DOI:
10.1039/D3LC00838J
(Paper)
Lab Chip, 2024,
24, 2080-2093
Microphysiological pancreas-on-chip platform with integrated sensors to model endocrine function and metabolism†
Received
3rd October 2023
, Accepted 18th February 2024
First published on 23rd February 2024
Abstract
Pancreatic in vitro research is of major importance to advance mechanistic understanding and development of treatment options for diseases such as diabetes mellitus. We present a thermoplastic-based microphysiological system aiming to model the complex microphysiological structure and function of the endocrine pancreas with concurrent real-time read-out capabilities. The specifically tailored platform enables self-guided trapping of single islets at defined locations: β-cells are assembled to pseudo-islets and injected into the tissue chamber using hydrostatic pressure-driven flow. The pseudo-islets can further be embedded in an ECM-like hydrogel mimicking the native microenvironment of pancreatic islets in vivo. Non-invasive real-time monitoring of the oxygen levels on-chip is realized by the integration of luminescence-based optical sensors to the platform. To monitor insulin secretion kinetics in response to glucose stimulation in a time-resolved manner, an automated cycling of different glucose conditions is implemented. The model's response to glucose stimulation can be monitored via offline analysis of insulin secretion and via specific changes in oxygen consumption due to higher metabolic activity of pseudo-islets at high glucose levels. To demonstrate applicability for drug testing, the effects of antidiabetic medications are assessed and changes in dynamic insulin secretion are observed in line with the respective mechanism of action. Finally, by integrating human pancreatic islet microtissues, we highlight the flexibility of the platform and demonstrate the preservation of long-term functionality of human endocrine pancreatic tissue.
1. Introduction
Diabetes mellitus (DM) is one of the fastest growing global health concerns with more than half a billion people currently estimated to be living with the disease worldwide.1 Consequently, research on pancreatic (patho)physiology is of major importance to advance mechanistic understanding, pharmaceutical research and treatment options in the field.2,3 To promote diabetes-related research, human in vitro platforms with physiological relevance as well as integrated non-invasive readout methods are urgently needed.
Over the last decade, organ-on-chip systems have emerged as a promising new in vitro technology, capable of recreating key biological processes and disease states; they hold great prospects in the fields of drug development, disease modelling and personalized medicine.4,5 Particularly, microphysiological pancreas-on-chip platforms can provide a powerful complement to current non-clinical models.6 By combining the newest advancements in 3-D tissue culture, microfluidics and sensor technology, organ-specific 3-D microenvironments, providing key physiological aspects such as cell–cell and cell–extracellular matrix (ECM) interactions, can be created and precise fluid control and online read-out methods implemented.7–9 The latter two are especially important in the case of dynamic hormone secretion studies assessing endocrine functionality.
The endocrine part of the pancreas, regulating glucose homeostasis, comprises the Islets of Langerhans, which are 3-D cell clusters of distinct endocrine cell types.10 The most prevalent cell type, the β-cells, secrete insulin in response to changing blood glucose levels.11 β-cells are key players for both major types of DM, being targeted by an autoimmune reaction in type 1 DM and experiencing a progressive dysfunction and cell death as a result of glucolipotoxicity and insulin resistance in type 2 DM.6 Functional model systems of pancreatic endocrine tissue preferably include controlled generation and culture of β-cells assembled into 3-D islets (so-called pseudo-islets) to closer mimic organ physiology incorporating important cell–cell interactions and functions.12–15 In recent years, a variety of microfluidic platforms have been developed to enable the integration of re-assembled 3-D tissues within microchannels.16–21 Pseudo-islets are either cultured separately using microwells16,17,19 or immobilized at defined positions applying nozzle- or damn-like array structures along the perfused channel utilizing a hydrodynamic trapping principle.2,22–27 Further approaches integrate spheroids scattered indiscriminately within a channel or on top of a membrane,18,28,29 harboring limitations with respect to reproducibility and high-resolution imaging of the tissues as the islets are not precisely located on the platform. Besides integrating 3-D pseudo-islets, surrounding them with a physiological ECM or an ECM-like hydrogel is important for mimicking the native microenvironment and providing biochemical cues;30–32 cell–ECM signaling has substantial influence on the functionality of cells. So far, most pancreas-on-chip systems have been developed with the general focus on assessing intrinsic pancreatic function in the context of transplantation therapy.33 Hence, the set-up commonly includes only one perfused channel and are not amenable for the integration of an ECM-like hydrogel.
Functional read-outs in the context of pancreas-on-chip systems most commonly focus on the evaluation of glucose-stimulated insulin secretion (GSIS) and intracellular Ca2+ oscillation monitoring.33,34 Complicated manual liquid handling followed by ELISA quantification are still primarily used in the field with a few exceptions employing on-chip analysis or automation of liquid handling.19,23 Functionality analysis via build-in sensors for real-time analysis has only rarely been explored but enables in situ assessment of the tissues on-chip at high temporal resolution. A key player in physiological processes and mitochondrial activity is oxygen, which is directly linked to glucose-stimulated insulin secretion in β-cells.35–37 Recent studies demonstrated that oxygen sensors integrated in microfluidic systems can be a promising read-out tool to assess cell functionality.8,38–41
Here, we introduce a novel pancreas-on-chip platform based on a tailored thermoplastic-based microfluidic system that addresses the key requirements on an in vitro model of the endocrine pancreas. The developed platform leverages a new and robust strategy based on hydrodynamic trapping for self-guided loading of spheroids, e.g. pseudo-islets, to defined positions enabling on-chip culture in a dynamic and controlled microenvironment with precise fluid control and supply of oxygen and nutrients. The multiple-layered device with separated media perfusion on top of the tissue chamber allows for a tailored microenvironment by integration of an ECM-like hydrogel enclosing the integrated pseudo-islets. β-cell functionality can be assessed via conventional GSIS assays using automated cycling of different glucose conditions through the media channel adjacent to the tissue and additionally via in situ monitoring of oxygen consumption. To this end, the chip design allows for the direct integration of optical luminescence-based sensors,8 enabling online, non-invasive real-time assessment of the oxygen concentration directly reflecting on the metabolic activity of the tissue on-chip. As proof-of-concept, the applicability of the system for diabetes-related compound research was demonstrated by two different antidiabetic medications. In line with their mechanism of action, the two drugs changed insulin secretion dynamics of the β-cells cultured on the platform. Further, human pancreatic islet microtissues were integrated to show the possibility of integrating human-based tissues and their functionality during long-term culture on the system.
2. Materials and methods
2.1. Microfluidic chip development
2.1.1. Chip design and fabrication.
All chip designs were executed using the computer-aided design software CorelCAD (Corel Cooperation, Canada). The chips were fabricated using polymers, including polymethylmethacrylate (PMMA), polycarbonate (PC) and polydimethylsiloxane (PDMS)). The set-up of the chip comprises 250 μm thick PMMA (PLEXIGLAS® 99
524, König-Kunststoffe, Germany) sheets for media and tissue layer, which entail the specific channel and chamber geometries and are separated by a thin, porous PC membrane (ipCellCulture™ track-etched PC membranes, 1000 M25/610 M303, it4ip S.A., Belgium; 22 μm thick, 3 μm pore size, 11.3% porosity, 1.6 × 106 cm−2 pore density). The media channel and tissue chamber both feature a width of 500 μm, with a tissue channel leading to the chamber being 250 μm wide. The 250 μm thick PMMA top layer was covered with an additional flexible 3 mm thick PDMS slab that serves as an interface for tubing connections and provides access to the in- and outlets of the microfluidic channel structures. The bottom layer is 175 μm thin PMMA (PLEXIGLAS® 99
524, König-Kunststoffe, Germany) and allows for optical accessibility of the tissue, while also serving as the sensor substrate when oxygen sensors were integrated to the systems. An overview of the dimensions of all parts of the microfluidic chip, dimensioned drawings and the layer alignment can be found in Table S1 and Fig. S1.†
PMMA layers and PC membranes were structured using a CO2- or UV-laser cutter, respectively. The specific trapping structures featured in the PC membrane were generated by laser cutting 70 ± 10 μm holes. The sheets were purchased with a protective backing foil to prevent scratches and dirt from accumulating on the plastic surfaces. Protective foils on both sides of the PMMA layers were only removed after the cutting process to prevent major contamination of the layers. All chip layers were cleaned thoroughly prior to chip assembly. The cleaning process included consecutive steps of (i) quickly wiping the layers with isopropanol using standard clean room wipes and Q-tips, (ii) immediately submersing them in DI-water and (iii) blow drying them with a nitrogen pistol. PDMS slabs for connection layers were fabricated by mixing PDMS pre-polymer and curing agent (SYLGARD™ 184 Silicone Elastomer Kit, Dow Corning, USA) in a 10:1 w/w ratio. To mold the slab 42 g of the uncured mix were poured into a squared petri dish (120 mm × 120 mm) and cured overnight at 60 °C. Then, the chip geometry was pre-structured into the PDMS using a laser cutter and cut out using a surgical knife. In- and outlets were punched using a 0.75 mm biopsy punch. For experiments involving glucose-stimulated insulin secretion (GSIS) assay in- and outlet of the media channel were punched using a 0.35 mm punch. Bonding of the PDMS connection layer to the PMMA top layer was achieved by O2 plasma activation of both layers and additional surface functionalization of the PMMA layer with 1% 3-aminopropyl triethoxysilane (APTES; 440
140, Merck KGaA, Germany) solution in Ultrapure™ water (Table S2†). To enhance bonding the chips were kept at 60 °C for at least 30 min afterwards.
