Market optimization and technoeconomic analysis of hydrogen-electricity coproduction systems†
Received
1st June 2024
, Accepted 9th October 2024
First published on 16th October 2024
Abstract
Decarbonization efforts across North America, Europe, and beyond rely on variable renewable energy sources such as wind and solar, as well as alternative fuels, such as hydrogen, to support the sustainable energy transition. These advancements have prompted a need for more flexibility in the electric grid to complement non-dispatchable energy sources and increased demand from electrification. Integrated energy systems are well suited to provide this flexibility, but conventional technoeconomic modeling paradigms neglect the time-varying dynamic nature of the grid and thus undervalue resource flexibility. In this work, we develop a computational optimization framework for dynamic market-based technoeconomic comparison of integrated energy systems that coproduce low-carbon electricity and hydrogen (e.g., solid oxide fuel cells, solid oxide electrolysis) against technologies that only produce electricity (e.g., natural gas combined cycle with carbon capture) or only produce hydrogen. Our framework starts with rigorous physics-based process models, built in the open-source Institute for the Design of Advanced Energy Systems (IDAES) modeling and optimization platform, for six energy process concepts. Using these rigorous models and a workflow to optimally design each technology, the framework is shown to be capable of evaluating new and emerging technologies in varying energy markets under a plethora of future scenarios (i.e., renewables penetration, carbon tax, etc.). Ultimately, our framework finds that solid oxide fuel cell-based coproduction systems achieve positive profits for 85% of the analyzed market scenarios. From these market optimization results, we use multivariate linear regression (R2 values up to 0.99) to determine which electricity price statistics are most significant to predict the optimized annual profit of each system. The proposed framework provides a powerful tool for directly comparing flexible, multi-product energy process concepts to help discern optimal technology and integration options.
Broader context
Integrated energy systems (IESs) provide power grid flexibility by coupling multiple technologies and products to create more efficient and dynamically responsive systems. Due to hydrogen's anticipated role in decarbonization, we investigate the integration of electricity and hydrogen production via solid oxide electrolysis. We develop a framework to directly compare technology options of varying flexibility and demonstrate its use via six process concepts. Our results indicate that solid oxide fuel cell (SOFC) and solid oxide electrolyzer cell (SOEC)-based IESs have significant economic advantages over standalone fuel cells and natural gas combined cycles. Integrated systems that coproduce electricity and hydrogen – SOFC + SOEC and reversible solid oxide cell (rSOC) – are profitable in 85% and the top performer in 74% of considered market scenarios. The SOFC and rSOC technologies also show extremely flexible performance in anticipated market scenarios with high levels of variable renewable energy penetration. These findings strongly encourage research investments in SOFC and SOEC technologies, especially flexible coproduction systems.
|
1. Introduction
Broad efforts across the U.S. and beyond to decarbonize the electric grid and energy-intensive industries have led to variable renewable energy (VRE) sources such as solar and wind growing faster than any other technology.1 VRE sources cannot control their energy production beyond curtailment, which exacerbates the difficulties in balancing supply and demand, often resulting in dramatic and undesirable ramping events for dispatchable generation resources which are generally designed for baseload operations.2–4 This being said, alternate energy carriers such as hydrogen (H2) have been investigated to support economy-wide decarbonization. Energy systems with increased flexibility, which can respond quickly to supply and demand imbalances, are needed to integrate VRE sources into the electric grid while maintaining efficiency, cost competitiveness, reliability, and resiliency. Integrated energy systems (IESs) provide grid flexibility by exploiting synergies from combining multiple technologies while switching rapidly between operating modes, e.g., switching from electricity to H2 production, in response to grid demands. As a result of this increased flexibility, IESs have been shown to have many benefits, including lower costs5 and reduced emissions6 compared to prevailing standalone technologies.
Prior work has highlighted the potential of H2 to decarbonize multiple industrial sectors7–9 and support the transition to higher VRE penetration.10–13 Bødal and colleagues showed that joint planning of H2 and electricity production can reduce the cost of grid expansion.14 Accounting for these benefits, H2 production is a great candidate for integration with electricity production processes by offering additional grid flexibility and an additional revenue opportunity for participants in increasingly volatile power markets.
Fuel cell technologies are becoming increasingly popular for power and H2 generation. In terms of electrical power, solid oxide fuel cells (SOFCs) are particularly interesting, as they have comparably better fuel flexibility, more capacity options, and higher efficiency than other fuel cell types.15,16 Additionally, while posing general operating challenges, their high-temperature operation makes them an ideal candidate for closely-coupled heat integration with other processes (i.e., H2 or liquid fuel) to improve overall process efficiencies.17 Prior reviews highlight current deployments of SOFCs,18 the outlook of SOFCs for grid-scale deployment and integration with other systems,19 as well as current research in improving SOFC technology, including materials advancements and systems design.20
Low temperature fuel cell (LTFC) technologies of present interest include alkaline fuel cell (AFC) and proton-exchange membrane fuel cell (PEMFC) systems.21–24 AFCs and PEMFCs, due to their short start-up times and portability, are generally utilized for small-scale applications in the 1–500 kW range such as backup power, transportation, and distributed generation. However, carbon dioxide (CO2) severely limits the performance and life of an AFC, while a PEMFC is susceptible to poisoning from a variety of impurities including carbon monoxide. Consequently, such systems cannot leverage a low-cost feedstock like natural gas without introducing pre-processing steps to produce pure H2 fuel with significant adverse impacts on overall system electrical efficiency. High temperature SOFC systems, on the other hand, can produce power operating directly on natural gas while utilizing both the process and waste heat effectively to result in much higher system efficiencies relative to any of the LTFCs.16,25,26 SOFC systems are also typically more electrically efficient than a molten carbon fuel cell (MCFC), which operates at a slightly lower temperature while eschewing the long-term durability implications of the corrosive MCFC electrolyte.
Concomitantly, high temperature solid oxide electrolyzer cell (SOEC) technologies can use both electricity and waste heat to produce H2 at efficiencies that can be 25 percentage points higher than low temperature proton exchange membrane (PEM) electrolysis.27,28 These heightened efficiencies hold immense promise in reducing the electricity demand, a primary cost driver in H2 production through electrolysis. Additionally, achieving the ambitious hydrogen cost target of 1.00 $ kg−1, as outlined in the Department of Energys Hydrogen Shot initiative,29 may necessitate such elevated efficiencies given that PEM-based H2 production, even after optimizing design and dynamic operation for time-varying electricity prices, is well-above this threshold.30
Accordingly, the present study focuses on SOFC and SOEC technologies with the potential to produce power and H2 at high efficiencies, which along with the capability to operate reversibly between the power and electrolysis modes can result in higher round trip efficiencies (cycling between power and electrolysis modes) than low temperature fuel cell technologies. Additionally, their higher temperature operation and ability to utilize waste heat make them particularly attractive for integration with existing technologies. There is a wide variety of prior work on novel SOFC-based IES (SOFC-IES) concepts and different technology options for these systems. Many of these works focus on developing highly detailed, physics-based models of the system and optimizing the steady-state operation for increased efficiency,31–35 reduced costs,32,34,36 less environmental impact,36 and other objectives.32,34,35,37,38
Traditional cost optimizations often hinge on the levelized cost of electricity (LCOE) or similar cost metrics. These static methods have sufficed for the historical grid, dominated by base-loaded systems. However, current and future grid optimization needs to consider the intermittency imposed by increased VRE penetration and electrification. Instead of considering a time-varying operating profile, LCOE calculates the system's lifetime costs and divides them by an assumed lifetime electricity production to find a cost per unit of energy produced.39 LCOE is one of the most popular technoeconomic analysis (TEA) metrics because it is easy to calculate and provides a means to compare power generation costs with prices in different regions quickly. However, because LCOE assumes a fixed capacity factor regardless of market dynamics, it does not value system flexibility and is a poor predictor for grid-parity for some technologies.40–42 As such, new TEA methods that go beyond LCOE and capture dynamic market forces are needed to capture the value of flexibility, especially for IESs.43–45 For example, Naeini et al.38 developed a detailed model of SOFC cell degradation over time which optimized cell size and cell replacement schedule for 20 years of the plant. Through these models, they found that the mode of operation of SOFCs significantly impacts LCOE. Glenk and colleagues evaluated reversible power-to-gas systems46 and forecast technology cost and the efficiency of such technology46,47 finding that even under current dynamic energy markets, power-to-gas systems can be profitable. However, integration and retrofitting with existing natural gas-based technologies and important operational constraints (i.e., minimum production constraints, minimum up and down time constraints) were not considered and only two electricity markets (Germany and Texas) were studied. To the authors’ knowledge, no prior studies have systematically evaluated the operation of SOFC-IESs in the context of a wide variety of both historical and forecast wholesale electricity market scenarios.
