DOI:
10.1039/D4NH00066H
(Communication)
Nanoscale Horiz., 2024,
9, 1023-1029
Heterointerface engineering of layered double hydroxide/MAPbBr3 heterostructures enabling tunable synapse behaviors in a two-terminal optoelectronic device†
Received
12th February 2024
, Accepted 3rd April 2024
First published on 4th April 2024
Abstract
Solution-processable semiconductor heterostructures enable scalable fabrication of high performance electronic and optoelectronic devices with tunable functions via heterointerface control. In particular, artificial optical synapses require interface manipulation for nonlinear signal processing. However, the limited combinations of materials for heterostructure construction have restricted the tunability of synaptic behaviors with simple device configurations. Herein, MAPbBr3 nanocrystals were hybridized with MgAl layered double hydroxide (LDH) nanoplates through a room temperature self-assembly process. The formation of such heterostructures, which exhibited an epitaxial relationship, enabled effective hole transfer from MAPbBr3 to LDH, and greatly reduced the defect states in MAPbBr3. Importantly, the ion-conductive nature of LDH and its ability to form a charged surface layer even under low humidity conditions allowed it to attract and trap holes from MAPbBr3. This imparted tunable synaptic behaviors and short-term plasticity (STP) to long-term plasticity (LTP) transition to a two-terminal device based on the LDH-MAPbBr3 heterostructures. The further neuromorphic computing simulation under varying humidity conditions showcased their potential in learning and recognition tasks under ambient conditions. Our work presents a new type of epitaxial heterostructure comprising metal halide perovskites and layered ion-conductive materials, and provides a new way of realizing charge-trapping induced synaptic behaviors.
New concepts
Artificial optical synapses are an emerging class of biomimetic devices that emulate visual sensing and neuromorphic computing. Although two-terminal optical synaptic devices are attractive for their simple device structures and easy fabrication, they show limited control over synaptic characteristics compared to three-terminal systems. Heterostructures of semiconductors are highly promising for realizing high performance optoelectronic devices through interface engineering. However, their solution-phase preparation remains challenging, leading to limited materials combinations and restricted property modulation. In this study, we explored the formation of epitaxial heterostructures between ion conductive LDH and metal halide perovskite nanostructures. We investigated the charge transfer and ion-electron coupling effect at the heterointerface, and demonstrated tunable optoelectronic properties under different conditions. By utilizing the strong coupling between the LDH and perovskite and the humidity endowed surface charging effect of LDH, we demonstrated a high performance artificial synapse using a simple two-terminal device that can work under both low and high humidity for operation under ambient conditions.
|
Introduction
The development of artificial intelligence (AI) and neuromorphic computing has led to the exploration of biologically neurally inspired artificial hardware for emulating synaptic functions.1 In contrast to the conventional von Neumann computing architecture, the bioinspired architectures of artificial synapses integrate both computing and memory functions within a single computing unit.2,3 Optoelectronic synapses offer advantages of fast information transmission and low-power consumption, showing their potential for neuromorphic vision systems that necessitate the integration of both photo-sensing and synaptic elements.4,5 Most reported optoelectronic synapses are categorized as two-terminal structures and three-terminal transistor geometries.6,7 Although two-terminal systems are attractive for their device structures and easier fabrication, they usually show poorer control over synaptic characteristics compared to three-terminal systems which can modulate paired-pulse facilitation (PPF), excitatory postsynaptic current (EPSC), and short/long-term plasticity (STP/LTP) through gate-induced modulation via electronic spikes. It remains a challenge to achieve high tunability with two-terminal optoelectronic devices.
Epitaxial heterostructures of semiconductors are important for optical and optoelectronic applications. The formation of such heterostructures is highly promising for realizing high performance optoelectronic devices through interface engineering. However, previous optoelectronic devices based on epitaxial heterostructures such as PtTe2/MoS2 and graphdiyne/graphene were usually fabricated through vapor-phased deposition methods, which are expensive and difficult for large-area fabrication.8,9 On the other hand, the solution-phase preparation methods are easy to scale up. However, the solution-phase preparation of epitaxial heterostructures remains challenging, leading to limited materials combinations.
