Understanding the importance of atmospheric transformation in assessing the hazards of liquid crystal monomers†
Received
28th September 2023
, Accepted 25th November 2023
First published on 30th November 2023
Abstract
Liquid crystal monomers (LCMs), a group of synthetic chemicals released from liquid crystal devices such as televisions and smartphones, have recently been recognized as emerging contaminants due to their widespread occurrence in the environment and potential negative impacts on human health. Airborne LCMs can undergo atmospheric oxidation reactions to form various transformation products. Despite the certainty of atmospheric transformation chemistry, the knowledge about the hazard properties of transformation products remains largely unknown. Here, we perform an in silico model-based evaluation of the persistence, bioaccumulation potential, mobility, and toxicity of two representative LCMs, namely, 1-ethyl-4-(4-(4-propylcyclohexyl)phenyl)benzene and 4′′-ethyl-2′-fluoro-4-propyl-1,1′:4′,1′′-terphenyl, and their transformation products. We found that, among the investigated transformation products, 38% have overall persistence greater than the minimum of 331 days among the persistent organic pollutants regulated by the Stockholm Convention, 62% meet the bioaccumulation threshold of 1000 L kg−1 used by the United States Environmental Protection Agency, 44% are classified “mobile” according to the criterion used by the German Environmental Agency, and 58% have the potential to induce unacceptable toxic effects in aquatic organisms. Furthermore, we identified several transformation products with increased persistence, bioaccumulation potential, and mobility compared to their parent compounds. These findings not only offer insights for prioritizing LCM transformation products for future risk assessment, but also underscore the significance of considering atmospheric transformation in the evaluation of environmental risks posed by emerging contaminants, including LCMs.
Environmental significance
Liquid crystal monomers (LCMs) are a class of emerging contaminants that are widely distributed in the environment. However, little is known about the hazard properties of their atmospheric transformation products. To address this knowledge gap, here, we evaluate the persistence, bioaccumulation potential, mobility, and toxicity of two representative LCMs and their atmospheric transformation products with in silico modeling. We find that the hazard properties of LCMs can be substantially changed by atmospheric oxidation process. Several transformation products possess higher persistence, bioaccumulation potential, and mobility than the parent LCMs. These results indicate that the assessment of environmental hazards associated with LCMs may be biased if atmospheric transformation processes are not considered.
|
Introduction
Liquid crystal monomers (LCMs) are synthetic chemicals widely used in liquid crystal display (LCD) devices such as laptops, smartphones, televisions, and computers.1,2 With the rapid development of the global LCD industry, the production volume of LCMs significantly increased over the past few years.3 The global production of LCD panels amounted to 198 million m2 in 2018 and was predicted to increase in the forthcoming years.3 During the manufacturing of LCDs, LCMs are physically filled into LCD screens and are not chemically bonded to base materials. As a result, LCMs can be constantly released into the environment during their production, utilization, and recycling, which leads to their ubiquitous presence in various environmental media such as indoor dust, municipal landfill sites, air, and marine sediments.4–9
Recent evidence suggests that LCMs can pose adverse impacts on human health. LCMs are demonstrated to be peroxisome proliferator-activated receptor gamma (PPARγ, a ligand-activated transcription factor of nuclear hormone receptor) antagonists, potentially inducing toxicological effects in humans.10 Given the widespread occurrence of LCMs in the environment and the recently demonstrated toxicity, LCMs have attracted increasing environmental and health concerns.7,11,12 It should be noted that previous studies mainly focus on the adverse impacts associated with LCMs, while limited consideration has been given to their atmospheric transformation products that may arise from their release.
Once emitted into the air, the physical and chemical properties of LCMs are likely to be changed. Our previous study indicates that LCMs will undergo complex transformation chemistry upon atmospheric hydroxyl radical (OH) oxidation, resulting in the formation of many structurally diverse transformation products.13 Thus, a complete hazard assessment of LCMs should consider not only LCMs themselves but also their atmospheric transformation products. This includes knowledge of the resistance of biotic and abiotic dissipation (persistence or P), the potential for accumulation and enrichment in biota (bioaccumulation potential or B), the tendency of permeation through natural barriers to contaminate surface and subsurface water systems (mobility or M),14 and the adverse impacts on biota (toxicity or T) of LCMs and their transformation products. However, there is currently limited understanding of the hazard properties of transformation products. It remains an open question whether the environmental hazards of LCMs will increase or decrease upon their introduction to the atmosphere.
To address this knowledge gap, here we illustrate the evaluation of the hazard properties of 66 transformation products resulting from the atmospheric oxidation of two typical LCMs, i.e., 1-ethyl-4-(4-(4-propylcyclohexyl)phenyl)benzene (EPPB; CAS registry number 84540-37-4) and 4′′-ethyl-2′-fluoro-4-propyl-1,1′:4′,1′′-terphenyl (EFPT; CAS registry number 95759-44-7). Our recent search indicates that chemical property data and environmental monitoring data are currently lacking for these two chemicals and their newly identified transformation products. In brief, the structural information of these transformation products serves as input to quantitative structure–activity and –property relationships (QSARs and QSPRs) to compute their phase partitioning and reactivity properties. Supplied with these property data, a comprehensive fate and exposure model named PROduction-to-EXposure (PROTEX) was operated to predict the P, B, M, and T indices.15,16 Our analysis aims to highlight the importance of atmospheric transformation in assessing the hazards of LCMs, which can inform future risk evaluation for these emerging contaminants.
Materials and methods
Transformation products of LCMs
As illustrated in our previous work, 66 transformation products were identified through an experimental study exploring the OH oxidation of two LCMs (EPPB and EFPT).13 Briefly, particulate LCMs were introduced into an oxidation flow reactor where they were exposed to OH radicals. The formed transformation products were identified with an advanced online mass spectrometry which can provide molecular information for organics. In general, these transformation products (see Table S1†) were formed from OH reactions with the alkyl group or the phenyl ring of LCMs.
In silico modeling
We assess the hazards P, B, and M using the PROTEX model.15,17 parameterized with chemical property data predicted by QSARs and QSPRs. We assess the hazard T using two QSAR toolkits, i.e., the Toxicity Estimation Software Tool (TEST) and VEGA-QSAR. These hazard indices are selected because they are usually used in assessing the potential adverse impacts on the environment and human health in regulatory frameworks such as Registration, Evaluation, Authorisation and Restriction of Chemicals (REACH). The use of hazard indices is advantageous in the assessment of “data-poor” chemicals with limited environmental monitoring data, as they are independent of the magnitudes of chemical emissions and concentrations in the environment.