In this work, we address these gaps by developing a novel computational optimization framework to predict the maximum possible profits of SOFC-IESs in the context of different energy markets. We also address important operational decisions such as minimum up and down times, minimum operating loads, and modular coproduction that were not considered previously.46,47 Using this framework, we directly compare the performance of multiple process concepts, each with 97% or greater carbon capture and storage (CCS), that hybridize SOFCs or natural gas combined cycles (NGCCs) for power generation with SOECs for H2 production. For each of the six technologies (Fig. 1), we optimize their operation within many historical and forecast real-time energy markets under many carbon tax and renewables penetration conditions. We find that SOFC and SOEC-based H2 production and coproduction IESs, even within historical conditions, provide a lucrative option at high throughput, promoting research into the scale-up of existing SOFC/SOEC technologies. As the framework allows for direct comparison of economic performance of energy process concepts, it has the potential to serve as a powerful tool to assist in discerning optimal technology and integration options for future energy system deployment and strategically guide R&D investments in enabling the systems.
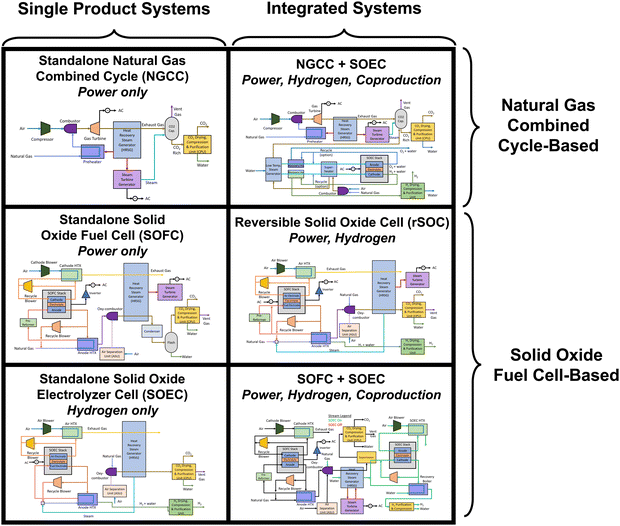 |
| Fig. 1 Process flow diagrams of process concepts compared in this work. The left column contains single product systems, including electricity (NGCC and SOFC) and H2 (SOEC) producers. The right column contains integrated concepts of those technologies. The top row are NGCC-based concepts, and the bottom two rows are SOFC-based. Each concept is fitted with greater than 97% carbon capture. For more information on the models see ref. 48 and 49. Larger images of each process concept is included in the ESI† (S9). | |
2. Methodology
2.1. Technology overview – process concepts
We compare six process concepts, shown in Fig. 1, that produce electric power, H2, or both. The (1) standalone NGCC system (Fig. 1 top-left) is based on case B31B in the U.S. Department of Energy (DOE) National Energy Technology Laboratory (NETL) fossil-energy baseline report50 and serves as a baseline for comparison. The hybrid (2) NGCC + SOEC system (Fig. 1 top-right) uses steam and electricity from the NGCC subsystem to produce H2 instead of electricity when market prices are low. The two NGCC-based concepts are equipped with a solvent-based post-combustion carbon capture system operating at 97% capture. The (3) standalone SOFC (Fig. 1 middle-left) and (4) standalone SOEC (Fig. 1 bottom-left) systems produce power-only and H2-only, respectively. The (5) SOFC + SOEC system (Fig. 1 bottom-right) has the flexibility to produce power from the SOFC when electricity prices are high or utilize some of the power, either from the SOFC or grid purchase, to produce H2 when electricity prices are low. The (6) reversible solid oxide cell (rSOC) system (Fig. 1 middle-right) is less flexible, able to produce electricity and H2 separately but not simultaneously.
The primary energy sources for all systems are natural gas, grid electricity, or both depending on the mode of operation. SOFC-based concepts assume complete internal reformation of the natural gas and employ a cryogenic distillation process to achieve at least 97% carbon capture. They are based on case ANGFC3B of NETL's natural gas fuel cells (NGFC) pathways study.48 Capacities range from 650 MW to 712 MW for the power generation systems, while the H2 generation capacities are all 5 kg s−1. The H2 specification is 6.479 MPa with less than 10 ppm water. Transport and storage costs for carbon dioxide and H2 were deemed outside the scope of this analysis, and tax credits for carbon sequestration or clean H2 production are not considered. More information on how H2 transportation costs can be incorporated is included at the end of Section 3.4. The capital costing approach assumes successful research and development and learning associated with mass-scale commercial deployment of SOFC technology (i.e., nth-of-a-kind plants) as previously described.48 Wherever possible, cost assumptions from the NGFC pathways study were used for the SOEC systems as well. Eslick et al.49 describes the process concepts, costing assumptions, and modeling approach in detail.
2.2. Modeling and optimization workflow
We utilize a multi-step computational framework to optimize and evaluate (see Fig. 2) the economic performance of process concepts (e.g., Fig. 1) and ultimately compare concepts against one another in various dynamic electricity price market scenarios. As described above, we start with rigorous steady-state process models developed in the open-source IDAES modeling and optimization platform.51 Then, algebraic surrogate models are trained using data from these rigorous models to emulate technology performance. Finally, these models, along with operational constraints, are combined with historical or forecasted market prices to optimize annual operation. These optimal operational schedules can then be compared to evaluate which process concept and market signal pairings could result in profitable investments.
 |
| Fig. 2 Framework for market optimization and technoeconomic analysis of process alternatives. (A) Algebraic surrogate models are trained from rigorous nonlinear steady-state process optimization results from the IDAES-PSE framework. Analyzing the partial derivatives of these surrogate models reveals the marginal costs of H2 and electricity for each system. (B) Past, present, and future hourly time-series electricity price data (historical, forecasts) are collected for six U.S. wholesale markets: CAISO, ERCOT, MISO, NYISO, PJM, SPP. (C) Over one thousand instances of the multi-period optimization problem (using the algebraic surrogates from box A) are solved to analyze all six energy process concepts using 61 electricity market scenarios and five fixed H2 price scenarios. (D) Energy process concepts are compared using annual net profit, electricity and H2 capacity factors, and other performance metrics. Linear regression analysis reveals which electricity market price statistics are most significant in predicting the annual net profit of each system. | |
2.3. Surrogate modeling
In this work, we go beyond the static LCOE analysis and include dynamic price signals, which require a detailed IES model (thousands of linear and nonlinear equations) at each hour. The high detail of the IES model equations for each time period (8760 hours in a one-year time horizon) make conventional simultaneous equation-oriented optimization intractable for year-long operational decision-making. Therefore, we train algebraic surrogates of the detailed IES models of the six process concepts using the Automatic Learning of Algebraic Models (ALAMO) modeling tool52 (available within the IDAES package). Training data were collected from the steady-state IDAES process models, which optimize system operation to minimize costs. Then, utilizing the IDAES surrogate application programming interface (API), the ALAMO framework determines the best algebraic surrogate form based on Bayesian information content and returns surrogate equations for fixed costs, fuel costs, other variable costs (e.g., water and chemicals purchasing, water treatment) and inequalities representing the feasible operating region. The IDAES surrogate API provides visualization, validation, and cross-validation to avoid over-fitting. Fixed cost equations were obtained by calculating each system's capital costs over a range of feasible plant sizes and fitting a power law equation to represent costs as a function of plant size.49,53 For this analysis, equipment sizes were fixed to 650 MW net max power and 5 kg s−1 H2. Variable cost equations (fuel and non-fuel) were developed by fitting a curve to the optimized operating costs at different plant operating points between the minimum turndown and maximum system capacity. These cubic equations contain co-linear terms when two products can be produced simultaneously (NGCC + SOEC and SOFC + SOEC). R2 values between 0.97 and 1.0 indicate an excellent fit (see ESI,† Table S6). For systems where electricity and H2 coproduction are possible (i.e., SOFC + SOEC and NGCC + SOEC), we determine a feasible operating region for H2 production for each system based on simultaneous energy production data and electricity purchase from the grid.
Besides improving computational tractability, the surrogate cost equations facilitate rapid grid-parity assessments, similar to an LCOE assessment. By taking the first derivative of cost with respect to power or H2 output, we can determine marginal cost for power or H2 at different operating points (or cost incurred by producing one unit more of a product). In Fig. 2 box A, the left plot represents the marginal cost of power, and the right plot represents the marginal cost of H2. The black contours on the plot report the marginal cost, corresponding to the colors on the grid behind it. The horizontal axis represents power output, and the vertical axis represents H2 output. The lighter shaded box represents the feasible operating range for simultaneous coproduction of both products. Values outside this range are within the system's capacity but not feasible coproduction outputs (meaning either H2 or power can be produced at those levels but not both). These plots are a single trend line instead of a surface for single output systems and modes. Fig. S2–S8 (ESI†) report marginal costs of additional systems. Similarly, Fig. S9 and S10 (ESI†) use the cost surrogates to determine the mean electricity and H2 prices for concepts to break even (analysis from ref. 49).
