DOI:
10.1039/D3LC00622K
(Critical Review)
Lab Chip, 2024,
24, 1088-1120
Expanding CAR-T cell immunotherapy horizons through microfluidics
Received
18th July 2023
, Accepted 20th December 2023
First published on 4th January 2024
Abstract
Chimeric antigen receptor (CAR)-T cell therapies have revolutionized cancer treatment, particularly in hematological malignancies. However, their application to solid tumors is limited, and they face challenges in safety, scalability, and cost. To enhance current CAR-T cell therapies, the integration of microfluidic technologies, harnessing their inherent advantages, such as reduced sample consumption, simplicity in operation, cost-effectiveness, automation, and high scalability, has emerged as a powerful solution. This review provides a comprehensive overview of the step-by-step manufacturing process of CAR-T cells, identifies existing difficulties at each production stage, and discusses the successful implementation of microfluidics and related technologies in addressing these challenges. Furthermore, this review investigates the potential of microfluidics-based methodologies in advancing cell-based therapy across various applications, including solid tumors, next-generation CAR constructs, T-cell receptors, and the development of allogeneic “off-the-shelf” CAR products.
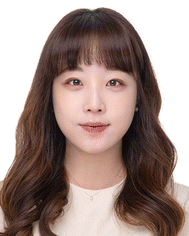 Hyelee Kim | Hyelee Kim earned her bachelor's degree in biomedical engineering from Korea University in 2022 and is currently enrolled in a master's degree program in the Department of Bioengineering at Korea University. Under the supervision of Prof. Aram Chung since 2022, she serves as a research assistant in the Biomicrofluidics Lab. Her research focuses on developing a microfluidic intracellular delivery platform to advance cell-based immunotherapy. |
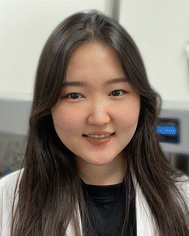 Suyeon (Sarah) Kim | Suyeon (Sarah) Kim graduated from the University of Illinois at Urbana-Champaign in 2021 with a Bachelor's degree in Bioengineering and from Korea University in 2023 with a Master's degree in Biomedical Engineering. Her master's thesis project focused on the fabrication of high-aspect-ratio silicon nanostructures for the intracellular delivery of biomolecules into hard-to-transfect cells. Currently, she is pursuing her Ph.D. at Carnegie Mellon University in the Department of Biomedical Engineering. Her research focuses on flexible oxygen generation devices in implantable immunoisolating devices for cancer cell therapy. |
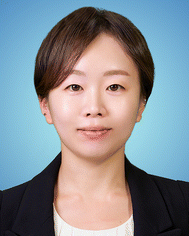 Hyunjung Lim | Hyunjung Lim earned her B.S. from Korea University in 2012 and completed her Ph.D. at Korea University in 2020. She currently serves as a Research Professor at the Interdisciplinary Program in Precision Public Health (PPH) at Korea University. Her research focuses on microfluidic devices for sample preparation and microfluidic platforms for intracellular delivery. |
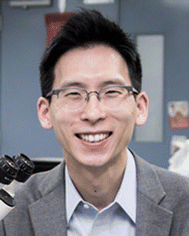 Aram Chung | Aram Chung received his B.S. from Seoul National University in 2006 and completed his Ph.D. at Cornell University in 2011. Following postdoctoral studies at UCLA from 2011 to 2013, he joined RPI as an Assistant Professor. In 2017, he moved to the School of Biomedical Engineering at Korea University, where he now serves as a Professor. His research pioneered the establishment of microfluidic platforms for immunotherapy and genome editing. He has also taken on a leadership role in technology entrepreneurship, founding a company (MxT Biotech) that commercializes intellectual property developed in his lab. |
1. Introduction
Historically, cancer treatment has involved the implementation of traditional therapeutic methods, including surgery, radiation therapy, and chemotherapy. These approaches, separately or in combination, have shown considerable success in the fight against cancer; however, overall cancer survival statistics have not significantly improved. Immunotherapy has recently gained significant recognition as a next-generation treatment modality that harnesses the inherent capabilities of the immune system of patients to combat cancer. This form of biological treatment employs various strategies, such as immune checkpoint inhibitors, cancer vaccines, and chimeric antigen receptor (CAR)-T cells. Among these, CAR-T cell therapy has emerged as a breakthrough, providing remarkable efficacy and renewed hope for patients with limited treatment options or relapsed/refractory disease.1 CAR-T cell therapy leverages genetically engineered T cells from patients to recognize and attack cancer cells. This method has been effective in treating hematological malignancies, such as leukemia, lymphoma, and multiple myeloma.2–4 As of January 2023, the US Food and Drug Administration (FDA) has approved six CAR-Tcell therapy products (Kymriah®, Breyanzi®, Abecma®, Carvykti®, Yescarta®, and Tecartus®), and more than 1000 clinical trials are currently underway.5 Moreover, CAR-T cell therapy has shown the ability to induce long-lasting complete remission that persists for over 10 years,6 thereby demonstrating its potential to revolutionize the current cancer treatment paradigm.
CAR-T cell production begins with the processing of whole blood collected from patients, as illustrated in Fig. 1. As T cells are derived from the peripheral blood of patients with cancer, achieving a large cell yield is crucial. Magnetic bead-conjugated antibodies are used for isolating T cells. The isolated autologous T cells are then non-antigen-specifically activated using anti-CD3/CD28 antibodies or nanoparticles. Following activation, the cells are transfected with CAR transgenes with gamma-retro- or lentiviral vectors. CAR-T cells are further expanded to achieve the required clinical dose. This expansion is typically accomplished by co-incubating the cells with anti-CD3/CD28 antibodies or nanoparticles, which were also used in the previous step of T cell activation. Finally, these CAR-T cells are cryopreserved and transported to clinical sites to reinject them into patients.7,8
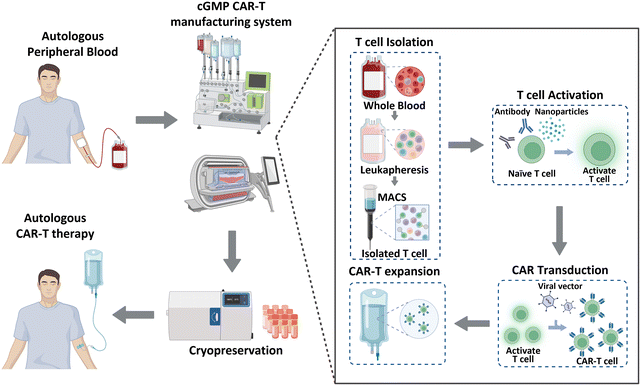 |
| Fig. 1 Overview of CAR-T cell manufacturing process. | |
Despite the promising outcomes, CAR-T-cell therapy still requires considerable development before it can be used as a viable alternative to existing cancer treatment approaches. Notably, the efficacy of CAR-T cell therapy is substantially low for solid tumors than that for blood cancers.9 Moreover, the high cost of CAR-T cell therapy is a major barrier to its widespread accessibility and benefit.10 Safety concerns related to mutagenesis and off-targeting effects due to the use of viruses, limited scalability, lack of standardization because of manufacturing challenges, along with other obstacles, hinder the democratization of the therapy.11 Thus, immediately addressing these limitations to boost the adoption of CAR-T cell therapy is crucial. We believe that microfluidics and microfluidics-associated technologies hold great potential to overcome or aid in overcoming these challenges and provide breakthrough advancements in CAR-T cell therapy.
Microfluidic lab-on-a-chip technologies offer numerous advantages, such as the reduction of the amount of substances needed for analysis, cost-effectiveness, operational simplicity, high-throughput processing, precise flow and cell control, and the potential for fully automated operations.12 These advantages position microfluidic technologies as a practical toolkit for addressing various challenges in biomedical research. Further, microfluidic technologies offer other advantages, such as improving point-of-care diagnosis,13 facilitating drug screening and development,14 simplifying sample preparation and control,15 advancing precision medicine,16 enabling single-cell and spatial multi-omics,17 and more.18 Therefore, microfluidics-based methods have found great utility in CAR-T cell therapy, thus potentially enhancing cancer immunotherapy research.
Several reviews are available on the current limitations and future prospects of CAR-T cell immunotherapy; however,1,5,19–21 only few reviews that are exclusively dedicated to exploring microfluidic approaches within CAR-T cell therapy have been published.
Therefore, this review aims to provide an overview of the current state-of-the-art CAR-T cell therapy, with particular emphasis on its production, while also highlighting existing challenges and bottlenecks. Further, it delves into how current and potential microfluidic technologies can specifically address these challenges, thereby contributing to the advancement of CAR-T cell therapy. Moreover, this review aims to explore the development of next-generation CAR-T cell approaches from a microfluidic perspective. By presenting the current landscape of CAR-T cell therapy and associated limitations and showcasing the potential solutions enabled by microfluidics, this review seeks to shed light on new opportunities for incorporating microfluidic technologies, thus expanding the horizon for CAR-T cell therapy (Fig. 2).
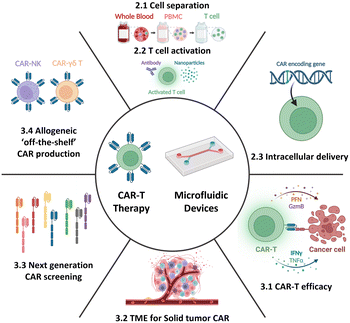 |
| Fig. 2 Microfluidic application for CAR-T cell production and cell-based immunotherapy. | |
2. CAR-T cell production overview and microfluidic opportunities
2.1. T cell isolation from whole blood
The peripheral blood is the primary source of material for CAR-T cell drug production. The initial ex vivo step in CAR-T cell production involves isolating T cells, a specific subset of white blood cells (WBCs) found within peripheral blood mononuclear cells (PBMCs). A 1 mL sample of whole blood derived from a healthy adult contains approximately 2 × 106 PBMCs, comprising 25–60% CD4-positive T cells and 5–30% CD8-positive T cells. PBMCs isolated from patients with cancer yield different proportions of CD4-positive T cell subtype with increased T helper 2-cells and low levels of cancer cell cytotoxic cytokines, including TNF-α and INF-γ.22 Given that the required dose of CAR-T cells per patient exceeds 1 × 108 CAR-expressing cells,4 achieving high scalability (i.e., processing of large cell numbers) is essential for blood cell sorting and classification platforms. In the following section, we discuss the separation of PBMCs from whole blood and subsequent isolation of T cells to manufacture CAR-T cells.
2.1.1. Conventional T cell isolation from whole blood.
2.1.1.1. PBMC isolation from whole blood.
The initial step of drug production in all FDA-approved CAR-T cell therapies involves the preparation of PBMCs from whole blood. PBMCs can be prepared via leukapheresis, which involves separating mononuclear cells, including lymphocytes, natural killer (NK) cells, and monocytes, from the anticoagulated peripheral blood. This separation can be achieved using two common benchtop methods (Fig. 3), namely density gradient centrifugation and elutriation centrifugation. During density gradient centrifugation, density gradient media are used to create layers with varying densities, ranging from low to high. These layers separate PBMCs from red blood cells (RBCs). PBMCs are then harvested from the thin layer between the plasma and gradient media during centrifugation.23 In contrast, elutriation centrifugation employs two opposite forces, fluid flow and centrifugation, to separate cells based on size, morphology, and density, which can be handled by the instrument without additional processes.24–26
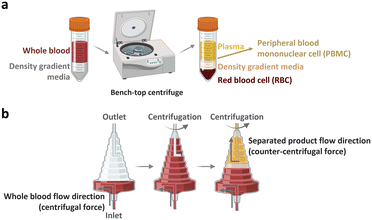 |
| Fig. 3 Benchtop cell separation methods. (a) Density gradient centrifugation. (b) Elutriation centrifugation. | |
2.1.1.2. T cell isolation from PBMCs.
In FDA-approved CAR-T cell products, direct activation of T cells occurs in the leukapheresis samples. However, in current fully automated CAR-T cell production instruments, such as CliniMACS Prodigy (Miltenyi Biotec, Germany) and Cocoon (Lonza, Switzerland), challenges arise due to cellular heterogeneity within PBMCs in leukapheresis samples.27,28 Therefore, further purification should be achieved through antibody-mediated approaches such as magnetic-activated cell sorting (MACS) and fluorescence-activated cell sorting (FACS). In MACS, columns packed with antibody-conjugated magnetic beads are placed between magnets.29 Within these columns, cells expressing the target antibodies are captured, and the remaining cells are extracted. Positive selection utilizes biomarkers expressed on T cells (e.g., CD3 and CD28) to specifically capture T cells, whereas negative selection uses biomarkers that are not expressed on T cells (e.g., CD56) to exclude unwanted cell populations. Alternatively, FACS enables T cell sorting using antibody-conjugated fluorophores.30,31 The emitted signal allows isolation of T cells within the electric field by activating fluorophores with a laser.
2.1.2. Microfluidic T cell and PBMC separation from whole blood.
Among the conventional technologies, apheresis systems, such as COBE Spectra (Terumo BCT, USA), can process approximately 1.93 × 108 CD34-positive cells in whole blood at a rate of 85 mL min−1.32 Moreover, automated MACS can isolate 2 × 108 labeled cells from up to 15 mL of whole blood.33 Furthermore, the fully automated CliniMACS Prodigy directly isolates CD4-positive or CD8-positive T cells from whole blood with 90% purity.34 Thus, microfluidics can play a pivotal role for improved scalability and purity.
Microfiltration membranes and microfluidic density gradients have been employed to classify PBMCs isolated from blood samples.35–37 The microfluidic density gradient approach leverages the equilibrium between centrifugal and buoyancy forces acting on blood cells within a rotating construct.38 Using this label-free microfluidic technology, PBMCs can be isolated from whole blood at a processing rate of approximately 30 μL min−1. Chen et al. presented compelling results, achieving high-throughput (<20 mL min−1) and high-purity (∼97%) separation for immunophenotyping subpopulations of immune cells using microfiltration membranes.35 When the blood sample was passed through a microfiltration membrane made of polydimethylsiloxane (PDMS), target cells larger than the filter's pore size were captured by antibody-conjugated beads. Moreover, direct T cell isolation from blood samples has been demonstrated, primarily for diagnostic purposes, such as for HIV, immunological change monitoring, and basic biological research (Fig. 4a and b).39–44 These studies used antibody-conjugated surfaces or magnetic beads for T cell separation, thus achieving purity greater than 95%. The throughput of these devices is insufficient for cell-based immunotherapy, via channel parallelization; however, higher scalability can potentially be expected.
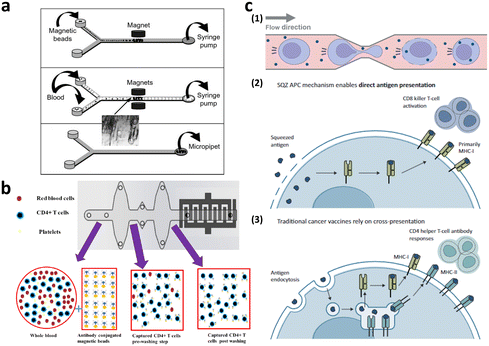 |
| Fig. 4 Microfluidic immune cell separation and T cell activation. (a) T cell separation from whole blood using the immunomagnetic microfluidic system for PCR analysis. Reproduced with ref. 43 with permission from The Royal Society of Chemistry. (b) Microfluidic CD4-positive T cell quantification from whole blood. Reproduced from ref. 40 (CC BY). (c) Microfluidic engineering of antigen presenting cell (APC) for T cell activation. Reproduced from ref. 60 (CC BY-NC-ND 4.0). | |
Recent advancements in microfluidic technologies have prompted significant research on label-free microfluidic cell or particle separation employing deterministic lateral displacement (DLD),45 dielectrophoresis (DEP),46 and acoustophoresis.47 However, these studies primarily emphasize on the separation of WBCs and RBCs, thus leading to a paucity of investigations that directly classify T cells or PBMCs from whole blood. Thus, prioritizing the development of complete solutions that can directly isolate T cells or PBMCs from whole blood with high scalability in a label-free manner is essential for the field of microfluidics. Microfluidic technologies can provide practical solutions, which can be immediately applied to CAR-T cell manufacturing, by shifting the focus to T cell and PBMC separation in a sample-in-and-sample-out format.
2.2. T cell activation and expansion
T cells intended for clinical use in CAR-T cell therapy are typically activated before and/or after gene transfer to meet the requirements for clinical doses. For in vivo activation of T cells, the use of autologous dendritic cells (DC) and B cells can be exploited, thus allowing for antigen-specific activation using various co-stimulatory molecules.48 However, owing to inherent patient variability and differences in culture systems between DCs and B cells, allogeneic exogenous antigen-loaded antigen-presenting cells (APCs), commonly referred to as feeder cells, are typically co-cultured with T cells ex vivo. Nevertheless, it's important to note that the use of artificial APCs in this manner has certain limitations in terms of scalability. Therefore, non-antigen-specific activation is often performed using anti-CD3/CD28 antibodies or beads.49,50 Six FDA-approved CAR-T cell products enrich T cells using this non-antigen-specific activation process.51,52 In addition to anti-CD3/CD28 activation, cytokines, such as interleukin (IL)-2, IL-4, IL-7, and IL-15, which play roles in T cell survival and activation, are incorporated into the ex vivo culture media.53
Conventional methods for T cell expansion and activation still has a limitation in that the interactions of T cells with other substances are neither controlled nor well-defined.54 To address this limitation, researchers have explored more precise approaches that are aimed at controlling the interactions of T cells with activation pathways. One such approach is the use of microfluidic platforms, which enable dynamic manipulation of the cells and cellular microenvironment55–57 and then allow T cell activation at the single-cell level.54,58,59 These microfluidic approaches not only precisely control T cell activation but also facilitate the study of T cell heterogeneity at the single-cell level. In addition, to address the issue of low scalability associated with feeder cell-based T cell activation, a microfluidic intracellular delivery platform for the large-scale production of feeder cells has been introduced as a practical solution (Fig. 4c).60
2.3. Gene transfer to T cells for CAR expression
To manufacture CAR-T cells, internalizing CAR genes into individual T cells is necessary. To achieve this, various techniques have been developed. These techniques can be broadly classified into two categories, namely viral transduction, and non-viral transfection technologies (Fig. 5). In the subsequent sections, we provide an overview of these methods and discuss how microfluidics-related technologies can help address current limitations.
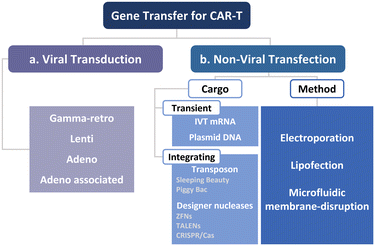 |
| Fig. 5 Two routes for the intracellular delivery for CAR-T cell production. (a) Viral transduction and (b) non-viral technologies, including electroporation, lipofection, and microfluidic membrane disruption-mediated approaches that deliver transient and integrated cargos. | |
2.3.1. Viral vectors for CAR-T cell production.
The current standard method for introducing CAR genes into T cells is viral transduction, which is utilized in approximately 94% of evaluable CAR-T cell manufacturing routes.61 Owing to their ability to integrate into the T cell genome, viral vectors exhibit high transduction efficiency, up to 70%,62 and provide long-lasting drug efficacy in the fight against cancer.6
Lentiviral and gammaretroviral vectors are the most commonly used viral vectors in CAR-T cell manufacturing, among a variety of viral vectors, including adenovirus and adeno-associated virus.63–65 All six FDA-approved CAR-T cell therapy products are based on lentiviral vector (Kymriah®, Breyanzi®, Abecma®, and Carvykti®) and gammaretroviral vector (Yescarta® and Tecartus®) as their methods of T cell transduction.
Virus-based T cell transduction can be divided into two discrete stages: the production of viral vector and subsequent transduction of T cells to enable CAR expression (Fig. 6). The initial step involves preparing a substantial quantity of viral vectors and T cells and allowing the introduction of viral vectors into target T cells on day 0. The former phase entails the production of viral vectors utilizing vector packaging cells and activation of T cells from day −6 to day −1.
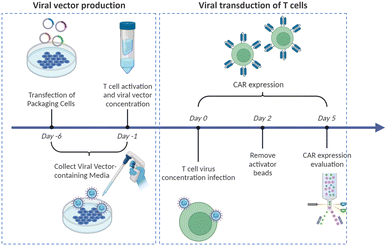 |
| Fig. 6 Timeline for generating CAR-T cells via viral transduction. | |
Stable packaging cell lines, namely, PA31713, PG1314, and 293GP15, have been extensively employed for gammaretroviral vectors.66 These cell lines express gag-pol-rev in a stable manner, thereby eliminating the need for the introduction of supplementary packaging constructs. This enhances compatibility with current good manufacturing practices (cGMP) by providing traceability of clinical vector producer cell lines. Moreover, these cell lines can enhance viral titers and safety. Conversely, there is currently a lack of stable packaging cell lines for lentiviral vectors, thus leading to the common usage of HEK293T cells for packaging purposes.67 Briefly, CAR vectors are produced by co-delivering vector plasmids and packaging plasmids that contain essential genes (gag, pol, rev, and env) required for viral replication and packaging. Owing to low transfection efficiency, co-incubating with significant quantities of plasmid DNA for transient transfection is necessary. The packaging cells produce the desired CAR vectors, which are then collected, filtered, and concentrated from the media over several days.
Upon completion of CAR vector preparation on day 0 (Fig. 6), these viral vectors are introduced into the activated T cells. Following 3–7 days of culture to enable transduction and proliferation, the transduced T cells are collected, filtered, and subjected to a validation assay to confirm CAR expression. The cells are finally cryopreserved.68
2.3.1.1. Current limitations of viral transduction.
As described above, viral vectors are primarily used in the production of CAR-T cells; however, there are certain challenges that prevent viral transduction to become a reliable and affordable cancer treatment option.40 One of the major concerns is related to biological complications associated with viral vector-mediated transduction. These complications include undesired effects on the expression of genes related to inflammation, metabolism, and cell cycle regulation,69,70 induction of cell stress, and influence on cell proliferation.71 Additionally, the tendency of retroviral vectors to integrate near transcriptional start sites may lead to their insertion adjacent to oncogenic promoters, which can potentially trigger cancer development.72 Furthermore, viral vectors have a limited packaging capacity that typically carry only a single type of cargo at a time, with a genome size typically ranging from 5 to 7.5 kilobase pairs (kbp).73 The most critical hindrance to the widespread use of viral transduction is its exceptionally high production cost and requirement of excessive number of packaging cells and specialized facilities. These limitations render viral vectors suboptimal for genome editing applications. Therefore, to advance CAR-T cell therapy as a safe, efficacious, and affordable cancer treatment option, an ideal transfection/transduction platform should be minimally invasive, scalable, adaptable to hard-to-transfect cells, dosage-controllable, and cost-effective.73
2.3.1.2. Microfluidic technologies to facilitate viral transduction.
Currently, the production of viral vectors for CAR-T cell therapy relies on transient and diffusion-limited transduction of packaging cells. This approach leads to costly and low-throughput production that is characterized by considerable wastage of virus mass.74 Furthermore, static well-based T cell cultures and exposure to viral vectors contribute to significant quantity of vector waste, thereby increasing the overall cost of CAR-T cell therapy. To overcome these limitations, researchers have explored the use of centrifugation to enhance transduction efficiency in target-cell–virus cultures, thus overcoming the diffusion-limited exposure to viral vectors.75,76 Moreover, the introduction of small-molecule and peptide additives has been investigated to enhance the interaction between the virus and target cells.77,78 However, the success of centrifugation relies on cell-specific stress responses and removal of unbound virus from the final product through labor-intensive processes.