PROTEX modeling
Supplied with information on chemical emission, chemical properties (partitioning and reactivity properties), environmental conditions (meteorological, hydrological, and land-use data), and organism data (growth, body composition, physiological and behavioral information), PROTEX simulates the transport, transformation, and accumulation of chemicals across multiple environmental compartments and aquatic and terrestrial organisms.15,17 As a modular tool, PROTEX's “environmental fate and transport” module simulates the fate of chemicals across various scales of a regional environment, including the rural areas, which encompass different compartments such as air and various land compartments (i.e., soil, vegetation, freshwater and freshwater sediment, estuarine water and estuarine sediment). A chemical can be released into one or more compartments at a given rate; a unit emission rate is assumed in this hazard assessment because the hazard properties are independent of the emission rate. The module then simulates its advective and diffusive transport across multiple environmental compartments, and its reactive loss from these environmental compartments. In this work, we assume the two LCMs and their atmospheric transformation products are released into the air compartment, given that (i) LCMs are primarily released from indoor sources through passive volatilization and have the potential to be ventilated into the outdoor air, and (ii) the transformation products are formed through atmospheric processes. PROTEX quantifies the rates of advection, diffusion, and reaction, as well as chemical concentrations in environmental compartments resulting from these processes. Furthermore, PROTEX's “food-web bioaccumulation” module simulates chemical concentrations in aquatic and terrestrial organisms, such as planktivorous and piscivorous fish, by quantifying the rates that these organisms bioaccumulate chemicals from the ambient environment, metabolize chemicals in their bodies, and excrete chemicals through various physiological processes.
PROTEX has several advantageous merits in hazard and risk assessments. First, PROTEX's performance has been well evaluated and validated in a series of earlier studies,15–20 where it succeeded in reproducing contamination of a diverse array of contaminants, ranging from mainly hydrophobic to hydrophilic and from recalcitrant to liable chemicals, that had been observed in environmental monitoring efforts. Second, PROTEX builds on mathematical descriptions of physical, chemical, biological, and toxicokinetic processes governing chemical fate and exposure. Such a mechanistic nature allows flexible parameterization of PROTEX for a wide range of chemicals, regions, and organisms of interest. In this illustrative assessment, we use the regional parameterization representative of the environment and biota of the Canadian side of the Lake Ontario basin because it was thoroughly evaluated in our earlier publication.16 An earlier study demonstrates that, compared to chemicals' physicochemical properties, the region-to-region difference in environmental conditions poses minor impacts on the modeled chemical fate.21 Therefore, our assessed results can be generalized to regions (e.g., polar, temperate, and subtropical climate zones) other than the modeled one.
We parameterize PROTEX with property data of the investigated chemicals, including partition coefficients of the neutral forms in the octanol-water-air system (KOW and KOA) at 25 °C, dissociation constants (pKa and pKb; for ionizable chemicals only), the rate constants for reactions with the hydroxyl radicals in the gas-phase (kOH), aquatic biodegradation half-life (HLbiodeg), and fish biotransformation half-life (HLbiotrans; see Dataset S1†). Since experimentally determined values are generally not available for these chemicals, we computed the chemical properties using four commonly applied QSPRs including the estimation programs interface (EPI) suite (for KOW, KOA, kOH, and HLbiodeg),22 iterative fragment selection-QSAR (IFS-QSAR) (for KOW, KOA, and HLbiotrans),23–25 OPEn structure–activity/property relationship app (OPERA) (for KOW, KOA, pKa and pKb, and kOH) and QSAR-INSubria (QSARINS) (for HLbiotrans),26–28 and used the “consensus values” of these predictions, following the recommended best practice of retrieving and evaluating chemical property data in chemical assessment.29 Following the recommended extrapolation factors by Fenner et al.,30 PROTEX applies a generic assumption that the biodegradation half-life in water is equal to HLbiodeg, that in soil is twice HLbiodeg, and the biodegradation half-life in sediment is 10 times HLbiodeg. In addition, we take a simplifying assumption of focusing only on the gas-phase atmospheric degradation and ignoring the heterogeneous degradation in airborne particles. Such a simplifying assumption is deemed to cause negligible impacts on the modeled persistence of the investigated chemicals, given our sensitivity analysis indicates that the modeled air concentrations of EPPB and EFPT are minimally sensitive to the atmospheric degradation rate constants, with respective sensitivity values of 0.06 and 0.09. This means that a change of approximately 3% in the atmospheric rate constant results in changes of only 0.18% for the airborne EPPB concentration and 0.27% for the airborne EFPT concentration. Here, the change of 3% reflects the consideration of heterogeneous OH reaction rate constants of 7.05 × 10−13 cm3 molecule−1 s−1 for EPPB and 4.67 × 10−13 cm3 molecule−1 s−1 for EFPT13 in addition to the already considered gas-phase OH reaction rate constants of 2.58 × 10−11 cm3 molecule−1 s−1 for EPPB and 1.40 × 10−11 cm3 molecule−1 s−1 for EFPT (see Dataset S1†).
Overall persistence
We quantify persistence using a chemical's overall persistence (POV; in hours),31–33 defined as a chemical's average residence time in PROTEX's multimedia rural environment. Here, the rural environment is used as it is consistent with the “natural” environment assumed in most current persistence assessment frameworks, e.g., the Organization for Economic Cooperation and Development (OECD) POV & LRTP screening tool. POV measures the total resistance to degradation across all environmental media including air and land compartments, as well as advective transport of chemicals from the source region. A long POV indicates a chemical's long-lasting impact on the ecosystem and lower reversibility of contamination if adverse effects are found. For each rural compartment, the residence time is calculated by dividing the steady-state mass and the rate of permanent removal from the compartment. The current version of PROTEX accounts for four main mechanisms of permanent removal for a chemical from rural areas, which include (1) its reaction with the hydroxyl radical in the gas phase of the air compartment, (2) biodegradation in land compartments, (3) sediment burial, and (4) advective transport of chemicals beyond the region.15,17
Bioaccumulation potential
The bioaccumulation potential is quantified using a chemical's bioaccumulation factor (BAF; L kg−1) of fish, defined as the ratio of a chemical's wet-weight-based concentration in fish tissue (gchemical/kgwet weight) to its total concentration in the water where the fish lives (gchemical/Lwater).34 A high BAF indicates a chemical's strong potential to be stored in fish and to pose a continuous threat to fish, seafood, and organisms that feed on fish such as birds, mammals and even humans. In this study, we calculate the BAF of a chemical using the modeled chemical concentration in the estuarine water compartment of PROTEX and the modeled chemical concentration in fish living in the estuary. Since the PROTEX-predicted BAFs of planktivorous and piscivorous fish are correlated with each other (correlation coefficient of 0.9 for the logarithms) and the choice between these two types of fish BAFs does not significantly impact the results, our subsequent analysis is based on the results for piscivorous fish.
Mobility
We assess the mobility of LCMs and their transformation products by calculating the minimum organic carbon normalized solid-water sorption coefficient (KOC) over the pH range of 4–9, according to the criterion proposed recently by the German Environmental Agency.35 A low minimum KOC means that a chemical exhibits a low potential for sorption by soil and sediment and hence a high potential for aquatic transport and contamination of the sources of drinking water if it is atmospherically deposited in aquatic environments such as lakes and rivers. PROTEX calculates the minimum KOC from a chemical's neutral form KOW and dissociation constants (pKa or pKb):36
Non-ionizable chemicals:
KOC = 100.679×logKOW+0.663 |
Acidic chemicals:
KOC = φ × 100.54×logKOW + 1.11 + (1 − φ) × 100.11×logKOW+1.54 |
Basic chemicals:
KOC = φ × 100.42×logKOW + 1.34 + (1 − φ) × 100.47×logKOW+1.95 |
where
φ denotes the fraction of neutral species (pH dependent), which is calculated based on dissociation constants and pH based on the Henderson–Hasselbalch equation. This approach mainly considers the sorption of chemicals to amorphous organic matter in soil or sediment and assumes a chemical's ionic species exhibit lower sorption onto soil or sediment solids.
