DOI:
10.1039/D3MA00924F
(Review Article)
Mater. Adv., 2024,
5, 394-408
Layered nanomaterials for renewable energy generation and storage
Received
30th October 2023
, Accepted 30th November 2023
First published on 11th December 2023
Abstract
This study focuses on potential applications of two-dimensional (2D) materials in renewable energy research. Additionally, we briefly discuss other implementations of 2D materials in smart systems like self-healing coatings and electrochemical reduction of carbon dioxide and nitrogen. We highlight the recent advancements in energy storage technology, phase change materials, materials' fundamental characteristics, and summarise perspectives of their future applications in energy technology and related fields. Furthermore, we discuss the use of machine learning and big data for optimisation and further development of 2D materials.
1. Introduction
Fossil fuels are the main energy sources in human society and account for about 85% of total energy consumption.1 However, burning fossil fuels led to a gradual depletion of their reserves, which caused an energy crisis and environmental problems.2–4 Therefore, it is important to search for implementation of renewable energy sources that are sustainable and environmentally friendly. Hydrogen, biofuels, solar, and wind energy are widely used to replace fossil energy sources. One of the main disadvantages of hydrogen energy is that most hydrogen is produced from fossil fuels using well-established technologies such as steam reforming, gasification, and partial oxidation, which are criticised for their high energy consumption and carbon emissions.5 Biofuels, typically produced through biomass fermentation, have low concentration in the final product and often form mix of liquids with water (azeotropes).6,7 This can significantly increase production costs and potentially impact the combustion process or pose security concerns during their application. Solar and wind energy disadvantages include production instability depending on the weather conditions and high installation and maintenance costs.8,9 Therefore, new multifunctional materials are required to solve currents issues of the green energy transition process.
Promising energy harvesting devices can accumulate energy from the environment, allowing for sustainable operation by storing more energy than consumed (Fig. 1).10 Their advantages have led to significant progress in portable energy harvesting systems containing 2D materials. First, 2D materials have a large surface to volume ratio, allowing full use of all available active electrode materials.11 This leads to an increase in contact area between the electrodes and electrolytes and a reduction of the pathway length for charge transfer. Second, atomic-level or nanoscale 2D materials are thin, which makes them flexible and attractive for devices requiring low thickness and high flexibility.12,13 Moreover, 2D materials have unique properties related to limiting in-plane charge and heat transfer, making them ideal for thermoelectric devices that convert heat into electricity.14,15 Third, many 2D materials are highly conductive with tunable electronic properties, making them ideal for batteries,16–18 supercapacitors19 and other energy storage devices.20 Remarkable optoelectronic characteristics of these nanomaterials include light emission, optical modulation, saturable adsorption and electrically modulated field effect characteristics, which have contributed to the various LEDs, lasers, optical modulators, photodetectors, and high-performance 2D field-effect transistors.21–23 The chemical stability of these materials makes it possible to achieve long-term resistance to degradation and corrosion.24,25 All of these characteristics make 2D materials potential candidates for the next generations of energy storage devices.
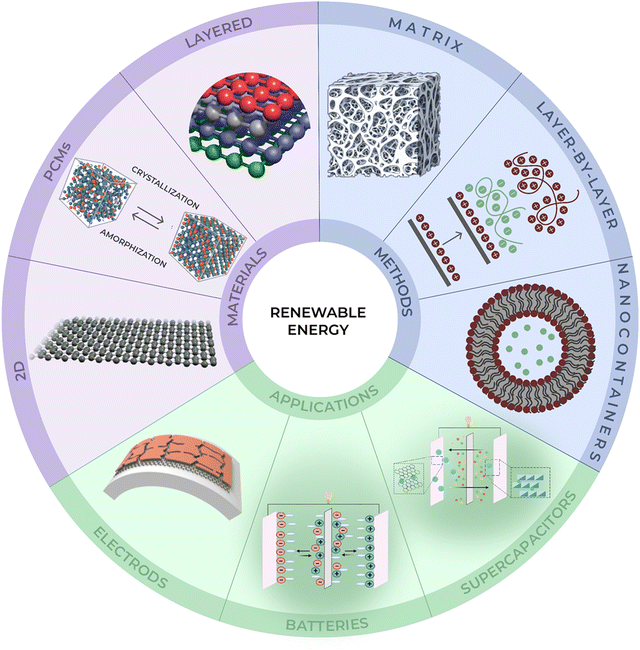 |
| Fig. 1 Schematic illustration of renewable energy materials, methods of their preparation and applications. | |
In recent years, thin 2D structures with exceptional electrical, optical, thermal and mechanical properties based on graphene and graphene oxide (GO),26–28 graphyne,29 graphdiyne (GDY),30 transition-metal dichalcogenides (TMDs),31,32 and transition metal carbide, nitride, or carbonitride (MXene),33,34 and 2D black phosphorus (BP)35 have demonstrated enormous potential for their applications in various fields of materials science and energy. 2D materials are divided into non-layered and layered materials. Layered 2D materials, unlike non-layered ones, have an isotropic volumetric crystal structure due to covalent bonds and have van der Waals gaps between adjacent layers.36,37 In particular, van der Waals forces plays significant role in determining the physical properties of substances, such as boiling and melting points, and are important in understanding intermolecular interactions.38,39
The use of 2D materials can significantly improve the properties of various types of electronic devices. Due to its high surface area (up to 2630 m2 g−1), electrical conductivity, electron transfer ratio (106 S cm−1), and modulus of elasticity, graphene has become a leading material in electrochemistry and battery storage.40,41 Graphdiyne differs from graphene in combining sp- and sp2-hybridized carbon atoms, while graphene consists of only sp2-hybridized carbon atoms. However, due to the absence of heteroatoms, graphdiyne has low intrinsic electrochemical activity.42 Therefore, to use graphene in energy storage and conversion, it is necessary to modify it by doping with heteroatoms or hybridise with other active materials. However, graphene cannot be used in high-performance, low-power FET devices due to the low turn-on/off ratios and high turn-off currents that arise from the lack of bandgap.43
In turn, some 2D TMDs have the same layered structure features as graphite. Still, most of their bandgap is within 1–2 eV, and they have strong covalent bonds within layers and weak van der Waals forces between layers, which provide an ideal space for Li intercalation.44 For batteries, this means better ion intercalation/deintercalation, control of the volumetric expansion of electrode materials and improved lithium storage surface/interface properties. Two-dimensional TMDs can also be used in solar cells to achieve tunable bandgap by adjusting the number of layers and ensure more efficient absorption of sunlight as compared to conventional materials like silicon or gallium arsenide.45,46 2D TMDs have a direct bandgap – leading to efficient charge separation and collection. Therefore, by controlling the thickness of the TMDs, their bandgap can be tailored to match most of the solar spectrum, allowing for more efficient absorption of sunlight.47 Moreover, TMDs have demonstrated multiple light-matter solid reflections, which means they can absorb a significant amount of light even in ultrathin layers.48
Using the unique MXenes structure for supercapacitors can provide a fast electron supply due to their individual conductive transition metal carbide layers, which have efficient electron transport.49 This enables increased charge storage and fast charging/discharging rates in supercapacitors through their versatile surface terminal groups, which act as active centres for rapid redox reactions.50 MXenes have high proton availability due to their hydrophilic surface terminal groups.
The unique puckered monolayer geometry gives 2D black phosphorus or phosphorene many unprecedented properties, making it a promising electrode material for electrochemical energy storage devices, such as lithium-ion batteries, supercapacitors, and emerging technologies like lithium–sulfur batteries, magnesium-ion batteries and sodium-ion batteries.51 The intrinsic in-plane anisotropy and moderate bandgap of phosphorene have stimulated considerable efforts in developing its potential in thermoelectric applications due to the large Seebeck coefficient and high carrier mobility. The phosphorene bandgap can be tuned from 0.3 to 2 eV by decreasing its thickness from bulk to single layer. Such an adjustment can be engineered by the plane strain and edge structures providing more flexibility in device design.52,53 It is important to note that, despite promising properties, phosphorene has challenges such as low yield in preparation and poor air stability.
This review summarises recent advances in 2D materials for energy sources, methods for the layered structures fromation, and applications of phase transition materials (PCMs) for thermal energy storage. We focus on using machine learning (ML) as a versatile and effective tool to predict the structural, electronic, mechanical, and chemical properties of 2D materials that have yet to be discovered.
2. Methods for the formation of layered structures
The development of functional micro- and nanocontainers is of great interest in various research and application areas, including biotechnology,54,55 medicine,56,57 cosmetics,58,59 catalysis,60,61 and the creation of multifunctional materials.62,63 Nanocontainers have a unique ability to isolate active substances from the surrounding environment and release them as needed. To achieve this goal, stable shell structures of nanocontainers should be designed to possess the required functionalities and enable the controlled release of active substances. Various external stimuli and methods can be used for this purpose, including changes in pH,64 temperature,65 magnetic fields,66 light irradiation.67,68
Currently, the following approaches for creating “smart” materials have gained wide popularity.69,70 The first approach is based on the self-assembly of lipid molecules or amphiphilic block copolymers into spherical bilayer structures known as vesicles.71,72 The second approach involves using dendrimers or hyperbranched polymers as nanocontainers.73,74 The third one includes suspension and emulsion polymerisation around nanodroplets of oil or water, forming a polymer shell around them. This method can be enhanced using ultrasound and allows for the creating of hollow nanostructures with a size of approximately 20 nm.75 It represents a simple one-step process and has been widely studied, with several reviews dedicated to the emulsion polymerisation technique.71,75,76 Inorganic frameworks of nanocontainers, such as mesoporous silica or nanotubes made of titanium dioxide and gallium zeolite equipped with nanogates with adjustable pores, exhibit higher mechanical strength and are more cost-effective comparing to polymer nanocontainers.77–79
2.1. Layer-by-layer method – protective coatings
Layer-by-layer (LbL) assembly has become a helpful tool for creating functional nanofilms with various applications.80,81 This universal method enables the fabrication of thin films on the surfaces of various shapes. One of the key benefits of LbL is the ability to tailor the chemical nature of the surface, making it flexible and adaptive.82–84 Additionally, LbL draws inspiration from processes occurring in living organisms, allowing the creation of biomimetic materials. The LbL method has been automated and is now used to develop new commercial products.85,86 Industry explores future directions for applying LbL, such as functional coatings on complex surfaces,87–90 membranes81,91,92 and material design,93,94 and for biomedical delivery applications.95–97
One of the most developed examples of LbL assembly application is fabrication of protective coatings, including anticorrosion and anti-graffiti coatings.98,99 Significant developments in this field include films that inhibit corrosion processes on metal surfaces and use of nanocarriers to store and release corrosion inhibitors. LbL assembly enables the development of intelligent coatings that can adapt to environmental changes and repair themselves if damaged.100
Shchukin et al. was the first to apply the LbL assembly method to deposit polystyrene sulfonate (PSS) polyelectrolyte multilayer films onto the surface of halloysite nanotubes, which were preloaded with corrosion inhibitors.101 After incorporating these halloysite nanocarriers into composite SiOx/ZrOy sol–gel coatings, the composite coatings demonstrated effective corrosion protection of aluminium over an extended period up to 10 years. Multilayer composite LbL films enable efficient storage of inhibitors and their long-term release (Fig. 2).