Cleaned PMMA layers were first aligned between two microscope glass slides and then bonded at 125 to 130 °C in a preheated convection oven (Memmert GmbH + Co. KG, Germany) by applying pressure from both sides using fold back clips. Chip assembly was achieved in two consecutive bonding steps for 15 minutes each: (i) first bonding of the PC membrane to the media layer and (ii) in the second step assembly of the full chip. For alignment and bonding of the first step, the PC membrane was placed on top of a 2 mm thick PDMS slab cut outs (fitting the microscope slide) that was placed on top of the microscope slide, serving as an alignment helper holding the fragile membrane in place as well as serving as a gasket during bonding.
For GSIS experiments, fabricated PDMS wells (h = 3 mm, Ø = 4 mm) were bonded on top of the outlet of the media channel using O2-plasma (Table S2†).
2.1.2. Contactless optical oxygen sensor integration.
A sensing layer was integrated in the bottom layer of the chip for online oxygen sensing. The bottom layer of the chip contained a recess (250 μm width, 75 μm depth) to host the oxygen sensitive layer. The space was created by additional bonding of another 75 μm thin PMMA sheet (PLEXIGLAS® 0F072, König-Kunststoffe, Germany) containing the laser cut structure of the recess. It is located at the area of the tissue chamber, 250 μm shifted to the location of the spheroids (sensor line integration performed by Stefanie Fuchs, TU Graz, Austria). The oxygen sensitive layer was prepared by slight modification of already published methods.42,43 In brief, the oxygen indicator dye platinum(II) meso-tetra(4-fluorophenyl)tetrabenzoporphyrin (Pt-TPTBPF) was dissolved in 10% PS Toluol solution. The solution was dispensed into the recess of the bottom layer using a CN controlled microdispensing device (MDS3200+, VERMES Microdispensing GmbH, Germany) equipped with a nozzle (70 μm width) and a tungsten tappet (0.7 mm width). The solution was applied as a line of 23 individual drops (spotting parameters: tapped lift: 35%, rising time 0.2 ms, opening time 0.1 ms, falling time 0.06 ms, delay 0.3 ms, number of pulses 2) with a center-to-center distance of 260 μm. The drops joined together forming a sensor line. The process was repeated three times to ensure sufficient signal strength. The sensors were allowed to dry for 5 s in between the repetitions. Afterwards, the sensors were allowed to fully dry before further integration into the system. The resulting sensor had a 1% dry weight ratio of sensitive dye to polymer.
Sensor calibration & measurements.
Chips were placed in custom-made PMMA holders in an ALS Incubator FlowBox™ (37 °C, 5% CO2, 60% humidity; ALS Automated Lab Solutions GmbH, Germany), the same set-up as the final experiments. The polished optical fibers (1 m length, core Ø 1 mm, SPFIB-BARE, PyroScience, Germany) were aligned to the center of the integrated sensor lines in the chip systems using fitted cut-outs in the custom-made chip holders. Oxygen measurements were performed using a customized 48-channel phase fluorometer (PyroScience GmbH, Germany) already described by Busche et al.43 For readouts the device was set to an illumination intensity of 100% and a detection amplification of 400×. Two-point calibration was performed in the final experimental set-up at 37 °C: For the 0% calibration point the phase shift (dphi) was determined by flushing the media channel of two designated calibration chips with the strong reductant sodium sulfite (Na2SO3; 100 g L−1) to create anoxic conditions (Fig. S3†). The mean dphi value after equilibration for 60 min of the calibration chips was used for all following experiments. For air-saturated conditions an individual calibration of each sensor was performed prior to tissue loading to the system. Chips were perfused with air-saturated PBS until equilibration and a stable dphi value was reached for each sensor.
Oxygen concentrations were measured during standard on-chip culture conditions and GSIS experiments. To ascribe the changes in oxygen concentration to the consumption of cells cultured on the chip, a blank chip with no cells was cultured with every experiment enabling to exclude changes caused by other factors. To calculate the oxygen consumption of the tissue cultured on-chip from the measured oxygen concentrations, values were subtracted from the blank chip serving as the baseline during each corresponding experiment.
2.1.3. Numerical modeling.
COMSOL Multiphysics 5.5 (COMSOL, Sweden) was used to model fluid flow, transport of diluted species and oxygen concentrations on-chip. A 3-D time-dependent model was constructed according to the chip design. Pseudo-islets were modeled as spheres with a diameter of 150 μm and one spheroid was positioned on each trap on the membrane. Physics modules “free and porous media flow” and “transport of diluted species” were coupled for the model. The “free and porous media flow” interface was selected to described free fluid flow in the microfluidic channels using the Navier–Stokes equations and the flow through the porous membrane (porosity: 11.3%) using Brinkman equations. The hydraulic permeability (K) of the membrane was calculated to K = 5.5 × 10−14 m2.44 For standard culture conditions on-chip incompressible free fluid flow was modelled in the media channel at a flow rate of Q = 5.56 × 10−12 m3 s−1 (20 μL h−1), as well as no slip boundaries at the walls of the modelled microfluidic channels. Dynamic viscosity (μ) and density (ρ) of the culture media were set to μ = 0.848 × 10−3 Pa s and ρ = 1002 kg m−3.45 The temperature was set to 310.15 K.
To model changes in the oxygen concentration on-chip, a time-dependent study was performed, modelling transport of diluted species in the system. Diffusion coefficients of oxygen in culture solutions, the collagen I hydrogel, and in the tissue were set to Doxy,media = 3.0 × 10−9 m2 s−1, Doxy,hydrogel = 2.5 × 10−9 m2 s−1 and Doxy,tissue = 2.0 × 10−9 m2 s−1, respectively.46,47 Oxygen consumption rates of the cells were set to calculated values derived from oxygen measurements performed with different glucose concentrations on-chip. The initial oxygen concentration in the system and the inflow of oxygen with the perfused media were presumed to be saturated and set to coxy,sat = 0.2 mM.
2.2. Cell culture
2.2.1. INS-1E cell culture.
The rat insulinoma-derived pancreatic β-cell line INS-1E was kindly provided by Prof. Maechler from the University of Geneva, Switzerland. Cells were cultured in T25 cell culture flasks (seeding density 40.000 cells per cm2) under standard conditions (37 °C, 5% CO2, 20% O2, 95% humidity) and passaged at a confluency of 70–80% using 0.05% trypsin/EDTA solution (Gibco). Culture media RPMI 1640 (21875-034, Gibco) was supplemented with 10 mM Hepes buffer solution (15630-056, Gibco), 1 mM sodium pyruvate (11360-070, Gibco), 50 μM β-mercaptoethanol (31350-010, Gibco), 5% FCS (SH30066.03, HyClone Fetal Clone II, GE Life Science), and 1% penicillin–streptomycin (stock: 10.000 U mL−1–10 mg mL−1; 15070063, Gibco).
2.2.2. Pseudo-islet generation.
Pseudo-islets were formed using 96 well ultra-low attachment (ULA) round bottom plates (650
970, Greiner Bio-One) at a concentration of 500 cells per well in 100 μL media per well as previously described.48 Pseudo-islets were formed over 72 hours under standard cell culture conditions and then injected to the chip system.
2.2.3. 3D InSight™ islet microtissues culture.
Commercially available human pancreatic islet microtissues (MT-04-002-01-60) from InSphero (Basel, Switzerland) were used as a human pancreatic islet model. After arrival, the islet microtissues were handled according to manufacturer's instructions. Donor was an African American male, 48 years, BMI of 23.7 and HbA1c of 5.5%. Islet microtissues were cultured in 3D InSight™ Human Islet Maintenance Medium (CS-07-005-02, InSphero) until loading to the chip systems and media was changed every 2–3 days according to manufacturer's instructions.
2.3. Tissue and hydrogel integration to the chip system and on-chip culture
Prior to experiments, chips were oxygen plasma-treated for hydrophilization for 5 min. Subsequently, the chips were flushed with 70% ethanol and then washed with PBS- 3 times, leaving PBS filled pipet tips in the tissue chamber inlet and the in- and outlet of the media channel. Chips were placed in an incubator (37 °C, 5% CO2, 95% humidity) to equilibrate.
For loading of the chips, pipet tips in the in- and outlet of the media channel were removed. INS-1E pseudo-islets or pancreatic islet microtissues were grouped in one well of the ULA plate and then injected to the inlet pipet tip of the tissue chamber. Once every trap was occupied, the hydrogel (FibriCol®, 5133, Advanced Biomatrix) at a concentration of 3 mg mL−1 was loaded to the microfluidic chip through the pipet tip located in the inlet of the tissue channel. Chips were then placed under standard cell culture conditions to allow the hydrogel to crosslink.
