DOI:
10.1039/D3CS00575E
(Review Article)
Chem. Soc. Rev., 2024,
53, 9059-9132
Computer-aided nanodrug discovery: recent progress and future prospects
Received
21st June 2024
First published on 16th August 2024
Abstract
Nanodrugs, which utilise nanomaterials in disease prevention and therapy, have attracted considerable interest since their initial conceptualisation in the 1990s. Substantial efforts have been made to develop nanodrugs for overcoming the limitations of conventional drugs, such as low targeting efficacy, high dosage and toxicity, and potential drug resistance. Despite the significant progress that has been made in nanodrug discovery, the precise design or screening of nanomaterials with desired biomedical functions prior to experimentation remains a significant challenge. This is particularly the case with regard to personalised precision nanodrugs, which require the simultaneous optimisation of the structures, compositions, and surface functionalities of nanodrugs. The development of powerful computer clusters and algorithms has made it possible to overcome this challenge through in silico methods, which provide a comprehensive understanding of the medical functions of nanodrugs in relation to their physicochemical properties. In addition, machine learning techniques have been widely employed in nanodrug research, significantly accelerating the understanding of bio–nano interactions and the development of nanodrugs. This review will present a summary of the computational advances in nanodrug discovery, focusing on the understanding of how the key interfacial interactions, namely, surface adsorption, supramolecular recognition, surface catalysis, and chemical conversion, affect the therapeutic efficacy of nanodrugs. Furthermore, this review will discuss the challenges and opportunities in computer-aided nanodrug discovery, with particular emphasis on the integrated “computation + machine learning + experimentation” strategy that can potentially accelerate the discovery of precision nanodrugs.
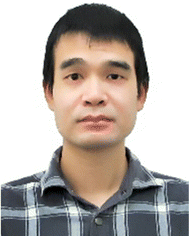
Jia-Jia Zheng
| Jia-Jia Zheng received his PhD in Materials Science and Engineering from Xi’an Jiaotong University in 2015. After working as a postdoctoral researcher at Kyoto University, he was appointed as an Assistant Professor at the same university in 2018. In 2020, he moved to the National Center for Nanoscience and Technology of China and became an Associate Professor. His research focuses on bio–nano interactions and catalytic transformation of bioactive molecules by nanomaterials. |
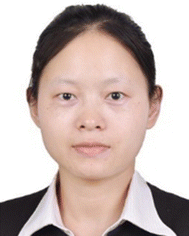
Qiao-Zhi Li
| Qiao-Zhi Li received her PhD from Xi’an Jiaotong University in 2018. After graduation, she worked as a postdoctoral researcher at the Fukui Institute for Fundamental Chemistry, Kyoto University. She is currently a postdoctoral researcher at the National Center for Nanoscience and Technology of China. Her research interests include the computer-aided design of catalysis-based nanodrugs and the development of activity prediction models for nanomaterial-catalysed hydrolysis of bioactive molecules. |
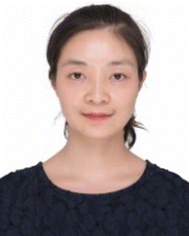
Zhenzhen Wang
| Zhenzhen Wang received her PhD from Institute of High Energy Physics, Chinese Academy of Sciences in 2019. She was a postdoctoral fellow (2019–2022) at National Center for Nanoscience and Technology (NCNST), and became an associate professor at NCNST in 2022. Her research interests include electronic structure, catalytic mechanism, and toxic mechanism of nanomaterials using first-principle calculations and molecular dynamics methods. |
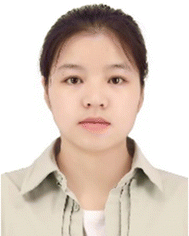
Xiaoli Wang
| Xiaoli Wang received her Master's degree from JiangXi Normal University in 2021. After graduation, she worked as a research assistant at Jiangxi Normal University in 2022. She is currently a PhD student at the National Center for Nanoscience and Technology of China under the supervision of Prof. Xingfa Gao. Her research focuses on the computer-aided design of nanozymes and the construction of energy model for noble metal-catalysed oxidation reaction. |
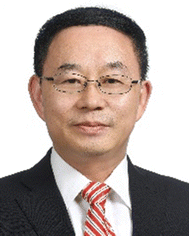
Yuliang Zhao
| Yuliang Zhao received his PhD at Tokyo Metropolitan University and worked at RIKEN (Japan). He moved to Chinese Academy of Sciences and became a full professor in 2001. His research interests include nanotoxicology, cancer nanotechnology, and radiochemistry. He is serving as Associated Editor or Editorial Broad Member for 8 international SCI journals in USA and Europe. |
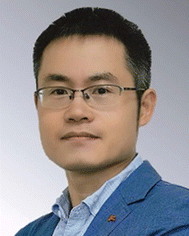
Xingfa Gao
| Xingfa Gao received his PhD from the Institute of High Energy Physics, Chinese Academy of Science (P. R. China) in 2006. Currently, he is a professor and principal investigator at National Center for Nanoscience and Technology (P. R. China). His research interests include theory and computer-aided design of nanodrugs. |
1. Introduction
Nanomaterials have shown great potential in medical applications. To date, a variety of nanomaterials have been employed in disease prevention and therapy.1 These nanomaterials can be used as either therapeutic agents or carriers of conventional drugs, both of which can be referred to as nanodrugs. Nanodrugs have demonstrated great promise in enhancing therapeutic efficacy and reducing drug dosage, drug toxicity, and side effects in comparison to conventional small molecule drugs. Furthermore, the designable structures, compositions, and surface functionalities of nanodrugs allow for the simultaneous optimisation of their therapeutic performance. Therefore, nanodrugs have attracted sustained attention over the past few decades.2–8
The most extensively studied nanodrugs are nanomaterials-based drug delivery systems, where nanomaterials act as carriers to deliver conventional drug cargos to the desired diseased tissues, typically tumours.9,10 A few such nanodrugs have been approved for clinical applications by the Food and Drug Administration.11,12 Nanomaterials can also directly participate in disease diagnosis and therapy like conventional drugs. These nanodrugs usually take advantage of the unique physicochemical properties of nanomaterials, such as their catalytic ability. The conceptualisation of “nanozyme”13 and “catalytic therapy”14 has led to the emergence of catalysis-based therapy as a branch of nanodrugs. Despite the significant progress that has been made, the clinical applications of nanodrugs remain very limited,15 likely due to a lack of clinician involvement in nanodrug development.16 This is particularly the case with regard to personalised precision nanodrugs, where the therapeutics can be suggested based on the patient's individual characteristics. The heterogeneous environments across patient populations and diseases present a significant challenge for the development of precision therapeutics.17 Future precision therapeutics requires the development of smart integrated nanodrugs with multiple medical functions, including efficient therapy, selective recognition, targeted delivery, efficient cellular uptake, a long circulation time, low toxicity, and easy degradation. To achieve this goal, it is essential to optimise the physicochemical properties of nanomaterials, including compositions, structures, surface functionalities (e.g. protecting and/or targeting moieties) and responsiveness,17 which collectively determine the medical functions of nanodrugs.
However, the large number of available nanomaterials and the complex bio–nano interactions make it extremely challenging to precisely design or screen nanostructures with desired therapeutic functions prior to experimentation. In this regard, a comprehensive understanding of how the physicochemical properties of nanomaterials influence their medical functions, i.e. the structure–activity relationships of nanodrugs, is of great importance. Theoretical and computational studies have been extensively conducted for nanodrug discovery, due to the appearance of powerful computer clusters and algorithms.18–20 From a theoretical point of view, there are four key interfacial interactions that may determine the medical functions of nanodrugs, namely, surface adsorption, supramolecular recognition, surface catalysis, and chemical conversion. As shown in Fig. 1, surface adsorption involves the interactions of nanomaterials with conventional molecular drugs, functional moieties, and proteins. The supramolecular recognition is typically represented by the interactions between functional moieties anchored on nanosurfaces and receptors on cell membranes. For comparison, the interfacial cell–nanomaterial interactions associated with the passive cellular uptake can also be considered as the supramolecular recognition. Surface catalysis represents the catalytic regulation of small bioactive molecules/species, such as reactive oxygen species, amino acids and phosphate toxins. Chemical conversion represents the structural transformation of nanodrugs, including the assembly/aggregation, deformation, ion release, and degradation/disassembly in physiological/pathological conditions. These interfacial interactions collectively affect the fate and cytotoxicity, the specificity to pathological locations, the ability to cross cell membranes and biological barriers, the intelligent release of active constituents, the pharmacokinetics, and thus the overall therapeutic efficacy of nanodrugs.
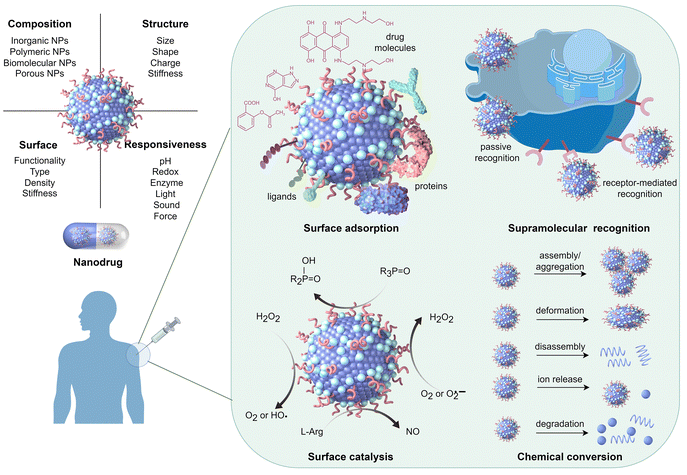 |
| Fig. 1 Physicochemical properties of nanomaterials that determine various interfacial interactions related to the medical functions of nanodrugs. Drawn by Figdraw (https://www.figdraw.com/#/). | |
Multi-scale computational approaches have been employed to investigate the interfacial interactions associated with the various functions of nanodrugs. For example, density functional theory (DFT) methods can be applied to investigate surface adsorption, surface catalysis, and chemical conversion, uncovering key factors that influence the interaction of nanomaterials with drug molecules or functional moieties, catalytic activity, dissolution ability, and many others related to therapeutic performance.20–23 However, DFT methods are not applicable to investigate the supramolecular recognition and several surface adsorption phenomena, such as protein adsorption onto nanosurfaces, which are usually investigated by all-atoms or coarse-grained molecular (AAMD/CGMD) dynamics simulations.18,19 In recent years, machine learning (ML) techniques have been widely employed to advance nanodrug discovery, but current studies are mainly experimental data-driven.24,25 With an understanding of the key interactions that govern the medical functions of nanomaterials, computation-driven machine learning has emerged as a promising technique for accelerating nanodrug discovery.26,27 Numerous reviews have been published, with a predominant focus on one or a few specific medical functions of nanomaterials.28–32 However, the interfacial interactions between nanodrugs and biological entities related to their various medical functions have yet to be categorised, which should be of crucial importance for the future precision nanodrug discovery.
