Differential biotransformation of micropollutants in conventional activated sludge and up-flow anaerobic sludge blanket processes†
Received
28th November 2023
, Accepted 16th February 2024
First published on 16th February 2024
Abstract
Biological wastewater treatment processes exhibit variable extents of micropollutant (MP) biotransformation. We hypothesize that the unique wastewater microbial communities in conventional activated sludge (CAS) and up-flow anaerobic sludge blanket (UASB) processes will perform different types of MP biotransformations at different rates. To test this hypothesis, we collected influent and effluent samples from a full-scale CAS and a pilot-scale UASB. We measured each sample by means of high-resolution mass spectrometry and frequently detected 39 MPs in both systems. We identify 17 MPs that exhibited better removal in the CAS, 15 MPs that exhibited better removal in the UASB, and 7 MPs that exhibited similar or no removal in both processes. We also conducted batch experiments in bioreactors seeded with wastewater microbial communities harvested from the CAS and UASB into which we spiked a mixture of 24 MPs of interest. Analysis of biotransformation products revealed that those formed exclusively in the batch CAS experiments were the result of oxidations and those formed in both batch CAS and UASB experiments were the result of redox-independent hydrolyses. Androsterone yielded different biotransformation products in the CAS and UASB experiments that align with the disparate redox conditions. Together, our data provide novel insights on the relative functioning of two wastewater microbial communities. Our study demonstrates the potential of the UASB to biotransform MPs and the relative biotransformation potential of CAS and UASB processes. We also present structures of eight biotransformation products, four of which have not been previously reported in the literature.
Water impact
In this study, we explore the differential biotransformation of micropollutants in conventional activated sludge and up-flow anaerobic sludge blanket processes. We couple field-scale measurements with laboratory-scale experiments to identify micropollutants that exhibit preferential biotransformation in each type of biological process. We also identify structures of biotransformation products and link putative biotransformations to the redox conditions of each process.
|
Introduction
Micropollutants (MPs) are defined as organic chemicals that can have a negative impact on the environment at concentrations in the μg L−1 range and lower.1,2 For example, pharmaceuticals and personal care products are down-the-drain chemicals that are conveyed to wastewater treatment plants (WWTPs) where they may only be partially removed before being discharged into receiving surface waters.1,3,4 Surface water systems that receive wastewater effluent often contain complex mixtures of organic MPs (and their biotransformation products) that are known to have adverse effects on aquatic ecosystems.5,6 A variety of studies have reported higher incidences of antibiotic resistance, endocrine effects, and reduced ecosystem diversity and functioning in effluent-dominated surface water systems.4,7,8 These observations motivate current research into enhanced removal of MPs during wastewater treatment.9
Conventional wastewater treatment relies on biological processes (e.g., conventional activated sludge (CAS) processes) to remove bulk carbon and nutrients from wastewater.10,11 Decades of studies on CAS processes have likewise demonstrated that some MPs can be removed during conventional wastewater treatment.12–16 There are two main mechanisms of MP removal: sorption to sludge17–19 and biotransformation.14,20–22 Sorption to sludge is relevant for more hydrophobic or apolar MPs (log
Kow > 4 or log
Kd > 1).18 Data have shown that adsorption can be a primary mechanism of MP removal for triclosan, perfluoroalkyl substances (PFASs), and fragrances used in personal care products.23,24 However, many MPs are polar or semi-polar and are not removed to a significant extent by means of adsorption.25 Previous research has demonstrated that some MPs are readily biotransformed by the wastewater microbial communities in CAS processes whereas other MPs are never biotransformed in CAS processes.12,14,16,26,27 The majority of MPs exhibit variable extents of biotransformation in CAS processes around the world reflecting some relationship to operational and environmental conditions that shape the structure and functioning of the wastewater microbial community.13,28–31 However, little is known about the operational and environmental conditions that might enhance the biotransformation of certain MPs and therefore it is not possible to optimize the performance of WWTPs for the removal of MPs.
Although most municipal WWTPs contain CAS processes, there is an increasing interest in alternative biological processes that might be better suited for certain wastewater characteristics or treatment objectives.32,33 One such alternative biological process is the up-flow anaerobic sludge blanket (UASB) reactor.29,32,34,35 UASB reactors can be advantageous in that they generate potentially valuable biogas during operation and consequently less biosolids for disposal.36 UASB reactors harbor microbial communities whose morphology and diversity are clearly distinct from those in the CAS process due to the formation of granular colonies.37–40 Whereas UASB reactors demonstrate competitive performance with CAS reactors when treating bulk carbon substrates, their potential to biotransform MPs has not been extensively studied.32 The UASB reactor is an interesting system in which to study MP biotransformations because of the unique morphology, structure, and functioning of the wastewater microbial community.32,41
The objectives of this research were to: (1) characterize MP removal (or biotransformation) at a full-scale CAS process and in a pilot-scale UASB reactor operating in parallel at the same WWTP; (2) identify MPs that exhibit comparatively faster biotransformation in one biological process over the other; and (3) identify biotransformation products for MPs that undergo differential biotransformations in the two systems. To meet these objectives, we first conducted a field sampling campaign in which we collected 1 L grab samples from the influent and effluent of both biological processes and quantified the MPs in each sample. We then conducted batch experiments with wastewater microbial communities harvested from each of the biological processes and spiked with 24 MPs that exhibited biotransformation in at least one of the biological processes. We found that several MPs exhibited differential biotransformation rates and products due to distinct redox conditions within each reactor. Our data reveal specific biotransformations that determine biotransformation rates in disparate biological processes operated in parallel.
Methods
Micropollutant standards and reagents
We selected 175 MPs for this study (the original mixture contained 184 MPs as previously described,42 but nine MPs could not be measured due to analytical issues related to their assigned isotope-labelled internal standard). The selected MPs are commonly observed in WWTPs and consist of pharmaceuticals, industrial chemicals, pesticides, human metabolites, and food additives. The selected MPs also encompass a broad range of chemical structures. Stock solutions of all MPs were prepared at 1 g L−1 in either LC-MS-grade methanol (OmniSolv, VWR), nanopure water (EMD Millipore), LC-MS-grade acetonitrile (Fisher Chemical), ethanol (Decon Labs), or dimethyl sulfoxide (Macron Fine Chemicals) and stored at −20 °C. A standard mixture of all MPs was created in nanopure water at 5 mg L−1 and stored at −20 °C. A list of the MPs, along with their CAS numbers, chemical formulas, and analytical parameters are provided in Table S1 of the ESI.† Similarly, a mixture of 51 isotope-labeled internal standards (ILIS) was created in nanopure water at 5 mg L−1 and stored at −20 °C. A list of the 51 ILISs is provided in Table S2.† Finally, an experimental mixture containing a subset of 24 of the MPs that exhibited biotransformation in at least one of the biological processes was prepared in nanopure water at a concentration of 20 mg L−1 and stored at −20 °C until use in batch experiments. A list of the 24 MPs in the experimental mixture is provided in Table S3.†
Field sampling campaign
We designed a field sampling campaign to characterize MP removal (or biotransformation) at a full-scale CAS within a municipal WWTP and in a pilot-scale UASB reactor operating at the same WWTP. The sampling campaign took place on September 20, 2022. Grab samples were collected in 4 L amber glass bottles that had been cleaned in an acid bath and pre-rinsed with methanol to remove any impurities and completely dried before use. Triplicate influent grab samples were collected at 1 hour intervals over two hours (three time points) to capture any temporal variability and triplicate effluent grab samples were offset from influent samples by the hydraulic retention time of the CAS (8 hours) and the UASB (9.5 hours). A total of three influent grab samples and three effluent grab samples were collected in this way from the CAS and the UASB process and processed individually as described in the following. The influent samples for the CAS process (noted as CAS_inf) were collected after primary settling and before mixing with the return activated sludge. The effluent samples for the CAS process (CAS_eff) were collected at the downstream end of the aeration basin. The UASB process is operated as a pilot-scale system at the same WWTP. The influent samples for the UASB process (UASB_inf) were collected from the outlet of a peristaltic pump that feeds untreated wastewater (after bar screening) to the UASB. The effluent samples from the UASB process (UASB_eff) were collected at the downstream end of the UASB. Schematics of the CAS and UASB processes along with sample locations are provided in Fig. S1 (ref. 43) and S2 of the ESI.† Bulk performance of the CAS and UASB processes is summarized in Table S4 of the ESI.†
Preparation of wastewater samples
All samples were filtered through Bunn coffee filters at the WWTP immediately after sampling to remove any unwanted solids and residue that would interfere with subsequent sample preparation. Triplicate influent samples (CAS_inf, UASB_inf) were collected in the morning, stored on ice until all triplicate samples were collected, and then immediately transported to the laboratory for sample preparation. Upon arrival in the laboratory, subsamples were collected to measure total suspended solids (TSS).42,43 The wastewater samples were then filtered with glass-fiber filters (grade GF/F, diameter 4.7 cm, pore size 0.7 μm, VWR) to remove suspended solids and subsequently with cellulose acetate filters (diameter 4.7 cm, pore size 0.45 μm, VWR) to generate sample filtrate for solid-phase extraction (SPE). CAS_inf and UASB_inf samples were diluted by a factor of ten in nanopure water. One liter of each triplicate influent sample (diluted and undiluted CAS_inf and UASB_inf) was titrated to a pH of 6.5 using dilute formic acid and spiked with 20 μL of the ILIS mixture such that each sample had an ILIS concentration of 100 ng L−1 before loading onto mixed-bed SPE cartridges to concentrate the samples by a factor of 1000 as previously described44,45 and as detailed in the ESI.† Triplicate effluent samples (CAS_eff, UASB_eff) were collected in the afternoon, stored on ice at the WWTP until all triplicate samples were collected, and then immediately transported to the laboratory for sample preparation and SPE.