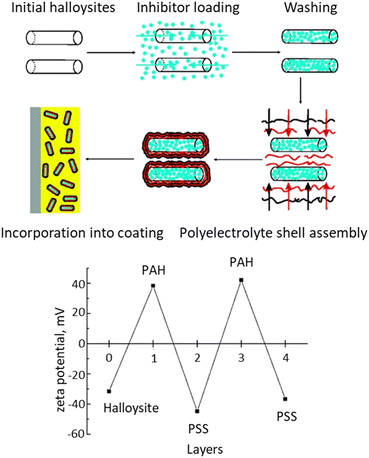 |
| Fig. 2 Synthesis nanocarriers from halloysite loaded with 2-mercaptobenzothiazole. Zeta potential during polyelectrolyte deposition on halloysite nanotubes at pH 7.5. Reproduced from ref. 101 with permission of publisher. Copyright 2008 American Chemical Society. | |
Similarly, SiO2 particles coated with LbL multilayer films containing inhibitors were used as nanocarriers for simultaneous self-regeneration and creation of anticorrosion composite coatings. Andreeva et al. applied the deposition of polyelectrolyte multilayer films with opposite charges onto the surfaces of aluminium materials.102 These polyelectrolyte multilayer LbL coatings effectively inhibited corrosion processes on aluminium surfaces due to their ability to maintain a stable pH level.
2.2. Matrix for thermal energy storage
The combination of natural nanocarriers like sepiolite and crystallohydrates represents an affordable and effective nanoscale energy storage system with significant potential for practical use and scalability due to their natural abundance.103,104 Aqueous crystallohydrate solutions were prepared and various amounts of sepiolite were added resulting in crystallohydrate/sepiolite dispersions with different crystallohydrate contents (50, 60, 70, 80, 90%). These dispersions were then subjected to ultrasound treatment at 40 °C for 30 minutes, followed by a 10-minute incubation at room temperature. The process was repeated twice to saturate the sepiolite. Composites containing 50% Na2HPO4·12H2O and 50% Na2SO4·10H2O demonstrated outstanding thermal energy storage capabilities during heat uptake and release. Sepiolite possesses a unique nanotubular structure, providing a high surface area and demonstrating the ability to accommodate many of hydrated salts. Water molecules and active groups on the sepiolite surface facilitate interactions with these salts.105,106 Sepiolite-based composites have great potential for thermal energy storage and utilisation, which could be beneficial for temperature regulation in household applications and harnessing low-temperature waste heat.
2.3. Nanocontainers for thermal energy storage
Various types of materials are available for thermal energy storage. Solid–liquid phase change materials (PCMs) are among the most promising options due to their high energy density and minimal volume changes during phase transitions. However, ideal PCMs that meet all the necessary criteria, such as high thermal conductivity and safety, have not yet been found. Organic paraffin and crystallohydrates are the most studied, but they also have their drawbacks, such as low thermal conductivity for paraffin and incongruent melting and corrosiveness for crystallohydrates.76
The energy properties of solid–liquid PCMs can be enhanced by encapsulating them into micro and nanocontainers. These containers must possess several smart properties, such as controlled release of thermal energy, protection against corrosion and damage, increased contact area with the surrounding environment, and improved thermal conductivity. Energy capsules can thus be used in various bulk systems to impart thermal energy storage properties suitable for practical applications. Graham et al.107 demonstrated a universal method for encapsulating crystallohydrates (Mg(NO3)2·6H2O and Na2SO4·10H2O) and their mixtures into capsules ranging in size from 100 to 300 nm with a poly(ethyl-2-cyanoacrylate), PECA, shell using in situ inversion mini-emulsification combined with ultrasound treatment (Fig. 3).
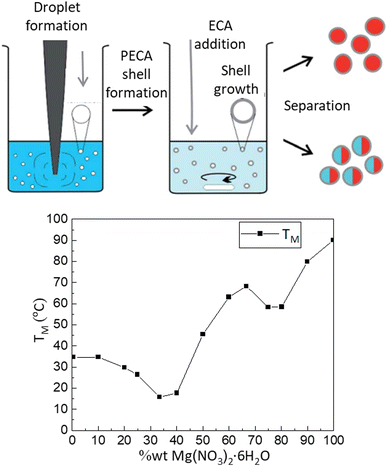 |
| Fig. 3 The process of encapsulating hydrate-loaded capsules: creating a mini-emulsion using ultrasound, forming a PECA shell around the aqueous phase, and the preparation of nanocapsules with either a single or mixed hydrate core. Reproduced from ref. 107 with permission of publisher. Copyright 2017 The Royal Society of Chemistry. | |
The encapsulated crystallohydrates and their mixtures exhibit high stability during energy storage/release processes (>100 cycles, monitored by differential scanning calorimetry, DSC) due to the functional properties of the capsule shell and spatial confinement preventing water loss and incongruent melting during phase transitions.
2.4. Microfluidic synthesis
Polyurethane capsules were synthesised via droplets formed inside a microfluidic reactor.108,109 The capabilities of Dolomite droplet formation are demonstrated in Fig. 4. However, due to the cost of each Dolomite device, it is not worth the risk of polymerisation within. This is where fused deposition modeling (FDM) printed devices would be beneficial in testing in-channel encapsulation.110 Microencapsulation of calcium chloride hexahydrate in resorcinol resin shell was also demonstrated in a microfluidic reactor.111 Microcapsules with uniform coating thickness were proved from the optical microscope as well as scanning electron microscope images. DSC results demonstrated that PCM melting in microencapsulated state occurred at a temperature which is within 1 °C from the melting point of bulk PCM. DSC profile after 50 heating/cooling cycles did not show significant changes.
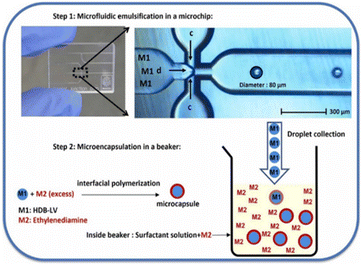 |
| Fig. 4 Microcapsule synthesis via droplet production in a Dolomite device before interfacial polymerisation for shell formation. Reproduced from ref. 110 with the permission of publisher. Copyright 2012, Elsevier. | |
Palazzo et al.112 demonstrated up-scaled encapsulation by supercritical CO2 emulsification using stearic acid and isopropyl myristate as PCMs in a polymethyl methacrylate (PMMA) shell. Continuous supercritical CO2 emulsion extraction technology was proposed for the first time to test the encapsulation of stearic acid and isopropyl myristate in PMMA. Spherical microcapsules with smooth surface and average diameter between 163–431 nm were produced. Encapsulation efficiency up to 91% was recorded. Thermograms of the obtained capsules indicated absence of chemical reaction between the core and shell and demonstrated thermal stability for at least 500 heat uptake/release cycles.
3. 2D materials for charge storage
The rapid development of technology and the need for more energy have significantly increased the demand for energy storage devices.113 Flexible supercapacitors (SCs), characterised by a simple manufacturing process, low cost, fast charging, discharge speed and long service life, are particularly interesting among other electrochemical energy storage devices (EES).114 Due to portability, SCs are easily integrated into wearable sensor devices115 or different types of EES. The efficiency of supercapacitors is based on the electron/ion transfer and the boundaries/surfaces behaviour during the electrochemical reaction, influencing on the reaction kinetics of the electrode material.116,117
Two-dimensional materials are considered innovative and promising candidates for various types of EES with excellent electrical, electrochemical and mechanical properties: (1) atomic or nanoscale thickness with excellent mechanical properties gives flexibility and durability, which is especially interesting in the development of novel energy storage devices; (2) 2D nanosheets have an ultra-large specific surface area and a better charge distribution, which contributes to the Faraday reaction rate of battery-type electrode materials and improves the storage capacity of capacitor-type electrode materials; (3) the variety of 2D materials allows the development of materials with different chemical compositions and adjustable electrical and electrochemical properties.118,119 There are many 2D materials with various properties, among which graphene and graphene derivatives are particularly distinguished: graphene oxide (GO), reduced graphene oxide (rGO),14,120 and graphene nanosheets.121 The following 2D-material are also widely used: MXenes,122,123 metal–organic frameworks (MOFs),124,125 transition metal dichalcogenides (TMDs),126 covalent organic frameworks (COFs),127 2D coordinated polymer.128
3.1. Graphene–graphene-based materials
Graphene is a single layer of hexagonally packed carbon atoms. The sp2-hybridization of carbon atoms allows delocalising electrons from π bonds, which allows free movement between carbon atoms and creates high electrical conductivity. Graphene also has high specific surface area, extraordinary strength, low density, flexibility and easy chemical processing. These characteristics make graphene and its derivatives ideal materials for electrochemical double-layer capacitor (EDLC) electrodes (Fig. 5). The capacitance characteristics of graphene-based EDLCs depend on several factors, such as specific surface area, pore size distribution, interlayer spacing, heteroatom doping, surface functionality and conductivity.14,129 Unlike other carbon materials, such as nanotubes, graphene is a strong electrode material due to the strong van der Waals inter-sheet force.130 However, such a strong attraction contributes to the self-rearrangement of graphene sheets, which decreases the surface area and ion paths for operational charge/discharge.38,39 Therefore, the actual specific capacity of graphene is lower than the theoretical one.131 This problem can be solved in two ways: physically increasing the gap between graphene nanosheets and chemical modification of graphene nanosheets to create repulsive forces between them.132 Various 2D materials can be used as spacers to prevent graphene stacking. According to Wang et al.,132 such spacers should: (1) have a thin and extended 2D network for the physical separation of two adjacent graphene nanosheets; (2) contain mesopores with high porosity to expose graphene surface for ion adsorption/desorption; (3) facilitate the unhindered ion-transportation; and (4) have excellent chemical stability without compromising cycling life. Thus, the formation of rGO/COF hybrids with a COF as a spacer prevented the laying of rGO nanolists and maximised their surface accessibility to electrolyte ions. GO and rGO were used in a similar way to avoid MXenes stacking.133,134
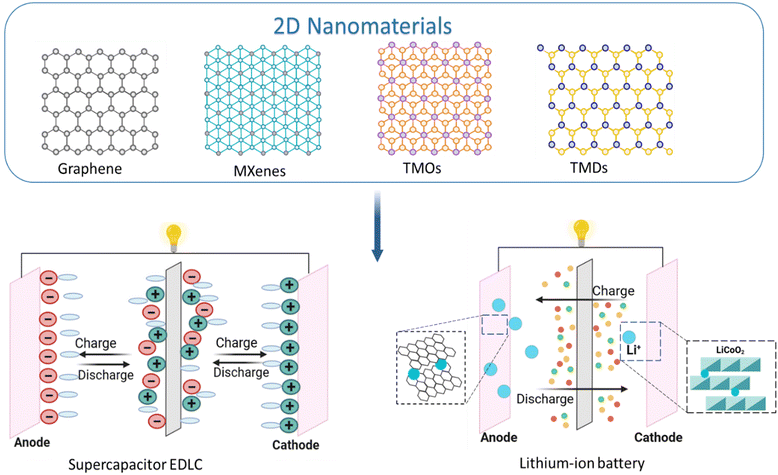 |
| Fig. 5 Various 2D nanomaterials for EDLC and LIB electrode applications. | |
3.2. Metal-ion batteries and supercapacitors
For a long time, metal-ion batteries have been widely used worldwide as energy storage devices. There are different types of batteries, depending on the metal.135 However, lithium-ion batteries have become mostly widespread (LIB) (Fig. 5). The characteristics of LIB are mostly influenced by the materials from which the electrodes are made. A traditional lithium-ion battery consists of two electrodes, typically lithium cobalt oxide (LiCoO2) cathode and graphite (C6) anode separated by a porous separator immersed in a non-aqueous liquid electrolyte. Li-ions move from the LiCoO2 lattice structure to the anode side during charging to form lithiated graphite (LiC6).18 Due to the limited storage capacity and moderate diffusion rate of lithium ions in electrode active materials, it is necessary to develop and use new materials to replace existing ones.136
