Label free, machine learning informed plasma-based elemental biomarkers of Alzheimer's disease†
Received
19th March 2024
, Accepted 18th June 2024
First published on 12th July 2024
Abstract
Using inductively coupled plasma mass spectrometry (ICP-MS), we have measured the elemental concentrations of Na, Fe, Cu, P, Mg, Zn, K in plasma samples of 25 Alzheimer's disease (AD) patients and 34 healthy individuals. Given the multidimensional nature of the ICP-MS data, we used support vector machines and logistic regression to illustrate the elemental distribution of each donor and seek key features that may differentiate plasma samples of AD patients from those of healthy individuals. We found that ratios of the elemental concentrations of Na over K, Fe over Na, and P over Zn yield specificity, sensitivity, and accuracy of 79%, 84% and 81% respectively. This information was then used to seek from the mass spectrometric data a differentiation of the plasma samples from AD and healthy donors. Plotted as a function of the Na/K, Fe/Na, and P/Zn, the ICP-MS data reveals a linear delineation between the two groups of samples yielding to the correct classification 21 of 25 AD and 28 of 34 HC plasma samples. These findings highlight the importance of elemental ratios present in plasma and suggest that the ratios of the elemental concentrations of blood metals may be considered as biomarkers that can distinguish plasma samples of AD patients from healthy subjects.
1 Introduction
Alzheimer's disease (AD) is an uncurable progressive neurodegenerative disorder that poses significant challenges to healthcare systems worldwide, individuals, families, and communities. Today, the gold standard for the diagnosis of AD involves pathological confirmation of amyloid-β-containing neuritic plaques and phosphorylated tau (p-tau)-containing neurofibrillary tangles in postmortem brain tissue. A panel of biomarkers corresponding to amyloid, tau and neurodegeneration, referred to as ATN, are mainly evaluated by invasive and costly methods that involve collection and analysis of cerebrospinal fluid (CSF) and positron emission tomography (PET) scans. ATN levels in CSF are analyzed by ultra-sensitive enzyme-linked immunosorbent assay (ELISA), and ATN burden in brains are often analyzed using neuroimaging techniques such as magnetic resonance imaging (MRI) and PET.1–4 As AD progresses gradually, the neurodegenerative processes are likely to initiate 18 years before the typical clinical symptoms of dementia appear.5 These transitional stages, clinically identified as preclinical AD and mild cognitive impairment (MCI) stages, are often examined for early indicator or precursor to the onset of dementia.6 Despite considerable advancements in both basic and clinical AD studies, the disease's etiology remains largely unclear. To effectively prevent or delay the onset of the disease, it is beneficial to identify biomarkers that can contribute to a better understanding of its etiology and its progression, preferably using minimally or noninvasive means. Blood-based biomarkers have the potential to meet this requirement.4,6,7 A wide range of blood-based biomarkers of AD have been explored,8 and the potential of using concentrations of elements present in blood has been highlighted and reported9–11 including for other diseases.12–14 Trace amount of elements known to be present in the brain, such as zinc, copper, iron, aluminum, and selenium have been the focus of multiple studies on AD.15–20 He et al.21 found an inverse correlation between increased serum Ca levels and decreased risk of AD. Similar studies, conducted by comparing the levels of individual elements in samples from donors known to have AD to those from healthy controls (HC), have not reached consistent conclusions.22,23 Li et al. conducted an analysis of 44 publications and found that there is a strong association of the serum levels of Cu, Zn, and Fe with AD; other studies show a weak or no association between levels of Cu and AD.24,25 Furthermore, additional studies have demonstrated reduced levels in serum of Mg, Mn, Fe, and Se in AD individuals compared to that of HC.26–29 Recently, an alternative direction based on ratios instead of absolute levels of element was pursued using univariate Receiver Operating Characteristic (ROC) curve analysis, and the results clearly showed >90% accuracy when the ratio of Cu to Mn in blood was used to distinguish between AD and healthy subjects.30 For patients with MCI, Cicero et al. reported that a higher ratio of Cu to non-heme Fe could predict the progression from MCI to AD over the duration of the study (5 years).23 Taken together, these studies suggest that there is a complex relationship between various elements (including trace metals) and AD,24 and that patients with AD may exhibit abnormal element concentrations in serum and brain.22
In this study, we sought to identify the main elements that contribute to the differentiation between AD and HC subjects using machine learning models. We have harnessed the power of machine learning to identify the contributions of multiple elements and their ratios in distinguishing plasma samples of AD patients from HC donors. Our approach consists of three steps. First, using ICP-MS, we measured the elemental concentrations of Na, Fe, Cu, P, Mg, Zn, K in plasma samples of 25 AD and 34 HC donors. Second, we computed p values (T-test) and used two machine learning algorithms, support vector machines (SVM) and logistics regression, to identify combinations of elements and their ratios that differentiate the plasma samples of AD from HC donors. Third, we used the information on the main features obtained from the machine learning analysis to visualize the ICP-MS data spectrometric signatures that yield differentiation between the AD and HC plasma samples. We found that the combinations of 3 elements ratios (Na/K, Fe/Na and P/Zn) yield classification with 79% specificity, 84% sensitivity, and 81% accuracy.