This review addresses the advances in computational nanodrug discovery, with a particular focus on the future computer-aided design of intelligent precision nanodrugs by simultaneous optimisation of various physicochemical properties of nanomaterials. We first summarise recent computational progress on four categories of nanodrugs, based on surface adsorption, supramolecular recognition, surface catalysis, and chemical conversion, respectively. The influence of the interfacial interactions on the therapeutic efficacy of nanodrugs is specifically focused on, with little attention paid to the cytotoxicity that has been well reviewed.33 Further, we summarise the machine learning-assisted discovery of nanodrugs based on experimental and/or computational data. Then, we discuss the necessity to combine traditional computational methods and emerging machine learning techniques in the design and screening of nanodrugs. The future perspectives and challenges associated with computer-aided nanodrug discovery are presented. This review is expected to provide an in-depth understanding of the underlying mechanisms associated with the medical functions of nanodrugs, thereby accelerating their development in the digital era.
2. Overview of computational pharmaceutics
Computational pharmaceutics has emerged as a new discipline in the last decade, with the aim of enhancing the efficiency of research and development process for new molecular entities.34 This discipline integrates multi-scale simulation and artificial intelligence techniques to enable the systematic simulation, analysis, and prediction of the behaviour of drugs. The first significant advancement of modern pharmaceutics occurred between the 1950s and 1980s with the emergence of “physical pharmacy”, which integrates principles of physical chemistry into pharmaceutical formulations. The second generation (1980s–2010s) was characterized by the development of sophisticated drug delivery systems. In recent decades, the significant growth in computational power and algorithmic sophistication has promoted computational pharmaceutics to the forefront of pharmaceutical innovation. By integrating machine learning, molecular modelling, process simulation, and physiologically-based pharmacokinetic (PBPK) modelling, computational pharmaceutics offers high-dimensional optimisation capabilities that significantly reduce the reliance on empirical methods.34,35
In the early stages, computational pharmaceutics mainly employed traditional computational methods, such as quantum mechanics (QM), molecular dynamics (MD), and Monte-Carlo (MC). QM can accurately describe atomic and molecular interactions, facilitating the prediction of structural and physicochemical properties of molecules. Conversely, MD simulates the physical motion of atoms and molecules, enabling the study of complex interactions over extended timescales. These methods have been pivotal in revealing the molecular mechanisms underlying drug-excipient interactions, stability, and drug release kinetics.34,35 A significant advancement in computational pharmaceutics has been the integration of machine learning algorithms. Techniques such as artificial neural networks, deep learning, and ensemble methods have been employed to enhance the accuracy of drug release predictions and stability assessments. For example, ML has facilitated the design of hydrophilic sustained-release matrix tablets and oral fast-disintegrating films by optimising the interaction of various formulation components.36
The combination of traditional computational methods with ML-driven models has been demonstrated to be particularly effective. Physiologically based pharmacokinetic modelling, which predicts the pharmacokinetic behaviour of formulations, is a representative example. By utilising in vitro and in silico data, PBPK models simulate the concentration-time profiles in the human body, facilitating the rational design of drug delivery systems.37 Moreover, data-intensive approaches using ML algorithms can refine PBPK models, ensuring more accurate predictions and enhancing regulatory compliance.38 The integration of classical simulation techniques and ML-driven methodologies represents a significant milestone in the field of computational pharmaceutics. This interdisciplinary integration not only improves the predictive capacity and robustness of pharmaceutical formulations but also accelerates the R&D processes by reducing the dependence on traditional trial-and-error methods.34,35
As computational pharmaceutics continues to evolve, the integration of multi-scale modelling techniques and advanced data analytics possesses a great promise in drug discovery, ultimately enhancing the quality and efficiency of pharmaceutical products. The toolboxes for computational pharmaceutics are also applicable to computational nanodrug discovery. As listed in Table 1, these methods can be employed individually or in combination18–20 to investigate the interfacial interactions between nanodrugs and biological entities, to predict the therapeutic efficacy, pharmacokinetics, and toxicity of nanodrugs, as well as to optimise nanodrug formulations, and so on.
Table 1 Computational toolboxes for multi-scale simulations of nanodrugs
Computation methods |
Interfacial interactions |
Target events |
Main computation outcomes |
DFT |
Surface adsorption |
Drug adsorption |
Adsorption energy |
Biomarker adsorption |
Adsorption structure |
Toxin adsorption |
Binding characteristics |
Surface catalysis |
Catalytic transformation of biomolecules |
Reaction process |
Reaction energy profile |
Activity descriptor |
Chemical conversion |
Ion release |
Cation formation energy |
Degradation |
Structural evolution |
MD/CGMD |
Surface adsorption |
Drug adsorption |
Adsorption energy |
Protein adsorption |
Adsorption structure |
Ligand adsorption |
Binding characteristics |
Time evolution of structure |
Supramolecular recognition |
Passive internalisation |
Transmembrane process |
Receptor-mediated internalisation |
Transmembrane kinetics |
Ligand–receptor binding |
Binding structure |
Protein–receptor binding |
Binding energy |
Binding characteristics |
Chemical conversion |
Assembly and aggregation |
Structural evolution |
Deformation and disassembly |
Degradation |
MC |
Surface adsorption |
Drug adsorption |
Adsorption position |
Adsorption structure |
Adsorption energy |
Supramolecular recognition |
Ligand–receptor interaction |
Binding structure |
ML |
Surface adsorption |
Drug adsorption |
Drug loading capacity |
Protein adsorption |
Nanodrug formulation |
Ligand adsorption |
Protein adsorption structure |
Protein component |
Ligand–nanoparticle optimisation |
Supramolecular recognition |
Cellular uptake |
Cell–particle interaction |
Cell recognition |
Ligand–receptor optimisation |
Surface catalysis |
Catalytic transformation of biomolecules |
Catalytic activity classification |
Catalytic activity prediction |
Activity descriptor prediction |
Catalysis optimisation |
Chemical conversion |
Aggregation |
Structural transformation assessment |
Degradation |
— |
General optimisation |
Drug solubility |
Particle size |
Stability |
Pharmacokinetics |
PBPK |
— |
— |
Absorption, distribution, metabolism, excretion of nanodrugs |
Data management |
— |
— |
Findable, accessible, interoperable, reusable datasets |
3. Surface adsorption-based nanodrugs
Nanomaterials, which typically possess large surface areas, can effectively adsorb small molecule drugs, functional ligands, and proteins via various intermolecular interactions (e.g. electrostatic, van der Waals, and coordination interactions), enabling their various medical functions. To enhance the efficacy of these surface adsorption-based nanodrugs, it is essential to understand the interactions between nanomaterials and various adsorbates. Such knowledge is of crucial importance for the future design and screening of these surface adsorption-based nanodrugs. Multi-scale calculations have been conducted to study the adsorption of small molecule drugs, functional ligands, and proteins on the surfaces of important nanomaterials, including carbons, metals, metal oxides, polymers, biomolecular assemblies, and porous metal/covalent-organic frameworks (MOFs/COFs).18,19,39
3.1. Adsorption of small molecule drugs on nanosurfaces
To reduce the dosage and potential side effects of conventional small molecule drugs, nanoparticles have been widely used as carriers to deliver those drug molecules for precise disease therapy. Interactions between nanosurfaces (host) and drug molecules (guest) are key factors that determine the drug loading and release from nanosurfaces. A few examples of drug molecules investigated in computational studies on their adsorption onto nanosurfaces are listed in Fig. 2.
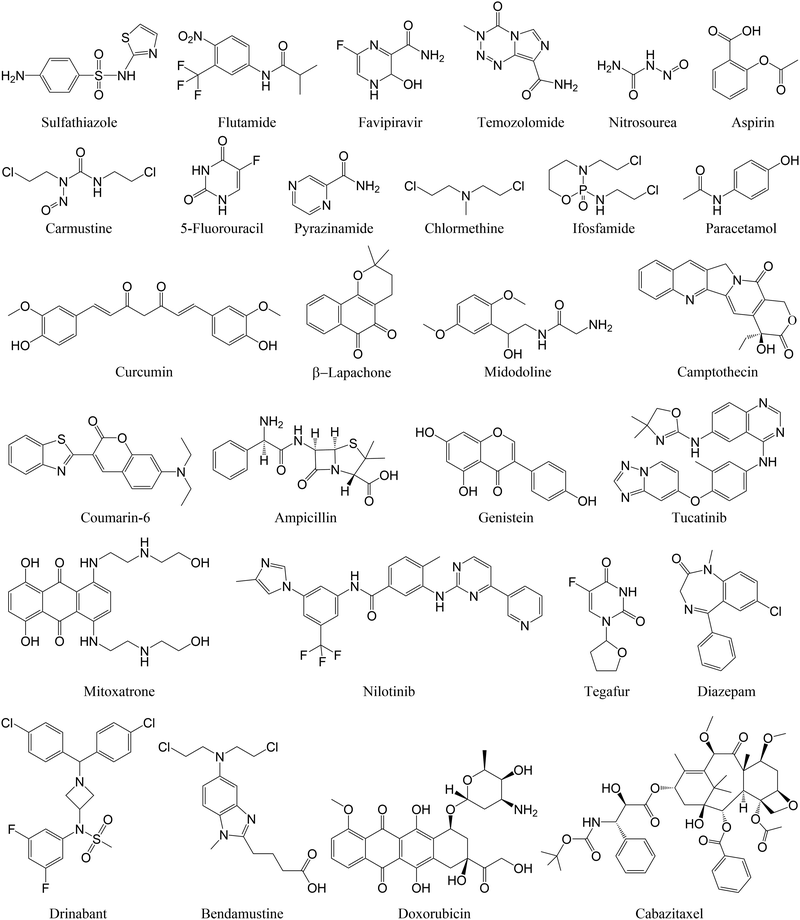 |
| Fig. 2 Representative examples of small molecule drugs adsorbed on nanosurfaces. | |
3.1.1. Inorganic nanosurfaces.
Metal and metal oxide nanomaterials.