The implementation of microfluidics offers promising solutions to the current limitations. Microfluidic cell culture systems have become increasingly popular owing to their ability to miniaturize sample volumes, facilitate convective mass transport, and offer precise control of external environmental factors,79–82 which can significantly benefit viral vector-mediated transduction.83–86 Quach et al. developed the lentiviral generation (LENGEN) system, which is an automated microfluidic system for lentiviral vector production and transduction (Fig. 7a).74 By reducing the need for manual handling of cells, such as pipetting and centrifugation, the system has achieved fully automated production. The increased surface-to-volume ratio of the LENGEN system has enabled faster gene transfer kinetics with a reduced working volume, thus resulting in a 100-fold reduction in lentiviral sample volume. This system integrates various processes into a microfluidic device, including vector generation and packaging, cell culture, transduction, and analysis. As a result, the lentiviral vector generation time significantly decreased from over 7 days to only 2 days, while maintaining a comparable functional titer of approximately 1 × 107 TU mL−1.
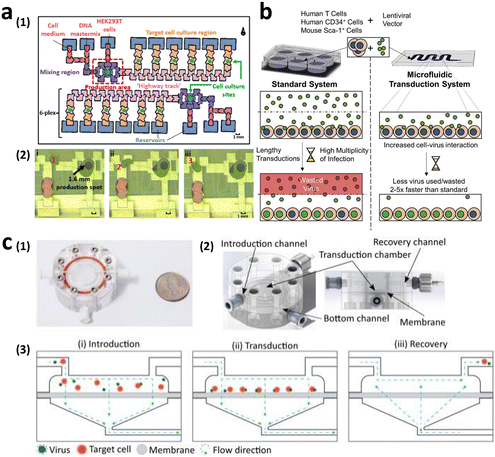 |
| Fig. 7 Microfluidic technologies to enhance viral transduction. (a) Lentiviral generation (LENGEN) system for lentiviral vector generation, packaging, and transduction. Reprinted with permission from ref. 74. Copyright 2022 American Chemical Society. (b) Ex vivo microfluidic lentiviral vector transduction platform for primary human T cells. Reprinted from ref. 87, Copyright 2017 with Elsevier. (c) Microfluidic transduction device (MTD) that combines microfluidic spatial confinement with advective flow through a membrane to enhance lentiviral transduction. Reproduced from ref. 86 (CC BY 4.0). | |
In addition to enhancing packaging cell transduction for higher-titer viral vector generation, researchers have explored the use of microfluidics to improve T cell transduction efficiency. Tran et al. demonstrated lentiviral transduction of primary human T cells at a processing rate up to 5-fold faster than that of conventional transduction protocols. This was achieved only with one-twentieth of the lentiviral vector volume, resulting in a titer of 8.9 × 107 TU mL−1, surpassing current clinical transduction platforms (Fig. 7b).87 Furthermore, Moore et al. achieved up to a 4-fold increase in lentiviral transduction efficiency of 2 × 106 T cells compared with that under static conditions, utilizing only half of the virus. This improvement was achieved by enhancing the colocalization of the virus and target cells through microfluidic spatial confinement and advective flow (Fig. 7c).86
These proof-of-concept implementations have demonstrated remarkable potential for integration into the manufacturing of adoptive cell therapy products; however, there are several other factors that still require optimization. These include addressing challenges related to high cell density, potential depletion of oxygen and nutrients due to the small volume of medium, and the impact of shear stress that is exerted on cells by medium flow. Despite advancements in highly efficient vector production methods, safety concerns; that is, off-targeting, genotoxicity, associated with viral transduction of immune cells still remain.
2.3.2. Non-viral technologies for CAR-T cell production.
To address the limitations associated with virus-based methods for CAR-T cell manufacturing, non-viral approaches for T cell transfection have attracted substantial attention.88 Non-viral intracellular delivery strategies encompass carrier-mediated and membrane disruption-mediated approaches. Carrier-mediated gene delivery methods, such as lipofection, utilize cationic lipid carriers to deliver cargos into cells through membrane fusion with subsequent delivery within the cells. Conversely, membrane disruption-mediated strategies involve direct penetration and/or permeabilization of the plasma membrane of cells, creating discontinuities that enable the cargos to gain intracellular access.89
An emerging technique for direct cell penetration for nucleic acid delivery involves the use of high-aspect-ratio nanostructures, which apply sufficient mechanical strain to induce plasma membrane penetration and/or permeabilization. High-aspect-ratio nanostructures (often combined with microfluidics) have demonstrated potential as effective tools for intracellular delivery.90–94 Moreover, they offer several advantages such as minimal perturbation of gene expression, cell type insensitiveness, scalability, and compatibility with cGMP sterilization processes.95 However, their translational potential is currently hindered by limitations, including low-throughput transfection, complex nanofabrication processes, and competition with well-established mainstream transfection technologies. Thus, further research is warranted to investigate the mechanism underlying cell–nanostructure interactions and long-term effects of nanostructure-mediated transfection on transfected cells.
Beyond high-aspect-ratio nanostructures, in the subsequent subsections, we focus on intracellular delivery technologies that hold promise for the effective transfection of primary immune cells with direct clinical implications on CAR-T cell therapy. First, we will introduce other key non-viral technologies and their applications in CAR-T cell production. Subsequently, we will explore T cell transfection or CAR-T cell production using electroporation, lipofection, and microfluidic mechanoporation transfection (Fig. 5b).
2.3.2.1. Cargo types for non-viral CAR-T cell production.
Prior to discussing the various non-viral intracellular delivery methods, we will briefly begin by examining types of cargo and their respective properties. Cargos employed for intracellular delivery encompass a wide range of sizes, shapes, chemical properties, and origins. They can originate from typical biomolecules, such as proteins, DNAs, and RNAs, and from synthetic nanomaterials.89 These cargos can be classified into two categories based on the genomic integration, namely transient and integrated cargo.
2.3.2.1.1. Transient cargos.
Transient cargos, such as RNAs and plasmid DNAs, serve as alternatives to viral vectors, thus addressing safety concerns related to unintended integration and permanent gene expression. This review encompasses the transfection of T cells with mRNAs and plasmid DNAs using non-viral technologies for the aforementioned safety reasons. However, the degradability of transient cargos in the cytosol and nucleus poses a clear limitation in terms of expression duration, which can impede effective CAR-T cell production. Therefore, non-viral technologies are now being explored for delivering integrated cargos.
2.3.2.1.2. Integrated cargos.
Transposon (transposable element).
In addition to FDA-approved CAR-T cell therapy products that utilize an integrative viral vector, efforts have been made to manufacture integrated CAR-T cells using non-viral vectors such as transposable elements (TEs), also known as jumping genes or transposons.96 These transposons are DNA sequences capable of repositioning a gene of interest (GOI) through a cut-and-paste mechanism that is facilitated by the enzyme transposase.97 The transposase gene can be delivered in the form of plasmid DNA or synthesized mRNA, along with plasmid DNA containing the GOI sequence that is flanked by terminal inverted repeats (TIRs). Once translated, transposase recognizes the TIRs (cut operation) and relocates the GOI to a specific site in the host's chromosomal sequence (paste operation). Among the transposons, Sleeping Beauty (SB)98 and piggyBac (PB)99 are well-known examples that exhibit integration efficiencies comparable to those of retroviral vectors.
Designer nuclease (genome editing technology).
Despite the success of autologous CAR-T cells, there are several advantages of using allogeneic CAR-T cells generated from healthy donors. These advantages include the immediate availability of “off-the-shelf” cryopreserved batches and reduced costs.100 However, when allogenic CAR-T cells are infused into a patient, the endogenous αβ T cell receptors on these cells may recognize histocompatibility antigens in the patient, potentially leading to graft-versus-host disease (GvHD). To address this issue, engineered nucleases, such as zinc-finger nucleases (ZFNs),101 transcription activator-like effector nucleases (TALENs),102 and clustered regularly interspaced short palindromic repeats (CRISPR)-Cas9, have garnered substantial attention as new solutions.103,104 Since detailed information on genome editing methods can be found elsewhere,105,106 our aim here is to highlight their potential applications in cell-based therapy through microfluidics alongside a brief introduction.107
Zinc-finger proteins (ZFPs) recognize specific target DNA sequences by binding amino acids at the α-helix contact. Synthetic zinc-finger proteins linked to the Flavobacterium okeanokoites (Fokl) nuclease can recognize 9–18 bp sequences. Two separate zinc-finger nucleases, referred to as left and right nucleases, cleave the target DNA between the recognition sites of the ZFPs. Similarly, TALENs utilize Fokl nucleases and TALE proteins that function as ZFPs in ZFNs. Each TALE protein consists of repeat domains composed of 33–35 amino acids, where each repeat, known as a repeat-variable di-residue, recognizes a single base pair of the target sequence. Compared with ZFN, TALENs offer the advantages of lower manufacturing cost/time as they can bind to a single sequence. In contrast, the CRISPR-Cas9 system includes comprises single guide RNA (sgRNA) that recognizes and binds to the target DNA, and a Cas9 nuclease that cleaves the target DNA. The sgRNA consists of CRISPR RNA (crRNA), which is complementary to the target sequence, and a transactivating crRNA (tracrRNA), which facilitates Cas9 binding. The Cas9 nuclease identifies and cleaves double-stranded DNA in a 3 bp sequence known as the protospacer adjacent motif (PAM). Thus, the CRISPR system provides a more accessible and modifiable approach, attributed to the utilization of RNA–DNA base pairing for target recognition, in contrast to the protein–DNA interactions employed by ZFNs and TALENs.
2.3.2.2. Electroporation for CAR-T cell production.
Electroporation (EP) is the most widely used non-viral transfection method, offering acceptable delivery–transfection efficiency with a simple operation. First introduced in 1982, electroporation is a physical transfection method that employs an electrical field to disrupt the cell membrane,108,109 allowing internalization of cargo. By applying external pulsed electric fields with magnitudes in the range of several kV cm−1, a potential difference is created across the cell membrane. When this potential difference reaches a threshold voltage, the transmembrane potential difference leads to the formation of membrane defects, known as hydrophobic pores, on the lipid bilayer. These hydrophobic pores, filled with water, function as capacitors and cause the phospholipid heads inside the bilayer to become hydrophilic. Ultimately, the hydrophilic pores formed by the external pulsed electric field facilitates the introduction of biomolecules (e.g., DNAs, mRNAs, and nanoparticles) through electrophoretic migration. To achieve successful intracellular delivery via electroporation, the magnitude, duration, and number of external electric field pulses are critical parameters that require optimization based on cell type and cargo properties.
This and the following subsections introduce the utilization of conventional cuvette electroporation for transfection of CAR-T cells with transient and integrated cargos (Fig. 8a). Although cuvette electroporation has significantly contributed to CAR-T cell production, certain challenges remain. Therefore, we first discuss the current landscape of cuvette electroporation-based CAR-T cell therapy and its limitations. Subsequently, we highlight the advancements in microfluidics that led to the development of microfluidic electroporation techniques (Fig. 8b), such as capillary electroporation (Fig. 8b-(1)) and flow electroporation (Fig. 8b-(2)).
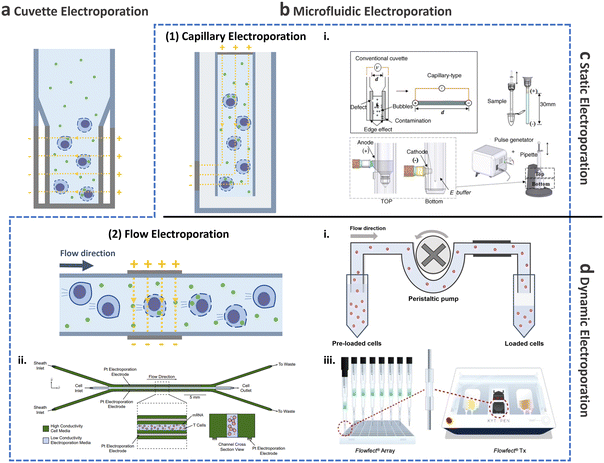 |
| Fig. 8 Electroporation for T cell transfection. Schematic of electroporation: (a) cuvette and (b) microfluidic electroporation. Microfluidic electroporation is divided into (1) capillary electroporation and (2) flow electroporation. (1)-i Capillary electroporation. Reprinted from ref. 125, Copyright 2008 with Elsevier. (2)-i Flow electroporation. Redrawn from ref. 130. (2)-ii Flow electroporation with high conductivity sheath flow. Reproduced from ref. 134 (CC BY). (2)-iii Electro-mechanical cell transfection. Reproduced from ref. 136 (CC BY-NC-ND 4.0). Electroporation can be further divided into (c) static electroporation and (d) dynamic electroporation. | |
2.3.2.2.1. Cuvette electroporation for CAR-T cell production.
Transient CAR-T cell production via cuvette electroporation.
In 2010, Carl June's research group reported a study demonstrating the use of RNA-electroporated CAR-T cells, which alleviated safety concerns associated with integrating viruses.110 The in vitro transcribed CAR-RNA with the optimized vector backbone was transfected into expanded T cells using cuvette electroporation (BTX, USA); although, specific details regarding pulse magnitude and duration were not disclosed. The transfection of CD19-BBz (4-1BB + CD3ζ) CAR-RNA resulted in 91.14% efficiency using 10 μg of in vitro transcribed RNA per 100 μL of T cells. Furthermore, the electroporated CAR-T cells exhibited enhanced functionality, as evidenced by the increased secretion of IL-2 and surface translocation of CD107a following co-culture with K562-CD19 cells.
To evaluate in vivo efficacy, researchers conducted experiments using mesothelin (ss1)-specific CARs in a mouse model (M108-Luc). Mice injected with ss1-specific CAR-T cells demonstrated median survival that was prolonged by 50% compared with those of the control group. Additionally, in vivo testing was performed using CD19-specific CAR-T cells.111 The expression of CD19-specific CAR on cytotoxic T cells transfected with the optimized mRNA persisted until day 6 and gradually declined toward baseline levels by day 10, indicating sustained expression. In a xenograft model of acute lymphocytic leukemia (ALL), mice inoculated with ALL cells were treated with 2.5 × 107 CD19 CAR-T cells on day 7, which resulted in a 2
log reduction in the bioluminescent tumor signal on day 8. Notably, the median survival within 80 days did not differ between lentivirus transduced and the RNA-transfected CAR-T cells.
The first clinical trial via electroporation involving non-viral mRNA CD19 CAR-T cells was conducted in patients with Hodgkin's lymphoma under trials NCT02277522 (adult) and NCT02624258 (pediatric).112 Of the five enrolled patients, CAR transfection rates greater than 99% were observed in T cells from four patients. Moreover, three of these patients survived after receiving six doses of the treatment.
In recent studies, plasmid DNA transfection has been used as an alternative approach for CAR-T cell production, which provides a prolonged duration of ex vivo expression compared with mRNA transfection.113 The efficiency of CD19-BBz plasmid DNA transfection was 49.4% with an optimized single pulse of 500 V for 20 ms with 2 × 106 cells per reaction.
The utilization of transient CAR-T cells through the electrotransfection of mRNA or plasmid DNA presents a promising solution to address the safety concerns associated with viral transduction, including achieving high CAR transfection efficiencies. However, the viability of T cells following transfection was observed to be 50–80%,111,113 which is substantially low considering the clinical dose of CAR-T cells. Consequently, it is necessary to explore alternative intracellular delivery strategies that can meet the requirements of improved viability while maintaining T-cell functionality, in addition to achieving high CAR transfection efficiency.
Integrated CAR-T cell production via cuvette electroporation.
In addition to FDA-approved CAR-T cell therapy, the utilization of integrated SB system-derived CD19-CAR-T cells via cuvette electroporation has been reported.114 To achieve this, a batch of 1 × 107 PBMCs and umbilical cord blood mononuclear cells (CBMCs) were electroporated using a Nucleofector (Lonza, Switzerland) with 5 μg of second-generation CAR (CD19RCD28) encoding transposon DNA plasmids and 5 μg of pCMV-SB11 DNA plasmids. In vitro testing demonstrated 50% and 60% lysis of CD19-positive Daudi cells and CD19-positive K562 cells, respectively. Notably, no significant SB11 transposase insertions were observed in the propagated T cells.
To enable clinical scale electro-transfection with improved transfection efficiency and T cell viability, mini-circles (MCs) encoding CD19-CAR and SB100X transposase were delivered to CD8+ T cells stimulated with CD3/CD28 on day 2.115 Compared with plasmids, the combination of SB100X mRNA and MCs achieved a 3.7-fold higher gene transfer rate, with T cell viability 48 h after transfection being 1.4-fold higher. These findings demonstrated significant advancements in non-viral integrated CAR-T cells, leading to tumor clearance by day 14 in an in vivo lymphoma xenograft model. However, it should be noted that the viability of the transfected T cells remained low at approximately 50%.
Simultaneously, efforts have been made to optimize the PB transposon system for T cells.116 A notable challenge associated with electro-transfected PB transposons is the substantial loss of viability. To address this, the addition of IL-15 before and after transfection was investigated, and a notable enhancement in cell viability was observed. Specifically, electroporation of 5 × 106 PBMCs with 5 μg of GFP-encoded PB transposon, along with varying concentrations of PB transposase, resulted in improved viability of 60% a day after electroporation.
It is worthwhile to mention, cuvette electroporation has facilitated the generation of integrated CAR-T cells that express CARs in long-term, providing a durable therapeutic alternative to viral transduction; however, the challenge of low viability in transfected T cells persists.
Allogeneic CAR-T cell production via cuvette electroporation.
Toward off-the-shelf cell-based therapy, allogeneic CAR-T cells were prepared via electroporation. In the context of universal T cell-based immunotherapy, two of the four ZFN mRNA molecules targeting the TCRα constant (TRAC) gene and TCRβ constant (TRBC) gene were delivered to CAR-positive T cells.117 The CAR-T cells were initially generated by a Nucleofector using 2 × 107 PBMCs/cuvette, wherein plasmids encoding the SB transposon CD19RCD28 and SB 11 transposase were transfected. Following 2–4 days of expansion, ZFN mRNA molecules were internalized into 5 × 106 T cells using a Nucleofector. Unlike other studies on allogeneic CAR-T cells, electroporation was employed for both CAR transfection and T cell receptor (TCR) gene knockout (KO). Both TRAC CD3-negative and TRBC CD3-negative CD19-CAR-T cells exhibited >40% cell lysis when exposed to CD19-positive EL4 cells; however, no significant effect was observed for CD19-negative cells.
In another study, TALEN mRNA was utilized for highly efficient multiplex gene editing in primary human T cells through electroporation, resulting in deficiencies in TRAC and CD52, a protein targeted by alemtuzumab.118 In an in vivo experiment using human CD19-positive lymphoma xenograft models, five of the seven mice injected with TRAC and CD52-KO CD19-negative CAR-T cells exhibited complete tumor control by day 13.
In addition to ZFN and TALEN, the CRISPR-Cas9 system enables the simultaneous disruption of two or three target genes, leading to enhanced therapeutic efficiency.119 To mitigate GvHD and minimize immunogenicity, it is necessary to eliminate not only TRAC but also human leukocyte antigen class I (HLA-I) from CAR-T cells.120 additionally, blockage of programmed death-1 (PD-1) signaling has been considered to reverse immunosuppression.121 Triple-knockout T cells exhibit a predominantly mutant phenotype, with the complete absence of beta-2 microglobulin (B2M) expression in 100% of the cells, 85% reduction in TRAC expression, and 64.7% decrease in PD-1 expression. Furthermore, triple-negative CAR-T cells demonstrate a high rate of tumor cell lysis, reaching approximately 60%. Despite high tumor cell killing ability of allogeneic CAR-T cells, the viability of cuvette electroporation remains a critical challenge. Notably, the fold expansion over a 10-day period following electroporation was substantially lower, approximately 5-fold, compared with that of the control group across three different donors.
In addition to lower T cell viability after cuvette electroporation, optimal sgRNA should be considered to achieve better transfection efficiency and therapeutic effect. As there are numerous potential sgRNAs targeting TRAC, TRBC, CD52, B2M, and other genes within the exon sequences, it is critical to screen for highly efficient sgRNAs and optimize the ratio of sgRNA to Cas9 protein. The screening process should involve the use of minimal cell and cargo volumes, making microfluidics-based electroporation techniques such as capillary electroporation preferable.
2.3.2.2.2. Microfluidic electroporation for CAR-T cell production.
Capillary electroporation.
As mentioned previously, conventional cuvette electroporation results in low viability and is not ideal for clinical applications.122 To address this limitation, microfluidic approaches have been explored to improve cell viability, delivery, and transfection efficiency.123,124 In 2008, Kim et al. introduced a novel electroporation technique that utilizes capillary and wire-type electrodes to increase cell viability (Fig. 8b-(2)i).125 This capillary electroporation system features increased electrode separation, ensuring high electrical resistance and minimal current flow, which in turn leads to a reduction in chemical reactions. Therefore, poor T cell viability post-engineering, which is the main limitation of cuvette electroporation, can be improved by capillary electroporation. Using capillary electroporation, a substantial improvement over conventional cuvette electroporation has been achieved. For example, an 80% EGFP plasmid transfection rate has been demonstrated for HeLa and COS-7 cell lines, with viability of 70–80%. Attempts has also been made to apply capillary electroporation for the direct transfection of flask-attached adherent cells.126–128 These technologies use an electrolyte-filled capillary with cargo, and they have demonstrated approximately 100% transfection for 4.6 kbp YOYO-stained plasmids while maintaining high cell viability after 24 h, which was comparable to that in the control group. It should be noted that this microfluidics-based work has been successfully commercialized as a product (Neon Transfection System; Thermo-Fisher, USA) and has been widely adopted by numerous researchers.
CCR5-negative CD19-CAR-T cells were subjected to homologous recombination (HDR) using the Neon Transfection System.129 The procedure involved electroporating expanded T cells with megaTAL nuclease mRNA, followed by lentiviral transduction to introduce CD19-CAR flanked by CCR5. By rendering T cells CCR5-negative, they become resistant to HIV-I infection, enabling CCR5-negative CD19-CAR-T cells to target HIV-associated B cell malignancies. In an in vitro CD19-K562 cell killing assay, CCR5-negative CD19-CAR-T cells exhibited comparable cell lysis rates with lentiviral CD19-CAR-T cells, as evidenced by CD107a and CD137 expression rates. The median survival time in the murine in vivo test was 38.5 days for lentiviral CD19-CAR-T cells and 42 days for CCR5-negative CD19-CAR-T cells. However, it is important to acknowledge that the throughput of capillary electroporation, approximately 2.5 × 105 cells per batch (run) of primary T cells, remains significantly limited, which is a fundamental drawback of this method.