2 Materials and methods
2.1 Sample collection
Subjects in this study were recruited from the Bedford VA Hospital Dementia Care Unit. The protocol was approved by the Bedford VA Hospital Institutional Review Board and written informed consent for each participant was obtained before initiation of the study and blood collection. Montreal Cognition Assessment (MoCA) was used to evaluate enrolled subjects, and healthy control subjects were scored over 27.31 Blood was collected with BD Vacutainer Blood Collection Steel Needle, 21 G × 1.25 inch into Vacutainer cell tubes (CPT, Becton Dickinson and Company, Franklin Lakes, NJ) and immediately centrifuged at 1500 × g for 20 min at room temperature. After centrifugation, the plasma was separated and frozen at −80 °C.32
2.2 Experimental
Plasma samples were diluted with 10 mL of 1% distilled nitric acid, followed by the addition of a purified indium spike solution to serve as a drift corrector. The plasma samples were transferred individually from the original tubes to centrifuge tubes with nitric acid by pipette. We weighed these tubes before and after the addition to determine the weight of plasma. The amount of plasma material varied slightly from sample to sample, but the mean was 0.135 g which amounts to about 0.13 mL. Samples were analyzed on an Agilent 7900 quadrupole-inductively coupled plasma-mass spectrometer (Q-ICP-MS) in the Core Research Facility at the University of Massachusetts Lowell under the operating parameters given in the Table 1. All masses were measured in helium collision mode. Serial dilutions of a customized mixed element standard solution from Inorganic Ventures were used as external calibrates, and the Seronorm Trace Elements Serum L-1 was analyzed repeatedly in each session for quality control. Results are reported in Table S1 (ESI†).
Table 1 Agilent 7900 quadrupole ICP-MS instrument parameters
For elements where multiple isotopes were monitored, isotopes in bold were used to calculate final concentrations.
|
RF power |
1550 W |
Plasma gas flow rate |
15 L per min Ar |
Auxiliary gas flow rate |
0.9 L per min Ar |
Sample gas flow rate |
1.05 L per min Ar |
Makeup gas flow rate |
0.14 L per min Ar |
Sample depth |
7.8 mm |
Spray chamber |
Apex IR desolvation system |
Collision cell He gas flow |
3.2 mL per min He |
Detector mode |
Dual |
Dwell time/mass |
Variable (10 to 30 ms) |
Acquisition |
3 points per peak, 10 sweeps per replicate, 3 replicates |
Isotopes and dwell time (in milliseconds)a |
23Na (50), 25Mg (100), 31P (100), 39K (100), 52Cr (100), 53Cr (100), 55Mn (100), 57Fe (100), 63Cu (100), 66Zn (150), 67Zn (150), 68Zn (150), 77Se (150), 78Se (150), 82Se (150), 115In (150) |
2.3 Data preprocessing
Using ICP-MS, we planned to measure the concentrations levels of 10 elements of 59 plasma samples (34 HC and 25 AD). We selected ten elements, Na, K, Mg, Ca, Fe, Zn, Mn, Cu, Cr, and Se, because they are known to be present in human blood plasma and their levels can potentially be measurable using ICP-MS. An initial assessment of the data for both groups revealed that the concentrations of chromium (Cr) and manganese (Mn) were not detectable by our instrument. We have therefore not considered Cr and Mn in our analysis. Additionally, Se exhibited a weak signal-to-noise ratio (SNR) and relatively high relative standard deviation (RSD) indicating a large variability in the measurements. Consequently, Se was excluded from our analysis. The ICP-MS signals of the remaining seven elements, Na, Fe, Cu, P, Mg, Zn, K, were well above the detection limit of our instrument. We further refined our dataset by calculating the total concentration of the seven elements for each sample and searched for potential outliers. For each sample, we calculated the z-score based on the total concentration. Using a z-score threshold of 2, we identified and eliminated a total of three samples (healthy controls) that exhibited significant deviations from the means of the data distribution. We also explored outlier detection by applying the z-score method to each element individually. However, this approach did not significantly alter the conclusions of the study. This step mitigated the potential bias introduced by outliers and ensured that our subsequent analyses, including machine learning and feature importance analysis, were based on representative data.
2.4 Machine learning approaches
To classify AD and HC, we used two machine learning algorithms: Support Vector Machine (SVM) with a linear kernel and logistic regression. SVM with a linear kernel is a powerful algorithm that performs well on high-dimensional data and is particularly suited for binary classification problems. To separate different classes, it constructs a hyperplane in a high-dimensional space.33 A key aspect of SVM is the selection of the hyperparameter C. We initially set C to be 1, a value often used in SVM implementations. However, as the optimal value of C depends on the specific dataset and problem at hand, we tested different values of C to find the value that yields the best performance on unseen data. This parameter controls the trade-off between reducing training error and minimizing model complexity to prevent overfitting.34
Logistic regression, a type of generalized linear model, is a widely used algorithm for binary classification.35 It models the probability of an individual sample belonging to a specific class based on their features. In this study, we used the scikit-learn library36 which employs the lbfgs solver, L2 regularization, and a regularization strength denoted by the hyperparameter C, set to 1. The parameter C in logistic regression plays a role analogous to that in SVMs, where it controls the inverse of the regularization strength, thereby aiding in the prevention of overfitting. This approach provides interpretable coefficients that helps identify the most influential features for AD classification.37 To address the issue of slightly imbalanced class samples in the dataset, we used the class_weight = “balanced” parameter in both the SVM and logistic regression implementation. This parameter adjusts the weights inversely proportional to class frequencies in the input data, which helps in handling the imbalance effectively. This approach ensures that the model does not bias its decisions towards the majority class and provides a fair chance for the minority class to be correctly classified. Statistical and machine learning analyses were conducted using a custom-built Python program, developed and executed in the Spyder IDE.
