DOI:
10.1039/D3FO02803H
(Paper)
Food Funct., 2024,
15, 2760-2771
Fried food consumption, genetic risk, and incident obesity: a prospective study†
Received
11th July 2023
, Accepted 25th January 2024
First published on 31st January 2024
Abstract
Background and aims: Genetic and dietary factors contribute to adiposity risk, but little evidence supports genetic personalization of fried food intake recommendations for the management of obesity. This study aimed to assess the associations between fried food consumption and adiposity incidence and whether the associations were modified by an individual's genotype. Methods: We included 27
427 participants who had dietary data assessed by a validated 24 h dietary recall and available anthropometric information from the UK Biobank study. The genetic risk score (GRS) was calculated using 940 BMI associated variants. Results: With an average of 8.1 years of follow-up, 1472 and 2893 participants were defined as having overall obesity and abdominal obesity, respectively. Individuals in the highest categories of fried food consumption were positively associated with the risk of obesity (HR = 1.31; 95% CI 1.10–1.56) and abdominal obesity (HR = 1.27; 95% CI 1.12–1.45) compared with the lowest categories. Moreover, fried food consumption had a significant interatction with obesity GRS for abdominal obesity risk (P interaction = 0.016). Fried food intake was associated with a higher abdominal obesity risk (HR = 1.59, 95% CI: 1.25–2.00) among participants with a lower genetic risk. Conclusions: Our findings indicated that fried food consumption had a higher abdominal obesity risk among individuals with a lower genetic risk, suggesting the restriction of fried food intake for this group of people.
Introduction
Obesity has become a major public health crisis affecting 13% of adults globally.1 Obesity substantially increases the risk of chronic diseases, including cardiometabolic diseases, dementia, and cancers,2 thereby leading to declined life quality and expectancy and a heavy economic burden.3 Overweight and obesity problems in European countries have attracted attention,4 and the huge consumption of fast foods is the significant difference in dietary habits between Europe and other countries.5 Frying is a popular cooking method in Western countries, particularly in restaurants. Nevertheless, the nutrient composition of food can be changed under high temperature and high oil conditions, such as generating more trans-fat,6 which promotes weight gain, thus increasing the obesity risk.7 Meanwhile, frying elevates the calories of food and improves its color and flavor,8 resulting in excessive intake of fried food in daily life. In addition, most fried foods, such as French fries, fried meat, and donuts, are often high in protein and carbohydrates, which are considered a significant contributor to the daily dietary harmful contaminants, including advanced glycation end products, acrylamide, and heterocyclic aromatic amines during the frying process,9,10 which interfere with inflammation and oxidative stress, thus leading to a high risk of obesity.11
The latest meta-analysis report states that fried food consumption increases obesity risk and subsequently leads to other cardiometabolic diseases.12 Recent epidemiological discoveries described significant and positive association of fried food intake with adiposity incidence in Europe.13,14 Such studies, however, used food frequency questionnaires to calculate the intake of specific fried food or total fried food, which are more subject to social desirability bias.15 Comparatively, a 24 h diet recall can reduce the impact of recall bias and better capture precise information of foods consumed, especially that of fried foods.16 In addition, previous studies failed to examine the influence of specific fried foods on adiposity risk due to the insufficient size of the population or incomplete dietary information.17 Thus, it is unclear whether different subtypes of fried foods confer divergent obesogenic effects.
Although dietary factors such as overeating have been thought to be a main cause of adiposity, an individual's genotype may interact with dietary components related to obesity development, also known as the gene–diet interaction.18,19 Despite previous studies indicating the effect of significant interactions between fried food and certain genetic variants on the body mass index (BMI),17 none of the interactions have been robustly replicated on obesity risk, which could be due to insufficient statistical data and limited genetic variants. Recent genome-wide association studies (GWAS) have identified 941 independent single-nucleotide polymorphisms (SNPs) associated with the BMI that determine genetic susceptibility to weight gain,20 which considerably increased the predictive ability for obesity by genetic factors. Unfortunately, little knowledge on whether the effect of fried food consumption on obesity development is modified by the overall genetic burden has been recognized.
To address these major gaps, we assessed the relationships between fried food consumption and incident adiposity, including overall and abdominal obesity among participants in the UK Biobank cohort. Furthermore, we tested the potential interaction of fried food consumption with genetic risk of adiposity.
Materials and methods
Study population
The UK Biobank is a large cohort with clinical and genetic information on approximately half a million participants aged 37–73 y who were recruited between 2006 and 2010 across the UK. Participants were required to complete a baseline questionnaire, undergo various anthropometric measurements, report medical conditions, and provide biological samples. A detailed description of the UK Biobank is available in the following website: https://www.ukbiobank.ac.uk. The UK Biobank protocol was approved by the NHS North West Multicentre Research Ethics Committee (21/NW/0157). All participants provided informed consent at recruitment, allowing for follow-up using data-linkage to health records. This research was conducted using the UK Biobank resource (project ID 47365).
Overall, 59
646 participants with available data on anthropometric data at baseline (2006 to 2010) and at least one of three repeated measurements (2012 to 2013, 2014 to date, and 2019 to date) during the follow-up were initially included. After excluding patients with cancer, cardiovascular diseases, or BMI ≥30 kg m−2 at baseline, individuals without 24 h-diet recall information, and participants who withdrew during the follow-up, 27
427 participants were selected to analyze the associations of fried food consumption with incident obesity. To further assess the potential interaction of fried food intake with the genetic predisposition to obesity, we excluded individuals without genetic data and not of white British descent. Finally, a total of 26
250 participants were eligible for the gene–diet interaction assessment (ESI Fig. S1†).
Obesity genetic risk score
Detailed information on the genotyping consideration, quality control, and genetic imputation were described previously.21 We selected 940 SNPs associated with the BMI based on the GWAS (ESI Table S1†).20 The GRS was computed using 940 SNPs and their corresponding β coefficients were used in the followed equation:
,22 where β is the coefficient for each individual SNP and i is the number of risk allele of SNPs. The overall GRS ranged between 801.7 and 971.4. The individuals with a higher GRS were suggested to have a higher genetic predisposition of obesity.
Assessment of fried food consumption and covariates
The dietary data were assessed by using the Oxford WebQ questionnaire consisting of nearly 200 kinds of food items, which has been validated using biomarkers including protein, potassium, and total sugars.23 Participants were required to complete the questionnaire on five separate occasions (2009–2012) to account for seasonal variation and provide relatively accurate measurement for each individual food component. Total fried food consumption was calculated by summing the following food items, including crumbed or deep-fried poultry, breaded or battered fish, fried potatoes or crisp potatoes, omelette, Indian snacks, and doughnuts. Among these, we defined crumbed or deep-fried poultry and breaded or battered fish as fried white meat. In this study, total fried food consumption was considered to be primary exposure, while the most consumed types of fried foods (fried white meat and fried potatoes) were considered to be part of secondary exposure.
We constructed a diet quality score based on the Alternate Mediterranean Diet (AMED) ranging from 0 to 9 as previously described, while a higher score indicated a higher quality of diet (ESI Table S2†).24 Furthermore, potential confounders were collected by using the touch screen questionnaire, including age, sex, ethnicity, BMI, average household income, education, Townsend deprivation index, physical activity, smoking, alcohol drinking, disease history, medications and dietary supplements. The metabolic equivalent of task (MET) was calculated by using the International Physical Activity Questionnaire short form.24 A detailed description of these covariates has been provided elsewhere (https://www.ukbiobank.ac.uk). The sleep pattern was classified as described in a previous study.25
Obesity ascertainment
Adiposity indicators including the BMI, waist circumference (WC), and percentage of body fat (BF%) were measured in physical assessments between 2006 and 2010 by trained staffs. The three repeated reassessments of these anthropometric data had been conducted during 2012 to 2013, 2014 to date, and 2019 to date. The BMI was calculated by dividing body weight by height squared (kg m−2). Individuals who had a BMI ≥30 kg m−2 were diagnosed as obesity cases, while incident abdominal obesity was defined as WC ≥102 cm for men or WC ≥88 cm for women.26 Besides, secondary outcomes were considered to be a 5% increase in the BMI, WC, or BF% from baseline to the follow-up.