Recently, metal halide perovskites (MHPs) have received considerable attention due to their many attractive properties such as large absorption coefficients,10 long charge carrier diffusion lengths,11 and high intrinsic charge carrier mobilities.12 They have been applied in various electronic and optoelectronic devices, such as solar cells,13 photodetectors,14 light-emitting diodes,15 and memory devices.16 In particular, many MHP based electronic devices exhibit hysteresis effects, making them capable of simulating biological synapses.17–19 Suitable interface engineering and charge manipulation are usually carried out to realize and enhance their synaptic behaviors. For example, a previously reported CsPbCl3/SnO2 based photonic synapse relied on the interfacial charge trapping effect between SnO2 and CsPbCl3 to realize PPF and short/long-term potentiation.20 Humidity is an important factor affecting the performance of artificial synapse devices working under ambient conditions. For electronic artificial synapses, while some devices showed impaired synaptic behavior due to reduced conductivity in humid air,21 those employing ion-conductive materials such as gelatin–hydrogel showed enhanced ion transport and thus improved EPSC.22,23 For optoelectronic synapses, humidity-dependent synaptic behaviors have been much less explored. Layered double hydroxides (LDHs) are a class of layered materials composed of positively charged hydroxide slabs and interlayer anions. They are typical ionic conductors that transport OH− ions24,25 supported by the polarized hydrogen-bond network on their surfaces under a wide range of humid conditions (e.g., from 11% RH to 97% RH).26 We therefore proposed to combine LDH with MHPs to realize synaptic behaviors through the interfacial charge trapping effect under humidity modulation. In this contribution, we show that MAPbBr3 nanocrystals could self-assemble on MgAl-LDH nanoplates epitaxially. The obtained LDH-MAPbBr3 heterostructures showed strong interfacial coupling. An artificial optoelectronic synapse was thus fabricated and demonstrated humidity enabled tunability of retention time from STP to LTP, and a high PPF of 158% for a two-terminal device. This is because water adsorbed on the surface of MgAl-LDH self-dissociated into protons and hydroxyl ions, forming a charged surface layer to attract photo-generated holes from MAPbBr3. Finally, a demonstration of neuromorphic computing simulation under both low and high humidity showed its potential in learning and recognition tasks under ambient conditions.
Results and discussion
MgAl-LDH nanoplates and MAPbBr3 nanocrystals were first synthesized separately (Fig. S1, ESI†) and then mixed in toluene through a self-assembly process27 at room temperature (Fig. 1a). Interestingly, the MgAl-LDH nanoplates which carry surface charges could not be dispersed by non-polar solvents such as toluene, but became dispersible after being mixed with MAPbBr3 (Fig. S2, ESI†), suggesting that MAPbBr3 might have been decorated on the surface of LDH nanoplates and made them dispersible in toluene. Indeed, the transmission electron microscopy (TEM) image in Fig. 1b and the X-ray diffraction (XRD) pattern of LDH-MAPbBr3 in Fig. 1c confirm the assembly of MAPbBr3 nanocrystals on MgAl-LDH. Note that before mixing, the as-synthesized MAPbBr3 nanocrystals were thoroughly washed to largely remove the oleylamine (OLA)/oleic acid (OA) molecules capped on their surfaces to ensure a clean interface between MAPbBr3 and LDH. If the OLA/OA molecules were not removed by washing, MAPbBr3 would have showed poor adhesion on LDH (Fig. S3, ESI†). The LDH-MAPbBr3 heterostructures were further characterized by high-resolution TEM (HRTEM) (Fig. 1d–g). Note that MAPbBr3 has a cubic crystal structure (space group of Pm
m) with a lattice parameter of a = 5.920 Å.28 The LDH nanoplates have a rhombohedral crystal structure (space group of R
m) with lattice parameters of a = b = 3.049 Å and c = 26.86 Å.26 A typical HRTEM image in Fig. 1d shows an area of overlapped lattice patterns of LDH and MAPbBr3. In the fast Fourier transformation (FFT)-generated diffraction pattern (Fig. 1e), there are two sets of diffraction spots: one with a hexagonal symmetry (highlighted as the vertices of the red hexagon) and the other with a square symmetry (highlighted as the vertices of the yellow square). By respectively selecting the two sets of spots, the LDH [001]-zone lattice pattern and the MAPbBr3 [001]-zone pattern were generated via inverse Fourier transform in Fig. 1f and g. This reveals an evident epitaxial relationship between LDH and MAPbBr3, where along the common direction of MAPbBr3 [100]/LDH [100], the MAPbBr3 (200) planes are parallel with the LDH (2–10) planes with a mismatch of only 1.8% (Fig. 1h).