Toxicity modeling
We assess both the acute toxicity (related to short-term chemical exposures) and genetic toxicity (related to long-term chemical exposures) of the LCMs and their transformation products.
Acute toxicity is assessed through the calculation of a 50% lethal concentration (LC50) for fathead minnow, a representative aquatic organism. The LC50 represents the concentration of a chemical in water that is statistically likely to cause half of the fathead minnow population to die after 96 hours of exposure. A lower LC50 value indicates a higher level of toxicity. We operate the TEST model with its four modules (i.e., hierarchical clustering, single model, group contribution, and nearest neighbor) and take the consensus values of the four predictions if available. In instances where the first three modules are unable to provide predictions (because a chemical's unique structural features prevent the modules from generating reasonable molecular descriptors), TEST does not calculate a consensus value since the model's “nearest neighbor” algorithm considers the result from such a read-across approach to be unreliable. The details of the four modules have been described in Martin et al.37
Genetic toxicity is assessed by whether the investigated chemicals are likely to cause cancer (“carcinogenicity”) or mutations in DNA (“mutagenicity”). A chemical is identified to be carcinogenic if it is possible to find a dose at which half of the rat population develops tumors through a lifetime of exposure to the chemical in in vivo studies with conditions similar to those described in the OECD test guideline (TG) for chemicals no. 451. Likewise, following OECD TG no. 471, a chemical is considered to be a potential mutagen if a chemical can induce mutations in the DNA of various strains of the bacterium Salmonella typhimurium, a model species found in animals such as rats.
In this work, the results for carcinogenicity and mutagenicity are presented as binary outcomes from VEGA-QSAR, categorized as either “yes” or “possibly not”. We include results from several modules of VEGA (the IRFMN/antares, CAESAR, and ISS modules for carcinogenicity, and the CAESAR and ISS modules for mutagenicity), given that each module adopts different training sets and classification approaches for prediction and may output inconsistent classification results. For example, the IRFMN/antares module (version 1.0.1) and the ISS module (version 1.0.3) classify a chemical as a carcinogen if it contains one or more structural features known to be associated with cancer development, referred to as “structural alerts”. These modules employ training sets that contain 127 and 54 structural alerts, respectively. In contrast, the CAESAR module (version 2.1.10) employs a counter propagation artificial neural network algorithm and classifies chemicals as carcinogens based on 12 structural, compositional, and topological variables, referred to as “descriptors”. The details of the algorithms and training sets of the VEGA modules have been described in the QSAR model reporting format (QMRF) documents, which are available at https://www.vegahub.eu/portfolio-item/vega-qsar-models-qrmf/.
Results and discussion
In the following sections, we first present an overview of the predicted physicochemical properties of EPPB and EFPT and their transformation products. We then compare the hazard properties of individual transformation products with those of their parent compounds, followed by an exploration of how the hazard properties of the transformation products evolve as photochemical oxidation continues along a representative reaction channel.
Distribution of predicted partitioning and reactivity properties
Before an in-depth analysis of the hazard indices of the two LCMs and their transformation products, we first present an overview of the distribution of their partitioning and reactivity properties (for details; see Dataset S1†). Overall, most of the investigated chemicals are moderately hydrophobic, with the predicted “consensus” KOW having a 25th percentile of 104.37, a median of 105.38, and a 75th percentile of 106.54. All the investigated chemicals fall within the applicability domain (AD) of at least one KOW QSPR used here. The investigated chemicals share low volatility, with the predicted “consensus” KOA having a 25th percentile of 1012.2, a median of 1013.2, and a 75th percentile of 1013.8. Among the investigated chemicals, 28 fall within the AD of at least one KOA QSPR used here.
Here, our predicted “consensus” KOW are 9.33 for EPPB and 7.93 for EFPT. For comparison, the REACH Registered Substances Database (https://echa.europa.eu/information-on-chemicals/registered-substances) reports experimental measurements as “>6.5” for EPPB and “>5.7” for EFPT. These experimental measurements are shown as ranges because they were derived using the HPLC method, which was limited by the KOW of the most hydrophobic calibration chemicals (6.5 and 5.7, respectively) used in the measurements. This comparison suggests that our predictions are reasonable.
The investigated chemicals' HLbiodeg have a 25th percentile of 27 days, a median of 45 days, and a 75th percentile of 73 days. All the investigated chemicals fall within the AD of the HLbiodeg QSPR used here. Our predicted HLbiodeg are 45 days for EPPB and 99 days for EFPT. To the best of our knowledge, HLbiodeg for these substances has not been experimentally measured before. However, results from ready biodegradability tests, as documented in the REACH Registered Substances Database, indicate that these chemicals were classified as “not readily biodegradable”, which means that they did not achieve 60% degradation within the 10 days window after 28 days of incubation. This lack of rapid biodegradability in the tests supports the plausibility of our predictions.
Finally, these chemicals are relatively susceptible to biotransformation in fish, with a median “consensus” HLbiotrans of 20 hours. All the investigated chemicals fall within the AD of at least one HLbiotrans QSPR used here.