Batch experiments with wastewater microbial communities from the UASB and CAS
Batch experiments with wastewater microbial communities harvested from the UASB and CAS were conducted in 100 mL clear glass serum bottles and 100 mL clear glass reactors (Corning), respectively. All reactors were covered in aluminum to block ambient light, and the experiments were carried out in triplicate. UASB microbial communities were harvested under anaerobic conditions from the base of the UASB reactor in 2 L amber glass bottles with no headspace. Harvested wastewater microbial communities were immediately transported to the laboratory and placed in an anaerobic chamber with a controlled atmosphere containing pure N2 and H2. The wastewater microbial communities were aseptically transferred into a 1 L clear glass bottle and diluted with deoxygenated wastewater effluent to a final TSS concentration of approximately 1 g L−1 to approximate the TSS of the CAS microbial communities and enable more robust comparisons of microbial activity (Table S4†). We then transferred 30 mL of the diluted wastewater microbial communities into triplicate serum bottles with a pipette and crimp sealed them with an airtight rubber stopper. CAS microbial communities were harvested from the middle of the CAS in 2 L amber glass bottles with ample headspace. We then transferred 30 mL of the collected microbial communities into triplicate glass reactors and capped them loosely to allow them to remain aerobic.
The respective reactors were then spiked with the mixture of 24 MPs using a hypodermic needle to achieve a starting concentration of 100 μg L−1 for each MP and placed on a rotary shaker at 20 °C; all incubation experiments were spiked within 5 h of sample collection. We collected 0.5 mL samples from each reactor after 5 min, 2 h, 6 h, 18 h, 30 h, 54 h, 69 h, 95 h, 118 h, 140 h, 165 h, and 190 h, transferred the samples to a 1.5 mL centrifuge tube (Eppendorf) and centrifuged the samples at 13
000 rpm for 5 min at 4 °C. Then, 400 μL of the supernatant was transferred to a 2 mL amber glass vial (VWR), capped, and stored at −20 °C until analysis. An adsorption control experiment was conducted with the same procedure, except reactors were autoclaved twice (120 °C, 1.3 bar, 20 min, 4 h apart) and spiked 24 h after the start of the active incubation experiment. A baseline MP control reactor was included to assess background concentrations of the 24 MPs and microbial activity in the absence of spiked MPs; this reactor contained the wastewater microbial community but was not spiked with MPs.
Sample analysis
We adopted a previously described analytical method for MP quantification and biotransformation product (TP) identification in samples collected in the field campaign and in the batch experiments46–50 as described in the ESI.† Analytical parameters for each target MP and its assigned ILIS are provided in Tables S1 and S2.†
Biotransformation product analysis
We used the Eawag-Pathway Prediction System (Eawag-PPS)51 to generate a list of predicted TPs with masses greater than 100 Da for select MPs that were biotransformed in the batch experiments. We generated SMILES for each of the predicted TPs and used ChemDraw Professional (2018 version 18.2.0.48) to calculate the exact mass of the [M + H]+ and [M − H]− ions for each predicted TP. We then used Xcalibur Qualbrowser (Thermo Scientific, Version 4.0.27.19) to visually screen HRMS acquisitions for evidence of TP formation in the samples from the batch experiment or the CAS_eff and UASB_eff samples. Evidence of biotransformation product formation includes: (i) peak areas greater than 1 × 105; (ii) reasonable peak shape; (iii) presence of a peak in the active reactors and absence of a peak (or a peak area less than 1 × 104) in control reactors; and (iv) increasing or increasing and then decreasing peak area over time (batch experiments).
Quality control
Because the wastewater samples from the field sampling campaign had a complex matrix, some of the 51 ILISs that we included in this study did not ionize efficiently. Therefore, we had to eliminate some ILISs from our processing method and reassign some of the target MPs to ILISs that could be measured across all samples. The results of this exercise are summarized in Table S2.† Reported concentrations from CAS_inf and UASB_inf samples are from the diluted samples and were corrected for the dilution. We used an in-house R script to match MS2 fragments to candidate target MP peaks in the wastewater samples from the sampling campaign (R script available at https://github.com/cmc493). Confirmed MP detection in any sample required at least one diagnostic fragment in one of the triplicate sample measurements. We only report concentrations of MPs where linear calibration curves consisted of at least three points and had an R2 greater than 0.85. Data quality parameters such as R2 and LOQ for target MPs are provided in Table S5.†
Data analysis
We used CAS_eff, UASB_eff, MP removal (%R_CAS or %R_UASB) and pseudo first-order biotransformation rate constants (kbio,CAS or kbio,UASB) as metrics to describe the performance of the two biological processes. The %R was calculated as: | 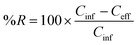 | (1) |
where Cinf is the concentration of an MP in the influent sample for the CAS or UASB (CAS_inf or UASB_inf) and Ceff is the concentration of an MP in the effluent sample for the CAS or UASB (CAS_eff or UASB_efff).
The kbio was calculated as:
| 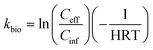 | (2) |
where HRT is the hydraulic retention time of the CAS or the UASB process. We also estimated a
kbio for each MP in the batch experiments by means of nonlinear regression and assuming pseudo-first order biotransformation kinetics. We found that the effect of sorption was limited for all of the MPs studied and we therefore used the first time point (
t = 5 min) in the active reactors as C(0) in the model.
48,52 Two-tailed
t-tests were performed to compare MP concentrations, removal percentages, and biotransformation kinetics between the CAS and UASB.