2.5 Cross-validation
We employed Leave-One-Out Cross-Validation (LOOCV), a resampling procedure used to evaluate machine learning models on a limited data sample.38 The procedure is appropriate for our dataset, which consists of 59 samples. LOOCV works by splitting the dataset into two parts: a single sample is used as the test set, and the remaining 58 samples serve as the training set. The model is trained on 58 samples and tested on the 1 left out. This process is repeated such that each observation in the dataset is used once as the test data. This is particularly advantageous for small datasets. The primary advantage of LOOCV is that it allows for the maximum possible amount of training data and uses each data point as a test data, thus reducing bias.
3 Results
In Table 2, we show demographic and age distribution of the donors considered in this study as well as the means and relative standard deviations concentrations of seven elements in the plasma samples measured using ICP-MS for HC and AD patients. The table shows that the average age of the AD group is higher than the HC group. As shown in Fig. S2,† the age distribution of our patients exhibits an overlap of 8 AD and 1 HC patients. The gender distribution shows more males than females in both groups due to the fact that most veterans are male.
Table 2 Demographic and mean and standard deviation values of elemental concentration data for healthy controls (HC) and patients with Alzheimer's disease (AD)
|
HC |
AD |
Gender |
Male = 32 |
Male = 24 |
Female = 2 |
Female = 1 |
Age (years) |
66.8 ± 7.8 |
82.2 ± 10.6 |
Na (ppm) |
5863.75 ± 3038.3 |
6614.4 ± 3094.2 |
Mg (ppm) |
29 ± 14.3 |
34.8 ± 17.4 |
P (ppm) |
125.2 ± 49 |
132.3 ± 53.7 |
K (ppm) |
205.2 ± 100.4 |
251.05 ± 123.8 |
Fe (ppm) |
1.18 ± 0.56 |
1.1 ± 0.9 |
Cu (ppm) |
1.3 ± 0.7 |
1.4 ± 0.7 |
Zn (ppm) |
1.8 ± 1 |
1.9 ± 1.2 |
3.1 Feature extraction
We used statistical analysis to select elemental features that can distinguish AD from HC plasma samples. The concentration of Na is significantly higher than the other elements. For all pairwise comparisons of these elements, we calculated the Pearson correlation coefficients. This step contributes to better understand the relationship between these elements and to identify those with low correlations that potentially be useful features. These calculations were conducted separately for each of the HC and AD patients. The results are shown in Fig. 1.
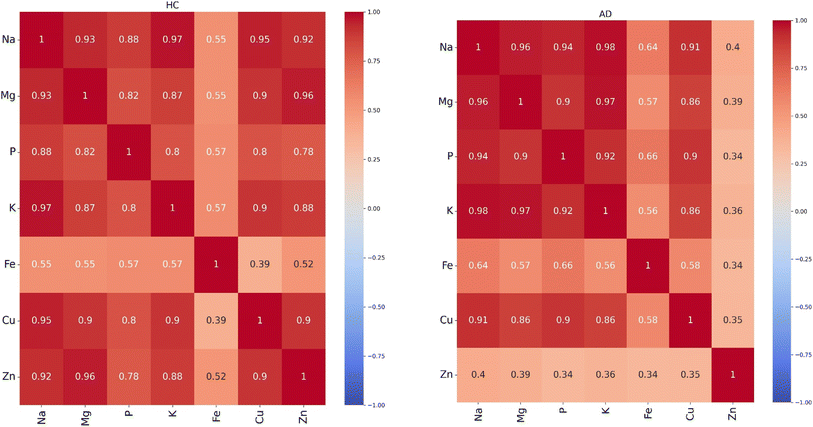 |
| Fig. 1 Pearson correlation coefficients for all pairwise comparisons of the seven elements for healthy controls (HC) and Alzheimer's disease (AD). | |
In both groups, strong positive interdependencies were observed among the elements, with the notable exceptions of Fe and Zn. Interestingly, Fe exhibited low correlation coefficients in both groups, suggesting a unique pattern distinct from the other elements. However, we noted a significant change between the AD and HC plasma samples in the correlation coefficients of Zn. In the HC group, Zn exhibited a high correlation with the other elements while in the AD group, the correlation of Zn with the other elements was low. A substantial difference was observed in the correlation between Zn and Mg, with a coefficient of 0.96 in the HC group and 0.39 in the AD group. These observations suggest distinct underlying relationships within each group, highlighting the potential influence of Zn and Mg in the context of AD and possibly their progression.
We note that high correlation among elements presents a challenge, as it could potentially induce multicollinearity in linear models.39 Multicollinearity can inflate the variance of the model's estimates and increase its sensitivity to minor changes in the training set. This sensitivity can, in turn, lead to less reliable coefficients. Therefore, despite the potential strong interpretive value of individual elements, their use as features posed a challenge. Recognizing this, we conducted a feature selection process based on ratios of concentrations of the seven elements rather their direct absolute concentration levels.
To test whether selected ratios of the seven elements can capture complex relationships and provide additional discriminatory power for classifying AD and HC plasma samples, we calculated the Pearson correlation coefficients for the said ratios. We note that, given the large number of the ratios in our dataset (42 in total), we have plotted a heatmap for only 21 by selecting a single ratio for every pair of elements (e.g.: we selected one ratio from Cu/Fe and Fe/Cu). The heatmap shown in Fig. 2 provides an overview of the correlation coefficients among the selected ratios. The ratio analysis reveals a surprising difference than the results from the analysis performed with the correlation coefficients of the individual elements. While the individual elements generally exhibited strong positive correlations, the outcome changed significantly when we considered elemental ratios. The majority of the ratio correlations fell within a wider range, from −1 to 1, as shown in Fig. 2. The heatmap shows that most cells carry low correlation coefficients. This also shows that HC and AD disease patients exhibit distinct patterns in their correlation coefficients.