Environmental persistence
Fig. 1A and B display the predicted POV for EPPB, EFPT, and their transformation products. EFPT shows a longer POV of 370 days, compared to that of 170 days for EPPB. Notably, EFPT's POV exceeds the POV of 331 days for γ-hexachlorocyclohexane (γ-HCH), which has shown to be the least persistent chemical among the 25 persistent organic pollutants (POPs) that are regulated by the Stockholm convention.38Fig. 1A and B show that 24 out of the 40 (60%) transformation products of EPPB are up to 2.6 times more persistent in the multimedia environment than EPPB, and 16 out of 26 (61%) transformation products of EFPT are up to 1.6 times more persistent than EFPT. Although EPPB is less persistent than the currently regulated POPs, 6 transformation products of EPPB and 19 transformation products of EFPT demonstrate a POV exceeding 331 days. This indicates that atmospheric oxidation generates chemicals that are comparably persistent to currently regulated POPs. In addition, the two LCMs investigated here and their transformation products possess POV (68–596 days) which are 29–252 times higher than those of organophosphate flame retardants (a class of globally ubiquitous emerging contaminants), such as tris(2-chloroisopropyl) phosphate and triphenyl phosphate.39,40
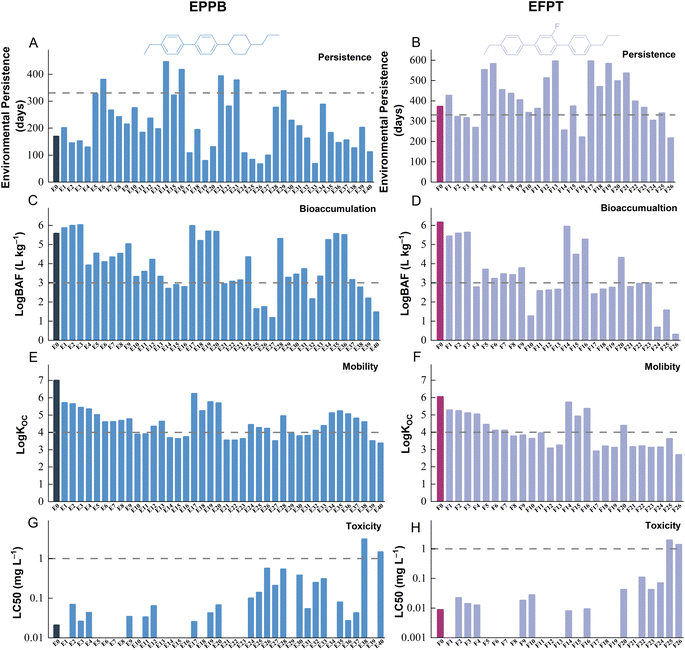 |
| Fig. 1 Persistence, bioaccumulation potential, mobility and acute toxicity of LCMs (EPPB and EFPT) and their transformation products. Environmental persistence (days) of (A) EPPB, and (B) EFPT and their corresponding transformation products. Bioaccumulation factor (BAF; L kg−1) of (C) EPPB, and (D) EFPT and their corresponding transformation products in fish. A higher BAF indicates a higher bioaccumulation potential. The minimum organic carbon normalized solid-water sorption coefficient (KOC) over the pH range of 4 to 9 of (E) EPPB and (F) EFPT and their corresponding transformation products. A lower KOC indicates higher mobility. Modelled fathead minnow LC50 values of (G) EPPB, and (H) EFPT. A lower fathead minnow LC50 indicates higher toxicity. LC50 predictions are missing for some transformation products because they contain structural features that cannot generate appropriate molecular descriptors by the TEST model and therefore cannot be predicted. Dark blue, and light blue represent EPPB and its transformation products, respectively. Dark purple and light purple represent EFPT and its transformation products, respectively. Dashed lines in A and B indicate the thresholds for overall persistence, i.e., the POV of γ-hexachlorocyclohexane (γ-HCH; 331 days), which has shown to be the least persistent chemical among the 25 POPs that are regulated by the Stockholm Convention.38 Dashed lines in C and D indicate the thresholds for BAF, i.e., chemicals with BAF higher than 1000 L kg−1 are considered to be bioaccumulative.42 Dashed lines in E and F indicate the thresholds for mobility, i.e., chemicals with KOC lower than 104 are considered to be mobile.35 Dashed lines in G and H indicate the thresholds for toxicity, i.e., chemicals with LC50 lower than 1 mg L−1 are considered to be highly toxic in aquatic organisms.49 | |
The increase in the overall persistence of these transformation products is because they are more enriched in land compartments (vegetation, water, soil, and sediment) compared to the air compartment; the degradation rate of products in surface media is typically two orders of magnitude slower than in air (Fig. S1†). This enrichment is facilitated by the lower volatility of transformation products, as evidenced by their higher KOA compared to the parent compounds (Fig. S2†), which favor their transfer from air to land compartments.
Bioaccumulation potential
As shown in Fig. 1C and D, both EPPB and EFPT have BAFs well above the threshold of 5000 L kg−1 that the Stockholm convention uses for bioaccumulation41 and the threshold of 1000 L kg−1 that the United States Environmental Protection Agency (EPA) uses for bioaccumulation.42Fig. 1C and D show that 83% and 100% of the transformation products of EPPB and EFPT, respectively, have estimated BAFs in fish lower than the corresponding parent compounds. In general, the BAF of a chemical depends on two “opposite” factors: (1) gastrointestinal and gill absorption of chemicals from the ambient environment and food web, and (2) metabolic and non-metabolic elimination (mainly gill elimination) of chemicals from the fish body.43 Both processes are impacted by the hydrophobicity of chemicals, characterized by the octanol–water partition coefficients (KOW). Atmospheric OH oxidation adds oxygen-containing functional groups (e.g., hydroxyl, carbonyl, and carboxyl groups) to LCMs, generating more hydrophilic transformation products, as suggested by the lower KOW for products compared to LCMs (Fig. S2†). In general, chemicals with high hydrophilicity, characterized by a KOW below 107, tend to have limited gill and gastrointestinal absorption because of the high transmembrane resistance to partition into lipid-rich gill and gastrointestinal epithelial tissues. Conversely, chemicals with very high hydrophobicity, characterized by a KOW above 109, may also have limited gill and gastrointestinal absorption because of (i) high transmembrane resistance posed by water-based boundary layers adjacent to the epithelial tissues,44 and (ii) low freely dissolved concentrations that are bioavailable.45 Notably, high transmembrane resistance in the gastrointestinal tract necessitates an extended period for these chemicals to reach equilibrium partitioning between fish and water, which may even exceed the residence time of digesta in the gastrointestinal tract and, therefore, limit gastrointestinal absorption.32 For these reasons, we may expect higher BAFs of certain investigated transformation products, i.e., those with KOW between 107 and 109, such as products E1–E3 (structures shown in Table S1†).
While the BAFs of most transformation products are lower than their parent compounds, this does not mean they are not bioaccumulative. Among the 66 transformation products investigated here, 44% of them (29 products) meet the “Stockholm convention criterion” (i.e., BAF > 5000 L kg−1), and 41 products meet the “EPA criterion” (i.e., BAF > 1000 L kg−1) and are considered to be bioaccumulative. Therefore, the bioaccumulation potential of transformation products warrants attention when assessing the environmental risks of LCMs.
Mobility
The high KOC of EPPB and EFPT indicate strong sorption of these chemicals on soil and sediment solids, which implies low mobility in the aquatic environments. However, the KOC for all investigated transformation products are one or two orders of magnitude lower than those of their parent compounds (Fig. 1E and F), indicating increased mobility. This can be expected given that atmospheric oxidation adds oxygen-containing functional groups (e.g., hydroxyl, carbonyl and carboxyl groups) to LCMs, generating more hydrophilic transformation products. While specific criteria for “mobility” are under discussion at this point, we tentatively adopt the German Environmental Agency's cut-off criterion (KOC lower than 104 over the pH range of 4–9) for illustration.35 With this criterion, the two parent compounds (EPPB and EFPT) are not identified to be “mobile”, while 35% of EPPB's and 58% of EFPT's transformation products become “mobile”. This contrast between the transformation products and their parent compounds emphasizes the necessity to further investigate their potential environmental impacts on aquatic ecosystem health and the safety of drinking water. Mobile chemicals, such as these transformation products, have a higher likelihood of being transported in the aqueous environment from their emission sources. In addition, they may pose challenges in terms of removal through drinking water and wastewater treatment procedures.46,47 Thus, understanding the environmental fate and potential risks of these transformation products is crucial for ensuring water quality and safeguarding aquatic ecosystems.35,48
Toxicity
Fig. 1G and H compare the toxicity to fathead minnow (representing aquatic ecological receptors) of the two LCMs and their transformation products. The predicted LC50 for the two parent LCMs are all well below 1 mg L−1. In general, the transformation products are predicted to possess slightly higher LC50 (i.e., less toxic) than EPPB and EFPT. Note that toxicity predictions are missing for certain transformation products because they contain structural features absent from the training set of the TEST model.