Statistical analyses
We calculated person-years from the date of attending the baseline assessment center until the date of diagnosis of adiposity, lost to follow-up, death, or end of follow-up (31 December 2020), whichever occurred first. To minimize random measurement errors, we calculated the cumulative average of fried food intake per day (2009–2012) and then grouped them into 0, 0–1, 1–1.5, and ≥1.5 servings per day. Multivariable Cox regression models were applied to estimate hazard ratios (HRs) and 95% confidence intervals (CIs) for adiposity in association with fried food intake. In the multivariable model, known or suspected confounders were considered according to previous literature and biological plausibility. Model 1 was adjusted for age and sex. Model 2 was further adjusted for centers, ethnicity, BMI, education, Townsend deprivation index, household income, physical activity, smoking status, alcohol consumption, sleep quality, total energy intake, and AMED score. Model 3 was additionally adjusted for the initial BMI, WC, or BF% where appropriate. All missing data were coded as an independent category if necessary. Tests for the P trend were calculated using the median value for each category of fried food intake as a continuous variable. The interaction between fried food consumption and GRS on the subsequent risk of developing adiposity was assessed by adding a multiplicative interaction term in our models. Continuous standardized values ([value-mean]/SD) of fried food consumption and GRS were utilized in the current study for appropriate scaling for clinical interpretation. Restricted cubic spline regression was used to determine the semi-parametric relationship of fried food consumption with adiposity. The GRS was also divided into low, medium, or high levels (tertiles) and we estimated HRs for general obesity and abdominal obesity according to the joint categories of fried food consumption and GRS (12 categories based on 4 categories of fried food intake and 3 categories of GRS, with low fried food intake and low GRS as the reference). In addition, the linear trend was tested by fitting the BMI, WC, or BF% as the continuous variable in the models.
We then conducted subgroup analyses to examine whether these associations varied by typical baseline characteristics. In sensitivity analyses, history of hypertension or diabetes, vitamin or mineral supplement, sedentary time, and the changes in lifestyle factors during the follow-up were further adjusted in the models. Second, we further excluded participants with a BMI ≤15 kg m−2, extreme energy intake (<500 or >3500 kcal d−1 for women and <800 or >4000 kcal d−1 for men),27 missing covariate data, or with cardiometabolic diseases during the follow-up.
All analyses were performed using SAS 9.4 software (SAS Institute, Cary, NC). A two-tailed P < 0.05 was defined as statistically significant.
Results
Population characteristics
The baseline characteristics of participants by different categories of fried food consumption are summarized in Table 1. Participants with higher fried food consumption tended to be men, younger, current smokers and alcohol drinkers, and had a higher total energy intake. They were less likely to be educated, had a high household income and sleep score, and used vitamin or mineral supplements (Table 1). Compared with non-consumers, those with higher fried potato or fried white meat consumption were more often men and smokers and had higher total energy intake, while they tended to be less educated and had lower sleep scores (ESI Table S3†). The average GRS of obesity in the current participants (n = 26
250) was 889.6 with a normal distribution (ESI Fig. S2†).
Table 1 Basic characteristics of participants from the UK Biobank according to fried food consumption
Characteristics |
Fried food consumption (servings per day) |
P value* |
0 |
0–1 |
1–1.5 |
≥1.5 |
BMI = body mass index, MET = metabolic equivalent, SBP = systolic blood pressure, DBP = diastolic blood pressure. Data are either percentages or means ± SDs unless indicated otherwise. *P value for continuous variables was estimated through unadjusted linear regression, treating quintile of fried food consumption as an ordinal variable, and Pearson's χ2 for categorical variables. £1.00 = $1.30, or €1.20. The Townsend deprivation index was an indicator of material deprivation that was calculated based on non-home ownership, non-car ownership, unemployment, and household over-crowding. |
N
|
10 232 |
10 408 |
4191 |
2596 |
|
Male (%) |
42.8 |
46.0 |
53.0 |
57.6 |
<0.001 |
Race (%) |
|
|
|
|
0.002 |
White |
97.1 |
97.5 |
96.9 |
96.0 |
|
Non-white |
2.7 |
2.2 |
2.8 |
3.7 |
|
Age (year) |
55.8 ± 7.4 |
55.6 ± 7.5 |
54.3 ± 7.7 |
53.2 ± 7.8 |
<0.001 |
BMI (kg m−2) |
24.9 ± 2.7 |
25.0 ± 2.7 |
25.2 ± 2.6 |
25.4 ± 2.6 |
<0.001 |
Physical activity (MET-h per wk) |
42.0 ± 38.9 |
40.2 ± 37.6 |
41.6 ± 40.1 |
42.0 ± 42.0 |
0.118 |
SBP (mmHg) |
136.6 ± 19.2 |
136.9 ± 19.1 |
136.1 ± 18.3 |
135.8 ± 18.4 |
0.030 |
DBP (mmHg) |
80.2 ± 10.3 |
80.6 ± 10.3 |
80.8 ± 10.6 |
80.8 ± 10.5 |
<0.001 |
Blood glucose (mmol L−1) |
5.0 ± 0.9 |
5.0 ± 0.8 |
4.9 ± 0.9 |
4.9 ± 0.9 |
0.111 |
Household income (£) (%)a |
|
|
|
|
<0.001 |
<18 000 |
10.4 |
9.8 |
9.0 |
9.4 |
|
18 000 to 30 999 |
20.1 |
21.0 |
20.6 |
18.3 |
|
31 000 to 51 999 |
27.4 |
27.2 |
28.9 |
30.7 |
|
52 000 to 100 000 |
26.3 |
26.5 |
26.8 |
27.8 |
|
>100 000 |
7.7 |
8.0 |
6.9 |
6.5 |
|
Education |
|
|
|
|
<0.001 |
College or University degree |
52.9 |
53.6 |
47.4 |
47.0 |
|
Vocational qualifications |
8.9 |
9.3 |
10.0 |
10.5 |
|
Optional national exams at age 17–18 years |
13.1 |
13.4 |
13.6 |
12.9 |
|
National exams at age 16 years |
20.2 |
19.4 |
23.3 |
24.8 |
|
Others |
4.7 |
4.1 |
5.5 |
4.6 |
|
Townsend deprivation indexb |
−2.0 ± 2.7 |
−2.1 ± 2.6 |
−2.0 ± 2.7 |
−1.9 ± 2.7 |
0.198 |
Smoking (%) |
|
|
|
|
<0.001 |
Never |
63.7 |
62.8 |
59.6 |
60.0 |
|
Previous |
30.8 |
31.7 |
33.2 |
31.9 |
|
Current |
5.4 |
5.3 |
6.9 |
7.9 |
|
Alcohol drinking (%) |
|
|
|
|
<0.001 |
Never or special occasions only |
23.1 |
20.9 |
21.2 |
21.6 |
|
1 to 3 times per month |
25.2 |
24.5 |
25.2 |
24.9 |
|
1 or 2 times per week |
29.2 |
29.4 |
29.9 |
29.1 |
|
3 or 4 times per week |
22.5 |
25.2 |
23.6 |
24.4 |
|
Sleep pattern |
|
|
|
|
<0.001 |
Poor |
4.0 |
3.3 |
4.2 |
5.2 |
|
Intermediate |
56.5 |
56.5 |
57.0 |
58.6 |
|
Healthy |
39.4 |
40.1 |
38.8 |
36.2 |
|
History of hypertension (%) |
45.7 |
46.8 |
44.5 |
44.3 |
0.016 |
History of high cholesterol (%) |
2.1 |
1.9 |
2.0 |
2.2 |
0.018 |
Mediterranean Diet Score |
3.9 ± 1.9 |
4.3 ± 1.8 |
3.7 ± 1.8 |
3.8 ± 1.8 |
<0.001 |
Energy intake (kcal) |
8359.4 ± 2446.3 |
8906.5 ± 2177.0 |
9594.2 ± 2515.0 |
10 559.0 ± 2974.4 |
<0.001 |
Vitamin use (%) |
32.9 |
30.9 |
31.7 |
30.6 |
0.008 |
Mineral use (%) |
14.3 |
12.9 |
11.2 |
10.7 |
<0.001 |
Relationship between fried food consumption and obesity risk
Over 222
158 person-years of follow-up (an average of 8.1 years), we documented 1472 and 2893 cases of obesity and abdominal obesity, respectively. In Model 1, fried food consumption was significantly and positively associated with incident obesity and abdominal obesity (Table 2). After further adjustment for lifestyle factors (Model 2), the HRs (95% CIs) comparing the highest frequent consumption of fried food (≥1.5 servings per day) with non-consumption were 1.41 (1.19–1.67) and 1.38 (1.21–1.57) for the risk of obesity and abdominal obesity, respectively. The HRs (95% CIs) across increasing categories of fried food were 1.10 (0.97–1.25), 1.06 (0.91–1.24), and 1.31 (1.10–1.56) for obesity risk and 1.09 (1.00–1.19), 1.06 (0.95–1.19) and 1.27 (1.12–1.45) for abdominal obesity risk in the fully adjusted models (Model 3), respectively. Each SD increment in fried food consumption was related to a 6% (1%–11%) higher obesity risk and a 5% (2%–9%) higher abdominal obesity risk. For different specific food groups, compared with the first categories, consumers in the highest categories of fried potato had a 45% higher risk of incident obesity and a 49% higher risk of abdominal obesity (ESI Table S4†). Nonetheless, non-significant associations were detected for fried white meat consumption (ESI Table S5†). The dose–response relationships for fried food consumption determined by the restricted-cubic-spline regression were all similar to those from the category analyses (Fig. 1).