Another widespread type of electrochemical energy storage device is supercapacitors.137 The energy storage mechanism in a supercapacitor originated from the reversible reaction on the surfaces of the electrode materials, which includes charge separation and a faradaic redox reaction at the electrode–electrolyte interface.138,139 Three types of supercapacitors can be distinguished depending on the mechanism of energy storing: electrical double-layer capacitor (EDLC), pseudocapacitor and hybrid EDLC supercapacitor.19
The energy storage process of EDLC (Fig. 5) is based on ion migration. Although the EDLC energy storage mechanism is almost similar to a traditional capacitor and pseudocapacitor, there is an important difference. The mechanism of EDLC does not involve the Faraday redox reaction. Due to this feature, the EDLC is characterised by high stability and charge/discharge rate. However, the EDLC does not have a large specific capacitance like the pseudocapacitor. A hybrid supercapacitor offers electrical double-layer and pseudocapacitor mechanisms providing high operating potential windows and improved energy densities without compromising power density.19,140
3.3. Methods of electrode fabrication
Several graphene-based films are prepared for supercapacitors by different methods: vacuum filtration, vacuum-assisted self-assembly, layer-by-layer self-assembly, mechanical compression and solvent evaporation. These methods allow the creation of films with excellent electrical conductivity and the necessary mechanical properties, but they have some disadvantages. Thus, the methods of vacuum filtration, vacuum-assisted self-assembly and mechanical compression require expensive laboratory equipment and are time-consuming. Fabrication of electrode materials by the solvent evaporation method takes 5–10 minutes. It allows the change of the parameters of the film, but there is a possibility of destruction of the film structure by the produced bubbles.141 Yun et al.,142 the LbL method was used to form layers of rGO and MXene on a wire substrate, which is not easy to do by vacuum filtration. It should also be noted that in comparison with conventional GO membranes prepared by vacuum filtration, multilayer GO membranes assembled by LbL differed in internal structure, wettability and ion permeability.143
Special attention should be paid to the combination of graphene-based materials with polyelectrolytes. Due to their electrical conductivity and chemical and mechanical strength, polyelectrolytes are used as materials for EDLCs electrodes in supercapacitors.144 In addition to these properties, polyelectrolytes can form various structures and layers depending on parameters such as their molecular weight, pH and ionic strength of the solution.145 Thus, it is possible to create layers of films with specific parameters using LbL. There are reports discussing the formation of thin films based on graphene nanosheets and graphene-based structures included in a polyelectrolyte matrix of poly(allylamine hydrochloride),146 polyaniline,147 and poly(3,4-ethylenedioxythiophene).148 Also, Kulandaivalu et al.149 demonstrated polypyrrole (PPy) to form PPy/GO|PPy/MnO2 layers by LbL. This film improves the electrochemical properties and performance of supercapacitor electrodes by combining the redox reactions of metal oxides with a high surface area/conductivity of graphene. The LbL was successfully used to form rGO/polyelectrolyte films. rGO sheets have a negative charge, due to which they can bind to various polycationic azopolyelectrolytes.150
4. Application of phase change materials for thermal energy storage
Phase change materials can store thermal energy by undergoing a phase transition, such as from solid to liquid or liquid to gas. PCMs have various applications, including cooling in large areas, regulating temperature fluctuations in buildings or containers, and storing solar energy for short or long periods.67 When thermal energy comes from periodic sources like solar radiation or waste heat, PCMs offer a compact and efficient way to store and access heat. This section discusses the properties of PCMs improved in combination with 2D materials,80 focusing on applications for heat storage and solar energy conversion. The safety and sustainability of PCMs are also considered, along with research challenges that need to be addressed for the widespread use of PCMs.151,152
4.1. PCM composites for controlled heat storage
PCMs can be classified into three main categories based on their chemical nature: organic (o-PCMs), inorganic (io-PCMs) and a combination of both called eutectic (eu-PCMs).76,153–159 PCMs can also be categorised based on their operating temperature range, latent heat of fusion and energy storage mode. An ideal PCM should have all the necessary characteristics for effective thermal energy storage, but often, a combination of materials is used to achieve the desired properties. The different subgroups of PCMs have a wide range of physical, thermal and chemical properties, which can be used to design thermal energy storage systems.151,160–163
Solid–solid PCMs are made of polymer-based materials, such as polyurethanes and polyalcohols. Liquid–gas and gas–solid PCMs are not commonly used because they require large volume and high-pressure systems. Solid–liquid PCMs are the most practical for thermal energy systems. Solid–liquid PCMs absorb heat and behave similarly to the sensible heat storage materials until they reach their phase transition temperature. At this point, they absorb energy without a significant temperature rise and transform into the liquid phase.152,164,165 PCMs solidify and release the up-taken latent heat when the temperature decreases. o-PCMs, such as paraffin and nonparaffins, have a temperature range of 5–160 °C. io-PCMs, such as salt hydrates and metallic-compounds-based materials, have an effective temperature range of 10–900 °C. Eu-PCMs fall between the temperature ranges of o-PCMs and io-PCMs.166–168
In a recent paper,155 PCMs were developed as microcapsules containing n-docosane (Fig. 6). Then, energy capsules were used to impregnate cotton fabrics for thermal energy storage. These fabrics had temperature buffering effect of 11 °C during heating when impregnated with 8 wt% of the microcapsules. They also showed a temperature increase of 6 °C during cooling over 100 cycles of heat uptake/release. This performance was maintained even after storing the fabrics at room temperature for 4 years. In aged fabric composites, the temperature buffering effect during heating increased to 14 °C, and the temperature increase effect during cooling reached 9 °C. The study suggests that microencapsulated n-docosane has great potential for thermal management applications in various industries.
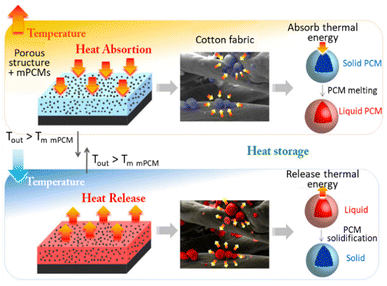 |
| Fig. 6 Microcapsules PCM integrated in cotton fabrics to improve the thermoregulating for commercial applications. Reproduced from ref. 155 with the permission of publisher. Copyright 2012 American Chemical Society. | |
4.2. 2D materials for solar energy conversion
Utilizing PCM for solar energy storage offers improved energy storage capacity, prolonged heat storage, increased energy conversion efficiency, flexibility in system design and environmental benefits. These advantages make them a promising solution for enhancing the performance and viability of solar energy systems.169–172 Solar energy storage using PCMs offers a solution to overcome the intermittent nature of solar radiation and enables continuous operation of heating-related processes. Tao et al.173 designed magnetically moving mesh-structured solar absorbers within molten salts to enhance the solar-thermal energy storage rates while maintaining 100% storage capacity. Current storage systems are limited by the low thermal conductivity of PCMs, which hinders their energy-harvesting performance. Presented magnetically movable charging strategy increases the latent heat solar-thermal energy harvesting rate by 107% and supports large-area charging and batch-to-batch storage. The system can be easily integrated into heat exchanging systems to provide abundant and clean solar-thermal energy for water and space heating.
Shi et al.174 conducted a study of photo-responsive PCM that can store latent heat and photon energy from sunlight. The ortho-substitution of azobenzene units allowed photo-switching by irradiation at >530 nm wavelength. Adding an aliphatic group provided a transition between solid and liquid states. The ability to achieve photo-controlled latent heat storage through solar irradiation represents a significant advancement in the field. It opens new avenues for solar energy harvesting utilising functional organic materials, which can be involved in existing systems such as photocatalysts and photovoltaic materials.
Composites based on 2D materials combined with PCM are very effective for solar energy storage. Fan et al.175 developed a new composite PCM based on PEG and Ti3C2Tx nanosheets. The material has high thermal energy storage density. It exhibits strong absorption of electromagnetic waves in the UV-Vis-NIR region due to the localised surface plasmon resonance effect of the Ti3C2Tx nanosheets. As a result, PCM has excellent photo-to-thermal storage efficiency, reaching up to 94.5% under solar light irradiation. Additionally, the composite maintains high energy storage density and remains stable before and after the phase transition. These findings demonstrate that synthesised PCM has superior properties that makes it suitable for solar energy storage applications. PCMs provide a compact and efficient way to store and access heat, especially when the thermal energy comes from intermittent sources like solar radiation or waste heat.176,177
5. Machine learning for the optimisation of the material
Machine learning (ML) has revolutionized various fields of science and technology, including materials science. Although ML technology appeared in the mid-20th century, its practical use became possible only after the computational power of personal computers significantly increased. ML algorithms require significant computational resources, which allow them to analyze huge volumes of data and identify patterns that would be difficult or impossible for humans to detect. In the field of material optimization, artificial intelligence can accelerate the discovery and development of new materials with desired properties.178–180 Therefore, many researchers in the field of materials science are interested in how to apply ML methods in their work. Fig. 7a shows growing interest in using machine learning for materials.