2.4. Curating market data
To analyze a diverse set of price markets, we collected 61 annual locational marginal price (LMP) signals to represent historical, present, and future wholesale electricity market scenarios. Table S7 in the ESI† summarizes these datasets, including key statistics. Historical and present LMPs were gathered from publicly available datasets published by independent system operators (ISOs) and regional transmission organizations (RTOs) across the United States, including the California ISO (CAISO – NP15, ZP26, and SP15 regions); Electric Reliability Council of Texas (ERCOT – North, South, West, and Houston regions); ISO New England (ISO-NE); New York ISO (NYISO); Midwest ISO (MISO – Indiana, Louisiana, and Minnesota regions); and the Pennsylvania Jersey Maryland Interconnection West, and Southwest Power Pool (SPP – North and South regions).54 We used data from 2019 to represent historical pricing (pre-COVID-19 pandemic) and data from 2022 to represent more current pricing trends. Natural gas prices for each region were selected by taking the average spot price reported by the Energy Information Administration.55 These prices ranged from 2.39 to 10.79 $ MMBtu−1 across the different regions.
We selected three price projection methods to represent possible future market scenarios. These projected market scenarios contain low to high VRE penetration conditions, as well as low to high carbon tax levels. First, projections from Durvasulu and Cohen56 used the National Renewable Energy Laboratory's (NREL) Regional Energy Deployment System (ReEDS) capacity planning tool57 and PLEXOS energy market simulation platform58 to simulate five regions of the U.S. (CAISO, ERCOT, MISO-W, NYISO, and PJM-W) under CO2 taxes of 100 and 150 $ tonne−1. Carbon taxes were implemented as increasing trajectories from 0 $ tonne−1 to the target value to drive capacity toward low carbon systems, but not allowing for CCS deployment to ensure LMPs were not affected. These price scenarios assumed natural gas prices between 1 and 3 $ MMBtu−1, depending on the region modeled. This dataset will be referred to as “NREL (2035)”.
Second, we considered projections by Jenkins et al.,59 which utilized GenX60 and PowerGenome61 to simulate prices in a single integrated planning model representing the U. S. under different levels of VRE penetration and CO2 tax policies. All these projections assumed a natural gas price of 2.94 $ MMBtu−1. This dataset will be referred to as “Princeton (2030)”.
Lastly, we performed a series of independent production cost simulations for the ERCOT region covering 2033 through 2037, assuming a range of CO2 tax rates ranging from 0 to 250 $ tonne−1. This enabled the exercise of simulations for a 2035 study year with sufficient run-in and run-out periods to ensure that unit commitment constraints did not artificially impact the results. These projections assumed a natural gas price of 4.42 $ MMBtu−1. See Section S1 (ESI†) for a detailed explanation of these projections.62,63 This dataset will be referred to as “NETL (2035)”.
A summary of the market scenarios can be seen in Fig. 3. We present the mean LMP value (panel (a)), the natural gas price for that scenario (panel (b)), and the two multimodality tests that were performed (see Section S4, ESI†) to indicate if a market should be considered multimodal (panel (c)). If the market passed both multimodality tests (above 1.0 in Fig. 3, panel (c)), the market was considered to be multimodal. To further visualize the price signal data from some of the markets, 25 of the 61 markets (5 from each reference) are shown as histograms in ESI,† Fig. S16.
 |
| Fig. 3 Summary statistics of the market characteristics with respect to (a) mean LMP, (b) natural gas price, and (c) multimodality test statistics. For market signal modality, only those that have both statistics above the normalized threshold are considered to be multimodal (indicated with markers). Markets have been broken down by reference and are sorted by increasing mean LMP. | |
While H2 is expected to be a key energy carrier in decarbonized economies, H2 markets remain in their infancy, currently dominated by private bilateral contracts between suppliers and consumers. Instead of speculating on the structure of future H2 markets, we selected five static H2 selling prices between 1.00 and 3.00 $ kg−1 and performed a sensitivity analysis. In 2019, the International Energy Agency (IEA) reported the global average levelized cost of H2 (LCOH) to be from 1.20 to 2.10 $ kg−1 for production from natural gas (via steam methane reforming (SMR)) and 3.2 to 7.7 $ kg−1 for renewables-based electrolysis.64 More recently, an NETL report estimated LCOH of 1.64 $ kg−1 for SMR with CCS, 1.59 $ kg−1 for auto thermal reforming (ATR) with CCS, 3.09 $ kg−1 for coal gasification with CCS, and 3.64 $ kg−1 for a coal and biomass co-gasification system with CCS.65 This is part of a broader DOE goal to reduce the cost of clean H2 to 1.00 $ kg−1 by 2030.29 Additional work has highlighted paths to achieve this goal, including integrating clean H2 production with other energy systems.29,66 Based on these guidelines, we select a nominal H2 selling price of 2.00 $ kg−1 and analyze additional prices of 1.00, 1.50, 2.50, and 3.00 $ kg−1 for each of the 61 LMP signals. This results in 305 different market scenarios assessed for each of the six process concepts.
We note the nominal H2 selling pricing is the selling price after taking into account both the transportation and storage costs and possible tax credits. Thus, this analysis allows technologies to be evaluated by how much net profit is required from each kilogram of H2 produced to justify technology investment. Nevertheless, we note that H2 transport and storage costs can vary widely depending on the chosen technologies. For example, whereas salt cavern storage coupled to pipeline transport may add as little as $0.2 kg−1 to the cost of H2, further compressing the H2, storing it in a tank, and transporting it by truck can add more than ten times that amount.67 Thus, we chose not to embed these costs directly into the optimization formulation to facilitate interpretation of the results. The sensitivity of the results to variation in H2 transport and storage costs can be explored, however, by comparing results generated assuming different selling prices of H2. Alternatively, one can directly incorporate these costs into the optimization problem (Section 3.5). However, since we consider that H2 price is static for the reasons given above, incorporation of static transportation and storage costs will lead to consistent conclusions.
2.5. Optimizing process concept operation
Proper valuation of the process concepts requires computing the optimal time-varying operation that responds to the fluctuations in market conditions. For each of the six process concepts and 305 market scenarios (1830 total scenarios), we solve a mixed-integer nonlinear programming (MINLP) optimization problem with the following general structure:
Maximize
|
Electricity revenue + H2 revenue − system costs (algebraic surrogates) − carbon taxes |
Subject to
|
Profit model constraints (algebraic surrogates), operating mode integer constraints, startup and shutdown costs and logic, minimum up and down time constraints, feasible operating constraints (algebraic surrogates) |
The optimization degrees of freedom (decision variables) are the hourly power and H2 production rates (continuous variables) and the operating decisions (discrete variables). The objective is to maximize the system's profit over the annual planning horizon. The electricity and H2 revenues are calculated by multiplying the production rates by the respective hourly prices. The system costs are calculated using an algebraic surrogate model (see Section 3.3). We define a set of possible operating modes for each system, depending on the process concept's flexibility. Possible modes include off (no output), power only, H2 only, or coproduction (producing both power and H2 simultaneously). A set of operating mode integer constraints enforces operation within the feasible region of the active mode at each operational time step. The systems are also subject to startup and shutdown costs, as well as time limits for how long a system must be down before starting back up again or up before it can shut down again.68 For coproduction systems, we also include feasible operating region constraints, in the form of linear inequalities, that bound system power production rate as a function of H2 production rate. This optimization formulation assumes the new system is operating as a price-taker meaning the addition of the modeled system does not significantly affect the LMPs or energy system makeup.69 See Fig. 2 box C (middle) for an example hourly operation schedule.
A detailed description and statement of the mathematical formulation used to solve these market scenarios is included in ESI,† Section S2. Therein, the MINLP is presented in detail with descriptions of each variable, set, and equation, as well as the solution procedure and solver tools used to optimize each MINLP instance.
2.6. Comparing system performance
The output of the optimization model is the best annual profit and system operating schedule, which includes hourly electricity and H2 production rates, as well as the best time to switch between operating modes, startups, and shutdowns. Fig. 2 box C (middle) shows an example system output. The red line depicts the hourly dispatch schedule, and the blue dots represent the LMPs plotted against the hour. When the LMP (blue dots) are low, the system chooses to shut down, and when the LMP is higher (typically, above the system's marginal costs), power is produced at its maximum capacity. The red lines may dip below zero power output for coproduction systems or H2-only systems, indicating a net purchase of electricity from the grid. A supplemental spreadsheet provides the annualized profit optimization results for each system and scenario and several dashboard-like plots that automatically update as the data are filtered and sorted.