The presence of metal sites enables drug molecules to form partial covalent interactions with metal or metal oxide nanomaterials, leading to charge transfer between drug molecules and nanosurfaces (Table 2). This strong interaction is beneficial for improving the drug loading capacity, but may also disrupt the structures of drug molecules, making them inactive upon adsorption onto these nanosurfaces. Noble metals such as gold nanoparticles (AuNPs) are representative examples of drug delivery nanocarriers. DFT calculations revealed that an antibiotic, ampicillin, can be readily adsorbed onto various gold surfaces with its sulphur and nitrogen atoms simultaneously bound to gold atoms.40 Although the adsorbed ampicillin took several configurations, the corresponding adsorption energies were very similar and sufficiently negative (−49.1 to −56.5 kcal mol−1). Specifically, the covalent binding character was observed for the ampicillin–gold nanoconjugate, where charge transfer occurs from the ampicillin to the gold nanosurfaces, contributing to the high stability. A similar binding character was found in the adsorption of sulfathiazole on AuNPs, where Au atoms were anchored to the nitrogen of the thiazole ring in sulfathiazole.41 Such an interaction can be further stabilised by the ring-surface coupling, with the benzene ring almost parallel to the gold surface. Although these antibiotics can be strongly adsorbed on nanosurfaces, making them difficult to release, their active sites were not blocked in the drug–gold nanoconjugate, which is favourable for maintaining the antibacterial activity of the resulting nanodrugs.
Table 2 Binding energies between different drugs and inorganic nanosurfaces calculated by DFT methods
Nanosurface |
Drug molecule |
Binding character |
Binding energy (kcal mol−1) |
DFT functional |
Ref. |
Au(111), (110), and (100) surfaces |
Ampicillin |
Partially covalent Au–O bond |
−49.1 to −56.5 |
opt-B86b-vdw |
40
|
Au6, Au8, and Au20 clusters |
Sulfathiazole |
Partially covalent Au–N bond |
−17.7 ∼ −27.2 |
PBE |
41
|
Au⋯π interaction |
ZnO nanosheet |
Flutamide |
Partially covalent Zn–O bond |
−20.6 |
vdw-DF |
42
|
(ZnO)12 Cluster |
Favipiravir |
Partially covalent Zn–O bond |
−34.8 |
PBE-D |
43
|
C60 |
Aspirin |
Non-covalent interaction |
−6.7 |
M06-2X |
44
|
C59B |
Partially covalent B–O bond |
−24.0 |
C60 |
Nitrosourea |
Non-covalent interaction |
−12.8 |
45
|
MC59 (M = Au, Hf, Hg, Ir) |
Partially covalent M–O bond |
−24.7 ∼ −32.3 |
Carbon dot |
Cytarabine |
Van der Waals interaction |
−24.3 |
ωB97XD |
46
|
Clofarabine |
−23.3 |
BN dot |
Cytarabine |
−17.3 |
Clofarabine |
−16.3 |
Carbon dot |
Gemcitabine |
−11.9 |
M06-2X |
47
|
N-Doped carbon dot |
−15.3 |
B40 |
5-Fluorouracil |
Partially covalent B–O bond |
−13.5 |
PBE-D2 |
48
|
M-Encapsulated B40 (M = Na and Ca) |
Partially covalent B–O bond |
−16.1 to −17.1 |
B12N12 cluster |
5-Fluorouracil |
Covalent B–O bond |
−27.0 |
DFT-D2 |
49
|
6-Mercaptopurine |
Covalent B–N bond |
−36.8 |
6-Thioguanine |
Covalent B–N bond |
−34.4 |
Graphene |
β-Lapachone |
Van der Waals interaction |
−21.2 |
PW91-D3 |
50
|
M-Doped graphene (M = Au and Pt) |
Partially covalent M–O bond |
−38.2 to −40.8 |
Graphdiyne nanosheet |
Hydroxyurea |
O–H⋯π interaction |
−13.8 |
DFT-D3 |
51
|
5-Fluorouracil |
N–H⋯π interaction |
−9.4 |
BN-Doped graphdiyne nanosheet |
Hydroxyurea |
O–H⋯π interaction |
−29.7 |
Partially covalent B–O bond |
5-Fluorouracil |
N–H⋯π interaction |
−13.9 |
BN nanotube |
5-Fluorouracil |
N–H⋯π interaction |
−5.63 |
B3LYP |
52
|
M-Doped BN nanotube (M = Sc and Ti) |
Partially covalent M–O bond |
−36.8 to −39.9 |
(8,0)-Carbon nanotube |
5-Fluorouracil |
Van der Waals interaction |
−7.9 |
DFT-D2 |
53
|
Chloromethylated (8,0)-carbon nanotube |
−10.7 |
(6,6)-Carbon nanotube |
−25.0 |
revPBE-D3BJ |
54
|
(7,7)-Carbon nanotube |
−29.3 |
(8,8)-Carbon nanotube |
−25.8 |
(7,7)-BN Nanotube |
Carmustine |
C–H⋯π interaction |
−36.5 |
PBE-D2 |
55
|
(8,8)-BN nanotube |
−37.8 |
(9,9)-BN nanotube |
−35.2 |
COOH-functionalised carbon nanotube |
Carmustine |
Intermolecular hydrogen bonds |
−8.2 |
B3LYP |
56
|
Silicon carbide nanosheet |
Pyrazinamide |
Partially covalent Si–O bond |
−33.9 |
PBE-D3BJ |
57
|
Chlormethine |
Partially covalent bond |
−29.1 |
B3LYP-D3 |
58
|
Silicon carbide nanotube |
Amphetamine |
Electrostatic interaction |
−8.5 |
TPSS-D2 |
59
|
Pt-Doped silicon carbide nanotube |
Pt⋯π complexation |
−34.4 |
Phosphorene |
Ifosfamide |
Van der Waals interaction |
−3.7 |
B3LYP |
60
|
M-Doped phosphorene (M = Be, Mg, and Ca) |
Partially covalent M–O bond |
−36.0 to −40.1 |
Metal oxide nanoparticles such as ZnO and Fe3O4 can also form strong interactions with drug molecules. The adsorption of flutamide on ZnO nanosheets was investigated using DFT methods with the dispersion correction.42 In the most stable adsorption structure, the O atoms of the –NO2 group of flutamide interact with a Zn atom of the nanosheet at 2.11 Å with an adsorption energy of −20.6 kcal mol−1. Another DFT calculation of ZnO nanoclusters revealed that the favipiravir molecule takes a similar binding configuration forming a Zn–O bond with a drug adsorption energy of −34.8 kcal mol−1.43 Although the calculated adsorption energy depends on the computational methods, these energy values suggest the thermal stability for drug adsorption on ZnO nanosurfaces.
MD simulations have been employed to investigate the effects of nanoparticle size on the adsorption of drug molecules onto metal (oxide) nanosurfaces. Depending on the particle size, different adsorption mechanisms have been observed. For example, drug molecules can alternately prefer the Fe2+ or Fe3+ sites of the biocompatible Fe3O4 nanoparticles with increasing particle size. This is likely due to the different surface structures, which are either oxygen-rich or iron-rich.61 More importantly, the drug adsorption energy depends on the nanoparticle size with stronger adsorption observed on smaller nanoparticles.62 Given that a small-sized nanoparticle may aggregate and has a limited number of adsorption sites, a medium-sized nanoparticles may be more suitable considering the adsorption affinity and the capability to load a relatively large number of drug molecules. However, it remains unclear how to identify the optimal nanoparticle size for a specific nanodrug formulation.63
Carbon nanomaterials and analogues.
Carbon nanomaterials and analogues have long been recognised as good carriers for drug molecules due to their versatile and tunable surface structures. DFT calculations have been employed to determine the binding structure and strength of drug molecules with these nanomaterials including zero-dimensional (0D) fullerenes and boron nitride nanocages/nanoclusters, one-dimensional (1D) carbon and boron nitride nanotubes, as well as two-dimensional (2D) graphene, graphdiyne, and boron nitride nanosheets. Drug molecules bind to these nanosurfaces mainly through non-covalent interactions, which necessitates the use of dispersion-corrected DFT methods. Such calculations have demonstrated the effectiveness of several surface engineering strategies, including the introduction of doping atoms or functional groups, to regulate the adsorption affinity of carbon nanomaterials and analogues to drug molecules.
The 0D carbon nanomaterials and analogues typically have a weak to moderate affinity to drug molecules with binding energies of less than −20.0 kcal mol−1, due to the lack of specific interaction sites with drug molecules. DFT calculations using the M06-2X functional revealed moderate binding energies for the adsorption of drug molecules such as nitrosourea (−12.8 kcal mol−1) and aspirin (−6.7 kcal mol−1)44,45 to C60 fullerene. Similar binding energy values were found for drug adsorption onto small-sized carbon quantum dots,46 boron nanocage48 and nanocluster.49 Introducing doping atoms provides additional interacting sites with drug molecules, leading to an enhanced binding affinity of carbon nanomaterials and analogues to drug molecules. For example, the binding energy for aspirin adsorption to C60 fullerene increased from −6.7 kcal mol−1 to −24.0 kcal mol−1 by B doping. This can be ascribed to the interaction between electron deficient B atom and electron rich O atoms in aspirin.44 In this regard, metal doping is more efficient due to the strong metal–oxygen interactions. Introducing doping atoms is also applicable to 1D and 2D carbon nanomaterials and analogues to enhance their binding affinities to drug molecules.47,50–52
Unlike the 0D nanocages/nanoclusters, 1D nanotubes and 2D nanosheets have extended structures and can adsorb drug molecules at both sides. The encapsulation of drug molecule into the inside of carbon or boron nitride nanotube is energetically more stable than adsorption onto the outside surface.53,54 Depending on the size of drug molecules, there is an optimal diameter for drug encapsulation. The CNT (7, 7) is the best for drug encapsulation of small drug molecule 5-fluorouracil.54 On the other hand, the BNNT (8, 8) and (9, 9) with larger diameters are better for carmustine and temozolomide encapsulation, respectively.55 To enhance drug adsorption onto the outside surface of CNTs or BNNTs, surface modification with functional groups such as –COOH is necessary. DFT calculations on the interactions of carmustine with pristine and COOH-functionalised CNTs showed that the binding energy for carmustine adsorption is significantly increased after COOH-functionalisation.56 This can be attributed to the formation of intermolecular hydrogen bonds between drug molecules and COOH groups. Similar trends were found in the adsorption of the 5-fluorouracil onto chloromethylated CNTs using the dispersion-corrected DFT method.53 These results demonstrate the importance of surface functionalisation in enhancing drug loading onto CNTs.