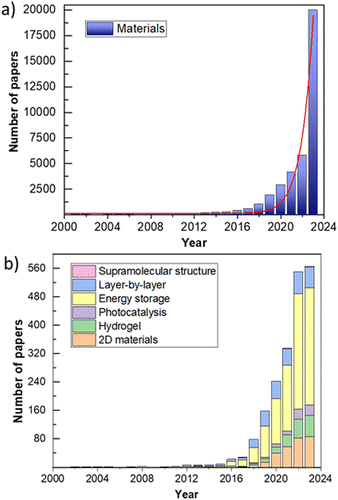 |
| Fig. 7 Histogram of number of scientific publications per year in the Scopus database on the topics (a) machine learning and materials (solid line is an exponential approximation); (b) machine learning and 2D materials/hydrogel/photocatalysis/energy storage/layer-by-layer/supramolecular structure. | |
Using ML methods, researchers can predict material properties before their synthesis, reducing the time and costs required for experiments. The main advantage of this approach is the minimization of harmful effects during the production of certain materials.181,182 Therefore, research on machine learning and materials optimization is rapidly developing, especially in the last 10 years, and is already for design of 2D materials,183–185 hydrogels,186–189 supramolecular structures,190 processes of photocatalysis,191–194 energy storage,195–197 layer-by-layer structures198,199 and others (Fig. 7b).200–203
In this section, we will discuss the current state of applications in optimizing various 2D materials and discuss the problems and opportunities in this rapidly developing field.
5.1. ML for 2D-materials
Currently, one of the most important directions in materials science is using machine learning as an effective tool for studying 2D materials for catalysis, energy storage, electronics, optics and biomedical applications. These materials form the basis of modern technologies, and therefore, developing desired properties and changing physical characteristics are important directions. Pure materials are rarely used, and most devices and technologies require careful design of material properties, which can be achieved through doping, creating heterostructures of composites, or controlled introduction of defects. Using computational or experimental data of material properties, machine learning algorithms can predict the structural, electronic, mechanical, and chemical properties of two-dimensional materials that are yet to be discovered. However, to solve such problems, developing an efficient learning model and creating a high-quality database are necessary.
In particular, Novoselov et al. demonstrated an approach to revealing the complex correlation between the structure and properties of defects in 2D materials based on high-throughput data sets.204 The main task is to compile the correct structured data set to establish and control the structure–property relationships of defects. In the study, the database was compiled using information on the electronic properties of defects obtained from Density Functional Theory calculations in MoS2 2D crystals. Defects are often used as a modification tool because they contribute to changes in the properties of solids and, as a result, the properties of 2D materials used in single-atom catalysis. Therefore, it is important to understand the electronic properties of defects in 2D materials. In this study, the distribution of properties of defect configurations was studied by creating a map of the dependence of the forbidden zone on the energy of formation. They also extensively studied the properties of double and triple defects, explaining the variations in properties using symmetry and quantum mechanical analysis of the electronic structure. This study allows for predicting changes in material properties when various defects are introduced by properly organising data and understanding the physics.
5.2. Big data analysis by computation methods
In addition to material optimisation tasks, machine learning can analyse large amounts of data. Modern detection methods are important tools for timely fighting various diseases caused by bacteria or viruses. However, many of them take time and require significant resources. In contrast, machine learning algorithms can quickly and accurately analyse large data sets and extract characteristic features, providing a more efficient and economical approach to pathogen detection. Skorb et al. demonstrated the use of machine learning for data analysis as a platform for rapid bacterial detection205 and tick-borne encephalitis detection.206 This system is based on using a soft hydrogel/eutectic gallium–indium alloy and allows registering nonlinear volt-ampere characteristics depending on the composition of the hydrogel. Hydrogel is a material that has a high water retention capacity and can be used to create an environment conducive to the growth of a pathogenic organisms. ML algorithms were trained on a database collected experimentally by electrochemical characteristics of the system, in particular voltammetric voltage.
Machine learning can also be one of the most effective ways to study and develop new photocatalysts that stimulate further theoretical and experimental research on multicomponent compounds in photocatalytic water splitting. One way to increase the photocatalytic activity of a material is by doping, which involves modifying its optical and electronic properties. Although studies on material doping have been conducted for decades, optimal dopants are still experimentally selected by trial and error. Machine learning can be a promising approach to determining the optimal dopants for high-performance systems by establishing correlations between dopant characteristics and doped materials, which may appear ambiguous when using traditional methods. This reduces the time and costs of experimental research and accelerates the process of discovering new promising materials for use in photocatalytic water splitting. Wang et al. demonstrated a machine learning model predicting changes in the efficiency of photoelectrodes.207 This work is based on studying changes in the properties of hematite by incorporating 17 different metallic dopants. It was found that when selecting a dopant to improve the material's efficiency, it is necessary to consider the chemical state, ionic radius, and enthalpy of the formation of the metal–oxygen bond. This study demonstrates the possibility of identifying and establishing correlations between dopant characteristics and the properties of doped photoelectrodes, which may be non-obvious for traditional experimental methods.
5.3. Usage ML for topological data analysis
Very interesting application for ML is imageanalysis. In recent papers the surface roughness of LbL polyelectrolytes was investigated by using atomic force microscopy.123,208 The analysis employs innovative techniques such as topological data analysis (TDA) and ML to establish a correlation between multiscale roughness and the number of bilayers as well as to identify the specific type of polyelectrolytes involved. Here researchers compared assemblies polyethyleneimine (PEI)/poly (sodium 4-styrene sulfonate) and PEI/MXene rigid flakes. (Fig. 8)
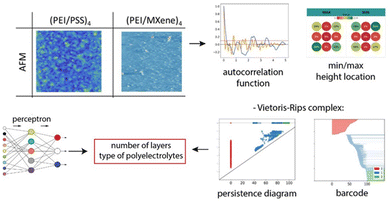 |
| Fig. 8 Schematic representation of the topological data analysis from atomic force microscope images. Reproduced from ref. 209 with the permission of publisher. Copyright 2023 American Chemical Society. | |
As the number of bilayers increases, the surface roughness shifts from a smooth profile to an equilibrium roughness. The AFM analysis reveals that the surface morphology exhibits multiscale roughness, with smaller features superimposed on larger ones. To ensure accurate roughness data, various methods are employed, such as correlation length calculations, statistical analysis of extreme values in trimmed images, and the use of TDA barcodes and persistence diagrams in an 8D data space. Additionally, ML algorithm is utilized to determine the number of bilayers in polyelectrolytes. The roughness analysis demonstrates a gradual shift from a smooth to a rough surface, reaching saturation at around three to four bilayers. Moreover, the existence of multiscale roughness invariance is observed.
The use of machine learning for material optimisation has opened up new opportunities for researchers to accelerate the discovery and development of new materials with desired properties. Artificial intelligence can be integrated at any stage of the material design process, depending on the goals set. This includes the selection of precursors, synthesis parameters, material formation conditions, and their properties. However, some challenges need to be addressed, such as the need for high-quality data and the development of practical machine learning models.
The database for training machine learning algorithms is typically formed using various methods such as experimental data, existing databases, simulations, and modelling. As this field continues to evolve, researchers need to collaborate and share data further to develop the capabilities of machine learning in material optimisation. Thanks to constant innovation and collaboration, the future of materials science with the integration of machine learning methods looks promising. In addition to tasks related to materials optimisation, machine learning can be used as a tool for analysing large volumes of data.
6. Conclusions and perspectives
In conclusion, this review highlights the potential of layered materials in the field of renewable energy and explores the use of machine learning techniques to enhance their performance. The following is some potential explorations:
1. 2D materials, such as graphene, transition metal dichalcogenides and black phosphorus, exhibit exceptional properties that make them promising candidates for various renewable energy applications and more. These materials possess high electrical conductivity, excellent mechanical strength, and unique optical properties, enabling their utilization in solar cells, batteries and supercapacitors.
2. The review emphasizes the importance of understanding the structure–property relationships of layered materials to optimize their performance in renewable energy devices. By manipulating the number of layers, doping, and defect engineering, researchers can tailor the properties of these materials to enhance their efficiency, stability, and functionality.
3. Encapsulated or layered PCMs demonstrate significant potential in energy storage applications. Their high surface area, ability to store large amount of heat in small volume and excellent electrical conductivity enable controlled thermal energy storage. The review highlights the advancements in PCMs energy storage systems, including increased energy density, longer cycle life, and faster energy exchange capabilities.
4. Machine learning algorithms offer a powerful tool for predicting and optimizing the properties of 2D materials. Through the application of supervised and unsupervised learning techniques, researchers can efficiently screen a vast number of potential materials, identify their optimal structures, and predict their performance for specific energy-related applications. This approach significantly accelerates the discovery and development of new materials with enhanced efficiency and functionality. Furthermore, the integration of 2D materials with machine learning techniques enables the development of smart energy storage systems. By leveraging the predictive capabilities of machine learning algorithms, researchers can optimize the charge–discharge behaviour of batteries and supercapacitors based on 2D materials. This leads to improved energy storage capacity, more heat uptake/release cycles, and enhanced overall performance.
In summary, our review demonstrates the immense potential of 2D materials in renewable energy applications. By combining their unique properties with machine learning techniques, researchers can accelerate the discovery of new materials, optimize their performance, and pave the way for the development of more efficient and sustainable energy technologies.
Author contributions
DGS and EVS were involved in writing original draft, funding acquisition and editing. Other co-authors participated in data curation, analysis and investigation. All co-authors equally contributed to this article.
Conflicts of interest
There are no conflicts to declare.
Acknowledgements
Authors acknowledge RSF grant no. 21-13-00403 for the support. We thank the Priority 2030 for infrastructural supported. DGS acknowledges support from Alexander von Humboldt foundation.
Notes and references
- H. Lu, J. Tournet, K. Dastafkan, Y. Liu, Y. H. Ng, S. K. Karuturi, C. Zhao and Z. Yin, Chem. Rev., 2021, 121, 10271–10366 CrossRef CAS PubMed.
- N. Abas, A. Kalair and N. Khan, Futures, 2015, 69, 31–49 CrossRef.
- S. Schwietzke, O. A. Sherwood, L. M. P. Bruhwiler, J. B. Miller, G. Etiope, E. J. Dlugokencky, S. E. Michel, V. A. Arling, B. H. Vaughn, J. W. C. White and P. P. Tans, Nature, 2016, 538, 88–91 CrossRef CAS PubMed.