Flow electroporation.
Despite the potential of capillary electroporation to address the low cell viability associated with cuvette electroporation, achieving high scalability (>1 × 108 cells per treatment) for clinical applications remains challenging. There has thus been growing interest in flow electroporation methods using microfluidics, which offer high-throughput capabilities.
The initial demonstration of continuous-flow electroporation technology with proven in vivo efficacy dates back to 2002 (Fig. 8b-(2)i).130 The system comprised a power/switch module and disposable flow pulsing chamber. Cells preloaded into the system were driven through the pulsing chamber that contained two parallel gold-coated stainless-steel electrodes, using a peristaltic pump. The mean cell velocity was 0.5 cm s−1, while the electrical fields were optimized to 1–2.3 kV cm−1, depending on the cell type, to achieve optimized efficiencies exceeding 70%. For instance, when 2.5 × 108 Jurkat cells suspended in 5 mL of electroporation buffer mixed with 500 kDa FITC dextran (0.5 mg mL−1) were processed in the system, delivery efficiency exceeding 90% and viability of approximately 90% was achieved. Subsequently, the system was scaled-up to process 50 mL of sample in 9 min treatment, resulting in delivery efficiency of approximately 60% and viability of approximately 80%.
To assess its potential for clinical scale applications, the disruption of the PD-1 gene using ZFN was conducted for the adoptive transfer of autologous tumor-infiltrating lymphocytes (TILs).131 On day 7 of expansion, TILs suspended in electroporation buffer at a concentration of 1 × 108 cells per mL were electroporated with 120 μg mL−1 of PD-1 ZFN mRNA. Targeting the PD-1 gene through ZFN resulted in a 75% disruption rate, as determined by the Cel-1 assay, and deep sequencing revealed biallelic disruption of 44%. Importantly, in vivo engraftment of PD-1 gene-edited T cells did not result in proliferative abnormalities or tumor formation during necropsy, indicating successful and safe editing of the target gene using flow electroporation.
In another study involving TILs, NY-ESO-1 TCR-transduced TCR-T cells were genetically edited using CRISPR-Cas9 RNP electroporation, which showed promising results in cancer treatment.132 NY-ESO-1 represents an antigen found in human refractory cancers. In vitro co-culture experiments with HLA-A2-positive tumor cells demonstrated that the edited NY-ESO-1 TCR-T cells exhibited approximately 80% specific cell lysis, surpassing the lysis rate of NY-ESO-1 TCR-T cells without gene editing (∼40%). Furthermore, an in vivo assessment conducted four months after the infusion of genetically edited NY-ESO-1 TCR-T cells in human subjects revealed 50% regression of a large abdominal mass, as observed by CT.
In conjunction with the success of TCR-T cells, CAR-T cell production has also been enabled via flow electroporation. Furthermore, as early flow electroporation technologies were carefully refined, a cGMP-compatible manufacturing process was established to generate highly efficient CAR-T cells.133 To minimize the cytotoxicity associated with the double-stranded template, a single-stranded HDR template incorporating the Cas9 target sequence (CTS) was employed to enhance knock-in (KI) efficiency. Expanded T cells (2 × 108 cells per mL) obtained from healthy donors were electroporated with single-stranded CTS HDR templates encoding Bcell maturation antigen (BCMA)-CAR and CRISPR RNPs targeting TRAC. The average KI efficiencies were 40.4% and 45.8% on days 7 and 10, respectively. In an in vitro killing assay using MM1s myeloma cells, BCMA-CAR-T cells exhibited target cell killing ability exceeding 90%.
Another microfluidic integration was demonstrated to enhance cell processing for flow electroporation. The microfluidic device consisted of a sample inlet for introducing T cells into low-conductivity electroporation media and two sheath inlets for high-conductivity cell culture media (Fig. 8b-(2)ii).134 Using this device, 5 × 108 T cells were processed within 25 min with 50 μg mL−1 mCherry-encoding mRNA, resulting in approximately 75% transfection efficiency and 91% cell viability. These recent advancements through microfluidics offer a potential to meet the clinical dose requirements for both autologous and allogenic therapies, delivering high transfection efficiency and viability.
Other microfluidic electroporation methods.
Owing to the inherent advantages of microfluidics, such as their simplicity of operation,135 the integration of a microfluidic electroporation device with other transfection–delivery techniques has become feasible. In a recent study by Sido et al., an electromechanical cell transfection system was developed in which suspended cells were directed through a flow cell cartridge. Within this system, the cells experience an electric field generated by electrodes positioned across the flow cell, as well as mechanical shear stress by high fluid flow (Fig. 8b-(2)iii).136 In contrast to conventional methods, such as cuvette electroporation, which result in the upregulation of proinflammatory cytokines, activation receptors, and exhaustion markers, leading to the upregulation of cytotoxic T-lymphocyte-associated protein (CTLA), transcriptional profiling revealed that electromechanically transfected cells did not exhibit any discernible changes. For potential clinical applications, a transfection experiment was conducted wherein 5 × 107 T cells were transfected with 1 mg of GFP mRNA, showing 94.3% transfection efficiency and 73.5% viability.
As previously mentioned, maintaining T cell function after transfection is crucial for clinically viable CAR-T cells. The newly introduced magnetic nano-electro-injection (MagNEI) platform was utilized to examine the biological attributes of T cells after transfection.91 In this platform, mechanical stimulation using magnetic modulation and nanoscale straw-like structures with electro-injection were adopted for EGFP-encoded plasmid DNA transfection into primary T cells, yielding delivery efficiency of 56% and a viability rate of 90%. Compared with virus-transduced and bulk electroporated primary T cells, MagNEI-transfected T cells showed a higher expansion rate for 2 weeks, lower cytokine release syndrome, and improved membrane repair after transfection.
In summary, as an alternative to viral transduction, the field of electroporation technology has evolved from cuvette electroporation to flow and microfluidic electroporation. Additionally, the issue of low T cell viability observed after cuvette electroporation111,113,115 has been addressed with capillary electroporation.125 To meet clinical dose requirements for CAR-T cells, microfluidics-based flow electroporation has been extensively investigated134 and is now commercially available. Despite these significant contributions of electroporation to CAR-T cell therapy, certain concerns persist. For instance, irreversible double-stranded breaks in T-cell DNA have been observed following electroporation,122 which is suboptimal, particularly for genome editing-based cell therapies. Furthermore, undesired changes in T cell functionality and perturbations in gene expression have been confirmed after electroporation treatment.137 The subsequent section introduces non-electrical stimulation methods as an alternative approach to enhance CAR-T cell production.
2.3.2.3. Lipid nanoparticles for CAR-T cell production.
Since the discovery of liposomes, an early version of lipid nanoparticles (LNPs),138 LNPs have been recognized as protective nanocarriers that encapsulate exogenous cargos for in vivo and ex vivo delivery in cancer therapy.139 LNPs facilitate effective and stable delivery by protecting encapsulated nucleic acid against degradation and promoting cellular uptake and controlled release.140,141 Notably, LNPs have gained approval from the FDA for encapsulating anticancer drugs, such as doxorubicin, amphotericin B, and daunorubicin. Moreover, Onpattro LNP, which encapsulates siRNA, was approved by the FDA and European Medicines Agency (EMA) in 2018.142 LNPs are being explored for not only cancer therapy but also the development of mRNA vaccines, which are currently undergoing clinical trials.143 In the context of ex vivo intracellular delivery for CAR-T cell production, LNPs offer advantages over viral transduction and electroporation, possibly addressing limitations related to cargo size, low cell viability, and cytotoxicity.96
LNPs can be broadly classified into two groups: cationic and ionizable lipids.144 Cationic lipids possess positively charged head groups, whereas ionizable LNPs are neutral at physiological pH. Neutral ionizable LNPs are advantageous because they exhibit minimal interaction with the anionic membrane of blood cells, thereby enhancing biocompatibility.145 In addition, under acidic conditions within endosomes, ionizable LNPs acquire a positive charge, enabling efficient endosomal escape by destabilizing the endosomal membrane.146
2.3.2.3.1. Microfluidic approach for LNP formation.
The scalability of CAR-T cell production using lipofection is influenced by the efficiency of LNP formation. Conventional methods for LNP synthesis, such as extrusion and sonication, have low-throughput, and need large sample volume. To overcome these limitations, microfluidic techniques have been introduced,147–149 which enable high-throughput processing and precise control of LNP production.
To achieve homogeneous LNP formation in continuous flow, a microfluidic mixing technique was implemented (Fig. 9a).147 The microchannel was designed with asymmetrically arranged periodic trenches and ridges (known as a chaotic mixer) made of polydimethylsiloxane (PDMS) for efficient mixing. First, an aqueous solution containing siRNA or mRNA was rapidly mixed with ethanol. Owing to the charge interaction between the cargo and lipids, the solubility of lipids decreases in the presence of water, facilitating the self-assembly of LNPs. By increasing the flow rates, the diameter of the formed LNPs decreased to approximately 70 nm at a flow rate of 200 μL min−1, which was much smaller, and more uniform compared to LNPs produced through pipette-based mixing (approximately 180 nm).
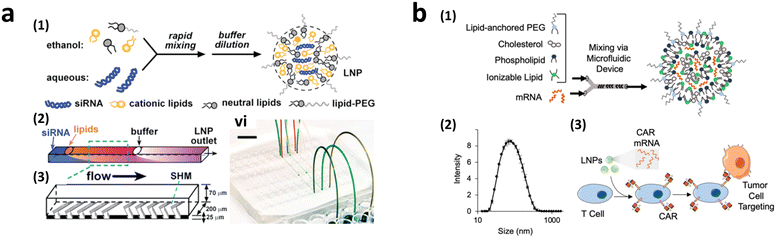 |
| Fig. 9 CAR-T cell production via lipofection. (a) Microfluidic formulation of siRNA-containing lipid nanoparticles. Reprinted with permission from ref. 147. Copyright 2012 American Chemical Society. (b) Ionizable lipid nanoparticle-mediated mRNA delivery via microfluidic mixing. Reprinted with permission from ref. 150. Copyright 2020 American Chemical Society. | |
2.3.2.3.2. Transient CAR-T cell production via LNP endocytosis.
Research on LNP for CAR-T cell production powered by microfluidics initially focused on mRNA transfection, highlighting the benefits of transient CAR expression in reducing the side effects associated with continuous CAR expression.110 The concept of LNP-delivered mRNA-CAR-T cell delivery was first reported in 2020 (Fig. 9b).150 Various ionizable LNPs incorporating lipids, such as dioleoyl phosphatidylethanolamine (DOPE), cholesterol, and lipid-anchored polyethylene glycol (PEG), were evaluated. The optimized LNPs formed via a microfluidic device demonstrated the highest luciferase expression rate following the transfection of luciferase mRNA into Jurkat cells (30 ng of mRNA/60
000 cells). Subsequently, purified LNPs with a diameter of approximately 70 nm were used to transfect CD19-CAR mRNA into expanded primary T cells. Based on in vitro Nalm6 ALL cell killing assay, CAR-T cells transfected with LNPs exhibited comparable killing efficacy while maintaining higher viability (76%) than CAR-T cells transfected by electroporation (31%). Follow-up studies were performed with the aim of optimizing the molar ratio of ionizable LNPs for CAR-T cell production.151 In addition, various cationic lipids produced using a microfluidic device were screened through LNP-mediated luciferase or CAR mRNA transfection efficiency.152 Primary T cells and macrophages were transfected with cationic lipids encapsulating CAR mRNA. Among the tested LNPs, 76-O17Se successfully transduced 22.6% of expanded CD8-positive T cells with N1-methlypseudouridine-anti-CD19-eGFP. An in vitro cell killing assay demonstrated a 74.53% reduction in the luminescence levels of luciferase-engineered B lymphoma. Moreover, bone marrow-derived macrophages and proinflammatory M1 macrophages were transfected with N1-methlypseudouridine-anti-CD19-eGFP for next-generation CAR, resulting in 32.54% and 22.5% reductions in luminescence levels of luciferase-engineered B lymphoma, respectively. Despite the relatively low mRNA concentration compared with electroporation, transient CAR-T cells transfected via lipofection with LNP formed in the microfluidic platform demonstrated comparable tumor killing ability with higher viability and preserved cell functionality. Nonetheless, it is evident that the efficacy of CAR-Tcell treatment strongly relies on the composition of LNPs. Identifying the optimal combination of major LNP components and target cargos remains challenging, as it involves numerous time-consuming and expensive trial-and-error experiments.153 Therefore, it is anticipated that the development of a high-throughput screening system through microfluidics could have a substantial impact on LNP-based CAR-T cell research.
2.3.2.3.3. Allogeneic TCR- or CAR-T cell production via LNP endocytosis.
In contrast to autologous transient CAR-T cells, the application of LNPs to Wilms' tumor gene 1 (WT1) TCR-T cells was proposed in 2021, specifically for NTLA-5001 (NCT05066165). LNPs formed in a microfluidic benchtop instrument154 have been used to encapsulate TRAC or TRBC gRNA and Cas9 mRNA, resulting in editing efficiency of up to 95% with increasing LNP concentration. Moreover, the relative cell viability was maintained at over 90%. Comparative analysis with electroporation revealed that post-edit expansion of LNP-based edited mice was significantly higher (p < 0.01), accompanied by a favorable 67.4% CD45RA+ CD27+ memory phenotype. In addition, LNP-based edited T cells demonstrated substantial secretion of IL-2 and IFN-γ in response to WT1-presenting tumor cell lines during expansion. To evaluate in vivo efficacy, 1 × 107 WT1-specific TCR-T cells were intravenously (IV) injected to an acute myeloid leukemia (AML) mouse model on days 0 and 20. Blood test results indicated 60% AML cells, whereas LNP-CRISPR-Cas9-edited-TCR-T-cell-injected mice showed only 10% AML cells. Based on these promising clinical outcomes, NTLA-5001 became the first LNP-CRISPR/Cas9-edited T cell therapy utilized in a first-in-human clinical study. Furthermore, the high efficiency of multiplex gene editing has been demonstrated using CRISPR-Cas9-encapsulated LNPs in allogeneic T cells. Sequential KO of HLA-Class II, Receptor X, TRAC, and TRBC achieved approximately 80% editing efficiency, along with 80% insertion efficiency in WT1-TCR-T cells.
Despite ongoing clinical trials involving LNPs encapsulating the multi-genome-targeted CRISPR-Cas9 system for allogeneic CAR-T cell production, viral vectors remain the current choice for CAR transduction. However, LNPs have been recognized for their superior biocompatibility compared with viral vectors or cuvette electroporation, making them a promising avenue for future research on CAR-T cell production. Moreover, with a growing focus on off-the-shelf allogeneic CAR-T cells, there is a need to develop LNPs capable of encapsulating large cargos with high transfection efficiency, emphasizing the importance of further studies in this area. To accelerate advancements in this research area, the use of microfluidic technologies to produce highly uniform LNPs with high scalability is crucial. Consequently, there has been significant growth in the field of microfluidics-based LNP research.155
2.3.2.4. Microfluidic membrane disruption-mediated approaches for CAR-T cell production.
Numerous membrane disruption-mediated non-viral delivery methods have been investigated as alternative. Mechanical permeabilization of the cell membrane (mechanoporation) can be accomplished through various mechanisms, including nanowires,156 scrape loading,157 fluid shear induced by sonoporation158 and laser-induced cavitation bubbles,159 and flowing cells through microconstrictions.160
Microfluidic mechanoporation technologies for T cell transfection have attracted considerable attention as potential alternatives to conventional viral transduction for T cell transfection.89 Notably, these technologies are highly applicable to primary immune cells.161 Achieving highly efficient transfection of T cells holds great significance, as it is essential for generating a sufficient number of potent CAR-T cells, which are critical for favorable treatment outcomes.61 Another advantage of microfluidic mechanoporation lies in the simplicity of the workflow, as it enables a sample-in-and-sample-out system to be realized. Furthermore, microfluidic transfection allows for continuous processing; that is, high scalability, potentially reducing the time required for viral transduction, which typically takes a minimum of 2 weeks for batch manufacture.7 In addition, owing of its operational simplicity, microfluidic transfection can be easily integrated with other transfection techniques such as electroporation,162 as well as other microfluidic modules for the sorting, isolation, and activation of T cells. This integration opens up the possibility for a fully automated, centrifuge-free CAR-T cell therapy manufacturing process, as discussed later in this review. Moreover, microfluidic transfection platforms are compatible with gene editing technologies, such as CRISPR-Cas9, allowing the development of off-the-shelf allogeneic CAR-T cells that require deletion of the TCR gene to avoid allorejection.100
General microfluidic mechanoporation-based intracellular delivery relies on the following sequence: cell deformation to create discontinuities in the membrane, internalization of exogenous cargos across the lipid bilayer, and recovery of the plasma membrane. Various microfluidic techniques have been adopted using this underlying mechanism, including cell squeezing,70,160,163–168 hydroporation,169–171 and vortex shedding.171,172
In 2013, Sharei et al. pioneered the use of cell squeezing via microfluidic channel constriction as a new intracellular delivery method for the internalization of diverse macromolecules into various cell types (Fig. 10a).160 This technique induces membrane disruption, leading to the formation of membrane discontinuities through which the cargos diffuse into the cells. Subsequent studies have demonstrated the efficacy of the cell squeezing method in delivering Janus kinase (JAK) inhibitors to human PBMCs,163 resulting in minimal aberrant transcriptional responses (<10-fold increase in proinflammatory cytokines) compared with electroporation (200-fold increase in interferon-γ (IFN-γ)).70 Researchers have also explored variations in constriction geometries, such as the implementation of parallel arrays of diamond microconstrictions. This approach achieved over 70% efficiency in EGFP KO SU-DHL-1 lymphoma cells using parallel arrays of diamond microconstrictions (Fig. 10b).164 Furthermore, sharp star-shaped constrictions demonstrated approximately 33% efficiency in delivering cargo into primary human T cells.167
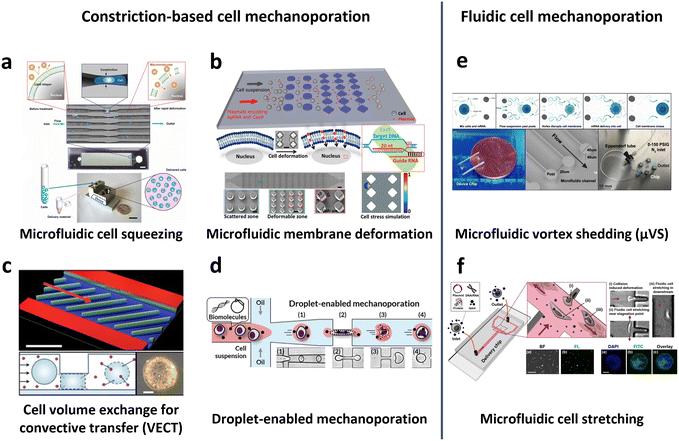 |
| Fig. 10 Microfluidic technologies for cell membrane disruption-mediated transfection of T cells. (a) Microfluidic cell squeezing for intracellular delivery. Reproduced from ref. 160. Copyright 2013 National Academy of Sciences. (b) Diamond-shaped pillar micro-constriction arrays for squeezing hard-to-transfect cells. Reproduced from ref. 164 (CC BY-NC 4.0). (c) Volume exchange for convective transfer (VECT) for rapid and repeated compression of cells for efficient intracellular delivery. Reprinted from ref. 166, Copyright 2018 with Elsevier. (d) Droplet-encapsulated cell squeezing for intracellular delivery of primary human T cells. Reprinted with permission from ref. 165. Copyright 2021 American Chemical Society. (e) Microfluidic vortex shedding (μVS) consisting of dense arrays of posts for cell deformation. Reproduced from ref. 172 (CC BY). (f) Microfluidic cell stretching at a T-junction microchannel with a cavity for elongational recirculating flow-mediated intracellular delivery. Reprinted with permission from ref. 169. Copyright 2020 American Chemical Society. | |
While the previously mentioned cell squeezing platforms enable the diffusion of biomaterials into the cytoplasm, alternative platforms utilize convective volume exchange to facilitate efficient delivery regardless of the cargo size. Recently, Liu et al. demonstrated the use of convectively driven delivery through transient cell volume exchange, which exhibited a 30% response to cell deformation (Fig. 10c).166 This approach achieved approximately 70% efficiency in mRNA delivery to primary T cells without adversely affecting T cell function, in terms of cell expansion and exhaustion. More recently, droplet-based cell squeezing has been reported to achieve approximately 90% mRNA transfection efficiency, with over 82% cell viability in primary human T cells (Fig. 10d).165 Interestingly, this approach requires only one-sixth of the cargo to achieve the same concentration of exogenous cargo in medium as cell squeezing, demonstrating a larger reduction in analyte consumption, i.e., cost. Furthermore, the transfection of plasmid DNA was demonstrated by leveraging droplet microfluidics, which was unachievable through conventional cell squeezing.
Microfluidic strategies for cell deformation have evolved beyond microconstrictions and now encompass techniques such as microfluidic vortex shedding (μVS) in densely arranged arrays of posts.172 This mechanism offers high-throughput (>2 × 106 cells per s) and highly efficient (∼63.6%) delivery of EGFP mRNA into primary human T cells while maintaining high cell viability (∼77.3%) and cell recovery (∼88.7%) without compromising T cell growth and expression profiles (Fig. 10e). As another representative approach, Hur et al. reported on a microfluidic device featuring a T-junction with a cavity that induces elongational recirculating flows, resulting in cell stretching and discontinuities in membrane formation (Fig. 10f).169 This approach enables high-throughput (>1 × 106 cells per min) and robust delivery of large cargos of up to 300 nm in size across cell types. It demonstrated 80% efficiency and 87% viability upon transfection of plasmid DNA into the K562 leukemic cell line. Moreover, it achieved highly efficient mRNA transfection in hard-to-transfect primary cells, such as human adipose-derived stem cells (ADSCs), Wharton's jelly human umbilical cord mesenchymal stem cells (WJ-MSCs), and murine bone marrow dendritic cells (BMDCs).
Notably, microfluidic transfection encounters several challenges in clinical translation despite its simple operation, low invasiveness, and high scalability. One primary challenge is the susceptibility of microfluidic channels to clogging, which is attributed to the presence of dust particles, cell clusters, and protein adsorption.173 Such clogging events can lead to lower delivery efficiency, cell loss, and cell death. To address this issue, several strategies have been explored in microfluidic systems, including the use of a parallel array of interconnected microchannels,164 droplet compartmentalization to inhibit nonspecific protein adsorption,165 cross-junction design without a constrictions,170 and post-arrays designed with spacing larger than the cell sizes.172 Another challenge that remains unresolved is the potential damage to the cytoskeleton and nucleus during the process, which can result in transient nuclear ruptures and DNA damage;174 thus, further investigations are necessary to assess the occurrence of off-target DNA damage across cell types.
