DOI:
10.1039/D4RA02217C
(Paper)
RSC Adv., 2024,
14, 13850-13861
Construction of a novel ternary synergistic CuFe2O4–SnO2-rGO heterojunction for efficient removal of cyanide from contaminated water
Received
23rd March 2024
, Accepted 8th April 2024
First published on 26th April 2024
Abstract
Many industrial effluents release cyanide, a well-known hazardous and bio-recalcitrant pollutant, and thus, the treatment of cyanide wastewater is a major challenge. In the current study, a CuFe2O4–SnO2-rGO nanocomposite was synthesized to remove cyanide from an aqueous system. The structural and morphological characterizations of the nanomaterials were investigated by X-ray diffraction (XRD), Fourier transform infrared spectroscopy (FTIR), scanning electron microscopy (SEM) and energy dispersive spectra (EDX) analysis. The results revealed that almost 97.7% cyanide removal occurred using the nanocomposite at an initial concentration of 100 mg L−1 within 1 h. The experimental data were fitted to various adsorption models, among which the Langmuir model fitted the data very well, confirming the monolayer adsorption process. The kinetic investigation revealed that the cyanide adsorption process followed a pseudo-second-order kinetic model, indicating a chemisorption process with a high cyanide adsorption capacity of 114 mg g−1. The result of the intraparticulate diffusion model fitting revealed a decreasing slope value (K) from stage 1 to stage 2, indicating that external mass transfer is the predominating step. Moreover, the CuFe2O4–SnO2-rGO nanocomposite shows excellent reusability.
1. Introduction
Cyanide, a typical contaminant, is commonly found in industrial wastewaters such as those from metal processing, photography, electroplating, ore leaching, and coke oven plants. Additionally, industries involved in producing nitrile, nylon, and acrylic plastics contribute cyanide to the receiving environment. Collectively, these industries discharge approximately 14 million kg of cyanide into the surrounding environment each year.1 According to a report, 1000 kg of gold concentrate discharges nearly 4 m3 of wastewater containing cyanide.2 It has been reported that the coke oven and steel-making industries discharge 10–50 mg L−1 of cyanide into the receiving environment.3 However, as per the United States Health Service, the allowable limit for cyanide is 0.2 mg L−1.4 Cyanide is a hazardous substance with resistance to chemical and biological processes. In general, cyanide inhibits the cytochrome oxidase enzyme system, consequently impeding the mitochondrial electron transport system and impairing the respiratory system. The harmful effects of cyanide on human health include severe impairment of the kidneys, skin, eyes, and central nervous system.3 Therefore, treatment of wastewater that contains cyanide has gained attention in recent years.
Various methods have been extensively discovered for the treatment of cyanide-containing wastewater, such as coagulation, flocculation, bioremediation, photocatalytic oxidation, electrochemical, ozonation, Fenton reaction, and ion exchange.5 Nevertheless, many of these technologies have drawbacks, such as increased electricity demands, high costs, low efficiency, and the generation of potentially harmful intermediate by-products.6 Similarly, although bioremediation is an eco-friendly and cost-effective technique, at a pH of less than 10, cyanide is converted to HCN gas and causes air pollution.7 On the other hand, microorganisms cannot grow and degrade pollutants at a pH greater than 10. On the other hand, adsorption is a traditional yet effective method for eliminating recalcitrant biological pollutants such as heavy metals or organic and inorganic substances from aqueous solutions and has thus gained popularity in wastewater treatment.8 Among the various potential adsorbents, the unique super-paramagnetic properties, substantial surface area-to-volume ratio and exceptional adsorption capabilities of magnetic nanoparticles have sparked significant interest. Among the key magnetic nanomaterials, spinel structures, which are often composed of ferrites, hold particular prominence.9 Among the various ferrites examined, copper ferrite (CuFe2O4), which is a spinel ferrite, stands out as a promising adsorbent due to its advantageous catalytic reactions, surface area, and exceptional magnetic properties, and thus can remove an inclusive range of contaminants from polluted wastewater.10–12 Furthermore, the enhanced adsorption performance is attributed to the tendency of spinels to occupy the octahedral and tetrahedral sites with trivalent and divalent cations13. Similarly, graphene-based materials have effectively improved the limited sensitivity of spinel CuFe2O4 and are thus widely used for pollutant removal.14 In addition, emphasis is placed on graphene-oxide-based nanomaterials due to their substantial surface area, stability, remarkable optical and electrical properties, and high thermal conductivity.14,15 Additionally, these materials are lightweight, less-toxic, and cost-effective compared to other intricate organic and inorganic adsorbents.16 Recently, reduced graphene oxide (rGO) gained tremendous attention as an adsorbent owing to its three-dimensional networks, which feature interpenetrating micropores and mesoporous structures that improve mass transfer capabilities. Its low density, robust mechanical strength, and adjustable surface functional groups make rGO effective in targeting various pollutants.17
SnO2 has garnered significant interest due to its various technological applications, such as serving as a catalyst for the oxidation of organics, possessing high conductivity, transparency, and thermal stability, and has thus gained popularity in environmental applications for the elimination of heavy metal ions and dyes, etc.18 The nanostructures of SnO2 possess intrinsic oxygen vacancies, including in-plane and bridging oxygen vacancies, resulting in the generation of shallow donor states beneath the minima of the conduction band (CBM). This electronic configuration promotes electron transfer, thereby enhancing electron mobility and pollutant removal capabilities.19 Additionally, it can be assembled into hollow spheres to serve as an effective adsorbent in water treatment.20 In the literature, a comparable observation has also been documented: the incorporation of SnO2 into the rGO sheet develops the extensive surface area of the SnO2-rGO nanocomposite, imparting a highly mesoporous structure and elevating the adsorption capacity of the SnO2-rGO.21
Therefore, in this investigation, a novel CuFe2O4–SnO2-rGO nanocomposite has been synthesized employing the hydrothermal method. The structural, morphological, and compositional characterizations of the nanomaterials were executed by XRD, FTIR, FESEM, and EDAX studies. The cyanide removal performance was evaluated in a batch study. The cyanide adsorption performance of the nanocomposite was assessed by applying different adsorption isotherms and adsorption kinetic models. Further, the reusability of the nanocomposite for cyanide adsorption was investigated.
2. Materials and methods
2.1. Chemicals and reagents
Analytical-grade graphite powder, ferric nitrate (Fe(NO3)3), purity 98%; tin chloride (SnCl2), purity 97%; copper nitrate (Cu(NO3)2), purity 98% and sodium sulfide (Na2S), purity 60% were purchased from LOBA, India. Sodium nitrate (NaNO3), purity 99.5%; potassium permanganate (KMnO4), purity 98.5%; hydrogen peroxide (H2O2), purity 30%, ammonium hydroxide (NH4OH), purity 25%; hydrazine hydrate (N2H4·H2O), purity 80%; ethylene glycol (C2H6O2), purity 99%; iron chloride (FeCl3), purity 98%; titanium butoxide (Ti(OBu)4), purity 97%; chloroform (CHCl3), purity 99%; and dimethyl formamide (C3H7NO), purity 99.5%; and tin oxide (SnO2), purity 99%, were purchased from Merck, India. Thioglycolic acid (C2H4O2S), purity 98%, was purchased from Sigma Aldrich. The remaining reagents and chemicals utilized in this investigation were of laboratory and analytical grade.
2.1.1. Synthesis of CuFe2O4. CuFe2O4 was prepared using a hydrothermal method as outlined in the literature.22,23 Briefly, a mixture of 12.08 g of Cu(NO3)2 and 24.186 g of Fe(NO3)3 was dissolved in 50 ml of distilled water and stirred for 3 h. Subsequently, 4 ml C2H4O2S was introduced into the solution, followed by an additional 2 h of stirring. The resultant solution was poured into a 100 ml stainless steel autoclave with a Teflon lining and subjected to a temperature of 230 °C in a muffle furnace for 8 h. After cooling, the obtained nanomaterial was thoroughly rinsed with distilled water and ethanol and dried at 60 °C. The obtained product was calcinated at 700 °C for 5 h in a muffle furnace to obtain the final product.
