showed similar percentage changes of −4.56 and −4.52, respectively (see Table 7). A greater negative percentage is an indication of increased reactivity of the system. As provided in Subsection 3.3.1 of the quantum descriptors, the increasing order of the energy gap is an increasing pattern of conductivity, which follows the order DCP-Ni@GP/AlN < DCP-Co@GP/AlN < DCP-Fe@GP/AlN < DCP-GP/AlN. This can be understood so that the least and greatest conductivity are found in the adsorption of DCP on Ni@GP/AlN and GP/AlN surfaces respectively. In furtherance, the GP/AlN surface without doping modification happened to have the least reactivity and most conducting. Lastly, since the energy gap falls within a close range of 1.693 to 2.335 eV, the electrical conductivities among the investigated surfaces are relatively close.75
3.6.2 Backdonation and FET patterns. Based on Pearson theory,76 electrical back-donation can be defined using a mathematical formula in such a way that chemical hardness is used therein. The eqn (10) shows the expression of electrical back donation in terms of chemical hardness. |
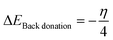 | (10) |
Based on literature reviewed, negative values of ΔEBack donation are indicative of good material. That is, those showcasing higher negative magnitude possesses an inherent adsorbing property that grants such material the gateway to be used in coupling a sensor device.77 As observed from the table, all studied systems are attributed to negative back-donation, with the highest negative value of −1.25911 eV for DCP-Ni@GP/AlN, indicating good material performance. Other studied systems are within a close range of −0.29194 to −0.24749 eV, depicting a relatively good sensing property. The electron mobility from the nanocomposite material to the DCP molecule can be elucidated using the fraction of electron transfer (FET).78 The Peason theory is used to calculate the electronegativity (χ) and chemical hardness (η) of FETs. The FET can be computed via eqn (11).
|
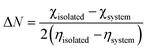 | (11) |
Thorough literature survey shows that lower η value is attributed to greater ΔN value, which in turn causes an increase in FET upon adsorption.79 A high FET indicates the movement of electrons from the nanocomposite materials to the DCP molecule.80 The highest FET value of 6.254571 is attributed to DCP-Ni@GP/AlN. Additionally, relatively high FETs of 0.011387 and 0.039285 corresponding to DCP-GP/AlN and DCP-Fe@GP/AlN, showcased good adsorption behaviour.
3.6.3 Effect of charge transfer, QNBO. The use of natural charge on an adsorbent material and adsorbate is an important concept in determining the charge transfer within a system. Generally, a positive magnitude of QNBO indicates charge transfer from the nanocomposite to the DCP molecule, whereas a transfer from the DCP molecule to the nanocomposite can be elucidated from the negative QNBO.81,82 Using the mathematical relation in eqn (12), the charge transfer can be calculated. |
QNBO = Qadsorption − Qisolated
| (12) |
Owing to the positive charge transfer observed in all cases, electrons are transferred from the nanocomposite to the DCP molecule. This scenario elucidates the presence of an excellent adsorbing materials.83
3.7 Computational insights and future directions
The theoretical investigation carried out in this study will help in the future experiments through screening new materials, optimizing adsorbent design, and tailoring the surface properties. Due to large library of potential materials, DFT model can be employed virtually to screen this large library. In the long run, the DFT investigation will guide in selecting the most promising candidates for further experimental study, while saving time and resources. Additionally, the optimization of the structure and composition of the adsorbent at the atomic level can be achieved by using the newly modelled materials. In line with this, one can simulate different configurations of metal dopants within the GP/AlN nanocomposite framework in order to identify the most effective arrangement for the adsorption of DCP. Lastly, this theoretical investigation creates room for the ease and flexibility of tailoring surface properties by analyzing the interaction between the DCP and the model adsorbent. With this, one can gain useful insight into the underlying mechanisms of adsorption. Conclusively, the knowledge gained in this computational inquiry can be a guide when tailoring the surface properties of the adsorbent for even stronger and highly selective detection of DCP.