After 60 min of incubation, the pipet tip in the tissue inlet was removed and the tissue chamber closed off with PCR foil. The media channel of the chip was then connected via Tygon tubing (VERNAAAD04103, VWR International GmbH, Germany) to a 12-channel syringe pump (Landgraf Laborsysteme HLL GmbH, Germany) set-up and perfused with 20 μL h−1 applying positive pressure. Chips were cultured in an incubator at 37 °C, 5% CO2 atmosphere and 95% humidity (Binder, Germany).
In experiments to investigate homogenous loading of the hydrogel to the tissue chamber FluoSpheres™ Carboxylate-Modified Microspheres (F8811, Invitrogen™) were added to the hydrogel prior to injection to the chip system. The chip was immediately placed under a Zeiss Observer 7 microscope (Carl Zeiss AG) to monitor the hydrogel filling the tissue chamber.
2.4. Tissue characterization on-chip
2.4.1. Cell viability staining.
Live-/dead staining was performed on-chip using fluorescein diacetate (FDA; F7378, Sigma-Aldrich) at 27 μg mL−1 visualizing living cells and propidium iodide (PI; P4170, Sigma-Aldrich) at 135 μg mL−1 marking dead cells. To assess viability, chips were disconnected from the perfusion set-up and washed with PBS+ (D8662, Sigma-Aldrich) by gravity flow. Subsequently, a staining solution with FDA, PI was prepared in PBS− (14190-094, Gibco) and injected to the media channel of the chip system by gravity flow and incubated for 15 min. Chips were washed with PBS− 3 times, and immediately imaged on the confocal microscope Axio Z.1 Cell Observer Spinning Disk (Carl Zeiss AG).
2.4.2. Immunofluorescence staining.
To investigate tissue integrity, structure, and function on-chip, staining to visualize e-cadherin, insulin and DAPI was applied. All washing and staining solutions were flushed through the media channel by gravity flow. Before fixation, chips were disconnected from the pump set-up and flushed with PBS+. Fixation was performed by a 20 min incubation step with 4% Roti® Histofix (P087.6, Carl Roth GmbH + Co. KG). After washing with PBS− 3 times chips were stored in PBS− at 4 °C until further processing. Blocking of unspecific binding and permeabilization was performed using 3% normal donkey serum (017-000-121, Jackson Immuno Research) and 0.1% Triton-X100 (T8787, Sigma-Aldrich) in PBS− for 1 h. Primary antibodies anti-insulin (1
:
100, ab181547, abcam) and anti-e-cadherin (1
:
50, BD610181, BD Bioscience) were diluted in antibody diluent (PBS− with final concentrations of 0.01% Trition-X100 and 0.3% normal donkey serum) and incubated for 2 h at RT and overnight at 4 °C. For chips integrating human pancreatic microtissues, primary antibody anti-glucagon (1
:
100, G2654, Sigma-Aldrich) was used instead of anti-e-cadherin. Chips were washed by flushing the media channel 3 times with washing buffer (PBS− with 0.01% Triton-X100 and 0.3% normal donkey serum) and then incubated with secondary and conjugated antibodies as well as DAPI (1
:
1000, MBD0015, Merck KGaA). Secondary antibodies Alexa Fluor 647 donkey anti-rabbit (1
:
100, A31573, Thermo Fisher) and Alexa Fluor 488 donkey anti-mouse (1
:
100, A21202, Thermo Fisher) and DAPI were diluted in antibody diluent and incubated for 2 h at RT and then thoroughly washed 3 times using washing buffer. Chips were stored in PBS− at 4 °C until imaging using the confocal microscope Axio Z.1 Cell Observer Spinning Disk (Carl Zeiss AG) or LSM 880 (Carl Zeiss AG).
2.4.3. Glucose-stimulated insulin secretion assay (GSIS) on-chip.
Chips were loaded and cultured overnight (20 μL h−1) as described above. To allow to switch between different glucose conditions the chips were connected to automatic 4-port microfluidic valves (AV202 uProcess™ Automated Valve, LabSmith). Prior to perfusion with low (3.3 mM in KREBS buffer) and high (16.7 mM in KREBS buffer) glucose (A24940, Gibco) conditions, a synchronization step was performed, perfusing the chips with 1x KREBS buffer containing 25 mM Hepes (15630-056, Gibco), 0.1% BSA (A9647, Sigma-Aldrich) and 0 mM glucose for 1 h. In case of chips integrating human pancreatic microtissues, low and high glucose solutions of 2.8 mM and 16.7 mM, respectively, were prepared in 3D InSight™ Krebs Ringer HEPES Buffer (CS-07-051-01, InSphero) according to manufacturer's instructions. Insulin secretion does not take place simultaneously and at the same rate in all beta cells, and glucose stimulation promotes the recruitment of actively secreting beta cells first.49 For this reason, in in vitro GSIS studies are preceded from a low glucose period, named synchronization before starting the quantification of basal insulin levels.
The chips were subsequently perfused with low, high and again low glucose conditions for 1 h each. Effluents were sampled from the well on top of the outlet of the media channel every 60 min and stored at −20 °C until analysis. Sampling time points were calculated based on volume of the chip, tubing and valves. Insulin secretion was quantified using rat insulin ELISA kits (10-1250-01, Mercodia) or human ultrasensitive insulin ELISA kits (80-INSHUU-E10, Alpco) following manufacturer's instructions. Glucagon was measured in the same effluents using the Lumit™ Glucagon immunoassay (W8020, Promega).
2.4.4. Drug testing.
To analyze the effect of antidiabetic drugs, exendin-4 (E7144, Sigma-Aldrich) and tolbutamide (46968, Sigma-Aldrich) were tested on-chip. Stock solutions were prepared in DMSO (D8418, Sigma-Aldrich) at concentrations of 100 μM (exendin-4) and 100 mM (tolbutamide). Drugs were diluted 1
:
1000 for use concentrations of 100 nM and 100 μM, respectively, and added to low (3.3 mM) & high glucose (16.7 mM) conditions to the respective chips. Control chips received low and high glucose solutions and 0.1% DMSO without the compounds.
2.5. Statistical analysis & image processing
For image processing Zen software (3.3 blue edition, Carl Zeiss Microscopy GmbH) and open source software Fiji (ImageJ version 1.53t) were used for standard deviation projections, 3D rendering, orthogonal views of z-stacks, and to insert scale bars. Descriptive graphs & statistics were generated using GraphPad Prism 9.3.1. GSIS data is presented as box plots showing all data points. Each data point represents one chip. Statistical significance was tested as indicated for each case individually. All oxygen measurement data is presented as mean ± standard deviation (SD).
3. Results & discussion
3.1. Microfluidic chip concept and design
To engineer a microphysiological platform modelling endocrine pancreas physiology several key features of the in vivo microenvironment were considered (Fig. 1A). In the pancreas, the insulin secreting β-cells are part of 3-D cell clusters, the Islets of Langerhans, which are embedded in a unique microenvironment and highly vascularized.16,50 Taking this into account the microfluidic platform facilitates the loading and culture of 3-D tissues (e.g. pseudo-islets) mimicking primary islet morphology more closely and supporting cell–cell interactions, which are especially important for proper β-cell function.13,51,52
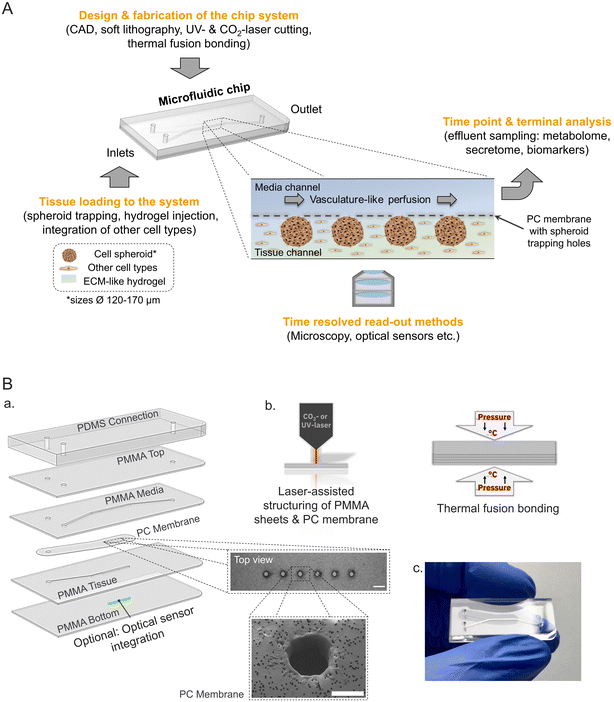 |
| Fig. 1 Pancreas-on-chip concept and design. (A) Schematic of underlying concept of the pancreas-chip including generation steps and read-out possibilities. (B) Microfluidic chip set-up and fabrication: (a) exploded view of the multilayered system with PMMA layers featuring channel structures and sandwiching a PC membrane with laser-cut trapping holes (scale bar: 200 μm) between media and tissue channel of the system. PDMS connection layer enabling tubing connections for perfusion set-up. Optional integration of an optical oxygen sensor to the bottom channel. (b) PMMA sheets and PC membrane with trapping structures are laser-structured and bonded via thermal fusion. (c) Photo of final assembled chip. | |
The developed chip is a tailored multiple-layered hybrid device featuring two microfluidic channels separated by a semi-permeable membrane. Geometries of the microstructures and channels were designed to trap and house 3-D islet models with an average diameter of 150 μm, which is representative of one islet equivalent (IEQ) for the average native pancreatic islet diameter.53 The chip set-up with separate media and tissue channels allows for the additional integration of an ECM-like hydrogel in the tissue chamber mimicking the physiological microenvironment with the option to integrate further tissue relevant cell types (e.g. endothelial cells) in direct proximity to the entrapped pseudo-islets (Fig. 1A). Media supply is implemented through dynamic micro-sale fluid flow at a flow rate of 20 μL h−1 in the overlaying channel using an external syringe pump. The controlled fluid flow not only allows for stable nutrient supply and waste removal mimicking vasculature-like perfusion, but also enables dynamic sampling of the effluent to examine secretion kinetics in a time-resolved manner; e.g. insulin secretion in response to glucose stimulation, a key function of β-cells.