The pristine carbon and boron nitride nanosheets also have weak affinities to drug molecules. MD simulations on the adsorption of tegafur on graphene flakes including graphene oxide, hydroxylated graphene (G-OH), carbonylated graphene (G-CO)64 demonstrated a decreasing order of GO > G-OH > G-CO for the affinity of these functionalised graphene nanosheets to tegafur. Although hydrogen bonding, π–π stacking, and hydrophilic interactions are present in all these graphene–tegafur systems, the number of hydrogen bonding interactions in the GO–tegafur system is greater than in the others, suggesting that graphene oxide could be a suitable carrier for tegafur. The impact of hydrogen bonding on drug loading was also observed for camptothecin and mitoxantrone adsorption on functionalised graphene. Mitoxantrone/nanographene showed a higher binding energy than camptothecin/nanographene due to the presence of a larger number of hydrogen bonding interactions.65
To more efficiently predict drug carriers without significant computational efforts, a combined quantum genetic algorithm (QCA) and QM/semi-empirical quantum mechanics (SQM)/force field (FF) method has been proposed. This method is able to globally and efficiently predict the optimal binding interactions between graphene/GO and drug molecules. For example, an appropriate functionalisation ratio of graphene is suggested to balance the stability and the solubility/biocompatibility of graphene-based drug delivery systems. In addition, four suitable drug delivery systems (midostaurin-GO, nilotinib-GO, tucatinib-GO and arzoxifene-GO) with stronger drug–GO interactions were found through screening the DrugBank database using the proposed method.66 Such a powerful and efficient method is expected to be useful in accelerating other nanodrug discovery.
Multi-scale simulations have also been conducted to investigate drug adsorption onto other inorganic nanosurfaces, such as Si- and P-based nanomaterials. Silicon carbide has a much stronger adsorption affinity to drug molecules than its analogue graphene or CNTs. This is mainly because Si atoms can form stronger interaction with negative charged atoms (e.g. O), typically existing in drug molecules.57,58 In addition, metal doping59 can significantly enhance the adsorption affinity of SiC to drug molecules, which also hold for drug adsorption onto phosphorene and silica nanoparticles.60,67 The oxygen atoms of ifosfamide drug coordinate with the doping metals, resulting much stronger binding energies (−36.0 to −40.1 kcal mol−1) than that for ifosfamide adsorption onto pristine phosphorene (−3.7 kcal mol−1).60
3.1.2. Polymeric nanosurfaces.
Polymers are another drug carriers that have attracted much interest because of their biodegradability, biocompatibility, and designable nanostructures. The compositions of these polymeric nanomaterials play significant roles in determining their drug loading efficiency.2 Regarding this, computational studies have been conducted to understand drug adsorption and release behaviour of polymeric nanomaterials. The force field-based MD and/or MC simulations have been mostly used, although DFT calculations can somehow describe the drug–polymer interactions using a monomer or a single short chain to model polymers. The estimated Gibbs free energy changes suggest that polyvinylchloride (PVC) can weakly adsorb paracetamol and diazepam drugs with small ΔG values (Fig. 3a–d), consistent with the non-detectable loss in drug concentrations after contacting with polyvinylchloride. On the other hand, polyethylene (PE) can strongly adsorb diazepam but weakly adsorb paracetamol, which were confirmed by experimentally measured drug loss.68,69 Based on these computational and experimental results, a threshold value (−7.2 kcal mol−1) of Gibbs free energy changes was suggested to evaluate whether a drug molecule could be adsorbed onto polymeric nanosurfaces.68–70 Because the adsorption capacity is also dependent on the drug concentration, a more negative threshold value can be expected if the target drug molecule has a lower solubility and vice versa.
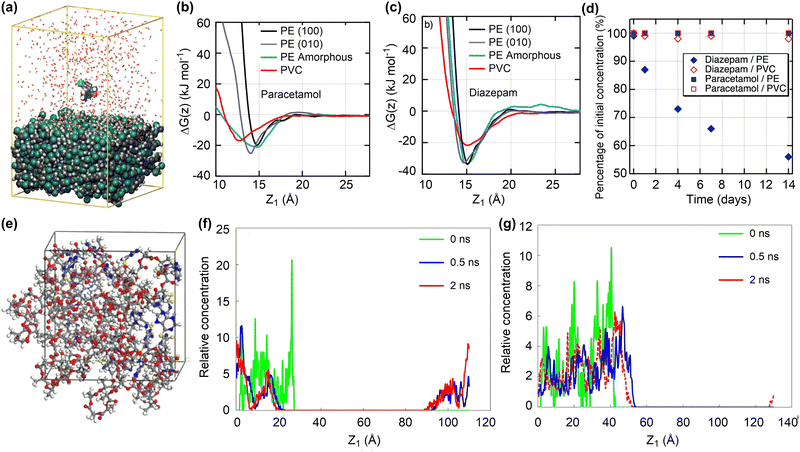 |
| Fig. 3 Drug adsorption and release on polymeric nanosurfaces. (a) Conformation of diazepam molecule adsorbed onto a PVC surface. (b) and (c) Gibbs free energy profiles for the adsorption of paracetamol and diazepam molecules onto PE and PVC surfaces. (d) Changes of paracetamol and diazepam concentrations upon contact with PVC and PE tubings. Reproduced with permission from ref. 69. Copyright 2021, American Chemical Society. (e) Conformation of 6-mercaptopurine adsorbed in PLA nanoparticles. (f) and (g) Concentration distribution for the release of drug molecule from PLA and PLA–PEG nanoparticles. reproduced with permission from ref. 71. Copyright 2019, Elsevier. Z1 is defined as the distance between the centres of mass of the drug molecule and surface. | |
Due to the structural flexibility of polymers, drug molecules tend to be encapsulated into polymer chains or form co-assembly composites. The adsorption energies of four drugs (doxorubicin, cabazitaxel, β-lapachone, and drinabant) on poly(lactic acid)–poly(ethylene glycol) (PLA–PEG) nanoparticles, representative polymeric nanodrug delivery systems, were investigated by the MC docking method. Such a computationally efficient method can estimate well the order of drug adsorption energies, consistent with their loading capacities.72 More complicated MD simulations were conducted to investigate the adsorption and release of 6-mercaptopurine in PLA (Fig. 3e).71 The drug loading capacity could reach as high as 35.8 wt% with sufficiently negative adsorption energies, ensuring the stability of resulting nanodrug composites. However, due to the hydrophobicity of PLA, the encapsulated drug molecules are hardly released (Fig. 3f). Introducing the hydrophilic PEG composition can significantly increase the drug release ability while maintaining the drug loading capacity (Fig. 3g).
Poly(lactic-co-glycolic acid) (PLGA) is another representative polymeric carrier for drug delivery. The ratio of LA to GA and the molecular weight of PLGA can affect the encapsulation of drug molecule into PLGA and stability of corresponding nanodrug complexes. Amongst the investigated polymeric systems (PLA, PLGA72, PLGA55, PLGA72, and PGA), PLGA55 (LA
:
GA = 50
:
50) exhibits the strongest interaction with coumarin-6 for all chain lengths because of the homogeneous spatial arrangements. However, the binding interaction between coumarin-6 and a single PLGA chain is not very strong.73 The weak interactions between drug molecules and PLGA make them susceptible to disassemble.74 The incorporation of clay minerals is an efficient strategy to enhance the drug loading capacity of polymer-based nanoparticles.75 The presence of montmorillonite can enhance the adsorption of curcumin due to the formation of strong silicon–oxygen interactions. Such interactions between curcumin and montmorillonite are dependent on ions, which may facilitate drug release in biological environment. These findings suggest that the mineral/polymer composites could be good candidates for nanodrug delivery.
3.1.3. Biomolecular nanosurfaces.
Biomolecular nanosurfaces are very promising for medical applications because of their natural abundance, biocompatibility and chemical versatility. Representative examples are polysaccharide-, peptide-, protein-, DNA- and lipid-based nanostructures.76 Similar to the polymer case, interactions between drug molecules and biomolecular nanosurfaces have been mainly investigated through MD simulations, despite that DFT calculations are useful to elucidate the driving force, the adsorption energy, and the binding nature for drug adsorption onto biomolecule nanosurfaces (Fig. 4a).77,78 Chitosan, a natural polysaccharide, is an example of biomolecules that can serve as a nanocarrier. To achieve the optimal therapeutic efficacy of drug molecules, chemical modification of the natural chitosan is necessary. Many tunable parameters, including the type, degree, and pattern of functional groups, the water–polymer ratio, as well as the drug payload, can be involved, hindering the rational design of modified chitosan hydrogels for drug adsorption and release. A flexible and transferable coarse-grained model was proposed to accurately capture the network structure of acetyl, butyryl or heptanoyl modified chitosan chains, and the transport of the drugs (doxorubicin and gemcitabine) through the network (Fig. 4b).79 Multi-scale simulations suggested that hydrophobic modifications significantly alter the conformation and spatial arrangement of the chains from a uniform distribution to a cluster-channel morphology. In addition, the diffusion of drug molecules with different polarity, hydrophobicity, and size show different dependence on the type, degree and pattern of modification. The diffusion coefficient of doxorubicin varies significantly, while that of gemcitabine is almost constant. Further development and application of such computational tool is expected to explore more types of modification and therapeutic payloads.