The finding that atmospheric oxidation slightly “detoxifies” EPPB and EFPT can be expected because the introduction of hydrophilic groups usually reduces the toxicity of chemicals. However, it is important to exercise caution when interpreting this finding, as the LC50 values of both parent compounds and transformation products are, in fact, quite close to each other, falling within a range of less than an order of magnitude. A closer examination of the TEST modeling procedures reveals two key factors contributing to this similarity: (i) TEST utilizes nearly identical sets of training chemicals for predicting both LCMs and transformation products, and (ii) the transformation products exhibit greater structural similarity to chemicals in the TEST training sets, in comparison to the parent compounds. As a result, the predictions for transformation products are considered to be relatively more reliable than those for parent compounds. By contrast, the observed subtle difference in predicted toxicity between transformation products and parent compounds can be attributed to the disparity in the similarity between these chemicals and TEST's training chemicals, which is highly dependent on the way that TEST defines and characterizes similarity between molecules. Therefore, it is essential not to over-interpret the difference in toxicity. Further investigation and validation are needed to gain a clearer understanding of the potential risks associated with the oxidation products of LCMs, which helps elucidate whether the observed detoxification effect is an artifact of the modeling process or a true representation of the evolution in toxicity of these chemicals.
Nevertheless, if we adopt the U.S. Fish and Wildlife Service (FWS) aquatic toxicity rating categorization based on LC50 values (“super toxic”, LC50: 0.01–0.1 mg L−1; “highly toxic”, LC50: 0.1–1 mg L−1; and “moderately toxic”, LC50: 1−10 mg L−1),49 26 transformation products are labeled to be “super toxic”, 8 transformation products are “highly toxic”, and 4 transformation products are “moderately toxic”, together accounting for 58% of the investigated transformation products.
We further analyze the carcinogenicity and mutagenicity of transformation products. As shown in Dataset S1,† a few LCM products are predicted to be potentially carcinogenic and mutagenic by the VEGA model. However, the model warns that the reliability for the predictions are low since the transformation products largely fall outside the applicability domains of the predictive models in VEGA. Therefore, future experimental and theoretical efforts are warranted to further elucidate the genetic toxicity of transformation products.
Impact of atmosphere photo-oxidation
With continued exposure to atmospheric oxidants, LCMs will be transformed into a series of oxidation products through multiple steps of reactions, generating early-generation (formed through less than three reaction steps) and later-generation products. Below we select one reaction channel of EFPT as a representative example to illustrate the impact of photo-oxidation on the properties of transformation products of different generations (Fig. 2A). In this reaction channel, EFPT reacts with OH radical and O2 to form alkylperoxy radicals, which can be transformed into product F1 (a hydroperoxide). With continued OH oxidation, product F1 leads to the formation of product F6 (a dihydroperoxide), which can then be converted to products F7 (a hydroxyl hydroperoxide) and F8 (a carbonyl hydroperoxide) through the Russell mechanism.50 Further reactions of products F7 and F8 would result in the formation of products F9 (a dicarbonyl) and F10 (a carboxylic acid).
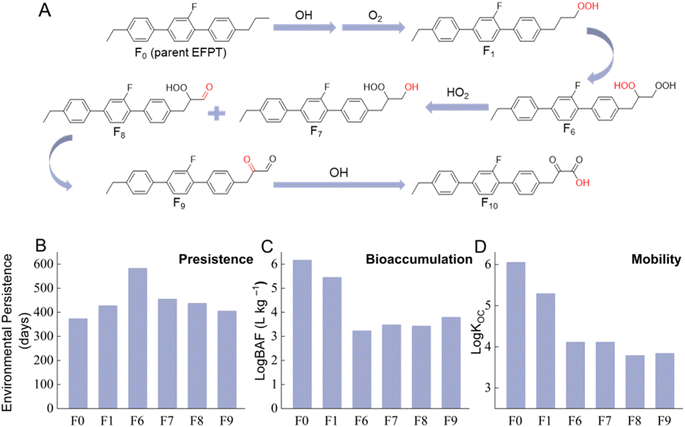 |
| Fig. 2 (A) A representative example of photo-oxidation reaction channel of EFPT. A comparison between the overall environmental persistence (B), bioaccumulation factor (C), and mobility (D) of EFPT transformation products formed from the reaction channel shown in A. | |
As shown in Fig. 2B, the POV of transformation products exhibits a gradually decreasing trend from F6 to F10 as oxidation reactions proceed. The first step of oxidation adds the OOH group to EFPT and generates a more hydrophilic transformation product (F1), which leads to an elevated POV. However, further oxidation does not significantly change the hydrophobicity of transformation products but lowers a chemical's resistance to biodegradation in the environment, as evidenced by the decreasing biodegradation half-lives of products F6–F10 (see Dataset S1†). Therefore, a decreasing trend for POV is observed as the oxidation reaction proceeds. It is noteworthy that, despite the observed decreasing trend for transformation products, the persistence of most products in Fig. 2A remained up to 56% higher than that of the parent EFPT.
Similar to the trend in POV, the BAFs of transformation products also exhibit a decreasing trend as oxidation reactions proceed (Fig. 2C). The BAF of product F10 (a later-generation product) is four orders of magnitude lower relative to that of F1 (an early-generation product). Compared to early-generation products, later-generation products F6–F10 exhibit lowered hydrophobicity and higher biotransformation potential (see Dataset S1†), both decreasing the BAF. In addition, since the transformation products' hydrophilicity increases with continued oxidation, the mobility of products increases as the reaction proceeds (Fig. 2D). With respect to the toxicity, the models cannot predict reliably for some transformation products in Fig. 2A (the model cannot generate appropriate molecular descriptors for predictions for F1, and F6–F8), preventing a definitive conclusion for the toxicity trend. Further study is warranted to investigate the dynamic evolution of transformation products' toxicity.
Uncertainties and limitations
This work presents an in silico model-based assessment of the hazard properties of LCM transformation products identified in the controlled laboratory experiments. Given the uncertainties in product identification and computational modeling, this work may suffer the following categories of limitations.