The multiple-layered platform is fabricated by laser-assisted structuring and thermal fusion bonding of thin sheets of the materials poly(methyl methacrylate) (PMMA) and polycarbonate (PC) (Fig. 1B, a and b). These thermoplastic materials provide the advantage of exhibiting far lower absorption of hydrophobic molecules compared to PDMS54,55 and enabling a controlled oxygen environment inside the microfluidic system due to minimal oxygen permeability.56
Loading of six pseudo-islets per chip is realized by trapping structures which are laser cut in the PC membrane that is separating media and tissue channel (Fig. 1B, a). The pseudo-islets are immobilized at defined positions in the tissue channel located on the bottom, which is sealed with a 175 μm thin bottom layer allowing for high-resolution microscopy and monitoring of the tissue during on-chip culture. In addition, an optical sensor for online, non-invasive real-time monitoring of oxygen can be integrated. The sensor material is applied as a line onto the bottom layer below the tissues and thereby enables continuous measurements of the oxygen concentration and consumption in close proximity to the tissue allowing to draw conclusions on their metabolic activity.
3.2. On-chip tissue generation: Guided immobilization of pseudo-islets
Pseudo-islets of the β-cell line INS-1E formed by spontaneous aggregation (500 cells per well, 72 hours) in U-bottom ultra-low attachment 96-wellplates (Fig. 2A) displayed an average diameter of 152.2 ± 5.8 μm (n = 32 pseudo-islets, Fig. S2†). Diffusion of oxygen and nutrients are limited in large cell aggregates, which lead to the formation of an hypoxic core. Zbinden et al. investigated physiological function and viability of INS-1E pseudo-islets with different sizes and showed that pseudo-islets with a cell seeding number of 500 INS-1E cells per well had a high reproducibility and were highly viable and glucose-responsive.48 The resulting diameter also matches one IEQ and was therefore chosen as a representative islet model.
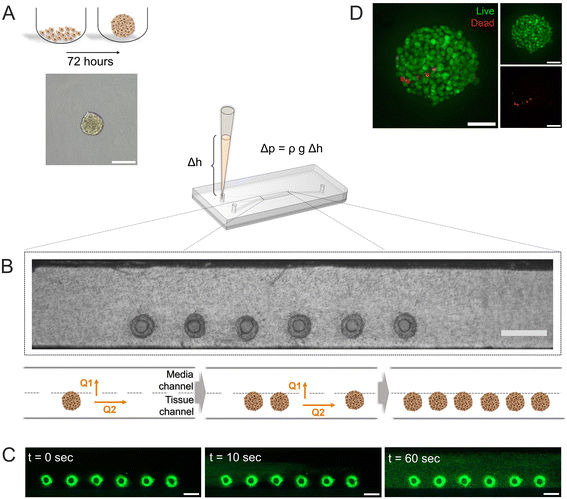 |
| Fig. 2 On-chip tissue generation. (A) Pseudo-islets were formed in 96 ultra-low attachment wellplates over 72 hours by seeding 500 cells per well in 100 μL per well (scale bar 150 μm). (B) Six pseudo-islets were grouped and injected into a pipet tip in the inlet of the tissue channel. Loading to the tissue channel by hydrostatic-pressure driven flow where the pressure difference (Δp) results from the height difference (Δh) between inlet and outlets considering the density (ρ) of the liquid and the acceleration due to gravity (g). Guided immobilization of islet models on-chip if Q1/Q2 > 1 (Q1: flow through trapping holes, Q2: flow along channel) the islets are guided to the trapping structures (scale bar 500 μm). (C) Subsequent filling of the tissue chamber with FibriCol® hydrogel injected again using hydrostatic pressure-driven flow. Invitrogen FluoSpheres™ were added to the hydrogel for visualization (scale bar 200 μm). (D) Viability staining (standard deviation projection) of pseudo-islet on-chip revealed mostly viable cells after loading ( , scale bar 50 μm). | |
Successfully formed pseudo-islets were loaded into the tissue chamber of the pancreas-on-chip utilizing hydrostatic pressure-driven flow employing protocols specifically tailored for ease-of-use and robustness: Six pseudo-islets were grouped and subsequently injected into a pipet tip in the inlet of the tissue channel. The resulting height difference of the liquid column in the inlet pipet tip with respect to the outlets of the media channel led to a hydrostatic pressure-driven flow achieving self-guided trapping of the pseudo-islets at the trapping sites on the membrane (Fig. 2B, Video S1†). The fluidic resistance of the membrane pores exceeds the one of the laser-cut structures, leading to a higher flow through the trapping sites dragging the pseudo-islets to these positions. Once sitting on the hole, the pseudo-islet blocks the flow, increasing the resistance, causing the next pseudo-islets to move to an empty trap. Once all trapping sites were loaded excess fluid can exit through the membrane pores to the media channel.
Right after pseudo-islet loading, the hydrogel was injected into the tissue chamber again using hydrostatic-pressure driven flow. Once crosslinked, the hydrogel preserves structural stability of the pseudo-islets, while at the same time mimicking the microenvironment of the cells on-chip. Collagen I has been shown to contribute to β-cell structure, survival and insulin expression in previous studies and was therefore chosen to model an in vivo microenvironment.31,48,57,58 To investigate the homogenous loading of the collagen I hydrogel to the tissue chamber, fluorescent microspheres were embedded in the gel prior to chip injection. The hydrogel filled the entire tissue channel within one minute in a homogeneous manner (Fig. 2C, Video S2†).
3.3. Characterization of viability, structure & function of the tissue on-chip
On-chip live/dead staining revealed predominantely viable pseudo-islets with few scattered dead cells demonstrating minimal impact of the loading process and on-chip culture on tissue viability (Fig. 2D). The immobilization of individual pseudo-islets at defined positions enables in situ immunofluorescence staining and high-resolution imaging. To assess pseudo-islet integrity and functionality on-chip, e-cadherin and insulin expression were analyzed: laser scanning confocal microscopy (LSM) confirmed preservation of pseudo-islet structure and expression of e-cadherin in all six pseudo-islets on-chip (Fig. 3A). As a key cell–cell contact protein, e-cadherin facilitates intra-islet communication promoting insulin secretion capacity.13,50,59,60 Staining for insulin itself underlined the pseudo-islet's intrinsic insulin production during on-chip culture (Fig. 3A).
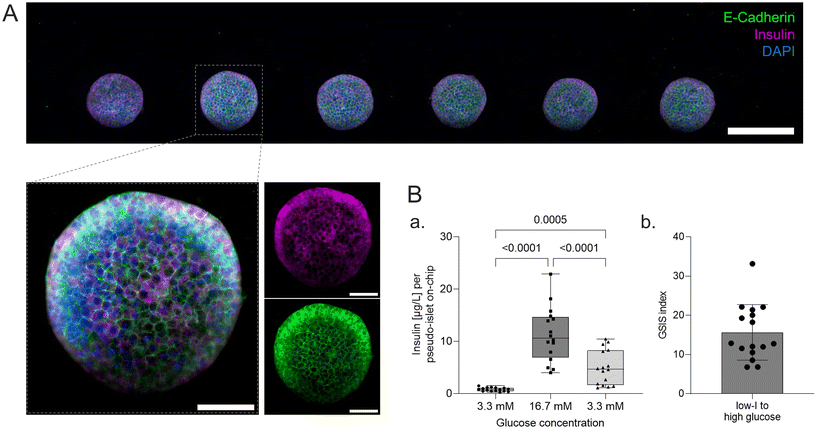 |
| Fig. 3 Structural & functional characterization on-chip. (A) LSM-imaging of a pancreas-on-chip system stained for e-cadherin, insulin and DAPI displaying the six pseudo-islets in the tissue channel (tile scan; standard deviation projection of z-stack). Scale bar 200 μm (tile scan) and 50 μm (zoom-in on single pseudo-islet). (B) (a) Glucose-stimulated insulin secretion of pseudo-islets on-chip (b) and corresponding GSIS index of high glucose normalized to first low glucose phase at 3.3 mM (one-way ANOVA, n = 16 chips). | |
Endocrine functionality was further investigated via dynamic on-chip GSIS assays: culture of the pseudo-islets under dynamic perfusion enables a controlled delivery of dissolved molecules to the tissue and analysis of secreted factors, e.g. hormones. Pancreas-on-chip systems were sequentially perfused with low (3.3 mM), high (16.7 mM) and again low glucose (3.3 mM) levels for 60 min each. The perfused effluent was collected from the outlet at specific timepoints considering dead volumes. The pseudo-islets on-chip demonstrated glucose responsiveness with a GSIS index of 15.7 ± 7.1 (n = 16 chips) featuring basal insulin levels of 0.8 ± 0.4 μg L−1 per pseudo-islet and hour during the first low glucose and an insulin release of 11.3 ± 5.2 μg L−1 per pseudo-islet and hour during the subsequent high glucose phase. Exposing the chips again to low glucose levels, insulin levels per pseudo-islet in an hour declined to 5.0 ± 3.3 μg L−1. These results confirmed cell functionality after loading and subsequent on-chip culture. Higher levels during the second low glucose phase were hypothesized to be attributed to insulin that was released to the hydrogel during the high glucose phase and was still diffusing to the media channel during the second low glucose phase as well as prolonged insulin secretion of the pseudo-islets after switching back to low glucose and therefore a delayed decrease.