2.4. Post-processing of CAR-T cells before injection
To achieve the required clinical dose, genetically transferred CAR-T cells undergo an additional expansion process, as mentioned previously. Following T cell isolation and activation, CAR-T cells can be expanded by co-incubation with anti-CD3/CD28 antibodies, anti-CD3/CD28 beads/nanoparticles, or artificial APCs. Furthermore, cytokines can be added to enhance enrichment.51 Subsequently, expanded CAR-T cells can be cryopreserved for transportation to clinical institutes. Previous investigations have shown that conventional cryopreservation of CAR-T cells with dimethyl sulfoxide (DMSO) in a controlled-rate freezer does not adversely affect the therapeutic effects or phenotype of CAR-T cells.175 However, the molecular-level modification that occurs in cryopreserved CAR-T cells after thawing remains unclear. Consequently, microfluidic approaches present an opportunity to develop novel tools for functional assays of cryopreserved CAR-T cells or to explore alternative cryopreservation methods that improve the production of effective CAR-T cells.
2.5. Fully automated cGMP CAR-T cell production
Fully automated cGMP technologies have been developed for CAR-T cell production, allowing integration of the aforementioned major processes. Cocoon platform and CliniMACS prodigy system are well-established cGMP technologies for the production of TCR-T cells and CAR-T cells. These platforms utilize viral transduction and electroporation. Starting from the leukapheresis product, T-cell separation, gene engineering, and subsequent expansion were conducted within a closed automated system. An average of 47.6 ± 14.9% of T cells were successfully lentiviral transduced with CAR. Starting with an initial cell count of 0.7–1.2 × 108 cells, the T cell population expanded to 1.58 × 109 cells within 9 days after transduction. In vivo testing demonstrated tumor clearance in three out of five mouse xenograft models.34 Despite notable advancements in automated CAR-T cell production and positive clinical outcomes with these CAR-T cells, there is still room for further improvement, particularly in CAR expression efficiency, cell viability, expansion rate, and cell functionality. As discussed in previous sections, microfluidic technology can be applied to enhance each step of CAR-T cell production, ultimately leading to more effective, safe (non-viral), and affordable CAR-T cell products. Moreover, microfluidics can be integrated into closed automated cGMP platforms to capitalize on their advantages and facilitate efficient and cost-effective CAR-T cell production.
3. Future of adoptive cell immunotherapy through microfluidic solutions
Herein we present an overview of the prospects of adoptive cell immunotherapies and the application of microfluidic approaches beyond the current individual steps of CAR-T cell production. The discussion specifically focuses on microfluidic solutions for immunotherapy, including single-cell-based evaluation and the microenvironment (TME) at the tissue or organ scale. Additionally, the advancements in next-generation CAR/TCR and allogeneic off-the-shelf immunotherapy will be explored (Fig. 2 and Table 1). Through comprehensive exploration, this section aims to provide insights into how microfluidics and microfluidics-based technologies can enhance and enrich current cell-based immunotherapy technologies.
Table 1 Microfluidic approaches for next-generation CAR-T/NK cell-based immunotherapy
|
Droplet microfluidics |
Static well plate and microwells |
Microfluidic tumor-on-a-chip |
Others |
Cell–cell interaction evaluation |
Droplet microfluidic chip for CAR-T cell cytotoxicity assessment189,190–197 |
Microfluidic cell trapping for cell interaction studies54 |
3D microfluidic cancer spheroid co-culture models for assessment of CAR-T cell cytotoxicity186 |
|
Made-to-order droplet ensembles for characterizing cell interactions188 |
Size difference-based hierarchical loading chip185 |
Droplet digital PCR for DNA copy number quantification194–197 |
Lymphocyte interaction monitoring via microfluidic cell pairing183,184 |
3D cell culture for tumor microenvironment characterization |
— |
Spheroid killing assay by CAR-T cells228–230 |
Microfluidic tumor model simulating hypoxia for evaluating CAR-T cell therapy241 |
|
Monitoring dynamic tumor cell–immune cell interaction236 |
Comparison of tumor models in 2D and 3D spheroid culture231 |
Tumor-on-a-chip for tumor cell extravasation, T cell migration, and cytotoxicity247 |
3D hanging spheroid plate for CAR-T cell cytotoxicity assay235 |
Microfluidic migration assay showing T cell recruitment238 |
Patient-derived glioblastoma organoids for CAR-T cell testing234 |
Tumor-on-a-chip to characterize the role of monocytes in TCR-T cell therapy239 |
Multilayered blood vessel/tumor-on-a-chip to investigate T cell infiltration240 |
Next-generation CAR construct/TCR screening and sorting |
Antigen-specific T cell functional screening190,192,248,249 |
— |
— |
|
Downstream droplet-compartmentalized sequencing250–252 |
Droplet microfluidics for integrated functional screening and TCR sequencing for next-generation cancer immunotherapy target discovery253–257 |
Allogeneic CAR development |
Droplet microfluidic platform for the single NK cell heterogeneity assay via killing assay with K562 (ref. 267) |
A microchip with microwells to study the lytic activity of individual NK cells against tumor target cells268 |
Breast cancer cell spheroid in 3D ECM with flanking lumen with vein endothelial cell281 |
Flow electroporation for transfection265,266 |
Size-tunable droplet for study of NK cell heterogeneity against various tumor cells193 |
3D NK cell cytotoxicity assay against HeLa cells282 |
Label-free NK cell subpopulation identification via viscoelastic flow269 |
Droplet microfluidic platforms to analyze the effector outcomes of NK cells against target cells at the single-cell level276–279 |
TME-mimicking 3D spheroid microarray for killing assay against MCF-7 cells283 |
NK cell migration assay in response to dendritic cell285 |
Paper-based microfluidic device for NK cell heterogeneity identification270 |
Micro-spray for formation of NK cell encapsulating microsphere286 |
3.1. Evaluation of CAR-T cell efficacy
The conventional evaluation of CAR-T cell therapy efficacy relies on bulk co-culture of CAR-T cells with cancer cells to facilitate cell–cell interactions (Fig. 11a). Subsequently, conventional measurements are taken to assess T cell cytotoxicity, proliferation kinetics,176 and metabolic profile.177 For the evaluation of CAR expression level, various techniques, such as qPCR, have been utilized to assess the mRNA expression level of CAR, ELISA to measure CAR protein expression, and flow cytometry to quantify CAR expression levels in the bulk population. Although these efficacy tests are robust, cost-effective, and easily implemented in biological laboratory settings, they depend on surrogate indicators of T cell cytotoxicity, which may not accurately reflect therapeutic outcomes. Chromium-51 (51Cr) release assays and xenograft mouse models are also commonly employed as direct indicators of CAR-T cell cytotoxicity.178 Nevertheless, utilization of radioactive chromium and the requirement for expensive and labor-intensive animal testing emphasizes the need for a straightforward, cost-effective, and high-throughput cell–cell interaction platform capable of delivering results that exhibit a strong correlation with therapeutic effects under physiological conditions. Moreover, in bulk co-culture settings, the inherent heterogeneity of immune cell responses is often neglected, as the diverse range of cell–cell interactions is averaged out.
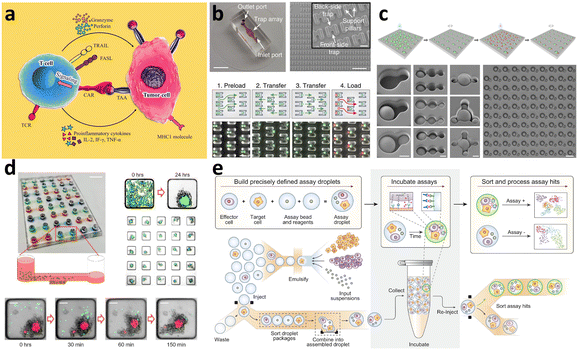 |
| Fig. 11 Microwell-based and microfluidic-based technologies for encapsulating individual cells for cell–cell interaction studies. (a) Schematic of cell interaction between CAR-T cell and tumor cell. Reproduced from ref. 19 (CC BY 4.0). (b) Microfluidic device for hydrodynamic cell trapping for profiling lymphocyte interactions. Reproduced from ref. 54 with permission of Springer. (c) Hierarchical loading microwell chip (HL-chip) for evaluating single-cell cytokine secretion and cell–cell interactions. Reproduced from ref. 185 (CC BY-NC-ND). (d) Microfluidic immunoassay of CAR-T cells and spheroid co-cultures in ultralow-adhesion microwells. Reproduced from ref. 186 (CC BY-NC-ND). (e) Made-to-order droplet ensembles (MODEs) for high-throughput cell interaction profiling. Reproduced from ref. 188 (CC BY). | |
It should be noted that these limitations can be addressed by evaluating CAR-T cells at the single-cell level using techniques such as microwell-based cell trapping and droplet microfluidics. This direction enables the interaction and variations across the population. Thus, microfluidic approaches offer a means of achieving cell compartmentalization, serving as spatial confinement for individual cell analyses.179 The next two sections mainly discuss microfluidics-based cell–cell interactions to assess the efficacy of CAR-T cells. While CAR-T cell efficacy evaluation typically involves tumor cell cytotoxicity assays and cytokine release measurements, efforts have been made to profile cytokines from individual CAR-T cells using microfluidic devices. Using optofluidic technologies, a 16-plex cytokine microfluidic device was used to capture four categories of cytokines (e.g., effector, stimulatory, regulatory, and inflammatory) secreted by single CD19 CAR-T cells labeled with secondary antibodies.180 This approach allowed a deeper understanding of the heterogeneity among activated and non-activated CAR-T cells from four different donors based on fluorescent intensities derived from antibody-captured cytokines. Furthermore, specific subsets of T cells, such as CXCR4-edited T cells via CRISPR/Cas9 transfer, could be housed in a single chamber with optoelectronic tweezers for selected cloning and phenotyping.181,182 In turn, microfluidic approaches can be applied to assess CAR-T cell efficacy at the molecular scale. As previously mentioned, the following sections will discuss microfluidic applications at the cellular scale for CAR-T cell efficacy evaluation.
3.1.1. Microwell-based cell–cell interaction studies.
Microwell-based cell compartmentalization has been extensively employed to investigate immune cell interactions, encompassing cytotoxicity183 and protein secretion.184 Dura et al. developed a microfluidic cell pairing platform that utilizes front- and back-side traps to optimize pairing efficiency with minimal clogging (Fig. 11b).54 With this approach, they achieved cell pairing efficiencies ranging from 40% to 85% in a sample-efficient manner with approximately 104 cells. CD8 T cells were paired with antigenic peptides-loaded B cells, enabling characterization of the early activation dynamics of CD8 T cells. Zhou et al. introduced a hierarchical loading microwell chip (HL-chip) based on size differences, featuring a high-throughput microwell array with single-cell precision (Fig. 11c).185 Their study demonstrated the evaluation of T cell functions, including longitudinal secretory profiling, cytolytic activity, and T cells and tumor cells interaction. The dual-well HL-chips allowed the co-culture and interaction between target cells and T cells, enabling the observation of T cell binding and cell killing.
In recent investigations, CAR-T cell-mediated cytotoxicity was assessed through the co-culture of CAR-T cells and 3D spheroids composed of cancer and stromal cells within ultralow-adhesion microwells (Fig. 11d).186 This approach involved two open wells connected by a microfluidic channel with an array of independent culture chambers, facilitating the sedimentation of cells into microwell traps below the channel level. Assessment of CAR-T cell targeting, and cytotoxicity revealed the ability of the cells to induce disaggregation of MDA-MB-468 spheroids and subsequent cell death.
Although microwell-based cell interaction studies facilitate efficient cell pairing and compatibility with live imaging, paracrine communication between neighboring cells is possible as the secreted proteins are not confined to individual wells.179 Therefore, for more precise evaluation at the single-cell level, including cytotoxicity, signaling and activation dynamics, and cytokine release studies, it is imperative to isolate cells within each microwell. Therefore, to achieve the same, droplet microfluidics can be a valuable tool for advanced analysis and assessment.
3.1.2. Droplet microfluidics for cell–cell interaction studies and vector copy number evaluation.
In contrast to microwell-based compartmentalization, droplet microfluidics leverages immiscible multiphase flows of media and oil in T-, Y-, or cross-shaped microchannel junctions to confine desired cells to water-in-oil droplets. Droplet microfluidics-based assays offer critical capabilities, such as compartmentalization of components at a high-throughput of thousands of pico- or nanoliter droplets per second. This approach has proven valuable in single-cell analysis, particularly within the field of immunology, as droplet compartmentalization can effectively account for the intrinsic heterogeneity exhibited by immune cells.187 Droplet microfluidics offer noise-free compartmentalization of cells and their secretions, making it suitable for assessing cell–cell interactions and cytotoxicity (Fig. 11e).188,189 Furthermore, this approach is also applicable to research involving cytokine release,190,191 activation dynamics,192 and cytotoxicity.189,193 Notably, droplet microfluidics is highly advantageous for accurately quantifying the actual number of vector copies integrated into transfected CAR-T cells.194–197
For viral transduction, precision in quantifying the average vector copy number per T cell is crucial. Relying only on assessing the average number may lead to inaccurate clinical potency evaluation. Furthermore, high transgene copy numbers carry the risk of genotoxicity and increased integration near oncogenes.198 To obtain FDA's approval for the clinical use of CAR-T cells, it is mandatory to ensure a vector copy number of less than five copies per transduced cell.199 The conventional method for measuring vector copy number in CAR-T cells involves qPCR followed by the construction of a standard curve.200 However, digital droplet-compartmentalized PCR (ddPCR) offers a simpler and more precise approach to measuring the average vector copy number per cell and its variation within the cell population, with a 7-fold improvement in reproducibility compared with real-time PCR.194,195 Furthermore, several studies have demonstrated the robustness of ddPCR as a safety measure for assessing the vector copy number of CAR-T cells, showing a linear correlation with flow cytometry measurements.196,197 Consequently, droplet-based cell compartmentalization has emerged as a reliable and straightforward analytical tool for evaluating the efficacy and safety of CAR-T cells at the single-cell level in its manufacturing and development.
3.2. CAR-T cell therapy for solid tumors
While CD19-targeted CAR-T cell therapy has exhibited remarkable success in the treatment of B cell ALL,201 its implementation in the treatment of solid tumors encounters challenges in recognizing, trafficking, and survival of the tumor.9 Optimal recognition of tumors can be achieved through the specific and uniform expression of a single target antigen on the tumor cell. Unfortunately, solid tumors often demonstrate significant heterogeneity in tumor antigens and evade destruction by expressing alternative antigens.202 Moreover, various characteristics of the TME, including hypoxia, immunosuppressive immune cells, soluble factors, and endothelial barriers, contribute to the limited infiltration and survival of CAR-T cells in tumors. Consequently, there is a substantial disparity in CAR-T cell therapy efficacy between hematological and non-hematologic malignancies. To address these challenges, targeting tumor-associated antigens (TAAs), such as prostate-specific membrane antigens (PSMA), mesothelin for pleural mesothelioma, ovarian pancreatic and lung cancers, EGFR for glioma, carcinoembryonic antigen (CEA) for adenocarcinoma liver metastases with CEA expression, and disialoganglioside GD2 for neuro-ectodermal originated tumors, has been employed to enable CAR-T cell therapy in solid tumors.203,204 However, the intrinsic properties of the solid TME hinder specific targeting and immune response efficacy, necessitating the development of novel strategies to successfully infiltrate and persist within the TME for precise targeting of such tumor-associated antigens. In the following subsection, we provided a brief overview of the challenges that lie within the TME and explored new opportunities to address them through microfluidics.
3.2.1. Tumor microenvironment (TME).
In contrast to hematological malignancies, a solid TME inherently possesses unfavorable characteristics that impede CAR-T cell therapy efficacy.205 The infiltration of CAR-T cells into the tumor is physically hindered by the vasculature, extracellular matrix (ECM), and blood vessels surrounding the tumor, as well as a dense network of cancer-associated fibroblasts.206 Moreover, TME exhibits hypoxic and immunosuppressive conditions. Reduced oxygen levels have been observed to negatively affect the antitumor immune response of T cells.207 Additionally, immune cells present within the TME create an immunosuppressive milieu by depleting IL-2, secreting immunosuppressive cytokines (such as IL-10 or TGF-β, suppressing antigen-presenting cells, hindering T cell activation), and inducing the lysis of effector cells through granzyme- or perforin-based mechanisms.208
Various approaches to and trials on CAR-T cell therapy have been performed to overcome the challenges posed by TME. Enhancing T cell trafficking into the tumor mass involves disrupting the tumor vasculature and targeting the ECM through the expression of matrix-degrading enzymes.209,210 Efforts are also being made to overcome TME immunosuppression and improve T cell survival by selecting appropriate co-stimulatory domains that enhance resistance against hypoxia and regulatory T cells,211,212 as well as by targeting antigens that are upregulated under hypoxic conditions.213
3.2.2. Microfluidic technologies as 3D cell culture to mimic the TME.
Conventional preclinical methods for assessing the therapeutic efficacy of CAR-T cell therapy involve in vitro 2-dimensional (2D) culture followed by subsequent in vivo studies. However, the reliance on 2D culture in a Petri dish, although convenient in laboratory settings, oversimplifies the complex physiological conditions, leading to inaccurate evaluations.214 Conversely, in vivo animal testing offers a closer approximation to the human TME, but is associated with high cost, time-consuming protocols, and limited immune response, while also often yielding misleading results.215
Microfluidics and lab-on-a-chip technologies have emerged as promising alternatives for addressing these limitations and the ethical concerns associated with animal models, aligning with recent FDA regulations that no longer require animal testing for new drug development.216 As briefly mentioned above, these microfluidic platforms enable high-fidelity simulation of complex physiological conditions in a dynamic and high-throughput manner.217 Notably, biomimetic microfluidic systems, such as organ-on-a-chip and tumor-on-a-chip platforms, facilitate TME reverse engineering and allow direct and real-time observation using microscopy techniques.218 In particular, organ-on-a-chip platforms offer a promising alternative to Petri dish-based cultures by addressing the limitations associated with oversimplification of TME. These platforms hold great potential for applications in drug screening and mechanistic studies of TME.
Accurate simulation of the physiological milieu requires careful consideration of several components of the cell and TME.219 One crucial aspect is the selection of materials that mimic the ECM, which is responsible for focal adhesion formation and mediates biochemical cues influencing cell survival, proliferation, and migration. The ECM also provides biophysical cues through structural orientation, mechanical stiffness, and pore presentation.220 Common protein components for ECM simulation include naturally derived hydrogels, such as collagen, fibrin, fibronectin, chitosan, alginate, and hyaluronic acid, and synthetic hydrogels, such as poly(acrylamide), poly(ethylene glycol), and hybrid hydrogels.221
Additionally, the dimensionality of the culture system plays a pivotal role in cell–ECM adhesion and the establishment of a concentration gradient of nutrients, oxygen, and soluble factors.219 In 3D culture, physical barriers hinder free diffusion and convective flow. Hypoxia due to low oxygen availability within the tissue is a hallmark of solid tumors. This hypoxic condition enhances chemoresistance and immunosuppression and induces a transcription program that promotes an aggressive tumor phenotype.222 Given that 2D Petri dish-based cell culture models are unable to replicate the oxygen gradient observed in the TME, the implementation of microfluidic 3D cell culture systems capable of recapitulating solid tumor hypoxia is anticipated to make significant contributions to the field.223
Furthermore, the difference between static medium perfusion in Petri dish-based cell cultures and dynamic perfusion in microfluidic-based cultures influences the shear stress, delivery of suspended immune cells, cancer cell metastasis, and material exchange with blood vessels in the TME. While 3D cell culture has evolved from hydrogel cultures to microwell-based tumor spheroids/organoids and biomimetic microfluidic channel-based culture platforms, its applications in immunotherapies have predominantly focused on fundamental biology research, including the characterization of cell behavior within the TME, cell–cell interaction, and cell migration.224 Despite the demonstrated potential of microfluidic 3D cell culture, it has yet to be rigorously explored as a robust preclinical evaluation tool for assessing CAR-T cell therapy efficacy.225 In the following sections, we will discuss the culture of spheroids and organoids as well as complex microfluidic physiological systems, as platforms for the characterization, evaluation, and screening for CAR-T cell therapy.
3.2.2.1. Spheroids and organoids.
Spheroids represent a basic form of 3D cell culture consisting of simple cell aggregates, whereas organoids encompass more complex cell aggregates that are often embedded in 3D hydrogels. Unlike 2D cell culture models, 3D spatial organization in the form of spheroids and organoids can recapitulate solid tumors' key features, such as the necrotic and hypoxic core and the proliferative outer layer. These structures can be cultured in various ways, including static suspension, hanging drop, microfluidic systems, and gel embedding.226 These culture systems enable cell–cell and cell–ECM interactions and the establishment of oxygen, nutrient, and cytokine gradients. Moreover, previous studies have shown that 3D spheroid culture systems better represent in vivo aspects of cancer cell signaling profiles.227
In the context of CAR-T cell therapy, spheroids and organoids have been employed to assess CAR-T cell cytotoxicity,228–230 investigate the role of hypoxia in CAR-T cell function,231 and study CAR-T cell function in 3D tumor models.232,233 Patient-derived organoid models have also been proposed to accurately evaluate patient-specific responses to CAR-T cells for personalized therapy.234
Chang et al. evaluated T cell anti-tumor activity using bispecific antibodies and PD-1 blockade in 3D spheroids of the MDA-MB-231 breast cancer cell line, suggesting that 3D spheroids could serve as an alternative to in vivo animal models for evaluating T cell potency in solid tumors.235 More recently, spheroids of the colon cancer cell line HCT 116 were used to evaluate CAR-T cell infiltration and cytotoxicity,230 further supporting the potential of spheroid-based assessment of cell therapy efficacy to prevent premature progression in in vivo studies. However, a major challenge in using spheroids as a tool for cytotoxicity assessment is the difficulty of separating unbound CAR-T cells and dead tumor cells from spheroids can result in additional staining steps and the possible loss of spheroids. To address this issue, a high-throughput HER2-T cell cytotoxicity assay was conducted on a 3D-hanging spheroid plate at a tilted angle,228 demonstrating a simplified approach for differentiating and quantifying the activity of CAR-T cell killing in HER2-positive breast cancer cell lines.
While spheroid and organoid cultures offer numerous advantages and potential, there do have certain limitations. Although they can preserve cellular diversity and hypoxic conditions within the core, they are often regarded as oversimplified models, particularly in CAR-T cell co-culture. This is due to their inability to accurately replicate the indirect access to tumor masses via blood vessels.234 Hence, the effector-target cell ratio observed in organoid culture may diverge significantly from the actual CAR-T cells that successfully infiltrate solid tumors in vivo.