- D. Welsby, J. Price, S. Pye and P. Ekins, Nature, 2021, 597, 230–234 CrossRef CAS PubMed.
- F. Zhang, P. Zhao, M. Niu and J. Maddy, Int. J. Hydrogen Energy, 2016, 41, 14535–14552 CrossRef CAS.
- Priya, P. S. Deora, Y. Verma, R. A. Muhal, C. Goswami and T. Singh, Mater. Today Proc., 2021, 48, 1178–1184 CrossRef.
- L. Dai, K. Huang, Y. Xia and Z. Xu, Green Energy Environ., 2021, 6, 193–211 CrossRef CAS.
-
P. Carpejani, É. T. de Jesus, B. L. S. Bonfim Catapan, S. E. Gouvea da Costa, E. Pinheiro de Lima, U. Tortato, C. G. Machado and B. K. Richter, International Business, Trade and Institutional Sustainability, 2020.
-
D. B. Pal and J. M. Jha, Sustainable and Clean Energy Production Technologies, 2022.
- F. Yi, H. Ren, J. Shan, X. Sun, D. Wei and Z. Liu, Chem. Soc. Rev., 2018, 47, 3152–3188 RSC.
- Q. Guo, N. Chen and L. Qu, Carbon Energy, 2020, 2, 54–71 CrossRef.
- O. A. Moses, L. Gao, H. Zhao, Z. Wang, M. Lawan Adam, Z. Sun, K. Liu, J. Wang, Y. Lu, Z. Yin and X. Yu, Mater. Today, 2021, 50, 116–148 CrossRef CAS.
- E. Tan, B. L. Li, K. Ariga, C.-T. Lim, S. Garaj and D. T. Leong, Bioconjug. Chem., 2019, 30, 2287–2299 CrossRef CAS PubMed.
- G. F. Smaisim, A. M. Abed, H. Al-Madhhachi, S. K. Hadrawi, H. M. M. Al-Khateeb and E. Kianfar, Bionanoscience, 2023, 13, 219–248 CrossRef.
- Y. Tian, Z. Yu, L. Cao, X. L. Zhang, C. Sun and D.-W. Wang, J. Energy Chem., 2021, 55, 323–344 CrossRef CAS.
- S. Wang, S. Zhao, X. Guo and G. Wang, Adv. Energy Mater., 2022, 12, 1–21 Search PubMed.
- X. Tian, Y. Yi, Z. Wu, G. Cheng, S. Zheng, B. Fang, T. Wang, D. G. Shchukin, F. Hai, J. Guo and M. Li, Chem. Eng. Sci., 2023, 266, 118271 CrossRef CAS.
- J. Xie and Y.-C. Lu, Nat. Commun., 2020, 11, 2499 CrossRef CAS PubMed.
- M. Tomy, A. Ambika Rajappan, V. VM and X. Thankappan Suryabai, Energy Fuels, 2021, 35, 19881–19900 CrossRef CAS.
- W. Zhang, R. Mazzarello, M. Wuttig and E. Ma, Nat. Rev. Mater., 2019, 4, 150–168 CrossRef CAS.
- H. Wang, Y. Wang, Z. Ni, N. Turetta, S. M. Gali, H. Peng, Y. Yao, Y. Chen, I. Janica, D. Beljonne, W. Hu, A. Ciesielski and P. Samorì, Adv. Mater., 2021, 33, 1–9 Search PubMed.
- F. Gao, H. Yang and P. A. Hu, Small Methods, 2018, 2, 1–13 Search PubMed.
- L. Spitthoff, P. R. Shearing and O. S. Burheim, Energies, 2021, 14, 1248 CrossRef CAS.
- D. V. A. K. Yang, Z. Hu, X. Li, K. Nikolaev, G. K. Hong, N. Mamchik, I. Erofeev, U. M. Mirsaidov, A. H. Castro Neto, D. J. Blackwood, D. G. Shchukin, M. Trushin and K. S. Novoselov, Proc. Natl. Acad. Sci. U. S. A., 2023, 120, e2307618120 CrossRef.
- A. T. Press, P. Babic, B. Hoffmann, T. Müller, W. Foo, W. Hauswald, J. Benecke, M. Beretta, Z. Cseresnyés, S. Hoeppener, I. Nischang, S. M. Coldewey, M. H. Gräler, R. Bauer, F. Gonnert, N. Gaßler, R. Wetzker, M. T. Figge, U. S. Schubert and M. Bauer, EMBO Mol. Med., 2021, 13, e14436 CrossRef CAS.
- R. M. Nauman Javed, A. Al-Othman, M. Tawalbeh and A. G. Olabi, Renew. Sustainable Energy Rev., 2022, 168, 112836 CrossRef CAS.
- O. Okhay and A. Tkach, Nanomaterials, 2021, 11, 1240 CrossRef CAS PubMed.
- F. Farjadian, S. Abbaspour, M. A. A. Sadatlu, S. Mirkiani, A. Ghasemi, M. Hoseini-Ghahfarokhi, N. Mozaffari, M. Karimi and M. R. Hamblin, ChemistrySelect, 2020, 5, 10200–10219 CrossRef CAS.
- M. Singh, A. Shukla and B. Chakraborty, Int. J. Hydrogen Energy, 2022, 1, 1–14 CrossRef.
- X. Gao, H. Liu, D. Wang and J. Zhang, Chem. Soc. Rev., 2019, 48, 908–936 RSC.
- J. Li, Z. Chen, H. Yang, Z. Yi, X. Chen, W. Yao, T. Duan, P. Wu, G. Li and Y. Yi, Nanomaterials, 2020, 10, 257 CrossRef CAS.
- V. H. Nguyen, T. P. Nguyen, T. H. Le, D. V. N. Vo, D. L. T. Nguyen, Q. T. Trinh, I. T. Kim and Q. Van Le, J. Chem. Technol. Biotechnol., 2020, 95, 2597–2607 CrossRef CAS.
- P. Lin, J. Xie, Y. He, X. Lu, W. Li, J. Fang, S. Yan, L. Zhang, X. Sheng and Y. Chen, Sol. Energy Mater. Sol. Cells, 2020, 206, 110229 CrossRef CAS.
- A. Bhat, S. Anwer, K. S. Bhat, M. I. H. Mohideen, K. Liao and A. Qurashi, npj 2D Mater. Appl., 2021, 5, 1–21 CrossRef.
- H. Huang, T. Shi, R. He, J. Wang, P. K. Chu and X. F. Yu, Adv. Sci., 2020, 7, 1–7 Search PubMed.
- S. Kaushik and R. Singh, Adv. Opt. Mater., 2021, 9, 1–20 Search PubMed.
- C. Cho, P. Kang, A. Taqieddin, Y. Jing, K. Yong, J. M. Kim, M. F. Haque, N. R. Aluru and S. Nam, Nat. Electron., 2021, 4, 126–133 CrossRef CAS PubMed.
- H. Hou, C. Anichini, P. Samorì, A. Criado and M. Prato, Adv. Funct. Mater., 2022, 32, 2207065 CrossRef CAS.
- X. Xu, Z. Lou, S. Cheng, P. C. Y. Chow, N. Koch and H.-M. Cheng, Chem, 2021, 7, 2989–3026 CAS.
- S. Noreen, M. B. Tahir, A. Hussain, T. Nawaz, J. U. Rehman, A. Dahshan, M. Alzaid and H. Alrobei, Int. J. Hydrogen Energy, 2022, 47, 1371–1389 CrossRef CAS.
- K. S. Novoselov, A. K. Geim, S. V. Morozov, D. Jiang, Y. Zhang, S. V. Dubonos, I. V. Grigorieva and A. A. Firsov, Science, 2004, 306, 666–669 CrossRef CAS PubMed.
- C. Yang, H. F. Wang and Q. Xu, Chem. Res. Chinese Univ., 2020, 36, 10–23 CrossRef CAS.
- H. Zhang, ACS Nano, 2015, 9, 9451–9469 CrossRef CAS PubMed.
- Q. Cui, Y. Zhong, L. Pan, H. Zhang, Y. Yang, D. Liu, F. Teng, Y. Bando, J. Yao and X. Wang, Adv. Sci., 2018, 5, 1700902 CrossRef PubMed.
- F. Yi, H. Ren, J. Shan, X. Sun, D. Wei and Z. Liu, Chem. Soc. Rev., 2018, 47, 3152–3188 RSC.
- C. Li, Q. Cao, F. Wang, Y. Xiao, Y. Li, J.-J. Delaunay and H. Zhu, Chem. Soc. Rev., 2018, 47, 4981–5037 RSC.
- Z. Xie, Y.-P. Peng, L. Yu, C. Xing, M. Qiu, J. Hu and H. Zhang, Sol. RRL, 2020, 4, 1900400 CrossRef.
- Z. Xiao, R. Wang, D. Jiang, Z. Qian, Y. Li, K. Yang, Y. Sun, Z. Zeng and F. Wu, ACS Appl. Energy Mater., 2021, 4, 7440–7461 CrossRef CAS.
- Y. Zhu, S. Wang, J. Ma, P. Das, S. Zheng and Z.-S. Wu, Energy Storage Mater., 2022, 51, 500–526 CrossRef.
- X. Li, Z. Huang, C. E. Shuck, G. Liang, Y. Gogotsi and C. Zhi, Nat. Rev. Chem., 2022, 6, 389–404 CrossRef PubMed.
- Y. Zhang, Y. Zheng, K. Rui, H. H. Hng, K. Hippalgaonkar, J. Xu, W. Sun, J. Zhu, Q. Yan and W. Huang, Small, 2017, 13, 1700661 CrossRef.
- Z. Zhang, X. Liu, J. Yu, Y. Hang, Y. Li, Y. Guo, Y. Xu, X. Sun, J. Zhou and W. Guo, WIREs Comput. Mol. Sci., 2016, 6, 324–350 CrossRef CAS.
- S. Wu, K. S. Hui and K. N. Hui, Adv. Sci., 2018, 5, 1700491 CrossRef PubMed.
- M. C. Jenkins and S. Lutz, ACS Synth. Biol., 2021, 10, 857–869 CrossRef CAS PubMed.
- D. Grigoriev, E. Shchukina and D. G. Shchukin, Adv. Funct. Mater., 2017, 4, 1600318 Search PubMed.
- S. Eiben, C. Koch, K. Altintoprak, G. Tovar, S. Laschat, I. M. Weiss and C. Wege, Adv. Drug Delivery Rev., 2019, 145, 96–118 CrossRef CAS PubMed.
- D. Diaz, A. Care and A. Sunna, Genes, 2018, 9, 370 CrossRef PubMed.