3.4. Assessing impact of compound treatment on insulin secretion
To demonstrate the applicability of the platform for drug testing, pseudo-islets cultured on the pancreas-on-chip systems were exposed to two different antidiabetic drugs: exendin-4, a glucagon-like peptide-1 (GLP-1) receptor agonist, and tolbutamide, a sulfonylurea, which are both classified as insulin secretagogue agents.61 Tolbutamide (n = 7 chips) and exendin-4 (n = 9 chips) were added during GSIS experiments at concentrations of 100 μM and 100 nM, respectively (Fig. 4A). Exendin-4 increased insulin secretion at high glucose levels, while it had little effect during the low glucose phase (Fig. 4B). In comparison, tolbutamide potentiated insulin secretion already during the first low glucose phase, raising insulin levels more than six times compared to control chips, while showing no significant difference in insulin release during high glucose phases (Fig. 4B). These findings are in line with expected drug responses. Sulfonylureas drugs, such as tolbutamide, are glucose-independent and lead to direct closure of ATP-sensitive K+ channels, skipping the usually necessary metabolic conversion of glucose to ATP to block these channels.62 Consequently, insulin is released independently of the present glucose concentration. The less pronounced insulin release during high glucose levels during the dynamic GSIS on-chip with tolbutamide treatment was also observed by Misun et al.19 and can be ascribed to the potentiated insulin release during the pre-stimulation phase supported by the hypothesis of the exhaustion of the readily releasable pool of insulin granules. Exendin-4 is an incretin mimetic drug and its effects are glucose-dependent.63 The compound potentiates already stimulated insulin secretion by influencing several key signaling mechanisms.62 This explains the increase in insulin secretion compared to non-treated chips during the perfusion with high glucose solution and little effect during low glucose phases. In line with their mechanism of action the two diabetic medications changed insulin secretion dynamics of the cells cultured on-chip and demonstrate the potential of the platform to be used for drug screening applications.
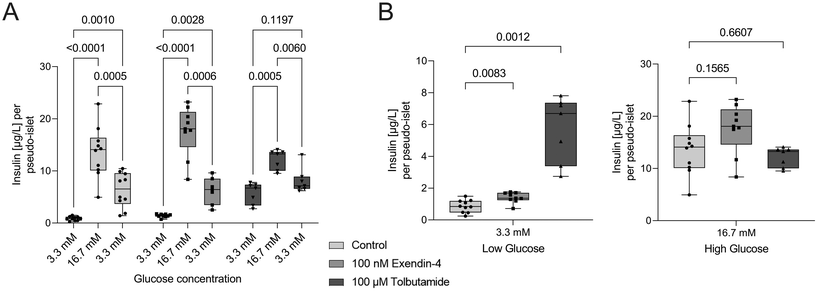 |
| Fig. 4 Proof-of-concept in vitro application: drug applicability. (A) Pseudo-islets on-chip were exposed to exendin-4 (100 nM) and tolbutamide (100 μM) during dynamic GSIS experiments (low-high-low glucose intervals for 60 min each) on-chip. (B) Both drugs changed insulin secretion dynamics. In line with their mechanism of action the treatment with exendin-4 resulted in a glucose-dependent increase, while the effect of tolbutamide is glucose-independent and already increased insulin secretion during the low glucose phase (two-way ANOVA, n = 10 chips for control, n = 9 chips for exendin-4, n = 7 chips for tolbutamide). | |
3.5. Oxygen sensor integration for monitoring of metabolic activity
3.5.1. Sensor integration for continuous monitoring of O2 concentrations.
Luminescence-based oxygen sensors were integrated into the pancreas-on-chip modules by depositing the sensor material onto the bottom layer prior to chip assembly. The sensor line was aligned with the tissue chamber flanking the line of trapping structures in the membrane to ensure measurements in close proximity to the tissue while maintaining optical accessibility to the tissue. The set-up enables non-invasive real-time monitoring of the O2 concentrations on-chip and thereby detection of changes in O2 consumption of the tissue. Fabricating the tissue compartments of the pancreas-on-chip modules from the thermoplastic material PMMA instead of the commonly used PDMS minimizes direct diffusion of oxygen from the outside of the chip. This enables controlled oxygen supply from the dissolved oxygen in the perfused media, diffusing over the membrane to the tissue. Thereby distortion of the sensor measurements due to O2 diffusion through the chip material was minimized and enabled read-out of the oxygen consumption of the tissue on-chip.
3.5.2. Oxygen consumption and metabolic activity on-chip.
Oxygen levels during homeostatic on-chip culture were at 97 μM ± 17 μM (Fig. 5A) for standard culture conditions (n = 32 chips) with a flow rate of 20 μL h−1 and glucose concentration of 11.1 mM. Lower glucose levels of 3.3 mM (n = 6 chips) in the perfused media led to a mean oxygen concentration of 143 μM ± 9 μM revealing lower O2 consumption of the tissue in response to lower glucose levels. The oxygen consumption rate (OCR) was determined to be about twice as high for the glucose levels of standard culture media compared to the low glucose condition, with values of 0.037 ± 0.008 mol m−3 s−1 and 0.017 ± 0.002 mol m−3 s−1, respectively (Fig. 5B). Values were calculated considering a standard islet equivalent IEQ volume of 1.77 × 10−12 m3.64 Modelling the oxygen level dynamics via finite element method (FEM) simulations based on these OCRs showed similar kinetics between the developed in silico model and experimental results (Fig. 5A and C).