Using these data, we perform statistical analysis to compare the economic performance across process concepts under each scenario. Fig. 2 box D (bottom) depicts the percentage of scenarios for each case that result in a positive profit for all scenarios with a H2 selling price of 2.00 $ kg−1. Analyses like this plot allow us to observe which systems are most robust to variation in market conditions. Moreover, we perform multivariate linear regression to correlate the system's optimal profit to market features such as natural gas price, carbon taxes, H2 price, and LMP statistics (e.g., mean, median, multimodality,70–73 unimodality, etc.74,75). Ultimately, this analysis helps us distill 1830 annual operation optimization results into a concise set of key findings about the relative economic competitiveness of the process concepts across diverse market scenarios.
3. Results
3.1 SOFC outperforms existing NGCC systems
Fig. 4 shows optimization results for all 61 market price signals at the nominal H2 price of 2.00 $ kg−1 for each process concept. Price scenarios are plotted by name along the horizontal axis and are divided into groups by source (see Section 2.4). The vertical axis shows the optimal annual profit, H2 capacity factor, and power capacity factor calculated in our rigorous optimization framework. High capacity factors stem from operating procedures that keep the process on and, while on, running near maximum capacity for a large part of the operating year. Technologies without H2 (NGCC, and SOFC) or power production (SOEC) will have a capacity factor of 0 for the missing process output. Scenarios within each source are sorted by increasing annual profit for the SOFC process concept to make comparison more clear. Versions of these plots at all the H2 selling prices, are in the ESI† (Fig. S11–S14).
 |
| Fig. 4 Optimized annual profits for all analyzed electricity price signals at a nominal H2 selling price of 2.00 $ kg−1. Price signals are separated by source and, within each source, sorted by SOFC annual profit. Also, panels (b) and (c) display the H2 and power capacity factors for the given optimal operating solution, respectively. Names of market signals are the region followed by the carbon tax for future scenarios (i.e., ERCOT_100 from the NREL (2035) data set is the ERCOT region with a carbon tax of 100 $ tonne−1). | |
From Fig. 4, we see that the standalone SOFC concept always outperforms the standalone NGCC. Additionally, whereas the SOFC system achieves a positive profit in 52% of the examined market scenarios, the NGCC achieves a positive profit in only 13%. This is due to the anticipated higher efficiency and lower capital cost for SOFC-based power plants with CCS compared to NGCC-based power plants with CCS leading to a lower LCOE. Also, SOFC has a higher power capacity factor than NGCC in every scenario analyzed, many times exceeding a 40% capacity factor increase (see Fig. 4 and ESI,† Fig. S11–S14). This dramatic result indicates that SOFC-based power plants can produce power at profit under significantly more market conditions than NGCC-based power plants. Also, at the nominal H2 price, the SOFC-based integrated systems and SOEC-based hydrogen producing system have positive profits in nearly all scenarios (97%, 98%, and 74%, respectively).
3.2. H2 price sensitivity analysis
Fig. 5 summarizes all 1830 process concept/market scenario pairs as a panel of histograms. Each histogram contains the optimal annual profit data from all 61 market scenarios at a given H2 price. Each column represents a different H2 price, and each row represents a unique process concept. As H2 increases across a row, profit either stays the same if the system produces only power (i.e., NGCC and SOFC) or increases if the system can produce H2 (i.e., SOEC, rSOC, NGCC + SOEC, SOFC + SOEC) for a given market scenario. The flexibility is obvious for the rSOC and SOFC + SOEC process concepts as almost all LMP signals result in profitability with a H2 price at or above 2.00 $ kg−1 (Fig. 5, rows 3, 4 and 5). Also, the benefit of adapting a NGCC system with an additional SOEC unit becomes clear at higher H2 prices. Finally, the SOEC profitability is on average increasing, but the spread of profitability also grows, indicating that although a standalone SOEC is quite profitable in many scenarios at high H2 prices, there are still a handful scenarios where little to no profit, or even losses, can occur.
 |
| Fig. 5 Optimized annual profits for each process concept at each H2 price presented as histograms. The height of the bars indicates how many scenarios achieve a profit in the range indicated by the horizontal axis profit values. Each column has the same H2 price, and each row is the same technology. Break even lines (solid black) and mean profit over all scenarios for a given H2 price and process concept (dashed line) are shown for reference. As H2 price increases, system profitability increases for H2 production. | |
Table 1 summarizes these histograms in terms of the percentage of profitable market scenarios for each technology at each H2 price is available. This table highlights the large benefit of integrated systems (rSOC and SOFC + SOEC), even at low H2 prices. Even at the DOE target price of 1.00 $ kg−1 H2, rSOC and SOFC + SOEC IESs are profitable in 54% and 46% of scenarios, respectively. Also, Table 1 reiterates that moving from NGCC systems to SOFC systems (both with 97% carbon capture) would result in significantly more profitable scenarios (from 13% with NGCC to 54% with SOFC). Finally, integrating current NGCC systems with an SOEC (NGCC + SOEC) results in profitability only when H2 exceeds a price of 2.00 $ kg−1, indicating that simply adding an SOEC to an existing NGCC does not result in enough H2 production to outweigh the capital investment at low H2 prices.
Table 1 Percentage of LMP scenarios each process concept achieves positive annual profits aggregated by H2 selling price
Process concept |
1.00 $ kg−1 |
1.50 $ kg−1 |
2.00 $ kg−1 |
2.50 $ kg−1 |
3.00 $ kg−1 |
NGCC |
13% |
13% |
13% |
13% |
13% |
SOFC |
54% |
54% |
54% |
54% |
54% |
NGCC + SOEC |
8% |
11% |
16% |
62% |
80% |
rSOC |
54% |
77% |
97% |
100% |
100% |
SOFC + SOEC |
46% |
79% |
98% |
100% |
100% |
SOEC |
10% |
49% |
74% |
87% |
98% |
3.3. Integrated IESs exploit multimodal LMP signals to increase profit
The increasing prevalence of VRE sources is shifting the distribution of LMPs away from Gaussian and similar unimodal distributions toward multimodal distributions with periods of very low76 (near zero or negative) prices and extreme prices, resulting in LMP distributions with two local maxima rather than one. Fig. 2 box B (top right) contains a histogram depicting a multimodal price distribution of a LMP signal. In wholesale electricity markets, LMPs are determined by a market clearing process where projected demand is assessed and subsequently met in order of increasing cost.77,78 This means low (or zero) marginal cost power production, like VRE sources (e.g., solar and wind), are cleared before higher marginal cost resources (e.g., NGCCs). The highest system marginal cost selected determines the LMP for a specific period. Specifically, under high VRE penetration, periods of ultra-low LMPs stem from abundant, low-cost renewable supply and high LMPs stem from limited supply of wind and solar, leaving only more expensive options to meet demand.79 As states across the U.S. move toward achieving renewables portfolio standard targets, multimodal price signals could become more common. Thus, analyzing systems that thrive under these conditions is increasingly important.
In markets that are considered strongly multimodal (see Section S5, ESI†), there is a high concentration of low electricity prices and high electricity prices around distribution modes. The scenarios that exhibited multimodal behavior were future scenarios from the NREL (2035)56 and Princeton (2030)59 datasets where prediction of higher renewables penetration leads to periods of inexpensive, and sometimes negative, power costs (see Fig. 3, panel (c)) in addition to price spikes during peak hours. For market distributions visualization for 5 markets from each reference, see Fig. S16 (ESI†) (multimodal distributions in orange and unimodal in blue).
Fig. 6 shows that the integrated SOFC + SOEC IESs enjoy higher profits when compared with standalone process concepts (e.g., SOFC and SOEC, respectively) within the same price market. This benefit is amplified within multimodal price markets (Fig. 6, orange portion of bars). The benefit is amplified because the integrated system can take advantage of high electricity prices (produce power only) and low electricity prices (produce hydrogen only), whereas the standalone systems can only operate in a single product space. The conclusion of integrated systems benefiting from multimodality generalizes also to the NGCC + SOEC system (see Fig. S17, ESI†).
