DOI:
10.1039/D3NR05966A
(Communication)
Nanoscale, 2024,
16, 3438-3447
Unravelling abnormal in-plane stretchability of two-dimensional metal–organic frameworks by machine learning potential molecular dynamics†
Received
24th November 2023
, Accepted 15th January 2024
First published on 16th January 2024
Abstract
Two-dimensional (2D) metal–organic frameworks (MOFs) hold immense potential for various applications due to their distinctive intrinsic properties compared to their 3D analogues. Herein, we designed a highly stable NiF2(pyrazine)2 2D MOF in silico with a two-dimensional periodic wine-rack architecture. Extensive first-principles calculations and molecular dynamics (MD) simulations based on a newly developed machine learning potential (MLP) revealed that this 2D MOF exhibits huge in-plane Poisson's ratio anisotropy. This results in anomalous negative in-plane stretchability, as evidenced by an uncommon decrease in its in-plane area upon the application of uniaxial tensile strain, which makes this 2D MOF particularly attractive for flexible wearable electronics and ultra-thin sensor applications. We further demonstrated the unique capability of MLP to accurately predict the finite-temperature properties of MOFs on a large scale, exemplified by MLP-MD simulations with a dimension of 28.2 × 28.2 nm2, relevant to the length scale experimentally attainable for the fabrication of MOF films.
Introduction
Metal–organic frameworks (MOFs) have attracted substantial interest from both academic and industrial perspectives owing to their unique features such as high porosity, tunable structures and versatile functionalities.1–3 An almost infinite number of MOF architectures with different pore sizes/shapes and a wide range of chemical functionalities can thus be designed by assembling a rational selection of inorganic and organic secondary building units.4–6 Highly flexible MOFs have emerged as an intriguing sub-class of porous hybrid porous materials that undergo reversible/irreversible changes in their crystalline structures and pore dimensions (ligand flip, gating, swelling, and breathing) in response to external stimuli such as guest adsorption, temperature or mechanical pressure.7–10 Some of these flexible three-dimensional (3D) MOFs have demonstrated unusual mechanical properties, i.e., superhigh flexibility, negative gas adsorption, and negative linear compressibility (NLC) among others, which have promoted new applications of this family of materials in the fields of gas separation, sensors and micromechanics.8,11,12 Typically, the wine-rack MIL-53 built up of infinite trans chains of corner-sharing MO4(OH)2 octahedra (M = Al3+, Cr3+, etc.) and other related flexible MOFs have been computationally and experimentally proved to exhibit significant anisotropy in their Young's modulus and/or shear modulus at the origin of their anomalous mechanical properties.13–20
Despite the fact that an exhaustive list of mechanical studies have been reported on 3D MOFs over the last 20 years, much less attention has been paid to two-dimensional (2D) MOFs. One of the main reasons for this is that 2D MOFs only make up a tiny portion of all known MOFs; this is especially true for 2D MOFs with the wine-rack pore geometry, of which only a very small number of instances have been reported with a perfect square lattice (sql) topology.21,22 Compared to their 3D analogues, 2D materials generally exhibit higher strength and toughness due to the limited number of constitutive atomic layers.23 As a result, they can undergo plastic deformation under high strain without fracturing, making them more flexible, highly resilient, and capable of withstanding high stresses without breaking, and therefore they can be adjustable into a variety of shapes.24,25 Therefore, the design of 2D MOFs with a wine-rack topology is anticipated to lead to inherent flexibility and potentially unusual mechanical properties, making them promising candidates for a wide range of applications, including flexible electronics like wearable devices, sensors, and smart patches.24–27
In this context, a 2D NiF2(pyz)2 (where pyz stands for pyrazine) with 2D wine-rack topology was constructed in silico and its flexible behaviour was systematically investigated at different length scales by combining high-precision first-principles calculations and large-scale machine learning potential (MLP)-based molecular dynamics (MD) simulations. The MLP strategy has been shown to significantly enhance the efficiency of molecular simulations by using high-quality datasets from first-principles calculations to accurately predict material properties while reducing computational costs dramatically.28–30 However, developing high-quality MLPs for MOFs is still a great challenge because the training time increases exponentially with the increase of system size and the number of chemical species.28 To date, only a few MLPs for MOFs have been reported, exclusively for 3D MOFs, including UiO-66, MOF-5, ZIF-8, and MOF-74.31–35 Herein, systematic phonon spectrum calculations and first-principles MD simulations first revealed that the proposed 2D MOF material does not exhibit any imaginary phonon modes in the whole Brillouin zone and that it can maintain its structural integrity at room temperature as well as in an aqueous solution. Large-scale modelling of the MOF system, i.e. length scale >10 × 10 nm2 with over thousands of atoms and a time scale of 1 ns, largely beyond the realm of first-principles calculations, was further achieved by developing the first MLP for a 2D MOF that was implemented into a MD scheme. This novel MLP was first validated by a fair reproduction of the first-principles calculations performed on 2D NiF2(pyz)2, e.g. energy profiles, phonon properties and thermodynamics data. Its implementation into a MD-scheme enabled the exploration of the structural responsiveness of the 2D MOF to external tension strain at finite temperatures. The MLP-based MD simulations revealed an enormous in-plane flexibility of the 2D NiF2(pyz)2 structure (originating from the notable difference in Poisson's ratio along the different directions), with a resulting simulated fracture strain up to 19% even at room temperature, similar to the value reported previously for graphene.36 Decisively, we revealed an anomalous negative in-plane stretchability of 2D NiF2(pyz)2 upon uniaxial stretching both at 0 K and finite temperature. This intriguing phenomenon implies a counterintuitive decrease in the MOF in-plane area upon the application of a wide range of uniaxial stretching modes below the fracture strain. Such an abnormal mechanical response may have potential applications in wearable devices and flexible electronic material applications. Beyond the design of a novel 2D MOF material with unconventional mechanical properties, we further applied the well-trained MLP to a large-scale 2D NiF2(pyz)2 atomistic model (size ∼28.2 × 28.2 nm2 corresponding to 36
800 atoms) that reduces the gap between experimental and theoretical attainable length scales in the field of MOFs, which is of key importance to venture into phenomena yet to be discovered.