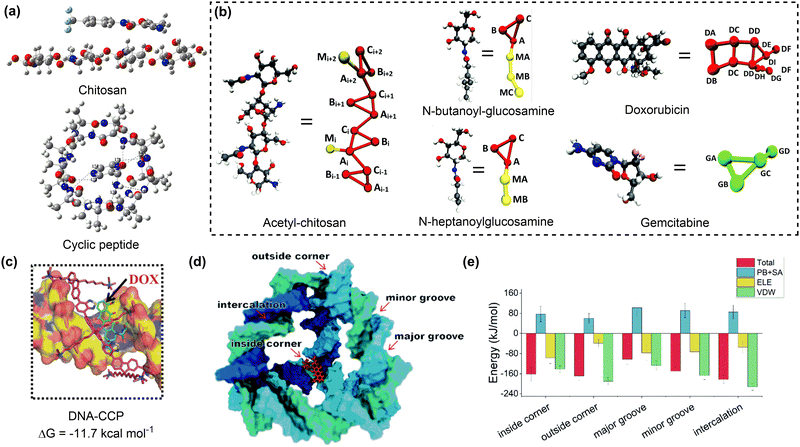 |
| Fig. 4 Drug adsorption on biomolecular nanosurfaces. (a) Structures of teriflunomide interacting with chitosan and pyrazinamide interacting with cyclic peptide. Reproduced with permission ref. 77 and 78. Copyright 2018 and 2023, Elsevier. (b) Coarse-grained mapping of atomic structures of acetyl-chitosan, n-butanoyl-glucosamine, n-heptanoylglucosamine, doxorubicin and gemcitabine. Reproduced with permission from ref. 79. Copyright 2020, CC BY 3.0. (c) The most dominant pose and corresponding binding energy of doxorubicin to DNA-CCP. Reproduced with permission from ref. 80. Copyright 2020, Wiley-VCH. (d) Possible binding sites of a doxorubicin to the tetrahedral DNA nanostructure. (e) The total binding energies and corresponding energy decomposition terms for the five binding modes. Reproduced with permission from ref. 81. Copyright 2022, CC BY-NC 3.0. | |
DNA or protein nanostructures are another representative biomolecular nanocarriers. The drug loading mechanisms of these nanostructures have been investigated through molecular docking and/or MD simulations.82,83 A single strained DNA chain exhibits a weak binding affinity to drug molecules due to the non-covalent interactions between them. The introduction of additional components such as chitosan, or the formation of nanocages may enhance the drug binding affinity of the resulting DNA nanostructures (Fig. 4c).80 The tetrahedral DNA nanocage (TDN) has five possible binding modes for doxorubicin loading. With the increase in the number of adsorbed doxorubicin molecules, the most favourable binding mode changed from the intercalation mode to the inside-corner mode. Importantly, the presence of more binding sites resulted in a strong binding affinity of TDN to doxorubicin molecules (approximately −45 kcal mol−1) at varying drug loading capacities (Fig. 4d and e).81 Similar calculations were conducted to investigate the mechanism for drug adsorption onto protein nanostructures, such as human heavy-chain ferritin (HFn), which shows an intrinsic active tumour-targeting ability due to its capacity to recognise and bind to human transferrin receptor 1 (TfR1).84 A combined molecular docking and MD simulation showed that encapsulating doxorubicin into the HFn cage is more stable than adsorbing it onto the outer surface due to the formation of more hydrogen bonds and salt bridges.85 To promote drug encapsulation into the cavity of proteins such as apoferritin (APO-Fr), the disassembly of openings on protein nanosurfaces in response to acidic conditions was proposed. Pores with more hydrophilic surfaces exhibit higher flexibility and tendency for opening to accommodate more drug molecules.86
Lipid-based nanostructures, such as nanodiscs and lipid vesicles, are emerging as promising biomolecules for drug delivery. Despite the complexity of their structures, multi-scale simulations can be employed to investigate the drug loading mechanisms of these biomolecular nanostructures. For instance, MD simulations were conducted to explore the drug–nanostructure interactions in nanodisc formulations.87 The free doxorubicin molecules are difficult to load on the nanodisc surface due to the unfavourable drug–lipid interactions. Conversely, the lipid conjugation of doxorubicin prodrugs demonstrated a sufficient conformational stability when incorporated into nanodiscs. This stability is influenced by the lipid moieties conjugated with the doxorubicin molecules, demonstrating that the formulation designs significantly impact the drug loading and the stability of drug–nanodisc complexes.
In order to efficiently describe drug loading into lipid vesicles such as liposomes, a coarse-grained model has been developed. Simulations conducted with this CG model reveal the impact of hypericin loading on the structural integrity of liposomes and the distribution of drug molecules within these vesicles. In particular, increasing drug loading would induce the structural expansion of liposomes and the aggregation of drug molecules near the polar headgroups of the outer lipid layer. The calculated potential of mean force further highlighted the disparities between drug loading on the inner and outer layer of liposomes. This research underscores the importance of CG simulations in understanding drug adsorption onto liposomes or membranes, paving the way for optimised strategies and future hybrid models for a deeper understanding of drug delivery mechanisms.88 Recently, a CG-based computational framework has been proposed to optimise drug loading in extracellular vesicles (EVs) through nanofluidic squeezing, which induces transient nanopores for drug encapsulation. The framework captures essential aspects, such as pore formation, EV deformation, fluidic properties, and drug loading efficiencies, which are dependent on the EV size, flow velocity, and channel dimensions. It was found that higher flow velocities and larger EVs can enhance drug uptake, while smaller constrictions resulted in more considerable EV deformation and even vesicle damage. A phase diagram derived from the simulations provides a practical guide for optimising nanochannel geometries and flow conditions to optimise drug loading without impairing the structural integrity of EVs. This study not only advances the field of EV-based drug delivery systems but also provides valuable insights into EV transport and fusion mechanisms.89
3.1.4. Porous nanosurfaces.
Porous nanomaterials, such as metal/covalent organic frameworks, are a new class of drug carriers with potential advantages of high loading capacity, low toxicity, biodegradability, specific targeting ability, as well as controlled/sustained drug release because of their tunable and designable compositions and pore structures. Various computational methods have been employed to investigate the host–guest interactions between porous nanomaterials and drug molecules, which determine the drug adsorption and release behaviour.90 The grand canonical Monte Carlo (GCMC) simulation, typically based on the universal force field, can be employed to study the drug loading position and capacity in porous nanomaterials. The simulated loading capacities of bendamustine and 5-fluorouracil in five MOFs (UiO-66, UiO-66-NH2, UiO-66-COOH, UiO-67, and UiO-66-NDC, UiO = University of Oslo) through this method are comparable to the experimental results. In addition, this method can well recognise the hydrogen bonding interactions (C–H⋯F, C–H⋯O, and N–H⋯O) between drug molecules and the framework.91 Likewise, GCMC simulations suggested that the remarkable drug loading of natural bioflavonoid genistein in mesoporous MIL-100(Fe) arises from the specific π–π stacking between the aromatic ring of genistein and the trimesate linker of the MIL-100(Fe).92 These results demonstrate the efficacy of GCMC simulations in describing drug loading in porous nanomaterials. However, it should be noted that the drug loading may be overestimated by GCMC simulations when the porous nanomaterials contain inaccessible pores due to the presence of solvents and/or very narrow channels for drug diffusion.93
To improve the drug loading capacity of nanomaterials based on surface adsorption, it is common to introduce additional interaction sites. For example, the amount of curcumin loading increased from 22.4 wt% to 28.3 wt% by introducing the –NO2 group into a MOF structure [Zn(BDC)(DABCO)0.5]n, (BDC2−: 1,4-benzene dicarboxylate, DABCO: 1,4-diazabicyclo[2.2.2]octane), providing additional C–H⋯O hydrogen bonding interaction.94 In addition, defect engineering is applied to increase the drug loading capacity of UiO-66 (Fig. 5a). The coulombic interaction between zirconium centres and pharmaceutical molecules plays a dominant role in stabilizing the host–guest interaction for UiO-66 with ligand defects. On the other hand, the van der Waals interaction is dominant for the ideal UiO-66 without open zirconium centre. Based on the calculated binding energies and molecular size of pharmaceutical molecule, the loading capacity can be qualitatively predicted (Fig. 5b).95 In addition to the host–guest interaction, geometrical parameters of porous nanomaterials, such as pore volume and cavity diameter, can affect the drug loading capacity.96 This is mainly because the limited size of channel may hinder drug diffusion into the pores.
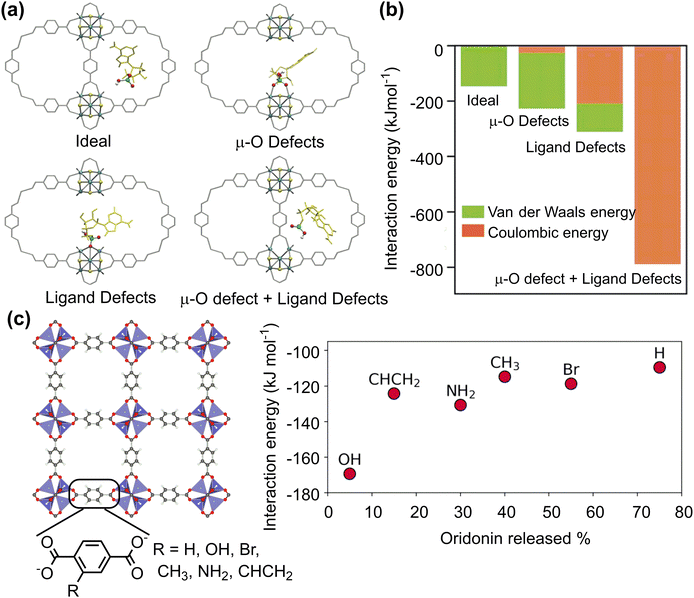 |
| Fig. 5 Drug adsorption on porous nanosurfaces. (a) and (b) Structures and interaction energies of adenosine monophosphate adsorbed in ideal and defected UiO-66. Reproduced with permission from ref. 95. Copyright 2021, Wiley-VCH. (c) Relationship between oridonin drug release and its interaction energy with functionalised MOF-5. Reproduced with permission from ref. 97. Copyright 2023, CC BY-NC 4.0. | |
The host–guest interaction can affect not only drug loading capacity but also drug release rate. A combined experimental and computational technique was employed to investigate the adsorption and release of acriflavine from four selected Zr-based MOFs (MOF-808, UiO-66, UiO-67, and NU-1000). The calculated adsorption energies agreed well with experimental release rates in water. A faster release rate was observed for MOF-808 and NU-1000 with weak adsorption energies of acriflavine whereas the release rate was slow for UiO-66 and UiO-67 with strong affinity to acriflavine.98 Similar results were for the drug release from a series of MOF-5 with different functionalised linkers. Among several possible descriptors, such as interaction energy, pore diameter, functional group volume, polarizabilities, and Hammett constants (σmeta), only the host–guest interaction energy shows a clear correlation with the amount of drug released; the stronger the interaction between the drug molecule and the framework, the longer the drug release (Fig. 5c).97