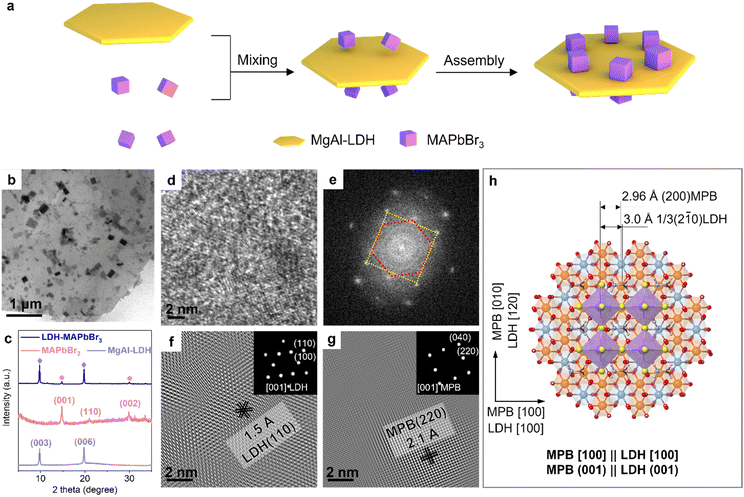 |
| Fig. 1 (a) Schematic illustration of the formation process of LDH-MAPbBr3 heterostructures via a self-assembly process. (b) TEM and (c) XRD pattern of LDH-MAPbBr3 heterostructures. (d) HRTEM image of an area with overlapped lattices of MAPbBr3 and LDH, and (e) the corresponding FFT-diffraction pattern. (f) LDH[001]-zone and (g) MPB[001]-zone lattice patterns generated by performing inverse-FFT of spots forming the red hexagon and the yellow square in (e), respectively (MAPbBr3, denoted as MPB). Inset: The corresponding selected spots. (h) Schematic model for the MPB[100]‖LDH[100] and MPB(001)‖LDH(001) epitaxial relationship. | |
The heterostructures were characterized by photoluminescence (PL) spectroscopy and ultraviolet-visible (UV-vis) absorption spectroscopy and compared with MAPbBr3 (Fig. 2a and Fig. S4a, ESI†). On the basis of equal mass of MAPbBr3, the LDH-MAPbBr3 film showed a much reduced PL intensity compared to MAPbBr3 at room temperature (300 K). This can be due to the charge transfer from MAPbBr3 to LDH that partially quenched the emission of MAPbBr3. As shown in Fig. 2b, the bandgap alignment of LDH and MAPbBr3 was obtained based on their conduction band and valence band positions (Fig. S4b–d, ESI†).29–31 Note that 405 nm light illumination cannot excite LDH with a bandgap of 4.6 eV, but can generate electrons and holes in MAPbBr3 having a bandgap of 2.19 eV. The photo-generated holes in MAPbBr3 were readily transferred to LDH, and hence the emission of MAPbBr3 was reduced at 300 K. However, at 80 K, the emission of MAPbBr3 in the heterostructure was stronger than that of MAPbBr3 alone (Fig. 2a, lower panel). The temperature-dependent PL intensity from 80 to 300 K was further plotted (Fig. 2c and Fig. S5, ESI†), which reveals that both MAPbBr3 and LDH-MAPbBr3 heterostructures showed enhanced PL emission at lower temperatures. This can be attributed to the decreased nonradiative recombination loss at low temperatures. At temperatures lower than 120 K, MAPbBr3 in the heterostructures showed stronger emission than MAPbBr3 alone (Fig. 2c), which suggests that in addition to interface charge transfer, another factor was influencing the emission behavior.
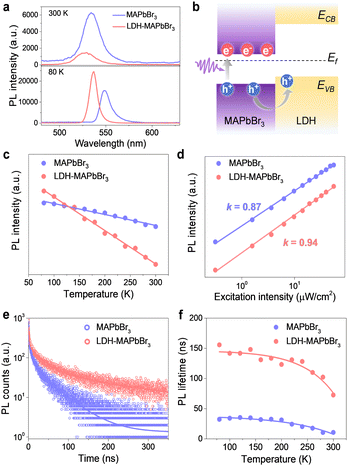 |
| Fig. 2 (a) PL spectra of MAPbBr3 and LDH-MAPbBr3 at 300 K (top panel) and 80 K (bottom panel). (b) Schematic illustration of charge transfer in the LDH-MAPbBr3 heterostructures under the excitation of a 405 nm laser. (c) PL intensity as a function of temperature for MAPbBr3 and LDH-MAPbBr3 heterostructures. (d) PL intensity as a function of excitation intensity for MAPbBr3 and LDH-MAPbBr3 heterostructures. (e) TRPL decay curves of MAPbBr3 and LDH-MAPbBr3 heterostructures at 80 K. (f) PL lifetime as a function of temperature for MAPbBr3 and LDH-MAPbBr3 heterostructures. | |
We further obtained the room temperature PL spectra of MAPbBr3 nanocrystals and LDH-MAPbBr3 heterostructures under 405 nm excitation with power intensities increasing from 0.23 to 509.55 μW cm−2 (Fig. S6, ESI†). The PL intensity against the excitation power intensity can be linearly fitted with the power law32,33 (Fig. 2d), where the slope (k) is the power coefficient. Based on the carrier recombination model, PL emission from free excitons usually gives a k value of 1, and k less than 1 is likely related to bound excitons due to trap states or defects.34 Therefore, an increased power coefficient from 0.87 to 0.94 after the formation of LDH-MAPbBr3 heterostructures indicates a reduced trap state. This is further supported by the longer PL lifetimes of LDH-MAPbBr3 compared to MAPbBr3 over the 80–300 K temperature range, as shown in their time-resolved photoluminescence (TRPL) decay curves (Fig. 2e and f and Fig. S7, ESI†). Furthermore, by fitting the decay curves under different power intensities (Fig. S8, ESI†) with the recombination kinetics model,35 the trap density in MAPbBr3 was found to be greatly reduced from 2.2 × 1015 to 3.4 × 1014 cm−3 after being hybridized with LDH. Therefore, the formation of LDH-MAPbBr3 epitaxial heterointerfaces enabled effective charge transfer from MAPbBr3 to LDH and reduced the trap defects on MAPbBr3. Considering the humidity-dependent ion conductive properties of LDH, the LDH-MAPbBr3 heterostructures were further applied in artificial synapses.