2.1.2. Synthesis of SnO2. SnO2 was prepared following the methodology outlined in the literature.24 The reaction mixture was made by dissolving 0.1 M of SnCl2 in 50 ml of distilled water. Subsequently, 1 M NaOH was gradually added to achieve a pH of 7, and then the mixture was stirred at 80 °C for 2 h. The precipitate was collected through centrifugation at 12
000 rpm for 20 min and washed thoroughly with acetone. The product was dried in a hot air oven at 150 °C for 1 h to remove moisture and obtain its Sn2+ state. The dried mixture was subsequently moved to a silica crucible for oxidation and underwent annealing in a muffle furnace for approximately 4 h at a temperature of 600 °C. The resulting product was SnO2 in the Sn4+ oxidation state.
2.1.3. Synthesis of rGO. Utilizing a modified Hummer process, graphene oxide (GO) was prepared.4 Briefly, 2 g of graphite powder was introduced into 80 ml of concentrated H2SO4 maintained at 0 °C using an ice bath. Subsequently, 4 g of NaNO3 and 8 g of KMnO4 were gradually incorporated with constant stirring, and the mixture temperature was maintained below 10 °C for 4 h. After another 4 h of stirring at 35 °C, the solution was diluted with 200 ml of distilled water and stirred for 1 h. Subsequently, 15 ml of a 30% H2O2 solution was added to the reaction mixture. The solution was centrifuged at 12
000 rpm for 20 min to separate the solid product. The filtrate was washed with water and ethanol to remove any impurities. The final product was dried in a vacuum oven at 60 °C for 24 h. The synthesis of rGO was performed as outlined in the literature.4 Briefly, 1 g of previously prepared GO was sonicated with 300 ml of distilled water for 20 min. Nitrogen gas was then purged into the solution for 15 min. Then, 0.5 ml of N2H4·H2O solution was added and the mixture was sonicated again for 10 min. The resultant suspension was transferred to a reflux condenser at 80 °C for 8 h. The resulting solution was centrifuged at 12
000 rpm for 20 min to obtain the precipitate, which was rinsed thoroughly with deionized water and ethanol many times and finally dried at 60 °C for 24 h.
2.1.4. Synthesis of CuFe2O4–SnO2-rGO nanocomposite. 12.08 g of Cu (NO3)2·3H2O and 40.4 g of Fe(NO3)3·9H2O were mixed with 50 ml of distilled water in a beaker to prepare solution A. In another beaker, 1 g of the previously synthesized rGO was added to 20 ml of distilled water and sonicated for 30 minutes to obtain solution B. Then, 5 ml of citric acid was added dropwise to the mixture of solutions A and B and stirred at 100 °C. After that, 3.918 g of previously prepared SnO2 was introduced to the solution. The resultant mixture was kept in a muffle furnace at 450 °C for 2 h to obtain the CuFe2O4–SnO2-rGO nanocomposite.
2.2. Experimental setup
The adsorption of cyanide by the nanocomposite was carried out in the batch shake flask mode of operation. In the present study, a cyanide solution with varying concentrations was taken in a 25 ml conical flask with 1 g L−1 of the nanocomposite for the adsorption experiments. 1 M NaOH solution was used to maintain the pH at ∼12.5 to avoid the evolution of HCN gas. The adsorption reaction was carried out under stirring at 300 rpm. The sample was collected at 15 min intervals and centrifuged at 12
000 rpm. The supernatant solutions of the centrifuged samples were employed to determine the residual concentration of cyanide using the colorimetric method as specified in the USEPA guidelines.25 The removal efficiency of cyanide was estimated as follows: |
Removal efficiency (%) = (C0 − C)/C0 × 100
| (1) |
C0 and C represent the initial and final concentrations of cyanide, respectively.
2.3. Analytical technique
The XRD characterization of the synthesized nanomaterials was performed using an X-ray powder diffractometer (Model: Rikagu Ultima Japan) with CuKα (λ = 1.5405 Å) over a broad range of Bragg angles (20° ≤ 2θ ≤ 80°) to verify the formation of the desired nanomaterial. The structural phase and cell parameters were computed by applying the X'Pert Highscore Plus software. The FTIR spectra of the produced nanomaterials were carried out using a JASCO-4100 IR spectrophotometer, with KBr pellets serving as the standard. The nanomaterials were subjected to scanning electron microscopy (SEM) for microstructural examination, utilizing a Zeiss Supra 40 instrument (Model: S-3400, Serial No. 340749-10) with an accelerating voltage of 15
000 kV. EDX analysis was executed to assess the elemental composition of the nanomaterials using identical Zeiss Supra 40 instruments.
3. Results and discussion
3.1. Characterization
3.1.1. XRD analysis of the synthesized nanomaterials. The XRD patterns of the synthesized nanomaterials CuFe2O4, SnO2, rGO, and CuFe2O4–SnO2-rGO are presented in Fig. 1. The diffraction peaks of CuFe2O4 at 2θ values of 18.36, 30.02°, 33.55°, 34.72°, 35.65°, 37.19°, 41.20°, 44.25°, 49.67°, 54.14°, 57.78°, 62.96°, and 64.15° correspond to the 101, 112, 200, 103, 211, 202, 004, 220, 301, 312, 303, 224, and 400 planes, respectively, which correspond to the tetragonal structure of the CuFe2O4. These observations are well matched with the standard values (references: JCPDS 01-072-1174, JCPDS 96-900-6319) and are also correlated with the previously reported literature.26–28 The diffraction peaks at 2θ values of 26.83°, 34.13°, 38.12°, 52.01°, 54.96°, 58.14°, 62.13°, 64.97°, 66.25°, 71.56°, and 78.97° of SnO2 correspond to the 110, 101, 200, 211, 220, 002, 310, 112, 301, 202, and 321 planes, respectively, which are correlated with the standard values (reference: JCPDS 96-153-3655) and are well supported by many reports in the literature.29–31 The sharp peaks obtained at 2θ values of 26.83°, 34.13°, and 52.01° indicate that the nanomaterial has good crystallinity. In the XRD pattern of rGO, the primary diffraction peaks at 25.8° and a secondary peak at 43.8° correspond to the (002) and (100) planes of graphite and are correlated with the standard reference values (JCPDS 01-075-1621, JCPDS 00-026-1075). In the literature, a comparable observation on rGO was also documented.32,33 The diffraction peaks at 2θ values of 26.72°, 34.01°, 35.60°, 37.89°, 38.34°, 52, 54.96°, 57.47°, 62.46°, 64.1°, 65.89°, 71.34°, and 78.62° of the nanocomposite CuFe2O4–SnO2-rGO correspond to the 110, 101, −311, 200, 111, 211, 220, 002, 310, 112, 301, 202, and 321 planes, respectively, which are correlated with JCPDS card numbers 01-088-0287 and 96-210-8028. The diffraction peaks of SnO2 at 2θ values of ∼26°, 34°, 38°, 52°, 54°, 58°, 62°, 64°, 66°, 71°, and 78° are well matched with the synthesized nanocomposite CuFe2O4–SnO2-rGO. The peak at a 2θ value of ∼35° of CuFe2O4 corresponds to the diffraction peak of the nanocomposite. The XRD data indicated that CuFe2O4 and SnO2 were successfully grown on the surface of rGO.