First, the transformation products in Table S1† were identified based upon the mass spectrometry data obtained from LCM oxidation experiments.13 Although this approach has been widely used for the identification of atmospheric transformation products of emerging contaminants such as organophosphates and synthetic antioxidants,51–53 it may still exhibit certain limitations. For example, the mass spectrometry used to detect LCM transformation products is unable to distinguish positional isomers and stereoisomers. When positional isomers exist, only a single isomer is selected for the hazard assessment in this work for simplicity. While this may introduce uncertainty into the property predictions, it should not affect the main conclusion of this work given that a previous study indicates that the positional isomers of organophosphate transformation products possess similar physicochemical properties.39 The absence of stereoisomerism does not pose an issue for in silico modeling, as QSARs and QSPRs used here are based on two-dimensional descriptors and do not take chirality into account.
Second, since QSARs and QSPRs rely on statistical relationships between chemical structures and the physicochemical and toxicity properties of interest, we acknowledge that their predictions can be subject to statistical errors. Such statistical errors can be quantified using metrics such as a root mean square error (RMSE) or a standard deviation (STD), and their magnitude can vary among different QSARs or QSPRs. For instance, when predicting KOW, the algorithms of OPERA, IFS-QSAR, and EPI Suite exhibit RMSEs or STDs of 0.79
log units, 0.15
log units, and 0.41
log units, respectively. Combining predictions from all three QSARs results in an overall uncertainty of
log units for KOW. Likewise, the calculated overall uncertainties for KOA, pKa, HLbiodeg, and HLbiotrans are 1.04
log units, 1.50
log units, 0.58
log units, and 0.57
log units, respectively. Furthermore, when using generic extrapolation ratios of 1
:
2
:
10 for degradation half-lives between water, surface soil, and sediment, additional uncertainties may be introduced. This is because these extrapolation ratios are statistical averages applied to a broad spectrum of chemicals and may not necessarily reflect the relationships for specific chemicals.
Third, we also need to acknowledge the uncertainties associated with PROTEX's algorithm. Models, by their nature, simplify the intricacies of the real-world environment. Therefore, the simplifying assumptions may cause uncertainties in estimates. For instance, when evaluating bioaccumulation, PROTEX relies on the assumption of a steady state, which represents a conservative “worst-case scenario” as recommended by the EU Technical Guidance Document to account for the potential maximum contamination in fish. Similarly, when assessing persistence, PROTEX assumes a well-mixing and homogeneous distribution of chemicals in different environmental compartments. Such simplifying assumptions might not fully capture the complexities of real-world conditions and can introduce biases.
For these reasons, it is advisable to interpret the hazard predictions outlined here with caution. It is also essential to underscore that these uncertainties are, for the most part, inevitable, primarily because of the lack of experimentally measured chemical properties and the inherent nature of in silico models, notably for “data-poor” chemicals with limited available experimental data, such as LCMs and their transformation products.
Environmental implications
This work provides new insights into the environmental hazards of LCMs. The results suggest that atmospheric transformation can significantly change the hazard properties of LCMs. Most transformation products are more persistent, less bioaccumulative, more mobile, and slightly less toxic than their parent compounds. Therefore, accounting for the impacts of atmospheric transformation should be considered critical for making accurate risk evaluations of LCMs. In addition, as noted above, a few transformation products are beyond the TEST model's capability of generating appropriate molecular descriptors in toxicity predictions, leading to unknown information for these products. This highlights the need to conduct in vitro and in vivo experiments in future studies to illustrate the health impacts of transformation products. Finally, we note that limited consideration has previously been given to atmospheric chemical transformation when evaluating the environmental and health risks of emerging contaminants. Recent studies indicate that the exposure risks associated with organophosphate flame retardants (another emerging contaminant) and bisphenols (a well-studied contaminant) may be underestimated due to the lack of consideration for their atmospheric chemical reactions.39,54 Together with the current LCM work, this underscores the necessity to include atmospheric transformations in the development of control measures for emerging contaminants.
Author contributions
Jinlong Wang formal analysis (lead), investigation (lead), writing-original draft (lead); Shenghong Wang methodology (lead), formal analysis (lead), investigation (lead), writing-original draft (lead); Zhizhen Zhang methodology (supporting), investigation (supporting), writing-review & editing (supporting); Xinkai Wang writing-review & editing (supporting); Kaihui Xia writing-review & editing (supporting); Li Li conceptualization (lead), methodology (lead), investigation (lead), writing-review & editing (lead), funding acquisition (lead), supervision (lead); Qifan Liu conceptualization (lead), investigation (lead), writing-review & editing (lead), funding acquisition (lead), supervision (lead).
Conflicts of interest
The authors declare no competing financial interest.
Acknowledgements
Q. L. is grateful for financial support from the National Natural Science Foundation of China (No. 42275101); S. W., Z. Z., and L. L. are grateful for financial support from Environment and Climate Change Canada (No. GCXE22S061).
References
- J. Li, G. Su, R. J. Letcher, W. Xu, M. Yang and Y. Zhang, Liquid crystal monomers (LCMs): a new generation of persistent bioaccumulative and toxic (PBT) compounds?, Environ. Sci. Technol., 2018, 52, 5005–5006 CrossRef CAS.
- H. Su, S. Shi, M. Zhu, D. Crump, R. J. Letcher, J. P. Giesy and G. Su, Persistent, bioaccumulative, and toxic properties of liquid crystal monomers and their detection in indoor residential dust, Proc. Natl. Acad. Sci. U. S. A., 2019, 116, 26450–26458 CrossRef CAS PubMed.
-
S&P Global, https://ihsmarkit.com/index.html, accessed 20 March 2019.
- Q. Liu and J. P. D. Abbatt, Liquid crystal display screens as a source for indoor volatile organic compounds, Proc. Natl. Acad. Sci. U. S. A., 2021, 118, 1–7 Search PubMed.
- Q. Jin, D. Y. Tao, Y. C. Lu, J. J. Sun, C. H. Lam, G. Su and Y. H. He, New insight on occurrence of liquid crystal monomers: a class of emerging e-waste pollutants in municipal landfill leachate, J. Hazard. Mater., 2022, 423, 1–8 Search PubMed.
- H. Su, S. Shi, M. Zhu, J. Li and G. Su, Liquid crystal monomers (LCMs) in sediments: method validation and detection in sediment samples from three typical areas, Environ. Sci. Technol., 2021, 55, 2336–2345 CrossRef CAS.
- S. Zhang, M. Yang, Y. Li, Y. Wang, Y. Lu, Z. Cheng and H. Sun, Occurrence, distribution, and human exposure of emerging liquid crystal monomers (LCMs) in indoor and outdoor dust: a nationwide study, Environ. Int., 2022, 164, 1–8 Search PubMed.
- M. Shen, Z. Feng, X. Liang, H. Chen, C. Zhu, B. Du, Q. Li and L. Zeng, Release and gas–particle partitioning behavior of liquid crystal monomers during the dismantling of waste liquid crystal display panels in e-waste recycling facilities, Environ. Sci. Technol., 2022, 56, 3106–3116 CrossRef CAS PubMed.
- X. Liang, R. Xie, C. Zhu, H. Chen, M. Shen, Q. Li, B. Du, D. Luo and L. Zeng, Comprehensive identification of liquid crystal monomers—Biphenyls, cyanobiphenyls, fluorinated biphenyls, and their analogues—in waste LCD panels and the first estimate of their global release into the environment, Environ. Sci. Technol., 2021, 55, 12424–12436 CrossRef CAS PubMed.