The feasibility in the synthesis of these materials can be accounted for by firstly putting into consideration, how the knowledge gained can be of use in the experimental. One among many others is the identification of active sites, where specific sites on the adsorbent materials with the most favourable for the adsorption of DCP can be pinpointed by the model. This aspect helps in experimental characterization practices on the active sites, thereby creating a deeper understanding of the adsorption process. Experimental observations like adsorption energy can be interpreted using this theoretical inquiry to better understand adsorption mechanism at the molecular level. Lastly, from the loaded adsorbent, desorption process of the adsorbate can be aided by this model, providing insights into the regeneration conditions usually required for efficient reusability of the adsorbent.
It is important to note that, as this model is a simplification of reality, the need for experimental validation cannot be jettisoned. This knowledge can be used to design experiments with techniques like scanning electron microscopy (SEM) or X-ray photoelectron spectroscopy (XPS) to focus on these active sites and validate the model's predictions. This information can also guide further experiments like temperature-programmed desorption (TPD) to measure the energy required to desorb DCP and validate the predicted mechanism, and many among others.
4 Conclusions
The intricacies of DCP molecule adsorption on newly tailored nanocomposite materials were explored in the present study. Using density functional theory, this study provides new insight into the adsorption potential of GP/AlN, Fe@GP/AlN, Co@GP/AlN, and Ni@GP/AlN nanocomposite surfaces for the detection of 2,4-dichlorophenoxyacetic acid (DCP) in humans. Knowledge on the chemistry of adsorption has been gained using various theoretical analyses, such as structural analysis, FMO and NBO analyses, determination of the effect of the d-band center, QTAIM and 3D RDG analyses, adsorption studies, and sensor mechanism studies, among others. The following conclusions are drawn:
I. As adsorption occurred, changes in the morphology of the nanocomposite materials were observed, as indicated by the contraction and stretching of bond lengths.
II. The phenomena of adsorption observed in most cases are physisorption and chemisorption for DCP-GP/AlN. This result demonstrated the suitability of the nanocomposite materials as detectors for DCM.
III. In all cases, the energy gap decreased upon adsorption, which is indicative of good sensor materials. During post-adsorption, the energy gap values were computed to be within a relatively close range of 1.693 to 2.336 eV.
IV. According to the QTAIM analysis, all the complexes exhibit a certain degree of stability and noncovalent interactions, which are required for outstanding sensor performance.
V. The green colour of the 3D RDG maps implies a significant intermolecular interaction. The DCP@GP/AlN complex exhibits only van der Waals forces of attraction, and the DCP-Fe@GP/AlN, DCP-Co@GP/AlN and DCP-Ni@GP/AlN complexes are dominated by green iso-surfaces, and a slight red colour appears around some regions, indicating the presence of strong van der Waals forces.
VI. Sensor mechanisms affirmed that the nanocomposite materials exhibit excellent detection potential for DCP through greater charge transfer, better sensitivity, conductivity, and enhanced adsorption capacity.
Data availability
All data are contained within the manuscript.
Author contributions
Chioma G. Apebende: project conceptualization, design and supervision. Ismail O. Amodu: methodology, validation, investigation, analysis. Miracle N. Ogbogu: writing, editing and data curation; Monsurat Alarape Raimi: review, editing and manuscript final draft; and Godwin O. Igomah: validation, methodology, reading, and editing.
Conflicts of interest
The authors declare no financial or competing interests.