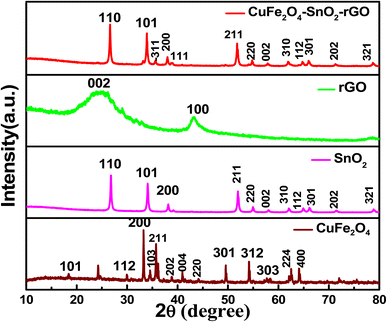 |
| Fig. 1 XRD patterns of the synthesized CuFe2O4, SnO2, RGO, and CuFe2O4–SnO2-rGO nanocomposite. | |
3.1.2. FTIR analysis of the synthesized nanomaterials. The FTIR spectra of the prepared nanomaterials are presented in Fig. 2. In Fig. 2(a), the peaks located at approximately 400–500 cm−1, i.e., at 472 cm−1 and 516 cm−1, represent the Cu–O bond.34 The Fe–O–H bending vibration appeared at 796.457 cm−1.35 The peaks appearing at 2359.48, 3484.74, and 3571.52 cm−1 were assigned to the stretching and bending vibrations of O–H, indicating the absorption of water molecules.36 The presence of peaks at 1107.9 cm−1 indicates stretching vibrations related to the C–O band.34 Fig. 2(b) represents the FTIR spectra of SnO2. In Fig. 2(b), the occurrence of the peak at 602.646 cm−1 reveals the existence of Sn–O–Sn bonds.33 The peaks at 3762.44 cm−1, 3693.01 cm−1, and 3423.03 cm−1 correspond to the stretching vibration of the O–H bond.37,38 The peak at 528.4 cm−1 matches the stretching vibrations of the terminal Sn–O.38 Fig. 2(c) demonstrates the FTIR spectrum of the rGO. The occurrence of peaks at 3173.29 cm−1 and 2358.52 cm−1 reveals the existence of C–OH and CO2 in rGO, indicating the hygroscopic and porous nature of graphite.39 In the FTIR spectra of rGO, the graphite bands observed beyond 3400 cm−1 (OH groups) are not prominent, and the C
O and C–O–C stretches are observed at 1712.48 cm−1 and 1220 cm−1.40 Additional peaks at 1044 cm−1 and 1220 cm−1 were detected, suggesting the existence of C–O stretching vibrations related to alkoxy and epoxy groups, respectively.41 Fig. 2(d) shows the FTIR spectra of the nanocomposite, in which the peaks at 512.97 cm−1 and 526.47 cm−1 match with SnO2, which reveals the stretching vibrations of the terminal Sn–OH. The peaks at 3449.06, 2358.52, 1630.52, and 1421.06 cm−1 are matched with CuFe2O4, SnO2, and rGO, which indicate the presence of an O–H group. This result confirms that the CuFe2O4–SnO2-rGO nanocomposite was chemically synthesized.
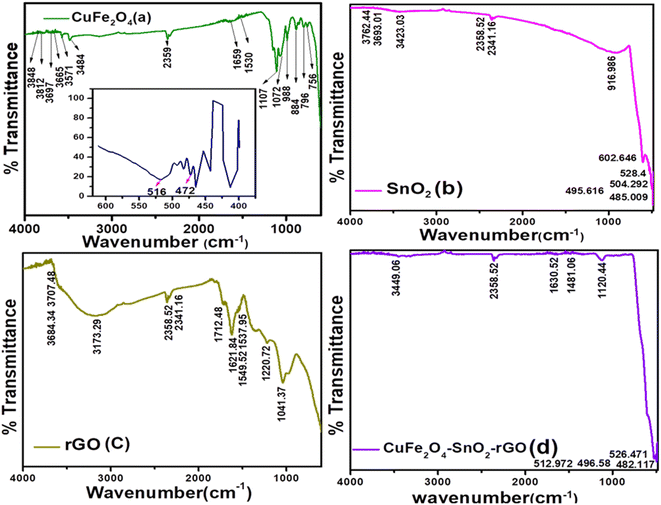 |
| Fig. 2 FTIR analysis of the synthesized nanomaterials (a) CuFe2O4, (b) SnO2, (c) rGO, and the (d) CuFe2O4–SnO2-RGO nanocomposite. | |
3.1.3. FE-SEM analysis of the synthesized nanomaterials. FE-SEM was utilized to examine the morphological structure, size, and distribution of the synthesized nanomaterials.42 The FESEM image of the CuFe2O4 is shown in Fig. 3(a), revealing agglomerated structures with limited particle distribution.43 The FESEM image of copper ferrite illustrates the spherical shape of the nanomaterials with an average size of less than 400 nm.44 The morphological characterization of SnO2 nanoparticles revealed a cluster of particles with well-defined edges, as illustrated in Fig. 3(b). Further, Fig. 3(b) shows the spherical shape of SnO2 nanoparticles with an average size of 100 nm and a slightly agglomerated surface.45 Agglomeration increases as the particle size moves towards the nanoscale.46 Fig. 3(c) presents the FESEM image of rGO, which displayed an interconnected, partially folded, three-dimensional porous, exfoliated, and corrugated structure. The reduction of GO to rGO is responsible for this corrugated structure, and the elimination of oxygen-containing groups from the GO surface causes aggregation. These observations are most likely caused by the π–π stacking phenomena in the graphene molecule.2 It was calculated that rGO was 200–300 nm in size on average. The observed 2D structure is crucial for maintaining the thermodynamic stability of graphene.47 Fig. 3(d) presents the FE-SEM image of the CuFe2O4–SnO2-rGO nanocomposite. From the figure, it is evident that CuFe2O4 and SnO2 are uniformly dispersed on the rGO sheet. The occurrence of rGO flakes in the nanocomposite is responsible for the characteristic wrinkled and exfoliated structure. This implies that the increased loading of SnO2 is mostly caused by the oxygen-carrying groups on the surface of rGO. Fig. 3(d) shows that the SnO2 and CuFe2O4 nanomaterials are attached to the rGO surface. The Williamson and Hall (W–H) plotting technique involves determining the peak width with 2θ. This relation is used to estimate parameters such as average lattice strain and crystalline size, as expressed below: |
β cos θ = 4ε sin θ + kλ/D
| (2) |
where β represents the full width at half maximum (FWHM), θ represents the angle of diffraction, λ denotes the wavelength (0.154 nm), k is a constant with a value of 0.89, ε denotes lattice strain, and D signifies the average crystalline size. This method was implemented by plotting the value of 4
sin
θ versus β
cos
θ. The slope of the curve provides the lattice strain, while the Y-intercept indicates the value of D. The calculated D values for CuInS2 and SnO2 were 225.90 nm and 118.53 nm, respectively.