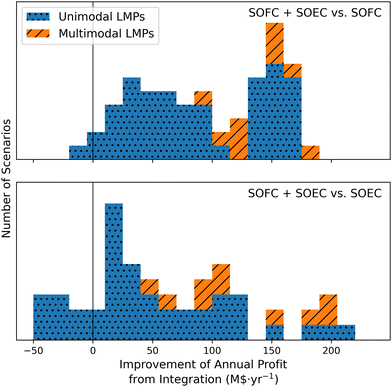 |
| Fig. 6 Here, the difference in optimized annual profits for standalone SOFC (top) and SOEC (bottom) process concepts when compared with the SOFC + SOEC integrated process concept is shown at a H2 price of 2.00 $ kg−1. The height of the bars indicates how many scenarios achieve a profit improvement through integration. Break even line is shown for reference (solid black). Multimodal scenarios are labeled differently to demonstrate that multimodal scenarios see large profit differences. | |
Fig. 7 shows the strong correlation of all process concepts with mean LMP, except SOEC. For the integrated technologies (right side of Fig. 7), the benefit from the multimodal scenarios (orange stars) is much greater than the benefit of multimodality within the standalone systems (left side of Fig. 7). This is because both peaks of a multimodal price signal can be taken advantage of by the integrated systems, i.e., H2 production at low energy prices and power production at high energy prices. The standalone technologies can only take advantage of one of these peaks.
 |
| Fig. 7 Optimized annual profits for each technology at an H2 price of 2.00 $ kg−1 plotted against the mean of the LMP signal distribution. The orange stars represent points that passed two multimodal tests and are considered multimodal, and the blue circles represents the markets that did not pass both tests. Higher mean LMP leads to higher profit in all process concepts except SOEC. | |
3.4. Linear regression predicts annual profit using market statistics across all scenarios
Finally, we train multivariate linear regression models to predict the maximum annual profit calculated from each optimization problem based on statistics of the input market data. Full regression coefficients (Table S5), feature correlation (Fig. S1), and methodological description (Sections S4 and S5) are available in the ESI.†Fig. 8 shows the parity between the regression model predictions (vertical axes) and rigorous market optimization results (horizontal axes). The high R2 values ranging from 0.88 to 0.99 and tight trending indicates a good fit. The SOEC (Fig. 8 bottom left) has a dip at lower profit values. This is explained because the lower bound (system always off) has total cost normalized at −0.82, resulting in a barrier in realizable losses even though the linear model predicts more losses.
 |
| Fig. 8 Parity between linear regression model and market optimization results for all six process concepts. Black stars represent a market that appears to be an outlier: the NETL scenario with carbon tax of 250 $ kg−1 which results in high volatility and extreme prices. | |
Examination of the linear regression coefficients further bolsters three key conclusions from the figures above. First, the rSOC process concept is much more sensitive to carbon tax than the other systems, as shown in regression coefficient Table S5 (ESI†). This is seen in Fig. 4 and in ESI,† Fig. S11–S14. At high hydrogen prices, electricity is more profitable to produce when the carbon tax is high, and at low hydrogen prices, electricity is more profitable to produce when carbon tax is low (note the power capacity factor, especially for the NREL (2035) reference). The integrated systems have less dependence because the feasible region to coproduce diminishes the benefit of single-product operation that the rSOC gains in these specific markets.
Second, the integrated process concepts (e.g., SOFC + SOEC and NGCC + SOEC) have a higher dependence on natural gas price. This can also be seen in Fig. 4 and ESI,† Fig. S11–S14, where the scenarios with high natural gas price (see Fig. 3, 2022 prices) show high variability in production capacity factor. The higher variability in H2 price for the 2022 scenarios leads to significantly higher dependence on natural gas price, which is a differentiating factor between the 2022 scenarios and other scenarios.
Lastly, H2 price has a lower impact on rSOC profit than the other H2-producing process concepts. This is explained by coproducing IESs (e.g., SOFC + SOEC and NGCC + SOEC) and H2 only IESs (e.g., SOEC standalone) have higher H2 capacity factors. The rSOC system has market conditions where producing only power is better than H2 because the integrated systems can coproduce H2 using self-produced power, which can exceed the marginal benefit of purchasing power from the grid. These three conclusions justify holistic analysis that includes optimizing individual systems alongside regression or other comparative studies to uncover subtle conclusions.
3.5. Power and H2 operational recommendations from marginal utility
From these results, we observe most units exhibit “on-and-off” dispatch. For a traditional thermal generator, it is either at full capacity (LMP is greater than the marginal cost of power) or off (LMP is less than the marginal cost of power). In Fig. S2–S8 (ESI†), we visualize the marginal cost for power and hydrogen for all the IESs. We hypothesize based on these data that it would be possible to extract simple heuristics that explain based on price when a unit should be producing power or hydrogen, coproduction, or neither, similar to the analysis in ref. 80.
4. Discussion
This analysis demonstrates the compelling advantages of co-generating electricity and H2 in a tightly coupled IES across diverse market scenarios. The economic performance of both NGCC and SOFC technologies is significantly enhanced through integration with H2 production, thereby creating a promising path toward the production of low-cost, low-carbon H2 that aligns with the DOE's hydrogen shot objective of achieving clean H2 for less than 1.00 $ kg−1 within a decade.29 The analysis reveals that the SOFC + SOEC system is profitable in 45% of the tested market scenarios with H2 capacity factors as high as 0.8 (>126 M kg year−1 for a 5.0 kg s−1 capacity H2 system). Similarly, the rSOC could profitably sell H2 at a price point of 1.00 $ kg−1 in 54% of the market scenarios, with H2 capacity factors as high as 0.68 (>107 M kg year−1 for a 5.0 kg s−1 H2 system). Moreover, one of the integrated systems that coproduce electricity and H2 – SOFC + SOEC or rSOC – is the top performer in 74% of the considered market scenarios. To summarize the entire analysis, Table 1 reports the percentage of LMP scenarios that achieve positive profits for each process concept and H2 selling price. These results clearly demonstrate the economic advantage of SOFC-IESs across a wide range of market scenarios. In addition, the IES concepts described herein enable higher annualized profits than similarly scaled SMR and ATR processes with CCS in scenarios with high natural gas prices (i.e., all 2022 scenarios), multimodal electricity pricing (i.e., all56 scenarios), or unimodal electricity pricing scenarios with high carbon taxes (e.g., all scenarios with a carbon tax of 100 $ tonne−1 and greater) as can be seen in the supplemental spreadsheet. Though beyond this study's scope, such systems’ value propositions could be enhanced even further through tax credits for carbon sequestration (45Q) and clean H2 production (45V).
Furthermore, the capabilities of our computational optimization framework have allowed us to directly compare a variety of process concepts and draw important conclusions. Along with these technology comparison results, we emphasize that this framework is generalizable beyond fuel cells, electrolysis, and even H2. The methodology we have employed, starting with detailed physics-based models (currently abundant in literature) or cost equations from any source and solving tractable market optimization problems, applies to many IES concepts with technologies including VRE sources, grid-scale storage, chemicals production, and residential heating and cooling. Based on our analysis, this work could be extended to explore the profitability of retrofit concepts instead of new construction concepts. Extensions to our market model may include analyzing faster timescales, such as real-time markets or ancillary service markets where prices are more volatile,44,81,82 and considering equipment degradation over longer time horizons.45,83,84 Also, we only consider static H2 selling prices (Section 2.4). One may improve this framework by including dynamic H2 pricing alongside dynamic transportation and storage costs. Finally, embedding this framework within analyses that include impact of the IES participating in the market (e.g., ref. 44) would improve the conclusions and prevent overestimating the benefit of emerging power generation and coproduction technologies.
5. Experimental procedures
5.1 Resource availability
5.1.1 Lead contact.
Further information and requests for resources and materials should be directed to and will be fulfilled by the lead contact, Prof. Alexander Dowling (adowling@nd.edu).
5.1.2 Materials availability.
This study did not generate new unique materials.
5.1.3 Data and code availability.
Description and sources of all LMP data can be found in Section 3.4 of this work. A more detailed description of how internal price projections were made is included in Section S1 (ESI†). Additional information about the IES concept process modeling can be found in ref. 49. IES concept models were generated in the IDAES-PSE open-source modeling platform51 version 2.0 which can be obtained for free on GitHub (https://github.com/IDAES/idaes-pse). Tools used for surrogate generation and market optimization modeling, including ALAMO version 2022.6.4, are available as part of the IDAES modeling platform. The market optimization model was built in Pyomo version 6.5.0 and solved using GAMS 41.3 with Dicopt 2. Visit https://github.com/IDAES/publications/ for all code used to generate the results presented in this work.
Disclaimer
This project was funded by the United States Department of Energy, National Energy Technology Laboratory, in part, through a site support contract. Neither the United States Government nor any agency thereof, nor any of their employees, nor the support contractor, nor any of their employees, makes any warranty, express or implied, or assumes any legal liability or responsibility for the accuracy, completeness, or usefulness of any information, apparatus, product, or process disclosed, or represents that its use would not infringe privately owned rights. Reference herein to any specific commercial product, process, or service by trade name, trademark, manufacturer, or otherwise does not necessarily constitute or imply its endorsement, recommendation, or favoring by the United States Government or any agency thereof. The views and opinions of authors expressed herein do not necessarily state or reflect those of the United States Government or any agency thereof.