Synapse performances
As shown in Fig. 3a, external stimuli can be perceived and processed by neuron networks, in which synaptic weights between pre-synaptic and post-synaptic neurons determine the response of a synapse to input stimuli. The change of synaptic weight modulated by the activity of the pre- and post-synaptic neurons is defined as the synaptic plasticity.4,6 For artificial optoelectronic synapses, light irradiation acts as the pre-synaptic stimulus, and causes the conductance of the device channel to increase. The ability (synaptic plasticity) of the device to convert and transmit such information is reflected in the change of channel current (i.e., EPSC). In our experiments, the LDH-MAPbBr3 heterostructures were drop-coated onto an interdigital electrode to construct a two-terminal optoelectronic synapse for measurements at room temperature. A fixed bias voltage (0.5 V) was applied between the electrodes to induce the current response of LDH-MAPbBr3 under 405 nm light stimulation (Fig. 3b). Under one spike stimulus (stimulus width Δt = 1 s), the EPSC increased as the humidity increased from 11% to 57% RH, which can be attributed to the enhanced ionic conductivity of LDH under higher humidity (Fig. S9, ESI†).24 In addition, an obviously elongated retention time of the current after the removal of the light stimulus was observed under humid air. In sharp contrast, no retention was observed for the device in a vacuum (Fig. S10, ESI†). The retention time is an important parameter of synaptic devices that the synaptic plasticity is classified into short-term plasticity (STP, several milliseconds of retention time) and long-term plasticity (LTP, tens to hundreds of seconds of retention time). Both STP and LTP play key roles in learning and forgetting and image recognition in humans. We found that the LDH-MAPbBr3 synapse device showed a humidity-modulated synaptic behavior, i.e., a transition from STP to LTP with increasing humidity.
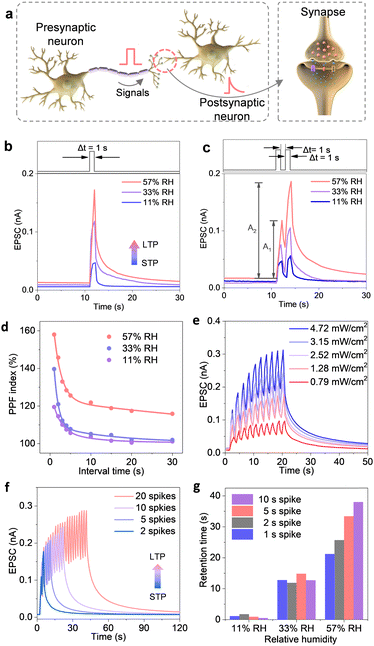 |
| Fig. 3 (a) Schematic illustration of a biological system in response to a stimulus. (b) EPSC and (c) PPF responses of LDH-MAPbBr3 as single and paired light stimuli were applied under various humidity conditions. (d) PPF index as a function of interval time under various humidity conditions. (e) Optical power intensity and (f) spike number dependent synaptic plasticity for LDH-MAPbBr3 heterostructures under 57% RH. (g) Comparison of retention time of LDH-MAPbBr3 under various humidity conditions for different durations of stimuli. | |
Paired pulse facilitation (PPF) is another typical synaptic behavior. As shown in Fig. 3c, a second pulse is rapidly excited after the first one with the same interval. The stimulus width and the interval between stimuli were equally set, denoted as Δt. After the second pulse, the EPSC level will increase from A1 to A2, and the PPF is thus defined by A2/A1, giving a value larger than 1. A high PPF index possibly indicates both high efficiency and accuracy for neuromorphic computing due to the higher temporal resolution.36 PPF behaviors were observed at different humidity levels, even at a low humidity of 11%. A PPF value of 158% was achieved under 57% RH, outperforming those of other solution processed 2-terminal optoelectronic synapse devices (Table S1, ESI†). As shown in Fig. 3d, the PPF index was plotted as a function of interval time under different humidities. It can be seen that the smaller the interval between two synaptic stimuli, the more significant the enhancement effect of the postsynaptic response.