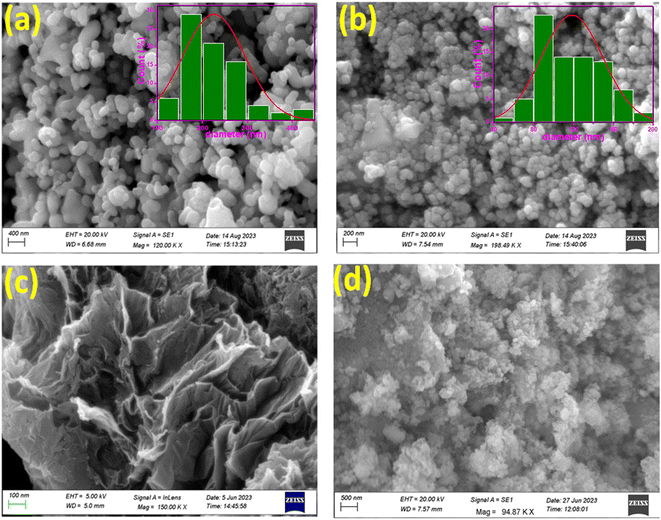 |
| Fig. 3 FE-SEM micrographs of (a) CuFe2O4, (b) SnO2, (c) rGO, and (d) CuFe2O4–SnO2-RGO. | |
3.1.4. EDX analysis of the synthesized nanomaterials. The elemental compositions of the prepared nanomaterials were analyzed using EDX spectroscopy. Fig. 4(a) presents the EDAX analysis of the synthesized CuFe2O4 nanomaterials. In the CuFe2O4 nanomaterial, the presence of stoichiometric percentages of Cu (16.3%), Fe (47%), and O (36%) without any impurities reveals the purity of the prepared nanomaterial. Fig. 4(b) illustrates the stoichiometric weight percentage of 83.4% tin and 16.6% oxygen in SnO2. This demonstrates that the SnO2 NPs are crystalline, which is their pure form. Fig. 4(c) depicts the EDX analysis of rGO and shows the occurrence of stoichiometric weight percentages of the elements C and O. EDAX analysis of rGO shows a higher weight percentage and atomic percentage of carbon, i.e., 66.6% and 72.7%, respectively. On the other hand, the lower weight percentage and atomic weight percentage of oxygen, i.e., 33.4% and 27.3%, respectively, confirm the successful synthesis of rGO. The EDAX spectrum of the CuFe2O4–SnO2-rGO nanocomposite confirms the existence of the elements Sn, C, O, Fe, and Cu in 48.5, 6.3, 12.2, 23.2, and 9.8 wt%, respectively, as illustrated in Fig. 4(d). Moreover, no other peaks were found in the spectrum, which confirms the purity of the prepared nanocomposite.
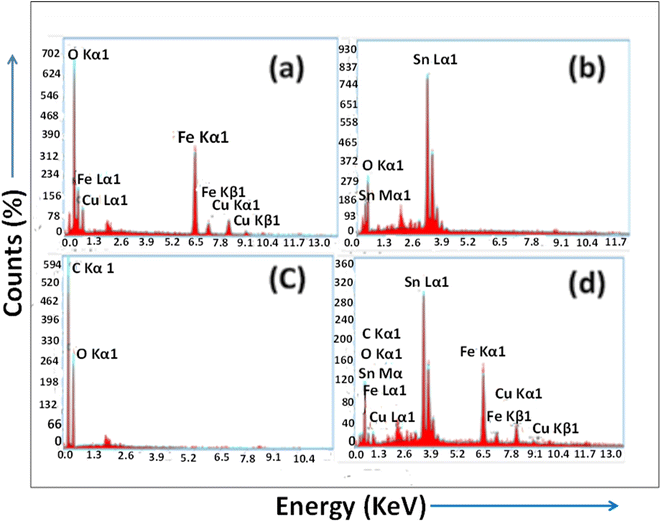 |
| Fig. 4 EDX spectra of the synthesized nanomaterials (a) CuFe2O4, (b) SnO2, (c) rGO, and (d) CuFe2O4–SnO2-RGO. | |
3.2. Adsorption isotherm model study
The adsorption isotherm models describe the sorption performance of the synthesized nano-materials, which defines the distribution of adsorbates among the liquid phase and solid phase at equilibrium. In the current work, the Langmuir, Freundlich, Temkin, and D–R isotherm models were used to evaluate the efficiency of cyanide removal.
3.2.1. Langmuir adsorption isotherm. The Langmuir isotherm models a monolayer homogenous sorption process in which no interaction occurs between adsorbate species.48,49 This, in turn, simplifies the model due to the fact that each binding site is occupied by only one atom or one molecule, and the adsorption energy is constant at all sites.50 The Langmuir isotherm can be expressed as shown in eqn (3). |
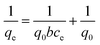 | (3) |
where q0 represents the adsorption capacity of the nanocomposite (mg g−1), and b represents the binding energy constant. Ce and qe are the adsorbate concentration and adsorption capacity at equilibrium conditions. The experimental data were plotted as 1/qe vs. 1/Ce, as presented in Fig. 5(a). The relatively higher correlation coefficient (R2 = 0.94) indicates that the Langmuir model best fits the cyanide sorption process. The q0 and b values were measured from the intercept and slope of the linear plot, as illustrated in Fig. 5(a), and were calculated to be 0.0016 l mg−1 and 84.96 mg g−1, respectively. The Langmuir model indicated that cyanide adsorption by the nanocomposite is well-modelled as a homogeneous monolayer adsorption process. This indicates that CuFe2O4 and SnO2 were uniformly distributed and grafted successfully onto the rGO surface. The dimensionless separation factor (RL) can express the main features of a Langmuir isotherm.51 The RL value can be calculated using the following formula: |
 | (4) |
where C0 represents the initial cyanide concentration. When the value of RL > 1, it indicates that the sorption process is unfavourable; if the RL value lies between 0 < RL < 1, then the adsorbent surface exhibits favourable conditions; when the RL value is 0, it indicates the irreversible nature of adsorption, and RL = 1 indicates a linear relationship.52 In the current study, the value of RL is 0.862 at a starting cyanide dose of 100 mg L−1, which suggests that the sorption process is favourable.
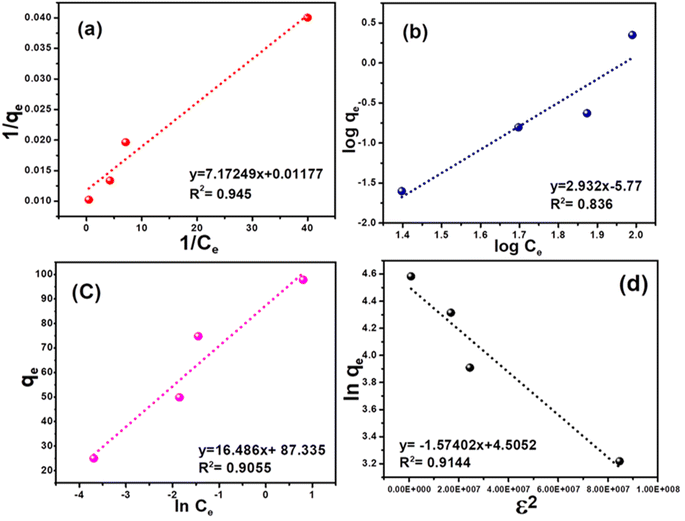 |
| Fig. 5 Adsorption isotherms for the removal of cyanide using the CuFe2O4–SnO2-rGO nano-composite: (a) Langmuir, (b) Freundlich, (c) Temkin, and (d) R–D isotherm. | |
3.2.2. Freundlich adsorption isotherm. The Freundlich isotherm model represents a monolayer heterogeneous adsorption process in which the quantity adsorbed increases with increasing solution concentration. The isotherm relationship can be presented as follows: |
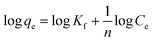 | (5) |
Here, qe denotes the adsorption capacity (mg g−1), and Ce represents the unadsorbed concentration of the adsorbate at equilibrium conditions. Kf and n are the relative adsorption capacity and adsorption intensity of the adsorbent, respectively. Fig. 5(b) presents the plot of log
qe vs. log Ce. When n > 1, it indicates attractive forces within the surface layer of the adsorbent, and the adsorbent binds firmly with the adsorbent;49 if n < 1, then the adsorbent surface layer exhibits a repulsive force.48,53 However, in the present study, the computed R2 value is 0.83, which indicates that the Freundlich isotherm model did not fit the experimental data.