Author contributions
D. J. Laky formal analysis, writing – original draft, writing – review & editing, visualization. N. P. Cortes conceptualization, methodology, software, formal analysis, investigation, writing – original draft, writing – review & editing, visualization. J. C. Eslick conceptualization, methodology, software, formal analysis, investigation, data curation, writing – original draft, writing – review & editing. A. Noring conceptualization, methodology, software, writing – review & editing. N. Susarla conceptualization, methodology, software, writing – review & editing. C. Okoli conceptualization, methodology, software, writing – review & editing. M. A. Zamarripa conceptualization, methodology, software, writing – review & editing. D. Allan conceptualization, methodology, software, writing – review & editing. J. H. Brewer methodology, software, data curation, resources, writing – original draft, writing – review & editing. A. K. S. Iyengar conceptualization, methodology, software, writing – review & editing. M. Wang formal analysis, software, writing – review & editing. A. P. Burgard conceptualization, methodology, writing – review & editing, supervision, project administration, funding acquisition. D. C. Miller conceptualization, project administration, funding acquisition, writing – review & editing. A. W. Dowling conceptualization, methodology, software, investigation, data curation, writing – original draft, writing – review & editing, visualization, supervision, project administration.
Data availability
A summary of all results used to generate the figures in this manuscript is included as an excel file entitled ‘optimal_summary_SOFC_results.xslx’. The data included are summarized input conditions (i.e., market signal used, technology being analyzed, carbon tax, etc.) and summarized outputs (i.e., annualized net profit). In addition, a compiled version of the inputs for the problem (i.e., energy system variables, LMP price signals for the 61 market scenarios, code for building and solving the optimization models) is available on GitHub: https://github.com/djlaky/idaes-publications/tree/cortes_joule_plotting/cortes_et_al_2023.
Conflicts of interest
The authors declare that they have no conflict of interest.
Acknowledgements
This work was conducted as part of the Institute for the Design of Advanced Energy Systems (IDAES) with support from the Simulation-Based Engineering and Reversible Solid Oxide Fuel Cells Programs within the U.S. Department of Energys Office of Fossil Energy and Carbon Management. We also thank Dr Radhakrishna Tumbalam Gooty and Dr Jaffer Ghouse at the National Energy Technology Laboratory for insightful feedback on this study.
References
- U. E. I. Administration, Annual Energy Outlook 2022, https://www.eia.gov/outlooks/aeo/ Accessed January 10, 2023 (March 2022).
-
J.-B. Rosenkranz, C. B. Martinez-Anido and B.-M. Hodge, Analyzing the impact of solar power on multi-hourly thermal generator ramping, in 2016 IEEE Green Technologies Conference (GreenTech), IEEE, 2016, pp. 153–158.
- California Independent System Operator, What the duck curve tells us about managing a green grid, https://www.caiso.com/documents/flexibleresourceshelprenewables_fastfacts.pdf Accessed January 10, 2023 ( 2016).
-
C. Kamath, Understanding wind ramp events through analysis of historical data, in IEEE PES T&D 2010, IEEE, 2010, pp. 1–6.
-
P. L. Denholm, R. M. Margolis and J. D. Eichman, Evaluating the Technical and Economic Performance of PV Plus Storage Power Plants, Tech. Rep. NREL/TP-6A20-68737, National Renewable Energy Laboratory, Golden, CO (United States) ( 2017) Search PubMed.
- Y. Wang, X. Wang, H. Yu, Y. Huang, H. Dong, C. Qi and N. Baptiste, Optimal design of integrated energy system considering economics, autonomy and carbon emissions, J. Cleaner Prod., 2019, 225, 563–578 CrossRef.
- M. Aminudin, S. Kamarudin, B. Lim, E. Majilan, M. Masdar and N. Shaari, An overview: Current progress on hydrogen fuel cell vehicles, Int. J. Hydrogen Energy, 2023, 48(11), 4371–4388 CrossRef CAS.
- S. Griffiths, B. K. Sovacool, J. Kim, M. Bazilian and J. M. Uratani, Industrial decarbonization via hydrogen: A critical and systematic review of developments, socio-technical systems and policy options, Energy Res. Soc. Sci., 2021, 80, 102208 CrossRef.
- M. Hirscher, V. A. Yartys, M. Baricco, J. B. von Colbe, D. Blanchard, R. C. Bowman Jr, D. P. Broom, C. E. Buckley, F. Chang, P. Chen, Y. W. Cho, J.-C. Crivello, F. Cuevas, W. I. David, P. E. de Jongh, R. V. Denys, M. Dornheim, M. Felderhoff, Y. Filinchuk, G. E. Froudakis, D. M. Grant, E. M. Gray, B. C. Hauback, T. He, T. D. Humphries, T. R. Jensen, S. Kim, Y. Kojima, M. Latroche, H.-W. Li, M. V. Lototskyy, J. W. Makepeace, K. T. Møller, L. Naheed, P. Ngene, D. Nóreus, M. M. Nygård, S.-I. Orimo, M. Paskevicius, L. Pasquini, D. B. Ravnsbæk, M. V. Sofianos, T. J. Udovic, T. Vegge, G. S. Walker, C. J. Webb, C. Weidenthaler and C. Zlotea, Materials for hydrogen-based energy storage-past, recent progress and future outlook, J. Alloys Compd., 2020, 827, 153548 CrossRef CAS.
- A. Chapman, K. Itaoka, K. Hirose, F. T. Davidson, K. Nagasawa, A. C. Lloyd, M. E. Webber, Z. Kurban, S. Managi, T. Tamaki, M. C. Lewis, R. E. Hebner and Y. Fjii, A review of four case studies assessing the potential for hydrogen penetration of the future energy system, Int. J. Hydrogen Energy, 2019, 44(13), 6371–6382 CrossRef.
- Hydrogen Council, How hydrogen empowers the energy transition, https://hydrogencouncil.com/en/study-how-hydrogen-empowers/ ( 2017).
- G. Nicoletti, N. Arcuri, G. Nicoletti and R. Bruno, A technical and environmental comparison between hydrogen and some fossil fuels, Energy Convers. Manage., 2015, 89, 205–213 CrossRef.
- P. Colombo, A. Saeedmanesh, M. Santarelli and J. Brouwer, Dynamic dispatch of solid oxide electrolysis system for high renewable energy penetration in a microgrid, Energy Convers. Manage., 2020, 204, 112322 CrossRef.
- E. F. Bødal, D. Mallapragada, A. Botterud and M. Korpås, Decarbonization synergies from joint planning of electricity and hydrogen production: a Texas case study, Int. J. Hydrogen Energy, 2020, 45(58), 32899–32915 CrossRef.
- N. Q. Minh, Solid oxide fuel cell technology—features and applications, Solid State Ionics, 2004, 174(1–4), 271–277 CrossRef CAS.
- S. Campanari, L. Mastropasqua, M. Gazzani, P. Chiesa and M. C. Romano, Predicting the ultimate potential of natural gas SOFC power cycles with CO2 capture-Part A: Methodology and reference cases, J. Power Sources, 2016, 324, 598–614 CrossRef CAS.
- P. Preuster, Q. Fang, R. Peters, R. Deja, L. Blum, D. Stolten and P. Wasserscheid,
et al., Solid oxide fuel cell operating on liquid organic hydrogen carrier-based hydrogen-making full use of heat integration potentials, Int. J. Hydrogen Energy, 2018, 43(3), 1758–1768 CrossRef CAS.
- M. C. Williams, S. D. Vora and G. Jesionowski, Worldwide status of solid oxide fuel cell technology, ECS Trans., 2020, 96(1), 1 CrossRef CAS.
- J. Nease and T. A. Adams, Systems for peaking power with 100% CO2 capture by integration of solid oxide fuel cells with compressed air energy storage, J. Power Sources, 2013, 228, 281–293, DOI:10.1016/j.jpowsour.2012.11.087.
- I. Sreedhar, B. Agarwal, P. Goyal and S. A. Singh, Recent advances in material and performance aspects of solid oxide fuel cells, J. Electroanal. Chem., 2019, 848, 113315 CrossRef CAS.
- C. Song, Fuel processing for low-temperature and high-temperature fuel cells challenges, and opportunities for sustainable development in the 21st century, Catal. Today, 2002, 77(1–2), 17–49, DOI:10.1016/s0920-5861(02)00231-6.
- T. Ferriday and P. H. Middleton, Alkaline fuel cell technology – a review, Int. J. Hydrogen Energy, 2021, 46(35), 18489–18510, DOI:10.1016/j.ijhydene.2021.02.203.