References
- T. C. Abrantes, A. R. S. Queiroz, F. R. Lucio, C. W. Mendes Junior, T. M. Kuplich, C. Bredemeier and A. Merotto Junior, The effects of dicamba and 2,4-dichlorophenoxyacetic acid (2,4D) on soybean plants were assessed through vegetation indices derived from unmanned aerial vehicle (UAV)-based RGB imagery, Int. J. Remote Sens., 2021, 42(7), 2740–2758 CrossRef
. - R. Avila Neto, A. A. Melo, A. D. R. Ulguim, R. M. Pedroso, G. F. Barbieri, E. F. Luchese and E. M. Leichtweiss, Mixtures of 2,4-D and dicamba with other pesticides and their influence on application parameters, Int. J. Pest Manage., 2021, 1–10 Search PubMed
. - N. P. Malindzisa, Evaluation of Glyphosate and 2,4-Dichlorophenoxyacetic Acid Combinations to Control Glyphosate-Resistant Hairy Fleabane [Conyza Bonariensis (L.) Cronquist], Master's thesis, University of Pretoria, 2022
. - D. K. Miller, T. M. Batts, J. T. Copes and D. C. Blouin, Reduced rates of glyphosate in combination with 2,4-D and dicamba impact sweet potato yield, HortTechnology, 2020, 30(3), 385–390 CAS
. - J. B. Muhammad, A. H. Jagaba, F. Yusuf, S. Usman, N. S. Yakubu, A. H. Birniwa and D. Shehu, Achromobacter xylosoxidans bacteria isolated from contaminated agricultural environment for a sustainable 2,4-dichlorophenoxyacetic acid herbicide degradation: an experimental study, Case Stud. Chem. Environ. Eng., 2024, 9, 100604 CrossRef CAS
. - A. Samanth, R. Vinayagam, G. Murugesan, T. Varadavenkatesan, R. Selvaraj and A. Pugazhendhi, Enhanced adsorption of 2,4-dichlorophenoxyacetic acid using low-temperature carbonized Peltophorum pterocarpum pods and its statistical physics modelling, Chemosphere, 2023, 336, 139143 CrossRef CAS PubMed
. - A. Shoneye, Photocatalytic Mineralization of Toxic Chlorophenols and Removal of Cr(VI) in Aqueous Solution Using Cocatalyst Decorated TiO2, Doctoral dissertation, UCL (University College London), 2021
. - Y. Xu, N. Qin, Z. Liu, H. Han, S. Y. Tee, G. Guan and M. Y. Han, Functionally Imprinted Orthorhombic WO3 H2O Nanoplates for Ultrasensitive Photoelectrochemical Sensing with Excellent Selectivity, ACS Mater. Lett., 2022, 4(11), 2394–2400 CrossRef CAS
. - Y. Han, M. Tai, Y. Yao, J. Li, Y. Wu, B. Hu and C. Liu, Iron-decorated covalent organic framework as efficient catalyst for activating peroxydisulfate to degrade 2,4-dichlorophenol: Performance and mechanism insight, J. Colloid Interface Sci., 2024, 663, 238–250 CrossRef CAS
. - J. S. Lee, J. S. Lee and H. S. Kim, Toxic effects of triclosan in aquatic organisms: a review focusing on single and combined exposure to environmental conditions and pollutants, Sci. Total Environ., 2024, 170902 CrossRef CAS PubMed
. - A. Kumari, S. Rajput, S. V. Singh, G. Sharma, A. Zhumbei, V. D. Rajput and R. Kaur, New Dimensions into the Removal of Pesticides Using an Innovative Ecofriendly Technique: Nanoremediation, in Nano-Bioremediation for Water and Soil Treatment, Apple Academic Press, 2024, pp. 211–235 Search PubMed
. - Z. Ji, W. Huang, T. Liu, W. Yang and W. Xu, A novel molecularly imprinted photoelectrochemical sensor utilizing Ag/SnO2 for the highly sensitive and selective detection of 2,4-dichlorophenoxyacetic acid, Microchem. J., 2024, 110278 CrossRef CAS
. - Environmental Contaminants: Impact, Assessment, and Remediation, ed. Ganguly, P., Mandal, J., Paramsivam, M. and Patra, S., CRC Press, 2024 Search PubMed