3.2.3. Temkin adsorption isotherm. The Temkin isotherm considers the interaction between the adsorbent and adsorbate and the heat of adsorption.54 In addition, unlike the Freundlich model, this isotherm model shows a linear decline in adsorption energy with increasing surface coverage. The Temkin isotherm can be represented as follows: |
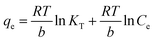 | (6) |
Here, qe is the adsorption capacity at equilibrium, RT/b is the molar interaction parameter, R represents the universal gas constant (8.314 J mol−1 K−1), T represents the absolute temperature (K), and b denotes the Temkin constant. Ce is the unadsorbed adsorbate at equilibrium, and KT is the equilibrium constant for the adsorption process. The Temkin isotherm excludes the very low and high adsorbate concentration ranges and only applies to an intermediate range. Fig. 5(c) demonstrates the plot of qe vs. ln
Ce. The KT and b values were determined from the intercept and slope of the linear plot, as shown in Fig. 5(c), and are calculated to be 17.81 and 7.81 L mg−1, respectively. However, the regression coefficient was found to be low (R2 = 0.91), indicating poor fitting of the experimental data with the model.
3.2.4. D–R adsorption isotherm. The D–R isotherm model makes assumptions about the typical adsorption behavior associated with the porous structure of the adsorbent. Also, in the D–R isotherm model, the mean free energy of the adsorption process is related to the chemical and physical adsorption processes.55 The D–R isotherm can be represented as shown below: |
ln qe = ln qm − βε2
| (7) |
Here, β represents the activity coefficient of adsorption energy, and ε denotes the Polanyi potential. |
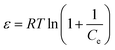 | (8) |
The physical and chemical adsorption characteristics can be expressed as the mean adsorption energy (E), as shown in eqn (9).
|
 | (9) |
Fig. 5(d) presents the D–R adsorption isotherm model graph of ln
qe versus ε2. The qm and β values are computed from the intercept and slope of the linear plot. However, the experimental data only moderately fitted the D–R isotherm model, with an R2 value of 0.91. A value of E < 8.0 kJ mol−1 signifies physisorption, and if the E value lies between 9–16 kJ mol−1, it indicates chemisorption.56 However, in this investigation, the calculated E value is 0.564 kJ mol−1, which is insignificant for the physical adsorption process.
3.3. Adsorption kinetic model
3.3.1. Pseudo first- and second-order kinetic models. The study of adsorption kinetics is a critical aspect that illuminates another essential facet of the adsorption phenomenon. It is imperative to explore the sorption kinetics of the adsorbent, as it delivers insights into the rate of adsorption and the controlling mechanisms governing the sorption process, particularly the mass transfer and physiochemical reactions.57,58 The pseudo-first-order kinetic model is applicable to explain the physical sorption rate and also describes the relation between the accessibility of vacant positions on the adsorbent surface and the sorption process.59 This model can be expressed by the Lagergren formula, which is presented below. |
ln(qe − qt) = ln qe − K1t
| (10) |
In this context, qt represents the sorption capacity at time t, and qe denotes the adsorption capacity at equilibrium conditions. K1 denotes the adsorption rate constant. Similarly, the pseudo-second-order kinetic model represents the chemisorption adsorption process. The linear form of this kinetic model is given below: |
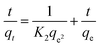 | (11) |
where K2 denotes the adsorption rate constant. Fig. (6) illustrates the kinetics of cyanide adsorption onto the CuFe2O4–SnO2-rGO nanocomposite. The computed cyanide sorption parameters of the different kinetic models are listed in Table 1. These findings showed that the experimental data fitted very well with the pseudo-second-order model with a higher R2 value of 0.996 compared to the pseudo-first-order reaction (R2 value = 0.98), as demonstrated in Fig. 6(a) and (b). The high R2 value observed for the pseudo-second-order kinetic model, compared to the pseudo-first-order model, indicates its predominance in the cyanide removal process. The alignment of the estimated data with the pseudo-second-order model suggests the occurrence of chemisorption in the adsorption method. Specifically, the unoccupied sites on the adsorbent surface exhibit chemical interactions by sharing or exchanging electrons with the adsorbate/cyanide ions in the solution,59,60 thus facilitating cyanide removal. The predicted theoretical cyanide sorption capacity applying the pseudo-second-order model (114.67 mg g−1) was in the vicinity of the experimental results (97.77 mg g−1), indicating that this kinetic model showed the best fit for cyanide adsorption. This is well correlated with reports in the literature.61 The elevated overall R2 value for the pseudo-second-order model suggests a substantial adsorbate uptake in the chemisorption process compared to physical adsorption. Table 2 presents a comparative analysis of the efficacy of various adsorbents for removing cyanide. The cyanide adsorption profile in this investigation proved to be better than those in the previously reported literature using various adsorbent systems such as modified zeolite-iron oxyhydroxide, Cu, Ag, Ni-impregnated activated carbon, calcinated eggshells, granular activated carbon, LTA zeolite-modified HDTMA, TiO2/Fe2O3/AC, and TiO2/AC, as shown in Table 2.
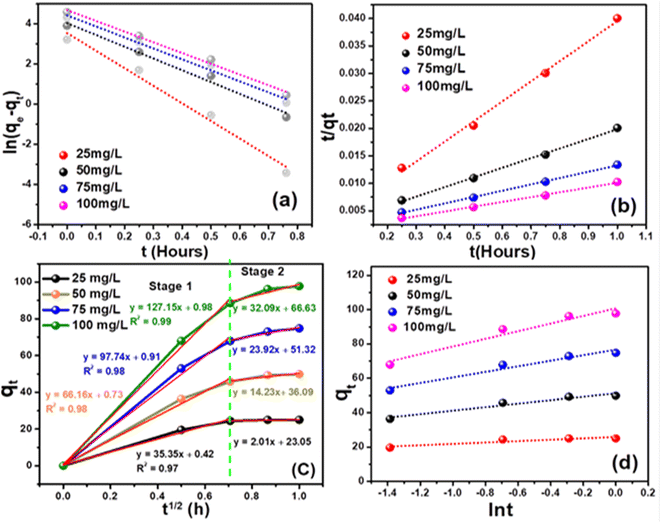 |
| Fig. 6 Study of the cyanide adsorption kinetics of the CuFe2O4–SnO2-rGO nano-composite: (a) pseudo-first-order model, (b) pseudo-second-order model, (c) intra-particle diffusion model and (d) Elovich model. | |
Table 1 Kinetic parameters for cyanide removal employing the CuFe2O4–SnO2-rGO nano-composite
Cyanide (mg L−1) |
Kinetic parametersa |
Intra-particle diffusion modela |
Elovicha |
k1 |
qe |
R2 b |
k2 |
k3 |
C1 |
R2 |
α |
β |
R2 |
Units for parameters: k1 (h−1), Qe (mg g−1), k2 (mg g−1 h−1), k3 (mg g−1 h−1-0.5), β (g mg−1), α (mg g−1 h−1). Pseudo-first-order rate constant denoted by K1 and pseudo-second-order kinetics denoted by K2. |
First-order |
5.354 |
108.60 |
0.98 |
Stage 1 |
127.1 |
0.98 |
0.99 |
2079.7 |
0.044 |
0.92 |
Second-order |
0.055 |
114.67 |
0.99 |
Stage 2 |
32.09 |
66.63 |
0.80 |
|
|
|
Table 2 Comparison of a list of parameters estimated from the kinetic models for the adsorption of various pollutants
SI no. |
Pollutant (mg L−1) |
Types of adsorbents |
K1 |
K2 |
Adsorption capacity qm (mg g−1) |
Reference |
1 |
0.5 mol L−1 |
Calcinated eggshells |
0.018 (min−1) |
0.0011 (g mg−1 min−1) |
3.27 |
62 |
2 |
102 |
Activated carbon |
0.193 (h−1) |
−0.002 (g mg−1 h−1) |
7.62 |
63 |
3 |
100 |
TiO2/AC |
0.055 (h−1) |
0.010 (h−1) |
90.9 |
64 |
4 |
75 |
LTA zeolite modified HDTMAB |
0.026 (min−1) |
0.0021 (g mg−1 min−1) |
24.09 |
65 |
5 |
0.5 |
Granular activated carbon |
0.0238 (min−1) |
0.881 (g mg−1 min−1) |
1.66 |
69 |
6 |
100 |
TiO2/Fe2O3/AC |
0.047 (h−1) |
0.011 (h−1) |
96.2 |
64 |
7 |
100 |
CuFe2O4–SnO2-rGO |
5.35 (h−1) |
0.0547 (mg g−1 h−1) |
114 |
This work |