- M. M. Tellez-Cruz, J. Escorihuela, O. Solorza-Feria and V. Compañ, Proton exchange membrane fuel cells (pemfcs): Advances and challenges, Polymers, 2021, 13(18), 3064, DOI:10.3390/polym13183064.
- K. Jiao, J. Xuan, Q. Du, Z. Bao, B. Xie, B. Wang, Y. Zhao, L. Fan, H. Wang and Z. Hou,
et al., Designing the next generation of proton-exchange membrane fuel cells, Nature, 2021, 595(7867), 361–369, DOI:10.1038/s41586-021-03482-7.
- S. Campanari, L. Mastropasqua, M. Gazzani, P. Chiesa and M. C. Romano, Predicting the ultimate potential of natural gas sofc power cycles with CO2 capture – part b: Applications, J. Power Sources, 2016, 325, 194–208, DOI:10.1016/j.jpowsour.2016.05.134.
- M. Singh, D. Zappa and E. Comini, Solid oxide fuel cell: Decade of progress, future perspectives and challenges, Int. J. Hydrogen Energy, 2021, 46(54), 27643–27674, DOI:10.1016/j.ijhydene.2021.06.020.
- A. Hauch, R. Küngas, P. Blennow, A. B. Hansen, J. B. Hansen, B. V. Mathiesen and M. B. Mogensen, Recent advances in solid oxide cell technology for electrolysis, Science, 2020, 370(6513), eaba6118 CrossRef CAS PubMed.
- Clean Air Task Force, Solid Oxide Electrolysis: A Technology Status Assessment, https://www.catf.us/resource/solid-oxide-electrolysis-technology-status-assessment/.
-
H. N. Dinh, Hydrogen production and hydrogen shot: Options for producing low-carbon hydrogen at scale, Tech. Rep. NREL/PR-5900-82933, National Renewable Energy Laboratory, Golden, CO (United States) (6 2022) Search PubMed.
- D. H. Chung, E. J. Graham, B. A. Paren, L. Schofield, Y. Shao-Horn and D. S. Mallapragada, Design space for pem electrolysis for cost-effective H2 production using grid electricity, Ind. Eng. Chem. Res., 2024, 63(16), 7258–7270 CAS.
- A. Hawkes and M. Leach, Solid oxide fuel cell systems for residential micro-combined heat and power in the UK: Key economic drivers, J. Power Sources, 2005, 149, 72–83 CrossRef CAS.
- A. Behzadi, A. Habibollahzade, V. Zare and M. Ashjaee, Multi-objective optimization of a hybrid biomass-based SOFC/GT/double effect absorption chiller/RO desalination system with CO2 recycle, Energy Convers. Manage., 2019, 181, 302–318 CrossRef CAS.
- M. Meratizaman, S. Monadizadeh and M. Amidpour, Techno-economic assessment of high efficient energy production (SOFC-GT) for residential application from natural gas, J. Nat. Gas Sci. Eng., 2014, 21, 118–133 CrossRef.
- A. Habibollahzade, E. Gholamian, E. Houshfar and A. Behzadi, Multi-objective optimization of biomass-based solid oxide fuel cell integrated with stirling engine and electrolyzer, Energy Convers. Manage., 2018, 171, 1116–1133 CrossRef CAS.
- M. A. Haghghi, S. G. Holagh, A. Chitsaz and K. Parham, Thermodynamic assessment of a novel multi-generation solid oxide fuel cell-based system for production of electrical power, cooling, fresh water, and hydrogen, Energy Convers. Manage., 2019, 197, 111895 CrossRef.
- T. Elmer, M. Worall, S. Wu and S. B. Riffat, Emission and economic performance assessment of a solid oxide fuel cell micro-combined heat and power system in a domestic building, Appl. Therm. Eng., 2015, 90, 1082–1089 CrossRef.
- M. Gandiglio, A. Lanzini, P. Leone, M. Santarelli and R. Borchiellini, Thermoeconomic analysis of large solid oxide fuel cell plants: Atmospheric vs. pressurized performance, Energy, 2013, 55, 142–155 CrossRef CAS.
- M. Naeini, J. S. Cotton and T. A. Adams, Economically optimal sizing and operation strategy for solid oxide fuel cells to effectively manage long-term degradation, Ind. Eng. Chem. Res., 2021, 60(47), 17128–17142 CrossRef CAS.
- W. Shen, X. Chen, J. Qiu, J. A. Hayward, S. Sayeef, P. Osman, K. Meng and Z. Y. Dong, A comprehensive review of variable renewable energy levelized cost of electricity, Renewable Sustainable Energy Rev., 2020, 133, 110301 CrossRef.
- U. Nissen and N. Harfst, Shortcomings of the traditional “levelized cost of energy”[LCOE] for the determination of grid parity, Energy, 2019, 171, 1009–1016 CrossRef.
- E. Veronese, G. Manzolini and D. Moser, Improving the traditional levelized cost of electricity approach by including the integration costs in the techno-economic evaluation of future photovoltaic plants, Int. J. Energy Res., 2021, 45(6), 9252–9269 CrossRef.
- A. W. Dowling, T. Zheng and V. M. Zavala, Economic assessment of concentrated solar power technologies: A review, Renewable Sustainable Energy Rev., 2017, 72, 1019–1032 CrossRef.
- D. J. Arent, S. M. Bragg-Sitton, D. C. Miller, T. J. Tarka, J. A. Engel-Cox, R. D. Boardman, P. C. Balash, M. F. Ruth, J. Cox and D. J. Garfield, Multi-input, multi-output hybrid energy systems, Joule, 2021, 5(1), 47–58 CrossRef.
- X. Gao, B. Knueven, J. D. Siirola, D. C. Miller and A. W. Dowling, Multiscale simulation of integrated energy system and electricity market interactions, Appl. Energy, 2022, 316, 119017 CrossRef.
- F. Sorourifar, V. M. Zavala and A. W. Dowling, Integrated multiscale design, market participation, and replacement strategies for battery energy storage systems, IEEE Trans. Sustainable Energy, 2018, 11(1), 84–92 Search PubMed.
- G. Glenk and S. Reichelstein, Reversible power-to-gas systems for energy conversion and storage, Nat. Commun., 2022, 13, 2010, DOI:10.1038/s41467-022-29520-0.
- G. Glenk, P. Holler and S. Reichelstein, Advances in power-to-gas technologies: cost and conversion efficiency, Energy Environ. Sci., 2023, 16, 6058–6070, 10.1039/D3EE01208E.
-
A. Iyengar, A. Noring, J. MacKay, D. Keairns and G. Hackett, Techno-economic analysis of natural gas fuel cell plant configurations, Tech. Rep. DOE/NETL-2022/3259, National Energy Technology Laboratory, Pittsburgh, PA, Morgantown, WV, Albany, OR (United States) ( 2022) Search PubMed.
-
J. C. Eslick, A. Noring, N. Susarla, C. Okoli, D. Allan, M. Wang, J. Ma, M. Zamarripa, A. Iyengar and A. P. Burgard, Technoeconomic evaluation of solid oxide fuel cell hydrogen-electricity co-generation concepts, Tech. Rep. DOE/NETL-2023/4322, National Energy Technology Laboratory, Pittsburgh, PA (United States) (March 2023) Search PubMed.
-
T. Schmitt, S. Leptinsky, M. Turner, A. Zoelle, C. White, S. Hughes, S. Homsy, M. Woods, H. Hoffman, T. Shultz and R. E. James, III, Cost and performance baseline for fossil energy plants volume 1: Bituminous coal and natural gas to electricity, Tech. Rep. DOE/NETL-2023/4320, National Energy Technology Laboratory, Pittsburgh, PA, Morgantown, WV, Albany, OR (United States) ( 2022) Search PubMed.
- A. Lee, J. H. Ghouse, J. C. Eslick, C. D. Laird, J. D. Siirola, M. A. Zamarripa, D. Gunter, J. H. Shinn, A. W. Dowling and D. Bhattacharyya,
et al., The IDAES process modeling framework and model library—Flexibility for process simulation and optimization, J. Adv. Manuf. Process., 2021, 3(3), e10095 CrossRef.
- A. Cozad, N. V. Sahinidis and D. C. Miller, Learning surrogate models for simulation-based optimization, AIChE J., 2014, 60(6), 2211–2227 CrossRef CAS.
-
J. Theis, Quality Guidelines for Energy Systems Studies: Cost Estimation Methodology for NETL Assessments of Power Plant Performance, Tech. Rep. NETL-PUB-22580, National Energy Technology Laboratory, Pittsburgh, PA, Morgantown, WV, Albany, OR (United States) (February 2021) Search PubMed.