3.1.5. Summary.
In conclusion, inorganic, polymeric, bimolecular and porous nanomaterials can serve as carriers of conventional drug molecules. Multi-scale simulations have been employed to investigate the adsorption and desorption of drug molecules on these nanosurfaces. DFT calculations can be employed to effectively characterise the structure, energy, and mechanism of drug adsorption onto nanosurfaces, using either a small cluster/fragment or crystal structure as the model system. In the case of large-sized inorganic nanoparticles, polymeric and biomolecular nanomaterials, MD/CGMD simulations are frequently required. These calculations have revealed that drug molecules can be adsorbed or embedded into nanomaterials, forming a variety of interactions with the carriers, including π–π, hydrogen bonding, electrostatic, and even partial covalent interactions. Surface functionalisation, heteroatom doping, and defect engineering are effective methods for regulating the binding affinity of drug molecules to nanosurfaces. Given that the sustained release of drug molecules is usually desired for disease therapy, it is crucial to precisely engineer the drug–carrier interaction in order to facilitate the practical applications of these nanomaterials as drug carriers. In addition to sustained drug release, the drug–carrier interaction can be altered in response to external stimuli, such as pH and biological ions, leading to a controllable drug release. Therefore, the drug–carrier interaction may be employed as an effective energy descriptor to screen nanomaterials with sufficient drug loading capacity and desirable release rate. However, the optimal value is still absent, probably due to the large number and diversity of drug molecules and nanomaterials to be explored. An efficient but reliable screening methodology is thus urgently needed to promote the development of nanocarriers.
3.2. Adsorption of proteins on nanosurfaces
3.2.1. Effect on protein aggregation.
Protein aggregation and liquid–liquid phase separation (LLPS) are fundamental biophysical processes associated with cellular compartmentalisation and the pathogenesis of various diseases. The regulation of protein aggregation states using nanomaterials has attracted considerable attention for disease therapy. For instance, the inhibition of protein aggregation and clearance of amyloid fibrils are essential for the treatment of diseases, such as Alzheimer's disease, Parkinson's disease, and type II diabetes.99 On the other hand, nanomaterials that are capable of promoting protein aggregation, specifically liquid–liquid phase separation, are also attractive for enhancing anti-tumour/viral immunity.100 The inhibitory or promotion effects of nanomaterials depends on the protein species and properties of nanomaterials.101 It is therefore crucial to understand the interaction between nanosurfaces and protein monomers in order to facilitate the design of nanodrugs capable of regulating protein aggregation for a range of applications. Molecular dynamic simulations have been conducted to elucidate the competitive interactions between nanomaterials and protein monomers, as well as those between protein monomers themselves.102
The inhibitory effects arise from the adsorption of protein monomers, such as α-synuclein (αS), tau, β-amyloid (Aβ) and human islet amyloid polypeptide (hIAPP) onto nanosurfaces, overriding the intermolecular interactions that cause the self-assembly of monomers into toxic oligomers or insoluble amyloid fibrils. Neutral graphene sheets have been demonstrated to efficiently inhibit Aβ fibrillation and break mature amyloid fibrils through penetration and extraction of Aβ peptides (Fig. 6a).103 Further analysis on the structural changes showed that the hydrophobic phenylalanine residues of extracted Aβ peptides can form strong π–π stacking and hydrophobic interactions with graphene sheets, thereby enabling their stronger interaction with graphene than with themselves.
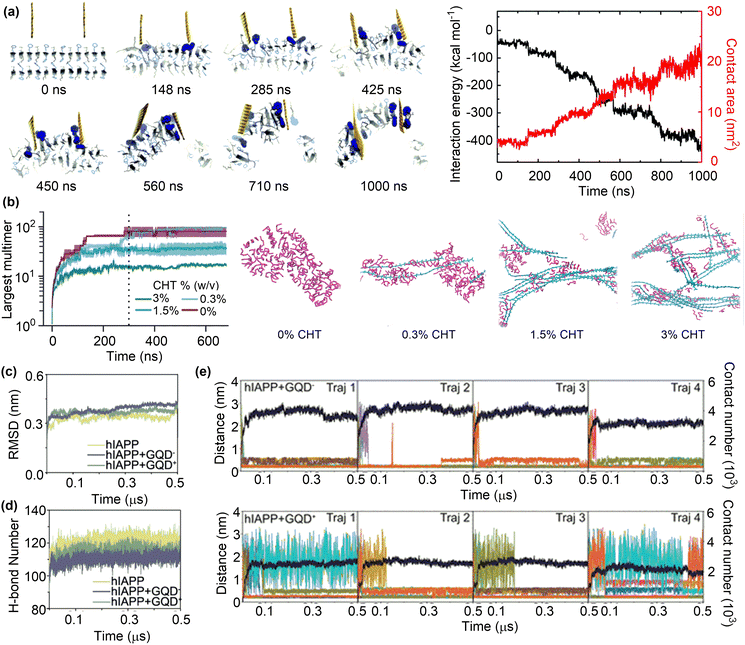 |
| Fig. 6 Inhibition of Aβ fibrillation by surface adsorption. (a) Trajectory and interaction energy profile for graphene nanosheet insertion into the Aβ amyloid fibril and peptide extraction. Reproduced with permission from ref. 103. Copyright 2015, the Royal Society of Chemistry. (b) Time evolution of Aβ aggregation in the presence of 0, 0.3, 1.5, and 3.0% chitosan and the snapshots of quasi-equilibrium conformations of Aβ self-assembly. Reproduced with permission from ref. 104. Copyright 2023, the Owner Societies. (c) and (d) Average time evolution of Cα-RMSD and average number of H-bonds between hIAPP backbones upon contact with GQDs. (e) Time evolution of the minimum distance and the total contact number between GQD− or GQD+ and hIAPP fibril. Reproduced with permission from ref. 105. Copyright 2023, American Chemical Society. | |
The role of nanomaterials in amyloid formation is potentially affected by a number of factors, such as concentration, charge and surface functionality. MD simulations were conducted to investigate the influence of chitosan concentrations on Aβ aggregation.104 Unsurprisingly, the population of Aβ monomers declined rapidly in the absence of chitosan (CHT) with the formation of Aβ oligomers and eventually a single large aggregate was formed (Fig. 6b). Conversely, the rate of monomer decay and the number of peptides in the largest oligomers decreased upon the addition of CHT at varying concentrations, demonstrating the inhibitory effects of CHT on Aβ aggregation.106 However, it is essential to carefully examine the concentrations of nanomaterials to specifically regulate protein aggregation. Low concentrations may have minimal influence on aggregation, whereas high concentrations could potentially promote aggregation.107 For instance, the maximum concentrations of graphene quantum dots for the inhibition of hIAPP and αS were found to be 400 and 200 μg mL−1, respectively.108,109
The charge state of nanomaterials is another factor that influences their role in protein aggregation. Different amount of cationic charge can be assigned to CHT's N-glucosamine saccharides by changing the pH conditions; 10% at pH 7.5 and 50% at pH 6.5. Due to the enrichment of electrostatic interactions between Aβ peptides and cationic units in CHT under lower pH conditions, the inhibitory effect is more pronounced at pH 6.5 than at pH 7.5. A similar study was conducted to investigate the inhibitory effects of charged GQDs on hIAPP aggregation (Fig. 6c).105 The addition of charged GQDs can sufficiently hinder the formation of inter-peptide and interfacial hydrogen bonds, as well as interfering with the hydrophobic packing of hIAPP side chains (Fig. 6d). The presence of negatively charged carboxyl groups in GQD− enabled the formation of electrostatic attractions, hydrogen bonds, and salt bridges, which collectively stabilize the interactions between hIAPP and GQD−. On the other hand, such attractive interactions were absent or very weak in the hIAPP + GQD+ system. These results demonstrate the stronger interaction between GQD− and hIAPP, as evidenced by the larger contact numbers and smaller distances in hIAPP–GQD− systems than in the hIAPP–GQD+ systems (Fig. 6e). Because GQD− can more strongly adsorb hIAPP than GQD+, the negatively charged GQD was suggested as a more effective candidate for amyloid inhibition than the positively charged one in disrupting hIAPP fibrils.
The functionalisation of nanosurfaces can not only alter the charge states, but also offer additional interaction sites with amyloid peptides. This could potentially affect the ability of nanomaterials to inhibit amyloid aggregation. A comparative analysis was conducted on the adsorption of Aβ peptide on carbon nanotubes (CNT) and functionalised ones with carboxyl and hydroxyl groups (CNT–COOH and CNT–OH).110 Although pristine CNT can alter the structure of Aβ peptide via π–π stacking and hydrophobic interactions, the structural changes of the Aβ peptide are more pronouncedly induced by CNT–COOH and CNT–OH. This is because the functional groups can form electrostatic interactions, H-bonds, and salt bridges with the Aβ peptide, which results in a reduction in the number of inter-peptide H-bonds and potentially the prevention of abnormal aggregation of the Aβ peptide.111 A similar mechanism was identified in the inhibition of hIAPP aggregation by fullerenol (C60–OH), suggesting that functionalisation can effectively modify the performance of nanosurfaces in preventing amyloid formation.112
The inhibition mechanisms for the amyloid aggregation were also revealed for other nanomaterials, such as metal–phenolic networks (MPNs)113 and covalent organic frameworks (COFs),114 possessing porous structures, metal sites or ionic functional groups to enhance the intermolecular interactions between nanomaterials and amyloid peptides. Such an enhanced intermolecular interaction is crucial for the inhibitory effect of nanomaterials on amyloid aggregation. Conversely, nanomaterials with a relatively weak attraction to proteins may either promote or inhibit protein aggregation.115,116 Specifically, protein aggregation can be promoted at a high protein/nanomaterial ratio but inhibited at a low protein/nanoparticle ratio. This could potentially clarify the complex size effect of nanomaterials on their ability to inhibit protein aggregation. For example, the 5 nm gold nanoparticles can inhibit the Aβ aggregation, whereas those of 20 nm or larger induced the formation of shorter protofibrils or β-sheet-rich structures, thereby promoting the aggregation.117 MD simulations of Aβ peptide adsorption onto Au(111) demonstrated that Aβ tended to relax into the flat and elongated conformers that are reminiscent of those observed in fibrils. These concentrated and fibril-like conformers of Aβ peptides on Au(111) could act as seeds to trigger the fibrillation process. On the other hand, small-sized Au nanoparticles (e.g. 5 nm) can selectively bind to the hydrophobic core of the Aβ peptide, which was not observed in the Aβ-Au(111) system, thereby supressing Aβ aggregation.118 The promotion effects on protein aggregation have also been observed in gold nano-butterflies, which represent a tool for the manipulation of biological structures, particularly LLPS droplets in biological systems. Unlike conventional spherical or rod-shaped nanoparticles, gold nano-butterflies, with their unique butterfly-like morphology, have been shown to induce LLPS in systems such as human IgG.119 This phenomenon is attributed to the concave structures interacting with the LLPS precursor beads. However, the detailed mechanistic insights into such protein aggregation remain elusive. Further attempts are warranted to predict the aggregation states of a specific protein upon contacting with nanomaterials, given the promising application of controlling protein aggregation in disease therapy.120,121