3.3.2. Intra-particle diffusion model. The intra-particle diffusion model elucidated additional insights into the mass transfer mechanisms between the adsorbate and adsorbent throughout the sorption process. This model also represents the adsorption properties of cyanide ions on a porous adsorbent.49,66 The Morris and Webber model describes the intra-particle diffusion properties of an adsorbent, and the linear equation is given below.Here, K3 is the intra-particle diffusion rate constant. According to this model, if a plot of qt versus t1/2 shows a straight line, then intraparticle diffusion governs the sorption process; however, if the data show multi-linear curves, then the adsorption process is controlled by two or more stages.49 As presented in Fig. 6(c), the curves showed a multi-linearity regression relationship, demonstrating that cyanide sorption onto the CuFe2O4–SnO2-rGO nano-composite transpired through two stages. The initial distinct segment signifies the external mass transfer of cyanide from the aqueous phase to the solid–liquid interface. The second phase of progressive adsorption represents the pore diffusion of cyanide into the inner surface of the nanocomposite. The steeper slope of the fitting line with a higher correlation coefficient in stage 1 (first linear segment) indicates a faster cyanide adsorption process. Thus, the external mass transfer significantly impacted the cyanide removal rate. This result indicates that the external mass transfer controls the sorption rate, as shown in Table 1. In the second stage, the lower slope of the fitting line suggests a lower intra-particle diffusion rate, as established in Fig. 6(c). Numerous reports in the literature strongly support this phenomenon.58 Further, the K1 value for cyanide removal was notably higher than K2, indicating that boundary diffusion/external mass transfer was the predominant step, followed by intraparticle diffusion. This observation aligns with findings reported in the literature,66,67 in which a lower value of K2 than K1 was reported. The decreasing nature of this slope agrees with the many reports in the literature.68
3.3.3. Elovich model. The interaction between the adsorbed species is represented by the Elovich kinetic model, where the adsorption rate exhibits an exponential decline as the number of adsorbates rises on the adsorbent surface. Further, the Elovich model provides a strong correlation for adsorption on very active heterogeneous surface functional groups and demonstrates that chemisorption often occurs in addition to surface adsorption as a prominent process. However, surface adsorption occurs in a highly heterogeneous process with chemisorption, ion exchange, intra-particle diffusion, and precipitation.69 In the Elovich model, the reaction rate and diffusion control the adsorption rate. The Elovich model is expressed as follows: |
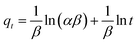 | (13) |
Here, the surface coverage and chemisorption activation energy are represented by β and α as the starting rate constant. The graph of qt vs. ln
t is displayed in Fig. 6(b). The slope of the straight line represented 1/β, and the intercept represents (1/β)ln(αβ). The calculated β values were 0.2487 and 0.044 g mg−1 for initial cyanide dosages of 25 and 100 mg L−1, as shown in Table 1. In the current investigation, the lower value of chemisorption activation energy (β) revealed that the feasibility of the chemisorption process is remarkably high. For starting concentrations of 100 mg L−1, the adsorption rate (α) was computed as 2079.74 × 103 mg g−1 h−1 with R2 values of 0.92. In the current investigation, these Elovich rate constants and activation energy values are superior to those reported in the literature. For instance, Inyinbor et al.70 computed a β value of 0.09 g mg−1 and an α value of 1159.89 mg g−1 h−1 using urea-modified Raphia hookerie epicarp for the degradation of 100 mg L−1 rhodamine dye. This observation revealed that the sorption of cyanide onto the CuFe2O4–SnO2-rGO nanocomposite is primarily governed by chemisorption rather than physical processes, which is also supported by the quick cyanide adsorption process.71 Therefore, it is understood that the adsorption of cyanide onto CuFe2O4–SnO2-rGO most likely occurs through surface exchange reactions until the point at which all of the surface functional sites are occupied.
3.4. Mechanism of cyanide removal by CuFe2O4–SnO2-rGO nanocomposite
The adsorption of CN− ions on the CuFe2O4–SnO2-rGO surface occurs through three main steps, which are electrostatic interaction, intra-particle diffusion, and external mass transfer. The detailed mechanism of cyanide removal using the CuFe2O4–SnO2-rGO nanocomposite is shown in Scheme 1. Electrostatic interactions occur between the negatively charged CN− ions and the positively charged ions present on the surface of the CuFe2O4–SnO2-rGO nanocomposite. The formula for CuFe2O4 is typically expressed as (Cu2+)1−x(Fe3+)x[(Cu2+)x (Fe3+)2−x]O42−. The A site is the tetrahedral site at which the metal ions outside the square brackets are located. In contrast, site B represents the ions inside the square bracket, which is an octahedral position. When the unit cell has a face-centered cubic structure, the oxygen ions are positioned at its face.72 In CuFe2O4, 3 CN− ions interact with 1 Fe3+ ion, and 2 CN− ions interact with 1 Cu2+. The nanostructures of SnO2 possess intrinsic oxygen vacancies, including in-plane and bridging oxygen vacancies, with 3D interconnected, size-tunable, trimodal pores, resulting in the generation of shallow donor states beneath the minima of the conduction band (CBM). This electronic configuration promotes electron transfer, thereby enhancing electron mobility and pollutant removal capabilities.19,73 In SnO2, 4 CN− ions interact with 1 Sn4+ ion through electrostatic interactions. The three-dimensional networks of rGO offer substantial surface area, stability, and remarkable optical and electrical properties, as they feature interpenetrating microporous and mesoporous structures that improve mass transfer capabilities.15,17 Further, due to the π–π interaction between the rGO surface and CN− ions, the adsorption capacity of rGO is high. The CN− ions from the aqueous phase are adsorbed onto the rGO surface under external mass transfer. Next, a process known as intraparticulate diffusion causes CN− ions to migrate from the exterior surface inside the microporous and mesoporous structures of rGO.
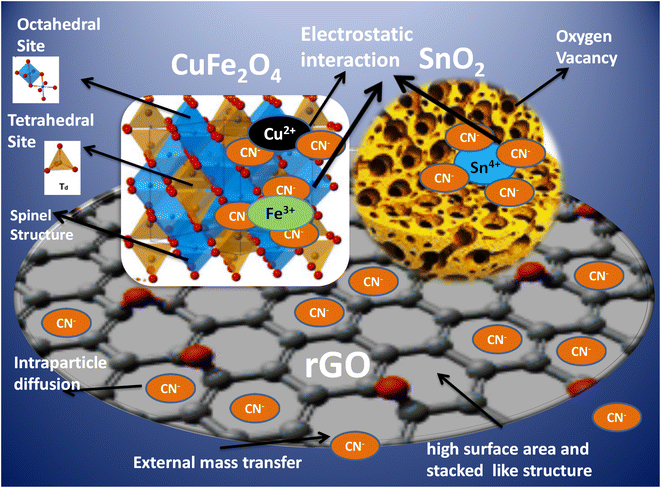 |
| Scheme 1 Mechanism of cyanide removal using the CuFe2O4–SnO2-rGO nanocomposite. | |
4. Conclusion
A CuFe2O4-rGO–SnO2 nanocomposite was created and characterized, and its cyanide removal efficiency was assessed. Almost 97.7% removal occurred within 1 h using the nanocomposite with a starting 100 mg L−1 cyanide dose. The CuFe2O4-rGO–SnO2 nanocomposite is a highly effective adsorbent for eliminating cyanide from polluted aqueous solutions. The experimental data were fitted well by the Langmuir model, signifying that cyanide was adsorbed as a monolayer on the homogeneous surface of the adsorbents. Furthermore, the cyanide adsorption method followed a pseudo-second-order kinetic model, which implies a chemisorption process. In comparison with other findings in the literature, the cyanide adsorption capacity of 114 mg g−1 in the current investigation is superior. The decreasing slope value (K) from stage 1 to stage 2 in the intra-particle diffusion model suggests that external mass transfer is the predominant phase. Moreover, the nanocomposite was successfully recycled without significantly decreasing the cyanide removal efficiency. Thus, the CuFe2O4-rGO–SnO2 nanocomposite could be an alternative adsorbent for removing cyanide-polluted wastewater.