3.2.2.2. Microfluidic physiological systems.
As discussed, despite 3D spheroid/organoid models being more similar to physiological conditions than 2D cultures, microfluidic TME models offer distinct advantages in capturing architectural complexities. These merits include the ability to perform co-culture with stromal and immune cells, facilitate extravasation from blood vessels, and enable infiltration into the tumor mass. Additionally, microfluidic models provide improved accessibility for live imaging and reduce reliance on reagents and biological components. Notably, several studies have employed microfluidic cell culture models to investigate the impact of extracellular matrix stiffness and hypoxia on T cell cytotoxicity, the role of monocytes, tumor cell extravasation, and the interactions between tumors and T cells (Fig. 12a).236–241
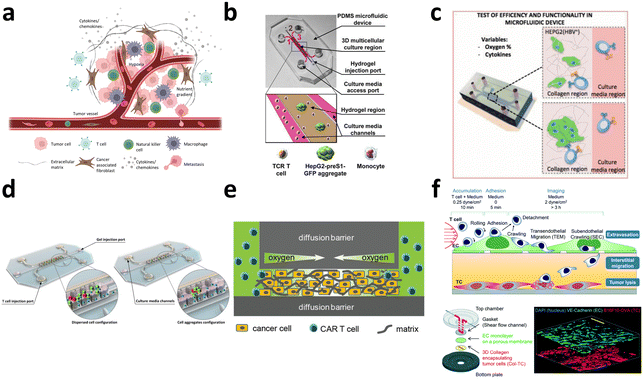 |
| Fig. 12 Microfluidic physiological systems to simulate the tumor microenvironment (TME). (a) Schematic of solid TME. (b) 3D static microfluidic model to characterize the role of monocytes in TCR-T cell therapy. Reproduced from ref. 239 (CC BY 4.0). (c) Microfluidic tumor-on-a-chip to study tumor cell extravasation and evaluate engineered T-cell immunotherapy efficiency. Reprinted, with permission, from ref. 237. Copyright 2015 IEEE. (d) Preclinical evaluation of TCR-T therapy against solid tumors. Used with permission of American Society for Clinical Investigation, from ref. 242; permission conveyed through Copyright Clearance Center, Inc. (e) Hypoxic 3D tumor model with oxygen gradient and matrix microenvironment for the evaluation of CAR-T therapy. Reproduced from ref. 241. Copyright 2019 John Wiley and Sons. (f) Multilayered blood vessel/tumor tissue chip to observe T cell extravasation, interstitial migration, and tumor lysis. Reproduced with ref. 240 with permission from The Royal Society of Chemistry. | |
To enable the co-culture of effector and target cells in a physiologically relevant environment, an integrated microfluidic platform was developed. The device utilized combined biosensors, including optical light scattering, and electrical impedance, to dynamically assess the cell–cell interaction between adherent cancer cells and nonadherent immune cells.236 In a separate study, Layer et al. employed a microfluidic migration assay to demonstrate that the proto-oncogene protein N-Myc impaired IFN pathway activity, chemokine expression, and immune cell recruitment.238 Lee et al. conducted a comparison between 2D and 3D microfluidic co-cultures to screen the target cell killing activity of TCR-T cells produced through different methods (Fig. 12b).239 Using a PDMS microfluidic device, they created three channels: a hydrogel region for seeding target cancer cell aggregates and monocytes, and two culture medium channels containing medium and TCR-T cells adjacent to the hydrogel channel. This setup closely mimicked the physical barriers encountered by T cells in vivo. Their study confirmed previous findings by Pavesi et al., which emphasized the advantage of 3D tumor models in screening various TCR-T-cell therapies, addressing the intrinsic limitations of 2D culture, such as overestimation of T-cell cytotoxicity and the inability to capture the oxygen gradients that influence T-cell behavior (Fig. 12c and d).237,242 They leveraged microfluidic channels to mimic the microvascular network, study tumor cell extravasation, and evaluate T cell immunotherapies. Meanwhile, the cytotoxicity mediated by CAR-T cells was evaluated in a microfluidic 3D tumor model that mimicked the physiological hypoxic environment (Fig. 12e).241 The study demonstrated that oxygen levels play a crucial role as a factor driving metabolic changes and enhance CAR-T cell cytotoxicity at physiologically relevant tissue oxygen levels.
Additionally, a multilayered blood vessel/tissue chip was developed to systematically investigate the therapeutic potential of CAR-T cells for solid tumors. This chip consists of a 3D collagen region for tumor cells, a porous membrane with an endothelial cell monolayer, and a fluidic channel with T cells (Fig. 12f).240 This simulation replicates the process of T cell adhesion to the endothelial layer of cells, extravasation, interstitial migration within the tumor tissue, and subsequent tumor lysis by T cells.
The transition of adoptive cell therapies from hematological to solid tumor treatments holds promise for cancer immunotherapy. Despite the promising results from microfluidic TME models, again, it remains unclear how accurately they approximate in vivo cancer biology.243 Complex in vivo biological processes cannot be fully recapitulated in a single microfluidic TME model. Thus, selecting the most suitable microfluidic system and conducting cross-validation between different microfluidic models are essential to study specific biological processes of interest.
Note that efforts have been made to enhance the complexity of microfluidic tumor models, including the introduction of microfluidic channels to simulate vascularization, the use of hydrogels for ECM, the creation of oxygen gradients for hypoxia, and the incorporation of multilayered vessels for endothelial cells to evaluate T cell infiltration, cytotoxicity, and interactions within the TME. For widespread adoption of microfluidic tumor models in therapeutic screening, several challenges must be addressed. These include the simulation of complex signal regulation functions, the industrial cGMP manufacturing of tumor-on-a-chip devices, the standardization of data, and the exploration of biocompatible materials other than PDMS.218
3.3. Next-generation CAR/TCR screening
The identification of antigen-binding TCRs plays a crucial role in uncovering potential targets, such as adoptive T-cell therapy, including CAR-, TCR-, and TIL-T-cell therapies, immune checkpoint blockade, and bispecific T-cell engager (BiTE) antibodies, for cancer immunotherapy. However, the main challenges in matching TCR sequences to the antigen specificities of T cells arise from the immense complexity of the T cell repertoire, which consists of billions of clonotypes combined with bulk, low-throughput functional screening technologies.244 Thus far, FACS, single-cell PCR, and Sanger sequencing have been employed in combination to screen TCR antigen specificity.245,246 However, these methods are often limited in their ability to identify only a few hundred clones at a time, and the prescreening process used to narrow down the candidate antibodies can result in the loss of certain target profiles. Additionally, the bulk analysis of T cells can overlook the molecular details of individual T cells.192 To effectively discover potential target antigens while preserving diversity, it is imperative to perform high-throughput functional screening of T cell heterogeneity at the single-cell level and subsequently sort the cells for TCR sequencing.
As mentioned, microfluidic technologies provide the advantage of precise cell manipulation and analysis, thereby enabling high-throughput single-cell processing. Droplet microfluidics-based assays are compatible with biochemical assays involving cell lysis and amplification within the droplets,247 allowing the incubation of viable cells for extended periods of up to 4 days. Additionally, droplet microfluidics-based assays offer high sensitivity and signal-to-noise ratio owing to their small volume, enabling multifunctional screening of single cells and real-time measurement.192 These features render droplet microfluidics suitable for antigen-specificity screening technology for immunotherapy.
Droplet microfluidics has advanced the detection, identification, and separation of antigen-specific T cells through various functional screening techniques,190,192,248,249 sequencing approaches,250–252 and their integration for next-generation cancer immunotherapy drug discovery.253,254 For example, Chokkalingam et al. employed droplet co-encapsulation of functionalized cytokine-capture beads to detect the secretion of IL-2, IFN-γ, and TNF-α from single activated T cells,255 while Segaliny et al. achieved real-time monitoring of single TCR-T cell activation and sorting of each clone at 100% specificity using droplet co-encapsulation of TCR-T cells with target cells, followed by downstream molecular analysis using single-cell sequencing of TCR.256 Moreover, Wang et al. identified functional antibodies for CD40 agonism with a frequency of at <0.02% after two rounds of functional screening, achieving high-throughput by processing millions of droplets within hours.257 By preserving the linkage between phenotype and genotype within the droplets, fluorescence-activated droplet sorting enabled the enrichment of droplets containing desirable activated reporter cells. In addition, a high-throughput droplet-based screening and sorting platform for antigen-specific TCRs was developed, demonstrating functional screening of TCR-pMHC interactions at a rate of 450 droplets per s.258
Droplet compartmentalization is widely used in single-cell sequencing, and notable technologies include inDrop,250 drop-seq,252 and scRNA-seq.259 These methods involve the separation and lysis of individual cells within droplets, followed by nucleic acid amplification, sequencing, and data analysis. Each droplet was barcoded to enable tracking of sequence reads back to the corresponding single cell. In the context of cancer immunotherapy, the high-throughput sequencing of TCR genes plays a role in the discovery of new targets. McDaniel et al. reported the sequencing of both TCRβ/δ-positive TCRα/γ chains from millions of cells with high precision (>97%), a level of detail that is lost in the conventional bulk sequencing where immune receptor chains are encoded by separate mRNAs.253 Additionally, the labeling of antigen-specific CD8-positive T cells using peptide–MHC tetramers was demonstrated for isolation,254 and the labeled TCR genes were directly amplified and sequenced in an integrated device, providing 1000-fold higher sensitivity than in conventional bulk analysis. Against this background, there is still ample opportunity for droplet microfluidics to play a significant role in CAR/TCR screening.
3.4. Allogeneic off-the-shelf CAR development
3.4.1. Microfluidic CAR-NK cell production.
NK cells are cytotoxic immune cells similar to CD8+ T cells and are characterized by the expression of CD56 without the co-expression of CD3 and TCRs.260 In general, NK cells recognize and kill tumors by integrating signals from inhibitory and activating receptors.261 Tumor cells lacking HLA, which are recognized by inhibitory receptors, are commonly targeted by NK cells. Moreover, NK cells kill tumors through antibody-dependent cell-mediated cytotoxicity (ADCC).262 The CD16 receptor on NK cells recognizes the Fc region of immunoglobulin G (IgG) bound to the surface of the target cells, activating the cytotoxic mechanism of NK cells.
It should be mentioned that NK cells, with their non-antigen-specific tumor killing capacity, are highly promising candidates for allogeneic immunotherapy.263,264 As mentioned earlier, the efficacy of CAR-T cell therapeutics remains unclear due to the scarcity and heterogeneous subpopulations of autologous T cells.4,22,100 Furthermore, the administration of allogeneic CAR-T cells to patients can result in GvHD and cytokine release syndrome (CRS), primarily attributed to the presence of TCR on T cells. In contrast, NK cells, lacking antigen specificity, have attracted interest for the development of off-the-shelf CAR-NK cells, which offer a reduced risk profile (Fig. 13a).
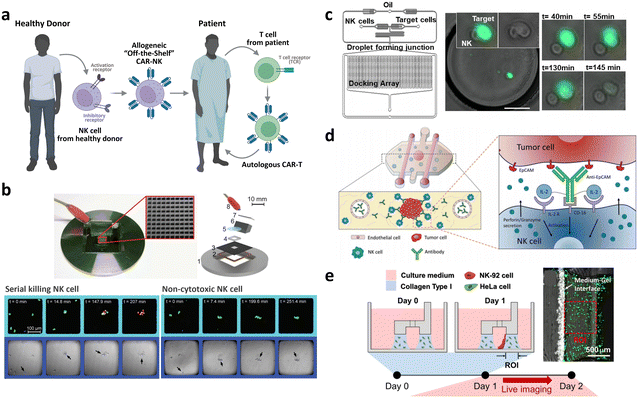 |
| Fig. 13 Allogeneic ‘off-the-shelf’ CAR development. (a) Comparison of CAR-T cell and ‘off-the-shelf’ CAR-NK cell. (b) Microarray of acoustic traps for quantification of natural killer cell heterogeneity. Reproduced from ref. 268 with permission. Copyright 2013 Oxford University Press. (c) Paper microfluidic cell chromatography for natural killer cell detection, quantification, and subpopulation identification. Reproduced from ref. 276 with permission from The Royal Society of Chemistry. (d) Evaluating natural killer cell cytotoxicity against solid tumors using a microfluidic model. Reproduced from ref. 281. Copyright 2019 Taylor and Francis. (e) 3D tumor spheroid microarray for high-throughput natural killer cell-mediated cytotoxicity. Reproduced from ref. 282 (CC BY). | |
Previously, CAR-NK cells were produced at a clinical scale using flow electroporation.265 Expanded NK cells from a 10-day culture were resuspended in 14 mL of EP buffer containing 20 μg mL−1 of mRNA. After 24 h, 18 samples collected in 800 μL fractions showed transfection efficiency of 94 ± 1%, demonstrating the scalability of flow electroporation for CAR-NK cell production. In the case of CD19-CAR mRNA transfection, ex vivo-expanded NK cells from 12 individuals showed 82% CAR expression and 87% cell viability.266In vivo studies using xenograft models of B cell leukemia showed that anti-CD19 CAR-expressing NK cells resulted in a 10-fold lower tumor burden. These results paved the way for a clinical trial of CAR-NK cells using flow electroporation (NCT01914479).
In the following sections, we discuss microfluidic approaches aimed at studying the unknown features and tumor killing abilities of NK cells to advance the production of clinically applicable CAR-NK cells.
3.4.2. Microfluidic approaches for NK cell characterization.
3.4.2.1. NK cell heterogeneity assay.
There are two types of NK cells: CD56dimCD16bright and CD56brightCD16dim.260 The former exhibits higher tumor cell cytotoxicity, whereas the latter acts as an immature NK cell with lower cytotoxicity. Understanding the heterogeneity in NK cell potency is of great interest for cell-based immunotherapy.
A droplet microfluidic platform was used for a single NK cell heterogeneity assay via a killing assay with K562, a myelogenous leukemia cell line.267 Approximately 3% of the visualized droplets (60
000 per experiment) exhibited 1
:
1 cell pairing. A large fraction (77%) of NK cells induced cytotoxicity within the first 4 h of interaction, corresponding to the proportion of NK cell subclasses. Additionally, a microchip containing 100 microwells was introduced to study the lytic activity of individual NK cells against MHC class I-deficient tumor target cells (Fig. 13b).268 IL-2 activated PBMC NK cells, and calcein-stained cells were seeded in the device, with approximately two-thirds of the NK cells exhibiting cytotoxicity. Live cell imaging reveled that several NK cells killed up to six target cells in the 4 h assay. For a time-efficient, high-throughput NK cell heterogeneity assay in a microfluidic platform, the droplet size for the cell killing assay was adjusted based on the ratio of effector NK cells to target cells (E
:
T ratio).193 NK cells were co-encapsulated with different hematological cancer cells (K562, Jurkat, and KG1a) and monitored for 6 h after droplet formation. For small-sized (∼67 μm) droplets, it was shown that half of the killing events occurred within the first hour of observation at an E
:
T ratio of 1
:
1, whereas large droplets (∼85 μm) were more suitable for containing three or more cells, providing a balance between the closest proximity of the effector and target cells. These studies demonstrated that the compartmentalization of microwells or droplets enables single NK cell analysis to study NK cell heterogeneity in a high-throughput and time-efficient manner. This highlights the versatility of droplet microfluidic platforms for examining donor-dependent heterogeneity of NK cells in precision medicine for cell-based immunotherapy and the study of heterogeneous NK cell cytotoxicity against different tumor types.
As mentioned above, CD56dim NK cells showed higher cytotoxicity than CD56bright ones. The isolation of NK cell subtypes is required to produce CAR-NK cells using highly cytotoxic CD56dim NK cells. Moreover, the classification of NK cells can serve as a parameter as the relative proportion of CD56dim cells among the total NK cells changes in conditions such as melanoma and autoimmune diseases.
Dannhauser et al. introduced a label-free microfluidic device that could identify NK cell subclasses based on biophysical single-cell features.269 Flowing in a viscoelastic medium at a relatively low rate of approximately 50 cells per s, CD56dim and CD56bright NK cells were classified with a significant difference between the refractive indices of the nucleus. The device achieved sensitivity of 94.1% for CD56bright cells and 76.4% for CD56dim cells.
Contrarily, a paper-based microfluidic device for cell chromatography was utilized to analyze the proportion of CD56bright and CD56dim NK-92 cells.270 The cells flowed through the paper-based device containing anti-CD56 fluorescent nanoparticles, and heterogeneous NK cells could be distinguished based on size differences because IL-2-starved CD56dim NK-92 cells were smaller than CD56bright ones. Consequently, CD56bright NK-92 cells were trapped near the inlet of the device owing to increased flow resistance. Microfluidic approaches have enabled the label-free, time-efficient, and high-throughput identification of NK cell subtypes. Therefore, in addition to therapeutic applications, microfluidic approaches can be used for diagnostic purposes to assess the proportion of cell subtypes, which can vary with the occurrence of cancer.271
3.4.2.2. NK cell cytotoxicity assay.
Owing to the differential expression of inhibitory MHC class I ligands by different types of tumor cells, the cytotoxicity of NK cells against tumors exhibits significant heterogeneity. Therefore, it is essential to gain a comprehensive understanding of heterogeneous NK cell cytotoxicity against various tumor cells to develop strategic immunotherapy approaches, including CAR-NK cells,265,266 high-affinity Fc receptor (CD16) NK cells for ADCC, CCR7-expressing NK cells for migration toward a solid tumor,272 as well as immune checkpoint inhibitors273 or targeted therapies such as doxorubicin274 and trastuzumab.275 Therefore, numerous studies have investigated the cytotoxicity of NK cells against various tumor cells.
Sarkar et al. introduced droplet microfluidic platforms to analyze the effector outcomes of NK cells against target cells at the single-cell level (Fig. 13c).276 Within each droplet, NK cells and target cells were encapsulated at a 1
:
1 ratio in a picoliter volume, thus minimizing the influence of neighboring NK cells or target cancer cells. The docking array in the region after droplet formation trapped approximately 4000 droplets per experiment. For the analysis of NK cell cytotoxicity, specific droplets of interest were monitored for up to 6 h, at 5 min intervals, demonstrating that prolonged contact between NK cells and target cells increased cell death, irrespective of the upregulation of NK-activating ligands. Furthermore, an NK cell-killing assay performed using this platform revealed that the NK cells were capable of killing target cells without the formation of immunological synapses. This platform was further applied to study NK-92 cells in combination with an immune checkpoint blockade or lenalidomide against multiple myeloma,277 NK-92 cells against HER2-positive solid tumor,278 and CD19-CAR natural killer cells against CD20-resistant non-Hodgkin lymphoma,279 maximizing its applications.
Screening donor-dependent heterogeneous NK cells in terms of cytotoxicity is crucial for the production of highly efficient off-the-shelf NK cells that target various tumors. With the modification of FDA regulations regarding animal testing,216 microfluidic cell cytotoxicity assay platforms have the potential to become new standards for screening and quality testing in the field of NK/Tcell-based immunotherapy for various tumors.
3.4.3. NK cell functional assay for solid tumor therapy.
Although NK cell cytotoxicity against solid tumors has been validated through single-cell analysis, TME remains a major obstacle, similar to CAR-T cell therapies.280 Consequently, microfluidic platforms offer new opportunities to evaluate NK cell cytotoxicity against solid tumors and enhance the therapeutic efficacy of NK cell-based immunotherapy.
Ayuso et al. introduced a 3D ECM model comprising breast cancer cell spheroids surrounded by lumens lined by human umbilical vein endothelial cells (HUVECs) (Fig. 13d).281 They demonstrated that tumor cell–cell interactions hinder antibody penetration, as the antibodies are taken up by tumor cells via endocytosis to evade immune surveillance. In the NK cell assay, despite their large size compared with that of the antibodies, NK cells were able to penetrate spheroids. However, even with a higher ratio of NK cells, their cytotoxicity was hindered by the ECM, as observed in other 3D toxicity assays using HeLa cells (Fig. 13e).282 A high-throughput microfluidic assay for a cancer immunotherapy platform was proposed to evaluate the killing ability of NK-92 cells in a 3D HeLa cell model. Compared with the 2D cytotoxicity assay in which approximately 90% of HeLa cells were killed, a lower percentage of HeLa cells were killed in the 3D TME despite the higher NK cell density. A microarray-based approach was also used for high-throughput NK cell cytotoxicity assays against 3D spheroids.283 The platform utilized micropillar–microwell sandwiched structures coated with dopamine hydrochloride in Tris-HCl to facilitate covalent bonding with MCF-7 cells. In this TME-mimicking 3D spheroid model, NK92-CD16 cells exhibited approximately 60% cytotoxicity toward MCF-7 cells under hypoxic conditions. This suggests that the presence of antibodies, such as trastuzumab, doxorubicin, and paclitaxel, can increase NK cell cytotoxicity against tumor cells.
Within the ECM, NK cells not only interact with tumor cells but also with DCs, which are APCs.284 A microfluidic device was proposed in 2014 to assess NK cell migration in response to crosstalk with DCs.285 This platform utilized two channels: one for the control culture medium and the other for chemokines or conditioned medium. Upon exposure to conditioned medium from immature DCs, approximately 66% of the NK cells exhibited chemotactic migration. Conversely, the presence of granulocyte macrophage colony-stimulating factor (GM-CSF) induces the repulsive migration of NK cells. This microfluidic platform enables the investigation of interactions between NK cells and APCs or other lymphoid cells, thereby opening new avenues for enhancing the therapeutic efficiency of NK cell immunotherapy.
As another study to address the limitations imposed by the TME in NK cell-based immunotherapy, a micro-spray technology was proposed for the production of porous microspheres encapsulating NK cells, allowing in situ injection.286 For this purpose, an alginate solution mixed with poly(ethylene oxide) (PEO) was used as the precursor solution for porous microspheres. An in vitro assay demonstrated that approximately 80% of the MDA-MB-231 cells and nearly 100% of the A375 cells were killed by the NK-92MI-encapsulated microspheres. Furthermore, an in vivo study showed that 75% of tumor cells underwent apoptosis following in situ injection of NK-92MI-encapsulated microspheres. Therefore, by leveraging microfluidics technology, not only can enhanced therapeutic strategies be screened, but more efficient drug delivery systems can also be established.
4. Conclusion and perspectives
This review discusses CAR-T cell immunotherapy, providing a detailed analysis of its current landscape, limitations, and potential solutions and opportunities through the integration of microfluidics and microfluidics-based approaches. Additionally, this review explores the application of microfluidics in advancing next-generation CAR-T cell therapies, including the emerging roles of allogeneic CAR-T and CAR-NK cells in the treatment of solid tumors.
To assess the impact of microfluidics on immunotherapy, a literature search was conducted using the keywords “microfluidic*” and “immunotherapy*” in the Web of Knowledge database. When performed in July 2023, the search yielded approximately 340 articles with over 7700 citations (Fig. 14). Although these numbers may underestimate the true potential of microfluidics in immunotherapy, it is noteworthy that the field itself has experienced rapid growth since FDA approved the first CAR-T cell therapy product (Kymriah®) in 2017. Another search with the keywords “microfluidic*” and “cell therapy*” retrieved approximately 4400 articles with over 84
000 citations in the Web of Knowledge database, confirming the increasing attention and potential impact of microfluidics.