Results and discussion
Structure and stability of 2D NiF2(pyz)2 at the quantum level
We first built a structure with a metal node and a pyz linker arranged in a 2D array. Three initial atomistic models were considered corresponding to the distinct orientations of pyz and Ni2+ in ab planes with dihedral angles (∠N–Ni–N–C) of ±45° and 90° leading to different space group symmetries as shown in Fig. S1.† These 3 models were geometry optimized at the density functional theory (DFT) level and converged towards a perfect square planar geometry (tetragonal space group P4/mmm) as shown in Fig. S2.† The resultant 2D NiF2(pyz)2 geometry, depicted in Fig. 1a, consists of pyz moieties coordinated to two Ni atoms via their opposite N atoms. Each Ni atom is coordinated to four pyz moieties and two fluorine atoms, featuring a perfect 2D sql topology with a = b = 7.061 Å and α = β = γ = 90° as defined at the DFT+U37 level (cf. Table S1† for the lattice parameters simulated by using other functionals). The proposed 2D NiF2(pyz)2 structure exhibits a pattern resembling a wine-rack motif that has been only rarely reported so far for 2D MOFs.21,22,38,39 We then computed the formation energy using the formula: Ef = EMOF − (2Epyz + ENiF2), where EMOF, ENiF2, and Epyz represent the total energies of the 2D NiF2(pyz)2, NiF2 cluster, and pyz ligand, respectively, to assess the stability of the proposed structure. The calculated formation energy of 2D NiF2(pyz)2 is −5.47 eV per f.u., which is comparable to or lower than that of Cr(pyz)2 and Cr(diarse)2 monolayers (−4.18–−5.57 eV per f.u.) reported before.40 The negative formation energy value indicates the energetically favorable formation of the structure. Therefore, the even lower formation energy suggests that 2D NiF2(pyz)2 can be synthesized.
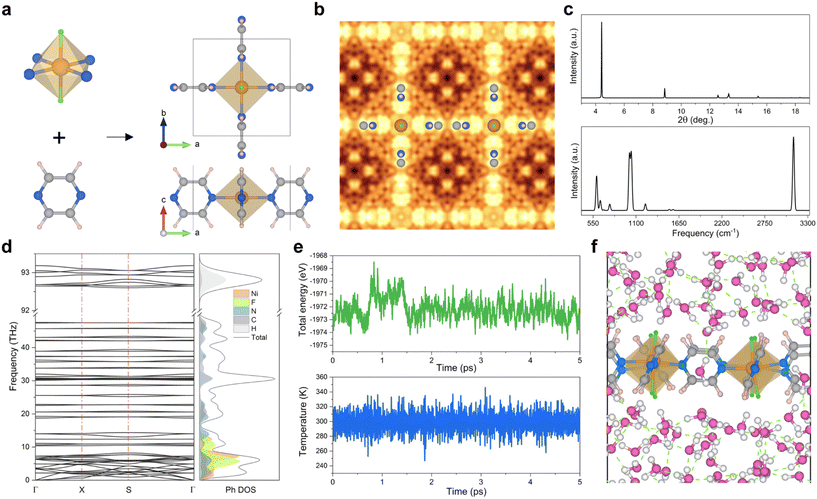 |
| Fig. 1 (a) Side and top views of the DFT+U-geometry optimized structure of 2D NiF2(pyz)2. Ni is shown in orange, N in blue, F in green, H in light pink, and C in dark-gray. The black line delimits the unit cell. (b) Simulated STM image of the DFT+U-geometry optimized 2D NiF2(pyz)2, by using the constant height mode at Vbias = 1.5 V. The corresponding atoms are also superimposed on the STM image with the same color code as in (a). (c) Simulated XRD pattern (top) and the Raman spectrum (bottom) of 2D NiF2(pyz)2. (d) Simulated phonon spectrum and the corresponding phonon density of state (Ph DOS) for 2D NiF2(pyz)2. (e) Evolution of the total energy (top) and temperature (bottom) obtained during the AIMD simulations for 2D NiF2(pyz)2 (2 × 2 supercell) in an aqueous solution. AIMD simulations conducted for 5 ps in the NVT ensemble (T = 300 K) with a timestep of 0.5 fs. (f) Side view of a representative snapshot of 2D NiF2(pyz)2 in an aqueous solution at 300 K after 5 ps-AIMD simulations. The color code of the atoms is the same as described before. Water molecules in the snapshot are marked by magenta (O) and white (H). The green dashed line represents hydrogen bonding interactions between water molecules. | |
To gain more structural details of 2D NiF2(pyz)2, we employed the Tersoff and Hamann approximation41 in constant height mode to simulate its scanning tunneling microscopy (STM) image, as depicted in Fig. 1b. This approach mimics the standard experimental setup applied to collect STM images of MOFs,42 delivering a precise description of the structure at the atomic level. The simulated STM image of NiF2(pyz)2 reveals a symmetrical square grid shape, resembling the 3D Nb-OFFIVE-Ni MOF along the [001] direction.43 Typically, the theoretical X-ray diffraction (XRD) pattern alongside the simulated Raman spectrum of 2D NiF2(pyz)2 is provided in Fig. 1c, to assist in the identification of such a 2D MOF in future experimental studies.
The dynamic and thermal stabilities of the resulting 2D NiF2(pyz)2 are key features prior to considering further applications. To evaluate these stabilities, high-precision calculations of the phonon spectrum were performed based on ab initio molecular dynamics (AIMD) simulations at 300 K. Notably, there is no observable imaginary frequency present in the phonon dispersion curves as shown in Fig. 1d, suggesting that 2D NiF2(pyz)2 is dynamically stable. The peak associated with the highest frequency at around 94.5 THz (∼3118 cm−1) corresponds to the vibrational stretching mode of the C–H bond (Movies S1–S3†), while the frequency of the metal node is mainly localized in the low frequency range (below 15 THz). AIMD simulations were further performed at 300 K for 10 picoseconds (ps) to further assess the thermal stability of 2D NiF2(pyz)2 under ambient conditions. The resulting energy fluctuation of the system over time, as shown in Fig. S3,† is very limited, suggesting that the 2D NiF2(pyz)2 structure remains intact without undergoing reconstruction during the simulation period. Therefore, 2D NiF2(pyz)2 exhibits both dynamic and thermal stabilities. Another important requirement for potential applications is the hydrolytic stability of the designed 2D NiF2(pyz)2. While many MOFs may not have long-term water stability,44 it is critical to investigate the structural integrity of 2D NiF2(pyz)2 in aqueous media using AIMD simulations. As shown in Fig. 1e and f, the resulting simulations performed in water at 300 K demonstrated that both temperature and total energy remained constant during the AIMD calculations, and analysis of the 2D NiF2(pyz)2 frameworks over the overall AIMD simulations evidences the absence of structural change, supporting the high robustness of 2D NiF2(pyz)2 (notes and Fig. S4† for details).