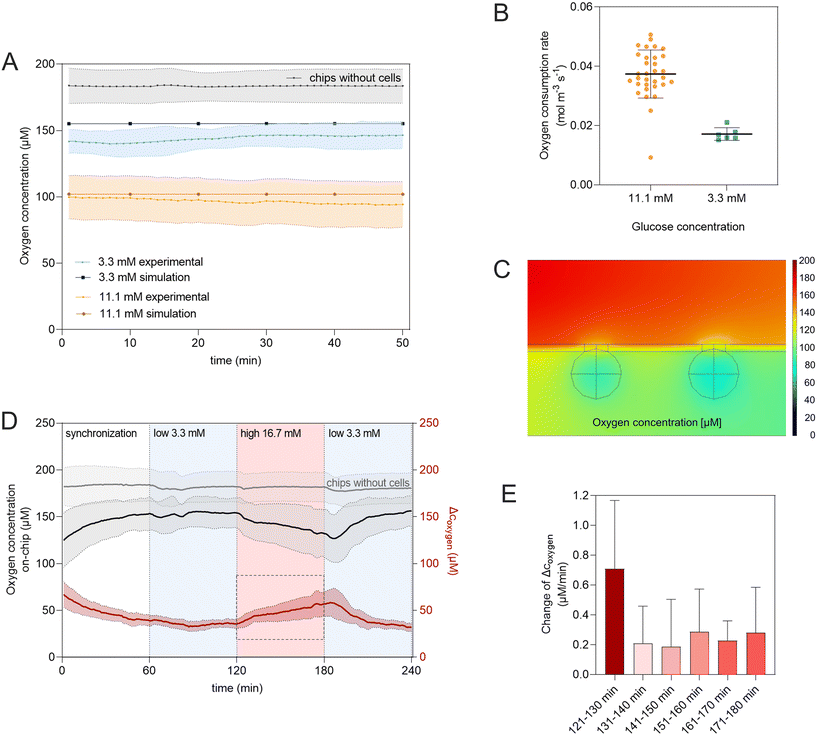 |
| Fig. 5 Monitoring metabolic activity on chip via integrated oxygen sensors. (A) Oxygen concentration in the tissue chamber from experimental measurements and in silico FEM simulations (point evaluation at sensor location) for standard media conditions (11.1 mM, orange) as well as low glucose levels (3.3 mM, blue); grey line indicates average oxygen concentration measured on perfused chips with hydrogel, but without cells – blank chips; (B) average oxygen consumption rate during on-chip culture with different glucose conditions (11.1 mM (n = 32 chips) and 3.3 mM (n = 6 chips)). (C) Cross-sectional view of two spheroids in FEM simulation modelling standard media perfusion (20 μL h−1, 11.1 mM glucose). (D) Oxygen kinetics during glucose-stimulated insulin secretion (GSIS) assay: mean oxygen concentration of chips (n = 6 tissue-chips: black line; n = 4 hydrogel only control chips: Grey line) perfused sequentially with low (3.3 mM, light blue background), high (16.7 mM, light red background) and low glucose media for 60 min each. Kinetics of consumed oxygen (red line) is calculated by subtracting the measured oxygen concentration (black line) at each time point of each chip from corresponding hydrogel blank (grey line). (E) Slopes of Δcoxygen averaged over 10 min intervals during high glucose perfusion phase. | |
Besides determining baseline levels during homeostatic culture, the integrated sensors also allow in situ monitoring of dynamic changes in oxygen consumption. Adjusting the glucose concentration in the perfused media stepwise from no glucose to low (3.3 mM), high (16.7 mM), and again low (3.3 mM) glucose levels, analogous to the GSIS-assays, resulted in almost immediate changes in O2 consumption (Fig. 5D); demonstrating that the integrated sensor approach provides a non-invasive live read-out of the metabolic state of the islets with high temporal resolution. The latter further allowed to analyze the kinetics of the response of the tissue to increased glucose levels by looking at 10 min intervals individually (Fig. 5E). In the first 10 min of the high glucose phase, the slope, directly relating to the change in OCR, is almost three-times higher (0.7 μM min−1) compared to the slope during the subsequent intervals (0.2–0.3 μM min−1). This can be associated with a biphasic oxygen consumption response to high glucose stimulation also described by Regeenes et al.22
Beta cells are highly metabolically active with a high demand of oxygen, especially to secrete insulin in response to high glucose levels.65 The oxygen consumption directly reflects on the mitochondrial activity of the cell and can be used as a useful parameter to assess functionality.22,66 Our proof-of-concept experiments showed that the integrated sensors enabled detailed analysis of oxygen consumption and therefore metabolic function in a non-invasive manner and with temporal resolution. This allows to study mitochondrial respiration in response to glucose stimulation especially valuable for studying impaired mitochondrial energy metabolism in T2DM.39,67
3.6. Human pancreatic islet microtissues maintain functionality over long-term on-chip
To demonstrate the flexibility of the platform, we integrated human pancreatic islet microtissues (MTs; 3D InSight™, InSphero). SOPs established for the pseudo-islets could be directly transferred to load six individual MTs (Fig. 6A) and culture them on-chip for two weeks. In contrast to the pseudo-islets, MTs feature both two most abundant endocrine cell types of pancreatic islets, β- and α-cells, as highlighted by on-chip immunofluorescence staining and confocal microscopy (Fig. 6B). The platform, moreover, maintains MT functionality over long-term culture as confirmed by GSIS assays performed on day 1, day 7 and day 14 of on-chip culture. Over the two week culture period, no decline in functionality was observed; on the contrary, over time insulin secretion actually increased both at basal levels (2.8 mM glucose) and during high-glucose (16.7 mM) conditions (Fig. 6C). The GSIS index thereby remained stable throughout the culture period displaying a 5.5 to 5.7-fold increase (Fig. 6D). Exposure to high glucose reduced glucagon level without further increase in low II condition (Fig. 6E).
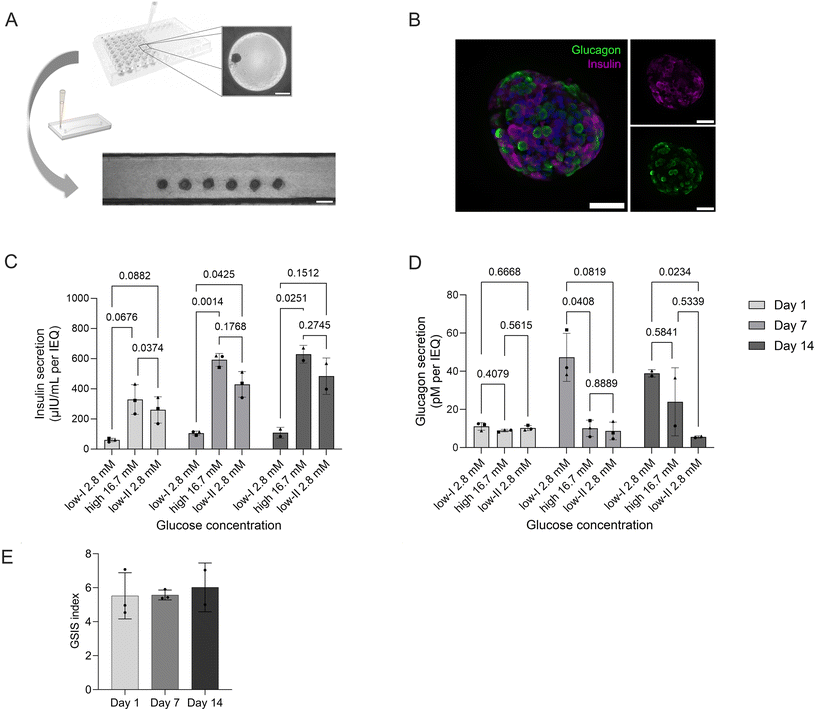 |
| Fig. 6 Integration and functionality assessment of human pancreatic islet microtissues on-chip. (A) Schematic depiction of the loading of pancreatic microtissues into the chip. Scale bars: 200 μm. (B) Expression of insulin and glucagon of an MT imaged on-chip (confocal imaging, standard deviation projection of z-stack). Scale bar 50 μm. (C) Consecutive GSIS assays throughout two weeks of on-chip culture demonstrating that human pancreatic MTs remained responsive for multiple stimulations over the 14 day culture period (two-way ANOVA, n = 3 chips for day 1 and 7 and n = 2 chips for day 14). (E) Corresponding GSIS index (with respect to first low glucose phase) on day 1, 7 and 14. (D) Glucagon secretion during consecutive GSIS assay. Glucagon secretion is glucose and insulin dependent at day 7 and 14 (two-way ANOVA, n = 3 chips for day 1 and 7 and n = 2 chips for day 14). For Fig. C and D, the same symbol reflects data collected from the same chip over the cell culture time. The same effluent samples were measured for insulin and glucagon. | |
These findings demonstrate that the developed platform provides a high level of flexibility and control for integrating and culturing human islet microtissues. The inclusion of the human model containing β-, α- and γ-cells also paves the way for mechanistic studies on the regulation of glucagon secretion by insulin and the role of not only insulin resistance but glucagon resistance in diabetes type II and obesity.68 The data on MT shows a healthy phenotype where insulin production is responding to glucose stimulation in a controlled fashion and glucagon secretion is not solely stimulated by a decrease in glucose secretion, but glucose and insulin dependent. In low II glucose condition, α-cells were recently sensing the insulin peak from high glucose (Fig. 6C) and were cultured in the absence of additional modulators of glucagon production such as fatty acids and aminoacids, explaining the low glucagon levels relative to low I condition.69
The defined and robust positioning enables reproducible culture conditions allowing comparison of results across chip systems. The MTs are integrated in an ECM-like hydrogel that can be modified in the future to study the contributions of specific ECM proteins and biomechanical properties as well as of other cell types to the (patho)physiology of pancreatic tissue.70–72 Next steps could also focus on increasing temporal resolution of effluent sampling to monitor the biphasic and oscillatory insulin secretion and correlate them to oxygen consumption, enabling mechanistic studies on the dynamics of insulin secretion and alterations in the secretory profile caused by diseases such as DM.62,73,74
4. Conclusion
We introduce a novel microphysiological system with integrated sensors for facile generation of endocrine pancreatic tissue and non-invasive in situ monitoring of insulin secretion and metabolism. The endocrine pancreas-on-chip platform enables robust self-guided immobilization and culture of pseudo-islets in a physiological microenvironment. To assess tissue function, the system features automated GSIS assays and in situ O2 sensing capabilities allowing to monitor insulin secretion kinetics and changes in metabolic activity on-chip with high temporal resolution. β-cell pseudo-islets cultured on the platform maintained viability and integrity as well as a high GSIS response. Integration of pancreatic islet MTs showed easy transferability of the system to another more relevant human model of pancreatic endocrine tissues and their functionality in long-term culture on the platform. By surrounding the islets with an ECM-like hydrogel amenable for integration of further tissue components (e.g., stromal, or endothelial cells) further increases physiological relevance of the tissue structure and microenvironment. In the future, the model may be advanced by addition of missing components such as the microvasculature, exocrine cells, and immune system. The overall geometries of the platform and immobilization method can also be adjusted to spheroids of different sizes and number making the underlying concept transferable to other tissue-chips using aggregates, spheroids, or organoids as building blocks. All in all, the presented microphysiological platform is a promising tool supporting 3-D tissue culture of pancreatic islet models in combination with non-invasive real-time and in situ monitoring of the tissue on-chip, while providing the opportunity to include further tissue-relevant components.