Data availability
Data will be made available on request.
Author contributions
Soumya Mishra: investigation, methodology, formal analysis, validation, data curation, writing – original draft, writing – review & editing, Naresh Kumar Sahoo: conceptualization, visualization, investigation, supervision, resources, writing – original draft, formal analysis, funding acquisition. Prasanta Kumar Sahoo: conceptualization, investigation, formal analysis, visualization, review & editing. Satyanjib Sahoo: investigation, formal data curation, formal analysis, writing – review & editing. Lopamudra Nayak: investigation, formal analysis, writing – original draft. Prangya Ranjan Rout: conceptualization, investigation, formal analysis, visualization, review & editing.
Conflicts of interest
There are no conflicts to declare.
Acknowledgements
The authors acknowledge the financial support received from the Department of Biotechnology, Ministry of Science and Technology, Government of India, BT/PR15242/BCE/8/1144/2015 and SAN No. 102/IFD/SAN/3612/2016-2017, for conducting this research work.
References
- J. Mishra, D. S. Pattanayak, A. A. Das, D. K. Mishra, D. Rath and N. K. Sahoo, J. Mol. Liq., 2019, 287, 110821 CrossRef CAS.
- S. Bhattacharya, A. A. Das, G. C. Dhal, P. K. Sahoo, A. Tripathi and N. K. Sahoo, J. Environ. Manage., 2022, 302, 114022 CrossRef CAS PubMed.
- U. Pathak, P. Mogalapalli, D. D. Mandal and T. Mandal, Biomass Convers. Biorefin., 2021, 1–16 Search PubMed.
- D. S. Pattanayak, J. Mishra, J. Nanda, P. K. Sahoo, R. Kumar and N. K. Sahoo, J. Environ. Manage., 2021, 297, 113312 CrossRef CAS PubMed.
- P. Gupta, T. R. Sreekrishnan and Z. A. Shaikh, J. Environ. Manage., 2018, 226, 448–456 CrossRef CAS PubMed.
- S. K. Sahoo, S. Bhattacharya and N. K. Sahoo, Biointerface Res. Appl. Chem., 2020, 10, 5048–5060 CAS.
- S. C. Curry and M. B. Spyres, Critical Care Toxicology, 2015, 1–21 Search PubMed.
- E. Abdelkader, L. Nadjia and V. Rose-Noëlle, Int. J. Ind. Chem., 2016, 7, 53–70 CrossRef CAS.
- K. K. Kefeni, B. B. Mamba and T. A. M. Msagati, Sep. Purif. Technol., 2017, 188, 399–422 CrossRef CAS.
- Y. Zhao and L. Tao, Chin. Chem. Lett., 2024, 35, 108571 CrossRef CAS.
- Y. Zhang, X.-A. Teng, Z.-Q. Ma, R.-M. Wang, W.-M. Lau and A.-X. Shan, Rare Met., 2023, 42, 1836–1846 CrossRef CAS.
- B. Mary, J. J. Vijaya, M. Bououdina, L. Khezami, A. Modwi, M. Ismail, S. Bellucci and S. Rather, Adsorpt. Sci. Technol., 2022, 1–24 Search PubMed.
- X. Shen, D. Wu, X.-Z. Fu and J.-L. Luo, Chin. Chem. Lett., 2022, 33, 390–393 CrossRef CAS.
- Z.-J. Zhang, H.-Y. Sun, Y.-F. Chen, Y.-W. Zhao, M.-M. Zhang, C.-S. Li, Y. Sun, Z. H. Gao, H.-J. Li and Y. Jiang, Rare Met., 2023, 42, 4039–4047 CrossRef CAS.
- N. M. Mahmoodi and M. H. Saffar-Dastgerdi, Appl. Catal., B, 2020, 268, 118443 CrossRef CAS.
- M. Oveisi, M. A. Asli and N. M. Mahmoodi, Inorg. Chim. Acta, 2019, 487, 169–176 CrossRef CAS.
- R. Xu, W. Liu, J. Cai and Z. Li, Sep. Purif. Technol., 2021, 257, 117876 CrossRef CAS.
- K. Y. Kumar, T. N. V. Raj, S. Archana, S. B. B. Prasad, S. Olivera and H. B. Muralidhara, J. Water Process Eng., 2016, 13, 44–52 CrossRef.
- V. Bonu, A. Das, S. Amirthapandian, S. Dhara and A. K. Tyagi, Phys. Chem. Chem. Phys., 2015, 17, 9794–9801 RSC.
- S. Yu, J. Wang and J. Cui, Int. J. Biol. Macromol., 2020, 156, 1474–1482 CrossRef CAS PubMed.
- D. Dutta, S. Thiyagarajan and D. Bahadur, Chem. Eng. J., 2016, 297, 55–65 CrossRef CAS.
- G. Raja, S. Gopinath, R. A. Raj, A. K. Shukla, M. S. Alhoshan and K. Sivakumar, Phys. E, 2016, 83, 69–73 CrossRef CAS.
- P. Paramasivan and P. Venkatesh, Phys. E, 2016, 84, 258–262 CrossRef CAS.
- D. C. Onwudiwe, O. A. Oyewo, O. E. Ogunjinmi and O. Ojelere, S. Afr. J. Chem. Eng., 2021, 38, 21–33 Search PubMed.
- A. M. Stern, Am. J. Public Health, 2005, 95, 1128–1138 CrossRef PubMed.
- S. Kumari, M. K. Manglam, L. K. Pradhan, L. Kumar, J. P. Borah and M. Kar, Appl. Phys. A, 2021, 127, 1–13 CrossRef.
- D. Thapa, N. Kulkarni, S. N. Mishra, P. L. Paulose and P. Ayyub, J. Phys. D Appl. Phys., 2010, 43, 195004 CrossRef.
- R. Dhyani, R. C. Srivastava, P. S. Rawat and G. Dixit, Int. J. Mater. Res., 2022, 113, 884–892 CrossRef CAS.
- R. N. Mariammal, K. Ramachandran, B. Renganathan and D. Sastikumar, Sens. Actuators, B, 2012, 169, 199–207 CrossRef CAS.
- S. T. Nipa, R. Akter, A. Raihan, S. bin Rasul, U. Som, S. Ahmed, J. Alam, M. R. Khan, S. Enzo and W. Rahman, Environ. Sci. Pollut. Res., 2022, 29, 10871–10893 CrossRef CAS PubMed.
- A. Ayeshamariam, C. Sanjeeviraja and R. P. Samy, J. Photonics Spintron., 2013, 2, 4 Search PubMed.
- S. S. Mehta, D. Y. Nadargi, M. S. Tamboli, T. Alshahrani, V. R. Minnam Reddy, E. S. Kim, I. S. Mulla, C. Park and S. S. Suryavanshi, Sci. Rep., 2021, 11, 5023 CrossRef CAS PubMed.