MLP training and validation for 2D NiF2(pyz)2
The target MLP was trained on the energy and force determined at the DFT level for 2D NiF2(pyz)2. We applied a variety of strategies, e.g. static calculations with different supercells, AIMD simulations at different temperatures, and datasets with the application of different stresses, to expand the diversity of the structure space (cf. ESI notes, Fig. S5 and Table S2†). To further validate the accuracy of the trained MLP, the energies and forces were calculated for a test set of 1000 structures initially generated by AIMD at 300 K and compared with the values obtained at the DFT level. The accuracy of the trained MLP is demonstrated by the nearly linear fit of the predicted MLP-derived energies (forces) versus the DFT-calculated energies (forces), as shown in Fig. 2a and b, respectively. The root mean square errors for the MLP-derived energy and force are 0.083 meV per atom and 0.013 eV Å−1, respectively. This result reveals the remarkable accuracy of the developed MLP in capturing the properties of the trained systems. The strain–energy relationship of 2D NiF2(pyz)2 was calculated for further validation of the trained MLP, as an accurate description of the energies under strain conduction is essential to avoid unphysical phase dissociation/reconstruction during the simulations. As depicted in Fig. 2c, our findings proved that the trained MLP describes very well the energetics of the strained phases referring to DFT data, suggesting that the trained MLP can be effectively applied to configurations not included in the training set. Fig. 2d shows that the phonon spectrum predicted by the MLP approach is in good accordance with the reference data obtained at the DFT level using the density functional perturbation theory method.45 The phonon density of states (Ph DOS) is also predicted accurately with peak positions well reproduced (Fig. 2e). This demonstrates that our MLP model predicts well not only pair potentials and forces but also collective phonon dynamics of the lattice. Thermal properties, such as the Helmholtz free energy and the specific heat capacity (CV), computed using the phonon relationships reproduced also very well the corresponding DFT data, as shown in Fig. 2f. Notably, our MLP was trained with AIMD simulations performed in the temperature range of 100–300 K, but we showed that it can still accurately predict thermal properties beyond room temperature, demonstrating its ability to anticipate properties over a wide range of temperatures accurately.
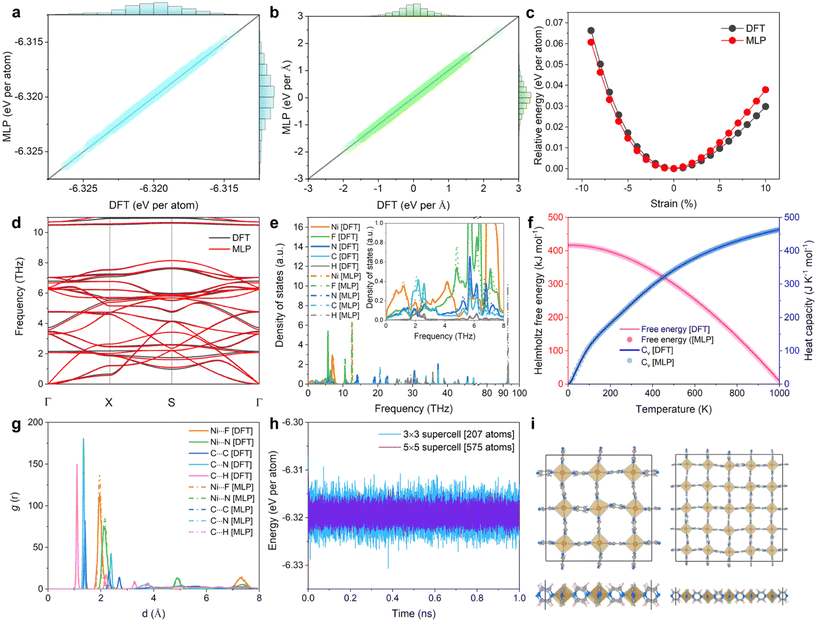 |
| Fig. 2 MLP-derived (a) energies and (b) forces vs. the corresponding DFT values. (c) Strain–energy curves for 2D NiF2(pyz)2 simulated using MLP and DFT calculations. (d) Phonon dispersion of 2D NiF2(pyz)2 computed using MLP and DFT calculations (for clarity only dispersion curves below 11 THz are displayed). (e) Phonon density of states (Ph DOS) computed using the DFT and MLP calculations, where the inset highlights the low frequency region. (f) Helmholtz free energy and specific heat capacity (Cv) computed using the DFT and MLP calculations as a function of temperature. (g) Radial distribution functions (RDFs) calculated for different atom pairs of 2D NiF2(pyz)2 at 300 K computed using MLP and AIMD, respectively. (h) Total energy fluctuations of 2D NiF2(pyz)2 during the MLP-MD simulations at 300 K with 3 × 3 and 5 × 5 supercells. (i) Snapshots of the MLP-MD-derived NiF2(pyz)2 with 3 × 3, (left) and 5 × 5 (right) supercells containing 207 and 575 atoms respectively after 1 ns MD simulation. Ni, F, N, C, and H atoms are marked with orange, green, blue, dark-gray, and light-pink, respectively. | |
We further evaluated the capability of the trained MLP to describe the lattice dynamics of the structure at finite temperatures (from 100 to 300 K). AIMD and MLP-MD simulations using the Nosé–Hoover thermostat46 were thus performed for 10 ps and 1.0 ns, respectively. The excellent agreement between the radial distribution functions (RDFs) calculated for different atom pairs of 2D NiF2(pyz)2 from both simulations at 300 K (see Fig. 2g) revealed the accuracy of our MLP in describing the structural behavior of 2D NiF2(pyz)2 at finite temperatures. It should be emphasized here that AIMD simulations for MOFs are computationally expensive and usually run for only a few ps.47 However, as evidenced in Fig. 2g and h, based on a well-trained MLP, we can scale up the AIMD simulations to the ns scale, while maintaining an accurate description of the structure. We demonstrated that MLP trained using a relatively small lattice for NiF2(pyz)2 (i.e., a 3 × 3 supercell with 207 atoms) is transferable to an accurate exploration of the structural properties of a larger system (i.e., 5 × 5 with 575 atoms) as shown in Fig. 2h and i. Therefore, given the high accuracy and good transferability of our MLP in capturing the interatomic forces of the 2D NiF2(pyz)2 overall system, one can expect to accurately predict the physical properties of this 2D MOF derived from the calculated force constants.