Results and discussion
Summary data from sampling of full-scale CAS and UASB
We collected triplicate grab samples from the influent and effluent of a full-scale CAS and pilot-scale UASB process at the same WWTP and measured each sample in triplicate (nine total data points for each sample location). The average TSS of the UASB was 21.8 g L−1 and the average TSS of the CAS was 1.2 g L−1 (Table S4†). We prepared the samples and measured them to assess the occurrence of up to 175 MPs. Our quality control requirement that MPs had linear calibration curves consisting of at least three points and an R2 greater than 0.85 reduced the number of possible MPs that could be quantified to 138. After matching MS2 fragments to candidate target MP peaks, we quantified 69 MPs in at least one of the CAS_inf samples and 68 MPs in at least one of the UASB_inf samples. Likewise, we quantified 79 MPs in at least one of the CAS_eff samples and 58 MPs in at least one of the UASB_eff samples. This accounting is summarized in Table S5† and average concentrations measured in each sample location is provided in Table S6.† Many of the MP quantifications were sporadic, and sometimes only one of the triplicate samples from a single sample location and time contained a quantifiable MP. When comparing the two systems, we identified 39 MPs that were consistently present in the influent samples from both the CAS and UASB processes. We summarized the concentrations of these 39 MPs in each of the samples as box plots in Fig. 1. The concentrations of all 39 MPs range from <LOQ to 169 μg L−1 (the highest observed concentration was for benzoylecgonine in a UASB_eff sample). In the UASB and CAS influent, the highest average MP concentrations were observed for acetaminophen at concentrations of 111 ± 14 μg L−1 and 61 ± 3 μg L−1, respectively. Whereas in the UASB and CAS effluent, benzotriazole methyl-1H displayed the highest average concentrations of 70 ± 8 μg L−1 and 47 ± 6 μg L−1, respectively, revealing a shift in the predominant compounds from the influent to the effluent of the UASB and CAS systems (see Fig. 1 for additional details).
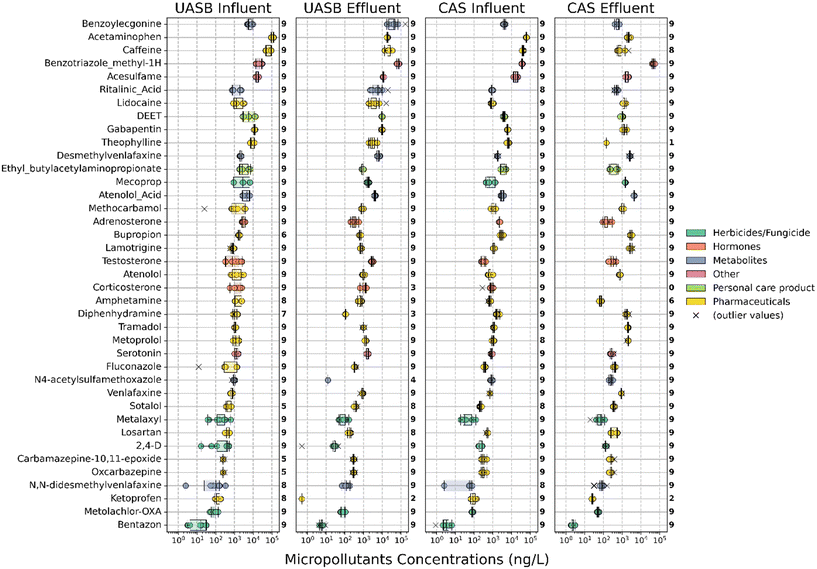 |
| Fig. 1 Concentrations of MPs in field sampling organized by chemical class. Each MP was subject to nine measurements (triplicate sampling and instrumental analysis) in UASB influent, UASB effluent, CAS influent, and CAS effluent. The number of measurements that exceeded the limit of quantification for each MP was indicated on the right of each box. The line inside the box represents the median of the dataset. The top and bottom edges of the box correspond to Q3 and Q1, respectively. The whiskers are lines that extend from the box and reach out to individual data points that are not considered outliers. | |
Comparing MP removal performance in both systems
We then evaluated the performance of the CAS and UASB for removing (or biotransforming) the 39 MPs that were measured in the influent samples from both processes. To do this we defined three metrics. First, we used a direct comparison of the average CAS_eff and UASB_eff data for each of the 39 MPs. Because we collected samples at three time points and measured each of the samples in triplicate, these data represent the average of nine measurements for each of the 39 MPs. We reasoned that the overall process (i.e., the CAS and UASB biological processes and all preceding treatment processes) that achieved a lower average Ceff for a given MP was performing better for that MP. Second, we calculated MP removal (%R_CAS or %R_UASB) as defined in eqn (1). MP removal was again calculated using the average of nine measurements for each MP. This metric allows us to compare the performance of each of the wastewater microbial communities and accounts for the different Cinf concentrations for each process. Third, we calculated pseudo-first order biotransformation rate constants (kbio,CAS or kbio,UASB) as defined in eqn (2). Biotransformation rate constants were calculated with the average of nine measurements and incorporates the hydraulic retention time (HRT) of each process. This metric allows us to compare the relative activity of the wastewater microbial communities by normalizing removal to the amount of time available for biotransformation.
A comparison of the average CAS_eff and UASB_eff data for each of the 39 MPs is provided as measures of significant differences in Table 1. These data demonstrate that eleven of the 39 MPs have significantly lower effluent concentrations in the UASB versus the CAS. These include ketoprofen, N4-acetylsulfamethosazole, 2,4-D, diphenhydramine, bupropion, methocarbamol, losartan, tramadol, lamotrigine, metoprolol, and fluconazole. It is difficult to assess whether the effluent concentrations are the result of sorption to sludge or biotransformation. One could expect more sorption in the UASB because the TSS was 18.2× higher than in the CAS. Further, more sorption would be expected for more hydrophobic MPs with relatively high log
Kow and log
Koc values. To test this expectation, we compared the log
Kow and log
Koc values (Table S3†) of the eleven MPs that had lower effluent concentrations in the UASB to the other 28 MPs and found no statistically significant difference. This finding suggests that adsorption is not likely to be a governing removal process in the UASB and that biotransformation in the UASB is likely important for at least for some of these eleven MPs. Six MPs did not exhibit significantly different effluent concentrations between the UASB and the CAS. These include atenolol acid, carbamazepine-10,11-epoxide, oxcarbazepine, sotalol, corticosterone, and venlafaxine which are MPs that are often found to be persistent during wastewater treatment.53,54 The remaining 22 MPs exhibited significantly lower effluent concentration in the CAS and are presumed to be better removed in the CAS process based on this metric.