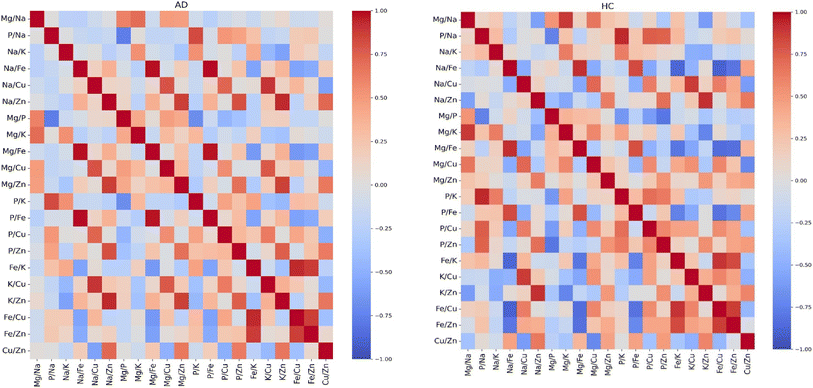 |
| Fig. 2 Pearson correlation coefficients for all pairwise comparisons of the elements ratio for healthy controls (HC) and Alzheimer's disease (AD). | |
3.2 Machine learning results
In this section, we explore the potential of two distinct machine learning models: Support Vector Machines (SVM) with a linear kernel and logistic regression. Using these models, we aim to consider separately individual elements and their ratios for classifying AD and HC samples. By comparing the performance of these models using both sets of features, we aim to uncover insights into the role of individual elements and their synergistic effects in distinguishing AD from HC. The comparative analysis will provide insights into how the relationships between these elements, both individually and in combination, contribute to the classification accuracy.
3.2.1 Individual elements as features.
First, we focused on using the concentrations of the seven individual elements as features. These features were fed into our machine learning models – Support Vector Machines (SVM) and logistic regression. Fig. 3A presents the performance of the model in terms of sensitivity, specificity, and accuracy. These metrics provide a comprehensive view of the model's ability to correctly classify AD and HC samples. We note that these results were obtained using a leave-one-out cross-validation approach. The performance metrics were then averaged over all iterations to yield an overall estimate of the model's performance. The SVM model achieved an accuracy of 64%, sensitivity of 56%, and specificity of 71%. On the other hand, the logistic regression model achieved the same accuracy of 64%, with the specificity of 74% and the slightly lower sensitivity of 52%.
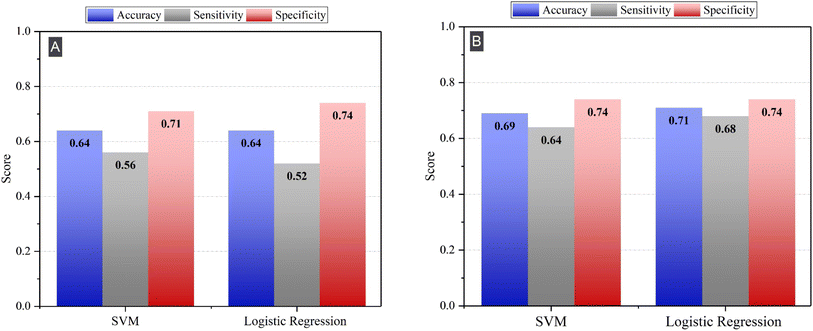 |
| Fig. 3 Sensitivity, specificity, and accuracy of SVM and logistic regression by using (A) individual elements and (B) elements ratios as feature. | |
While both models demonstrated high specificity, indicating a strong ability to correctly identify HC samples, the sensitivity obtained with both models was relatively low. This means that the models had a lower success rate in correctly identifying AD samples. Low sensitivity could lead to a high rate of false negatives, meaning that AD patients could be incorrectly classified as healthy.
3.2.2 Element's ratios as features.
Next, we focused on the concentration ratios of the seven elements. The result of the analysis is shown in Fig. 3B. It shows an improvement in the performance of our models compared to using individual elements as features. The accuracy of the SVM model increased from 64% to 69%, and the sensitivity improved from 56% to 64%. Similarly, for the logistic regression model, the accuracy increased from 64% to 71%, and the sensitivity improved from 52% to 68%.
We note that the specificity of both models remained relatively high, indicating that the models were effective at correctly identifying HC samples. These results support our hypothesis that the ratios of the elements better capture complex relationships and provide additional discriminatory power for classifying AD and HC samples. Therefore, using ratios of element concentrations as features rather than absolute elemental concentrations is more effective at classifying HC and AD plasma samples.
3.3 Feature importance
In this section, we examined the degree of influence that each feature had on the classification that the SVM or logistic regression models provided. In our previous sections, we utilized the elements concentrations and their ratios as features. Our findings indicated that for both SVM and logistic regression models, the ratio of elements yielded superior results. Building upon this, we sought to evaluate the importance of several of these features to ascertain which ones played a significant role in the classification of AD. We note that, before we turned our attention to the ratios of the seven elements, we initially evaluated all possible subsets of individual element concentration in an attempt to improve the performance of our models. We found that no subset of individual features yielded any significant improvement over using all individual elements as features.