- S. Pugh, R. Mckenna, I. Halloum and D. R. Nielsen, Metab. Eng. Commun., 2015, 2, 39–45 CrossRef PubMed.
- C. S. Ramirez-Barria, M. Isaacs, C. Parlett, K. Wilson, A. Guerrero-Ruiz and I. Rodríguez-Ramos, Catal. Today, 2020, 357, 8–14 CrossRef CAS.
- S. Bera, F. Banerjee and S. K. Samanta, ChemNanoMat, 2023, 9, 1–6 CrossRef.
- H. Liu, X. Wang and D. Wu, Appl. Therm. Eng., 2018, 134, 603–614 CrossRef CAS.
- D. G. Shchukin, Polym. Chem., 2013, 4, 4871–4877 RSC.
- D. I. Njoku, M. Cui, H. Xiao, B. Shang and Y. Li, Sci. Rep., 2017, 1–15 CAS.
- N. Imoro, V. V. Shilovskikh, P. V. Nesterov, A. A. Timralieva, D. Gets, A. Nebalueva, F. V. Lavrentev, A. S. Novikov, N. D. Kondratyuk, N. D. Orekhov and E. V. Skorb, ACS Omega, 2021, 6, 17267–17275 CrossRef CAS PubMed.
- L. Ma, J. Wang, D. Zhang, Y. Huang, L. Huang, P. Wang, H. Qian, X. Li, H. A. Terryn and J. M. C. Mol, Chem. Eng. J., 2021, 404, 127118 CrossRef CAS.
- W. Wang, W. Li, W. Fan, X. Zhang, L. Song and C. Xiong, Chem. Eng. J., 2018, 332, 658–670 CrossRef CAS.
- B. Gholipour, Science, 2019, 366, 186–187 CrossRef CAS PubMed.
- A. A. Nikitina, S. A. Ulasevich, I. S. Kassirov, E. A. Bryushkova, E. I. Koshel and E. V. Skorb, Bioconjug. Chem., 2018, 20, 3793–3799 CrossRef.
- D. Grigoriev, D. Akcakayiran, M. Schenderlein and D. Shchukin, Corrosion, 2014, 70, 446–463 CrossRef CAS.
- E. Shchukina and D. G. Shchukin, Langmuir, 2019, 35, 8603–8611 CrossRef CAS.
- D. G. Shchukin and H. Möhwald, Small, 2007, 3, 926–943 CrossRef CAS.
- D. Lombardo, M. A. Kiselev, S. Magazù and P. Calandra, Adv. Condens. Matter. Phys., 2015, 1–22 Search PubMed.
- K. A. Zahidah, S. Kakooei, M. C. Ismail and P. Bothi Raja, Prog. Org. Coat., 2017, 111, 175–185 CrossRef CAS.
- G. L. Li, H. Möhwald and D. G. Shchukin, Chem. Soc. Rev., 2013, 42, 3628–3646 RSC.
- E. V. Skorb, D. G. Shchukin, H. Möhwald and D. V. Andreeva, Nanoscale, 2010, 2, 722–727 RSC.
- E. M. Shchukina, M. Graham, Z. Zheng and D. G. Shchukin, Chem. Soc. Rev., 2018, 47, 4156–4175 RSC.
- N. Brezhneva, A. Nikitina, N. Ryzhkov, A. Klestova, A. V. Vinogradov and E. V. Skorb, J. Sol-Gel Sci. Technol., 2019, 89, 92–100 CrossRef CAS.
- V. Y. Yurova, P. I. Zyrianova, P. V. Nesterov, V. V. Goncharov, E. V. Skorb and S. A. Ulasevich, Catalysts, 2023, 13, 993 CrossRef CAS.
- A. Sharsheeva, V. A. Iglin, P. V. Nesterov, O. A. Kuchur, E. Garifullina, E. Hey-Hawkins, S. A. Ulasevich, E. V. Skorb, A. V. Vinogradov and M. I. Morozov, J. Mater. Chem. B, 2019, 7, 6810–6821 RSC.
- K. Ariga, E. Ahn, M. Park and B. Kim, Chem. – Asian J., 2019, 2553–2566 CrossRef CAS PubMed.
- A. S. Ivanov, L. V. Pershina, K. G. Nikolaev and E. V. Skorb, Macromol. Biosci., 2021, 21, 1–16 CrossRef PubMed.
- A. M. Yola, J. Campbell and D. Volodkin, Appl. Surf. Sci. Adv., 2021, 5, 100091 CrossRef.
- A. Arkhangelskiy, D. Maniglio, A. Bucciarelli, V. K. Yadavalli and A. Quaranta, Adv. Mater. Interfaces, 2021, 8, 2100324 CrossRef CAS.
- A. M. Díez-Pascual and A. Rahdar, Nanomaterials, 2022, 12, 949 CrossRef PubMed.
- C. Xu, A. R. Puente-Santiago, D. Rodríguez-Padrón, M. J. Muñoz-Batista, M. A. Ahsan, J. C. Noveron and R. Luque, Chem. Soc. Rev., 2021, 50, 4856–4871 RSC.
- S. Zhao, F. Caruso, L. Dahne, G. Decher, B. G. De Geest, J. Fan, N. Feliu, Y. Gogotsi, P. T. Hammond, M. C. Hersam, A. Khademhosseini, N. Kotov, S. Leporatti, Y. Li, F. Lisdat, L. M. Liz-Marzan, S. Moya, P. Mulvaney, A. L. Rogach, S. Roy, D. G. Shchukin, A. G. Skirtach, M. M. Stevens, G. B. Sukhorukov, P. S. Weiss, Z. Yue, D. Zhu and W. J. Parak, ACS Nano, 2019, 13, 6151–6169 CrossRef CAS PubMed.
- J. Lee, D. Kyeong, J. Kim and W. Choi, Int. J. Heat Mass Transf., 2022, 184, 122344 CrossRef CAS.
- Q. Fan, L. Wen, R. Dong, X. Hu, J. Ma, W. Zhang, X. Li and G. Carmen, Appl. Surf. Sci., 2023, 638, 158108 CrossRef CAS.
- J. Lee, J. Kim, B. Seo, D. Shin, S. Hwang and W. Choi, Int. J. Heat Mass Transf., 2023, 209, 124067 CrossRef CAS.
- N. V. Ryzhkov, N. Brezhneva and E. V. Skorb, Surf. Innov., 2019, 7, 145–167 CrossRef.
- D. V. Andreeva, M. Trushin, A. Nikitina, M. C. F. Costa, P. V. Cherepanov, M. Holwill, S. Chen, K. Yang, S. W. Chee, U. Mirsaidov, A. H. Castro Neto and K. S. Novoselov, Nat. Nanotechnol., 2020, 16, 174–180 CrossRef PubMed.
- D. Yu, X. Xiao, C. Shokoohi, Y. Wang, L. Sun, Z. Juan, M. J. Kipper, J. Tang, L. Huang, G. S. Han, H. S. Jung and J. Chen, Adv. Funct. Mater., 2023, 33, 1–35 Search PubMed.
- Y. Lanchuk, A. Nikitina, N. Brezhneva, S. A. Ulasevich, S. N. Semenov and E. V. Skorb, ChemCatChem, 2018, 10, 1798–1803 CrossRef CAS.
- N. V. Ryzhkov, N. A. Mamchik and E. V. Skorb, J. R.Soc., Interface, 2019, 16, 20180626 CrossRef PubMed.
- S. A. Ulasevich, N. Brezhneva, Y. Zhukova, H. Möhwald, P. Fratzl, F. H. Schacher, D. V. Sviridov, D. V. Andreeva and E. V. Skorb, Macromol. Biosci., 2016, 1422–1431 CrossRef CAS PubMed.
- B. V. Parakhonskiy, W. J. Parak, D. Volodkin and A. G. Skirtach, Langmuir, 2019, 35, 8574–8583 CrossRef CAS PubMed.
- E. V. Skorb and H. Möhwald, Adv. Mater., 2013, 25, 5029–5043 CrossRef CAS PubMed.
- Z. Zheng, X. Huang, M. Schenderlein, H. Moehwald, G. K. Xu and D. G. Shchukin, Nanoscale, 2015, 7, 2409–2416 RSC.
- Z. Zheng, M. Schenderlein, X. Huang, N. J. Brownbill, F. Blanc and D. Shchukin, ACS Appl. Mater. Interfaces, 2015, 7, 22756–22766 CrossRef CAS.
- E. Shchukina, H. Wang and D. G. Shchukin, Chem. Commun., 2019, 55, 3859–3867 RSC.
- D. G. Shchukin, S. V. Lamaka, K. A. Yasakau, M. L. Zheludkevich, M. G. S. Ferreira and H. Möhwald, J. Phys. Chem. C, 2008, 112, 958–964 CrossRef CAS.
- D. V. Andreeva, D. Fix, H. Möhwald and D. G. Shchukin, J. Mater. Chem., 2008, 18, 1738–1740 RSC.
- X. Zhu, V. Vinokurov, D. Kopitsyn and D. G. Shchukin, ACS Omega, 2021, 6, 25828–25834 CrossRef CAS PubMed.
- X. Zhu and D. Shchukin, Adv. Eng. Mater., 2018, 20, 1800618 CrossRef.
- Y. Luo, S. Xiong, J. Huang, F. Zhang, C. Li, Y. Min, R. Peng and Y. Liu, Sol. Energy Mater. Sol. Cells, 2021, 231, 111300 CrossRef CAS.
-
G. Tian, G. Han, F. Wang and J. Liang, Sepiolite nanomaterials: Structure, properties and functional applications, 2019.
- M. Graham, J. A. Coca-Clemente, E. Shchukina and D. Shchukin, J. Mater. Chem. A, 2017, 5, 13683–13691 RSC.
- J. Li, M. A. J. Mazumder, H. D. H. Stöver, A. P. Hitchcock and I. M. Shirley, J. Polym. Sci., Part A: Polym. Chem., 2011, 49, 3038–3047 CrossRef CAS.
- G. Hao, C. Yu, Y. Chen, X. Liu and Y. Chen, Int. J. Heat Mass Transf., 2022, 190, 122738 CrossRef CAS.
- J. Du, N. Ibaseta and P. Guichardon, Chem. Eng. Res. Des., 2022, 182, 256–272 CrossRef CAS.
- A. Dey and S. Ganguly, Therm. Sci. Eng. Prog., 2023, 40, 101776 CrossRef CAS.
- I. Palazzo and E. Reverchon, J. Supercrit. Fluids, 2023, 193, 105807 CrossRef CAS.
- S. Koohi-Fayegh and M. A. Rosen, J. Energy Storage, 2020, 27, 101047 CrossRef.