Table 1 MPs that were determined to be better removed in the CAS or UASB across 3 metrics
Micropollutant (MP) |
Effluent concentration |
Percent removal |
First order rate kinetic |
CAS |
UASB |
NS |
CAS |
UASB |
NS |
CAS |
UASB |
NS |
Abbreviations: N4 = N4-acetylsulfamethoxazole, EBAP = ethyl_butylacetylaminopropionate, CBZ-10,11-E = carbamazepine-10,11-epoxide, N,N = N,N-didesmethylvenlafaxine, BTM1H = benzotriazole_methyl-1H, DMVF = desmethylvenlafaxine. NS = no significant difference between UASB and CAS performance. *p < 0.05, **p < 0.01, ***p < 0.001, X = not statistically significant, XX = no removal, negative removal, or negative rate constant. |
Ketoprofen |
|
** |
|
|
*** |
|
|
* |
|
N4 |
|
** |
|
|
** |
|
|
|
X |
2,4-D |
|
*** |
|
|
*** |
|
|
|
X |
Diphenhydramine |
|
*** |
|
|
*** |
|
|
** |
|
Adrenosterone |
* |
|
|
* |
|
|
|
|
X |
Acetaminophen |
*** |
|
|
** |
|
|
*** |
|
|
Bupropion |
|
** |
|
|
** |
|
|
* |
|
Methocarbamol |
|
* |
|
|
** |
|
|
|
X |
EBAP |
*** |
|
|
** |
|
|
* |
|
|
Losartan |
|
* |
|
|
|
X |
|
|
X |
Caffeine |
** |
|
|
** |
|
|
** |
|
|
Theophylline |
** |
|
|
** |
|
|
* |
|
|
Acesulfame |
*** |
|
|
** |
|
|
* |
|
|
Amphetamine |
** |
|
|
*** |
|
|
** |
|
|
Metalaxyl |
* |
|
|
|
* |
|
|
* |
|
Bentazon |
** |
|
|
|
|
X |
|
|
X |
Gabapentin |
** |
|
|
** |
|
|
* |
|
|
Sotalol |
|
|
X |
|
*** |
|
|
* |
|
Atenolol |
* |
|
|
|
* |
|
|
|
X |
Mecoprop |
** |
|
|
|
* |
|
|
|
X |
Tramadol |
|
** |
|
|
*** |
|
|
** |
|
Lamotrigine |
|
*** |
|
|
* |
|
|
* |
|
Atenolol_acid |
|
|
X |
|
|
XX |
|
|
X |
CBZ-10,11-E |
|
|
X |
* |
|
|
* |
|
|
Oxcarbazepine |
|
|
X |
* |
|
|
* |
|
|
Metoprolol |
|
*** |
|
|
|
XX |
|
|
XX |
N,N |
* |
|
|
|
|
XX |
|
|
XX |
Venlafaxine |
|
|
X |
|
|
XX |
|
|
XX |
Serotonin |
*** |
|
|
** |
|
|
** |
|
|
Metolachlor-OXA |
* |
|
|
** |
|
|
|
|
X |
Corticosterone |
|
|
X |
|
|
X |
|
|
X |
DEET |
*** |
|
|
* |
|
|
* |
|
|
Fluconazole |
|
** |
|
|
|
XX |
|
|
X |
BTM1H |
* |
|
|
|
|
XX |
|
|
XX |
DMVF |
** |
|
|
|
|
XX |
|
|
XX |
Testosterone |
*** |
|
|
|
|
XX |
|
|
X |
Lidocaine |
** |
|
|
|
|
XX |
|
|
XX |
Ritalinic_acid |
** |
|
|
** |
|
|
* |
|
|
Benzoylecgonine |
** |
|
|
** |
|
|
** |
|
|
The middle columns in Table 1 can be used to compare the average percent removal between the CAS and UASB. These data demonstrate that twelve of the 39 MPs have significantly higher %R_UASB than %R_CAS. These include ketoprofen, N4-acetylsulfamethosazole, 2,4-D, diphenhydramine, bupropion, methocarbamol, metalaxyl, sotalol, atenolol, mecoprop, tramadol, and lamotrigine. Eight of these also had lower UASB_eff than CAS_eff highlighting the better performance of the UASB for these MPs using two independent metrics. The other four MPs did not have significantly different or lower effluent concentrations in CAS_eff compared to UASB_eff (metalaxyl, sotalol, atenolol, and mecoprop). In these cases, accounting for the higher UASB_inf concentration (relative to CAS_inf) demonstrates better removal of these MPs. We believe that the lower concentration of these MPs in the CAS_inf sample is due to some removal during primary sedimentation.55 Interestingly, losartan, metoprolol, and fluconazole were found to have lower effluent concentrations in the UASB than the CAS, but the percent removal was not significantly different in the UASB and CAS. Indeed, there were nine MPs that exhibited no removal or negative removal in both systems including atenolol acid, metoprolol, N,N-didesmethylvenlafaxine, venlafaxine, fluconazole, benzotriazole-methyl-1H, desmethylvenlafaxine, testosterone, and lidocaine. The phenomenon of negative removal has been reported before for some of these MPs53,54 and is attributed to formation resulting from back-transformation of human metabolites or the MP being a TP itself56–58 or variable biotransformation within a biological process.42 The remaining 15 MPs exhibited significantly higher removal in the CAS and are presumed to be better removed in the CAS based on this metric (t-test, p < 0.05).
A direct comparison of the pseudo first-order biotransformation rate constants (kbio,CAS or kbio,UASB) for each of the 39 MPs is provided in the last three columns of Table 1. These data demonstrate that seven of the 39 MPs have significantly higher kbio,UASB than kbio,CAS. These include ketoprofen, diphenhydramine, bupropion, metalaxyl, sotalol, tramadol, and lamotrigine. Five of these MPs also had lower average MP concentrations in UASB_eff than CAS_eff and higher %R_UASB than %R_CAS highlighting the better performance of the UASB for these five MPs using all three of our defined metrics. In fact, ten of the 15 MPs were identified as being better removed in the UASB by at least two separate metrics; the exceptions are losartan, fluconazole, atenolol, metoprolol, and mecoprop which were only identified by one metric. We also report on six MPs that exhibited pseudo first-order biotransformation rate constants equal to zero (or a negative value suggesting formation, which is a phenomenon previously reported in the literature as described in the preceding).56–58 These include metoprolol, N,N-didesmethylvenlafaxine, venlafaxine, benzotriazole-methyl-1H, desmethylvenlafaxine, and lidocaine. We also report on 13 MPs that did not exhibit significantly different rate constants between the UASB and the CAS, and the remaining 13 MPs exhibited significantly higher kbio,CAS than kbio,UASB. The 13 MPs that exhibited significantly higher kbio,CAS than kbio,UASB were identified by at least one of our other metrics as MPs that are better removed in the CAS system.
In summary, our analysis identified 15 MPs that exhibited significantly better removal in the UASB based on at least one of our metrics (ketoprofen, N4-acetylsulfamethosazole, 2,4-D, diphenhydramine, bupropion, methocarbamol, losartan, metalaxyl, sotalol, atenolol, metoprolol, mecoprop, tramadol, lamotrigine, and fluconazole) and 17 MPs that exhibited significantly better removal in the CAS based on at least one of our metrics (adrenosterone, acetaminophen, ethyl-butylacetylaminopropionic acid, caffeine, theophylline, acesulfame, amphetamine, gabapentin, carbamazepine-10,11-epoxide, oxcarbazepine, serotonin, metolachlor-oxa, corticosterone, DEET, testosterone, ritalinic acid, and benzoylecgonine). Seven of the MPs exhibited no removal or similar removal in both systems. It is interesting to note that there was nearly an even split of MPs that were better removed by the two processes. This was also somewhat unexpected because previous studies show that MPs are readily biotransformed in aerobic biological processes whereas anaerobic biological processes often perform MP biotransformations more slowly or not at all.50,59,60 To investigate these findings further, we performed batch experiments to assess biotransformation rates and explore the formation of biotransformation products.
Batch experiments – biotransformation kinetics
We performed batch experiments in bioreactors seeded with microbial communities harvested from the UASB and CAS processes. We selected 12 MPs that were found to be better removed in the pilot-scale UASB (ketoprofen, N4-acetylsulfamethosazole, 2,4-D, diphenhydramine, bupropion, methocarbamol, losartan, bentazone, sotalol, mecoprop, tramadol, and lamotrigine) and 12 MPs that were found to be better removed in the full-scale CAS (adrenosterone, acetaminophen, ethyl-butylacetylaminopropionic acid, caffeine, theophylline, acesulfame, amphetamine, gabapentin, serotonin, DEET, ritalinic acid, and benzoylecgonine). A mixture of the 24 MPs was spiked into each of the triplicate prepared bioreactors to reach a starting concertation of each MP of 100 μg L−1. Samples were collected over a period of eight days and the resulting data were used to estimate pseudo-first order biotransformation rate constants. The results of this analysis demonstrate that 18 of the 24 MPs were biotransformed in the UASB batch experiments and all 24 of the MPs were biotransformed in the CAS batch experiments. The six MPs that exhibited no biotransformation in the UASB batch experiments include three that were removed to greater extents in the pilot-scale UASB (mecoprop, tramadol, and lamotrigine) and three that were removed to greater extents in the full-scale CAS (amphetamine, gabapentin, and ritalinic acid). Notably, only 2,4-D exhibited faster biotransformation kinetics in the UASB batch experiments compared to the CAS batch experiments. Together, these observations suggest that the batch systems are not replicating the MP biotransformation rates that were observed in the pilot-scale UASB and the full-scale CAS. There are several likely reasons for this discrepancy. First, we diluted the UASB sludge by a factor of 33.3× to ensure that we have equivalent biomass densities in the respective batch experiments to allow us to compare the relative activities of the two microbial communities. However, dilution can lower the diversity of the microbial community through the bottleneck effect and can eliminate low abundance members of the microbial community that may participate in MP biotransformations.61 This could explain why we observed no biotransformation of mecoprop, tramadol, and lamotrigine in the batch experiments with the UASB sludge. It is also possible that some of the removal observed in the pilot-scale system was driven by adsorption to sludge and that removal by means of adsorption significantly decreased when we diluted the sludge. Although this is a plausible explanation for some MPs, an analysis of log
Kow and log
Koc values (Table S3†) along with literature reported sludge–water distribution coefficients18,23,62 suggests that sorption is not a major removal mechanism for these MPs. Finally, we note that harvesting wastewater microbial communities from full-scale or pilot-scale systems that are operating at steady-state and placing them in batch reactor in the lab is likely to cause some changes in microbial community structure and function. This has been discussed in the literature to some extent when evaluating MP biotransformations in bioreactors seeded with sludge from CAS processes,63,64 and is likewise relevant here for the experiments with the UASB sludge. In the latter case, the bioreactors were established under anaerobic conditions in an atmosphere consisting of N2 and H2. These conditions might not align with those in the pilot-scale system which further complicates this comparison.