. - M. Yadav, N. K. Singh, N. K. Singh, T. Jagwani and S. Yadav, Pesticides and fertilizers contamination of groundwater: health effects, treatment approaches, and legal aspects, in Contaminants of Emerging Concerns and Reigning Removal Technologies, CRC Press, 2022, pp. 349–376 Search PubMed
. - H. B. Habeeb Rahuman, R. Dhandapani, S. Narayanan, V. Palanivel, R. Paramasivam, R. Subbarayalu and S. Muthupandian, Medicinal plants mediated the green synthesis of silver nanoparticles and their biomedical applications, IET Nanobiotechnol., 2022, 16(4), 115–144 CrossRef PubMed
. - A. Bano, A. Gupta, S. Rai, T. Fatima, S. Sharma and N. Pathak, Mechanistic role of reactive oxygen species and its regulation via the antioxidant system under environmental stress, Plant stress physiology—perspectives in agriculture, 2021, pp. 1–18 Search PubMed
. - A. K. Gautam, P. K. Singh and M. Aravind, Defensive role of plant phenolics against pathogenic microbes for sustainable agriculture, Plant Phenolics in Sustainable Agriculture, 2020, vol. 1, pp. 579–594 Search PubMed
. - N. Panigrahy, A. Priyadarshini, M. M. Sahoo, A. K. Verma, A. Daverey and N. K. Sahoo, A comprehensive review on eco-toxicity and biodegradation of phenolics: Recent progress and future outlook, Environ. Technol. Innovation, 2022, 27, 102423 CrossRef CAS
. - A. Kamal, F. Ahmad and M. A. Shafeeque, Toxicity of pesticides to plants and nontarget organism: A comprehensive review, Iran. J. Plant Physiol., 2020, 10(4), 3299–3313 Search PubMed
. - S. Ince, H. H. Demirel, F. Zemheri-Navruz, D. Arslan-Acaroz, I. Kucukkurt, U. Acaroz and E. N. Demirkapi, Synergistic toxicity of ethanol and 2,4-dichlorophenoxyacetic acid enhances oxidant status, DNA damage, inflammation, and apoptosis in rats, Environ. Sci. Pollut. Res., 2023, 30(4), 10710–10723 CrossRef CAS
. - A. Verma, R. Bandyopadhyay, C. S. Tiwary, B. K. Das and J. Bhattacharya, Engineering defect concentration and morphology of green graphene for the development of hard and anticorrosive coating on carbon steel, Corros. Sci., 2023, 224, 111523 CrossRef CAS
. - S. Hassan, A. Y. Nadeem, H. Qaiser, A. S. Kashif, A. Ahmed, K. Khan and A. Altaf, A review of carbon-based materials and their coating techniques for biomedical implants applications, Carbon Letters, 2023, 33(4), 1171–1188 CrossRef
. - N. Baig, Two-dimensional nanomaterials: a critical review of recent progress, properties, applications, and future directions, Composites, Part A, 2023, 165, 107362 CrossRef CAS
. - W. Han, J. Zhou and Q. Shi, Research progress on enhancement mechanism and mechanical properties of FRP composites reinforced with graphene and carbon nanotubes, Alexandria Eng. J., 2023, 64, 541–579 CrossRef
. - Y. Hua, F. Li, N. Hu and S. Y. Fu, Frictional characteristics of graphene oxide-modified continuous glass fiber reinforced epoxy composite, Compos. Sci. Technol., 2022, 223, 109446 CrossRef CAS
. - K. V. Balaji, K. Shirvanimoghaddam and M. Naebe, Multifunctional basalt fibre polymer composites enabled by carbon nanotubes and graphene, Composites, Part B, 2024, 268, 111070 CrossRef CAS
. - B. Merillas, T. E. G. Álvarez-Arenas, F. Villafañe and M. Á. Rodríguez-Pérez, Reaching a near zero radiative heat transfer by the inclusion of modified multiwalled-carbon nanotubes (MWCNTs) in polyurethane-polyisocyanurate aerogels, Mater. Today, 2023, 34, 101789 CAS