- Y. Zhang, H. Mei, Y. Cao, X. Yan, J. Yan, H. Gao, H. Luo, S. Wang, X. Jia, L. Kachalova, J. Yang, S. Xue, C. Zhou, L. Wang and Y. Gui, Coord. Chem. Rev., 2021, 438, 213910 CrossRef CAS.
- J. Liu, M. Liu, Y. Bai, J. Zhang, H. Liu and W. Zhu, Sensors, 2020, 20, 4009 CrossRef PubMed.
- C. Meng, P. Das, X. Shi, Q. Fu, K. Müllen and Z.-S. Wu, Small Sci., 2021, 1, 2000076 CrossRef CAS.
- D. Zhang, J. Lu, C. Pei and S. Ni, Adv. Energy Mater., 2022, 12, 2103689 CrossRef CAS.
- D. Zhou, L. Zhao and B. Li, J. Energy Chem., 2021, 62, 27–42 CrossRef CAS.
- M. Wu, W. Zheng, X. Hu, F. Zhan, Q. He, H. Wang, Q. Zhang and L. Chen, Small, 2022, 18, 2205101 CrossRef CAS PubMed.
- C. Wang, M. Muni, V. Strauss, A. Borenstein, X. Chang, A. Huang, S. Qu, K. Sung, T. Gilham and R. B. Kaner, Small, 2021, 17, 2006875 CrossRef CAS PubMed.
- D. A. Oliveira, R. A. da Silva, M. O. Orlandi and J. R. Siqueira, J. Mater. Sci., 2022, 57, 7023–7034 CrossRef CAS.
- A. Aglikov, O. Volkova, A. Bondar, I. Moskalenko, A. Novikov, E. V. Skorb and E. Smirnov, Chem. Phys. Chem., 2023, e202300187 CrossRef CAS PubMed.
- M. Sychov, A. Eruzin, A. Semenova, P. Katashev, S. Mjakin, M. V. Zhukov, A. Aglikov, M. Nosonovsky and E. V. Skorb, Langmuir, 2023, 39, 12336–12345 CrossRef CAS PubMed.
- A. S. Efimova, P. V. Alekseevskiy, M. V. Timofeeva, Y. A. Kenzhebayeva, A. O. Kuleshova, I. G. Koryakina, D. I. Pavlov, T. S. Sukhikh, A. S. Potapov, S. A. Shipilovskikh, N. Li and V. A. Milichko, Small Methods, 2023, 2300752 CrossRef CAS PubMed.
- V. Shrivastav Mansi, B. Gupta, P. Dubey, A. Deep, W. Nogala, V. Shrivastav and S. Sundriyal, Adv. Colloid Interface Sci., 2023, 318, 102967 CrossRef PubMed.
- S. Ali, S. S. Ahmad Shah, M. Sufyan Javed, T. Najam, A. Parkash, S. Khan, M. A. Bajaber, S. M. M. Eldin, R. A. Tayeb, M. M. Rahman and J. Qi, Chem. Rec., 2023, e202300145 Search PubMed.
- R. Iqbal, G. Yasin, M. Hamza, S. Ibraheem, B. Ullah, A. Saleem, S. Ali, S. Hussain, T. Anh Nguyen, Y. Slimani and R. Pathak, Coord. Chem. Rev., 2021, 447, 214152 CrossRef CAS.
- B. Pepió, N. Contreras-Pereda, S. Suárez-García, P. Hayati, S. Benmansour, P. Retailleau, A. Morsali and D. Ruiz-Molina, Ultrason. Sonochem., 2021, 72, 105425 CrossRef PubMed.
- W. Yang, M. Ni, X. Ren, Y. Tian, N. Li, Y. Su and X. Zhang, Curr. Opin. Colloid Interface Sci., 2015, 20, 416–428 CrossRef CAS.
- O. D. Salahdin, H. Sayadi, R. Solanki, R. M. R. Parra, M. Al-Thamir, A. T. Jalil, S. E. Izzat, A. T. Hammid, L. A. B. Arenas and E. Kianfar, Appl. Phys. A: Mater. Sci. Process., 2022, 128, 703 CrossRef CAS.
- Y. B. Pottathara, H. R. Tiyyagura, Z. Ahmad and K. K. Sadasivuni, J. Energy Storage, 2020, 30, 101549 CrossRef.
- C. Wang, F. Liu, J. Chen, Z. Yuan, C. Liu, X. Zhang, M. Xu, L. Wei and Y. Chen, Energy Storage Mater., 2020, 32, 448–457 CrossRef.
- K. Nasrin, M. Arunkumar, N. Koushik Kumar, V. Sudharshan, S. Rajasekar, D. Mukhilan, M. Arshad and M. Sathish, Chem. Eng. J., 2023, 474, 145505 CrossRef CAS.
- M. Zhao, N. Trainor, C. E. Ren, M. Torelli, B. Anasori and Y. Gogotsi, Adv. Mater. Technol., 2019, 4, 1800639 CrossRef.
- Y. Liang, H. Dong, D. Aurbach and Y. Yao, Nat. Energy, 2020, 5, 646–656 CrossRef CAS.
- Y. Bahari, B. Mortazavi, A. Rajabpour, X. Zhuang and T. Rabczuk, Energy Storage Mater., 2021, 35, 203–282 CrossRef.
- W. Tian, A. VahidMohammadi, M. S. Reid, Z. Wang, L. Ouyang, J. Erlandsson, T. Pettersson, L. Wågberg, M. Beidaghi and M. M. Hamedi, Adv. Mater., 2019, 31, 1902977 CrossRef PubMed.
- X. Lang, A. Hirata, T. Fujita and M. Chen, Nat. Nanotechnol., 2011, 6, 232–236 CrossRef CAS PubMed.
- M. Salanne, B. Rotenberg, K. Naoi, K. Kaneko, P.-L. Taberna, C. P. Grey, B. Dunn and P. Simon, Nat. Energy, 2016, 1, 16070 CrossRef CAS.
- Q. Zhu, J. Li, P. Simon and B. Xu, Energy Storage Mater., 2021, 35, 630–660 CrossRef.
- S. Zhao, M. Li, X. Wu, S. H. Yu, W. Zhang, J. Luo, J. Wang, Y. Geng, Q. Gou and K. Sun, Mater. Today Adv., 2020, 6, 100060 CrossRef.
- J. Yun, I. Echols, P. Flouda, Y. Chen, S. Wang, X. Zhao, D. Holta, M. Radovic, M. J. Green, M. Naraghi and J. L. Lutkenhaus, ACS Appl. Mater. Interfaces, 2021, 13, 14068–14076 CrossRef CAS PubMed.
- E. Ahn, H. Gaiji, T. Kim, M. Abderrabba, H.-W. Lee and B.-S. Kim, J. Memb. Sci., 2019, 585, 191–198 CrossRef CAS.
- S. Bashir, K. Hasan, M. Hina, R. Ali Soomro, M. A. Mujtaba, S. Ramesh, K. Ramesh, N. Duraisamy and R. Manikam, J. Electroanal. Chem., 2021, 898, 115626 CrossRef CAS.
- E. V. Lengert, S. I. Koltsov, J. Li, A. V. Ermakov, B. V. Parakhonskiy, E. V. Skorb and A. G. Skirtach, Coatings, 2020, 10, 1131 CrossRef CAS.
- D. A. Oliveira, J. L. Lutkenhaus and J. R. Siqueira, Thin Solid Films, 2021, 718, 138483 CrossRef CAS.
- F. Balqis, B. Prakoso, N. Hanif Hawari, C. Eldona and A. Sumboja, ChemNanoMat, 2022, 8, e202200151 CrossRef CAS.
- O. Faruk and B. Adak, Synth. Met., 2023, 297, 117384 CrossRef CAS.
- S. Kulandaivalu, N. Suhaimi and Y. Sulaiman, Sci. Rep., 2019, 9, 4884 CrossRef PubMed.
- X.-W. Yun, B. Tang, Z.-Y. Xiong and X.-G. Wang, Chinese J. Polym. Sci., 2020, 38, 423–434 CrossRef CAS.
- A. S. Yang, T. Y. Cai, L. Su, Y. S. Li, F. F. He, Q. P. Zhang, Y. L. Zhou, R. He, K. Zhang and W. Bin Yang, Sustainable Energy Fuels, 2022, 6, 5045–5071 RSC.
- K. Shportko, S. Kremers, M. Woda, D. Lencer, J. Robertson and M. Wuttig, Nat. Mater., 2008, 7, 653–658 CrossRef CAS PubMed.
- D. V. Voronin, R. I. Mendgaziev, M. I. Rubtsova, K. A. Cherednichenko, P. A. Demina, A. M. Abramova, D. G. Shchukin and V. Vinokurov, Mater. Chem. Front., 2022, 6, 1033–1045 RSC.
- W. Aftab, X. Huang, W. Wu, Z. Liang, A. Mahmood and R. Zou, Energy Environ. Sci., 2018, 11, 1392–1424 RSC.
- P. F. De Castro, S. Minko, V. Vinokurov, K. Cherednichenko and D. G. Shchukin, ACS Appl. Energy Mater., 2021, 4, 12789–12797 CrossRef PubMed.
- Q. Qin, W. Cao, D. W. Zhaxi, X. Chen, D. V. Andreeva, K. Chen, S. Yang, H. Tian, M. Shaker, Z. Jin and K. S. Novoselov, J. Mater. Eng. Perform., 2023, 1, 1–12 CrossRef.
- M. Wieckowski and M. Krolikowski, J. Chem. Eng. Data, 2022, 67, 727–738 CrossRef CAS.
- L. Abdolmaleki, S. M. Sadrameli and A. Pirvaram, Renew. Energy, 2020, 145, 233–241 CrossRef CAS.
- M. Więckowski, M. Królikowski, L. Scheller and M. Dzida, Phys. Chem. Chem. Phys., 2023, 25, 16979–16990 RSC.
- W. Xu, L. Xu, W. Jia, X. Mao, S. Liu, H. Dong, H. Zhang and Y. Zhang, Biomater. Sci., 2022, 10, 6388–6398 RSC.
- B. Nie, A. Palacios, B. Zou, J. Liu, T. Zhang and Y. Li, Renew. Sustainable Energy Rev., 2020, 134, 513–533 Search PubMed.
-
S. Amin, Z. Ma and M. Arici, Building Energy Flexibility and Demand Management, 2023 Search PubMed.
- Y. Feng, R. Wei, Z. Huang, X. Zhang and G. Wang, Phys. Chem. Chem. Phys., 2018, 20, 7772–7780 RSC.
- M. Zhu, O. Cojocaru-mirédin, A. M. Mio, J. Keutgen, M. Küpers, Y. Yu, J. Cho, R. Dronskowski and M. Wuttig, Adv. Mater., 2018, 30, 1706735 CrossRef PubMed.