To identify the ratio of elemental features that can optimally differentiate and classify HC and AD plasma samples, we evaluated the performance of the model using all possible subsets of 1 to 5 features from the dataset. As our dataset consisted of 59 samples, this approach was feasible and allowed for thorough exploration of the feature space. To mitigate the extensive processing time required for feature subsets larger than 5, we employed forward feature selection to evaluate the model's performance. This process involved adding another feature to the combination of features that yielded the highest accuracy, to find the best subset of 6 features. We followed the same procedure for larger subsets. To avoid multicollinearity, we introduced a threshold value of 0.6 for the absolute value of the correlation. If the Pearson correlation value of any pair of features exceeded this threshold, indicating high correlation, we removed that feature combination from consideration. This step ensured that our model was not affected by multicollinearity. For each subset of features, we identified those that yielded the highest accuracy, sensitivity, and specificity. Results of this analysis for the logistic regression and SVM models when using subsets of 1 to 9 features are illustrated in Fig. 4. This figure shows that sensitivity, specificity and accuracy of classification improve as the number of features increase from 1 to 4 and remain relatively stable before it starts to drop. We also note that in the SVM model, the maximum accuracy, 83%, is achieved when employing 4 features.
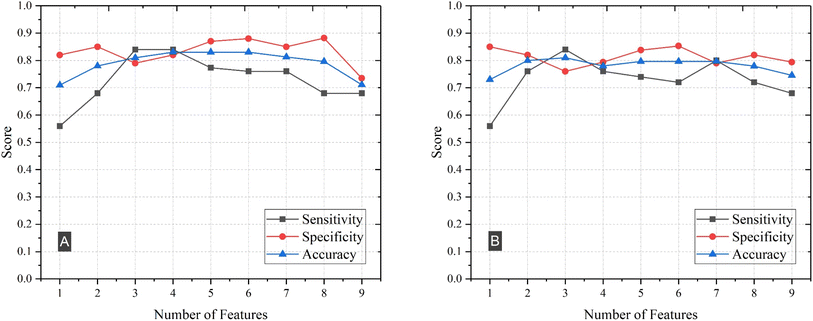 |
| Fig. 4 Sensitivity, specificity, and accuracy of (A) SVM and (B) logistic regression by using subsets of 1 to 9 features. | |
Table 3 shows the features that exhibit the highest performance when we use subsets of 1 to 3 features for both logistic regression and SVM models. We note that for subsets of 1, 2, and 3 features, we obtain the highest accuracy for both SVM and logistic regression with the same elemental features.
Table 3 Top-performing features in subsets of 1 to 3 for logistic regression and SVM models
|
Logistic regression |
Support vector machine (SVM) |
Features |
Accuracy |
Sensitivity |
Specificity |
Features |
Accuracy |
Sensitivity |
Specificity |
1 feature |
|
0.73 |
0.56 |
0.85 |
|
0.71 |
0.56 |
0.82 |
2 features |
|
0.8 |
0.76 |
0.82 |
|
0.78 |
0.68 |
0.85 |
3 features |
|
0.8 |
0.84 |
0.76 |
|
0.81 |
0.84 |
0.79 |
3.4 From elemental features to mass spectrometric data
In our subsequent analysis, we used the results of machine learning and applied to the ICP-MS data to seek differentiation between the HC and AD plasma samples. We used a t-test to compute the p-value for each feature (Fig. 5) and we compared HC with AD for each feature listed in Table 3. Fig. 5 shows that box plots for the HC and AD plasma samples, the means with their respective uncertainty bars, and the computed p-values of several elemental ratios. It shows that the Na/K ratio is lower in the plasma blood samples of the AD than that for HC.
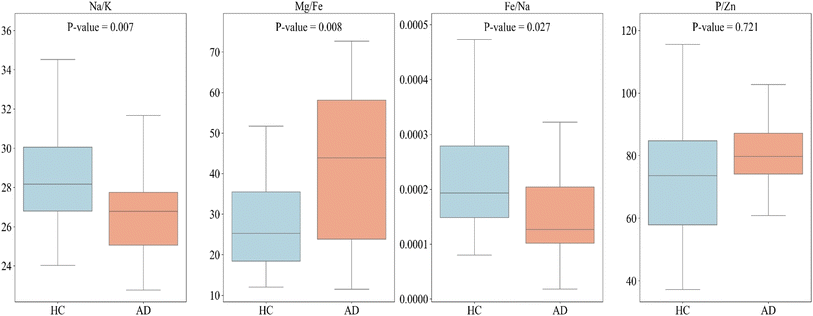 |
| Fig. 5 Box plots representing each feature listed in Table 3, used to compare healthy control (HC) vs. Alzheimer's disease (AD). Each graph includes the p-value for the respective feature. | |
We found statistical significance in differences and showed that the p-values for the combination of 1 to 3 features were less than 0.05 except P/Zn. We note that these p values can be smaller if the uncertainties on the measurements of the various elemental concentrations are reduced. We conducted a similar comparison between HC and AD for each element. These comparisons are illustrated in Fig. S1 (ESI†). Univariate analysis revealed that the concentration of all examined elements, except for Fe, exhibited a slight increase in AD compared to HC. In contrast, Fe showed a decrease in concentration in AD compared to HC. This observation is in agreement with the findings of Hare et al., who also reported an association between lower plasma iron levels and Alzheimer's disease.40 When we utilized the ratio of these elements, the difference between AD and HC became more pronounced.
Fig. 6 shows 2D and 3D visualizations of the differentiation between HC and AD plasma samples using (Na/K and Mg/Fe), and (Na/K, Fe/Na, and P/Zn) as features respectively. Use of three features, namely, (Na/K, Fe/Na, and P/Zn), the data points of plasma samples from HC and AD are differentiable as they fall into two different spaces. Our results demonstrate the performance and classification power of the linear models with respect to the selected features.