. - Y. Lv, T. Yang, X. Hou, Z. Fang, K. Rajan, Y. Di and T. Liang, Zirconia nanofibers-loaded reduced graphene oxide fabrication for specific electrochemical detection of methyl parathion, J. Alloys Compd., 2022, 904, 163798 CrossRef
. - A. Subasi, S. Subasi, M. Bayram, A. Sarı, G. Hekimoğlu, A. Ustaoglu and T. Ozbakkaloglu, Effect of carbon nanotube and microencapsulated phase change material utilization on the thermal energy storage performance in UV cured (photoinitiated) unsaturated polyester composites, J. Energy Storage, 2023, 61, 106780 CrossRef
. - M. Bhong, T. K. Khan, K. Devade, B. V. Krishna, S. Sura, H. K. Eftikhaar and N. Gupta, Review of composite materials and applications, Mater. Today: Proc., 2023 DOI:10.1016/j.matpr.2023.10.026
. - G. Luo, J. Xie, J. Liu, Y. Luo, M. Li, Z. Li and Z. Jiang, Highly stretchable, knitable, wearable fiberform hydrovoltaic generators driven by water transpiration for portable self-power supply and self-powered strain sensor, Small, 2024, 20(12), 2306318 CrossRef CAS PubMed
. - G. Chakraborty, R. Padmashree and A. Prasad, Recent advancement of surface modification techniques of 2-D nanomaterials, Mater. Sci. Eng., C, 2023, 297, 116817 CrossRef CAS
. - D. Meher, S. Acharya and B. P. Sahoo, Influence of reduced graphene oxide content on dielectric, optical energy band gap, and I–V characteristics of PVDF/PANI/RGO nanocomposites, J. Mater. Sci.: Mater. Electron., 2023, 34(31), 2064 CrossRef CAS
. - Y. Xue, Y. Zheng, E. Wang, T. Yang, H. Wang and X. Hou, Ti3C2Tx (MXene)/Pt nanoparticle electrode for the accurate detection of DA coexisting with AA and UA, Dalton Trans., 2022, 51(11), 4549–4559 RSC
. - S. T. Haider, M. A. Shah, D. G. Lee and S. Hur, A review of the recent applications of aluminum nitride-based piezoelectric devices, Ieee Access, 2023, 11, 58779–58795 Search PubMed
. - D. Jia, T. Yang, K. Wang, L. Zhou, E. Wang, K. C. Chou and X. Hou, Facile in-situ synthesis of Ti3C2Tx/TiO2 nanowires toward simultaneous determination of ascorbic acid, dopamine and uric acid, J. Alloys Compd., 2024, 173392 CrossRef CAS
. - M. Chhattal, A. Rosenkranz, S. Zaki, K. Ren, A. Ghaffar, Z. Gong and P. G. Grützmacher, Unveiling the tribological potential of MXenes-current understanding and future perspectives, Adv. Colloid Interface Sci., 2023, 103021 CrossRef CAS PubMed
. - Z. Wang, X. Wang, S. Wang, J. He, T. Zhang, J. Wang and G. Wu, Simultaneously, enhanced thermal conductivity and dielectric breakdown strength in sandwich AlN/epoxy composites, Nanomaterials, 2021, 11(8), 1898 CrossRef CAS PubMed
. - H. Wang, T. Tian, D. Wang, F. Xu and W. Ren, Adsorption of bisphenol A and 2,4-dichlorophenol onto cetylpyridinium chloride-modified pine sawdust: a kinetic and thermodynamic study, Environ. Sci. Pollut. Res., 2022, 1–12 Search PubMed
. - Y. Liu, B. Men, A. Hu, Q. You, G. Liao and D. Wang, Facile synthesis of graphene-based hypercross-linked porous carbon composite with superior adsorption capability for chlorophenols, J. Environ. Sci., 2020, 90, 395–407 CrossRef CAS PubMed
. - Y. Song, Y. Wang and R. Han, Adsorption of chlorophenols on activated pine sawdust-activated carbon from solution in batch mode, Environ. Sci. Pollut. Res., 2023, 30(11), 31294–31308 CrossRef CAS PubMed