- Hitachi Energy – Velocity Suite, ISO Real Time Day Ahead LMP Pricing – All Price Nodes Hourly (Non-Optimized), website, https://www.hitachienergy.com/us/en/products-and-solutions/energy-portfolio-management/market-intelligence-services/velocity-suite Accessed April 10, 2023 ( 2023).
- U.S. Energy Information Administration, Natural gas – data, Website, https://www.eia.gov/dnav/ng/ng_pri_sum_dcu_nus_m.htm Accessed January 10, 2023.
-
S. Cohen and V. Durvasulu, NREL Price Series Developed for the ARPA-E FLECCS Program, Tech. Rep. NREL/TP-6A20-78195, National Renewable Energy Laboratory – Data (NREL-DATA), Golden, CO (United States) (December 2021) Search PubMed.
-
J. Ho, J. Becker, M. Brown, P. Brown, I. Chernyakhovskiy, S. Cohen, W. Cole, S. Corcoran, K. Eurek, W. Frazier, P. Gagnon, N. Gates, D. Greer, P. Jadun, S. Khanal, S. Machen, M. Macmillan, T. Mai, M. Mowers, C. Murphy, A. Rose, A. Schleifer, B. Sergi, D. Steinberg, Y. Sun and E. Zhou, Regional energy deployment system (reeds) model documentation (version 2020), Tech. Rep. NREL/TP-6A20-78195, National Renewable Energy Laboratory, Golden, CO (United States) (6 2021) Search PubMed.
- Energy Exemplar, Plexos, website, https://www.energyexemplar.com/plexos Accessed April 10, 2023.
-
J. D. Jenkins, S. Chakrabarti, F. Cheng and N. Patankar, Summary Report of the GenX and PowerGenome runs for generating Price Series (for ARPA-E FLECCS Project) DOI:10.5281/zenodo.5765798 (Dec. 2021).
-
J. D. Jenkins and N. A. Sepulveda, Enhanced Design for a Changing Electricity Landscape: The GenX Configurable Electricity Resource Capacity Expansion Model, Tech. Rep. MITEI-WP-2017-10, Massachusets Institute of Technology Energy Initiative (MITei), Cambridge, MA (November 2017) Search PubMed.
- Massachusetts Institute of Technology (MIT), PowerGenome, website, https://github.com/PowerGenome/PowerGenome Accessed April 10, 2023.
-
J. Brewer and K. Labarbara, Quality Guideline for Energy Systems Studies: Guideline for Developing Security Constrained Unit Commitment and Dispatch Models for NETL Studies, Tech. Rep. DOE/NETL-2019/1905, National Energy Technology Laboratory, Morgantown, WV ( 2021) Search PubMed.
- Electric Reliability Council of Texas, Report on the Capacity, Demand and Reserves in the ERCOT Region, 2022–2031, https://www.ercot.com/files/docs/2021/12/29/CapacityDemandandReservesReport_December2021.pdf Accessed January 10, 2023.
- International Energy Agency, Global average levelised cost of hydrogen production by energy source and technology, 2019 and 2050 ( 2022).
-
E. Lewis, S. McNaul, M. Jamieson, M. S. Henriksen, H. S. Matthews, L. Walsh, J. Grove, T. Shultz, T. J. Skone and R. Stevens, Comparison of commercial, state-of-the-art, fossil-based hydrogen production technologies, Tech. Rep. DOE/NETL-2022/3241, National Energy Technology
Laboratory, Pittsburgh, PA, Morgantown, WV, Albany, OR (United States) (April 2022) Search PubMed.
-
S. McNaul, C. White, B. Wallace, T. Warner, H. S. Matthews, J. N. Ma, M. Ramezan, E. Lewis, D. Morgan, M. Henriksen, J. White, C. Munson, R. Stevens and T. Shultz, Strategies for Achieving the DOE Hydrogen Shot Goal: Thermal Conversion Approaches, Tech. Rep. DOE/NETL-2023/3824, National Energy Technology Laboratory, Pittsburgh, PA, Morgantown, WV, Albany, OR (United States) (January 2023) Search PubMed.
- U. D. of Energy, Pathways to commercial liftoff: Clean hydrogen, Tech. rep., US Department of Energy ( 2023).
-
D. Rajan and S. Takriti, Minimum Up/Down Polytopes of the Unit Commitment Problem with Start-Up Costs, Tech. Rep. W0506-050, IBM Research Division, Yorktown Heights, NY (United States) (06 2005). https://dominoweb.draco.res.ibm.com/cdcb02a7c809d89e8525702300502ac0.html Search PubMed.
- J. Jalving, J. Ghouse, N. Cortes, X. Gao, B. Knueven, D. Agi, S. Martin, X. Chen, D. Guittet, R. Tumbalam-Gooty, L. Bianchi, K. Beattie, D. Gunter, J. D. Siirola, D. C. Miller and A. W. Dowling, Beyond price taker: Conceptual design and optimization of integrated energy systems using machine learning market surrogates, Appl. Energy, 2023, 351, 121767 CrossRef.
- I. Eisenberger, Genesis of bimodal distributions, Technometrics, 1964, 6(4), 357–363 CrossRef.
- T. R. Knapp, Bimodality revisited, J. Mod. Appl. Stat. Methods, 2007, 6(1), 3 Search PubMed.
- J. A. Hartigan and P. M. Hartigan, The dip test of unimodality, Ann. Stat., 1985, 70–84 Search PubMed.
- R. Pfister, K. A. Schwarz, M. Janczyk, R. Dale and J. B. Freeman, Good things peak in pairs: a note on the bimodality coefficient, Front. Psychol., 2013, 4, 700 Search PubMed.
-
S. Institute, SAS/STAT User's Guide: version 6, SAS Institute Incorporated, 1990, vol. 2 Search PubMed.
- J. B. Freeman and R. Dale, Assessing bimodality to detect the presence of a dual cognitive process, Behav. Res. Methods, 2013, 45, 83–97 CrossRef PubMed.
- A. W. Dowling and V. M. Zavala, Economic opportunities for industrial systems from frequency regulation markets, Comput. Chem. Eng., 2018, 114, 254–264, DOI:10.1016/j.compchemeng.2017.09.018 , fOCAPO/CPC 2017.
- K. Imran and I. Kockar, A technical comparison of wholesale electricity markets in north america and europe, Electr. Power Syst. Res., 2014, 108, 59–67, DOI:10.1016/j.epsr.2013.10.016.
- A. W. Dowling, R. Kumar and V. M. Zavala, A multi-scale optimization framework for electricity market participation, Appl. Energy, 2017, 190, 147–164, DOI:10.1016/j.apenergy.2016.12.081.
-
R. B. Hytowitz, B. Frew, G. Stephen, E. Ela, N. Singhal, A. Bloom and J. Lau, Impacts of price formation efforts considering high renewable penetration levels and system resource adequacy targets, Tech. Rep. NREL/TP-6A20-74230, National Renewable Energy Laboratory, Golden, CO (United States) ( 2020) Search PubMed.
-
M. Watson, A. Veronese da Silva, P. G. Machado, C. D. O. Ribeiro, C. A. Oller de Nascimento and A. Dowling, The case for bio-jet fuel from bioethanol in brazil: An optimization-based analysis using historical market data, ChemRxiv, 2024, preprint. DOI:10.26434/chemrxiv-2024-j5l9x.
- A. W. Dowling, R. Kumar and V. M. Zavala, A multi-scale optimization framework for electricity market participation, Appl. Energy, 2017, 190, 147–164 CrossRef.
- A. W. Dowling and V. M. Zavala, Economic opportunities for industrial systems from frequency regulation markets, Comput. Chem. Eng., 2018, 114, 254–264 CrossRef CAS.
- H. Lai, N. F. Harun, D. Tucker and T. A. Adams II, Design and eco-technoeconomic analyses of sofc/gt hybrid systems accounting for long-term degradation effects, Int. J. Hydrogen Energy, 2021, 46(7), 5612–5629 CrossRef CAS.
- M. Naeini, J. S. Cotton and T. A. Adams II, Dynamic life cycle assessment of solid oxide fuel cell system considering long-term degradation effects, Energy Convers. Manage., 2022, 255, 115336 CrossRef CAS.
Footnotes |
† Electronic supplementary information (ESI) available. See DOI: https://doi.org/10.1039/d4ee02394c |
‡ These authors contributed equally to this work. |
§ Current address: Carrier Global Corporation, Palm Beach Gardens, FL 33418. |
¶ Current address: Air Products, Allentown, Pennsylvania, 18106. |
|| Current address: Procter & Gamble, Cincinnati, OH 45202. |
** Current address: OLI Systems, Inc., Parsippany, NJ 07054. |
|
This journal is © The Royal Society of Chemistry 2024 |
Click here to see how this site uses Cookies. View our privacy policy here.