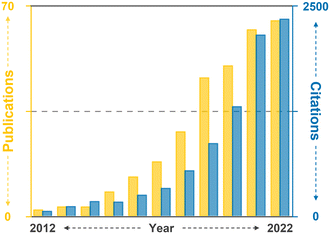 |
| Fig. 14 Growth of field. Bar graph representing the publications (yellow) and citations (blue) of each year including words “microfluidic*” and “immunotherapy*” according to the Web of Knowledge database, as of July 2023. | |
However, for microfluidics to have a significant impact, it must progress beyond proof-of-concept and advance toward commercialization efforts. In this context, the commercialization of previous electroporators and ongoing microfluidic cell transfection platforms by numerous companies are worth mentioning. Additionally, current developments in organ-on-a-chip technology for immunological research and drug development by various startups have signified the correct steps taken in this direction. These endeavors are pivotal for optimizing CAR-T cell manufacturing and ensuring its practical implementation.
In conclusion, although numerous challenges remain in realizing fully automated microfluidics-based CAR-T/NK cell production, it is very true that considerable progress has been made through the application of microfluidics in immunotherapy. With growing opportunities in cell-based therapies, increasing attention from the medical community and public, engagement of major pharmaceutical companies, and commercialization efforts, microfluidic platforms have the potential to revolutionize cancer treatment. Continued collaborative development across academia, industry, and the medical field will pave the way for the integration of microfluidics and associated methodologies into cellular engineering and immunotherapy, decisively shaping the future of these disciplines.
Conflicts of interest
There are no conflicts of interest to declare.
Acknowledgements
The authors would like to thank all members of the Biomicrofluidics Laboratory at Korea University for their useful discussions. This study was supported by the National Research Foundation of Korea (NRF) grants funded by the Korean government (MSIT; Ministry of Science and ICT) (2021R1A2C2006224, RS-2023-00242443, and RS-2023-00218543), the Technological Innovation R&D Program (RS-2023-00262758) funded by the Ministry of SMEs and Startups (MSS, Republic of Korea), and the Materials/Parts Technology Development Program (20020278) funded by the Ministry of Trade, Industry & Energy (MOTIE, Republic of Korea). The schematics in Fig. 1–3, 4c (1), 6, 12a, and 13a were created using https://BioRender.com.
References
- C. H. June, R. S. O'Connor, O. U. Kawalekar, S. Ghassemi and M. C. Milone, Science, 2018, 359, 1361–1365 CrossRef CAS PubMed
.
- M. Kalos, B. L. Levine, D. L. Porter, S. Katz, S. A. Grupp, A. Bagg and C. H. June, Sci. Transl. Med., 2011, 3, 95ra73 CAS
.
- D. L. Porter, B. L. Levine, M. Kalos, A. Bagg and C. H. June, N. Engl. J. Med., 2011, 365, 725–733 CrossRef CAS PubMed
.
- S. A. Grupp, M. Kalos, D. Barrett, R. Aplenc, D. L. Porter, S. R. Rheingold, D. T. Teachey, A. Chew, B. Hauck, J. F. Wright, M. C. Milone, B. L. Levine and C. H. June, N. Engl. J. Med., 2013, 368, 1509–1518 CrossRef CAS PubMed
.
- V. Wang, M. Gauthier, V. Decot, L. Reppel and D. Bensoussan, Cancers, 2023, 15, 1003 CrossRef CAS PubMed
.
- J. J. Melenhorst, G. M. Chen, M. Wang, D. L. Porter, C. Chen, M. A. Collins, P. Gao, S. Bandyopadhyay, H. Sun and Z. Zhao, Nature, 2022, 602, 503–509 CrossRef CAS PubMed
.
- B. L. Levine, J. Miskin, K. Wonnacott and C. Keir, Mol. Ther.--Methods Clin. Dev., 2017, 4, 92–101 CrossRef CAS PubMed
.
- A. Aijaz, M. Li, D. Smith, D. Khong, C. LeBlon, O. S. Fenton, R. M. Olabisi, S. Libutti, J. Tischfield, M. V. Maus, R. Deans, R. N. Barcia, D. G. Anderson, J. Ritz, R. Preti and B. Parekkadan, Nat. Biomed. Eng., 2018, 2, 362–376 CrossRef CAS PubMed
.
- F. Marofi, S. Tahmasebi, H. S. Rahman, D. Kaigorodov, A. Markov, A. V. Yumashev, N. Shomali, M. S. Chartrand, Y. Pathak, R. N. Mohammed, M. Jarahian, R. Motavalli and F. M. Khiavi, Stem Cell Res. Ther., 2021, 12, 443 CrossRef PubMed
.
- G. Choi, G. Shin and S. Bae, Int. J. Environ. Res. Public Health, 2022, 19, 12366 CrossRef CAS PubMed
.
- J. T. Bulcha, Y. Wang, H. Ma, P. W. L. Tai and G. P. Gao, Signal Transduction Targeted Ther., 2021, 6, 53 CrossRef CAS PubMed
.
- E. K. Sackmann, A. L. Fulton and D. J. Beebe, Nature, 2014, 507, 181–189 CrossRef CAS PubMed
.
- P. Yager, T. Edwards, E. Fu, K. Helton, K. Nelson, M. R. Tam and B. H. Weigl, Nature, 2006, 442, 412–418 CrossRef CAS PubMed
.
- P. S. Dittrich and A. Manz, Nat. Rev. Drug Discovery, 2006, 5, 210–218 CrossRef CAS PubMed
.
- A. J. Demello, Nature, 2006, 442, 394–402 CrossRef CAS PubMed
.
- J. M. Ayuso, M. Virumbrales-Muñoz, J. M. Lang and D. J. Beebe, Nat. Commun., 2022, 13, 3086 CrossRef CAS PubMed
.
- Y. Deng, Z. Bai and R. Fan, Nat. Rev. Bioeng., 2023, 1, 769–784 CrossRef
.
- T. A. Duncombe, A. M. Tentori and A. E. Herr, Nat. Rev. Mol. Cell Biol., 2015, 16, 554–567 CrossRef CAS PubMed
.
- F. Marofi, R. Motavalli, V. A. Safonov, L. Thangavelu, A. V. Yumashev, M. Alexander, N. Shomali, M. S. Chartrand, Y. Pathak and M. Jarahian, Stem Cell Res. Ther., 2021, 12, 1–16 CrossRef PubMed
.
- R. C. Sterner and R. M. Sterner, Blood Cancer J., 2021, 11, 69 CrossRef PubMed
.
- A. Mitra, A. Barua, L. Huang, S. Ganguly, Q. Feng and B. He, Front. Immunol., 2023, 14, 1188049 CrossRef CAS PubMed
.
- M. Sato, S. Goto, R. Kaneko, M. Ito, S. Sato and S. Takeuchi, Anticancer Res., 1998, 18, 3951–3955 CAS
.
- S. K. Panda and B. Ravindran, Bio-Protoc., 2013, 3, e323 Search PubMed
.
- D. J. Powell Jr, A. L. Brennan, Z. Zheng, H. Huynh, J. Cotte and B. L. Levine, Cytotherapy, 2009, 11, 923–935 CrossRef PubMed
.
- D. F. Stroncek, V. Fellowes, C. Pham, H. Khuu, D. H. Fowler, L. V. Wood and M. Sabatino, J. Transl. Med., 2014, 12, 1–8 CrossRef PubMed
.
- D. F. Stroncek, D. W. Lee, J. Ren, M. Sabatino, S. Highfill, H. Khuu, N. N. Shah, R. N. Kaplan, T. J. Fry and C. L. Mackall, J. Transl. Med., 2017, 15, 1–8 CrossRef PubMed
.
- M. Ruella, J. Xu, D. M. Barrett, J. A. Fraietta, T. J. Reich, D. E. Ambrose, M. Klichinsky, O. Shestova, P. R. Patel and I. Kulikovskaya, Nat. Med., 2018, 24, 1499–1503 CrossRef CAS PubMed
.
- E. Noaks, C. Peticone, E. Kotsopoulou and D. G. Bracewell, Mol. Ther.--Methods Clin. Dev., 2021, 20, 675–687 CrossRef CAS PubMed
.
- S. Miltenyi, W. Müller, W. Weichel and A. Radbruch, Cytometry, 1990, 11, 231–238 CrossRef CAS PubMed
.
- W. Bonner, H. Hulett, R. Sweet and L. Herzenberg, Rev. Sci. Instrum., 1972, 43, 404–409 CrossRef CAS PubMed
.
-
K. Milward, J. Hester and K. J. Wood, Immunological Tolerance: Methods and Protocols, 2019, vol. 1899, pp. 43–54 Search PubMed
.
- A. Moller, E. Dickmeiss, C. Geisler and L. Christensen, J. Hematother. Stem Cell Res., 2001, 10, 837–853 CrossRef CAS PubMed
.
- S. K. Bedoya, T. D. Wilson, E. L. Collins, K. Lau and J. Larkin III, J. Visualized Exp., 2013, e50765 Search PubMed
.
- U. Mock, L. Nickolay, B. Philip, G. W.-K. Cheung, H. Zhan, I. C. Johnston, A. D. Kaiser, K. Peggs, M. Pule and A. J. Thrasher, Cytotherapy, 2016, 18, 1002–1011 CrossRef CAS PubMed
.
- W. Chen, N. T. Huang, B. Oh, R. H. Lam, R. Fan, T. T. Cornell, T. P. Shanley, K. Kurabayashi and J. Fu, Adv. Healthcare Mater., 2013, 2, 965–975 CrossRef CAS PubMed
.
- D. J. Kinahan, S. M. Kearney, N. A. Kilcawley, P. L. Early, M. T. Glynn and J. Ducree, PLoS One, 2016, 11, e0155545 CrossRef PubMed
.
- Y. Sun and P. Sethu, Biomed. Microdevices, 2018, 20, 1–10 CrossRef CAS PubMed
.
- Y. Sun and P. Sethu, Biomed. Microdevices, 2018, 20, 77 CrossRef PubMed
.
- J. Darabi and C. Guo, Biomicrofluidics, 2013, 7, 054106 CrossRef PubMed
.
- P. L. Chiu, C. H. Chang, Y. L. Lin, P. H. Tsou and B. R. Li, Sci. Rep., 2019, 9, 8145 CrossRef PubMed
.
- R. D. Fennell, M. Sher and W. Asghar, Biosensors, 2021, 12, 12 CrossRef PubMed
.
- A. E. Rosenbach, P. Koria, J. Goverman, K. T. Kotz, A. Gupta, M. Yu, S. P. Fagan, D. Irimia and R. G. Tompkins, Clin. Transl. Sci., 2011, 4, 63–68 CrossRef CAS PubMed
.
- V. I. Furdui and D. J. Harrison, Lab Chip, 2004, 4, 614–618 RSC
.
- M. Sher and W. Asghar, Biosens. Bioelectron., 2019, 142, 111490 CrossRef CAS PubMed
.
- L. R. Huang, E. C. Cox, R. H. Austin and J. C. Sturm, Science, 2004, 304, 987–990 CrossRef CAS PubMed
.
- J. Voldman, Annu. Rev. Biomed. Eng., 2006, 8, 425–454 CrossRef CAS PubMed
.
- F. Petersson, L. Aberg, A. M. Swärd-Nilsson and T. Laurell, Anal. Chem., 2007, 79, 5117–5123 CrossRef CAS PubMed
.
- B. L. Levine, Cancer Gene Ther., 2015, 22, 79–84 CrossRef CAS PubMed
.
- B. L. Levine, W. B. Bernstein, M. Connors, N. Craighead, T. Lindsten, C. B. Thompson and C. H. June, J. Immunol., 1997, 159, 5921–5930 CrossRef CAS
.
- L. S. Hami, C. Green, N. Leshinsky, E. Markham, K. Miller and S. Craig, Cytotherapy, 2004, 6, 554–562 CrossRef CAS PubMed
.
- A. Trickett and Y. L. Kwan, J. Immunol. Methods, 2003, 275, 251–255 CrossRef CAS PubMed
.
- D. Hollyman, J. Stefanski, M. Przybylowski, S. Bartido, O. Borquez-Ojeda, C. Taylor, R. Yeh, V. Capacio, M. Olszewska, J. Hosey, M. Sadelain, R. J. Brentjens and I. Rivière, J. Immunother., 2009, 32, 169–180 CrossRef CAS PubMed
.
- J. H. Cho, H. O. Kim, K. S. Kim, D. H. Yang, C. D. Surh and J. Sprent, J. Immunol., 2013, 191, 5559–5573 CrossRef CAS PubMed
.
- B. Dura, S. K. Dougan, M. Barisa, M. M. Hoehl, C. T. Lo, H. L. Ploegh and J. Voldman, Nat. Commun., 2015, 6, 5940 CrossRef CAS PubMed
.
- J. P. Frimat, M. Becker, Y. Y. Chiang, U. Marggraf, D. Janasek, J. G. Hengstler, J. Franzke and J. West, Lab Chip, 2011, 11, 231–237 RSC
.
- S. Hong, Q. Pan and L. P. Lee, Integr. Biol., 2012, 4, 374–380 CrossRef CAS PubMed
.
- A. M. Skelley, O. Kirak, H. Suh, R. Jaenisch and J. Voldman, Nat. Methods, 2009, 6, 147–152 CrossRef CAS PubMed
.
- P. Friedl and M. Gunzer, Trends Immunol., 2001, 22, 187–191 CrossRef CAS PubMed
.
- C. Chan, J. Stark and A. J. George, J. Comput. Appl. Math., 2005, 184, 101–120 CrossRef
.
- J. C. Park, H. Bernstein, S. Loughhead, R. Zwirtes, J. Jennings, V. Nicolini, C. Klein, L. C. Deak, P. Umana, C. Trumpfheller and A. Sharei, Immuno-Oncol. Technol., 2022, 16, 100091 CrossRef CAS PubMed
.
- P. Vormittag, R. Gunn, S. Ghorashian and F. S. Veraitch, Curr. Opin. Biotechnol., 2018, 53, 164–181 CrossRef CAS PubMed
.
- R. J. Brentjens, I. Rivière, J. H. Park, M. L. Davila, X. Wang, J. Stefanski, C. Taylor, R. Yeh, S. Bartido, O. Borquez-Ojeda, M. Olszewska, Y. Bernal, H. Pegram, M. Przybylowski, D. Hollyman, Y. Usachenko, D. Pirraglia, J. Hosey, E. Santos, E. Halton, P. Maslak, D. Scheinberg, J. Jurcic, M. Heaney, G. Heller, M. Frattini and M. Sadelain, Blood, 2011, 118, 4817–4828 CrossRef CAS PubMed
.
- C. Elsner and J. Bohne, Virus Genes, 2017, 53, 714–722 CrossRef CAS PubMed
.
- A. I. Salter, M. J. Pont and S. R. Riddell, Blood, 2018, 131, 2621–2629 CrossRef CAS PubMed
.
- X. Dai, J. J. Park, Y. Du, H. R. Kim, G. Wang, Y. Errami and S. Chen, Nat. Methods, 2019, 16, 247–254 CrossRef CAS PubMed
.
- J. Park, S. Inwood, S. Kruthiventi, J. Jenkins, J. Shiloach and M. Betenbaugh, Curr. Opin. Chem. Eng., 2018, 22, 128–137 CrossRef
.
- W. S. Pear, G. P. Nolan, M. L. Scott and D. Baltimore, Proc. Natl. Acad. Sci., 1993, 90, 8392–8396 CrossRef CAS PubMed
.
-
A. Okuma, in Mammalian Cell Engineering: Methods and Protocols, Springer, 2021, pp. 3–14 Search PubMed
.
- M. K. Cromer, S. Vaidyanathan, D. E. Ryan, B. Curry, A. B. Lucas, J. Camarena, M. Kaushik, S. R. Hay, R. M. Martin, I. Steinfeld, R. O. Bak, D. P. Dever, A. Hendel, L. Bruhn and M. H. Porteus, Mol. Ther., 2018, 26, 2431–2442 CrossRef CAS PubMed
.
- T. DiTommaso, J. M. Cole, L. Cassereau, J. A. Buggé, J. L. S. Hanson, D. T. Bridgen, B. D. Stokes, S. M. Loughhead, B. A. Beutel, J. B. Gilbert, K. Nussbaum, A. Sorrentino, J. Toggweiler, T. Schmidt, G. Gyuelveszi, H. Bernstein and A. Sharei, Proc. Natl. Acad. Sci., 2018, 115, E10907–E10914 CrossRef CAS PubMed
.
- A. Tay and N. Melosh, Adv. Ther., 2019, 2, 1900133 CrossRef CAS PubMed
.
- Z. Li, J. Düllmann, B. Schiedlmeier, M. Schmidt, C. Von Kalle, J. Meyer, M. Forster, C. Stocking, A. Wahlers, O. Frank, W. Ostertag, K. Kühlcke, H. G. Eckert, B. Fehse and C. Baum, Science, 2002, 296, 497–497 CrossRef CAS PubMed
.
- M. P. Stewart, A. Sharei, X. Ding, G. Sahay, R. Langer and K. F. Jensen, Nature, 2016, 538, 183–192 CrossRef CAS PubMed
.
- A. B. V. Quach, S. R. Little and S. C. C. Shih, Anal. Chem., 2022, 94, 4039–4047 CrossRef CAS PubMed
.
- U. O'Doherty, W. J. Swiggard and M. H. Malim, J. Virol., 2000, 74, 10074–10080 CrossRef PubMed
.
- J. Guo, W. Wang, D. Yu and Y. Wu, J. Virol., 2011, 85, 9824–9833 CrossRef CAS PubMed
.
- H. Liu, Y. Hung, S. D. Wissink and C. M. Verfaillie, Leukemia, 2000, 14, 307–311 CrossRef CAS PubMed
.
- H. E. Davis, J. R. Morgan and M. L. Yarmush, Biophys. Chem., 2002, 97, 159–172 CrossRef CAS PubMed
.
- X. J. Li, A. V. Valadez, P. Zuo and Z. Nie, Bioanalysis, 2012, 4, 1509–1525 CrossRef CAS PubMed
.
- G. M. Nocera, G. Viscido, S. Criscuolo, S. Brillante, F. Carbone, L. Staiano, S. Carrella and D. di Bernardo, Commun. Biol., 2022, 5, 1034 CrossRef PubMed
.
- A. D. Castiaux, D. M. Spence and R. S. Martin, Anal. Methods, 2019, 11, 4220–4232 RSC
.
- J. S. Kuo and D. T. Chiu, Annu. Rev. Anal. Chem., 2011, 4, 275–296 CrossRef CAS PubMed
.
- H. N. Vu, Y. Li, M. Casali, D. Irimia, Z. Megeed and M. L. Yarmush, Lab Chip, 2008, 8, 75–80 RSC
.
- E. Cimetta, M. Franzoso, M. Trevisan, E. Serena, A. Zambon, S. Giulitti, L. Barzon and N. Elvassore, Biomicrofluidics, 2012, 6, 24127–2412712 CrossRef PubMed
.
- P. N. Silva, Z. Atto, R. Regeenes, U. Tufa, Y. Y. Chen, W. C. Chan, A. Volchuk, D. M. Kilkenny and J. V. Rocheleau, Lab Chip, 2016, 16, 2921–2934 RSC
.
- N. Moore, J. R. Chevillet, L. J. Healey, C. McBrine, D. Doty, J. Santos, B. Teece, J. Truslow, V. Mott, P. Hsi, V. Tandon, J. T. Borenstein, J. Balestrini and K. Kotz, Sci. Rep., 2019, 9, 15101 CrossRef PubMed
.
- R. Tran, D. R. Myers, G. Denning, J. E. Shields, A. M. Lytle, H. Alrowais, Y. Qiu, Y. Sakurai, W. C. Li, O. Brand, J. M. Le Doux, H. T. Spencer, C. B. Doering and W. A. Lam, Mol. Ther., 2017, 25, 2372–2382 CrossRef CAS PubMed
.
- A. R. K. Kumar, Y. Shou, B. Chan and A. Tay, Adv. Mater., 2021, 33, 2007421 CrossRef CAS PubMed
.
- M. P. Stewart, R. Langer and K. F. Jensen, Chem. Rev., 2018, 118, 7409–7531 CrossRef CAS PubMed
.
- X. Xie, A. M. Xu, S. Leal-Ortiz, Y. Cao, C. C. Garner and N. A. Melosh, ACS Nano, 2013, 7, 4351–4358 CrossRef CAS PubMed
.
- A. Tay and N. Melosh, Small, 2021, 17, 2103198 CrossRef CAS PubMed
.
- A. K. Shalek, J. T. Gaublomme, L. Wang, N. Yosef, N. Chevrier, M. S. Andersen, J. T. Robinson, N. Pochet, D. Neuberg, R. S. Gertner, I. Amit, J. R. Brown, N. Hacohen, A. Regev, C. J. Wu and H. Park, Nano Lett., 2012, 12, 6498–6504 CrossRef CAS PubMed
.
- Y. Chen, S. Aslanoglou, G. Gervinskas, H. Abdelmaksoud, N. H. Voelcker and R. Elnathan, Small, 2019, 15, 1904819 CrossRef CAS PubMed
.
- C. Zhao, T. Man, X. Xu, Q. Yang, W. Liu, S. J. Jonas, M. A. Teitell, P.-Y. Chiou and P. S. Weiss, ACS Mater. Lett., 2020, 2, 1475–1483 CrossRef CAS PubMed
.
- A. Tay, ACS Nano, 2020, 14, 7714–7721 CrossRef CAS PubMed
.
- C. E. Thomas, A. Ehrhardt and M. A. Kay, Nat. Rev. Genet., 2003, 4, 346–358 CrossRef CAS PubMed
.
- N. Sandoval-Villegas, W. Nurieva, M. Amberger and Z. Ivics, Int. J. Mol. Sci., 2021, 22, 5084 CrossRef CAS PubMed
.
- Z. Ivics, P. B. Hackett, R. H. Plasterk and Z. Izsvák, Cell, 1997, 91, 501–510 CrossRef CAS PubMed
.
- K. Woltjen, I. P. Michael, P. Mohseni, R. Desai, M. Mileikovsky, R. Hämäläinen, R. Cowling, W. Wang, P. Liu, M. Gertsenstein, K. Kaji, H. K. Sung and A. Nagy, Nature, 2009, 458, 766–770 CrossRef CAS PubMed
.
- S. Depil, P. Duchateau, S. A. Grupp, G. Mufti and L. Poirot, Nat. Rev. Drug Discovery, 2020, 19, 185–199 CrossRef CAS PubMed
.
- R. R. Beerli and C. F. Barbas III, Nat. Biotechnol., 2002, 20, 135–141 CrossRef CAS PubMed
.
- J. Boch, H. Scholze, S. Schornack, A. Landgraf, S. Hahn, S. Kay, T. Lahaye, A. Nickstadt and U. Bonas, Science, 2009, 326, 1509–1512 CrossRef CAS PubMed
.
- T. Gaj, C. A. Gersbach and C. F. Barbas III, Trends Biotechnol., 2013, 31, 397–405 CrossRef CAS PubMed
.