The PPF index can be fitted using a dual exponential decay function, as shown in the following equation:37
|  | (1) |
where
C0 is the initial facilitation magnitude,
C1 and
C2 are the rapid- and slow-phase initial facilitation magnitude, respectively.
τ1 and
τ2 are the relaxation times of the two phases, respectively, and
τ2 is crucial for determining the retention time.
38 The fitted curves and parameters are shown in
Fig. 3d and Table S2 (ESI
†). The larger
τ2 obtained at a higher humidity indicates a longer retention time after stimulus removal, which is consistent with the observed transition from STP to LTP as the humidity increased (
Fig. 3b).
The synaptic plasticity of the optoelectronic synapse was also modulated by optical power intensity (Fig. 3e), spike number (Fig. 3f) and optical duration time (Fig. S11, ESI†) under 57% RH. Obviously, a higher optical power could enhance the EPSC (Fig. 3e), and a transition from STP to LTP occurred with increasing spike number (Fig. 3f). When the duration time increased from 1 to 10 s, the EPSC increased by two times at ten spikes (Fig. S11, ESI†). We summarized the dependency of retention time on humidity and spike duration in Fig. 3g. A long retention time of 38 s was achieved at 57% RH and 10 s duration. This phenomenon indicates that humidity plays a key role in enhancement of the synaptic behavior and modulation of the retention time.
Our proposed mechanism for the humidity enabled synaptic behavior is illustrated in Fig. 4a. It is well accepted that a layer of water adsorbed on the surface of LDH can self-dissolve and form a hydrogen bond network as per the following reaction:39
| M–OH + H2O = M–OH2+ + OH− | (2) |
where M represents the metal in LDH. The photo-generated holes in MAPbBr
3 were attracted to the OH
− ions on the surface of LDH and trapped by the polarized hydrogen bond network. When the light stimulus was removed, the photo-generated holes were retained at the interface for a certain period of time, resulting in the increased retention time at higher humidity and no retention under vacuum conditions. To further verify the abovementioned mechanism, the surface potential of the LDH-MAPbBr
3 heterostructure under low and high humidity was measured by Kelvin probe force microscopy (KPFM). As shown in
Fig. 4b–e, the surface potentials of the heterostructure under 25% and 57% RH were −39 and −86 mV, respectively. The more negative surface potential under higher humidity suggests a more polarized surface of MAPbBr
3 due to the attraction of more holes towards LDH. In addition, the photo-response of a device based on the randomly assembled LDH-MAPbBr
3 composite without well-defined heterointerfaces (Fig. S3, ESI
†) showed no retention (Fig. S12, ESI
†). This again suggests the importance of having a well-defined heterointerface for charge modulation.