Anisotropic in-plane mechanical properties of 2D NiF2(pyz)2
Fig. 3a predicts the highly anisotropic in-plane flexibility of 2D NiF2(pyz)2 once applying uniaxial tensile strain is applied, which is unique for such a 2D-wine-rack topology. To gain insight into this intriguing behaviour, the ideal tensile strength and critical strain were further explored by applying uniaxial strain along the Γ–M and Γ–X directions (cf. Fig. S7† for the definition of the strain directions), as shown in Fig. 3b. The uniaxial strain is defined as ε = (a − a0)/a0, where a0 and a are the lattice parameters of the original and strained 2D NiF2(pyz)2, respectively. For each applied strain, the lattice is also relaxed along the direction normal to the layer during the MLP-geometry optimization to ensure that the forces are minimal. The resulting strain–stress relationship is shown in Fig. 3c. The 2D NiF2(pyz)2 structure exhibits ideal strengths of 13 N m−1 and 23 N m−1 in the Γ–X and Γ–M directions, respectively, with critical strains of 12% (Γ–X) and 40% (Γ–M). Evidently, the critical strain along the Γ–M direction is three times higher than that along the Γ–X direction, indicating significant in-plane anisotropy of the mechanical properties of 2D NiF2(pyz)2.
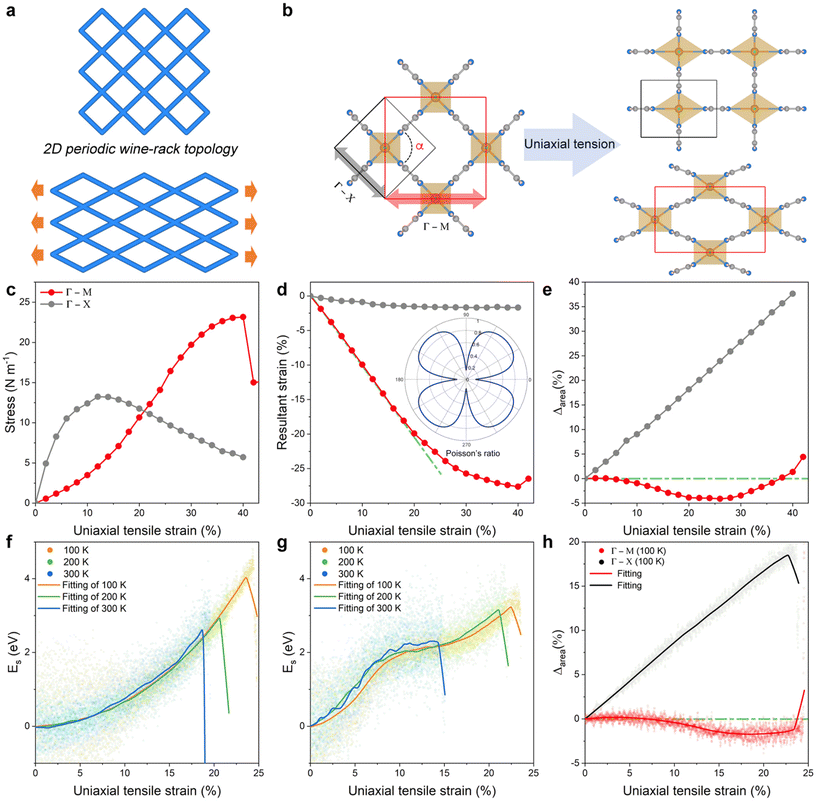 |
| Fig. 3 (a) Illustration of the deformation of the 2D periodic wine-rack structure upon applying uniaxial tensile strain. The blue stick is analogous to the ligand in 2D NiF2(pyz)2, while the intersection of the hinges is the location of the metal nodes. (b) Schematic representation of 2D NiF2(pyz)2 with uniaxial strain applied along different directions (Γ–X and Γ–M according to the Brillouin zone of the lattice) of the plane. Color code: Ni: orange; N: blue; C: dark-gray; F: green; H: light pink. (c) Stress–strain responses of 2D NiF2(pyz)2 upon applying strains. (d) Resultant strain induced by the uniaxial strain along the Γ–X and Γ–M directions, respectively. The inset shows the polar diagrams for Poisson's ratio of 2D NiF2(pyz)2. (e) Relative in-plane area changes (Δarea, %) under a strain deformation along the two different directions. MLP-MD derived strain–energy (Es) curves along the (f) Γ–M and (g) Γ–X directions upon applying uniaxial tensile strain at different temperatures (100, 200, and 300 K). Es is defined as Es = Estrain − E0, where Estrain is the total energy of the strained system and E0 is the total energy of the original system. (h) MLP-MD derived Δarea upon a strain deformation applied along the two different directions at 100 K. The colored dots represent the real MLP-MD simulation data points, and the solid lines represent the corresponding fitted data. | |
Fig. 3d and Fig. S6† show that the resultant strain (with Poisson's ratio) and shear modulus along the Γ–M direction are ∼6 and ∼20 times higher than those along the Γ–X direction, respectively, in line with the very large in-plane anisotropy of the mechanical properties of 2D NiF2(pyz)2. Such anisotropy is a signature of a substantial difference in terms of flexibility of NiF2(pyz)2 along different directions. It should be noted that the Poisson's ratios calculated from the linear fits of the resultant strain curve are consistent with those calculated using the elastic constant method, as shown in Fig. S8.† The resulting difference of 0.72 (0.14 along the Γ–X direction and 0.86 along the Γ–M direction) is considerably larger than those reported for other systems including tetraoxa[8]circulene-based COFs (0.04–0.384),48 Fe2(TCNQ)2 MOF (0.41),49 and M3(C6X6)2 (M = Co, Cr, Cu, Fe, Mn, Ni, Pd, Rh and X = O, S, Se) MOF (0–0.04),50 that highlight an ultra-high in-plane flexibility of 2D NiF2(pyz)2. Such ultra-high in-plane Poisson's ratio combined with high anisotropy makes 2D NiF2(pyz)2 a good candidate for practical large-magnitude strain engineering.51
Negative in-plane stretchability of 2D NiF2(pyz)2
Typically, when an in-plane tension strain (below the fracture strain) is applied to a 2D crystal, it will elongate in the direction of the applied tension and shrink on the other side, while resulting in an increase in the change of the in plane-area (Δarea). This conclusion remains valid even for some materials with negative Poisson's ratio, called auxetic materials.52,53 In this respect, 2D-NiF2(pyz)2 exhibits a standard behaviour with a DFT-predicted increase of Δarea when a stretching is applied along the Γ–X direction (Fig. 3e). However, the situation is reversed when the stretching is applied along the Γ–M direction with 2D NiF2(pyz)2 predicted to show an anomalous decrease of Δarea as shown in Fig. 3e. In this later case, even at >30% strain, Δarea still decreases. This unexpected phenomenon originates from the very high anisotropic in-plane flexibility of this 2D wine-rack MOF featuring high Poisson's ratio and high shear modulus along the Γ–M direction (cf. Fig. S8†). As depicted in Fig. S9,† when the material is stretched, i.e. 24% along the direction of uniaxial tensile strain applied, the lattice parameters become significantly shorter on the opposite side along the Γ–M direction, i.e. a decrease of 20% and 1.8% along the Γ–M and Γ–X directions, respectively, leading to a decrease of Δarea. We further revealed that this variation is primarily due to structural changes occurring at the connections between the ligand and the metal node (namely Ni–N bonds), while the strain has almost no impact on the pyz ligand both in the Γ–X and Γ–M directions (cf. Fig. S10 and S11†). These conclusions were drawn from DFT calculations performed at 0 K, while experimental nanoindentation measurements are usually performed at finite temperatures. However, incorporating the temperature effect into DFT calculations to investigate the structural changes of materials under strain is theoretically highly challenging. Interestingly, we revealed above that our MLP-MD simulations performed at finite temperatures were also able to capture the same trend as that obtained by DFT at 0 K (Movies S5 and S6†). Fig. 3f and g show that in the temperature range 100–300 K, the MLP-derived energy of the structure increases more rapidly along the Γ–X direction than that along the Γ–M direction, as the applied uniaxial tensile strain increases, in line with the DFT calculations. In addition, we found that even at 300 K, 2D-NiF2(pyz)2 still has a high fracture strain of 19% (14%) along the Γ–M ( Γ–X) directions, indicating its exceptional flexibility under finite temperature conditions (see comparison with other 2D materials in Fig. S12†). We also observed the negative in-plane stretchability phenomenon in the Δarea calculation at 100 K, as shown in Fig. 3h. At the same time, such a phenomenon gradually becomes imperceptible as the temperature rises, as shown in Fig. S13.† This is because the free-energy landscape of transitions typically gets smoother with increasing temperature and the probability of being trapped in local minima decreases. However, the material still exhibits a noticeably different response to tension changes along the Γ–X and Γ–M directions, even at 300 K. Therefore, both DFT and MLP-MD simulations demonstrated the unique negative in-plane stretchability phenomenon exhibited by the 2D NiF2(pyz)2 structure.
Large-scale MLP applied to extended 2D NiF2(pyz)2 MOF models
Our MLP strategy was applied to a 2D NiF2(pyz)2 model with moderate size (up to a 5 × 5 supercell with 575 atoms, ∼3.5 × 3.5 nm2); however, the dimensions of experimentally prepared 2D films are generally above 10 × 10 nm2. Therefore, we extended the use of our MLP to 2D NiF2(pyz)2 models of much larger size, from 7.1 × 7.1 (2300 atoms) to 28.2 × 28.2 (36
800 atoms) nm2, as described in Fig. 4a–f. It is clear that no energy jump was detected in the energy fluctuations of the 2D MOF framework over the whole simulation time-scale (100 ps, as shown in Fig. 4a–c), whatever the dimension of the system considered, while the out-of-plane displacements of the structure tend to become apparent as the system size increases. We also evidenced that the 2D MOF containing >10
000 atoms reaches its equilibrium state only after ∼20 ps of MLP MD simulations, which is almost unattainable in AIMD calculations with this size of the system. Fig. 4g and h show the RDFs calculated for different atom pairs of 2D NiF2(pyz)2 MOF models from the MLP-MD simulations performed on the 28.2 × 28.2 nm2 model that compare very well with the AIMD-calculated profile on the small 2.0 × 2.0 nm2 model. The MLP-MD simulated angular distribution function (ADF) reported in Fig. 4i also shows a single peak at ∼90°, consistent with the angles formed by the pzy ligand and Ni metal in the pristine 2D NiF2(pyz)2 configuration. The atoms are more mobile at a higher temperature, resulting in slightly broader features in the RDF and ADF at 300 K compared to those calculated at 100 and 200 K, but there is no quantitative change between these different temperature settings, and the MLP-based simulations reproduce all features obtained by AIMD in the small system. All these results demonstrated the effectiveness and accuracy of our derived MLP in handling a large 2D NiF2(pyz)2 system.
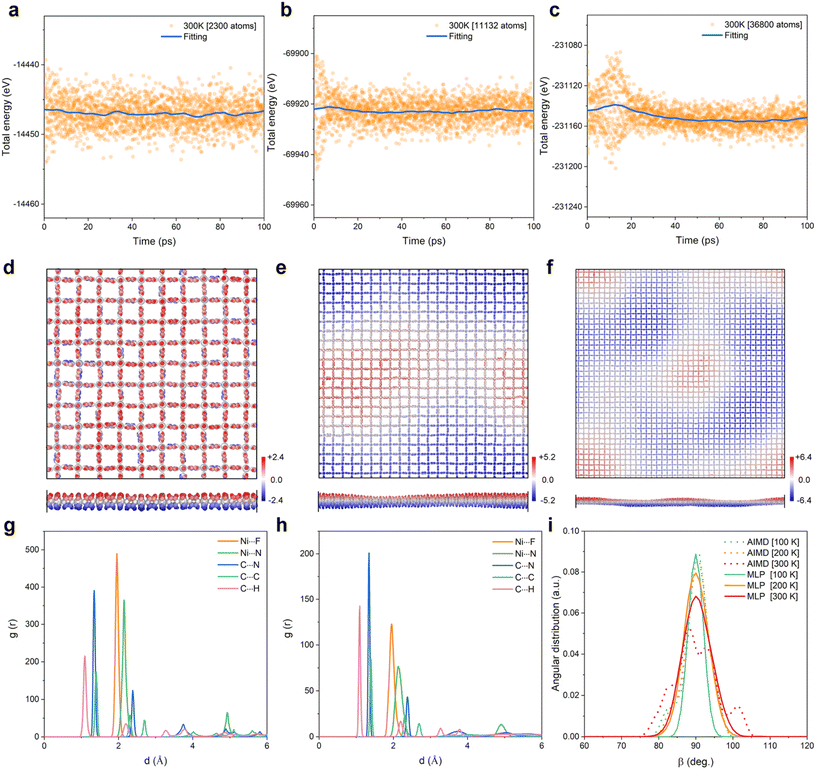 |
| Fig. 4 MLP-MD simulated fluctuation of the total energy of 2D NiF2(pzy)2 with different sizes and a simulation time of 100 ps at 300 K, (a) 7.1 × 7.1 nm2, (b) 15.5 × 15.5 nm2, and (c) 28.2 × 28.2 nm2, respectively. (d–f) The corresponding color coding represents the out-of-plane displacement in each system. The black boxes show the boundary of the periodic cell. Radial distribution functions (RDF) for different atom pairs of 2D NiF2(pzy)2 at 300 K computed via (g) MLP-MD (28.2 × 28.2 nm2) and (h) AIMD (2.0 × 2.0 nm2) simulations in the NVT ensemble. (i) ADF (∠αN–Ni–N) of 2D NiF2(pzy)2 at different temperatures from MLP-MD and AIMD simulations in the same 2D NiF2(pzy)2 systems as described in (g and h). The slightly more “jagged” appearance of the AIMD data in panel i is due to the smaller number of configurations that are sampled from the MD trajectory compared to MLP data. | |
Conclusions