- H. Zhao, C. Li, M. Y. Naik, J. Wu, A. Cardilla, M. Liu, F. Zhao, S. A. Snyder, Y. Xia, G. Su and M. Fang, Liquid crystal monomer: a potential PPARγ antagonist, Environ. Sci. Technol., 2023, 57, 3758–3771 CrossRef CAS PubMed.
- L. Y. Liu, J. F. Xie, Z. M. Yu and E. Y. Zeng, Liquid crystal monomers in multimedia environments and potential human exposure risk: a short review, Curr. Opin. Environ. Sci. Health, 2023, 32, 1–7 Search PubMed.
- C. Li, Y. Huang, X. Zhang, Y. H. Zhao and Y. Huo, Atmospheric fate and risk investigation of typical liquid crystal monomers, ACS Sustainable Chem. Eng., 2021, 9, 3600–3607 CrossRef CAS.
- Q. Liu, J. Liggio, J. Wentzell, P. Lee, K. Li and S. M. Li, Atmospheric OH oxidation chemistry of particulate liquid crystal monomers: an emerging persistent organic pollutant in air, Environ. Sci. Technol. Lett., 2020, 7, 646–652 CrossRef CAS.
-
European Union (EU), Regulation (EC) No 1272/2008 of the European Parliament and of the Council of 16 December 2008 on Classification, Labelling and Packaging of Substances and Mixtures, Amending and Repealing Directives 67/548/EEC and 1999/45/EC, and Amending Regulation (EC) No 1907/2006, 2023 Search PubMed.
- L. Li, J. A. Arnot and F. Wania, Towards a systematic understanding of the dynamic fate of polychlorinated biphenyls in indoor, urban and rural environments, Environ. Int., 2018, 117, 57–68 CrossRef CAS PubMed.
- L. Li, C. Hoang, J. A. Arnot and F. Wania, Clarifying temporal trend variability in human
biomonitoring of polybrominated diphenyl ethers through mechanistic modeling, Environ. Sci. Technol., 2020, 54, 166–175 CrossRef CAS PubMed.
- L. Li, J. A. Arnot and F. Wania, Revisiting the contributions of far- and near-field routes to aggregate human exposure to polychlorinated biphenyls (PCBs), Environ. Sci. Technol., 2018, 52, 6974–6984 CrossRef CAS PubMed.
- D. Li, A. Sangion and L. Li, Evaluating consumer exposure to disinfecting chemicals against coronavirus disease 2019 (COVID-19) and associated health risks, Environ. Int., 2020, 145, 106108 CrossRef CAS PubMed.
- S. Niu, X. Chen, R. Chen, Y. Zou, Z. Zhang, L. Li, K. J. Hageman, C. Ng and L. Dong, Understanding inter-individual variability in short-chain chlorinated paraffin concentrations in human blood, J. Hazard. Mater., 2023, 443, 130235 CrossRef CAS.
- L. Li and D. Li, Inter-individual variability and non-linear dose-response relationship in assessing human health impact from chemicals in lca: addressing uncertainties in exposure and toxicological susceptibility, Front. Sustain., 2021, 2, 648138 CrossRef.
- E. Webster, D. Mackay, A. Di Guardo, D. Kane and D. Woodfine, Regional differences in chemical fate model outcome, Chemosphere, 2004, 55, 1361–1376 CrossRef CAS PubMed.
-
U.S. Environmental Protection Agency (US EPA), Toxics, Exposure Assessment Tools and Models, Estimation Program Interface (EPI) Suite, Version 4.11, https://www.epa.gov/opptintr/exposure/pubs/episuite.htm Search PubMed.
- T. N. Brown, J. A. Arnot and F. Wania, Iterative fragment selection: a group contribution approach to predicting fish biotransformation half-lives, Environ. Sci. Technol., 2012, 46, 8253–8260 CrossRef CAS.
- T. N. Brown, QSPRs for predicting equilibrium partitioning in solvent-air systems from the chemical structures of solutes and solvents, J. Solut. Chem., 2022, 51, 1101–1132 CrossRef CAS.
- T. N. Brown, J. M. Armitage and J. A. Arnot, Application of an iterative fragment selection (IFS) method to estimate entropies of fusion and melting points of organic chemicals, Mol. Inf., 2019, 38, 10 Search PubMed.
- K. Mansouri, C. Grulke, R. Judson and A. Williams, OPERA: a free and open source QSAR tool for predicting physicochemical properties and environmental fate endpoints, Abstr. Pap. Am. Chem. Soc., 2018, 255, 1 Search PubMed.
- P. Gramatica, S. Cassani and N. Chirico, QSARINS-Chem: insubria datasets and new QSAR/QSPR models for environmental pollutants in QSARINS, J. Comput. Chem., 2014, 35, 1036–1044 CrossRef CAS PubMed.
- P. Gramatica, N. Chirico, E. Papa, S. Cassani and S. Kovarich, QSARINS: a new software for the development, analysis, and validation of QSAR MLR models, J. Comput. Chem., 2013, 34, 2121–2132 CrossRef CAS.
- L. Li, Z. Zhang, Y. Men, S. Baskaran, A. Sangion, S. Wang, J. A. Arnot and F. Wania, Retrieval, selection, and evaluation of chemical property data for assessments of chemical emissions, fate, hazard, exposure, and risks, ACS Environ. Au, 2022, 2, 376–395 CrossRef CAS PubMed.
- K. Fenner, M. Scheringer, M. MacLeod, M. Matthies, T. McKone, M. Stroebe, A. Beyer, M. Bonnell, A. C. Le Gall, J. Klasmeier, D. Mackay, D. Van De Meent, D. Pennington, B. Scharenberg, N. Suzuki and F. Wania, Comparing estimates of persistence and long-range transport potential among multimedia models, Environ. Sci. Technol., 2005, 39, 1932–1942 CrossRef CAS PubMed.
- E. Webster, D. Mackay and F. Wania, Evaluating environmental persistence, Environ. Toxicol. Chem., 1998, 17, 2148–2158 CrossRef CAS.
- D. Mackay and E. Webster, Environmental persistence of chemicals, Environ. Sci. Pollut. Res., 2006, 13, 43–49 CrossRef CAS.
- M. Scheringer, K. C. Jones, M. Matthies, S. Simonich and D. van de Meent, Multimedia partitioning, overall persistence, and long-range transport potential in the context of POPs and PBT chemical assessments, Integr. Environ. Assess. Manage., 2009, 5, 557–576 CrossRef CAS.
- J. A. Arnot and F. A. Gobas, A review of bioconcentration factor (BCF) and bioaccumulation factor (BAF) assessments for organic chemicals in aquatic organisms, Environ. Rev., 2006, 14, 257–297 CrossRef CAS.