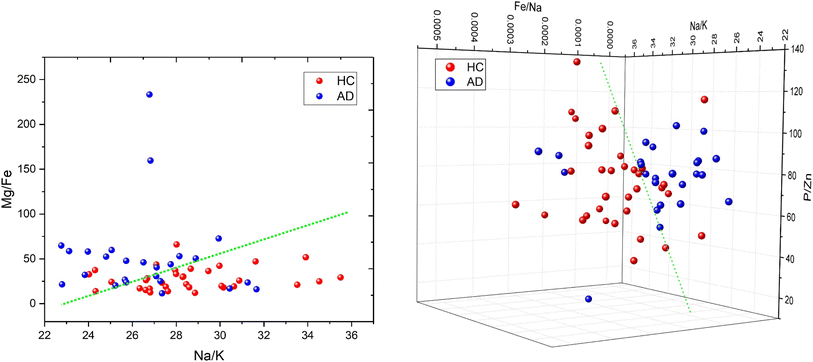 |
| Fig. 6 2D (left) and 3D (right) visualizations utilizing the two (Na/K and Mg/Fe) and three features (Na/K, Fe/Na and P/Zn), respectively, that yielded the highest accuracy in our analysis. The dotted lines are provided to illustrate the linear classification of HC and AD blood plasma samples. | |
The information of the key elemental features obtained was used to seek from the mass spectrometric data a differentiation of the plasma samples from AD and healthy donors. To better visualize the results on the impact of the ratio of elemental features on the differentiation of the AD and HC plasma samples, we illustrated the Na/K, Mg/Fe (Fig. 6 left) and Na/K, Fe/Na, and P/Zn (Fig. 6 right) for each donor. We found that a linear delineation between the two groups of samples led to the correct identification of 21 of 25 AD and 28 of 34 HC plasma samples. This shows that the elemental concentration ratios, Na/K, Fe/Na, and P/Zn, differentiated blood plasma samples of HC and AD donors using SVM and logistics regression on the ICP-MS data. The specificity, sensitivity, and accuracy obtained are 0.79, 0.84 and 0.81 respectively. This study suggests that these ratios can be used as a panel of biomarkers to distinguish with a high level of accuracy these samples.
4 Discussion
We have identified ratios of concentrations of elements that yield excellent classification accuracy of HC and AD plasma samples.
Our result (Fig. 5) is consistent with the previous studies on a connection between the Na to K ratio to neurodegenerative diseases.41–43 A similar finding was obtained on concentrations ratios Mg/Fe and Zn/Fe that are higher for AD samples.
This study demonstrates that the selection of element concentration ratios as features using statistics and machine learning models can significantly aid in unraveling the underlying mechanisms of AD. We have shown that the correlation between the paired features is relatively low, which implies that they independently contribute to the differentiation of HC and AD plasma samples. For instance, for the combination of 3 features, Na/K, Fe/Na, and P/Zn, which yielded the highest accuracy with both SVM, the Pearson correlation coefficient is 0.13, 0.05 and −0.03 respectively for the pairs (Na/K, Fe/Na), (Fe/Na, P/Zn), and (Na/K, P/Zn). This suggests that each feature may contribute unique information independently to the classification of AD, thereby enhancing the reliability and interpretability of our approach. This study also suggests that the relationships between concentrations of Na and K, Fe and Na, and P and Zn may yield to a better separation of AD from HC. It highlights the importance of considering the correlation among elemental concentrations that could potentially induce multicollinearity in linear models. We found that the elemental concertation ratios, (Na/K, Mg/Fe) or (Na/K, Fe/Na, P/Zn) taken together, can be used as biomarkers of AD.
5 Limitations
This study was performed with 59 blood plasma samples acquired from 34 HC and 25 AD donors. Ideally, we would have conducted it with a larger number of blood plasma samples but securing samples of AD and HC donors is challenging. However, despite this limitation we have obtained statistically significant results to within the reported uncertainties. This was possible because we collected 3 mass spectrometry data for each sample and performed a careful uncertainty analysis. Another limitation to this study stems from the fact that our blood plasma samples were collected from patients with partially overlapping ages as shown in Fig. S2.† We note that age alone cannot be good differentiator of HC and AD blood plasma donors. To test the effect of age on our results, we incorporated it in the SVM model as an additional feature. We observed that with a single feature, the model's accuracy was 85% when age was included, compared to 71% without it, a change of 14%. However, as we expanded the feature set from 1 to 4, the difference in accuracy caused by the inclusion of age decreased to about 7%. This suggests a diminishing influence of age on the model's performance as more features are used.
Despite these limitations, our study provides a valuable foundation for further research into the classification of AD using blood samples and machine learning. Finally, we note that the use of ratios as independent variables must be taken with great care as such an approach may lead to spurious conclusions.44 As pointed out by Tu et al., when there is a significant correlation between denominator and numerator, as is the case in this study, then the correlation between the two ratio variables is considerably less prone to spuriousness.45 In addition, by taking the step of going back to spectroscopic data to differentiate the HC from AD samples (Fig. 6), we show that these results of this study are solid albeit caution must be taken as the number of samples considered for this study is limited.
6 Conclusions
The aim of this study was to identify elemental biomarkers of Alzheimer's disease (AD) present in plasma samples. Using ICP-MS, we first measured the elemental concentrations of plasma samples of 25 AD patients and 34 healthy individuals. We then used p values (T-test), support vector machines and logistic regression to seek key elemental features that may differentiate plasma samples of AD patients from those of healthy individuals. We found that ratios of the elemental concentrations, more than absolute concentrations, of Na, Fe, Cu, Se, P, Mg, Zn, K yielded significant differentiation of the plasma samples. Three ratios, Na/K, Fe/Na and P/Zn, yielded specificity, sensitivity, and accuracy of 79%, 84% and 81% respectively. With this information, we sought to understand whether the ICP-MS data could reveal the same classification results as those obtained using machine learning. To do this, we went back to the ICP-MS data and plotted it in a new space: as a function of the Na/K, Fe/Na, and P/Zn ratios previously identified. We found a clear differentiation of the AD and healthy plasma samples: 21 of 25 AD and 28 of 34 HC plasma samples were correctly identified (see Fig. 6 of the manuscript).