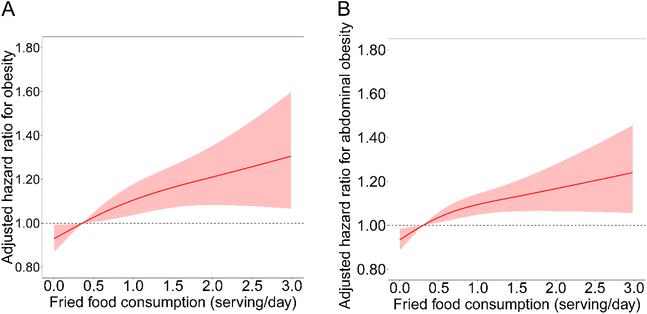 |
| Fig. 1 Dose–response relationships between fried food consumption and obesity/abdominal obesity risk. HRs for fried food consumption associated with obesity (A) and abdominal obesity (B) were estimated by restricted cubic-spline regression adjusted for age, sex, race, centers, education, Townsend deprivation index, household income, smoking, alcohol consumption, physical activity, sleep pattern, energy intake, and BMI (for obesity) or WC (for abdominal obesity) at baseline. | |
Table 2 Multivariable-adjusted HRs (95% CIs) for the association of fried food consumption with obesity incidence
|
Fried food intake (servings per day) |
P-trenda |
Per 1-SD |
P value |
0 |
0–1 |
1–1.5 |
≥1.5 |
BMI = body mass index, WC = waist circumference, BF = body fat, HRs = hazard ratios, CIs = confidence intervals. Cox proportional hazards regression analyses were used to calculate the HRs and 95% CIs. P-trend was obtained by including the categories of fried food intake as a continuous variable in the model. Model 1 was adjusted for age (continues) and sex (male or female). Model 2 was further adjusted for race (White or non-White), centers (22 categories), education (college or university degree, vocational qualifications, optional national exams at age 17–18 years, national exams at age 16 years, others, or missing), Townsend deprivation index (quartiles), household income (<£18 000, £18 000–£30 999, £31 000–£51 999, £52 000–£100 000, >£100 000, or missing), smoking (never, former, current, or missing), alcohol consumption (never or special occasions only, 1 or 2 times per week, 3 or 4 times per week, ≥5 times per week, or missing), physical activity (quartiles), sleep pattern (poor, medium or healthy), Mediterranean diet score (quartiles), and energy intake (quartiles). Model 3 was further adjusted for baseline BMI (in kg m−2; <18.5, 18.5 to 25, or 25 to 30) for obesity or adjusted for the baseline BMI (in kg m−2; <18.5, 18.5 to 25, or 25 to 30) and WC (quartiles) for abdominal obesity. Excluding 3 participants without data of WC at baseline or follow-up duration. Excluding 710 participants without data of BF% at baseline or follow-up duration. |
N
|
10 232 |
10 408 |
4191 |
2596 |
|
|
|
Obesity case
|
499 |
527 |
244 |
202 |
|
|
|
Model 1b |
1.00 (Ref.) |
1.09 (0.96–1.23) |
1.19 (1.02–1.38) |
1.59 (1.35–1.87) |
<0.001 |
1.12 (1.07–1.16) |
<0.001 |
Model 2c |
1.00 (Ref.) |
1.15 (1.02–1.30) |
1.15 (0.98–1.34) |
1.41 (1.19–1.67) |
<0.001 |
1.08 (1.03–1.12) |
<0.001 |
Model 3d |
1.00 (Ref.) |
1.10 (0.97–1.25) |
1.06 (0.91–1.24) |
1.31 (1.10–1.56) |
0.009 |
1.06 (1.01–1.11) |
0.018 |
Abdominal obesity case
|
1019 |
1074 |
467 |
333 |
|
|
|
Model 1b |
1.00 (Ref.) |
1.14 (1.04–1.24) |
1.26 (1.13–1.41) |
1.58 (1.39–1.79) |
<0.001 |
1.13 (1.09–1.16) |
<0.001 |
Model 2c |
1.00 (Ref.) |
1.16 (1.06–1.26) |
1.17 (1.04–1.30) |
1.38 (1.21–1.57) |
<0.001 |
1.09 (1.05–1.12) |
<0.001 |
Model 3d |
1.00 (Ref.) |
1.09 (1.00–1.19) |
1.06 (0.95–1.19) |
1.27 (1.12–1.45) |
0.002 |
1.05 (1.02–1.09) |
0.007 |
Case having a ≥5% BMI increase
|
1925 |
1946 |
884 |
629 |
|
|
|
Model 1b |
1.00 (Ref.) |
1.04 (0.98–1.11) |
1.13 (1.04–1.22) |
1.34 (1.23–1.47) |
<0.001 |
1.08 (1.05–1.10) |
<0.001 |
Model 2c |
1.00 (Ref.) |
1.06 (1.00–1.13) |
1.08 (1.00–1.18) |
1.22 (1.11–1.35) |
<0.001 |
1.05 (1.02–1.07) |
<0.001 |
Model 3d |
1.00 (Ref.) |
1.06 (1.00–1.13) |
1.09 (1.00–1.18) |
1.23 (1.12–1.35) |
<0.001 |
1.05 (1.02–1.07) |
<0.001 |
Case having a ≥5% WC increase
|
3357 |
3326 |
1420 |
898 |
|
|
|
Model 1b |
1.00 (Ref.) |
1.04 (0.99–1.09) |
1.09 (1.03–1.16) |
1.18 (1.09–1.27) |
<0.001 |
1.05 (1.03–1.07) |
<0.001 |
Model 2c |
1.00 (Ref.) |
1.05 (1.00–1.11) |
1.04 (0.98–1.11) |
1.10 (1.02–1.19) |
0.014 |
1.02 (1.00–1.04) |
0.055 |
Model 3d |
1.00 (Ref.) |
1.08 (1.03–1.13) |
1.08 (1.01–1.15) |
1.15 (1.07–1.25) |
<0.001 |
1.04 (1.01–1.06) |
0.003 |
Case having a ≥ 5% BF% increase
|
4740 |
4903 |
2080 |
1364 |
|
|
|
Model 1b |
1.00 (Ref.) |
1.07 (1.03–1.11) |
1.06 (1.01–1.12) |
1.13 (1.06–1.20) |
<0.001 |
1.03 (1.01–1.04) |
0.002 |
Model 2c |
1.00 (Ref.) |
1.07 (1.03–1.11) |
1.02 (0.96–1.07) |
1.05 (0.99–1.12) |
0.154 |
1.01 (0.99–1.02) |
0.172 |
Model 3d |
1.00 (Ref.) |
1.09 (1.05–1.14) |
1.05 (0.99–1.10) |
1.09 (1.03–1.16) |
0.004 |
1.01 (1.00–1.03) |
0.113 |
For adiposity indicators, we detected a positive association between fried food intake and participants having more than a 5% increase of the BMI (HRC4 vs. C1: 1.23, 95% CI: 1.12–1.35), WC (HRC4 vs. C1: 1.15, 95% CI: 1.07–1.25), and BF% (HRC4 vs. C1: 1.09, 95% CI: 1.03–1.16) in the fully adjusted model (Model 3) (Table 2). We also observed that fried potato consumption was positively associated with a higher risk for the BMI and BF% increase (ESI Table S4†). However, fried white meat consumption was not significantly associated with these adiposity indicators (ESI Table S5†).
Interactions of fried food consumption with the genetic risk of obesity
In the analyses according to continuous standardized values, we observed that the intake of fried food and fried potatoes and GRS were individually and positively associated with developing adiposity. Notably, we detected a significant interplay between fried food intake and GRS on future incident abdominal obesity (P interaction = 0.016) (Table 3).