- M. Jinek, K. Chylinski, I. Fonfara, M. Hauer, J. A. Doudna and E. Charpentier, Science, 2012, 337, 816–821 CrossRef CAS PubMed
.
- D. B. T. Cox, R. J. Platt and F. Zhang, Nat. Med., 2015, 21, 121–131 CrossRef CAS PubMed
.
- S. H. Khan, Mol. Ther.–Nucleic Acids, 2019, 16, 326–334 CrossRef CAS PubMed
.
- Y. X. Deng, M. Kizer, M. Rada, J. Sage, X. Wang, D. J. Cheon and A. J. Chung, Nano Lett., 2018, 18, 2705–2710 CrossRef CAS PubMed
.
- E. Neumann, M. Schaefer-Ridder, Y. Wang and P. Hofschneider, EMBO J., 1982, 1, 841–845 CrossRef CAS PubMed
.
- M. S. Venslauskas and S. Šatkauskas, Eur. Biophys. J., 2015, 44, 277–289 CrossRef PubMed
.
- Y. Zhao, E. Moon, C. Carpenito, C. M. Paulos, X. Liu, A. L. Brennan, A. Chew, R. G. Carroll, J. Scholler, B. L. Levine, S. M. Albelda and C. H. June, Cancer Res., 2010, 70(22), 9053–9061 CrossRef CAS PubMed
.
- D. M. Barrett, Y. Zhao, X. Liu, S. Jiang, C. Carpenito, M. Kalos, R. G. Carroll, C. H. June and S. A. Grupp, Hum. Gene Ther., 2011, 22, 1575–1586 CrossRef CAS PubMed
.
- J. Svoboda, S. R. Rheingold, S. I. Gill, S. A. Grupp, S. F. Lacey, I. Kulikovskaya, M. M. Suhoski, J. J. Melenhorst, B. Loudon, A. R. Mato, S. D. Nasta, D. J. Landsburg, M. R. Youngman, B. L. Levine, D. L. Porter, C. H. June and S. J. Schuster, Blood, 2018, 132, 1022–1026 CrossRef CAS PubMed
.
- G. Doenz, S. Dorn, N. Aghaallaei, B. Bajoghli, E. Riegel, M. Aigner, H. Bock, B. Werner, T. Lindhorst and T. Czerny, BMC Biotechnol., 2018, 18, 1 CrossRef PubMed
.
- H. Singh, P. R. Manuri, S. Olivares, N. Dara, M. J. Dawson, H. Huls, P. B. Hackett, D. B. Kohn, E. J. Shpall, R. E. Champlin and L. J. N. Cooper, Cancer Res., 2008, 68, 2961–2971 CrossRef CAS PubMed
.
- R. Monjezi, C. Miskey, T. Gogishvili, M. Schleef, M. Schmeer, H. Einsele, Z. Ivics and M. Hudecek, Leukemia, 2017, 31, 186–194 CrossRef CAS PubMed
.
- D. Morita, N. Nishio, S. Saito, M. Tanaka, N. Kawashima, Y. Okuno, S. Suzuki, K. Matsuda, Y. Maeda and M. H. Wilson, Mol. Ther., 2018, 8, 131–140 CAS
.
- A. Chang, T. Dao, A. Scott, L. Dubrovsky, C. Liu and D. A. Scheinberg, Blood, 2015, 126, 2527 CrossRef
.
- L. Poirot, B. Philip, C. Schiffer-Mannioui, D. Le Clerre, I. Chion-Sotinel, S. Derniame, P. Potrel, C. Bas, L. Lemaire, R. Galetto, C. Lebuhotel, J. Eyquem, G. W. Cheung, A. Duclert, A. Gouble, S. Arnould, K. Peggs, M. Pule, A. M. Scharenberg and J. Smith, Cancer Res., 2015, 75, 3853–3864 CrossRef CAS PubMed
.
- A. K. Fajrial, Q. Q. He, N. I. Wirusanti, J. E. Slansky and X. Y. Ding, Theranostics, 2020, 10, 5532–5549 CrossRef CAS PubMed
.
- X. Liu, Y. Zhang, C. Cheng, A. W. Cheng, X. Zhang, N. Li, C. Xia, X. Wei, X. Liu and H. Wang, Cell Res., 2017, 27, 154–157 CrossRef PubMed
.
- X. M. Wu, Z. K. Gu, Y. Chen, B. R. Chen, W. Chen, L. Q. Weng and X. L. Liu, Comput. Struct. Biotechnol. J., 2019, 17, 661–674 CrossRef CAS PubMed
.
- T. B. Napotnik, T. Polajzer and D. Miklavcic, Bioelectrochemistry, 2021, 141, 107871 CrossRef PubMed
.
-
Z. Dong, T. Yang, H. Wu, J. Brooks, R. Yang, Y. Huang and L. Chang, in Micro and Nanosystems for Biophysical Studies of Cells and Small Organisms, Elsevier, 2021, pp. 105–123 Search PubMed
.
- M. B. Fox, D. C. Esveld, A. Valero, R. Luttge, H. C. Mastwijk, P. V. Bartels, A. Van Den
Berg and R. M. Boom, Anal. Bioanal. Chem., 2006, 385, 474–485 CrossRef CAS PubMed
.
- J. A. Kim, K. Cho, M. S. Shin, W. G. Lee, N. Jung, C. Chung and J. K. Chang, Biosens. Bioelectron., 2008, 23, 1353–1360 CrossRef CAS PubMed
.
- K. Nolkrantz, C. Farre, A. Brederlau, R. I. D. Karlsson, C. Brennan, P. S. Eriksson, S. G. Weber, M. Sandberg and O. Orwar, Anal. Chem., 2001, 73, 4469–4477 CrossRef CAS PubMed
.
- I. Zudans, A. Agarwal, O. Orwar and S. G. Weber, Biophys. J., 2007, 92, 3696–3705 CrossRef CAS PubMed
.
- M. Y. Wang, O. Orwar and S. G. Weber, Anal. Chem., 2009, 81, 4060–4067 CrossRef CAS PubMed
.
- M. Hale, B. Lee, Y. Honaker, W.-H. Leung, A. E. Grier, H. M. Jacobs, K. Sommer, J. Sahni, S. W. Jackson and A. M. Scharenberg, Mol. Ther., 2017, 4, 192–203 CAS
.
- L. H. Li, R. Shivakumar, S. Feller, C. Allen, J. M. Weiss, S. Dzekunov, V. Singh, J. Holaday, J. Fratantoni and L. N. Liu, Technol. Cancer Res. Treat., 2002, 1, 341–350 CrossRef CAS PubMed
.
- J. D. Beane, G. Lee, Z. Zheng, M. Mendel, D. Abate-Daga, M. Bharathan, M. Black, N. Gandhi, Z. Yu, S. Chandran, M. Giedlin, D. Ando, J. Miller, D. Paschon, D. Guschin, E. J. Rebar, A. Reik, M. C. Holmes, P. D. Gregory, N. P. Restifo, S. A. Rosenberg, R. A. Morgan and S. A. Feldman, Mol. Ther., 2015, 23, 1380–1390 CrossRef CAS PubMed
.
- E. A. Stadtmauer, J. A. Fraietta, M. M. Davis, A. D. Cohen, K. L. Weber, E. Lancaster, P. A. Mangan, I. Kulikovskaya, M. Gupta, F. Chen, L. Tian, V. E. Gonzalez, J. Xu, I. Y. Jung, J. J. Melenhorst, G. Plesa, J. Shea, T. Matlawski, A. Cervini, A. L. Gaymon, S. Desjardins, A. Lamontagne, J. Salas-Mckee, A. Fesnak, D. L. Siegel, B. L. Levine, J. K. Jadlowsky, R. M. Young, A. Chew, W. T. Hwang, E. O. Hexner, B. M. Carreno, C. L. Nobles, F. D. Bushman, K. R. Parker, Y. Qi, A. T. Satpathy, H. Y. Chang, Y. Zhao, S. F. Lacey and C. H. June, Science, 2020, 367, eaba7365 CrossRef CAS PubMed
.
- MaxCyt Enables a CGMP-Compatible Manufacturing Process for Highly Efficient T Cell Engineering, DOI: https://maxcyte.com/resource/maxcyte-enables-a-cgmp-compatible-manufacturing-process-for-highly-efficient-t-cell-engineering/.
- C. A. Lissandrello, J. A. Santos, P. Hsi, M. Welch, V. L. Mott, E. S. Kim, J. Chesin, N. J. Haroutunian, A. G. Stoddard, A. Czarnecki, J. R. Coppeta, D. K. Freeman, D. A. Flusberg, J. L. Balestrini and V. Tandon, Sci. Rep., 2020, 10, 18045 CrossRef CAS PubMed
.
- A. G. Niculescu, C. Chircov, A. C. Bîrcă and A. M. Grumezescu, Int. J. Mol. Sci., 2021, 22, 2011 CrossRef CAS PubMed
.
-
J. M. Sido, J. B. Hemphill, R. N. McCormack, R. D. Beighley, B. F. Grant, C. R. Buie and P. A. Garcia, bioRxiv, 2021, preprint, DOI:10.1101/2021.10.26.465897.
- J. Hur, H. Kim, U. Kim, G. B. Kim, J. Kim, B. Joo, D. Cho, D. S. Lee and A. J. Chung, Nano Lett., 2023, 23, 7341–7349 CrossRef CAS PubMed
.
- A. D. Bangham, M. M. Standish and J. C. Watkins, J. Mol. Biol., 1965, 13, 238–252 CrossRef CAS PubMed
.
- R. Tenchov, R. Bird, A. E. Curtze and Q. Zhou, ACS Nano, 2021, 15, 16982–17015 CrossRef CAS PubMed
.
- X. C. Hou, T. Zaks, R. Langer and Y. Z. Dong, Nat. Rev. Mater., 2021, 6, 1078–1094 CrossRef CAS PubMed
.
- M. J. Byun, J. Lim, S. N. Kim, D. H. Park, T. H. Kim, W. Park and C. G. Park, BioChip J., 2022, 16, 128–145 CrossRef CAS PubMed
.
- A. Akinc, M. A. Maier, M. Manoharan, K. Fitzgerald, M. Jayaraman, S. Barros, S. Ansell, X. Du, M. J. Hope, T. D. Madden, B. L. Mui, S. C. Semple, Y. K. Tam, M. Ciufolini, D. Witzigmann, J. A. Kulkarni, R. van der Meel and P. R. Cullis, Nat. Nanotechnol., 2019, 14, 1084–1087 CrossRef CAS PubMed
.
- X. Wang, S. Liu, Y. Sun, X. Yu, S. M. Lee, Q. Cheng, T. Wei, J. Gong, J. Robinson, D. Zhang, X. Lian, P. Basak and D. J. Siegwart, Nat. Protoc., 2023, 18, 265–291 CrossRef CAS PubMed
.
- D. Sun and Z. R. Lu, Pharm. Res., 2023, 40, 27–46 CrossRef CAS PubMed
.
- M. Hajiaghapour Asr, F. Dayani, F. Saedi Segherloo, A. Kamedi, A. O. Neill, R. MacLoughlin and M. Doroudian, Pharmaceutics, 2023, 15, 1127 CrossRef CAS PubMed
.
-
L. Zheng, S. R. Bandara and C. Leal, bioRxiv, 2022, DOI:10.1101/2022.05.20.492895.
- D. Chen, K. T. Love, Y. Chen, A. A. Eltoukhy, C. Kastrup, G. Sahay, A. Jeon, Y. Dong, K. A. Whitehead and D. G. Anderson, J. Am. Chem. Soc., 2012, 134, 6948–6951 CrossRef CAS PubMed
.
- B. G. Carvalho, B. T. Ceccato, M. Michelon, S. W. Han and L. G. de la Torre, Pharmaceutics, 2022, 14, 141 CrossRef CAS PubMed
.
- M. Maeki, T. Saito, Y. Sato, T. Yasui, N. Kaji, A. Ishida, H. Tani, Y. Baba, H. Harashima and M. Tokeshi, RSC Adv., 2015, 5, 46181–46185 RSC
.
- M. M. Billingsley, N. Singh, P. Ravikumar, R. Zhang, C. H. June and M. J. Mitchell, Nano Lett., 2020, 20, 1578–1589 CrossRef CAS PubMed
.
- M. M. Billingsley, A. G. Hamilton, D. Mai, S. K. Patel, K. L. Swingle, N. C. Sheppard, C. H. June and M. J. Mitchell, Nano Lett., 2022, 22, 533–542 CrossRef CAS PubMed
.
- Z. Ye, J. Chen, X. Zhao, Y. Li, J. Harmon, C. Huang, J. Chen and Q. Xu, ACS Biomater. Sci. Eng., 2022, 8, 722–733 CrossRef CAS PubMed
.
- M. Maeki, S. Uno, A. Niwa, Y. Okada and M. Tokeshi, J. Controlled Release, 2022, 344, 80–96 CrossRef CAS PubMed
.
- J. D. Finn, A. R. Smith, M. C. Patel, L. Shaw, M. R. Youniss, J. van Heteren, T. Dirstine, C. Ciullo, R. Lescarbeau, J. Seitzer, R. R. Shah, A. Shah, D. D. Ling, J. Growe, M. Pink, E. Rohde, K. M. Wood, W. E. Salomon, W. F. Harrington, C. Dombrowski, W. R. Strapps, Y. Chang and D. V. Morrissey, Cell Rep., 2018, 22, 2227–2235 CrossRef CAS PubMed
.
- S. J. Shepherd, D. Issadore and M. J. Mitchell, Biomaterials, 2021, 274, 120826 CrossRef CAS PubMed
.
- A. K. Shalek, J. T. Robinson, E. S. Karp, J. S. Lee, D.-R. Ahn, M.-H. Yoon, A. Sutton, M. Jorgolli, R. S. Gertner, T. S. Gujral, G. MacBeath, E. G. Yang and H. Park, Proc. Natl. Acad. Sci., 2010, 107, 1870–1875 CrossRef CAS PubMed
.
- P. L. McNeil, R. F. Murphy, F. Lanni and D. L. Taylor, J. Cell Biol., 1984, 98, 1556–1564 CrossRef CAS PubMed
.
- I. Lentacker, I. De Cock, R. Deckers, S. C. De Smedt and C. T. Moonen, Adv. Drug Delivery Rev., 2014, 72, 49–64 CrossRef CAS PubMed
.
- R. Xiong, S. K. Samal, J. Demeester, A. G. Skirtach, S. C. De Smedt and K. Braeckmans, Adv. Phys.: X, 2016, 1, 596–620 CAS
.
- A. Sharei, J. Zoldan, A. Adamo, W. Y. Sim, N. Cho, E. Jackson, S. Mao, S. Schneider, M.-J. Han, A. Lytton-Jean, P. A. Basto, S. Jhunjhunwala, J. Lee, D. A. Heller, J. W. Kang, G. C. Hartoularos, K. Kim, D. G. Anderson, R. Langer and K. F. Jensen, Proc. Natl. Acad. Sci., 2013, 110, 2082–2087 CrossRef CAS PubMed
.
- T. DiTommaso, J. M. Cole, L. Cassereau, J. A. Bugge, J. L. S. Hanson, D. T. Bridgen, B. D. Stokes, S. M. Loughhead, B. A. Beutel, J. B. Gilbert, K. Nussbaum, A. Sorrentino, J. Toggweiler, T. Schmidt, G. Gyuelveszi, H. Bernstein and A. Sharei, Proc. Natl. Acad. Sci. U. S. A., 2018, 115, E10907–E10914 CrossRef CAS PubMed
.
- X. Ding, M. P. Stewart, A. Sharei, J. C. Weaver, R. S. Langer and K. F. Jensen, Nat. Biomed. Eng., 2017, 1, 0039 CrossRef CAS PubMed
.
- J. Li, B. Wang, B. M. Juba, M. Vazquez, S. W. Kortum, B. S. Pierce, M. Pacheco, L. Roberts, J. W. Strohbach, L. H. Jones, E. Hett, A. Thorarensen, J. B. Telliez, A. Sharei, M. Bunnage and J. B. Gilbert, ACS Chem. Biol., 2017, 12, 2970–2974 CrossRef CAS PubMed
.
- X. Han, Z. Liu, M. C. Jo, K. Zhang, Y. Li, Z. Zeng, N. Li, Y. Zu and L. Qin, Sci. Adv., 2015, 1, e1500454 CrossRef PubMed
.
- B. Joo, J. Hur, G. B. Kim, S. G. Yun and A. J. Chung, ACS Nano, 2021, 15, 12888–12898 CrossRef CAS PubMed
.
- A. Liu, M. Islam, N. Stone, V. Varadarajan, J. Jeong, S. Bowie, P. Qiu, E. K. Waller, A. Alexeev and T. Sulchek, Mater. Today, 2018, 21, 703–712 CrossRef CAS PubMed
.
- X. Han, Z. Liu, Y. Ma, K. Zhang and L. Qin, Adv. Biosyst., 2017, 1, 1600007 CrossRef PubMed
.
- C. Kwon and A. J. Chung, Lab Chip, 2023, 23, 1758–1767 RSC
.
- J. Hur, I. Park, K. M. Lim, J. Doh, S. G. Cho and A. J. Chung, ACS Nano, 2020, 14, 15094–15106 CrossRef CAS PubMed
.
- M. E. Kizer, Y. Deng, G. Kang, P. E. Mikael, X. Wang and A. J. Chung, Lab Chip, 2019, 19, 1747–1754 RSC
.
- G. Kang, D. W. Carlson, T. H. Kang, S. Lee, S. J. Haward, I. Choi, A. Q. Shen and A. J. Chung, ACS Nano, 2020, 14, 3048–3058 CrossRef CAS PubMed
.
- J. A. Jarrell, A. A. Twite, K. H. W. J. Lau, M. N. Kashani, A. A. Lievano, J. Acevedo, C. Priest, J. Nieva, D. Gottlieb and R. S. Pawell, Sci. Rep., 2019, 9, 3214 CrossRef PubMed
.
- N. Delouche, A. B. Schofield and H. Tabuteau, Soft Matter, 2020, 16, 9899–9907 RSC
.
- M. Raab, M. Gentili, H. de Belly, H. R. Thiam, P. Vargas, A. J. Jimenez, F. Lautenschlaeger, R. Voituriez, A. M. Lennon-Duménil, N. Manel and M. Piel, Science, 2016, 352, 359–362 CrossRef CAS PubMed
.
- S. R. Panch, S. K. Srivastava, N. Elavia, A. McManus, S. T. Liu, P. Jin, S. L. Highfill, X. B. Li, P. Dagur, J. N. Kochenderfer, T. J. Fry, C. L. Mackall, D. Lee, N. N. Shah and D. F. Stroncek, Mol. Ther., 2019, 27, 1275–1285 CrossRef CAS PubMed
.
- C. Liu, V. S. Ayyar, X. Zheng, W. Chen, S. Zheng, H. Mody, W. Wang, D. Heald, A. P. Singh and Y. Cao, Clin. Pharmacol. Ther., 2021, 109, 716–727 CrossRef CAS PubMed
.
- S. Joaquina, C. Forcados, B. Caulier, E. M. Inderberg and S. Wälchli, Front. Bioeng. Biotechnol., 2023, 11, 1207576 CrossRef PubMed
.
- S. Kiesgen, J. C. Messinger, N. K. Chintala, Z. Tano and P. S. Adusumilli, Nat. Protoc., 2021, 16, 1331–1342 CrossRef CAS PubMed
.
- N. Sinha, N. Subedi and J. Tel, Front. Immunol., 2018, 9, 2373 CrossRef PubMed
.
- Q. Xue, E. Bettini, P. Paczkowski, C. Ng, A. Kaiser, T. McConnell, O. Kodrasi, M. F. Quigley, J. Heath, R. Fan, S. Mackay, M. E. Dudley, S. H. Kassim and J. Zhou, J. Immunother. Cancer, 2017, 5, 85 CrossRef PubMed
.
- A. Mocciaro, T. L. Roth, H. M. Bennett, M. Soumillon, A. Shah, J. Hiatt, K. Chapman, A. Marson and G. Lavieu, Commun. Biol., 2018, 1, 41 CrossRef PubMed
.
- M. Jorgolli, T. Nevill, A. Winters, I. Chen, S. Chong, F. F. Lin, M. Mock, C. Chen, K. Le, C. Tan, P. Jess, H. Xu, A. Hamburger, J. Stevens, T. Munro, M. Wu, P. Tagari and L. P. Miranda, Biotechnol. Bioeng., 2019, 116, 2393–2411 CrossRef CAS PubMed
.
- Y. J. Yamanaka, C. T. Berger, M. Sips, P. C. Cheney, G. Alter and J. C. Love, Integr. Biol., 2012, 4, 1175–1184 CrossRef CAS PubMed
.
- B. Vanherberghen, P. E. Olofsson, E. Forslund, M. Sternberg-Simon, M. A. Khorshidi, S. Pacouret, K. Guldevall, M. Enqvist, K. J. Malmberg, R. Mehr and B. Önfelt, Blood, 2013, 121, 1326–1334 CrossRef CAS PubMed
.
- Y. Zhou, N. Shao, R. B. Bessa de Castro, P. Zhang, Y. Ma, X. Liu, F. Huang, R. F. Wang and L. Qin, Cell Rep., 2020, 31, 107574 CrossRef CAS PubMed
.
- K. Paterson, S. Paterson, T. Mulholland, S. B. Coffelt and M. Zagnoni, IEEE Open J. Eng. Med. Biol., 2022, 3, 86–95 Search PubMed
.
- L. Shang, Y. Cheng and Y. Zhao, Chem. Rev., 2017, 117, 7964–8040 CrossRef CAS PubMed
.
- J. L. Madrigal, N. G. Schoepp, L. F. Xu, C. S. Powell, C. L. Delley, C. A. Siltanen, J. Danao, M. Srinivasan, R. H. Cole and A. R. Abate, Proc. Natl. Acad. Sci. U. S. A., 2022, 119 Search PubMed
.
- K. U. Wong, J. Shi, P. Li, H. Wang, Y. Jia, C. Deng, L. Jiang and A. H. Wong, Antibiot. Ther., 2022, 5, 85–99 CAS
.
- V. Chokkalingam, J. Tel, F. Wimmers, X. Liu, S. Semenov, J. Thiele, C. G. Figdor and W. T. S. Huck, Lab Chip, 2013, 13, 4740–4744 RSC
.
- J. L. Madrigal, N. G. Schoepp, L. F. Xu, C. S. Powell, C. L. Delley, C. A. Siltanen, J. Danao, M. Srinivasan, R. H. Cole and A. R. Abate, Proc. Natl. Acad. Sci. U. S. A., 2022, 119, e2110867119 CrossRef CAS PubMed
.
- A. I. Segaliny, G. D. Li, L. S. Kong, C. Ren, X. M. Chen, J. K. Wang, D. Baltimore, G. K. Wu and W. A. Zhao, Lab Chip, 2018, 18, 3733–3749 RSC
.
- S. Antona, I. Platzman and J. P. Spatz, ACS Omega, 2020, 5, 24674–24683 CrossRef CAS PubMed
.