These findings highlight the importance of elemental ratios present in plasma and suggest that the ratios of the elemental concentrations of Na over K, Fe over Na, and P over Zn may be considered as biomarkers that can distinguish plasma samples of AD patients from healthy subjects. We believe that although the number of samples used in this study is limited, we have shown that ratios of elements present in blood may have the potential to be used as biomarkers of AD. This study also shows that machine learning algorithms can be used to seek paths for the analysis of complex multidimensional spectroscopic data.
Ethical statement
All experiments were performed in accordance with the Guidelines of “the Declaration of Helsinki 1964 and its later amendments”, and experiments were approved by the ethics committee at “Bedford VA Hospital Institutional Review Board”. Informed consents were obtained from human participants of this study.
Author contributions
AS, WX and NM: led the conceptualization and led the preparation of the original draft, laying the foundation for the study and ensuring the manuscript's initial coherence and direction. RG: led, designed, and conducted the ICP-MS measurements and provided review of the original manuscript. AS, KEE and NM: collaboratively conducted the formal analysis, investigation, and methodology analysis. AS, NM and WX: played a critical role in the manuscript's final preparations. NM and XW: were instrumental in funding acquisition, securing the financial support necessary for the research's execution and dissemination.
Conflicts of interest
There are no conflicts to declare.
Acknowledgements
We greatly thank Omar Melikechi and Jong Soo Lee for discussions on the analysis of the ICP-MS data.
References
- M. Citron, D. Westaway, W. Xia, G. Carlson, T. Diehl, G. Levesque, K. Johnson-wood, M. Lee, P. Seubert, A. Davis, D. Kholodenko, R. Motter, R. Sherrington, B. Perry, H. Yao, R. Strome, I. Lieberburg, J. Rommens, S. Kim, D. Schenk, P. Fraser, P. St George Hyslop and D. J. Selkoe, Nat. Med., 1997, 3, 67–72 CrossRef CAS.
- D. S. Knopman, H. Amieva, R. C. Petersen, G. Chételat, D. M. Holtzman, B. T. Hyman, R. A. Nixon and D. T. Jones, Nat. Rev. Dis. Primers, 2021, 7, 33 CrossRef.
- W. Jagust, Nat. Rev. Neurosci., 2018, 19, 687–700 CrossRef CAS.
- H. Hampel, S. E. O'Bryant, J. L. Molinuevo, H. Zetterberg, C. L. Masters, S. Lista, S. J. Kiddle, R. Batrla and K. Blennow, Nat. Rev. Neurol., 2018, 14, 639–652 CrossRef PubMed.
- J. Jia, Y. Ning, M. Chen, S. Wang, H. Yang, F. Li, J. Ding, Y. Li, B. Zhao, J. Lyu, S. Yang, X. Yan, Y. Wang, W. Qin, Q. Wang, Y. Li, J. Zhang, F. Liang, Z. Liao and S. Wang, N. Engl. J. Med., 2024, 390, 712–722 CrossRef CAS.
- S. Lista, B. Dubois and H. Hampel, J. Nutr., Health Aging, 2015, 19, 154–163 CrossRef CAS PubMed.
- K. Blennow, Neurol. Ther., 2017, 6, 15–24 CrossRef PubMed.
- C. E. Teunissen, I. M. W. Verberk, E. H. Thijssen, L. Vermunt, O. Hansson, H. Zetterberg, W. M. van der Flier, M. M. Mielke and M. del Campo, Lancet Neurol., 2022, 21, 66–77 CrossRef CAS PubMed.
- P. Lei, S. Ayton and A. I. Bush, J. Biol. Chem., 2021, 296, 100105 CrossRef CAS PubMed.
- K. Cilliers, Clin. Anat., 2021, 34, 766–773 CrossRef PubMed.
- R. Gaudiuso, E. Ewusi-Annan, W. Xia and N. Melikechi, Spectrochim. Acta, Part B, 2020, 171, 105931 CrossRef CAS.
- N. Melikechi, Y. Markushin, D. C. Connolly, J. Lasue, E. Ewusi-Annan and S. Makrogiannis, Spectrochim. Acta, Part B, 2016, 123, 33–41 CrossRef CAS.
- N. Melikechi, H. G. Adler, A. Safi, J. E. Landis, F. Pourkamali-Anaraki, K. E. Eseller, K. Berlo, D. Bonito, G. R. Chiklis and W. Xia, Biomed. Opt. Express, 2024, 15, 446 CrossRef CAS.
- Y. Markushin, P. Sivakumar, D. Donnolly and N. Melikechi, Anal. Bioanal. Chem., 2015, 407, 1849–1855 CrossRef CAS.
- A. Bush, W. Pettingell, G. Multhaup, M. d Paradis, J.-P. Vonsattel, J. Gusella, K. Beyreuther, C. Masters and R. Tanzi, Science, 1994, 265, 1464–1467 CrossRef CAS.
- N. T. Watt, I. J. Whitehouse and N. M. Hooper, Int. J. Alzheimer's Dis., 2011, 2011, 971021 CrossRef.
- F.
N. C. Vaz, B. L. Fermino, M. V. L. Haskel, J. Wouk, G. B. L. de Freitas, R. Fabbri, E. Montagna, J. B. T. Rocha and J. S. Bonini, Biol. Trace Elem. Res., 2018, 181, 185–191 CrossRef CAS PubMed.