. - M. J. Frisch, G. W. Trucks, H. B. Schlegel, G. E. Scuseria, M. A. Robb, J. R. Cheeseman, G. Scalmani, V. Barone, G. A. Petersson, H. Nakatsuji, X. Li, M. Caricato, A. V. Marenich, J. Bloino, B. G. Janesko, R. Gomperts, B. Mennucci, H. P. Hratchian, J. V. Ortiz, A. F. Izmaylov, J. L. Sonnenberg, D. Williams-Young, F. Ding, F. Lipparini, F. Egidi, J. Goings, B. Peng, A. Petrone, T. Henderson, D. Ranasinghe, V. G. Zakrzewski, J. Gao, N. Rega, G. Zheng, W. Liang, M. Hada, M. Ehara, K. Toyota, R. Fukuda, J. Hasegawa, M. Ishida and T. Nakajima, Gaussian 16, Revision C 01 Search PubMed
. - T. A. Keith and J. M. Millam, GaussView, Version 6.1, Roy Dennington, Semichem Inc., Shawnee Mission, KS, 2016 Search PubMed
. - J. Tirado-Rives and W. L. Jorgensen, Performance of B3LYP density functional methods for a large set of organic molecules, J. Chem. Theory Comput., 2008, 4(2), 297–306 CrossRef CAS PubMed
. - T. Lu and F. Chen, Multiwfn: A Multifunctional Wavefunction Analyser, J. Comput. Chem., 2012, 33, 580–592, DOI:10.1002/jcc.22885
. - Origin(Pro), Version 2018. OriginLab Corporation, Northampton, MA, USA Search PubMed
. - Chemcraft - Graphical Software for Visualization of Quantum Chemistry Computations, Version 1.8, build 682, https://www.chemcraftprog.com Search PubMed
. - W. Humphrey, A. Dalke and K. Schulten, VMD - Visual Molecular Dynamics, J. Mol. Graphics, 1996, 14, 33–38 CrossRef CAS PubMed
. - F. J. Benítez, S. Gutiérrez-Oliva, B. Herrera and A. Toro-Labbé, Basis Electronic Activity of Molecular Systems. A Theory of Bond Reactivity, J. Phys. Chem. A, 2024, 128(10), 1902–1912 CrossRef
. - R. Liu, L. Qin, Z. Zhang, L. Zhao, F. Sagan, M. Mitoraj and G. Frenking, Genuine quadruple bonds between two main-group atoms. Chemical bonding in AeF-(Ae = Be–Ba) and isoelectronic EF (E = B–Tl) and the particular role of d orbitals in covalent interactions of heavier alkaline-earth atoms, Chem. Sci., 2023, 14(18), 4872–4887 RSC
. - J. S. Wenger, M. Weng, G. N. George and T. C. Johnstone, Isolation, bonding and reactivity of a monomeric stibine oxide, Nat. Chem., 2023, 15(5), 633–640 CrossRef CAS PubMed
. - D. Singh, Insights into Adsorbents: Activated Carbon for Effective Adsorption, J. Appl. Pharm. Res., 2024, 1(2), 11–21 Search PubMed
. - N. Blankevoort, P. Bastante, R. J. Davidson, R. J. Salthouse, A. H. Daaoub, P. Cea and H. Sadeghi, Exploring the Impact of the HOMO–LUMO Gap on Molecular Thermoelectric Properties: A Comparative Study of Conjugated Aromatic, Quinoidal, and Donor–Acceptor Core Systems, ACS Omega, 2024, 9(7), 8471–8477 CAS
. - P. Dhali and A. A. Hossain, Investigating the adsorption potential and sensitivity of pristine along with carbon, boron, and nitrogen substituted heteronanocages towards azacitidine drug, J. Mol. Liq., 2024, 396, 124051 CrossRef CAS
. - A. Saral, A. Manikandan, S. Javed and S. Muthu, Vibrational spectra, molecular level solvent interaction, stabilization, donor-acceptor energies, thermodynamic, noncovalent interaction and electronic behaviors of 6-methoxyisoquinoline-anti tubercular agent, Chem. Phys. Impact., 2024, 8, 100392 CrossRef
. - S. K. Yilmaz, A. A. Agar, E. B. Cinar, N. Dege, V. G. Vidya and V. V. Kumar, Deciphering noncovalent interactions in unprecedented binuclear copper complex: spectroscopic, Hirshfeld surface and DFT investigation, J. Mol. Struct., 2024, 1299, 137111 CrossRef CAS
. - R. Pal and P. Chattaraj, Structure, Stability, Reactivity and Bonding in Noble Gas Compounds, Phys. Chem. Chem. Phys., 2024, 26(13), 9856–9866 RSC