Table 3 Interactions between fried food consumption and GRS on the risk of obesity and abdominal obesity and increase in adiposity indicator levels
|
GRS (n Loci = 940) |
|
Diet |
GRS |
Diet × GRS |
|
β* |
SE |
P
|
β* |
SE |
P
|
β* |
SE |
P
|
BMI = body mass index, WC = waist circumference, BF = body fat, GRS = genetic risk score. Cox proportional hazards regression analyses were used to calculate the HRs and 95% CIs. The model was adjusted for age (continuous), sex (male or female), race (White or non-White), centers (22 categories), education (college or university degree, vocational qualifications, optional national exams at age 17–18 years, national exams at age 16 years, others, or missing), Townsend deprivation index (quartiles), household income (<£18 000, £18 000–£30 999, £31 000–£51 999, £52 000–£100 000, >£100 000, or missing), smoking (never, former, current, or missing), alcohol consumption (never or special occasions only, 1 or 2 times per week, 3 or 4 times per week, ≥5 times per week, or missing), physical activity (quartiles), sleep pattern (poor, medium or healthy), Mediterranean diet score (quartiles), and energy intake (quartiles). The model was adjusted for age (continuous), sex (male or female), race (White or non-White), centers (22 categories), education (college or university degree, vocational qualifications, optional national exams at age 17–18 years, national exams at age 16 years, others, or missing), Townsend deprivation index (quartiles), household income (<£18 000, £18 000–£30 999, £31 000–£51 999, £52 000–£100 000, >£100 000, or missing), smoking (never, former, current, or missing), alcohol consumption (never or special occasions only, 1 or 2 times per week, 3 or 4 times per week, ≥5 times per week, or missing), physical activity (quartiles), sleep pattern (poor, medium, healthy), Mediterranean diet score (quartiles), energy intake (quartiles), and baseline BMI (in kg m−2; <18.5, 18.5 to 25 or 25 to 30) for obesity or adjusted for the baseline BMI (in kg m−2; <18.5, 18.5 to 25 or 25 to 30) and WC (quartiles) for abdominal obesity. Excluding 3 participants without data of WC at baseline or follow-up duration. Excluding 710 participants without data of BF% at baseline or follow-up duration. |
Fried food
|
Obesity |
0.070 |
0.025 |
0.005 |
0.156 |
0.027 |
<0.001 |
0.009 |
0.023 |
0.693 |
Abdominal obesity |
0.052 |
0.018 |
0.005 |
0.088 |
0.019 |
<0.001 |
−0.039 |
0.016 |
0.016
|
BMI increase |
0.103 |
0.014 |
<0.001 |
0.164 |
0.013 |
<0.001 |
0.012 |
0.012 |
0.337 |
WC increasea |
0.265 |
0.044 |
<0.001 |
0.318 |
0.042 |
<0.001 |
0.017 |
0.040 |
0.664 |
BF% increaseb |
0.181 |
0.025 |
<0.001 |
0.151 |
0.024 |
<0.001 |
0.004 |
0.023 |
0.855 |
Fried potato
|
Obesity |
0.062 |
0.025 |
0.012 |
0.158 |
0.028 |
<0.001 |
−0.002 |
0.022 |
0.918 |
Abdominal obesity |
0.071 |
0.019 |
<0.001 |
0.088 |
0.019 |
<0.001 |
−0.026 |
0.017 |
0.117 |
BMI increase |
0.109 |
0.014 |
<0.001 |
0.165 |
0.013 |
<.0001 |
−0.00001 |
0.013 |
1.000 |
WC increasea |
0.288 |
0.045 |
<0.001 |
0.320 |
0.042 |
<0.001 |
−0.011 |
0.042 |
0.783 |
BF% increaseb |
0.214 |
0.026 |
<0.001 |
0.153 |
0.024 |
<0.001 |
−0.005 |
0.024 |
0.839 |
Fried white meat
|
Obesity |
0.042 |
0.022 |
0.061 |
0.158 |
0.027 |
<0.001 |
0.013 |
0.020 |
0.519 |
Abdominal obesity |
−0.006 |
0.020 |
0.749 |
0.086 |
0.019 |
<0.001 |
−0.017 |
0.016 |
0.288 |
BMI increase |
0.015 |
0.013 |
0.255 |
0.164 |
0.013 |
<0.001 |
0.005 |
0.011 |
0.679 |
WC increasea |
−0.020 |
0.043 |
0.637 |
0.319 |
0.042 |
<0.001 |
0.0003 |
0.036 |
0.993 |
BF% increaseb |
0.017 |
0.025 |
0.501 |
0.152 |
0.024 |
<0.001 |
0.010 |
0.020 |
0.631 |
In category analyses, similar positive relationships were observed between fried food consumption and obesity risk across all genetic risk groups (Fig. 2A). Increased fried food was related to more increases in abdominal obesity among individuals with a low GRS risk (Fig. 2B). The HRs (95% CIs) of abdominal obesity risk across increasing categories of fried food were 0.99 (0.83–1.17), 1.17 (0.96–1.44), and 1.59 (1.25–2.00) in the fully adjusted model (P interaction = 0.015) (Fig. 2B). For the adiposity indicators, the consumption of fried food was positively associated with the BMI, WC, and BF% across all genetic risk groups (C4vs. C1: β = 0.74 for BMI, β = 1.78 for WC, β = 1.06 for BF%, all P < 0.001) (Fig. 3A–D).
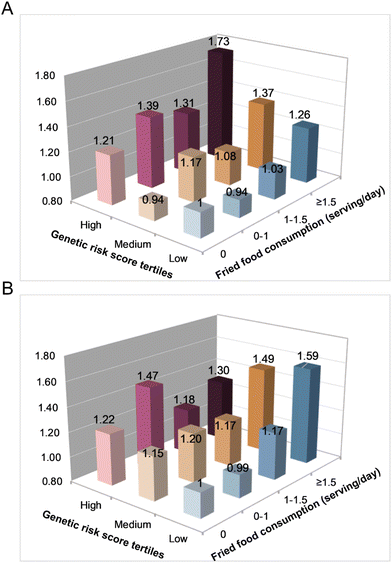 |
| Fig. 2 HRs of obesity and abdominal obesity according to the joint categories of the fried food consumption and the overall-obesity GRS. Cox proportional hazards regression analyses were used to calculated the HRs of obesity (A) and abdominal obesity (B) adjusting for age, sex, race, centres, education level, Townsend deprivation index, household income, smoking status, alcohol consumption, physical activity, sleep pattern, energy intake, and BMI (for obesity)/WC (for abdominal obesity) at baseline. | |
 |
| Fig. 3 The relationship between fried food consumption and adiposity indicators. The associations of fried food intake with the BMI, WC and BF% (A). The joint association of fried food consumption with the overall-obesity GRS on the BMI (B), WC (C), and BF% (D). General linear models were used to calculate the β and SE. BMI = body mass index, WC = waist circumference, BF = body fat, SE = standard error. The generalize linear model was adjusted for age, sex, race, centers, education, Townsend deprivation index, household income, smoking, alcohol consumption, physical activity, sleep pattern, energy intake, and BMI, WC or BF% at baseline (according to the outcome). Horizontal lines represent 95% CIs. Exclusion: 183 participants without data of WC at baseline or follow-up duration for the analysis of WC. Exclusion: 710 participants without data of BF% at baseline or follow-up duration for the analysis of BF%. | |
Subgroup analyses and sensitivity analyses
In subgroup analyses, the positive associations of fried food consumption with adiposity incidence were stronger among active alcohol drinkers (P for interaction = 0.040 for obesity and 0.005 for abdominal obesity) (ESI Fig. S3†). The relationships between the intake of fried potatoes or fried white meat and obesity risk were consistent regardless of baseline characteristics (ESI Fig. S4 and S5†).
In sensitivity analyses, the relationships between fried food intake and adiposity development did not change obviously after further adjustment for the history of hypertension or diabetes, the use of vitamin or mineral supplements, sedentary time, or changes in lifestyle factors (ESI Tables S6–S8†). Moreover, the results also appeared similar after excluding participants who developed cardiometabolic diseases during the follow-up, participants with an extremely low BMI (≤15 kg m−2) or extreme energy intake, or participants with missing covariate data (ESI Tables S6–S8†).
Discussion
In this large prospective cohort, we systematically reported the associations of the intake of total fried food and its main subtypes with the risk of developing adiposity and further investigated whether these relationships were modified by genetic predisposition with the BMI. We found that increased fried food consumption, especially fried potato consumption, was positively associated with adiposity risk. Findings from our current study demonstrated that a higher fried food intake was related to 31% and 27% higher risk of general obesity and abdominal obesity, respectively. Besides, the consumption of fried foods was also positively associated with adiposity indicators, including BMI, WC, and BF%. Notably, we first observed that individuals with a lower genetic predisposition to obesity might be more susceptible to the detrimental influence of excessive fried food consumption on abdominal obesity.