In conclusion, we proposed and in-depth explored a super-flexible 2D MOF with wine-track architecture, 2D NiF2(pyz)2, by combining systematic first-principles calculations and MLP-large-scale MD simulations. The mechanical, thermal and water stabilities of 2D NiF2(pyz)2 were first evidenced through systematic phonon spectrum AIMD calculations. Interestingly, benefitting from the unique 2D wine-rack motif, 2D NiF2(pyz)2 was shown to exhibit anisotropic in-plane flexibility with a fracture strain of up to 40% (0 K) and 17% even at room temperature, suggesting that this material can be used for wearable devices and flexible sensors. We also identified a counterintuitive negative in-plane stretchability phenomenon in 2D NiF2(pyz)2, whereby the surface area decreases with the applied tensile strain. Our systematic theoretical investigation showed that this behaviour originates from its unique 2D sql topology and anisotropic Poisson's ratio. Finally, we demonstrated that well-trained MLP can yield nanosecond MD simulations while maintaining first-principles accuracy and can scale the MD simulation to more than tens of thousands of atoms. We believe that the multi-scale computational approach presented here can greatly accelerate the theoretical design and understanding of novel flexible 2D MOF materials.
Methods
First-principles calculations
The Vienna ab initio simulation package54 (VASP, version: 5.4.4) was used to perform structural relaxation and total energy calculations with an energy cut-off of 900 eV (see more information regarding convergence testing in the ESI†). Exchange–correlation potentials were treated within the generalized gradient approximation (GGA) employing the Perdew–Burke–Ernzerhof (PBE) functional.55 The density functional theory (DFT) calculations used 6 × 6 × 1 G-centered Monkhorst–Pack k-point sampling.56 The DFT+U (U = 6.4 eV) scheme37 was used to account for the strong correlation of an unfilled d orbital of the Ni atom. The spin polarization was fully considered for all calculation processes unless specifically mentioned. A 300 K AIMD simulation was run in the NVT (canonical ensemble) employing the Nosé–Hoover thermostat46 to check the stability of the 2D NiF2(pyz)2 structure at room temperature.
Dataset preparation
To obtain training data for all the MLPs presented in this paper, finite temperature ab initio molecular dynamics (AIMD) simulations based on DFT were performed using the VASP.54 The PAW method and the GGA of the PBE type for the exchange–correlation functional were used during the AIMD simulations. The simulations consisted of more than 10
000 time steps with a time step of 0.5 fs in the 3 × 3 supercell. Brillouin zone sampling was performed using a Monkhorst–Pack k-point grid size of 1 × 1 × 1. All AIMD simulations were conducted in the NVT (canonical ensemble) employing the Nosé–Hoover thermostat46 with fixed temperatures of 100 K, 200 K and 300 K.
MLP development
A deep neural network based approach was utilized to develop a MLP for the proposed NiF2(pyz)2 by using the DeePMD-kit package (version 2.0.1).57 A total of 14
788 snapshots were collected, with 13
788 used for MLP training and 1000 used for MLP validation. The DeepPot-SE model was used to train the MLP using the DeePMD-kit code.57,58 The model contains two networks: the embedding network and the fitting network. Both networks utilize the ResNet architecture.59 The size of the embedding network was set to {25, 50, 100}. The size of the fitting network was set to {240, 240, 240}. The cutoff radius was set to 6.0 Å and the smoothing parameter was set to 4.9 Å. It should be noted that the choice of cutoff in the MLP training is important and significantly affects the speed of training and the accuracy of the trained MLP, and we point out here that our choice of cutoff of 6 Å is sufficient for our 2D system to contain sufficient many-body information between adjacent atoms.60,61 Throughout the training, we employed three layers of NN, where each layer consisted of 240 neurons. The loss is defined by:
where Δ means the difference between the MLP results and the training data during the training process, ε is the energy per atoms, N is the total number of atoms, Fi is the force of atom i, and η is the tensor divided by N. The number of batches and the steps of decay were set to 1
000
000 and 5000, respectively. The initial learning rate was set to 0.001 and decays every 5000 steps. The number of batches was set to 1
000
000.
MLP-MD simulations
The MLP obtained from the training process was utilized for MLP-MD simulations using the DeepMD-kit interface with the LAMMPS code.57,62 To compute energy and force during MD simulations, the trained model was used as a pair style in LAMMPS.62 We performed 1 ns long-time simulations in two systems, one system consistent with the AIMD simulation and one larger model initially, namely 3 × 3 and 5 × 5 supercells. To examine the applicability of our trained MLP, we conducted large-scale molecular dynamics simulations for three large systems (including 2300, 11
132, and 36
800 atoms, respectively) of 100 ps length. MLP-MD simulations were executed within the NVT ensemble with the Nosé–Hoover thermostat46 at 300 K. The time step was set to 0.5 fs and the periodic boundary conditions were employed along all three dimensions in the simulations. Two python-based tools, Phonopy and phonoLAMMPS,63,64 were utilized to compute phonons for the 2D-NiF2(pyz)2. The phonon bands for a 2 × 2 supercell were calculated with a consistent Brillouin-zone phonon sampling of a 22 × 22 mesh. The path inside the Brillouin zone was from Γ (0.0, 0.0, 0.0) to X (0.5, 0.0, 0.0) to S (0.5, 0.5, 0.0) to Γ (0.0, 0.0, 0.0). VESTA and OVITO packages65,66 were employed to plot the atomistic results.
Author contributions
D. F. and G. M. designed the research. D. F. carried out all the simulations with the help of A. O. and P. L. D. F., A. O., P. L., and G. M. wrote the manuscript. G. M. supervised the research.