. - T. Li, L. Zhang, L. Zhang, J. Ke, T. Du, L. Zhang and T. Qian, Tailoring the Chemisorption Manner of Fe d-Band Center with La2O3 for Enhanced Oxygen Reduction in Anion Exchange Membrane Fuel Cells, Adv. Funct. Mater., 2024, 34(9), 2309886 CrossRef CAS
. - S. Xu, K. Xu, M. Liu, X. Song and K. Xie, Tuning the Fe–N4 electrocatalytic CH3NO2 reduction reaction based on axial ligands: a computational study, Mater. Today, 2024, 25, 100683 Search PubMed
. - Y. Jia, Y. Zhang, H. Xu, J. Li, M. Gao and X. Yang, Recent Advances in Doping Strategies to Improve Electrocatalytic Hydrogen Evolution Performance of Molybdenum Disulfide, ACS Catal., 2024, 14, 4601–4637 CrossRef CAS
. - M. Javed, M. U. Khan, R. Hussain, F. Abbas and T. Ahamad, Deciphering the detection and electrochemical sensing of the environmental pollutant CO gas with Ga12As12 and Al12As12 nanostructured materials: an insight from first-principle calculations, J. Mater. Sci., 2024, 1–23 CAS
. - Y. Malik, N. Naseem, F. Tariq, K. Ayub, S. A. Alsalhi, S. A. Abdelmohsen and J. Iqbal, Unveiling the unique properties of carbon nitride (C6N8) monolayer as a novel flexible sensor for hydrogen cyanide and hydrogen fluoride: a DFT study, Diamond Relat. Mater., 2024, 110930 CrossRef CAS
. - F. Tariq, Y. Malik, N. Naseem, W. A. Zahid, A. Younis, R. A. Khera and J. Iqbal, Sensing Potential of Carbon Nitride (C6N8) for the Detection of Hydrogen Sulfide (H2S) and Nitrogen Trichloride (NCl3): A DFT approach, IEEE Sens. J., 2024, 24(7), 9383–9389 CAS
. - N. A. Hassanuddin, E. Normaya, H. Ismail, A. Iqbal, M. B. M. Piah, S. Abd Hamid and M. N. Ahmad, Methyl 4-pyridyl ketone thiosemicarbazone (4-PT) as an effective and safe inhibitor of mushroom tyrosinase and antibrowning agent, Int. J. Biol. Macromol., 2024, 255, 128229 CrossRef CAS
. - N. Wang, X. Wang, S. Zhang, T. Wang and D. Yu, Noncovalent interaction between soybean protein isolate and naringenin: focused on binding mechanism, interface behavior, and functional properties, Food Hydrocolloids, 2024, 109975 CrossRef CAS
. - Y. Jiang, C. M. Deane, G. M. Morris and E. P. O'Brien, It is theoretically possible to avoid misfolding into noncovalent lasso entanglements using small molecule drugs, PLoS Comput. Biol., 2024, 20(3), e1011901 CrossRef CAS PubMed
. - R. J. Grams, W. L. Santos, I. R. Scorei, A. Abad-García, C. A. Rosenblum, A. Bita and M. A. Soriano-Ursúa, The Rise of Boron-Containing Compounds: Advancements in Synthesis, Medicinal Chemistry, and Emerging Pharmacology, Chem. Rev., 2024, 124(5), 2441–2511 CrossRef CAS PubMed
. - T. Noor, M. Waqas, M. Shaban, S. Hameed, Ateeq-ur-Rehman, S. B. Ahmed and A. A. Hassan, Designing Thieno [3,4-c] pyrrole-4,6-dione Core-Based, A2–D–A1–D–A2-Type Acceptor Molecules for Promising Photovoltaic Parameters in Organic Photovoltaic Cells, ACS Omega, 2024, 9(9), 6403–6422 CrossRef CAS PubMed
. - R. S. Odera, A. C. Ezika, G. J. Adekoya, E. R. Sadiku, V. U. Okpechi and H. C. Oyeoka, Computational investigation of the interaction mechanisms of low-density polyethylene (LDPE)/polyurethane and low-density polyethylene (LDPE)/hexane systems as absorbents for oil spill remediation: A DFT study, Polym. Eng. Sci., 2024, 64(3), 1274–1285 CrossRef CAS
. - M. J. Saadh, S. A. Jasim, S. M. Dhiaa, S. Chandra, H. A. Alwan, H. M. Baeissa and E. H. Kadhum, A DFT study the role of pristine and metal doped of g-CN nanosheet for drug delivery system, Comput. Theor. Chem., 2024, 1232, 114448 CrossRef CAS