Since frying leads to the property of processed food with high fat and high energy density and thus links to the occurrence of adiposity,28 restricting fried food consumption is widely recommended to reduce obesity-related diseases.12 Few and inconsistent evidence has focused on the relationships between fried food consumption and obesity development in previous studies. A cross-sectional study has reported a 26% higher obesity risk with increased fried food intake among Spanish population.13 A previous twin cohort study based on the SUN project has also found that fried food intake had an increased obesity risk.14 However, we are simultaneously aware that a cross-sectional Korean study failed to detect a significant association of fried food consumption with obesity.29 These inconsistent results can partly be explained by differences in frying methods and foods in different countries. For example, fried meat was the most consumed fried food (45%), followed by vegetables (31%), fish (12%), and seaweed (8%) among Korean adults.29 High intake of healthy food with less energy such as vegetables among Korean population might attenuate the associations of fried food with obesity development.30 In the present study, we also found that fried potato but not fried white meat consumption was positively associated with obesity and abdominal obesity risk. This is in line with findings from a systematic review that increased consumption of French fries was related to a higher BMI and weight gain.31 The null associations between fried white meat intake and incident obesity may be attributed to diverse cooking methods and types of oil used.32 In the United Kingdom, fish or chicken is prepared in battered or breaded form, which might inhibit fat absorption and retain the quality attributes of fried fish or chicken.33 Evidence also showed that higher consumption of fish and marine n-3 PUFAs could reduce long-term weight gain.34 The high content of animal-sourced protein in chicken increases satiety, which may simultaneously attenuate energy intake.35 In addition, fried poultry and fish were usually cooked using butter that is rich in bone-building calcium, and the intake of these two fried foods was associated with a lower risk of obesity.36 Our findings, together with other previous studies,37 further highlighted the obesogenic role of fried food, especially fried potatoes, in developing adiposity risk.
Our current findings added to the existing literature on the interaction between genetic and dietary factors by assessing whether obesity-capturing GRS could interact with fried food on obesity risk. It is well-known that genetic factors modulate the associations between dietary factors and incident obesity.38 In terms of fried food, limited studies have examined the interplay between fried food intake and genetic susceptibility to BMI.17 In three US cohort studies, higher adherence to fried food consumption was related to an increased BMI among participants with a high GRS using 32 variants.17 However, the GRS captured by limited variants only reflects 1.5% of the variation in the BMI and might not precisely predict inherent risk for obesity.39 Our study used 940 BMI-associated SNPs based on the largest GWAS study, which could explain 6% of BMI variance20 and unprecedentedly enhance the accuracy of an individual's genetic risk on future development of adiposity. Our findings emphasized the synergistic genetic effect on abdominal obesity among individuals with a lower genetic risk who consumed more than 1.5 servings per day, which was about a 59% higher abdominal obesity risk compared with non-consumers. Moreover, individuals with a low GRS had a sharp increase in abdominal obesity risk related to higher consumption of fried food, which largely explained the significant interaction in the analysis using continuous variables (Table 3). A previous study indicated that the abdominal adipose tissue is the primary site for immediate storage of diet-derived fat.40 Among participants with a low genetic risk of obesity, higher intake of fried food might prefer affecting the body's fat distribution rather than the total weight, as the latter is genetically more difficult to change. Unfortunately, few GWAS studies have been conducted for WC so far; thus, studies on whether the association between fried food intake and the risk of abdominal obesity is modified by the variants of WC are warranted in the future. Overall, our present results of a significant interaction between diet and genetic predisposition to obesity risk emphasize the importance of sample size and the accuracy of obesity risk prediction by the GRS. Moreover, our results underlying the assessment of genetic risk could identify that individuals at increased disease risk and persons at a low GRS may be more susceptible to fried food intake, thus causing their inherited obesity risk.
There are several possible explanations for the positive associations of fried food intake with adiposity risk. Frying process increases fat content but dehydrates greatly,41 which produces fried foods with a high energy density. Frying also advances food palatability by improving its flavor and texture, which can lead to excess energy intake, thus increasing adiposity development.13 In addition, edible oil would be deteriorated by oxidation and hydrogenation reactions during frying, resulting in the decline of unsaturated fatty acids but the increase of trans fatty acids associated with a higher risk of obesity.42 Another explanation is that acrylamide is generated from foods rich in protein and carbohydrate, such as French fries and potato chips, during the frying process,43 which could play a vital role in the development of obesity, mainly driven by involving inflammation and oxidative stress.44,45 The significant positive relationships between fried potato consumption and adiposity risk in our study could be implicated by the association of acrylamide intake with obesity incidence. Previous epidemiological studies have supported this evidence that greater consumption of food containing a high acrylamide content was related to a higher risk of obesity and atherosclerotic lipid changes.46,47
The biological mechanisms underlying the observed interactions between fried food consumption and genetic predisposition to abdominal obesity risk remain unclear. Previous studies observed that fried food consumption was correlated with poor physical activity and excessive energy intake.17,41 Consistently, we also found that participants with greater fried food intake were more likely to have an unhealthier lifestyle, lower household income, poor sleep score, and higher total energy intake.17 Nevertheless, the documented interaction did not change after further adjustment for multiple lifestyle and dietary factors. Genetic variants such as FTO and MC4R associated with the regulation of appetite might personalize the effect of fried food consumption on adiposity.48,49 Previous evidence has also reported that FTO genetic variants could modify the function of total fat,50 saturated fat,51 and total energy intake on adiposity development.52 In this study, we found that fried food intake significantly interacts with individual's genetic risk on obesity incidence by calculating the cumulative effects of BMI-associated genetic variants including 940 SNPs. Notably, fried food intake affected individuals with a lower genetic risk more pronouncedly. Among individuals with high genetic burden of obesity, the influence of fried food intake might be weak, because the genetic factor may be the dominant factor, whereas individuals with a lower genetic risk may be mainly affected by dietary factors.53 However, the biological mechanism requires more research studies, especially experimental studies.
Our study had important strengths, including the use of a validated 24 h recall questionnaire for comprehensive assessment of dietary intake, objectively measured biomarkers of adiposity free of recall error from self-reports, a large sample size, and a long follow-up duration. We also had detailed information on multiple covariates that could potentially modify the relationships between fried food intake and obesity risk, which provided adequate statistical power to investigate the impact of fried food. Notably, we constructed novel polygenic scores of adiposity that capture 940 SNPs identified to be associated with BMI, which provided an accurate genetic risk prediction to fully examine the potential interactions in the gene–diet analyses.
Several limitations deserve attention. First, because our study was observational, the causal relationship between fried food intake and genetic risk and the development of obesity could not be inferred. Unmeasured or residual confounding factors could also not be completely ruled out, although we have comprehensively adjusted for potential confounding factors. Second, despite 24 h recall being acknowledged as the priority method for comprehensively recording dietary data, the information on the types of oil used for frying, frying time and temperature, frying method (deep, stir-frying, and griddling), and how often oil had been reused remains unclear. Although we further adjusted for the total energy intake, it was not possible to examine whether the interactions between fried food consumption and genetic predisposition to general obesity and abdominal obesity risk differed by these factors. Besides, inevitable measurement errors remained for 24 h dietary recall, such as recall bias and misreporting, though we had calculated an intake level of each food for all participants. Third, previous evidence indicated that obese individuals were more likely to underestimate the intake of unhealthy foods, such as snacks and French fries.54 Such underestimation of fried food consumption would dilute or attenuate the real relationships between fried food consumption and adiposity risk toward the null. Nevertheless, online questionnaires would have helped improve the accuracy of dietary intake, which is expected to minimize reporting bias due to societal expectations. Finally, due to the inclusion of European descent, our findings might not be immediately generalized to other ethnic groups.
Conclusion
In summary, our results demonstrated that the positive association of fried food consumption with adiposity risk was modified by an individual's genetic makeup. These results demonstrated that individuals with a low genetic risk might more likely suffer from adherence to fried food intake in developing adiposity. Our findings provide important evidence on limiting fried food consumption for adiposity prevention, especially among individuals genetically predisposed to abdominal obesity. More future research studies utilizing large population-wide biobanks and precise dietary information are warranted to corroborate our findings.
Abbreviations
BMI | Body mass index |
WC | Waist circumference |
BF% | Percentage of body fat |
GRS | Genetic risk score |
HR | Hazard ratio |
CI | Confidence interval |
GWAS | Genome-wide association study |
SNP | Single-nucleotide polymorphism |
AMED | Alternate Mediterranean diet |
MET | Metabolic equivalent of task |
Author contributions
JJJ and YZ conceived and designed the study. XZW, YA, XHL, PZ, and YYH performed the data cleaning, analysis and interpretation. XZW wrote the manuscript. YA and XHL provided statistical expertise and assistance. XHL, PZ, and HBS helped with the interpretation of the results and provided critical comments on the manuscript. All authors contributed to the interpretation of the data and critical revision of the manuscript for important intellectual content and approved the final draft. JJJ and YZ were involved in data acquisition. YZ is the guarantor.