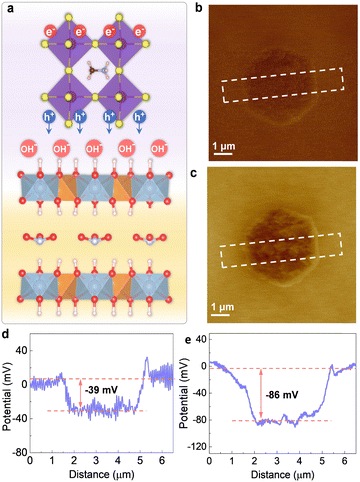 |
| Fig. 4 (a) Schematic illustration of photo-generated holes in MAPbBr3 attracted to the negatively charged LDH surface. (b) and (c) KPFM images and (d) and (e) the corresponding surface potentials of LDH-MAPbBr3 under (b) and (d) 25% RH and (c) and (e) 57% RH. | |
The LDH-MAPbBr3 optical synapse was further applied to simulate the learning process with a spike duration of 30 s (Fig. 5a). After the relaxation, it only took 13.3 s to reach the same EPSC, i.e., relearning process. This phenomenon resembles the human learning process in which relearning the same information takes less time compared with the initial learning.40,41 The high PPF index of LDH-MAPbBr3 heterostructures under humid conditions enabled their use in neuromorphic computing. A 3 × 3 LDH-MAPbBr3 synapse array was thus exposed under an “H”-patterned light stimulation (4.72 mW cm−2) with the spike duration time ranging from 1 to 30 s under 57%, 33% and 11% RH. The color mapping images corresponding to the EPSC values are shown in Fig. 5b–d. A clearer “H” image was achieved for both 33% and 57% RH compared to the blurred image under 11% RH, indicating that the device is a good demonstration of artificial eyes which lose the recognition ability under dry conditions.23
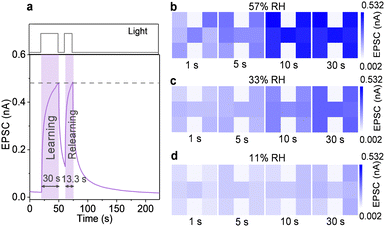 |
| Fig. 5 (a) Learning and relearning behavior of the LDH-MAPbBr3 optoelectronic synapse. (b) and (c) EPSC responses of LDH-MAPbBr3 as 10 successive light pulses with 1 s, 5 s, 10 s, and 30 s duration were applied under (b) 57% RH, (c) 33% RH and (d) 11% RH condition. | |
Neuromorphic computing simulation
To further demonstrate the potential of the LDH-MAPbBr3 optical synapse for neuromorphic computing, it was employed for the recognition of the MNIST handwritten digital image42via a multilayer perceptron (MLP) simulator NeruroSimV3.0.43 A fully connected 2-layer MLP neural network was constructed as shown in Fig. 6a, including 400 neurons in one input layer and 100 hidden neurons in another hidden layer, as well as 10 neurons in the output layer. The LTP curves and the corresponding nonlinearity (NL) which were extracted from the LTP curves for long-term potentiation are shown in Fig. S13 (see the Experimental section for detailed simulation, ESI†). After 35 training epochs, the cognitive accuracy reached a considerably high value up to 80% under both 33% and 57% RH (Fig. 6b), indicating the potential of our optoelectronic synapse for learning and recognition tasks under ambient conditions.
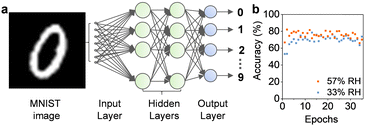 |
| Fig. 6 (a) Schematic illustration of a neural network. (b) Accuracy calculation of the network under different humidity conditions. | |
Conclusions
In this work, LDH-MAPbBr3 heterostructures were prepared via a self-assembly process. An epitaxial relationship was observed between LDH and MAPbBr3, and such an epitaxial interface enabled effective charge transfer from MAPbBr3 to LDH and passivated defects on MAPbBr3. The LDH-MAPbBr3 optoelectronic synapse showed optical interval time, duration, power, and spike number dependent plasticity. The EPSC and retention time of LDH-MAPbBr3 increased with increasing RH with an evident STP-to-LTP transition, indicating the humidity-modulated synaptic performance. It was suggested that the photo-generated holes might be transferred from MAPbBr3 to LDH and trapped on the polarized LDH surface due to the self-dissociation of water molecules on LDH, resulting in the potentiation properties. In addition, learning and relearning processes were also demonstrated with the LDH-MAPbBr3 synapse, and a neuromorphic computing simulation was realized showing high accuracy. Our work introduces a new type of hybrid functional material, and may provide a new way of emulating environmental adaptive behavior of human eyes based on electronic and ionic conductors.
Author contributions
This study was conceived and designed by X. Huang and Q. Chen. Q. Chen synthesized the samples, conducted the characterization and analyzed the results. H. Li, and S. Yu conducted KPFM measurements. J. Cao, N. Wang, and C. Hao conducted TRPL measurements. X. Huang, Q. Chen, Z. Yang, Z. Wang and J. Wang wrote the manuscript with input from all authors.
Conflicts of interest
There are no conflicts to declare.
Acknowledgements
This work was supported by the National Natural Science Foundation of China (grant no. 62374086 and 62288102).
Notes and references
- Q. H. Liu, L. Yin, C. Zhao, J. Y. Wang, Z. Wu, H. Lei, Y. A. Liu, B. W. Tian, Z. Y. Zhang, Z. S. Zhao, R. F. Liu, C. Z. Ding, Y. F. Han, C. Q. Ma, P. F. Song, E. G. Lim and Z. Wen, Nano Energy, 2022, 102, 107686 CrossRef CAS
.
- D. Hao, Z. Yang, J. Huang and F. Shan, Adv. Funct. Mater., 2022, 33, 2211467 CrossRef
.
- D. Sharma, D. Rao and B. Saha, Nanoscale Horiz., 2023, 8, 543–549 RSC
.