3.2.2. Inhibition of protein activity.
The regulation of protein activity is an important strategy for disease therapy in both conventional drugs and nanodrugs. It has been found that some nanoparticles can effectively inhibit protein activity through their various interactions with proteins at the nanosurfaces. MD simulations have been performed to provide an atomic insight into the inhibition mechanism with detailed intermolecular interactions and atomic information. In general, there are two possible mechanisms for the inhibitory effect of nanoparticles on protein activity. Nanoparticles can either bind to the active sites or disrupt protein conformations to interfere with its activity.
The α-chymotrypsin (α-ChT) is a representative example that has been used to study the inhibitory effect of nanoparticles on protein activity. MD simulations on the adsorption of α-ChT on pristine and carboxylated CNT(14, 14) (Fig. 7a) demonstrated that the adsorbed α-ChT can maintain its conformation but took different orientations on pristine and carboxylated CNTs.122 The active site is far from the pristine CNT surface but close to those of carboxylated CNTs (Fig. 7b), suggesting that the carboxylated CNTs can inhibit the activity of α-ChT by blocking the active site of α-ChT to prevent substrate entry without significantly disrupting the protein structure. Such different effects of pristine and carboxylated CNTs arise from their intermolecular interactions with the α-ChT. For pristine CNT, van der Waals and hydrophobic interactions are dominant, showing non-competitive characteristic. However, the electrostatic interactions between the carboxylate groups and the cationic residues around the active site are dominant in the adsorption of α-ChT onto carboxylated CNTs, promoting the selective binding of the active site with carboxylated CNTs. A recent study suggested that the pristine CNTs may also block the active site of α-ChT.123 These discrepant results may arise from the different size or curvature of CNTs. The (10, 10)-CNT with a small size and large curvature can match well with the active pocket of α-ChT. On the other hand, the (14, 14)-CNT has a larger size and smaller curvature, which could hinder the selective binding between CNTs and the active pocket of α-ChT.
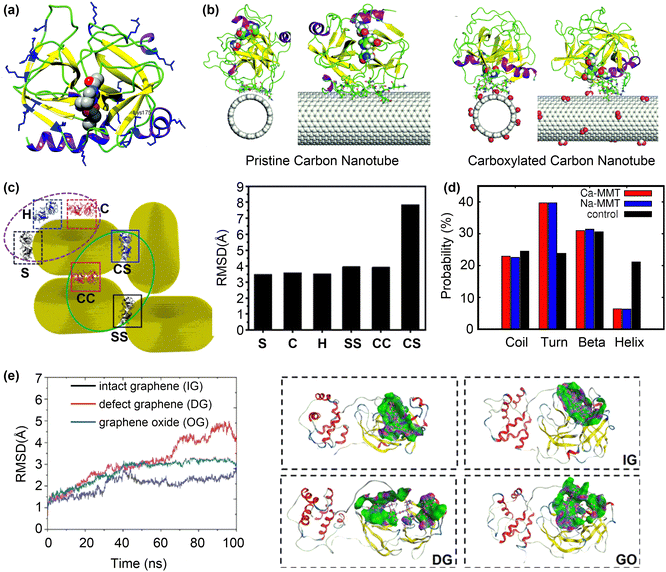 |
| Fig. 7 Inhibition of protein activity by surface adsorption. (a) The structure of α-ChT with the active site residues represented by the vdW spheres. (b) The final snapshots for the adsorption of α-ChT on pristine and carboxylated carbon nanotubes. Reproduced with permission from ref. 122. Copyright 2017, the Owner Societies. (c) Different interaction types of AuNPs with MMP-9 protein and the corresponding RMSD of adsorbed MMP-9 protein on AuNRs compared to the wild-type one. Reproduced with permission from ref. 124. Copyright 2023, American Chemical Society. (d) Probability of the secondary structures in S protein in control, Na-MMT and Ca-MMT systems. Reproduced with permission from ref. 125. Copyright 2022, American Chemical Society. (e) Backbone RMSD and structural change of Mpro upon contact with different graphene systems. Purple, green, and blue represent the H-bond region, hydrophobic region, and polar region, respectively. Reproduced with permission from ref. 126. Copyright 2022, American Chemical Society. | |
Changing the structure of nanoparticles not only affects their size/shape complementarity with the active pockets of proteins, but also induces additional intermolecular interactions that enhance their binding to proteins. This phenomenon has recently been investigated with regard to the binding between planar/curved polycyclic aromatic hydrocarbons and lysozyme (LSZ).127 The curved corannulene and its planar analogue perylene can readily bind to the active pocket of LSZ with moderate binding free energies (ΔG°) of −7.7 and −4.0 kcal mol−1, respectively. The stronger ΔG° for curved corannulene mainly arises from the electrostatic interactions due to the asymmetrical charge distribution on its convex and concave surfaces. As a result, the inhibitory effect of curved corannulene on the LSZ activity is stronger than that of perylene.
The activity inhibition mechanism by selective binding of nanoparticles to the active site/pocket of proteins has been also observed for other proteins such as matrix metalloproteinases128 and tyrosine phosphatases.129 Due to their versatile and tunable surface properties, nanoparticles can bind to other regions of proteins and cause severe vitiations of protein structures to supress the protein activity. For example, gold nanorods (AuNRs) can sufficiently inhibit tumour metastasis by regulating the activity of matrix metalloproteinase (MMP-9), a protein associated with tumour invasion and metastasis.124 The activity of MMP-9 decreases after exposure to Au nanoparticles, arising from the change in the secondary structure of MMP-9 caused the interactions with AuNRs, as evidenced by the large RMSD values of adsorbed MMP-9 with respect to the wild one (Fig. 7c).
The disruption of protein structures to inhibit their activity is useful for antiviral applications. For instance, the spike (S) glycoprotein of SARS-CoV-2 plays a crucial role in its invasion of host cells via the interaction with human angiotensin-converting enzyme. Recent MD simulations125 suggested that the S protein undergoes structural changes in the presence of montmorillonite nano clay surfaces (Na-MMT and Ca-MMT). An analysis of the secondary structure of adsorbed S protein revealed a decrease in the helical content and an increase in the average probability for turns in the presence of MMT surfaces (Fig. 7d). Such severer changes in the secondary structure arise from the strong interactions between MMT surfaces and the three chains of S protein. Given that most of the contacting residues with MMT surfaces come from the N-terminus and the receptor binding domain, the disrupted S protein may lose its function in viral invasion.
In addition to interfere with the whole protein structure, nanoparticles may destroy only the active pocket of protein to inhibit its activity. MD simulations revealed that graphene-related materials including intact graphene (IG), defect graphene (DG), and graphene oxide (GO) can inhibit the activity of Mpro of SARS-Cov-2 and its expression.126 Furthermore, DG and GO had stronger inhibitory effects due to their more intensive interactions with the Mpro, according to the larger RMSD values, interaction energies and contact areas in the “Mpro-DG” and “Mpro-GO” systems than in the “Mpro-IG” system. Conformational change analysis showed that the whole active pocket was adsorbed onto the surfaces of DG and GO with large RMSD values of 4.1 and 3.0 Å (Fig. 7e), respectively. On the other hand, only one terminal of the active pocket was adsorbed onto the surface of IG. These results suggest that more residues of the active pocket are adsorbed onto the surfaces of DG and GO than that of IG, changing the posture and conformation of the active pocket and thus inhibiting the activity of Mpro.
3.2.3. Immobilisation of protein.
Proteins can be protected from denaturation and biological degradation through immobilization onto nanosurfaces, making nanoparticles promising carriers for functional proteins like enzymes, antigens, and antibodies with various medical applications.130–132 To preserve the bioactivity of proteins, the adsorbed proteins must retain their native structures and take proper orientations on nanosurfaces, leaving their active sites and/or receptor binding domains accessible. As discussed above, the interactions between nanoparticles and proteins play significant roles in determining the activity of proteins upon deposition onto nanosurfaces.
Enzymes are representative proteins that can be immobilized on nanosurfaces to enhance their catalytic activities. MD simulations suggested a surface-dependent manner for the orientation of adsorbed acetylcholinesterase from Torpedo californica (TcAChE) on carbon nanotubes.133 On amino-functionalised CNTs, the active site and substrate tunnel of TcAChE were close to the surface due to the electrostatic interaction between the positively charged surface and residues of TcAChE. Conversely, the active site and substrate tunnel of TcAChE took an opposite orientation on pristine or carboxylate-functionalised CNTs. Because the adsorbed TcAChE is stable and its active site is not blocked by nanosurfaces, such different orientations result in different enzyme activity. The adsorption of other proteins, such as antigens and antibodies, onto nanosurfaces in order to improve their functions has also been investigated using MD simulations.134 For example, immunoglobulin G (IgGs) adsorbed on graphene layers can maintain their native structures with negligible changes in the secondary and tertiary structures.135 The robustness of IgGs is attributed to its large size, resulting in high internal strength, thereby making them resistant to structure disruption caused by surface adsorption. In addition, IgGs can strongly bound to graphene with preferable vertical orientations, where the antigen binding fragments are accessible. These results render graphene an excellent candidate for constructing artificial antibodies.