Nevertheless, it is important to emphasize that we did see biotransformation of 16 MPs in the batch experiments seeded with sludge from the UASB. This is an important observation in its own right because the performance of wastewater microbial communities from UASB systems to biotransform MPs has not been studied to a significant extent. Also, we note that the difference in the magnitudes of the rate constants between the UASB and CAS batch experiments are smaller for the MPs that were observed to be better removed in the pilot-scale UASB. To explore this latter observation more deeply, we corrected the rate constants for the MPs in the UASB experiment to account for the 33.3× dilution factor used to equalize the biomass concentrations. The results of this analysis are presented in Fig. 2. Here we can see that six of the nine MPs that were biotransformed in our batch experiments and were better removed in the pilot-scale UASB have significantly greater rate constants in the UASB batch experiments than in the CAS batch experiments (t-test, p < 0.05). The other three MPs do not have significantly different rate constants in both sets of batch experiments. In contrast, only two of nine MPs that were biotransformed in our batch experiments and were better removed in the full-scale CAS have significantly greater rate constants in the UASB experiments than in the CAS experiments. This correction demonstrates that biotransformation rates measured in batch can represent trends observed at pilot- and full-scale when one corrects for biomass dilution.
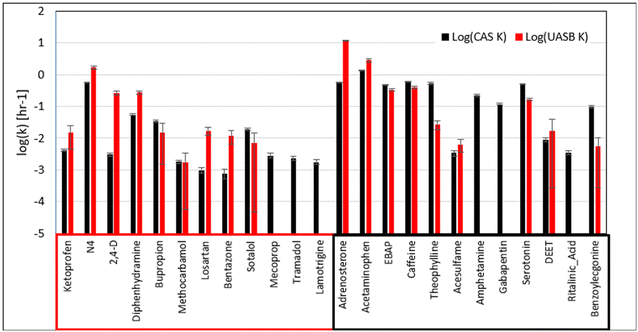 |
| Fig. 2 Log rate constants of the MPs in batch tests after correcting for dilution of the UASB sludge. The red box indicates MPs that are predicted to be better removed in the UASB, while the MPs in the black box are predicted to be better removed in the CAS according to our respective metrics for evaluating reactor performance. | |
Batch experiments – biotransformation products
Our final objective was to screen the high-resolution mass spectral acquisitions from the batch experiments for evidence of biotransformation product formation. This evidence will allow us to infer whether there were differential metabolic functions performed between the two types of wastewater microbial communities. To do this, we used the Eawag-PPS to predict biotransformation products for 14 of the 24 parent MPs (2,4-D, acetaminophen, adrenosterone, amphetamine, benzoylecgonine, bupropion, caffeine, diphenhydramine, ethyl-butylacetylaminopropionate, gabapentin, N4-acetylsulfamethoxazole, theophylline, serotonin, and sotalol). The selected 14 MPs were observed to have a pseudo-first order rate constant higher than 0.01 h−1 in the CAS batch test, and a pseudo-first order rate constant higher than 0.005 h−1 in the UASB batch test. We reasoned that only those MPs with a measurable rate constant would generate TPs that we could actually measure. We predicted TPs with relative reasoning turned off and allowed the Eawag-PPS to predict both aerobic and anaerobic biotransformations. This resulted in a list of 104 predicted first-generation biotransformation products for the 14 parent MPs. We used the exact masses of the [M + H]+ and [M − H]− parent ions for each of the predicted TPs to screen our high-resolution mass spectral acquisitions. More details on our approach to biotransformation product screening is provided elsewhere.42,50
We identified eight TPs for six of the 14 parent MPs in at least one of the batch experiments. All TPs were identified with a confidence of level 3.65 A summary of the TP structures is provided in Fig. 3 and 4 and additional details are provided in Table S7.† It must be noted that no evidence of predicted biotransformation products was observed for eight of the 14 parent MPs. These included 2,4-D, acetaminophen, benzoylecgonine, bupropion, gabapentin, theophylline, serotonin, sotalol. We contend that biotransformation products for these TPs were either not accurately predicted or subsequently biotransformed into other products before they could be measured.66Fig. 3a–c shows TPs for diphenhydramine, caffeine, and amphetamine. TPs for these MPs were measured exclusively in the experiments seeded with wastewater microbial communities harvested from the CAS system. The observed biotransformations include monohydroxylations (diphenhydramine and amphetamine), ether dealkylation (diphenhydramine), and amine dealkylation (caffeine). These are all oxidations that have previously been reported in aerobic environments.67 However, to the best of our knowledge, this is the first report of transformation products for diphenhydramine (monohydroxylation and ether dealkylation) in a CAS system. Fig. 3d and e shows biotransformation pathways for N-acetylsulfamethoxazole and ethyl-butylacetylaminopropionate. TPs for these MPs were measured in the experiments seeded with both wastewater microbial communities harvested from the CAS or UASB system. The observed biotransformations are ester hydrolysis (ethyl-butylacetylamino-propionate) and amide hydrolyses (acetylsulfamethoxazole). These biotransformations are not expected to be redox dependent which can explain why we see these types of biotransformations in experiments seeded with wastewater microbial communities harvested from CAS and UASB systems. Both of these biotransformations have been previously reported in other types of biological wastewater processes, further highlighting the ubiquity at which these biotransformations may be performed.68Fig. 4 shows the biotransformation pathways for adrenosterone which exhibited different TPs in CAS and UASB experiments. In the CAS experiment we observe the formation of a monohydroxylation product which is an oxidative biotransformation one would expect in an aerobic environment, but to the best of our knowledge has not been previously reported.69 In the UASB we observe a hydrogenation reaction which has not been previously reported but could be expected in an anaerobic environment.70 This latter observation is of particular interest because it demonstrates rapid yet differential biotransformation of a MP in CAS and UASB processes. Together, data from our batch experiments demonstrate that MPs can be biotransformed with relevant rates in batch experiments and the observed biotransformation products align with the expected metabolisms of the respective wastewater microbial communities. Previous research has demonstrated differential extents of micropollutant biotransformation in wastewater treatment exhibiting variable redox zones (e.g., biological nutrient removal systems),71–73 a phenomenon often linked to more functionally diverse microbial communities.64,71 Our study provides further context to previous work by demonstrating differential biotransformation resulting in the formation of biotransformation products that align with the expected metabolisms of the respective wastewater microbial communities. Finally, we returned to our UASB_eff and CAS_eff samples to screen for the TPs that formed in our batch experiments. We found evidence of the ether dealkylation product of diphenhydramine and the amide hydrolysis product of N-acetylsulfamethoxazole in UASB_eff and CAS_eff samples and the ester hydrolysis product of ethyl-butylacetylamino-propionate in CAS_eff samples. These observations agree only in part with our data from the batch experiments and the interpretation linked to redox conditions. This may be due to a variety of analytical factors (e.g., concentrations in full- or pilot-scale system too low to observe TP) or biological factors (e.g., insufficient time to completely biotransform a MP). Nevertheless, taken together, our data demonstrate the differential biotransformation of MPs in the two processes.