Data availability
The UK Biobank data are available on application to the UK Biobank (https://www.ukbiobank.ac.uk/).
Ethics approval
The UK Biobank protocol was approved by the NHS North West Multicentre Research Ethics Committee (21/NW/0157).
Consent to participate
All participants provided informed consent at recruitment, allowing for follow-up using data-linkage to health records.
Conflicts of interest
The authors declare that they have no conflict of interest.
Acknowledgements
This research was conducted using the UK Biobank resource. The authors thank the participants of the UK Biobank. This research has been conducted using the UK Biobank Resource under Application Number 47365. This research was supported by the Zhejiang Provincial Natural Science Foundation of China (grant no. LZ20C200001).
References
-
World Health Organization, Factsheet on Obesity and overweight, 2018. Available from: https://www.who.int/news-room/fact-sheets/detail/obesity-andoverweight.
- M. Blüher, Obesity: global epidemiology and pathogenesis, Nat. Rev. Endocrinol., 2019, 15(5), 288–298 CrossRef PubMed.
- Y. Wang, L. Zhao, L. Gao, A. Pan and H. Xue, Health policy and public health implications of obesity in China, Lancet Diabetes Endocrinol., 2021, 9(7), 446–461 CrossRef PubMed.
- J. Krzysztoszek, I. Laudańska-Krzemińska and M. Bronikowski, Assessment of epidemiological obesity among adults in EU countries, Ann. Agric. Environ. Med., 2019, 26(2), 341–349 CrossRef PubMed.
-
Basicplanet, Top 10 countries with highest fast food consuming rates. Available from: https://www.basicplanet.com/top-10-countries-with-highest-fast-food-consuming-rates/.
- S. Bhat, D. Maganja, L. Huang, J. H. Y. Wu and M. Marklund, Influence of heating during cooking on trans fatty acid content of edible oils: a systematic review and meta-analysis, Nutrients, 2022, 14(7), 1489 CrossRef CAS PubMed.
- D. Mozaffarian, Dietary and policy priorities for cardiovascular disease, diabetes, and obesity: a comprehensive review, Circulation, 2016, 133(2), 187–225 CrossRef CAS PubMed.
- C. Chang, G. Wu, H. Zhang, Q. Jin and X. Wang, Deep-fried flavor: characteristics, formation mechanisms, and influencing factors, Crit. Rev. Food Sci. Nutr., 2020, 60(9), 1496–1514 CrossRef CAS PubMed.
- D. S. Mottram, B. L. Wedzicha and A. T. Dodson, Acrylamide is formed in the Maillard reaction, Nature, 2002, 419(6906), 448–449 CrossRef CAS PubMed.
- M. G. Knize, C. P. Salmon, P. Pais and J. S. Felton, Food heating and the formation of heterocyclic aromatic amine and polycyclic aromatic hydrocarbon mutagens/carcinogens, Adv. Exp. Med. Biol., 1999, 459, 179–193 CrossRef CAS PubMed.
- N. ALjahdali and F. Carbonero, Impact of Maillard reaction products on nutrition and health: current knowledge and need to understand their fate in the human digestive system, Crit. Rev. Food Sci. Nutr., 2019, 59(3), 474–487 CrossRef CAS PubMed.
- P. Qin, D. Liu, X. Wu, Y. Zeng, X. Sun, Y. Zhang, Y. Li, Y. Wu, M. Han, R. Qie, S. Huang, Y. Zhao, Y. Feng, X. Yang, Y. Liu, H. Li, M. Zhang, D. Hu and F. Hu, Fried-food consumption and risk of overweight/obesity, type 2 diabetes mellitus, and hypertension in adults: a meta-analysis of observational studies, Crit. Rev. Food Sci. Nutr., 2022, 62(24), 6809–6820 CrossRef CAS PubMed.
- P. Guallar-Castillón, F. Rodríguez-Artalejo, N. S. Fornés, J. R. Banegas, P. A. Etxezarreta, E. Ardanaz, A. Barricarte, M. D. Chirlaque, M. D. Iraeta, N. L. Larrañaga, A. Losada, M. Mendez, C. Martínez, J. R. Quirós, C. Navarro, P. Jakszyn, M. J. Sánchez, M. J. Tormo and C. A. González, Intake of fried foods is associated with obesity in the cohort of Spanish adults from the European Prospective Investigation into Cancer and Nutrition, Am. J. Clin. Nutr., 2007, 86(1), 198–205 CrossRef PubMed.
- C. Sayon-Orea, M. Bes-Rastrollo, F. J. Basterra-Gortari, J. J. Beunza, P. Guallar-Castillon, C. de la Fuente-Arrillaga and M. A. Martinez-Gonzalez, Consumption of fried foods and weight gain in a Mediterranean cohort: the SUN project, Nutr. Metab. Cardiovasc. Dis., 2013, 23(2), 144–150 CrossRef CAS PubMed.
- J. Cade, R. Thompson, V. Burley and D. Warm, Development, validation and utilisation of food-frequency questionnaires – a review, Public Health Nutr., 2002, 5(4), 567–587 CrossRef PubMed.
- Y. Park, K. W. Dodd, V. Kipnis, F. E. Thompson, N. Potischman, D. A. Schoeller, D. J. Baer, D. Midthune, R. P. Troiano, H. Bowles and A. F. Subar, Comparison of self-reported dietary intakes from the automated self-administered 24 h recall, 4-d food records, and food-frequency questionnaires against recovery biomarkers, Am. J. Clin. Nutr., 2018, 107(1), 80–93 CrossRef PubMed.
- Q. Qi, A. Y. Chu, J. H. Kang, J. Huang, L. M. Rose, M. K. Jensen, L. Liang, G. C. Curhan, L. R. Pasquale, J. L. Wiggs, I. De Vivo, A. T. Chan, H. K. Choi, R. M. Tamimi, P. M. Ridker, D. J. Hunter, W. C. Willett, E. B. Rimm, D. I. Chasman, F. B. Hu and L. Qi, Fried food consumption, genetic risk, and body mass index: gene-diet interaction analysis in three US cohort studies, Br. Med. J., 2014, 348, g1610 CrossRef PubMed.
- M. Song, Y. Zheng, L. Qi, F. B. Hu, A. T. Chan and E. L. Giovannucci, Associations between genetic variants associated with body mass index and trajectories of body fatness across the life course: a longitudinal analysis, Int. J. Epidemiol., 2018, 47(2), 506–515 CrossRef PubMed.
- S. Walter, I. Mejía-Guevara, K. Estrada, S. Y. Liu and M. M. Glymour, Association of a genetic risk score with body mass index across different birth cohorts, J. Am. Med. Assoc., 2016, 316(1), 63–69 CrossRef CAS PubMed.
- L. Yengo, J. Sidorenko, K. E. Kemper, Z. Zheng, A. R. Wood, M. N. Weedon, T. M. Frayling, J. Hirschhorn, J. Yang and P. M. Visscher, Meta-analysis of genome-wide association studies for height and body mass index in ∼700000 individuals of European ancestry, Hum. Mol. Genet., 2018, 27(20), 3641–3649 CrossRef CAS PubMed.
- C. Bycroft, C. Freeman, D. Petkova, G. Band, L. T. Elliott, K. Sharp, A. Motyer, D. Vukcevic, O. Delaneau, J. O'Connell, A. Cortes, S. Welsh, A. Young, M. Effingham, G. McVean, S. Leslie, N. Allen, P. Donnelly and J. Marchini, The UK Biobank resource with deep phenotyping and genomic data, Nature, 2018, 562(7726), 203–209 CrossRef CAS PubMed.
- T. Huang, Q. Qi, Y. Zheng, S. H. Ley, J. E. Manson, F. B. Hu and L. Qi, Genetic predisposition to central obesity and risk of type 2 diabetes: two independent cohort studies, Diabetes Care, 2015, 38(7), 1306–1311 CrossRef CAS PubMed.
- D. C. Greenwood, L. J. Hardie, G. S. Frost, N. A. Alwan, K. E. Bradbury, M. Carter, P. Elliott, C. E. L. Evans, H. E. Ford, N. Hancock, T. J. Key, B. Liu, M. A. Morris, U. Z. Mulla, K. Petropoulou, G. D. M. Potter, E. Riboli, H. Young, P. A. Wark and J. E. Cade, Validation of the Oxford WebQ online 24-hour dietary questionnaire using biomarkers, Am. J. Epidemiol., 2019, 188(10), 1858–1867 CrossRef PubMed.