-
I. S. Michael Neumann, Protecting the sources of Our Drinking Water: The Criteria for identifying Persistent, Mobile and Toxic (PMT) Substances and Very Persistent and Very Mobile (vPvM) Substances Under EU Regulation REACH (EC) No 1907/2006, Umweltbundesamt Wörlitzer Platz 1, 2019 Search PubMed.
- A. Franco and S. Trapp, Estimation of the soil-water partition coefficient normalized to organic carbon for ionizable organic chemicals, Environ. Toxicol. Chem., 2008, 27, 1995–2004 CrossRef CAS.
-
T. Martin, User's Guide for T.E.S.T. (Version 4.2) Toxicity Estimation Software Tool: A Program to Estimate Toxicity from Molecular Structure, Sustainable Technology Division, National Risk Management Research Laboratory, U.S. Environmental Protection Agency, Cincinnati, 2016 Search PubMed.
- L. Li, C. Chen, D. Li, K. Breivik, G. Abbasi and Y.-F. Li, What do we know about the production and release of persistent organic pollutants in the global environment?, Environ. Sci.: Adv., 2023, 2, 55–68 CAS.
- Q. Liu, L. Li, X. Zhang, A. Saini, W. Li, H. Hung, C. Hao, K. Li, P. Lee, J. J. B. Wentzell, C. Huo, S. M. Li, T. Harner and J. Liggio, Uncovering global-scale risks from commercial chemicals in air, Nature, 2021, 600, 456–461 CrossRef CAS.
- A. Saini, T. Harner, S. Chinnadhurai, J. K. Schuster, A. Yates, A. Sweetman, B. H. Aristizabal-Zuluaga, B. Jimenez, C. A. Manzano, E. O. Gaga, G. Stevenson, J. Falandysz, J. Ma, K. S. B. Miglioranza, K. Kannan, M. Tominaga, N. Jariyasopit, N. Y. Rojas, O. Amador-Munoz, R. Sinha, R. Alani, R. Suresh, T. Nishino and T. Shoeib, GAPS-megacities: a new global platform for investigating persistent organic pollutants and chemicals of emerging concern in urban air, Environ. Pollut., 2020, 267, 1–10 CrossRef PubMed.
-
United Nations (UN), Stockholm Convention, https://www.pops.int/, accessed 2004 Search PubMed.
-
U.S. Environmental Protection Agency (US EPA), 40 CFR Part 372 Persistent Bioaccumulative Toxic (PBT) Chemicals; Proposed Rule, U.S. Environmental Protection Agency, Washington, 1999, vol. 64, pp. 624–637 Search PubMed.
- Z. Zhang, S. Wang and L. Li, Emerging investigator series: the role of chemical properties in human exposure to environmental chemicals, Environ. Sci. Process. Impacts, 2021, 23, 1839–1862 RSC.
- J. A. Arnot and F. Gobas, A food web bioaccumulation model for organic chemicals in aquatic ecosystems, Environ. Toxicol. Chem., 2004, 23, 2343–2355 CrossRef CAS PubMed.
- J. Glüge, B. I. Escher and M. Scheringer, How error-prone bioaccumulation experiments affect the risk assessment of hydrophobic chemicals and what could be improved, Integr. Environ. Assess. Manage., 2023, 19, 792–803 CrossRef PubMed.
- S. E. Hale, H. P. H. Arp, I. Schliebner and M. Neumann, Persistent, mobile and toxic (PMT) and very persistent and very mobile (vPvM) substances pose an equivalent level of concern to persistent, bioaccumulative and toxic (PBT) and very persistent and very bioaccumulative (vPvB) substances under REACH, Environ. Sci Eur., 2020, 32, 1–15 CrossRef.
- E. Fries, T. Grewal and R. Sühring, Persistent, mobile, and toxic plastic additives in Canada: properties and prioritization, Environ. Sci.-Process Impacts, 2022, 24, 1945–1956 RSC.
- T. Reemtsma, U. Berger, H. P. H. Arp, H. Gallard, T. P. Knepper, M. Neumann, J. B. Quintana and P. D. Voogt, Mind the gap: persistent and mobile organic compounds—water contaminants that slip through, Environ. Sci. Technol., 2016, 50, 10308–10315 CrossRef CAS PubMed.
-
M. El-Harbawi, Toxicity measurement of imidazolium ionic liquids using acute toxicity test, in 1st UNPAR International Conference and Workshop on Chemical Engineering and Process Safety (ICCE UNPAR), Elsevier Science Bv, Kuta, indonesia, 2012, pp. 40–52 Search PubMed.
- S. Miyamoto, G. R. Martinez, M. H. G. Medeiros and P. Di Mascio, Singlet molecular oxygen generated from lipid hydroperoxides by the Russell Mechanism: studies using o-18-labeled linoleic acid hydroperoxide and monomol light emission measurements, J. Am. Chem. Soc., 2003, 125, 6172–6179 CrossRef CAS PubMed.
- Q. Liu, J. Liggio, D. M. Wu, A. Saini, S. Halappanavar, J. J. B. Wentzell, T. Harner, K. Li, P. Lee and S. M. Li, Experimental study of OH-Initiated heterogeneous oxidation of organophosphate flame retardants: kinetics, mechanism, and toxicity, Environ. Sci. Technol., 2019, 53, 14398–14408 CrossRef CAS PubMed.
- H. N. Zhao, X. M. Hu, Z. Y. Tian, M. Gonzalez, C. A. Rideout, K. T. Peter, M. C. Dodd and E. P. Kolodziej, Transformation products of tire rubber antioxidant 6PPD in heterogeneous gas-phase ozonation: identification and environmental occurrence, Environ. Sci. Technol., 2023, 57, 5621–5632 CrossRef CAS PubMed.
- Q. Liu, R. Liu, X. Zhang, W. Li, T. Harner, A. Saini, H. Y. Liu, F. Yue, L. Zeng, Y. Zhu, C. Y. Xing, L. Li, P. Lee, S. R. Tong, W. G. Wang, M. F. Ge, J. J. Wang, X. G. Wu, C. Johannessen, J. Liggio, S. M. Li, H. Y. Hung, Z. G. Xie, S. A. Mabury and J. P. D. Abbatt, Oxidation of commercial antioxidants is driving increasing atmospheric abundance of organophosphate esters implication for global regulation, One Earth, 2023, 6, 1202–1212 CrossRef.
- D. Yang, Q. Liu, S. Wang, M. Bozorg, J. Liu, P. Nair, P. Balaguer, D. Song, H. Krause, B. Ouazia, J. P. D. Abbatt and H. Peng, Widespread formation of toxic nitrated bisphenols indoors by heterogeneous reactions with HONO, Sci. Adv., 2022, 8, 1–12 Search PubMed.
Footnotes |
† Electronic supplementary information (ESI) available: Chemical structures of LCM transformation products; additional details on the physicochemical properties of LCM transformation products. Dataset S1: persistence, bioaccumulation potential, mobility, and toxicity data of LCMs and their transformation products. See DOI: https://doi.org/10.1039/d3em00424d |
‡ These authors contributed equally to this work. |
|
This journal is © The Royal Society of Chemistry 2024 |