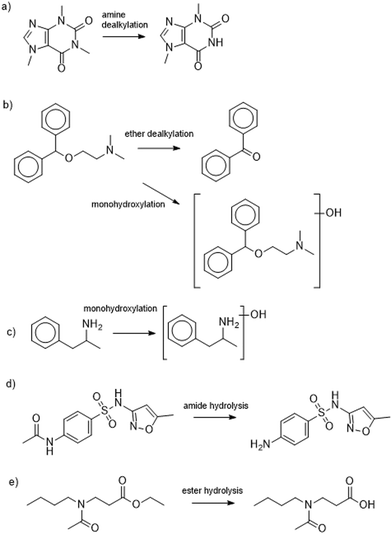 |
| Fig. 3 Biotransformation pathways observed in CAS: (a) caffeine, (b) diphenhydramine, (c) amphetamine. Biotransformation pathways observed in both UASB & CAS: (d) N-acetylsulfamethoxazole and (e) ethyl-butylacetylamino-propionate. | |
 |
| Fig. 4 Differential biotransformation pathways observed in UASB and CAS for adrenosterone. | |
Conclusion
This study aimed to investigate the differential biotransformations of micropollutants in conventional activated sludge (CAS) and up-flow anaerobic sludge blanket (UASB) processes. Our study is timely because alternative biological processes are being developed for wastewater treatment applications focused on resource recovery and solid waste elimination. Overall, our data provide novel insights on the relative functioning of two wastewater microbial communities operated under different redox conditions. Our study demonstrates the potential of the UASB reactor and reports on four TPs that have not been previously reported in the literature. These findings support the continued development of UASB reactors and other alternative biological processes for wastewater treatment around the world.
Conflicts of interest
There are no conflicts to declare.
Acknowledgements
We thank the team of operators at the Ithaca Area Wastewater Treatment Facility, especially Ed Gottlieb and Roxanne Roberts for assisting us in the sampling process and measuring physical and chemical properties of the collected samples. This work was supported by the United States National Science Foundation, United States (CBET-1748982). We acknowledge Guangyu Li for his code contribution in Fig. 1, where his custom Python library, ‘mpllayout’, was used (https://github.com/lguangyu/MatplotlibLayout).
References
- S. D. Richardson and T. A. Ternes, Water Analysis: Emerging Contaminants and Current Issues, Anal. Chem., 2022, 94, 382–416 CrossRef CAS PubMed.
- R. P. Schwarzenbach, B. I. Escher, K. Fenner, T. B. Hofstetter, C. A. Johnson, U. von Gunten, B. Wehrli, U. Von Gunten and B. Wehrli, The challenge of micropollutants in aquatic systems, Science, 2006, 313, 1072–1077 CrossRef CAS PubMed.
- A. S. Oberoi, Y. Jia, H. Zhang, S. K. Khanal and H. Lu, Insights into the Fate and Removal of Antibiotics in Engineered Biological Treatment Systems: A Critical Review, Environ. Sci. Technol., 2019, 53, 7234–7264 CrossRef CAS PubMed.
- P. Gago-Ferrero, A. A. Bletsou, D. E. Damalas, R. Aalizadeh, N. A. Alygizakis, H. P. Singer, J. Hollender and N. S. Thomaidis, Wide-scope target screening of >2000 emerging contaminants in wastewater samples with UPLC-Q-ToF-HRMS/MS and smart evaluation of its performance through the validation of 195 selected representative analytes, J. Hazard. Mater., 2020, 387, 121712 CrossRef CAS PubMed.
- L. B. Stadler, A. S. Ernstoff, D. S. Aga and N. G. Love, Micropollutant Fate in Wastewater Treatment: Redefining ‘Removal’, Environ. Sci. Technol., 2012, 46, 10485–10486 CrossRef CAS PubMed.
- A. Sherman, W. A. G. Mejia, N. R. Padilla, C. M. G. Carpenter, S. L. Rich, E. T. Moncada and D. E. Helbling, Target and suspect screening for biocides in drinking water resources of Honduras, H2Open J., 2022, 5, 84–97 CrossRef.
- W. Q. Fan, T. Yanase, H. Morinaga, S. Gondo, T. Okabe, M. Nomura, T. Komatsu, K. I. Morohashi, T. B. Hayes, R. Takayanagi and H. Nawata, Atrazine-induced aromatase expression is SF-1 dependent: Implications for endocrine disruption in wildlife and reproductive cancers in humans, Environ. Health Perspect., 2007, 115, 720–727 CrossRef PubMed.
- F. J. Burdon, Y. Bai, M. Reyes, M. Tamminen, P. Staudacher, S. Mangold, H. Singer, K. Räsänen, A. Joss, S. D. Tiegs, J. Jokela, R. I. L. Eggen and C. Stamm, Stream microbial communities and ecosystem functioning show complex responses to multiple stressors in wastewater, Global Change Biol., 2020, 26, 6363–6382 CrossRef PubMed.
- N. A. Zhou and H. L. Gough, Enhanced Biological Trace Organic Contaminant Removal: A Lab-Scale Demonstration with Bisphenol A-Degrading Bacteria Sphingobium sp. BiD32, Environ. Sci. Technol., 2016, 50, 8057–8066 CrossRef CAS PubMed.
- M. Oosterhuis, D. Ringoot, A. Hendriks and P. Roeleveld, Thermal hydrolysis of waste activated sludge at Hengelo Wastewater Treatment Plant, the Netherlands, Water Sci. Technol., 2014, 70, 1–7 CrossRef CAS PubMed.
- D. C. Vuono, J. Benecke, J. Henkel, W. C. Navidi, T. Y. Cath, J. Munakata-Marr, J. R. Spear and J. E. Drewes, Disturbance and temporal partitioning of the activated sludge metacommunity, ISME J., 2015, 9, 425–435 CrossRef CAS PubMed.
- D. Krah, A. Ghattas, A. Wick, K. Bröder and T. Ternes, Micropollutant degradation via extracted native enzymes from activated sludge, Water Res., 2016, 95, 348–360 CrossRef CAS PubMed.
- M. K. H. Winkler, R. Kleerebezem, L. M. M. De Bruin, P. J. T. Verheijen, B. Abbas, J. Habermacher and M. C. M. Van Loosdrecht, Microbial diversity differences within aerobic granular sludge and activated sludge flocs, Appl. Microbiol. Biotechnol., 2013, 97, 7447–7458 CrossRef CAS PubMed.
- K. Fischer and M. Majewsky, Cometabolic degradation of organic wastewater micropollutants by activated sludge and sludge-inherent microorganisms, Appl. Microbiol. Biotechnol., 2014, 98, 6583–6597 CrossRef CAS PubMed.
- N. A. Zhou, A. C. Lutovsky, G.
L. Andaker, H. L. Gough and J. F. Ferguson, Cultivation and characterization of bacterial isolates capable of degrading pharmaceutical and personal care products for improved removal in activated sludge wastewater treatment, Biodegradation, 2013, 24, 813–827 CrossRef CAS PubMed.
- Y. Men, S. Achermann, D. E. Helbling, D. R. Johnson and K. Fenner, Relative contribution of ammonia oxidizing bacteria and other members of nitrifying activated sludge communities to micropollutant biotransformation, Water Res., 2017, 109, 217–226 CrossRef CAS PubMed.
- M. Hörsing, A. Ledin, R. Grabic, J. Fick, M. Tysklind, J. la C. Jansen and H. R. Andersen, Determination of sorption of seventy-five pharmaceuticals in sewage sludge, Water Res., 2011, 45, 4470–4482 CrossRef PubMed.
- Z. Ying and R. L. Droste, Sorption of microconstituents onto primary sludge, Water Sci. Technol., 2015, 72, 779–784 CrossRef CAS PubMed.
- J. Stevens-Garmon, J. E. Drewes, S. J. Khan, J. A. McDonald and E. R. V. Dickenson, Sorption of emerging trace organic compounds onto wastewater sludge solids, Water Res., 2011, 45, 3417–3426 CrossRef CAS PubMed.
- M. Nsenga Kumwimba and F. Meng, Roles of ammonia-oxidizing bacteria in improving metabolism and cometabolism of trace organic chemicals in biological wastewater treatment processes: A review, Sci. Total Environ., 2019, 659, 419–441 CrossRef CAS PubMed.