- F. C. Bull, S. S. Al-Ansari, S. Biddle, K. Borodulin, M. P. Buman, G. Cardon, C. Carty, J. P. Chaput, S. Chastin, R. Chou, P. C. Dempsey, L. DiPietro, U. Ekelund, J. Firth, C. M. Friedenreich, L. Garcia, M. Gichu, R. Jago, P. T. Katzmarzyk, E. Lambert, M. Leitzmann, K. Milton, F. B. Ortega, C. Ranasinghe, E. Stamatakis, A. Tiedemann, R. P. Troiano, H. P. van der Ploeg, V. Wari and J. F. Willumsen, World Health Organization 2020 guidelines on physical activity and sedentary behaviour, Br. J. Sports Med., 2020, 54(24), 1451–1462 CrossRef PubMed.
- M. Fan, D. Sun, T. Zhou, Y. Heianza, J. Lv, L. Li and L. Qi, Sleep patterns, genetic susceptibility, and incident cardiovascular disease: a prospective study of 385 292 UK biobank participants, Eur. Heart J., 2020, 41(11), 1182–1189 CrossRef PubMed.
- C. Nishida, G. T. Ko and S. Kumanyika, Body fat distribution and noncommunicable diseases in populations: overview of the 2008 WHO expert consultation on waist circumference and waist-hip ratio, Eur. J. Clin. Nutr., 2010, 64(1), 2–5 CrossRef CAS PubMed.
-
W. Willett, Nutritional Epidemiology, Oxford University Press, 2012 Search PubMed.
- L. Fillion and C. J. Henry, Nutrient losses and gains during frying: a review, Int. J. Food Sci. Nutr., 1998, 49(2), 157–168 CrossRef CAS PubMed.
- Y. Kang and J. Kim, Association between fried food consumption and hypertension in Korean adults, Br. J. Nutr., 2016, 115(1), 87–94 CrossRef CAS PubMed.
- M. Nour, S. A. Lutze, A. Grech and M. Allman-Farinelli, The relationship between vegetable intake and weight outcomes: a systematic review of cohort studies, Nutrients, 2018, 10(11), 1626 CrossRef PubMed.
- D. Borch, N. Juul-Hindsgaul, M. Veller, A. Astrup, J. Jaskolowski and A. Raben, Potatoes and risk of obesity, type 2 diabetes, and cardiovascular disease in apparently healthy adults: a systematic review of clinical intervention and observational studies, Am. J. Clin. Nutr., 2016, 104(2), 489–498 CrossRef CAS PubMed.
- T. V. Gadiraju, Y. Patel, J. M. Gaziano and L. Djoussé, Fried food consumption and cardiovascular health: a review of current evidence, Nutrients, 2015, 7(10), 8424–8430 CrossRef CAS PubMed.
- C. Chen, J. Chen, Z. Yuan, E. Liao, W. Xia, H. Wang and Y. L. Xiong, Effect of the wheat starch/wheat protein ratio in a batter on fat absorption and quality attributes of fried battered and breaded fish nuggets, J. Food Sci., 2020, 85(7), 2098–2104 CrossRef CAS PubMed.
- T. Huang, T. Wang, Y. Heianza, Y. Zheng, D. Sun, J. H. Kang, L. R. Pasquale, E. B. Rimm, J. E. Manson, F. B. Hu and L. Qi, Habitual consumption of long-chain n-3 PUFAs and fish attenuates genetically associated long-term weight gain, Am. J. Clin. Nutr., 2019, 109(3), 665–673 CrossRef PubMed.
- J. Moon and G. Koh, Clinical evidence and mechanisms of high-protein diet-induced weight loss, J. Obes. Metab. Syndr., 2020, 29(3), 166–173 CrossRef PubMed.
- G. C. Major, J. P. Chaput, M. Ledoux, S. St-Pierre, G. H. Anderson, M. B. Zemel and A. Tremblay, Recent developments in calcium-related obesity research, Obes. Rev., 2008, 9(5), 428–445 CrossRef CAS PubMed.
- D. Mozaffarian, T. Hao, E. B. Rimm, W. C. Willett and F. B. Hu, Changes in diet and lifestyle and long-term weight gain in women and men, N. Engl. J. Med., 2011, 364(25), 2392–2404 CrossRef CAS PubMed.
- B. de Lauzon-Guillain, E. A. Clifton, F. R. Day, K. Clément, S. Brage, N. G. Forouhi, S. J. Griffin, Y. A. Koudou, V. Pelloux, N. J. Wareham, M. A. Charles, B. Heude and K. K. Ong, Mediation and modification of genetic susceptibility to obesity by eating behaviors, Am. J. Clin. Nutr., 2017, 106(4), 996–1004 CrossRef CAS PubMed.
- E. K. Speliotes, C. J. Willer, S. I. Berndt, K. L. Monda, G. Thorleifsson, A. U. Jackson, H. L. Allen, C. M. Lindgren, J. Luan, R. Mägi, J. C. Randall, S. Vedantam, T. W. Winkler, L. Qi, T. Workalemahu, I. M. Heid, V. Steinthorsdottir, H. M. Stringham, M. N. Weedon, E. Wheeler, A. R. Wood, T. Ferreira, R. J. Weyant, A. V. Segrè, K. Estrada, L. Liang, J. Nemesh, J. H. Park, S. Gustafsson, T. O. Kilpeläinen, J. Yang, N. Bouatia-Naji, T. Esko, M. F. Feitosa, Z. Kutalik, M. Mangino, S. Raychaudhuri, A. Scherag, A. V. Smith, R. Welch, J. H. Zhao, K. K. Aben, D. M. Absher, N. Amin, A. L. Dixon, E. Fisher, N. L. Glazer, M. E. Goddard, N. L. Heard-Costa, V. Hoesel, J. J. Hottenga, A. Johansson, T. Johnson, S. Ketkar, C. Lamina, S. Li, M. F. Moffatt, R. H. Myers, N. Narisu, J. R. Perry, M. J. Peters, M. Preuss, S. Ripatti, F. Rivadeneira, C. Sandholt, L. J. Scott, N. J. Timpson, J. P. Tyrer, S. van Wingerden, R. M. Watanabe, C. C. White, F. Wiklund, C. Barlassina, D. I. Chasman, M. N. Cooper, J. O. Jansson, R. W. Lawrence, N. Pellikka, I. Prokopenko, J. Shi, E. Thiering, H. Alavere, M. T. Alibrandi, P. Almgren, A. M. Arnold, T. Aspelund, L. D. Atwood, B. Balkau, A. J. Balmforth, A. J. Bennett, Y. Ben-Shlomo, R. N. Bergman, S. Bergmann, H. Biebermann, A. I. Blakemore, T. Boes, L. L. Bonnycastle, S. R. Bornstein, M. J. Brown, T. A. Buchanan, F. Busonero, H. Campbell, F. P. Cappuccio, C. Cavalcanti-Proença, Y. D. Chen, C. M. Chen, P. S. Chines, R. Clarke, L. Coin, J. Connell, I. N. Day, M. den Heijer, J. Duan, S. Ebrahim, P. Elliott, R. Elosua, G. Eiriksdottir, M. R. Erdos, J. G. Eriksson, M. F. Facheris, S. B. Felix, P. Fischer-Posovszky, A. R. Folsom, N. Friedrich, N. B. Freimer, M. Fu, S. Gaget, P. V. Gejman, E. J. Geus, C. Gieger, A. P. Gjesing, A. Goel, P. Goyette, H. Grallert, J. Grässler, D. M. Greenawalt, C. J. Groves, V. Gudnason, C. Guiducci, A. L. Hartikainen, N. Hassanali, A. S. Hall, A. S. Havulinna, C. Hayward, A. C. Heath, C. Hengstenberg, A. A. Hicks, A. Hinney, A. Hofman, G. Homuth, J. Hui, W. Igl, C. Iribarren, B. Isomaa, K. B. Jacobs, I. Jarick, E. Jewell, U. John, T. Jørgensen, P. Jousilahti, A. Jula, M. Kaakinen, E. Kajantie, L. M. Kaplan, S. Kathiresan, J. Kettunen, L. Kinnunen, J. W. Knowles, I. Kolcic, I. R. König, S. Koskinen, P. Kovacs, J. Kuusisto, P. Kraft, K. Kvaløy, J. Laitinen, O. Lantieri, C. Lanzani, L. J. Launer, C. Lecoeur, T. Lehtimäki, G. Lettre, J. Liu, M. L. Lokki, M. Lorentzon, R. N. Luben, B. Ludwig, P. Manunta, D. Marek, M. Marre, N. G. Martin, W. L. McArdle, A. McCarthy, B. McKnight, T. Meitinger, O. Melander, D. Meyre, K. Midthjell, G. W. Montgomery, M. A. Morken, A. P. Morris, R. Mulic, J. S. Ngwa, M. Nelis, M. J. Neville, D. R. Nyholt, C. J. O’Donnell, S. O’Rahilly, K. K. Ong, B. Oostra, G. Paré, A. N. Parker, M. Perola, I. Pichler, K. H. Pietiläinen, C. G. Platou, O. Polasek, A. Pouta, S. Rafelt, O. Raitakari, N. W. Rayner, M. Ridderstråle, W. Rief, A. Ruokonen, N. R. Robertson, P. Rzehak, V. Salomaa, A. R. Sanders, M. S. Sandhu, S. Sanna, J. Saramies, M. J. Savolainen, S. Scherag, S. Schipf, S. Schreiber, H. Schunkert, K. Silander, J. Sinisalo, D. S. Siscovick, J. H. Smit, N. Soranzo, U. Sovio, J. Stephens, I. Surakka, A. J. Swift, M. L. Tammesoo, J. C. Tardif, M. Teder-Laving, T. M. Teslovich, J. R. Thompson, B. Thomson, A. Tönjes, T. Tuomi, J. B. van