Conflicts of interest
There are no conflicts to declare.
Acknowledgements
The computational work was performed using HPC resources from GENCI-CINES (grant A0140907613).
References
- H. Furukawa, K. E. Cordova, M. O'Keeffe and O. M. Yaghi, Science, 2013, 341, 1230444 CrossRef PubMed
.
- J.-B. Lin, T. T. T. Nguyen, R. Vaidhyanathan, J. Burner, J. M. Taylor, H. Durekova, F. Akhtar, R. K. Mah, O. Ghaffari-Nik, S. Marx, N. Fylstra, S. S. Iremonger, K. W. Dawson, P. Sarkar, P. Hovington, A. Rajendran, T. K. Woo and G. K. H. Shimizu, Science, 2021, 374, 1464–1469 CrossRef CAS PubMed
.
- L. S. Xie, G. Skorupskii and M. Dincă, Chem. Rev., 2020, 120, 8536–8580 CrossRef CAS PubMed
.
- O. M. Yaghi, M. O'Keeffe, N. W. Ockwig, H. K. Chae, M. Eddaoudi and J. Kim, Nature, 2003, 423, 705–714 CrossRef CAS PubMed
.
- O. M. Yaghi and Z. Rong, Science, 2023, 379, 330–331 CrossRef CAS
.
- H. Jiang, D. Alezi and M. Eddaoudi, Nat. Rev. Mater., 2021, 6, 466–487 CrossRef CAS
.
- A. Schneemann, V. Bon, I. Schwedler, I. Senkovska, S. Kaskel and R. A. Fischer, Chem. Soc. Rev., 2014, 43, 6062–6096 RSC
.
- S. Krause, V. Bon, I. Senkovska, U. Stoeck, D. Wallacher, D. M. Többens, S. Zander, R. S. Pillai, G. Maurin, F.-X. Coudert and S. Kaskel, Nature, 2016, 532, 348–352 CrossRef CAS PubMed
.
- J. H. Lee, S. Jeoung, Y. G. Chung and H. R. Moon, Coord. Chem. Rev., 2019, 389, 161–188 CrossRef CAS
.
- S. Horike, S. Shimomura and S. Kitagawa, Nat. Chem., 2009, 1, 695–704 CrossRef CAS PubMed
.
- L. R. Redfern and O. K. Farha, Chem. Sci., 2019, 10, 10666–10679 RSC
.
- F.-X. Coudert and J. D. Evans, Coord. Chem. Rev., 2019, 388, 48–62 CrossRef CAS
.
- P. Serra-Crespo, E. Stavitski, F. Kapteijn and J. Gascon, RSC Adv., 2012, 2, 5051 RSC
.
- P. Serra-Crespo, A. Dikhtiarenko, E. Stavitski, J. Juan-Alcañiz, F. Kapteijn, F.-X. Coudert and J. Gascon, CrystEngComm, 2015, 17, 276–280 RSC
.
- A. U. Ortiz, A. Boutin, A. H. Fuchs and F.-X. Coudert, Phys. Rev. Lett., 2012, 109, 195502 CrossRef PubMed
.
- A. U. Ortiz, A. Boutin, A. H. Fuchs and F.-X. Coudert, J. Chem. Phys., 2013, 138, 174703 CrossRef PubMed
.
- I. Senkovska, V. Bon, L. Abylgazina, M. Mendt, J. Berger, G. Kieslich, P. Petkov, J. Luiz Fiorio, J. Joswig, T. Heine, L. Schaper, C. Bachetzky, R. Schmid, R. A. Fischer, A. Pöppl, E. Brunner and S. Kaskel, Angew. Chem., Int. Ed., 2023, e202218076 CAS
.
-
J.-C. Tan, Mechanical Behaviour of Metal–Organic Framework Materials, Royal Society of Chemistry, 2023 Search PubMed
.
- L. Vanduyfhuys, S. M. J. Rogge, J. Wieme, S. Vandenbrande, G. Maurin, M. Waroquier and V. Van Speybroeck, Nat. Commun., 2018, 9, 204 CrossRef CAS
.
- J. Wieme, K. Lejaeghere, G. Kresse and V. Van Speybroeck, Nat. Commun., 2018, 9, 4899 CrossRef CAS
.
- K. S. Pedersen, P. Perlepe, M. L. Aubrey, D. N. Woodruff, S. E. Reyes-Lillo, A. Reinholdt, L. Voigt, Z. Li, K. Borup, M. Rouzières, D. Samohvalov, F. Wilhelm, A. Rogalev, J. B. Neaton, J. R. Long and R. Clérac, Nat. Chem., 2018, 10, 1056–1061 CrossRef CAS PubMed
.
- P. Perlepe, I. Oyarzabal, A. Mailman, M. Yquel, M. Platunov, I. Dovgaliuk, M. Rouzières, P. Négrier, D. Mondieig, E. A. Suturina, M.-A. Dourges, S. Bonhommeau, R. A. Musgrave, K. S. Pedersen, D. Chernyshov, F. Wilhelm, A. Rogalev, C. Mathonière and R. Clérac, Science, 2020, 370, 587–592 CrossRef CAS
.
- H. Zhang, ACS Nano, 2015, 9, 9451–9469 CrossRef CAS PubMed
.
- D. Akinwande, N. Petrone and J. Hone, Nat. Commun., 2014, 5, 5678 CrossRef CAS PubMed
.
- S. Pinilla, J. Coelho, K. Li, J. Liu and V. Nicolosi, Nat. Rev. Mater., 2022, 7, 717–735 CrossRef
.
- G. Fiori, F. Bonaccorso, G. Iannaccone, T. Palacios, D. Neumaier, A. Seabaugh, S. K. Banerjee and L. Colombo, Nat. Nanotechnol., 2014, 9, 768–779 CrossRef CAS PubMed
.
- H. Jiang, L. Zheng, Z. Liu and X. Wang, InfoMat, 2020, 2, 1077–1094 CrossRef CAS
.
- O. T. Unke, S. Chmiela, H. E. Sauceda, M. Gastegger, I. Poltavsky, K. T. Schütt, A. Tkatchenko and K.-R. Müller, Chem. Rev., 2021, 121, 10142–10186 CrossRef CAS PubMed
.
- G. Carleo, I. Cirac, K. Cranmer, L. Daudet, M. Schuld, N. Tishby, L. Vogt-Maranto and L. Zdeborová, Rev. Mod. Phys., 2019, 91, 045002 CrossRef CAS
.