- N. H. Tran, T. Urase, H. H. Ngo, J. Hu and S. L. Ong, Insight into metabolic and cometabolic activities of autotrophic and heterotrophic microorganisms in the biodegradation of emerging trace organic contaminants, Bioresour. Technol., 2013, 146, 721–731 CrossRef CAS PubMed.
- D. M. Kennes-Veiga, B. Vogler, K. Fenner, M. Carballa and J. M. Lema, Heterotrophic enzymatic biotransformations of organic micropollutants in activated sludge, Sci. Total Environ., 2021, 780, 146564 CrossRef CAS PubMed.
- T. A. Ternes, N. Herrmann, M. Bonerz, T. Knacker, H. Siegrist and A. Joss, A rapid method to measure the solid-water distribution coefficient (Kd) for pharmaceuticals and musk fragrances in sewage sludge, Water Res., 2004, 38, 4075–4084 CrossRef CAS PubMed.
- T. Alvarino, S. Suarez, J. Lema and F. Omil, Understanding the sorption and biotransformation of organic micropollutants in innovative biological wastewater treatment technologies, Sci. Total Environ., 2018, 615, 297–306 CrossRef CAS PubMed.
- S.-W. Nam, D.-J. Choi, S.-K. Kim, N. Her and K.-D. Zoh, Adsorption characteristics of selected hydrophilic and hydrophobic micropollutants in water using activated carbon, J. Hazard. Mater., 2014, 270, 144–152 CrossRef CAS PubMed.
- R. Gulde, U. Meier, E. L. Schymanski, H. E. Kohler, D. E. Helbling, S. Derrer, D. Rentsch and K. Fenner, Systematic Exploration of Biotransformation Reactions of Amine- Containing Micropollutants in Activated Sludge, Environ. Sci. Technol., 2016, 50, 2908–2920 CrossRef CAS PubMed.
- Y. Men, P. Han, D. E. Helbling, N. Jehmlich, C. Herbold, R. Gulde, A. Onnis-hayden, A. Z. Gu, D. R. Johnson, M. Wagner and K. Fenner, Biotransformation of Two Pharmaceuticals by the Ammonia- Oxidizing Archaeon Nitrososphaera gargensis, Environ. Sci. Technol., 2016, 50, 4682–4692 CrossRef CAS PubMed.
- K. M. Onesios, J. T. Yu and E. J. Bouwer, Biodegradation and removal of pharmaceuticals and personal care products in treatment systems: a review, Biodegradation, 2009, 20, 441–466 CrossRef CAS PubMed.
- M. K. H. Winkler, C. Meunier, O. Henriet, J. Mahillon, M. E. Suárez-Ojeda, G. Del Moro, M. De Sanctis, C. Di Iaconi and D. G. Weissbrodt, An integrative review of granular sludge for the biological removal of nutrients and recalcitrant organic matter from wastewater, Chem. Eng. J., 2018, 336, 489–502 CrossRef CAS.
- L. Wu, D. Ning, B. Zhang, Y. Li, P. Zhang, X. Shan, Q. Zhang, M. Brown, Z. Li, J. D. Van Nostrand, F. Ling, N. Xiao, Y. Zhang, J. Vierheilig, G. F. Wells, Y. Yang, Y. Deng, Q. Tu, A. Wang, T. Zhang, Z. He, J. Keller, P. H. Nielsen, P. J. J. Alvarez, C. S. Criddle, M. Wagner, J. M. Tiedje, Q. He, T. P. Curtis, D. A. Stahl, L. Alvarez-Cohen, B. E. Rittmann, X. Wen and J. Zhou, Global diversity and biogeography of bacterial communities in wastewater treatment plants, Nat. Microbiol., 2019, 4, 1183–1195 CrossRef CAS PubMed.
- L. F. Stadlmair, T. Letzel, J. E. Drewes and J. Grassmann, Enzymes in removal of pharmaceuticals from wastewater: A critical review of challenges, applications and screening methods for their selection, Chemosphere, 2018, 205, 649–661 CrossRef CAS PubMed.
- M. Pronk, A. Giesen, A. Thompson, S. Robertson and M. Van Loosdrecht, Aerobic granular biomass technology: Advancements in design, applications and further developments, Water Pract. Technol., 2017, 12, 987–996 CrossRef.
- W. W. Li and H. Q. Yu, Anaerobic granule technologies for hydrogen recovery from wastes: The way forward, Crit. Rev. Environ. Sci. Technol., 2013, 43, 1246–1280 CrossRef CAS.
- S. J. Lim and T. H. Kim, Applicability and trends of anaerobic granular sludge treatment processes, Biomass Bioenergy, 2014, 60, 189–202 CrossRef CAS.
- C. Burzio, J. Ekholm, O. Modin, P. Falås, O. Svahn, F. Persson, T. van Erp, D. J. I. Gustavsson and B. M. Wilén, Removal of organic micropollutants from municipal wastewater by aerobic granular sludge and conventional activated sludge, J. Hazard. Mater., 2022, 438, 129528 CrossRef CAS PubMed.
- T. Abbasi and S. A. Abbasi, Formation and impact of granules in fostering clean energy production and wastewater treatment in upflow anaerobic sludge blanket (UASB) reactors, Renewable Sustainable Energy Rev., 2012, 16, 1696–1708 CrossRef CAS.
- R. Subramanyam, Physicochemical and morphological characteristics of granular sludge in upflow anaerobic sludge blanket reactors, Environ. Eng. Sci., 2013, 30, 201–212 CrossRef CAS.
- M. X. Zheng, K. J. Wang, J. E. Zuo, Z. Yan, H. Fang and J. W. Yu, Flow pattern analysis of a full-scale expanded granular sludge bed-type reactor under different organic loading rates, Bioresour. Technol., 2012, 107, 33–40 CrossRef CAS PubMed.
- J. Margot, S. Lochmatter, D. A. Barry and C. Holliger, Role of ammonia-oxidizing bacteria in micropollutant removal from wastewater with aerobic granular sludge, Water Sci. Technol., 2016, 73, 564–575 CrossRef CAS PubMed.
- S. J. Lim and P. Fox, Evaluation of a static granular bed reactor using a chemical oxygen demand balance and mathematical modeling, Bioresour. Technol., 2011, 102, 6399–6404 CrossRef CAS PubMed.
- C. V. Faria, B. C. Ricci, A. F. R. Silva, M. C. S. Amaral and F. V. Fonseca, Removal of micropollutants in domestic wastewater by expanded granular sludge bed membrane bioreactor, Process Saf. Environ. Prot., 2020, 136, 223–233 CrossRef CAS.
- S. L. Rich and D. E. Helbling, Daily Monitoring at a Full-Scale Wastewater Treatment Plant Reveals Temporally Variable Micropollutant Biotransformations, Environ. Sci. Technol., 2023, 57, 10404–10414 CrossRef CAS PubMed.
- Plant Schematic | Ithaca, NY - Official Website, https://www.cityofithaca.org/687/Plant-Schematic, (accessed 8 October 2023).
- C. M. G. Carpenter and D. E. Helbling, Widespread Micropollutant Monitoring in the Hudson River Estuary Reveals Spatiotemporal Micropollutant Clusters and Their Sources, Environ. Sci. Technol., 2018, 52, 6187–6196 CrossRef CAS PubMed.
- A. L. Pochodylo and D. E. Helbling, Emerging Investigators Series: Prioritization of suspect hits in a sensitive suspect screening workflow for comprehensive micropollutant characterization in environmental samples, Environ. Sci.: Water Res. Technol., 2017, 3, 54–65 RSC.
- M. T. Zumstein and D. E. Helbling, Biotransformation of antibiotics: exploring the activity of extracellular and intracellular enzymes derived from wastewater microbial communities, Water Res., 2019, 155, 115–123 CrossRef CAS PubMed.
- D. E. Helbling, J. Hollender, H.-P. E. Kohler, H. Singer and K. Fenner, High-throughput identification of microbial transformation products of organic micropollutants, Environ. Sci. Technol., 2010, 44, 6621–6627 CrossRef CAS PubMed.