- M. Zhou, Y. Zhao, X. Gu, Q. Zhang, J. Zhang, M. Jiang and S. Lu, APL Photonics, 2023, 8, 076107 CrossRef CAS
.
- C. Zhu, W. Huang, W. Li, X. Yu and X. a Li, Research, 2022, 2022, 9786023 CrossRef CAS
.
- X. Han, Z. S. Xu, W. Q. Wu, X. H. Liu, P. G. Yan and C. F. Pan, Small Struct., 2020, 1, 2000029 CrossRef
.
- J. Zhang, S. Dai, Y. Zhao, J. Zhang and J. Huang, Adv. Intell. Syst., 2020, 2, 1900136 CrossRef
.
- M. M. Islam, A. Krishnaprasad, D. Dev, R. Martinez-Martinez, V. Okonkwo, B. Wu, S. S. Han, T. S. Bae, H. S. Chung, J. Touma, Y. Jung and T. Roy, ACS Nano, 2022, 16, 10188–10198 CrossRef CAS PubMed
.
- Z. Zhang, Y. Li, J. Wang, D. Qi, B. Yao, M. Yu, X. Chen and T. Lu, Nano Res., 2021, 14, 4591–4600 CrossRef CAS
.
- M. B. Johnston and L. M. Herz, Accounts Chem. Res., 2016, 49, 146–154 CrossRef CAS PubMed
.
- S. D. Stranks, G. E. Eperon, G. Grancini, C. Menelaou, M. J. Alcocer, T. Leijtens, L. M. Herz, A. Petrozza and H. J. Snaith, Science, 2013, 342, 341–344 CrossRef CAS PubMed
.
- D. Shi, V. Adinolfi, R. Comin, M. Yuan, E. Alarousu, A. Buin, Y. Chen, S. Hoogland, A. Rothenberger, K. Katsiev, Y. Losovyj, X. Zhang, P. A. Dowben, O. F. Mohammed, E. H. Sargent and O. M. Bakr, Science, 2015, 347, 519–522 CrossRef CAS PubMed
.
- S. T. Ha, R. Su, J. Xing, Q. Zhang and Q. Xiong, Chem. Sci., 2017, 8, 2522–2536 RSC
.
- H. Deng, X. Yang, D. Dong, B. Li, D. Yang, S. Yuan, K. Qiao, Y. B. Cheng, J. Tang and H. Song, Nano Lett., 2015, 15, 7963–7969 CrossRef CAS PubMed
.
- X. Shen, K. Kang, Z. Yu, W. H. Jeong, H. Choi, S. H. Park, S. D. Stranks, H. J. Snaith, R. H. Friend and B. R. Lee, Joule, 2023, 7, 272–308 CrossRef CAS
.
- G. Vats, B. Hodges, A. J. Ferguson, L. M. Wheeler and J. L. Blackburn, Adv. Mater., 2023, 35, e2205459 CrossRef PubMed
.
- X. Hong, X. Liu, L. Liao and X. Zou, Photonics Res., 2023, 11, 787–807 CrossRef CAS
.
- L. Qian, Y. Sun, M. Wu, C. Li, D. Xie, L. Ding and G. Shi, Nanoscale, 2018, 10, 6837–6843 RSC
.
- G. K. Gupta, I. J. Kim, Y. Park, M. K. Kim and J. S. Lee, ACS Appl. Mater. Interfaces, 2023, 15, 18055–18064 CrossRef CAS PubMed
.
- L. Yang, M. Singh, S. W. Shen, K. Y. Chih, S. W. Liu, C. I. Wu, C. W. Chu and H. W. Lin, Adv. Funct. Mater., 2020, 31, 2008259 CrossRef
.
- P. Y. Le, H. Nagib, L. A. Sylvander, M. W. Allen, D. G. McCulloch, J. G. Partridge and H. N. Tran, ACS Appl. Electron. Mater., 2023, 5, 2885–2892 CrossRef CAS
.
- J.-R. Chen, Z.-N. Lu, C.-H. Zhu, J.-W. Cai, Z.-D. Zhang, Y.-N. Zhong, J.-L. Xu, X. Gao and S.-D. Wang, Microelectron. Eng., 2023, 277, 112028 CrossRef CAS
.
- X. Han, X. Zhao, T. Zeng, Y. Yang, H. Yu, C. Zhang, B. Wang, X. Liu, T. Zhang, J. Sun, X. Li, T. Zhao, M. Zhang, Y. Ni, Y. Tong, Q. Tang and Y. Liu, Small, 2023, 19, e2206181 CrossRef PubMed
.
- P. Sun, R. Ma, X. Bai, K. Wang, H. Zhu and T. Sasaki, Sci. Adv., 2017, 3, e1602629 CrossRef PubMed
.