- L. B. Pinheiro, V. A. Coleman, C. M. Hindson, J. Herrmann, B. J. Hindson, S. Bhat and K. R. Emslie, Anal. Chem., 2012, 84, 1003–1011 CrossRef CAS PubMed
.
- C. M. Hindson, J. R. Chevillet, H. A. Briggs, E. N. Gallichotte, I. K. Ruf, B. J. Hindson, R. L. Vessella and M. Tewari, Nat. Methods, 2013, 10, 1003–1005 CrossRef CAS PubMed
.
- R. Haderbache, W. Warda, E. Hervouet, M. N. da Rocha, R. Trad, V. Allain, C. Nicod, C. Thieblemeont, N. Boissel, P. Varlet, I. Y. Agha, L. Bouquet, M. Guiot, F. Venet, P. Sujobert, X. Roussel, P. O. Rouzaire, D. Caillot, O. Casasnovas, J. C. Bories, E. Bachy, S. Caillat-Zucman, M. Deschamps and C. Ferrand, J. Transl. Med., 2021, 19, 265 CrossRef CAS PubMed
.
- B. Cacho-Díaz, D. R. García-Botello, T. Wegman-Ostrosky, G. Reyes-Soto, E. Ortiz-Sánchez and L. A. Herrera-Montalvo, J. Transl. Med., 2020, 18, 1 CrossRef PubMed
.
- Y. Zhao, H. Stepto and C. K. Schneider, Hum. Gene Ther: Methods., 2017, 28, 205–214 CrossRef CAS PubMed
.
- U. FDA, Guidance for Industry, 2020.
- A. Chen, Z. Velickovic and J. Rasko, Cytotherapy, 2020, 22, S142 CrossRef
.
- J. Cao, G. Wang, H. Cheng, C. Wei, K. Qi, W. Sang, L. Zhenyu, M. Shi, H. Li, J. Qiao, B. Pan, J. Zhao, Q. Wu, L. Zeng, M. Niu, G. Jing, J. Zheng and K. Xu, Am. J. Hematol., 2018, 93, 851–858 CrossRef CAS PubMed
.
- R. G. Majzner and C. L. Mackall, Cancer Discovery, 2018, 8, 1219–1226 CrossRef CAS PubMed
.
- H. J. Jackson, S. Rafiq and R. J. Brentjens, Nat. Rev. Clin. Oncol., 2016, 13, 370–383 CrossRef CAS PubMed
.
- M. Martinez and E. K. Moon, Front. Immunol., 2019, 10, 128 CrossRef CAS PubMed
.
- A. Rodriguez-Garcia, A. Palazon, E. Noguera-Ortega, D. J. Powell Jr and S. Guedan, Front. Immunol., 2020, 11, 1109 CrossRef CAS PubMed
.
- J. Yu, X. Wu, J. Yan, H. Yu, L. Xu, Z. Chi, X. Sheng, L. Si, C. Cui, J. Dai, M. Ma, T. Xu, Y. Kong and J. Guo, J. Hematol. Oncol., 2018, 11, 1 CrossRef CAS PubMed
.
- M. Z. Noman, M. Hasmim, Y. Messai, S. Terry, C. Kieda, B. Janji and S. Chouaib, Am. J. Physiol., 2015, 309, C569–C579 CrossRef PubMed
.
- Y. Togashi, K. Shitara and H. Nishikawa, Nat. Rev. Clin. Oncol., 2019, 16, 356–371 CrossRef CAS PubMed
.
- Z. Liu, Y. Zhang, N. Shen, J. Sun, Z. Tang and X. Chen, Adv. Drug Delivery Rev., 2022, 183, 114138 CrossRef CAS PubMed
.
- I. Caruana, B. Savoldo, V. Hoyos, G. Weber, H. Liu, E. S. Kim, M. M. Ittmann, D. Marchetti and G. Dotti, Nat. Med., 2015, 21, 524–529 CrossRef CAS PubMed
.
- O. U. Kawalekar, R. S. O'Connor, J. A. Fraietta, L. Guo, S. E. McGettigan, A. D. Posey Jr, P. R. Patel, S. Guedan, J. Scholler, B. Keith, N. W. Snyder, I. A. Blair, M. C. Milone and C. H. June, Immunity, 2016, 44, 380–390 CrossRef CAS PubMed
.
- S. Guedan, X. Chen, A. Madar, C. Carpenito, S. E. McGettigan, M. J. Frigault, J. Lee, A. D. Posey Jr, J. Scholler, N. Scholler, R. Bonneau and C. H. June, Blood, 2014, 124, 1070–1080 CrossRef CAS PubMed
.
- J. Cui, Q. Zhang, Q. Song, H. Wang, P. Dmitriev, M. Y. Sun, X. Cao, Y. Wang, L. Guo, I. H. Indig, J. S. Rosenblum, C. Ji, D. Cao, K. Yang, M. R. Gilbert, Y. Yao and Z. Zhuang, Neuro-Oncology, 2019, 21, 1436–1446 CrossRef CAS PubMed
.
- M. Kapałczyńska, T. Kolenda, W. Przybyła, M. Zajączkowska, A. Teresiak, V. Filas, M. Ibbs, R. Bliźniak, Ł. Łuczewski and K. Lamperska, Arch. Med. Sci., 2018, 14, 910–919 Search PubMed
.
- L. Meigs, L. Smirnova, C. Rovida, M. Leist and T. Hartung, ALTEX, 2018, 35, 275–305 CrossRef PubMed
.
- M. Wadman, Science, 2023, 379, 6628 Search PubMed
.
- C. M. Leung, P. De Haan, K. Ronaldson-Bouchard, G.-A. Kim, J. Ko, H. S. Rho, Z. Chen, P. Habibovic, N. L. Jeon, S. Takayama, M. L. Shuler, G. Vunjak-Novakovic, O. Frey, E. Verpoorte and Y. Toh, Nat. Rev. Methods Primers, 2022, 2, 33 CrossRef CAS
.
- X. Liu, J. Fang, S. Huang, X. Wu, X. Xie, J. Wang, F. Liu, M. Zhang, Z. Peng and N. Hu, Microsyst. Nanoeng., 2021, 7, 50 CrossRef CAS PubMed
.
- G. Huang, F. Li, X. Zhao, Y. Ma, Y. Li, M. Lin, G. Jin, T. J. Lu, G. M. Genin and F. Xu, Chem. Rev., 2017, 117, 12764–12850 CrossRef CAS PubMed
.
- Y. Yang, K. Wang, X. Gu and K. W. Leong, Engineering, 2017, 3, 36–54 CrossRef CAS PubMed
.
- E. C. González-Díaz and S. Varghese, Gels, 2016, 2, 20 CrossRef PubMed
.
- A. L. Harris, Nat. Rev. Cancer, 2002, 2, 38–47 CrossRef CAS PubMed
.
- S. Bhattacharya, K. Calar and P. de la Puente, J. Exp. Clin. Cancer Res., 2020, 39, 1–16 CrossRef PubMed
.
- K. Paterson, S. Zanivan, R. Glasspool, S. Coffelt and M. Zagnoni, Lab Chip, 2021, 21, 2306–2329 RSC
.
- M. Campisi, S. E. Shelton, M. Chen, R. D. Kamm, D. A. Barbie and E. H. Knelson, Cancers, 2022, 14, 3561 CrossRef CAS PubMed
.
- C. Jensen and Y. Teng, Front. Mol. Biosci., 2020, 7, 33 CrossRef CAS PubMed
.
- M. Pickl and C. H. Ries, Oncogene, 2009, 28, 461–468 CrossRef CAS PubMed
.
- X. Ma, C. Lee, T. Zhang, J. Cai, H. Wang, F. Jiang, Z. Wu, J. Xie, G. Jiang and Z. Li, J. Nanobiotechnol., 2022, 20, 1 CrossRef PubMed
.
- T. E. Schnalzger, M. H. de Groot, C. Zhang, M. H. Mosa, B. E. Michels, J. Röder, T. Darvishi, W. S. Wels and H. F. Farin, EMBO journal, 2019, 38, e100928 CrossRef PubMed
.
- P. Dillard, H. Köksal, E. M. Inderberg and S. Wälchli, J. Visualized Exp., 2018, 142, e58785 Search PubMed
.
- Y. Ando, J. M. Oh, W. Zhao, M. Tran and K. Shen, Cells, 2021, 10, 2201 CrossRef CAS PubMed
.
- S. Herter, L. Morra, R. Schlenker, J. Sulcova, L. Fahrni, I. Waldhauer, S. Lehmann, T. Reisländer, I. Agarkova, J. M. Kelm, C. Klein, P. Umana and M. Bacac, Cancer Immunol., Immunother., 2017, 66, 129–140 CrossRef CAS PubMed
.
- L. Wallstabe, C. Göttlich, L. C. Nelke, J. Kühnemundt, T. Schwarz, T. Nerreter, H. Einsele, H. Walles, G. Dandekar, S. L. Nietzer and M. Hudecek, JCI Insight, 2019, 4, e126345 CrossRef PubMed
.
- F. Jacob, G. L. Ming and H. Song, Nat. Protoc., 2020, 15, 4000–4033 CrossRef CAS PubMed
.
- C. H. Chang, Y. Wang, R. Li, D. L. Rossi, D. Liu, E. A. Rossi, T. M. Cardillo and D. M. Goldenberg, Cancer Res., 2017, 77, 5384–5394 CrossRef CAS PubMed
.
- V. Charwat, M. Rothbauer, S. F. Tedde, O. Hayden, J. J. Bosch, P. Muellner, R. Hainberger and P. Ertl, Anal. Chem., 2013, 85, 11471–11478 CrossRef CAS PubMed
.
-
A. Pavesi, A. T. Tan, M. B. Chen, G. Adriani, A. Bertoletti and R. D. Kamm, Using Microfluidics to Investigate Tumor Cell Extravasation and T-Cell Immunotherapies, Milan, Italy, 2015 Search PubMed.
- J. P. Layer, M. T. Kronmüller, T. Quast, D. van den Boorn-Konijnenberg, M. Effern, D. Hinze, K. Althoff, A. Schramm, F. Westermann, M. Peifer, G. Hartmann, T. Tüting, W. Kolanus, M. Fischer, J. Schulte and M. Hölzel, Oncoimmunology, 2017, 6, e1320626 CrossRef PubMed
.
- S. W. L. Lee, G. Adriani, E. Ceccarello, A. Pavesi, A. T. Tan, A. Bertoletti, R. D. Kamm and S. C. Wong, Front. Immunol., 2018, 9, 416 CrossRef PubMed
.
- J. Lee, S. E. Kim, D. Moon and J. Doh, Lab Chip, 2021, 21, 2142–2152 RSC
.
- Y. Ando, E. L. Siegler, H. P. Ta, G. E. Cinay, H. Zhou, K. A. Gorrell, H. Au, B. M. Jarvis, P. Wang and K. Shen, Adv. Healthcare Mater., 2019, 8, e1900001 CrossRef PubMed
.
- A. Pavesi, A. T. Tan, S. Koh, A. Chia, M. Colombo, E. Antonecchia, C. Miccolis, E. Ceccarello, G. Adriani, M. T. Raimondi, R. D. Kamm and A. Bertoletti, JCI Insight, 2017, 2, e89762 CrossRef PubMed
.
- H. Xie, J. W. Appelt and R. W. Jenkins, Cancers, 2021, 13, 6052 CrossRef CAS PubMed
.
- A. Han, J. Glanville, L. Hansmann and M. M. Davis, Nat. Biotechnol., 2014, 32, 684–692 CrossRef CAS PubMed
.
- M. J. T. Stubbington, O. Rozenblatt-Rosen, A. Regev and S. A. Teichmann, Science, 2017, 358, 58–63 CrossRef CAS PubMed
.
- M. De Simone, G. Rossetti and M. Pagani, Front. Immunol., 2018, 9, 1638 CrossRef PubMed
.
- L. Mazutis, J. Gilbert, W. L. Ung, D. A. Weitz, A. D. Griffiths and J. A. Heyman, Nat. Protoc., 2013, 8, 870–891 CrossRef CAS PubMed
.
- S. Y. Wang, Y. Liu, Y. J. Li, M. H. Lv, K. Gao, Y. He, W. B. Wei, Y. G. Zhu, X. Dong, X. Xu, Z. D. Li, L. Q. Liu and Y. Liu, Anal. Chem., 2022, 94, 918–926 CrossRef CAS PubMed
.
- Y. Wang, R. N. Jin, B. Q. Shen, N. Li, H. Zhou, W. Wang, Y. J. Zhao, M. S. Huang, P. Fang, S. S. Wang, P. Mary, R. K. Wang, P. X. Ma, R. N. Li, Y. J. Tian, Y. J. Cao, F. B. Li, L. Schweizer and H. K. Zhang, Sci. Adv., 2021, 7, eabe3839 CrossRef CAS PubMed
.
- A. M. Klein, L. Mazutis, I. Akartuna, N. Tallapragada, A. Veres, V. Li, L. Peshkin, D. A. Weitz and M. W. Kirschner, Cell, 2015, 161, 1187–1201 CrossRef CAS PubMed
.
- G. X. Y. Zheng, J. M. Terry, P. Belgrader, P. Ryvkin, Z. W. Bent, R. Wilson, S. B. Ziraldo, T. D. Wheeler, G. P. McDermott, J. J. Zhu, M. T. Gregory, J. Shuga, L. Montesclaros, J. G. Underwood, D. A. Masquelier, S. Y. Nishimura, M. Schnall-Levin, P. W. Wyatt, C. M. Hindson, R. Bharadwaj, A. Wong, K. D. Ness, L. W. Beppu, H. J. Deeg, C. McFarland, K. R. Loeb, W. J. Valente, N. G. Ericson, E. A. Stevens, J. P. Radich, T. S. Mikkelsen, B. J. Hindson and J. H. Bielas, Nat. Commun., 2017, 8, 14049 CrossRef CAS PubMed
.
- E. Z. Macosko, A. Basu, R. Satija, J. Nemesh, K. Shekhar, M. Goldman, I. Tirosh, A. R. Bialas, N. Kamitaki, E. M. Martersteck, J. J. Trombetta, D. A. Weitz, J. R. Sanes, A. K. Shalek, A. Regev and S. A. McCarroll, Cell, 2015, 161, 1202–1214 CrossRef CAS PubMed
.
- J. R. McDaniel, B. J. DeKosky, H. Tanno, A. D. Ellington and G. Georgiou, Nat. Protoc., 2016, 11, 429–442 CrossRef CAS PubMed
.
- A. H. C. Ng, S. M. Peng, A. M. Xu, W. J. Noh, K. Guo, M. T. Bethune, W. Chour, J. Choi, S. Yang, D. Baltimore and J. R. Heath, Lab Chip, 2019, 19, 3011–3021 RSC
.
- V. Chokkalingam, J. Tel, F. Wimmers, X. Liu, S. Semenov, J. Thiele, C. G. Figdor and W. T. Huck, Lab Chip, 2013, 13, 4740–4744 RSC
.
- A. I. Segaliny, G. Li, L. Kong, C. Ren, X. Chen, J. K. Wang, D. Baltimore, G. Wu and W. Zhao, Lab Chip, 2018, 18, 3733–3749 RSC
.
- Y. Wang, R. Jin, B. Shen, N. Li, H. Zhou, W. Wang, Y. Zhao, M. Huang, P. Fang, S. Wang, P. Mary, R. Wang, P. Ma, R. Li, Y. Tian, Y. Cao, F. Li, L. Schweizer and H. Zhang, Sci. Adv., 2021, 7, eabe3839 CrossRef CAS PubMed
.
- S. Wang, Y. Liu, Y. Li, M. Lv, K. Gao, Y. He, W. Wei, Y. Zhu, X. Dong, X. Xu, Z. Li, L. Liu and Y. Liu, Anal. Chem., 2022, 94, 918–926 CrossRef CAS PubMed
.
- G. X. Zheng, J. M. Terry, P. Belgrader, P. Ryvkin, Z. W. Bent, R. Wilson, S. B. Ziraldo, T. D. Wheeler, G. P. McDermott, J. Zhu, M. T. Gregory, J. Shuga, L. Montesclaros, J. G. Underwood, D. A. Masquelier, S. Y. Nishimura, M. Schnall-Levin, P. W. Wyatt, C. M. Hindson, R. Bharadwaj, A. Wong, K. D. Ness, L. W. Beppu, H. J. Deeg, C. McFarland, K. R. Loeb, W. J. Valente, N. G. Ericson, E. A. Stevens, J. P. Radich, T. S. Mikkelsen, B. J. Hindson and J. H. Bielas, Nat. Commun., 2017, 8, 14049 CrossRef CAS PubMed
.
- J. C. Roder and H. F. Pross, J. Clin. Immunol., 1982, 2, 249–263 CrossRef CAS PubMed
.
- A. Moretta, C. Bottino, M. Vitale, D. Pende, R. Biassoni, M. C. Mingari and L. Moretta, Annu. Rev. Immunol., 1996, 14, 619–648 CrossRef CAS PubMed
.
- E. Vivier, E. Tomasello, M. Baratin, T. Walzer and S. Ugolini, Nat. Immunol., 2008, 9, 503–510 CrossRef CAS PubMed
.
- T. J. Laskowski, A. Biederstadt and K. Rezvani, Nat. Rev. Cancer, 2022, 22, 557–575 CrossRef CAS PubMed
.
- G. Xie, H. Dong, Y. Liang, J. D. Ham, R. Rizwan and J. Chen, EBiomedicine, 2020, 59, 102975 CrossRef CAS PubMed
.
- L. Li, L. N. Liu, S. Feller, C. Allen, R. Shivakumar, J. Fratantoni, L. A. Wolfraim, H. Fujisaki, D. Campana, N. Chopas, S. Dzekunov and M. Peshwa, Cancer Gene Ther., 2010, 17, 147–154 CrossRef CAS PubMed
.
- N. Shimasaki, H. Fujisaki, D. Cho, M. Masselli, T. Lockey, P. Eldridge, W. Leung and D. Campana, Cytotherapy, 2012, 14, 830–840 CrossRef CAS PubMed
.
- N. Subedi, L. C. Van Eyndhoven, A. M. Hokke, L. Houben, M. C. Van Turnhout, C. V. C. Bouten, K. Eyer and J. Tel, Sci. Rep., 2021, 11, 17084 CrossRef CAS PubMed
.
- A. E. Christakou, M. Ohlin, B. Vanherberghen, M. A. Khorshidi, N. Kadri, T. Frisk, M. Wiklund and B. Önfelt, Integr. Biol., 2013, 5, 712–719 CrossRef CAS PubMed
.
- D. Dannhauser, D. Rossi, A. T. Palatucci, V. Rubino, F. Carriero, G. Ruggiero, M. Ripaldi, M. Toriello, G. Maisto, P. A. Netti, G. Terrazzano and F. Causa, Lab Chip, 2021, 21, 4144–4154 RSC
.
- R. Zenhausern, A. S. Day, B. Safavinia, S. Han, P. E. Rudy, Y. W. Won and J. Y. Yoon, Biosens. Bioelectron., 2022, 200, 113916 CrossRef CAS PubMed
.
- S. J. Xu, Z. H. Shao, R. Fu, H. Q. Wang, H. Liu, C. Y. Liu and W. Zhang, Zhongguo Shiyan Xueyexue Zazhi, 2017, 25, 832–836 Search PubMed
.
- M. Carlsten, E. Levy, A. Karambelkar, L. Li, R. Reger, M. Berg, M. V. Peshwa and R. W. Childs, Front. Immunol., 2016, 7, 105 Search PubMed
.
- T. Kamiya, S. V. Seow, D. Wong, M. Robinson and D. Campana, J. Clin. Invest., 2019, 129, 2094–2106 CrossRef PubMed
.
- E. Wennerberg, D. Sarhan, M. Carlsten, V. O. Kaminskyy, P. D'Arcy, B. Zhivotovsky, R. Childs and A. Lundqvist, Int. J. Cancer, 2013, 133, 1643–1652 CrossRef CAS PubMed
.
- A. Muntasell, M. Cabo, S. Servitja, I. Tusquets, M. Martínez-García, A. Rovira, F. Rojo, J. Albanell and M. López-Botet, Front. Immunol., 2017, 8, 1544 CrossRef PubMed
.
- S. Sarkar, P. Sabhachandani, D. Ravi, S. Potdar, S. Purvey, A. Beheshti, A. M. Evens and T. Konry, Front. Immunol., 2017, 8, 1736 CrossRef PubMed
.
- S. Sarkar, S. McKenney, P. Sabhachandani, J. Adler, X. Z. Hu, D. Stroopinksy, J. Rosenblatt, D. Avigan and T. Konry, Sens. Actuators, B, 2019, 282, 580–589 CrossRef CAS PubMed
.
- S. Sarkar, W. J. Kang, S. Y. Jiang, K. P. Li, S. Ray, E. Luther, A. R. Ivanov, Y. Fu and T. Konry, Lab Chip, 2020, 20, 2317–2327 RSC
.
- D. Ravi, S. Sarkar, S. Purvey, F. Passero, A. Beheshti, Y. Chen, M. Mokhtar, K. David, T. Konry and A. M. Evens, Leukemia, 2020, 34, 1291–1304 CrossRef CAS PubMed
.
- J. M. Pitt, A. Marabelle, A. Eggermont, J. C. Soria, G. Kroemer and L. Zitvogel, Ann. Oncol., 2016, 27, 1482–1492 CrossRef CAS PubMed
.
- J. M. Ayuso, R. Truttschel, M. M. Gong, M. Humayun, M. Virumbrales-Munoz, R. Vitek, M. Felder, S. D. Gillies, P. Sondel, K. B. Wisinski, M. Patankar, D. J. Beebe and M. C. Skala, Oncoimmunology, 2019, 8, 1553477 CrossRef PubMed
.
- D. Park, K. Son, Y. Hwang, J. Ko, Y. Lee, J. Doh and N. L. Jeon, Front. Immunol., 2019, 10, 1133 CrossRef CAS PubMed
.
- S. Gopal, S. J. Kwon, B. Ku, D. W. Lee, J. Kim and J. S. Dordick, Commun. Biol., 2021, 4, 893 CrossRef CAS PubMed
.
- R. Wehner, K. Dietze, M. Bachmann and M. Schmitz, J. Innate Immun., 2011, 3, 258–263 CrossRef CAS PubMed
.
- S. Mahmood, S. Nandagopal, I. Sow, F. Lin and S. K. P. Kung, Eur. J. Immunol., 2014, 44, 2737–2748 CrossRef CAS PubMed
.
- D. Wu, Y. R. Yu, C. Zhao, X. Shou, Y. Piao, X. Zhao, Y. J. Zhao and S. Q. Wang, ACS Appl. Mater. Interfaces, 2019, 11, 33716–33724 CrossRef CAS PubMed
.
Footnote |
† These authors contributed equally to this work. |
|
This journal is © The Royal Society of Chemistry 2024 |
Click here to see how this site uses Cookies. View our privacy policy here.