- J. R. do Nascimento, M. R. D'Oliveira, A. G. Veiga, C. A. Chagas and M. Schmal, ACS Omega, 2020, 5, 25568–25581 CrossRef CAS PubMed.
- A. Meidanchi and H. Ansari, J. Cluster Sci., 2021, 32, 657–663 CrossRef CAS.
- S. Kanagesan, M. Hashim, S. AB Aziz, I. Ismail, S. Tamilselvan, N. B. Alitheen, M. K. Swamy and B. P. C Rao, Appl. Sci., 2016, 6, 184 CrossRef.
- R. Peymanfar, F. Azadi and Y. Yassi, Proceedings, 2018, 2, 1155 Search PubMed.
- P. A. Luque, O. Nava, C. A. Soto-Robles, H. E. Garrafa-Galvez, M. E. Martinez-Rosas, M. J. Chinchillas-Chinchillas, A. R. Vilchis-Nestor and A. Castro-Beltrán, J. Mater. Sci.: Mater. Electron., 2020, 31, 16859–16866 CrossRef CAS.
- N. Shahzad, N. Ali, A. Shahid, S. Khan and H. Alrobei, Digest J. Nanomater. Biostruct., 2021, 16, 41–4916 CrossRef.
- I. O. Faniyi, O. Fasakin, B. Olofinjana, A. S. Adekunle, T. V Oluwasusi, M. A. Eleruja and E. O. B. Ajayi, SN Appl. Sci., 2019, 1, 1–7 Search PubMed.
- D. Strankowski Michałand Włodarczyk, Ł. Piszczyk and J. Strankowska, et al., J. Spectrosc., 2016, 2016, 7520741–6 Search PubMed.
- M. Gupta, H. F. Hawari, P. Kumar and Z. A. Burhanudin, Crystals, 2022, 12, 264 CrossRef CAS.
- S. K. Sahoo, A. A. Das, D. Deka, B. Naik and N. K. Sahoo, J. Mol. Liq., 2021, 339, 116721 CrossRef CAS.
- E. M. Elsayed, M. M. Rashad, M. R. Hussein, M. M. B. El-Sabbah and I. A. Ibrahim, Renewable Energy Sustainable Dev., 2017, 3, 267–276 CrossRef.
- A. Shiri, A. Khorramabadi-zad, H. Bahiraei and F. Saeedian, Res. Chem. Intermed., 2022, 48, 1331–1345 CrossRef CAS.
- S. Asaithambi, P. Sakthivel, M. Karuppaiah, Y. Hayakawa, A. Loganathan and G. Ravi, Appl. Phys. A, 2020, 126, 1–12 CrossRef.
- J. Mayandi, M. Marikkannan, V. Ragavendran and P. Jayabal, J. Nanosci. Nanotechnol., 2014, 2, 707–710 Search PubMed.
- A. Raza, U. Qumar, J. Hassan, M. Ikram, A. Ul-Hamid, J. Haider, M. Imran and S. Ali, Appl. Nanosci., 2020, 10, 3875–3899 CrossRef CAS.
- A. Priyadarshini, S. Mishra, N. K. Sahoo, S. Raut, A. Daverey and B. C. Tripathy, Appl. Biochem. Biotechnol., 2023, 1–21 DOI:10.1007/s12010-023-04508-8.
- L. Yin, B. Hu, L. Zhuang, D. Fu, J. Li, T. Hayat, A. Alsaedi and X. Wang, Chem. Eng. J., 2020, 381, 122744 CrossRef CAS.
- L. Dolatyari, M. R. Yaftian and S. Rostamnia, J. Taiwan Inst. Chem. Eng., 2016, 60, 174–184 CrossRef CAS.
- D. Naidu, P. Pattanaik, A. A. Das and N. K. Sahoo, Int. J. Innov. Technol. Explor. Eng., 2019, 8, 316–323 Search PubMed.
- M. Z. Afzal, P. Zu, C.-M. Zhang, J. Guan, C. Song, X.-F. Sun and S.-G. Wang, J. Hazard. Mater., 2022, 434, 128879 CrossRef CAS PubMed.
- P. Pattanaik, N. Panigrahi, J. Mishra, N. K. Sahoo, B. P. Dash and D. Rath, J. Hazard., Toxic Radioact. Waste, 2018, 22, 4018001 CrossRef.
- M. R. Mahmoud, G. M. Rashad, A. M. Elewa, E. Metwally and E. A. Saad, J. Mol. Liq., 2019, 291, 111257 CrossRef CAS.
- K. Alizadeh, E. Khaledyan and Y. Mansourpanah, J. Water Environ. Nanotechnol., 2018, 3, 243–253 CAS.
- Y. Liu, L. Ting, Z. He, T. Li, W. Hui, X. Hu, Y. Guo and H. E. Yuan, Trans. Nonferrous Met. Soc. China, 2013, 23, 1804–1814 CrossRef CAS.
- K. Y. Koh, Z. Chen, S. Zhang and J. P. Chen, Chemosphere, 2022, 286, 131458 CrossRef CAS.
- F. Liu, W. Huang, S. Wang and B. Hu, Appl. Surf. Sci., 2022, 594, 153376 CrossRef CAS.
- N. Singh and C. Balomajumder, Appl. Water Sci., 2021, 11, 144 CrossRef CAS.
- S. Singh, M. Kaur, B. S. Bajwa and I. Kaur, J. Mol. Liq., 2022, 346, 117061 CrossRef CAS.
- Y. Yang, Y. Xie, L. Pang, M. Li, X. Song, J. Wen and H. Zhao, Langmuir, 2013, 29, 10727–10736 CrossRef CAS PubMed.
- O. A. A. Eletta, O. A. Ajayi, O. O. Ogunleye and I. C. Akpan, J. Environ. Chem. Eng., 2016, 4, 1367–1375 CrossRef CAS.
- A. Behnamfard and M. M. Salarirad, J. Hazard. Mater., 2009, 170, 127–133 CrossRef CAS PubMed.
- P. Eskandari, M. Farhadian, A. R. Solaimany Nazar and A. Goshadrou, Int. J. Environ. Sci. Technol., 2021, 18, 297–316 CrossRef CAS.
- H. S. Hassan, L. Attia and G. A. Dakroury, Appl. Radiat. Isot., 2020, 16109278 Search PubMed.
- Y. Huang, X. Lee, M. Grattieri, F. C. Macazo and R. Cai, et al., J. Mater. Sci., 2018, 53, 12641–12649 CrossRef CAS.
- M. Bhaumik, S. Agarwal, V. K. Gupta and A. Maity, J. Colloid Interface Sci., 2016, 470, 257–267 CrossRef CAS PubMed.
- C. S. Cheng, J. Deng, B. Lei, A. He, X. Zhang, L. Ma, S. Li and C. Zhao, J. Hazard. Mater., 2013, 263, 467–478 CrossRef CAS PubMed.
- K. Riahi, S. Chaabane and B. B. Thayer, J. Saudi Chem. Soc., 2017, 21, S143–S152 CrossRef CAS.
- A. A. Inyinbor, F. A. Adekola and G. A. Olatunji, Appl. Water Sci., 2017, 7, 3257–3266 CrossRef CAS.
- P. Zong, M. Shao, D. Cao, X. Xu, S. Wang and H. Zhang, Environ. Res., 2021, 196, 110375 CrossRef CAS PubMed.
- N. Masunga, O. K. Mmelesi, K. K. Kefeni and B. B. Mamba, J. Environ. Chem. Eng., 2019, 7(3), 103179 CrossRef CAS.
- J. W. Yoon, S. H. Choi, J. S. Kim, H. W. Jang, Y. C. Kang and J. H. Lee, NPG Asia Mater., 2016, 8(3), 244 CrossRef.
|
This journal is © The Royal Society of Chemistry 2024 |