- R. González-Domínguez, T. García-Barrera and J. L. Gómez-Ariza, Metallomics, 2014, 6, 292–300 CrossRef.
- J.-Y. Lee, J.-H. Kim, D.-W. Choi, D.-W. Lee, J.-H. Park, H.-J. Yoon, H.-S. Pyo, H.-J. Kwon and K.-S. Park, Toxicol. Res., 2012, 28, 93–98 CrossRef CAS PubMed.
- E. Andrási, É. Farkas, H. Scheibler, A. Réffy and L. Bezúr, Arch. Gerontol. Geriatr., 1995, 21, 89–97 CrossRef.
- Y. He, H. Zhang, T. Wang, Z. Han, Q. Ni, K. Wang, L. Wang, Y. Zhang, Y. Hu, S. Jin, B. Sun and G. Liu, J. Alzheimer's Dis., 2020, 76, 713–724 CAS.
- K. Li, A. Li, Y. Mei, J. Zhao, Q. Zhou, Y. Li, M. Yang and Q. Xu, Environ. Pollut., 2023, 318, 120782 CrossRef CAS.
- C. E. Cicero, G. Mostile, R. Vasta, V. Rapisarda, S. S. Signorelli, M. Ferrante, M. Zappia and A. Nicoletti, Environ. Res., 2017, 159, 82–94 CrossRef CAS.
- D. D. Li, W. Zhang, Z.-Y. Wang and P Zhao, Front. Aging Neurosci., 2017, 9, 300 CrossRef CAS.
- H. Kessler, T. A. Bayer, D. Bach, T. Schneider-Axmann, T. Supprian, W. Hermann, M. Haber, G. Multhaup, P. Falkai and F.-G. Pajonk, J. Neural Transm., 2008, 67, 1181–1187 CrossRef.
- B. R. Cardoso, S. Braat and R. M. Graham, Front. Nutr., 2021, 8, 1–7 Search PubMed.
- Z. Gong, W. Song, M. Gu, X. Zhou and C. Tian, PLoS One, 2021, 16, e0255595 CrossRef CAS.
- K. Du, M. Liu, Y. Pan, X. Zhong and M. Wei, Nutrients, 2017, 9(3), 231 CrossRef.
- K. Du, X. Zheng, Z. T. Ma, J. Y. Lv, W. J. Jiang and M. Y. Liu, Front. Aging Neurosci., 2022, 13, 1–12 Search PubMed.
- G. Paglia, O. Miedico, A. Cristofano, M. Vitale, A. Angiolillo, A. E. Chiaravalle, G. Corso and A. Di Costanzo, Sci. Rep., 2016, 6, 22769 CrossRef CAS PubMed.
- M. Chen and W. Xia, J. Alzheimer's Dis., 2020, 76, 349–368 CAS.
- H.-K. Lee, C. Velazquez Sanchez, M. Chen, P. J. Morin, J. M. Wells, E. B. Hanlon and W. Xia, PLoS One, 2016, 11, e0163072 CrossRef.
-
D. D. Pokrajac, in Optical Spectroscopy and Imaging for Cancer Diagnostics, World Scientific, 2023, pp. 99–135 Search PubMed.
- M. A. Hearst, S. T. Dumais, E. Osuna, J. Platt and B. Scholkopf, IEEE Intelligent Systems and their Applications, 1998, 13, 18–28 Search PubMed.
- D. R. Cox, J. R. Stat. Soc. Ser. B Methodol., 1958, 20, 215–242 CrossRef.
- P. Fabian, V. Gaël, G. Alexandre, M. Vincent, T. Bertrand, G. Olivier, B. Mathieu, P. Peter, W. Ron, D. Vincent, V. Jake, P. Alexandre, C. David, B. Matthieu, P. Matthieu and D. Édouard, J. Mach. Learn. Res., 2011, 12, 2825–2830 Search PubMed.
- C.-Y. J. Peng, K. L. Lee and G. M. Ingersoll, J. Educ. Res., 2002, 96, 3–14 CrossRef.
- A. Vehtari, A. Gelman and J. Gabry, Stat. Comput., 2017, 27, 1413–1432 CrossRef.
-
D. S. Young, Handbook of Regression Methods, Chapman and Hall/CRC, Chapman and Hall/CRC, CRC Press, Boca Raton, 2017 Search PubMed.
- D. J. Hare, J. D. Doecke, N. G. Faux, A. Rembach, I. Volitakis, C. J. Fowler, R. Grimm, P. A. Doble, R. A. Cherny, C. L. Masters, A. I. Bush and B. R. Roberts, ACS Chem. Neurosci., 2015, 6, 398–402 CrossRef CAS.
- S. Y. Hwang and J. Kim, J. Clin. Nurs., 2016, 25, 1766–1776 CrossRef.
- Z. Wang, N. Li, M. Heizhati, L. Wang, M. Li, F. Pan, Z. Yang, R. Abudureyimu, J. Hong, L. Sun, J. Li and W. Li, Public Health Nutr., 2021, 24, 5795–5804 CrossRef.
- X. Na, M. Xi, Y. Zhou, J. Yang, J. Zhang, Y. Xi, Y. Yang, H. Yang and A. Zhao, Glob. Transit., 2022, 4, 28–39 CrossRef.
- R. A. Kronmal, J. R. Stat. Soc. Ser. A Stat. Soc., 1993, 156, 379 CrossRef.
- Y.-K. Tu, V. Clerehugh and M. S. Gilthorpe, J. Dent., 2004, 32, 143–151 CrossRef.
|
This journal is © The Royal Society of Chemistry 2024 |
Click here to see how this site uses Cookies. View our privacy policy here.