Meurs, G. J. van Ommen, V. Vatin, J. Viikari, S. Visvikis-Siest, V. Vitart, C. I. Vogel, B. F. Voight, L. L. Waite, H. Wallaschofski, G. B. Walters, E. Widen, S. Wiegand, S. H. Wild, G. Willemsen, D. R. Witte, J. C. Witteman, J. Xu, Q. Zhang, L. Zgaga, A. Ziegler, P. Zitting, J. P. Beilby, I. S. Farooqi, J. Hebebrand, H. V. Huikuri, A. L. James, M. Kähönen, D. F. Levinson, F. Macciardi, M. S. Nieminen, C. Ohlsson, L. J. Palmer, P. M. Ridker, M. Stumvoll, J. S. Beckmann, H. Boeing, E. Boerwinkle, D. I. Boomsma, M. J. Caulfield, S. J. Chanock, F. S. Collins, L. A. Cupples, G. D. Smith, J. Erdmann, P. Froguel, H. Grönberg, U. Gyllensten, P. Hall, T. Hansen, T. B. Harris, A. T. Hattersley, R. B. Hayes, J. Heinrich, F. B. Hu, K. Hveem, T. Illig, M. R. Jarvelin, J. Kaprio, F. Karpe, K. T. Khaw, L. A. Kiemeney, H. Krude, M. Laakso, D. A. Lawlor, A. Metspalu, P. B. Munroe, W. H. Ouwehand, O. Pedersen, B. W. Penninx, A. Peters, P. P. Pramstaller, T. Quertermous, T. Reinehr, A. Rissanen, I. Rudan, N. J. Samani, P. E. Schwarz, A. R. Shuldiner, T. D. Spector, J. Tuomilehto, M. Uda, A. Uitterlinden, T. T. Valle, M. Wabitsch, G. Waeber, N. J. Wareham, H. Watkins, J. F. Wilson, A. F. Wright, M. C. Zillikens, N. Chatterjee, S. A. McCarroll, S. Purcell, E. E. Schadt, P. M. Visscher, T. L. Assimes, I. B. Borecki, P. Deloukas, C. S. Fox, L. C. Groop, T. Haritunians, D. J. Hunter, R. C. Kaplan, K. L. Mohlke, J. R. O’Connell, L. Peltonen, D. Schlessinger, D. P. Strachan, C. M. van Duijn, H. E. Wichmann, T. M. Frayling, U. Thorsteinsdottir, G. R. Abecasis, I. Barroso, M. Boehnke, K. Stefansson, K. E. North, M. I. McCarthy, J. N. Hirschhorn, E. Ingelsson and R. J. Loos, Association analyses of 249,796 individuals reveal 18 new loci associated with body mass index, Nat. Genet., 2010, 42(11), 937–948 CrossRef CAS PubMed.
- F. Karpe and K. E. Pinnick, Biology of upper-body and lower-body adipose tissue – link to whole-body phenotypes, Nat. Rev. Endocrinol., 2015, 11(2), 90–100 CrossRef CAS PubMed.
- Y. Sun, B. Liu, L. G. Snetselaar, J. G. Robinson, R. B. Wallace, L. L. Peterson and W. Bao, Association of fried food consumption with all cause, cardiovascular, and cancer mortality: prospective cohort study, Br. Med. J., 2019, 364, k5420 CrossRef PubMed.
- A. K. Thompson, A. M. Minihane and C. M. Williams, Trans fatty acids and weight gain, Int. J. Obes., 2011, 35(3), 315–324 CrossRef CAS PubMed.
- A. Koszucka, A. Nowak, I. Nowak and I. Motyl, Acrylamide in human diet, its metabolism, toxicity, inactivation and the associated European Union legal regulations in food industry, Crit. Rev. Food Sci. Nutr., 2020, 60(10), 1677–1692 CrossRef CAS PubMed.
- G. Jiang, A. Lei, Y. Chen, Q. Yu, J. Xie, Y. Yang, T. Yuan and D. Su, The protective effects of the Ganoderma atrum polysaccharide against acrylamide-induced inflammation and oxidative damage in rats, Food Funct., 2021, 12(1), 397–407 RSC.
- L. Zhang, L. Yang, Y. Luo, L. Dong and F. Chen, Acrylamide induced hepatotoxicity through oxidative stress: mechanisms and interventions, Antioxid. Redox Signal., 2022, 38(16–18), 1122–1137 Search PubMed.
- X. Wan, F. Zhu, P. Zhuang, X. Liu, L. Zhang, W. Jia, J. Jiao, C. Xu and Y. Zhang, Associations of hemoglobin adducts of acrylamide and glycidamide with prevalent metabolic syndrome in a nationwide population-based study, J. Agric. Food Chem., 2022, 70(28), 8755–8766 CrossRef CAS PubMed.
- I. Cheang, S. Liao, X. Zhu, X. Lu, Q. Zhu, W. Yao, Y. Zhou, H. Zhang and X. Li, Association of acrylamide hemoglobin biomarkers with serum lipid levels in general US population: NHANES 2013–2016, Ecotoxicol. Environ. Saf., 2021, 214, 112111 CrossRef CAS PubMed.
- B. Olson, X. Zhu, M. A. Norgard, P. R. Levasseur, J. T. Butler, A. Buenafe, K. G. Burfeind, K. A. Michaelis, K. R. Pelz, H. Mendez, J. Edwards, S. M. Krasnow, A. J. Grossberg and D. L. Marks, Lipocalin 2 mediates appetite suppression during pancreatic cancer cachexia, Nat. Commun., 2021, 12(1), 2057 CrossRef CAS PubMed.
- A. Dougkas, P. Yaqoob, D. I. Givens, C. K. Reynolds and A. M. Minihane, The impact of obesity-related SNP on appetite and energy intake, Br. J. Nutr., 2013, 110(6), 1151–1156 CrossRef CAS PubMed.
- E. Sonestedt, C. Roos, B. Gullberg, U. Ericson, E. Wirfält and M. Orho-Melander, Fat and carbohydrate intake modify the association between genetic variation in the FTO genotype and obesity, Am. J. Clin. Nutr., 2009, 90(5), 1418–1425 CrossRef CAS PubMed.
- D. Corella, D. K. Arnett, K. L. Tucker, E. K. Kabagambe, M. Tsai, L. D. Parnell, C. Q. Lai, Y. C. Lee, D. Warodomwichit, P. N. Hopkins and J. M. Ordovas, A high intake of saturated fatty acids strengthens the association between the fat mass and obesity-associated gene and BMI, J. Nutr., 2011, 141(12), 2219–2225 CrossRef CAS PubMed.
- T. Ahmad, I. M. Lee, G. Paré, D. I. Chasman, L. Rose, P. M. Ridker and S. Mora, Lifestyle interaction with fat mass and obesity-associated (FTO) genotype and risk of obesity in apparently healthy U.S. women, Diabetes Care, 2011, 34(3), 675–680 CrossRef PubMed.
- K. E. Mason, L. Palla, N. Pearce, J. Phelan and S. Cummins, Genetic risk of obesity as a modifier of associations between neighbourhood environment and body mass index: an observational study of 335 046 UK Biobank participants, BMJ Nutr. Prev. Health, 2020, 3(2), 247–255 CrossRef PubMed.
- F. Juul, E. Martinez-Steele, N. Parekh, C. A. Monteiro and V. W. Chang, Ultra-processed food consumption and excess weight among US adults, Br. J. Nutr., 2018, 120(1), 90–100 CrossRef CAS PubMed.
|
This journal is © The Royal Society of Chemistry 2024 |
Click here to see how this site uses Cookies. View our privacy policy here.