- D. E. Helbling, J. Hollender, H.-P. E. Kohler and K. Fenner, Structure-based interpretation of biotransformation pathways of amide-containing compounds in sludge-seeded bioreactors, Environ. Sci. Technol., 2010, 44, 6628–6635 CrossRef CAS PubMed.
- D. E. Helbling, D. R. Johnson, M. Honti and K. Fenner, Micropollutant biotransformation kinetics associate with WWTP process parameters and microbial community characteristics, Environ. Sci. Technol., 2012, 46, 10579–10588 CrossRef CAS PubMed.
- S. Rich, M. T. Zumstein and D. E. Helbling, Identifying functional groups that determine rates of micropollutant biotransformations performed by wastewater microbial communities, Environ. Sci. Technol., 2022, 56, 984–994 CrossRef CAS PubMed.
- J. Gao, L. B. M. Ellis and L. P. Wackett, The University of Minnesota Biocatalysis/Biodegradation Database: improving public access, Nucleic Acids Res., 2010, 38, D488–D491 CrossRef CAS PubMed.
- D. E. Helbling, D. R. Johnson, T. K. Lee, A. Scheidegger and K. Fenner, A framework for establishing predictive relationships between specific bacterial 16S rRNA sequence abundances and biotransformation rates, Water Res., 2015, 70, 471–484 CrossRef CAS PubMed.
- K. G. Aukema, D. E. Escalante, M. M. Maltby, A. K. Bera, A. Aksan and L. P. Wackett, In silico identification of bioremediation potential: Carbamazepine and other recalcitrant personal care products, Environ. Sci. Technol., 2017, 51, 880–888 CrossRef CAS PubMed.
- E. Kaiser, C. Prasse, M. Wagner, K. Broeder and T. A. Ternes, Transformation of Oxcarbazepine and Human Metabolites of Carbamazepine and Oxcarbazepine in Wastewater Treatment and Sand Filters, Environ. Sci. Technol., 2014, 48, 10208–10216 CrossRef CAS PubMed.
- M. Carballa, F. Omil, J. Lema, M. Llompart, C. García-Jares, I. Rodríguez, M. Gómez and T. Ternes, Behavior of pharmaceuticals, cosmetics and hormones in a sewage treatment plant, Water Res., 2004, 38, 2918–2926 CrossRef CAS PubMed.
- A. Jelic, M. Gros, A. Ginebreda, R. Cespedes-Sánchez, F. Ventura, M. Petrovic and D. Barcelo, Occurrence, partition and removal of pharmaceuticals in sewage water and sludge during wastewater treatment, Water Res., 2011, 45, 1165–1176 CrossRef CAS PubMed.
- P. Paíga, M. Correia, M. J. Fernandes, A. Silva, M. Carvalho, J. Vieira, S. Jorge, J. G. Silva, C. Freire and C. Delerue-Matos, Assessment of 83 pharmaceuticals in WWTP influent and effluent samples by UHPLC-MS/MS: Hourly variation, Sci. Total Environ., 2019, 648, 582–600 CrossRef PubMed.
- F. Polesel, H. R. Andersen, S. Trapp and B. G. Plósz, Removal of Antibiotics in Biological Wastewater Treatment Systems - A Critical Assessment Using the Activated Sludge Modeling Framework for Xenobiotics (ASM-X), Environ. Sci. Technol., 2016, 50, 10316–10334 CrossRef CAS PubMed.
- D. E. Helbling, M. Ackermann, K. Fenner, H.-P. E. Kohler and D. R. Johnson, The activity level of a microbial community function can be predicted from its metatranscriptome, ISME J., 2012, 6, 902–904 CrossRef CAS PubMed.
- L. Gonzalez-Gil, D. Krah, A. K. Ghattas, M. Carballa, A. Wick, L. Helmholz, J. M. Lema and T. A. Ternes, Biotransformation of organic micropollutants by anaerobic sludge enzymes, Water Res., 2019, 152, 202–214 CrossRef CAS PubMed.
- J. S. LeClair and L. M. Wahl, The Impact of Population Bottlenecks on Microbial Adaptation, J. Stat. Phys., 2018, 172, 114–125 CrossRef.
- I. López and L. Borzacconi, UASB reactor hydrodynamics: Residence time distribution and proposed modelling tools, Environ. Technol., 2010, 31, 591–600 CrossRef PubMed.
- D. R. Johnson, D. E. Helbling, T. K. Lee, J. Park, K. Fenner, H.-P. E. Kohler and M. Ackermann, Association of biodiversity with the rates of micropollutant biotransformations among full-scale wastewater treatment plant communities, Appl. Environ. Microbiol., 2015, 81, 666–675 CrossRef PubMed.
- D. R. Johnson, T. K. Lee, J. Park, K. Fenner and D. E. Helbling, The functional and taxonomic richness of wastewater treatment plant microbial communities are associated with each other and with ambient nitrogen and carbon availability, Environ. Microbiol., 2015, 17, 4851–4860 CrossRef CAS PubMed.
- E. L. Schymanski, J. Jeon, R. Gulde, K. Fenner, M. Ru, H. P. Singer, J. Hollender, M. Ruff, H. P. Singer and J. Hollender, Identifying Small Molecules via High Resolution Mass Spectrometry: Communicating Confidence, Environ. Sci. Technol., 2014, 48, 2097–2098 CrossRef CAS PubMed.
- H. Cui, H. Chang, H. Zheng and Y. Wan, Determination and occurrence of sulfonamide transformation products in surface waters, Sci. Total Environ., 2021, 779, 146562 CrossRef CAS PubMed.
- X. Ma, H. Chen, R. Chen and X. Hu, Direct and Activated Chlorine Dioxide Oxidation for Micropollutant Abatement: A Review on Kinetics, Reactive Sites, and Degradation Pathway, Water, 2022, 14(13), 2028 CrossRef CAS.
- S. Achermann, P. Falås, A. A. Joss, C. B. Mansfeldt, Y. Men, B. Vogler, K. Fenner, P. Falas, A. A. Joss, C. B. Mansfeldt, Y. Men, B. Vogler and K. Fenner, Trends in Micropollutant Biotransformation along a Solids Retention Time Gradient, Environ. Sci. Technol., 2018, 52, 11601–11611 CrossRef CAS PubMed.
- Y. H. Kim, C. J. Cha, K. H. Engesser and S. J. Kim, Degradation of various alkyl ethers by alkyl ether-degrading Actinobacteria isolated from activated sludge of a mixed wastewater treatment, Chemosphere, 2008, 73, 1442–1447 CrossRef CAS PubMed.
- R. M. Wilson, M. M. Tfaily, V. I. Rich, J. K. Keller, S. D. Bridgham, C. M. Zalman, L. Meredith, P. J. Hanson, M. Hines, L. Pfeifer-Meister, S. R. Saleska, P. Crill, W. T. Cooper, J. P. Chanton and J. E. Kostka, Hydrogenation of organic matter as a terminal electron sink sustains high CO2:CH4 production ratios during anaerobic decomposition, Org. Geochem., 2017, 112, 22–32 CrossRef CAS.
- P. Falås, A. Wick, S. Castronovo, J. Habermacher, T. A. Ternes and A. Joss, Tracing the limits of organic micropollutant removal in biological wastewater treatment, Water Res., 2016, 95, 240–249 CrossRef PubMed.
- E. Edefell, P. Falås, E. Torresi, M. Hagman, M. Cimbritz, K. Bester and M. Christensson, Promoting the degradation of organic micropollutants in tertiary moving bed biofilm reactors by controlling growth and redox conditions, J. Hazard. Mater., 2021, 414, 125535 CrossRef CAS PubMed.
- N. Lakshminarasimman, O. Quiñones, B. J. Vanderford, P. Campo-Moreno, E. V. Dickenson and D. C. McAvoy, Biotransformation and sorption of trace organic compounds in biological nutrient removal treatment systems, Sci. Total Environ., 2018, 640–641, 62–72 CrossRef CAS PubMed.
|
This journal is © The Royal Society of Chemistry 2024 |
Click here to see how this site uses Cookies. View our privacy policy here.