Data availability
The data that support the findings of this study are available from the corresponding author upon reasonable request.
Author contributions
KS: conceptualization, formal analysis, investigation, methodology, visualization, data curation, writing – original draft, writing – review & editing. MC: formal analysis, investigation, writing – review & editing, supervision. AZ: methodology, writing – review & editing. Stefanie Fuchs: methodology, writing – review & editing. TM: conceptualization, supervision, writing – review & editing. KSL: supervision, writing – review & editing. PL: conceptualization, methodology, supervision, funding acquisition, writing – review & editing.
Conflicts of interest
P. L. and K. S. are named as inventors on patent application PCT/EP2023/061827 (“Microfluidic device”) filed by Eberhard Karls University Tübingen. T. M. is a founder of and holds equity in PyroScience GmbH, and is the CEO of the Austrian branch, PyroScience AT GmbH. PyroScience is a developer, producer, and vendor of sensor technology.
Acknowledgements
This research has been supported by the European Union's Horizon 2020 research and innovation programme under grant agreement No. 812954.
References
- H. Sun, P. Saeedi, S. Karuranga, M. Pinkepank, K. Ogurtsova, B. B. Duncan, C. Stein, A. Basit, J. C. N. Chan, J. C. Mbanya, M. E. Pavkov, A. Ramachandaran, S. H. Wild, S. James, W. H. Herman, P. Zhang, C. Bommer, S. Kuo, E. J. Boyko and D. J. Magliano, Diabetes Res. Clin. Pract., 2022, 183, 109119 CrossRef PubMed
.
- A. Zbinden, J. Marzi, K. Schlünder, C. Probst, M. Urbanczyk, S. Black, E. M. Brauchle, S. L. Layland, U. Kraushaar, G. Duffy, K. Schenke-Layland and P. Loskill, Matrix Biol., 2020, 85, 205–220 CrossRef
.
- J. Rogal, A. Zbinden, K. Schenke-Layland and P. Loskill, Adv. Drug Delivery Rev., 2019, 140, 101–128 CrossRef CAS PubMed
.
- D. E. Ingber, Cell, 2016, 164, 1105–1109 CrossRef CAS PubMed
.
- P. Loskill, R. Hardwick and A. Roth, Stem Cell Rep., 2021, 16, 2033–2037 CrossRef PubMed
.
- M. Bakhti, A. Böttcher and H. Lickert, Nat. Rev. Endocrinol., 2019, 15, 155–171 CrossRef CAS PubMed
.
- D. E. Ingber, Nat. Rev. Genet., 2022, 23(8), 467–491 CrossRef CAS PubMed
.
- S. Fuchs, S. Johansson, A. Tjell, G. Werr, T. Mayr and M. Tenje, ACS Biomater. Sci. Eng., 2021, 7, 2926–2948 CrossRef CAS
.
- E. Ferrari, C. Palma, S. Vesentini, P. Occhetta and M. Rasponi, Biosensors, 2020, 10, 110 CrossRef CAS PubMed
.
- X. W. Ng, Y. H. Chung and D. W. Piston, Compr. Physiol., 2021, 11, 2191 Search PubMed
.
- G. Da Silva Xavier, J. Clin. Med., 2018, 7, 54 CrossRef PubMed
.
- J. Hilderink, S. Spijker, F. Carlotti, L. Lange, M. Engelse, C. van Blitterswijk, E. de Koning, M. Karperien and A. van Apeldoorn, J. Cell. Mol. Med., 2015, 19, 1836–1846 CrossRef CAS PubMed
.
- M.-J. Lecomte, S. Pechberty, C. Machado, S. Da Barroca, P. Ravassard, R. Scharfmann, P. Czernichow and B. Duvillié, Cell Med., 2016, 8, 99–112 CrossRef PubMed
.
- R. A. Zuellig, G. Cavallari, P. Gerber, O. Tschopp, G. A. Spinas, W. Moritz and R. Lehmann, J. Tissue Eng. Regen. Med., 2017, 11, 109–120 CrossRef CAS PubMed
.
- J. Mir-Coll, T. Moede, M. Paschen, A. Neelakandhan, I. Valladolid-Acebes, B. Leibiger, A. Biernath, C. Ämmälä, I. B. Leibiger, B. Yesildag and P. O. Berggren, Int. J. Mol. Sci., 2021, 22, 1813 CrossRef CAS PubMed
.
- S. H. Lee, S. Hong, J. Song, B. Cho, E. J. Han, S. Kondapavulur, D. Kim and L. P. Lee, Adv. Healthcare Mater., 2018, 7, 1701111 CrossRef PubMed
.
- Y. Jun, J. S. Lee, S. Choi, J. H. Yang, M. Sander, S. Chung and S. H. Lee, Sci. Adv., 2019, 5, eaax4520 CrossRef CAS PubMed
.
- S. Bauer, C. Wennberg Huldt, K. P. Kanebratt, I. Durieux, D. Gunne, S. Andersson, L. Ewart, W. G. Haynes, I. Maschmeyer, A. Winter, C. Ämmälä, U. Marx and T. B. Andersson, Sci. Rep., 2017, 7, 14620 CrossRef
.
- P. M. Misun, B. Yesildag, F. Forschler, A. Neelakandhan, N. Rousset, A. Biernath, A. Hierlemann and O. Frey, Adv. Biosyst., 2020, 4, e1900291 CrossRef PubMed
.
- T. Tao, Y. Wang, W. Chen, Z. Li, W. Su, Y. Guo, P. Deng and J. Qin, Lab Chip, 2019, 19, 948–958 RSC
.
- T. Tao, P. Deng, Y. Wang, X. Zhang, Y. Guo, W. Chen, J. Qin, T. Tao, P. Deng, Y. Wang, X. Zhang, Y. Guo, W. Chen and J. Qin, Adv. Sci., 2022, 9, 2103495 CrossRef PubMed
.
- R. Regeenes, Y. Wang, A. Piro, A. Au, C. M. Yip, M. B. Wheeler and J. V. Rocheleau, Biosens. Bioelectron. X, 2023, 13, 100285 CAS
.
- A. L. Glieberman, B. D. Pope, J. F. Zimmerman, Q. Liu, J. P. Ferrier, J. H. R. Kenty, A. M. Schrell, N. Mukhitov, K. L. Shores, A. B. Tepole, D. A. Melton, M. G. Roper and K. K. Parker, Lab Chip, 2019, 19, 2993–3010 RSC
.
- P. N. Silva, B. J. Green, S. M. Altamentova and J. V. Rocheleau, Lab Chip, 2013, 13, 4374 RSC
.
- K. S. Sankar, B. J. Green, A. R. Crocker, J. E. Verity, S. M. Altamentova and J. V. Rocheleau, PLoS One, 2011, 6, e24904 CrossRef CAS
.
- M. Nourmohammadzadeh, Y. Xing, J. W. Lee, M. A. Bochenek, J. E. Mendoza-Elias, J. J. McGarrigle, E. Marchese, Y. Chun-Chieh, D. T. Eddington, J. Oberholzer and Y. Wang, Lab Chip, 2016, 16, 1466–1472 RSC
.
- J. V. Rocheleau and D. W. Piston, Methods Cell Biol., 2008, 89, 71–92 CAS
.
- T. Hori, K. Yamane, T. Anazawa, O. Kurosawa and H. Iwata, Biomed. Microdevices, 2019, 21, 1–9 CrossRef
.
- K. Shik Mun, K. Arora, Y. Huang, F. Yang, S. Yarlagadda, Y. Ramananda, M. Abu-El-Haija, J. J. Palermo, B. N. Appakalai, J. D. Nathan and A. P. Naren, Nat. Commun., 2019, 10, 3124 CrossRef
.
- N. Navarro-Alvarez, J. D. Rivas-Carrillo, A. Soto-Gutierrez, T. Yuasa, T. Okitsu, H. Noguchi, S. Matsumoto, J. Takei, N. Tanaka and N. Kobayashi, Cell Transplant., 2008, 17, 111–119 Search PubMed
.
- L. A. Llacua, M. M. Faas and P. de Vos, Diabetologia, 2018, 61(6), 1261–1272 CrossRef CAS
.
- M. Urbanczyk, S. L. Layland and K. Schenke-Layland, Matrix Biol., 2020, 85, 1–14 CrossRef
.
- S. Abadpour, A. Aizenshtadt, P. A. Olsen, K. Shoji, S. R. Wilson, S. Krauss and H. Scholz, Curr. Diabetes Rep., 2020, 20, 1–13 CrossRef
.
- J. V. Rocheleau, G. M. Walker, S. S. Head, O. P. McGuinness and D. W. Piston, Proc. Natl. Acad. Sci. U. S. A., 2004, 101, 12899–12903 CrossRef CAS
.