- J. Hu, X. Tang, Q. Dai, Z. Liu, H. Zhang, A. Zheng, Z. Yuan and X. Li, Nat. Commun., 2021, 12, 3409 CrossRef CAS PubMed
.
- Q. Chen, X. Wang, Z. Wang, J. Cao, J. Dai, J. Wang, E. Fatima-Ezzahra and X. Huang, ACS Appl. Nano Mater., 2022, 5, 4991–4997 CrossRef CAS
.
- Z. Zhu, C. Zhu, L. Yang, Q. Chen, L. Zhang, J. Dai, J. Cao, S. Zeng, Z. Wang, Z. Wang, W. Zhang, J. Bao, L. Yang, Y. Yang, B. Chen, C. Yin, H. Chen, Y. Cao, H. Gu, J. Yan, N. Wang, G. Xing, H. Li, X. Wang, S. Li, Z. Liu, H. Zhang, L. Wang, X. Huang and W. Huang, Nat. Mater., 2022, 21, 1042–1049 CrossRef CAS PubMed
.
- G. A. Elbaz, D. B. Straus, O. E. Semonin, T. D. Hull, D. W. Paley, P. Kim, J. S. Owen, C. R. Kagan and X. Roy, Nano Lett., 2017, 17, 1727–1732 CrossRef CAS PubMed
.
- L. Kong, G. Liu, J. Gong, Q. Hu, R. D. Schaller, P. Dera, D. Zhang, Z. Liu, W. Yang, K. Zhu, Y. Tang, C. Wang, S. H. Wei, T. Xu and H. K. Mao, Proc. Natl. Acad. Sci. U. S. A., 2016, 113, 8910–8915 CrossRef CAS PubMed
.
- G. Mannino, I. Deretzis, E. Smecca, A. La Magna, A. Alberti, D. Ceratti and D. Cahen, J. Phys. Chem. Lett., 2020, 11, 2490–2496 CrossRef CAS PubMed
.
- S. M. Xu, T. Pan, Y. B. Dou, H. Yan, S. T. Zhang, F. Y. Ning, W. Y. Shi and M. Wei, J. Phys. Chem. C, 2015, 119, 18823–18834 CrossRef CAS
.
- T. Schmidt, K. Lischka and W. Zulehner, Phys. Rev. B: Condens. Matter., 1992, 45, 8989–8994 CrossRef PubMed
.
- F. Luckert, M. V. Yakushev, C. Faugeras, A. V. Karotki, A. V. Mudryi and R. W. Martin, J. Appl. Phys., 2012, 111, 093507 CrossRef
.
- L. Yang, Y. Li, W. Zhang, Y. Liu, J. Cao, Y. Cao, J. Bao, Z. Wang, L. Wang and X. Huang, Chem. Commun., 2022, 58, 7765–7768 RSC
.
- S. D. Stranks, V. M. Burlakov, T. Leijtens, J. M. Ball, A. Goriely and H. J. Snaith, Phys. Rev. Appl., 2014, 2, 034007 CrossRef CAS
.
- H. S. Zhang, X. M. Dong, Z. C. Zhang, Z. P. Zhang, C. Y. Ban, Z. Zhou, C. Song, S. Q. Yan, Q. Xin, J. Q. Liu, Y. X. Li and W. Huang, Nat. Commun., 2022, 13, 4996 CrossRef CAS PubMed
.
- C. Han, X. Han, J. Han, M. He, S. Peng, C. Zhang, X. Liu, J. Gou and J. Wang, Adv. Funct. Mater., 2022, 32, 2113053 CrossRef CAS
.
- Y. Liu, Y. Wu, H. Han, Y. Wang, R. Peng, K. Liu, D. Yi, C. W. Nan and J. Ma, Adv. Funct. Mater., 2023, 34, 2306945 CrossRef
.
- T. Yamasaki, S. Iimura, H. Hosono and S. Yamaguchi, J. Phys. Chem. C, 2023, 127, 6045–6053 CrossRef CAS
.
- H. Ebbinghaus, Ann. Neurosci., 1913, 20, 155–156 Search PubMed
.
- B. J. Underwood, Psychol. Rev., 1957, 64, 49–60 CAS
.
- Y. Lecun, L. Bottou, Y. Bengio and P. Haffner, Proc. IEEE Inst. Electr. Electron. Eng., 1998, 86, 2278–2324 CrossRef
.
-
P. Y. Chen, X. Peng and S. Yu, San Francisco, CA, USA, 2017: 6.1.1–6.1.4.
|
This journal is © The Royal Society of Chemistry 2024 |