- N. Ravikiran, B. Padya, A. Rao, R. Kali and P. K. Jain, Multifunct. Phase Chang. Mater. Fundam. Prop. Appl., 2023, 95–112 Search PubMed.
- K. Du, J. Calautit, Z. Wang, Y. Wu and H. Liu, Appl. Energy, 2018, 220, 242–273 CrossRef CAS.
- V. Kulish, N. Aslfattahi, M. Schmirler and P. Sláma, Sci. Rep., 2023, 1–12 Search PubMed.
- S. Fatahi, H. Claverie and J. Poncet, Appl. Sci., 2022, 12, 12019 CrossRef.
- K. Matuszek, M. Kar, J. M. Pringle and D. R. Macfarlane, Chem. Rev., 2023, 123, 491–514 CrossRef CAS PubMed.
- Y. Zhang, J. Tang, J. Chen, Y. Zhang, X. Chen, M. Ding, W. Zhou, X. Xu, H. Liu and G. Xue, Nat. Commun., 2023, 14, 1–10 CAS.
- H. Cho, J. Kwon, I. Ha, J. Jung, Y. Rho, H. Lee, S. Han, S. Hong, C. P. Grigoropoulos and S. H. Ko, Sci. Adv., 2019, 5, 1–9 Search PubMed.
- S. S. Mali, J. V. Patil, J. Y. Shao, Y. W. Zhong, S. R. Rondiya, N. Y. Dzade and C. K. Hong, Nat. Energy, 2023, 8, 1–13 Search PubMed.
- P. Tao, C. Chang, Z. Tong, H. Bao, C. Song, J. Wu, W. Shang and T. Deng, Energy Environ. Sci., 2019, 12, 1613–1621 RSC.
- Y. Shi, M. A. Gerkman, Q. Qiu, S. Zhang and G. G. D. Han, J. Mater. Chem. A, 2021, 9, 9798–9808 RSC.
- X. Fan, L. Liu, X. Jin, W. Wang, S. Zhang and B. Tang, J. Mater. Chem. A, 2019, 7, 14319–14327 RSC.
- B. Gupta, J. Bhalavi, S. Sharma and A. Bisen, Mater. Today Proc., 2020, 46, 5550–5554 CrossRef.
- V. Chalkia, N. Tachos, P. K. Pandis, A. Giannakas, M. K. Koukou, M. G. Vrachopoulos, L. Coelho, A. Ladavos and V. N. Stathopoulos, RSC Adv., 2018, 8, 27438–27447 RSC.
- A. S. Rosen, S. M. Iyer, D. Ray, Z. Yao, A. Aspuru-Guzik, L. Gagliardi, J. M. Notestein and R. Q. Snurr, Matter, 2021, 4, 1578–1597 CrossRef CAS.
- Z. Yao, B. Sánchez-Lengeling, N. S. Bobbitt, B. J. Bucior, S. G. H. Kumar, S. P. Collins, T. Burns, T. K. Woo, O. K. Farha, R. Q. Snurr and A. Aspuru-Guzik, Nat. Mach. Intell., 2021, 3, 76–86 CrossRef.
- G. Pilania, J. E. Gubernatis and T. Lookman, Comput. Mater. Sci., 2017, 129, 156–163 CrossRef CAS.
- C. Gao, X. Min, M. Fang, T. Tao, X. Zheng, Y. Liu, X. Wu and Z. Huang, Adv. Funct. Mater., 2022, 32, 2108044 CrossRef CAS.
- C. Liu, E. Fujita, Y. Katsura, Y. Inada, A. Ishikawa, R. Tamura, K. Kimura and R. Yoshida, Adv. Mater., 2021, 33, 2102507 CrossRef CAS PubMed.
- H. Ning, Z. Yu, Q. Zhang, H. Wen, B. Gao, Y. Mao, Y. Li, Y. Zhou, Y. Zhou, J. Chen, L. Liu, W. Wang, T. Li, Y. Li, W. Meng, W. Li, Y. Li, H. Qiu, Y. Shi, Y. Chai, H. Wu and X. Wang, Nat. Nanotechnol., 2023, 18, 493–500 CrossRef CAS PubMed.
- S. Wu, Z. Wang, H. Zhang, J. Cai and J. Li, Energy Environ. Mater., 2023, 6, e12259 CrossRef CAS.
- H. J. Kim, M. Chong, T. G. Rhee, Y. G. Khim, M.-H. Jung, Y.-M. Kim, H. Y. Jeong, B. K. Choi and Y. J. Chang, Nano Converg., 2023, 10, 10 CrossRef CAS.
- M. Seifermann, P. Reiser, P. Friederich and P. A. Levkin, Small Methods, 2023, 7, 2300553 CrossRef CAS PubMed.
- T. Xu, J. Wang, S. Zhao, D. Chen, H. Zhang, Y. Fang, N. Kong, Z. Zhou, W. Li and H. Wang, Nat. Commun., 2023, 14, 3880 CrossRef CAS PubMed.
- N. Madadian Bozorg, M. Leclercq, T. Lescot, M. Bazin, N. Gaudreault, A. Dikpati, M.-A. Fortin, A. Droit and N. Bertrand, Biomater. Adv., 2023, 153, 213533 CrossRef CAS PubMed.
- J. Zhang, Y. Liu, D. Chandra Sekhar, M. Singh, Y. Tong, E. Kucukdeger, H. Y. Yoon, A. P. Haring, M. Roman, Z. (James) Kong and B. N. Johnson, Appl. Mater. Today, 2023, 30, 101720 CrossRef.
- E. Vargo, J. C. Dahl, K. M. Evans, T. Khan, P. Alivisatos and T. Xu, Adv. Mater., 2022, 34, 2203168 CrossRef CAS PubMed.
- Z. H. Jaffari, A. Abbas, S.-M. Lam, S. Park, K. Chon, E.-S. Kim and K. H. Cho, J. Hazard. Mater., 2023, 442, 130031 CrossRef CAS PubMed.
- Z. Zhao, Y. Shen, H. Zhu, Q. Zhang, Y. Zhang, X. Yang, P. Liang and L. Chen, Appl. Surf. Sci., 2023, 640, 158400 CrossRef CAS.
- A. Esmaeili, S. Pourranjabar Hasan Kiadeh, A. Ebrahimian Pirbazari, F. Esmaeili Khalil Saraei, A. Ebrahimian Pirbazari, A. Derakhshesh and F.-S. Tabatabai-Yazdi, Chemosphere, 2023, 332, 138852 CrossRef CAS PubMed.
- J. Li, X. Liu, H. Wang, Y. Sun and F. Dong, Chinese Chem. Lett., 2023, 108596 Search PubMed.
- T. Lemaoui, A. S. Darwish, G. Almustafa, A. Boublia, P. R. Sarika, N. A. Jabbar, T. Ibrahim, P. Nancarrow, K. K. Yadav, A. M. Fallatah, M. Abbas, J. S. Algethami, Y. Benguerba, B.-H. Jeon, F. Banat and I. M. AlNashef, Energy Storage Mater., 2023, 59, 102795 CrossRef.
- H. Yang, L. Fang, Z. Yuan, X. Teng, H. Qin, Z. He, Y. Wan, X. Wu, Y. Zhang, L. Guan, C. Meng, Q. Zhou, C. Wang, P. Ding, H. Hu and M. Wu, Carbon, 2023, 201, 408–414 CrossRef CAS.
- Y. Zhang and M. Zhao, Energy Storage Mater., 2023, 57, 346–359 CrossRef.
- T. Šušteršič, V. Gribova, M. Nikolic, P. Lavalle, N. Filipovic and N. E. Vrana, ACS Omega, 2023, 8, 4677–4686 CrossRef PubMed.
- L. Pilz, C. Natzeck, J. Wohlgemuth, N. Scheuermann, P. G. Weidler, I. Wagner, C. Wöll and M. Tsotsalas, Adv. Mater. Interfaces, 2023, 10, 2201771 CrossRef CAS.
- E. E. Ondar, M. V. Polynski and V. P. Ananikov, ChemPhysChem, 2023, 24, e202200940 CrossRef CAS PubMed.
- D. A. Boiko, K. S. Kozlov, J. V. Burykina, V. V. Ilyushenkova and V. P. Ananikov, J. Am. Chem. Soc., 2022, 144, 14590–14606 CrossRef CAS PubMed.
- J. Razlivina, N. Serov, O. Shapovalova and V. Vinogradov, Small, 2022, 18, 2105673 CrossRef CAS PubMed.
- N. Shirokii, Y. Din, I. Petrov, Y. Seregin, S. Sirotenko, J. Razlivina, N. Serov and V. Vinogradov, Small, 2023, 19, 2207106 CrossRef CAS PubMed.
- P. Huang, R. Lukin, M. Faleev, N. Kazeev, A. R. Al-Maeeni, D. V. Andreeva, A. Ustyuzhanin, A. Tormasov, A. H. Castro Neto and K. S. Novoselov, npj 2D Mater. Appl., 2023, 7, 6 CrossRef.
- F. V. Lavrentev, I. S. Rumyantsev, A. S. Ivanov, V. V. Shilovskikh, O. Y. Orlova, K. G. Nikolaev, D. V. Andreeva and E. V. Skorb, ACS Appl. Mater. Interfaces, 2022, 14, 7321–7328 CrossRef CAS PubMed.
- A. S. Ivanov, K. G. Nikolaev, A. A. Stekolshchikova, W. T. Tesfatsion, S. O. Yurchenko, K. S. Novoselov, D. V. Andreeva, M. Y. Rubtsova, M. F. Vorovitch, A. A. Ishmukhametov, A. M. Egorov and E. V. Skorb, ACS Appl. Bio Mater., 2020, 3, 7352–7356 CrossRef CAS PubMed.
- Z. Wang, Y. Gu, L. Zheng, J. Hou, H. Zheng, S. Sun and L. Wang, Adv. Mater., 2022, 34, 2106776 CrossRef CAS PubMed.
- M. Zhukov, M. S. Hasan, P. Nesterov, M. Sabbouh, O. Burdulenko, E. V. Skorb and M. Nosonovsky, ACS Appl. Mater. Interfaces, 2022, 14, 2351–2359 CrossRef CAS PubMed.
- A. S. Aglikov, A. T. Aliev, M. V. Zhukov, A. A. Nikitina, E. A. Smirnov, D. A. Kozodaev, M. I. Nosonovsky and E. V. Skorb, ACS Appl. Electr. Mater., 2023 DOI:10.1021/acsaem.3c01358.
|
This journal is © The Royal Society of Chemistry 2024 |
Click here to see how this site uses Cookies. View our privacy policy here.