- I. R. Sweet, G. Khalil, A. R. Wallen, M. Steedman, K. A. Schenkman, J. A. Reems, S. E. Kahn and J. B. Callis, Diabetes Technol. Ther., 2002, 4, 661–672 CrossRef
.
- W. Wang, L. Upshaw, D. M. Strong, R. P. Robertson and J. A. Reems, J. Endocrinol., 2005, 185, 445–455 CAS
.
- P. Maechler, Mol. Cell. Endocrinol., 2013, 379, 12–18 CrossRef CAS
.
- H. Zirath, M. Rothbauer, S. Spitz, B. Bachmann, C. Jordan, B. Müller, J. Ehgartner, E. Priglinger, S. Mühleder, H. Redl, W. Holnthoner, M. Harasek, T. Mayr and P. Ertl, Front. Physiol., 2018, 9, 815 CrossRef
.
- E. Haythorne, M. Rohm, M. van de Bunt, M. F. Brereton, A. I. Tarasov, T. S. Blacker, G. Sachse, M. Silva dos Santos, R. Terron Exposito, S. Davis, O. Baba, R. Fischer, M. R. Duchen, P. Rorsman, J. I. MacRae and F. M. Ashcroft, Nat. Commun., 2019, 10, 2474 CrossRef
.
- A. Bussooa, E. Tubbs, F. Revol-Cavalier, A. Chmayssem, M. Alessio, M. L. Cosnier and N. Verplanck, Biosens. Bioelectron. X, 2022, 11, 100198 CAS
.
- S. Fuchs, R. W. J. van Helden, M. Wiendels, M. N. S. de Graaf, V. V. Orlova, C. L. Mummery, B. J. van Meer and T. Mayr, Mater. Today Bio, 2022, 17, 100475 CrossRef CAS
.
- O. Schneider, A. Moruzzi, S. Fuchs, A. Grobel, H. S. Schulze, T. Mayr and P. Loskill, Mater. Today Bio, 2022, 15, 100280 CrossRef CAS PubMed
.
- M. Busche, D. Rabl, J. Fischer, C. Schmees, T. Mayr, R. Gebhardt and M. Stelzle, EXCLI J., 2022, 21, 144 Search PubMed
.
- P. Loskill, T. Sezhian, K. M. Tharp, F. T. Lee-Montiel, S. Jeeawoody, W. M. Reese, P.-J. H. Zushin, A. Stahl and K. E. Healy, Lab Chip, 2017, 17, 1645–1654 RSC
.
- C. Poon, J. Mech. Behav. Biomed. Mater., 2022, 126, 105024 CrossRef CAS PubMed
.
- P. Buchwald, A. Tamayo-Garcia, V. Manzoli, A. A. Tomei and C. L. Stabler, Biotechnol. Bioeng., 2018, 115, 232–245 CrossRef CAS PubMed
.
- A. Colom, R. Galgoczy, I. Almendros, A. Xaubet, R. Farré and J. Alcaraz, J. Biomed. Mater. Res., Part A, 2014, 102, 2776–2784 CrossRef PubMed
.
- A. Zbinden, M. Urbanczyk, S. L. Layland, L. Becker, J. Marzi, M. Bosch, P. Loskill, G. P. Duffy and K. Schenke-Layland, Tissue Eng., Part A, 2021, 27, 977–991 CrossRef CAS PubMed
.
- D. Pipeleers, R. Kiekens, Z. Ling, A. Wilikens and F. Schuit, Diabetologia, 1994, 37, S57–S64 CrossRef
.
- J. C. Stendahl, D. B. Kaufman and S. I. Stupp, Cell Transplant., 2009, 18, 1–12 Search PubMed
.
- R. K. P. Benninger, W. S. Head, M. Zhang, L. S. Satin and D. W. Piston, J. Physiol., 2011, 589, 5453–5466 CrossRef CAS PubMed
.
- N. J. Hart, C. Weber, N. Price, A. Banuelos, M. Schultz, B. Huey, E. Harnois, C. Gibson, L. V. Steyn, K. K. Papas and R. M. Lynch, Am. J. Physiol., 2021, 321, C247–C256 CrossRef CAS PubMed
.
- C. Ricordi, D. W. R. Gray, B. J. Hering, D. B. Kaufman, G. L. Warnock, N. M. Kneteman, S. P. Lake, N. J. M. London, C. Socci, R. Alejandro, Y. Zeng, D. W. Scharp, G. Viviani, L. Falqui, A. Tzakis, R. G. Bretzel, K. Federlin, G. Pozza, R. F. L. James, R. V. Rajotte, V. Di Carlo, P. J. Morris, D. E. R. Sutherland, T. E. Starzl, D. H. Mintz and P. E. Lacy, Acta Diabetol. Lat., 1990, 27(3), 185–195 CrossRef CAS
.
- J. Chuchuy, J. Rogal, T. Ngo, K. Stadelmann, L. Antkowiak, K. Achberger, S. Liebau, K. Schenke-Layland and P. Loskill, ACS Biomater. Sci. Eng., 2021, 7, 3006–3017 CrossRef CAS PubMed
.
- S. Halldorsson, E. Lucumi, R. Gómez-Sjöberg and R. M. T. Fleming, Biosens. Bioelectron., 2015, 63, 218–231 CrossRef CAS PubMed
.
- V. Palacio-Castañeda, N. Velthuijs, S. Le Gac and W. P. R. Verdurmen, Lab Chip, 2022, 22, 1068–1092 RSC
.
- M. Riopel and R. Wang, Front. Biosci.-Landmark, 2014, 19, 77–90 CrossRef
.
- M. D. Hunckler and A. J. García, Adv. Funct. Mater., 2020, 30, 2000134 CrossRef CAS
.
- M. Urbanczyk, A. Zbinden, S. L. Layland, G. Duffy and K. Schenke-Layland, Tissue Eng., Part A, 2020, 26, 387–399 CrossRef CAS PubMed
.
- W. C. Dissanayake, B. Sorrenson and P. R. Shepherd, Biosci. Rep., 2018, 38, 20170989 CrossRef PubMed
.
- A. Chaudhury, C. Duvoor, V. S. Reddy Dendi, S. Kraleti, A. Chada, R. Ravilla, A. Marco, N. S. Shekhawat, M. T. Montales, K. Kuriakose, A. Sasapu, A. Beebe, N. Patil, C. K. Musham, G. P. Lohani and W. Mirza, Front. Endocrinol., 2017, 8, 6 Search PubMed
.
- J. E. Campbell and C. B. Newgard, Nat. Rev. Mol. Cell Biol., 2021, 22(2), 142–158 CrossRef CAS PubMed
.
- L. Prasad-Reddy and D. Isaacs, Drugs Context, 2015, 4, 212283 Search PubMed
.
- P. Buchwald, Theor. Biol. Med. Modell., 2009, 6, 5 CrossRef PubMed
.
- P. Maechler and C. B. Wollheim, J. Physiol., 2000, 529(Pt 1), 49–56 CrossRef CAS
.
- K. J. Morten, M. Potter, L. Badder, P. Sivathondan, R. Dragovic, A. Neumann, J. Gavin, R. Shrestha, S. Reilly, K. Phadwa, T. A. Lodge, A. Borzychowski, S. Cookson, C. Mitchell, A. Morovat, A. K. Simon, J. Uusimaa, J. Hynes and J. Poulton, Wellcome Open Res., 2019, 2, 14 Search PubMed
.
- I. Chareyron, S. Christen, S. Moco, A. Valsesia, S. Lassueur, L. Dayon, C. B. Wollheim, J. Santo Domingo and A. Wiederkehr, Diabetologia, 2020, 63, 2628–2640 CrossRef CAS PubMed
.
- N. B. Kramer, C. Lubaczeuski, M. Blandino-Rosano, G. Barker, G. K. Gittes, A. Caicedo and E. Bernal-Mizrachi, Diabetes, 2021, 70, 477–491 CrossRef CAS PubMed
.
- E. Lorza-Gil, F. Gerst, M. B. Oquendo, U. Deschl, H.-U. Häring, M. Beilmann and S. Ullrich, Sci. Rep., 2019, 9, 10261 CrossRef
.
- K. I. Aamodt and A. C. Powers, Diabetes, Obes. Metab., 2017, 19, 124–136 CrossRef CAS PubMed
.
- L. A. Llacua, M. M. Faas and P. de Vos, Diabetologia, 2018, 61(6), 1261–1272 CrossRef CAS PubMed
.
- C. Pignatelli, F. Campo, A. Neroni, L. Piemonti and A. Citro, Transplant Int., 2022, 35, 168 CrossRef PubMed
.
- J.-C. Henquin, D. Dufrane, J. Kerr-Conte and M. Nenquin, Am. J. Physiol., 2015, 309, E640–E650 CAS
.
- L. S. Satin, P. C. Butler, J. Ha and A. S. Sherman, Mol. Aspects Med., 2015, 42, 61–77 CrossRef CAS PubMed
.
|
This journal is © The Royal Society of Chemistry 2024 |
Click here to see how this site uses Cookies. View our privacy policy here.