- P. Friederich, F. Häse, J. Proppe and A. Aspuru-Guzik, Nat. Mater., 2021, 20, 750–761 CrossRef CAS PubMed
.
- S. K. Achar, J. J. Wardzala, L. Bernasconi, L. Zhang and J. K. Johnson, J. Chem. Theory Comput., 2022, 18, 3593–3606 CrossRef CAS PubMed
.
- M. Eckhoff and J. Behler, J. Chem. Theory Comput., 2019, 15, 3793–3809 CrossRef CAS PubMed
.
- B. Zheng, F. L. Oliveira, R. Neumann Barros Ferreira, M. Steiner, H. Hamann, G. X. Gu and B. Luan, ACS Nano, 2023, 17, 5579–5587 CrossRef CAS PubMed
.
- Y. Shaidu, A. Smith, E. Taw and J. B. Neaton, PRX Energy, 2023, 2, 023005 CrossRef
.
- P. Ying, T. Liang, K. Xu, J. Zhang, J. Xu, Z. Zhong and Z. Fan, Appl. Mater. Interfaces, 2023, 15, 36412–36422 CrossRef CAS PubMed
.
- Z. Zhang, Y. Hong, B. Hou, Z. Zhang, M. Negahban and J. Zhang, Carbon, 2019, 148, 115–123 CrossRef CAS
.
- A. A. Emery and C. Wolverton, Sci. Data, 2017, 4, 170153 CrossRef CAS
.
- V. Guillerm, D. Kim, J. F. Eubank, R. Luebke, X. Liu, K. Adil, M. S. Lah and M. Eddaoudi, Chem. Soc. Rev., 2014, 43, 6141–6172 RSC
.
- P. M. Bhatt, V. Guillerm, S. J. Datta, A. Shkurenko and M. Eddaoudi, Chem, 2020, 6, 1613–1633 CAS
.
- H. Lv, X. Li, D. Wu, Y. Liu, X. Li, X. Wu and J. Yang, Nano Lett., 2022, 22, 1573–1579 CrossRef CAS PubMed
.
- J. Tersoff and D. R. Hamann, Phys. Rev. Lett., 1983, 50, 1998–2001 CrossRef CAS
.
- A. Kumar, K. Banerjee, A. S. Foster and P. Liljeroth, Nano Lett., 2018, 18, 5596–5602 CrossRef CAS PubMed
.
- A. Cadiau, K. Adil, P. M. Bhatt, Y. Belmabkhout and M. Eddaoudi, Science, 2016, 353, 137–140 CrossRef CAS
.
- N. Hanikel, M. S. Prévot and O. M. Yaghi, Nat. Nanotechnol., 2020, 15, 348–355 CrossRef CAS PubMed
.
- S. Baroni, S. de Gironcoli, A. Dal Corso and P. Giannozzi, Rev. Mod. Phys., 2001, 73, 515–562 CrossRef CAS
.
- S. Nosé, J. Chem. Phys., 1984, 81, 511–519 CrossRef
.
- D. J. Vogel, J. M. Rimsza and T. M. Nenoff, Angew. Chem., 2021, 133, 11615–11623 CrossRef
.
- A. V. Kuklin, G. V. Baryshnikov, B. F. Minaev, N. Ignatova and H. Ågren, J. Phys. Chem. C, 2018, 122, 22216–22222 CrossRef CAS
.
- A. Wang, J. Peng, N. Ren, L. Ding, X. Yu, Z. Wang and M. Zhao, J. Phys. Chem. Lett., 2021, 12, 7921–7927 CrossRef CAS PubMed
.
- B. Mortazavi, M. Shahrokhi, T. Hussain, X. Zhuang and T. Rabczuk, Appl. Mater. Today, 2019, 15, 405–415 CrossRef
.
- Y. Qi, M. A. Sadi, D. Hu, M. Zheng, Z. Wu, Y. Jiang and Y. P. Chen, Adv. Mater., 2023, 2205714 CrossRef CAS PubMed
.
- J.-W. Jiang and H. S. Park, Nat. Commun., 2014, 5, 4727 CrossRef CAS PubMed
.
- C. Huang and L. Chen, Adv. Mater., 2016, 28, 8079–8096 CrossRef CAS PubMed
.
- G. Kresse and J. Furthmüller, Phys. Rev. B: Condens. Matter Mater. Phys., 1996, 54, 11169–11186 CrossRef CAS PubMed
.
- J. P. Perdew, K. Burke and M. Ernzerhof, Phys. Rev. Lett., 1996, 77, 3865–3868 CrossRef CAS PubMed
.
- H. J. Monkhorst and J. D. Pack, Phys. Rev. B: Solid State, 1976, 13, 5188–5192 CrossRef
.
- H. Wang, L. Zhang, J. Han and W. E, Comput. Phys. Commun., 2018, 228, 178–184 CrossRef CAS
.
- L. Zhang, J. Han, H. Wang, R. Car and W. E, Phys. Rev. Lett., 2018, 120, 143001 CrossRef CAS PubMed
.
-
K. He, X. Zhang, S. Ren and J. Sun, Proceedings of the IEEE Conference on Computer Vision and Pattern Recognition (CVPR), 2016, pp. 770–778.
- Z. Fan, Z. Zeng, C. Zhang, Y. Wang, K. Song, H. Dong, Y. Chen and T. Ala-Nissila, Phys. Rev. B, 2021, 104, 104309 CrossRef CAS
.
- S. Yue, M. C. Muniz, M. F. Calegari Andrade, L. Zhang, R. Car and A. Z. Panagiotopoulos, J. Chem. Phys., 2021, 154, 034111 CrossRef CAS PubMed
.
- A. P. Thompson, H. M. Aktulga, R. Berger, D. S. Bolintineanu, W. M. Brown, P. S. Crozier, P. J. in ‘t Veld, A. Kohlmeyer, S. G. Moore, T. D. Nguyen, R. Shan, M. J. Stevens, J. Tranchida, C. Trott and S. J. Plimpton, Comput. Phys. Commun., 2022, 271, 108171 CrossRef CAS
.
- A. Togo and I. Tanaka, Scr. Mater., 2015, 108, 1–5 CrossRef CAS
.
-
A. Carreras, Zenodo, DOI:10.5281/zenodo.3940626
.
- K. Momma and F. Izumi, J. Appl. Crystallogr., 2008, 41, 653–658 CrossRef CAS
.
- A. Stukowski, Modell. Simul. Mater. Sci. Eng., 2010, 18, 015012 CrossRef
.
|
This journal is © The Royal Society of Chemistry 2024 |