. - V. Palermo, M. Palma and P. Samorì, Electronic characterization of organic thin films by Kelvin probe force microscopy, Adv. Mater., 2006, 18(2), 145–164 CrossRef CAS
. - M. Goudarzi, J. Karamdel, H. Hassanabadi and S. Zorriasatein, Study of electronic, optical, thermodynamic, and thermoelectric properties of GeC bulk and monolayer structure, Mod. Phys. Lett. B, 2024, 2450215 CrossRef CAS
. - H. Wang, Z. Huang, X. Zeng, J. Li, Y. Zhang and Q. Hu, Enhanced anticarbonization and electrical performance of epoxy resin via densified spherical boron nitride networks, ACS Appl. Electron. Mater., 2023, 5(7), 3726–3732 CrossRef CAS
. - N. Erum, R. Sharma, N. Anwar, S. Al-Qaisi, M. M. Al-Anazy and E. S. Yousef, Enhanced mechanical, optoelectronic and thermoelectric properties of binary CdO by under pressure: An ab initio study, Int. J. Quantum Chem., 2024, 124(5), e27360 CrossRef CAS
. - S. Yang, Y. Zhang, Z. Sha, Z. Huang, H. Wang, F. Wang and J. Li, Deterministic manipulation of heat flow via three-dimensional-printed thermal meta-materials for multiple protection of critical components, ACS Appl. Mater. Interfaces, 2022, 14(34), 39354–39363 CrossRef CAS PubMed
. - A. Toghan, A. A. Farag, O. K. Alduaij, H. M. Elabbasy, H. M. Dardeer, E. M. Masoud and H. S. Gadow, Electrochemical, gravimetric, quantum chemical and computational investigations on an effective synthetic chlorinated cyclic imide derivative as a corrosion inhibitor for carbon steel in sulfuric acid solution, J. Mol. Struct., 2024, 138040 CrossRef CAS
. - A. ElsharifR. AlyamiS. A. HaladuD. W. AlmagribiR. AlmalihD. K. ShalabiM. Abd El-LateefA Comprehensive Study on the Synthesis of Novel Chitosan Derived Schiff Base Derivatives as Effective Corrosion Inhibitors in Acidic EnvironmentsEnvironments 2024preprint DOI:10.2139/ssrn.4743941
. - L. Saya, B. Arya, K. Rastogi, M. Verma, S. Rani, P. K. Sahu and S. Hooda, Recent advances in sensing toxic nerve agents through DMMP model simulant using diverse nanomaterials-based chemical sensors, Talanta, 2024, 272, 125785 CrossRef CAS PubMed
. - H. Hrichi, A. M. Ali, N. A. Elkanzi and A. Abdou, A selective colorimetric chemosensor for detecting Ni(II) in aqueous solutions based on 4-[{[4-(3-chlorophenyl)-1,3-thiazol-2-yl] hydrazono} methyl] phenyl 4-methyl benzene sulfonate (CTHMBS), Anal. Sci., 2024, 1–14 Search PubMed
. - L. Saya, B. Arya, K. Rastogi, M. Verma, S. Rani, P. K. Sahu and S. Hooda, Recent advances in sensing toxic nerve agents through DMMP model simulant using diverse nanomaterials-based chemical sensors, Talanta, 2024, 272, 125785 CrossRef CAS PubMed
. - X. Wang, Y. Y. Xin, Y. Liu, Y. M. Xu, X. L. Cheng, X. F. Zhang and L. H. Huo, Growth of Ni5P4/Ni2P/C nanosheet arrays on ITO as dual-function electrochemical sensor for efficient Cu2+ and paracetamol detection, Ceram. Int., 2024, 50(11), 18584–18593 CrossRef CAS
. - H. Wang, M. Ye, J. Wu, Y. Li, L. Guo and D. Yang, Development of amine functionalized graphene oxide/fluorinated polyurethane topcoat with integrated anti-corrosion, anti-aging, and antibacterial performance, Prog. Org. Coat., 2024, 189, 108337 CrossRef CAS
. - Y. Lu, M. Stegmaier, P. Nukala, M. A. Giambra, S. Ferrari, A. Busacca and R. Agarwal, Mixed-mode operation of hybrid phase-change nanophotonic circuits, Nano Lett., 2017, 17(1), 150–155 CrossRef CAS PubMed
.
|
This journal is © The Royal Society of Chemistry 2024 |
Click here to see how this site uses Cookies. View our privacy policy here.