Various interactions contribute to stabilizing protein adsorption on nanosurfaces and determining protein orientation. Among these interactions, the electrostatic interaction usually dominates over others, such as van der Waals, hydrophobic/hydrophilic, π–π stacking, and cation–/anion–π interactions. Accordingly, tuning the surface charge of nanoparticles has proven an effective strategy to regulate the orientation of proteins on nanosurfaces.136 The orientations of the adsorbed α-Syn on gold nanoparticles can be regulated by coating cationic or anionic ligands, which prefers the C-terminus and N-terminus, respectively.137 In addition to coating ligands, variation of pH can alter the charge states of nanoparticles and proteins, influencing their interactions and thus changing the protein orientation on nanosurfaces. The pH-induced protein reorientation has been reported on silica nanoparticles, resulting from the change in surface electrostatic interactions between silica and proteins.138,139
Although MD simulation is useful to find the preferable protein adsorption on nanosurfaces, modelling protein-surface interactions is very time-consuming, particularly when the protein is large and take a large number of possible orientations. To efficiently screen nanoparticles for protein immobilisation, simplified simulation models that are able to predict the preferable protein orientation are particularly required. In this regard, the method based on a united-residue modelling of protein–surface interactions is promising, which has recently been applied to predict the orientation of the SARS-CoV-2 spike protein receptor binding domain (RBD) on a few prototypical surfaces.140 It was revealed that the antigen orientation is unfavourable for antibody recognition when immobilised on either a positively or negatively charged self-assembled monolayer (SAM). Conversely, immobilisation on the negatively charged silica yields favourable orientation compatible with antibody recognition. These results suggest that the orientation of immobilised proteins may depend not only on the charge states but also on the charge density of nanosurfaces. This simple yet effective model is highly valuable in predicting protein orientation on nanosurfaces. However, improving the prediction accuracy and conducting experimental verification of protein orientations are necessary to enable medical applications through protein immobilisation, which remains a challenging task.
3.2.4. Formation of protein corona.
When nanoparticles enter an organism, they quickly bind to components in body fluids or blood, particularly proteins, forming a composite structure of nanoparticles and proteins known as “protein corona”. The formation of protein corona alters the intrinsic properties of nanoparticles and influences their biological characteristics, such as the blood circulation time and targeting ability.141 These biological properties of protein corona significantly depend on the protein components adsorbed on nanoparticles. Due to the complex structure of the protein corona and its surrounding microenvironment, protein corona has still been poorly understood, although a large number of analytical and characterization techniques, such as dynamic light scattering, plug-in centrifugal sedimentation, fluorescence correlation spectroscopy, and molecular dynamics simulations have been applied.142
Studying the protein–nanoparticle and protein–protein interactions helps to understand the structure of the protein corona and its biological effects, which is crucial for nanodrug design. As discussed above, these interactions significantly depend on the surface properties of both proteins and nanoparticles, and the physiological environment surrounding them, such as pH and salinity.143 A “hard” or “soft” protein corona can be formed according to the strength of protein–nanoparticle interactions. For instance, a “hard corona” is formed on the surface of super-paramagnetic iron oxide nanoparticles (SPIONs), with a strongly adsorbed monolayer of bovine serum albumin (BSA). MD simulations showed that the BSA molecules can be gradually adsorbed onto a SPION nanoparticle of 6 nm until reaching a maximum of 10 protein molecules. The excess proteins retained in solution rather than forming a second layer of adsorbed proteins on the SPION surface (Fig. 8a). These results suggest that the preformed monolayer of BSA can serve as a protective shell for the SPION surface.144 Likewise, the monolayer BSA shell has been found on L-/D-chiral gold nanosurfaces with similar adsorption strength but different orientation.145 These adsorbed BSA proteins (corona) may influence the cellular uptake and protect the targeting ability of nanodrugs.
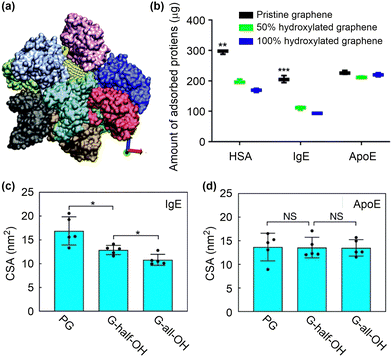 |
| Fig. 8 Protein corona formation on nanosurfaces. (a) Snapshot of ten BSA proteins adsorbed onto a nanoparticle. Reproduced with permission from ref. 144. Copyright 2016, CC BY-NC 4.0. (b) In vitro analysis of the number of proteins adsorbed on functionalised graphene. (c) and (d) The contact surface area between IgE or ApoE and the graphene with different surface modifications. Reproduced with permission from ref. 146. Copyright 2019 CC BY 4.0. | |
Because surface adsorption may alter protein structure, the protein corona on nanosurfaces can be regulated by tuning surface structures. It has been shown that the apolipoprotein-cIII (apo-c3) undergoes denaturation upon adsorption to graphene oxide, driven by the large electrostatic interactions between the positively charged or polar residues and the oxygen-containing functional groups.147,148 Structural analysis showed that the α-helix character decreased with the formation of β-turns, which could promote subsequent aggregation of serum proteins, thereby complicating the predesigned targeting ability of nanodrugs. Conversely, the azide- and alkyne-double functionalised GO (C2GO) did not induce notable changes in the structure of apo-c3. This is because the binding between C2GO and apo-c3 is dominated by van der Waals interactions dispersed over the entire protein, which cannot compromise the intra-protein interactions that maintain the tertiary structure of apo-c3. As a result, the lipid-binding function is preserved in C2GO-adsorbed apo-c3, resulting in the preferential uptake of corona-coated C2GO to corona-coated GO by J744 cells.
Not only the structures of adsorbed proteins but also the protein species can be changed by tuning surface structures. The influences of hydrophilic functional groups on protein adsorption, conformation and residues have been investigated by proteomics and all-atom MD simulations (Fig. 8b).146 The study revealed that the incorporation of hydrophilic hydroxyl groups can considerably suppress the adsorption of opsonins, such as immunoglobulin E (IgE), by reducing the contact surface area and the amount of adsorbed residues (Fig. 8c). This effect, however, has only a minimal impact on the adsorption of apolipoprotein E (ApoE) (Fig. 8d). Theoretical analyses further suggested that the Janus-type distribution of hydroxyl groups on nanosurfaces might be the best choice to maximize the adsorption of ApoE proteins. Based on these results, a nanocarrier was fabricated by applying a layer of ApoE protein as a pre-coating on the surface. This protein layer exhibited resistance against complementary proteins and immunoglobins, leading to enhanced blood circulation time and improved delivery efficiency to tumour tissues. These findings imply that controlling protein corona is becoming increasingly crucial for achieving optimal function of nanodrugs, rather than eliminating it completely. Poly(ethylene glycol) (PEG) has been widely used to tune interactions of nanoparticles with proteins. By tuning the architecture of shape persistent amphiphilic bottlebrush block copolymer building blocks, the surface topography of PEGylated nanoparticles can be precisely tailored, which further influence their protein adsorption and cell uptake performances. Specifically, the well-tailed nanoparticles with rough surface and narrow terminal PEG block brush width shows a low protein adsorption but a high cell uptake compared to conventional PEGylated nanoparticles with a high-density PEG shell.149 Despite the progress made so far, it remains difficult to precisely predict the formation of protein corona based on the physicochemical properties of nanoparticles and the types of proteins involved.150–152
3.2.5. Summary.
In conclusion, protein adsorption on nanosurfaces can directly or indirectly influence the therapeutic efficacy of nanodrugs. MD/CGMD simulations have been extensively employed to investigate the interaction between proteins and nanosurfaces, which are influenced by the properties of both the proteins and the nanosurfaces. It has been demonstrated that nanosurfaces may inhibit or promote protein aggregation, protect or destroy protein structure or active pocket, depending on the competition between inter-/intro-protein interactions and protein–nanosurface interactions. All of these protein states are associated with their respective medical functions. For example, the aggregation of Aβ should be prevented, whereas that of STING should be promoted, in the development of nanodrugs for relevant diseases. The antigens and antibodies should retain their native structure when they bind to nanoparticles. Conversely, nanomaterials with antiviral properties are expected to disrupt the structures of viral proteins. In addition, a protein shell (protein corona) can be formed on nanosurfaces, which may alter the pre-designed functions of nanodrugs. It is therefore of great importance that protein–nanosurface interactions should be precisely engineered in the future in order to facilitate the discovery of nanodrugs. MD simulations have indicated that a variety of factors, including size, shape, concentration, charge, and surface functionality, can affect the orientation and binding strength of protein adsorption on nanosurfaces. However, it remains unclear how to simultaneously optimise these properties to regulate the species and amount of protein adsorbed on nanosurfaces. Further development in computational methods, potentially integrating with machine learning techniques, is therefore warranted to accelerate precise design of nanodrugs.
3.3. Adsorption of functional ligands on nanosurfaces
Anchoring functional ligands on nanosurfaces is often necessary to achieve the desired therapeutic efficacy of nanodrugs. The anchored functional ligands can alter the surface structures and properties that related to medical functions, such as adsorption of small molecule drugs and proteins, supramolecular binding with targeted sites, catalytic performance, as well as stability and biocompatibility.153 These properties depend not only on the binding strength between functional ligands and nanosurfaces but also on the distribution and orientation of functional ligands on nanosurfaces.
3.3.1. Adsorption structure.
Peptides are representative functional ligands that may improve the stability, biocompatibility, and targeting ability of nanodrugs. The sequence of peptides plays a crucial role in determining their binding strength with nanoparticles. For example, rearranging the sequence of the Pd4 peptide (TSNAVHPTLRHL) to HHTSNAVPTLRL (Fig. 9a) increased the binding free energy of the peptide with the Au(111) surface from −7.5 to −8.5 kcal mol−1.154 Although there is no direct correlation between the binding affinity and the colloidal stability (size) of resulting nanoparticles, the number of anchor residues for peptide adsorption may govern the stability of peptide-capped NPs. Designing peptide sequences with more anchor residues and small conformational entropy was suggested to obtain stable nanoparticles. However, for adsorption of targeting peptides such as the pH low insertion peptide (pHLIP), the targeting residues must remain in solution. Therefore, the adsorption structure and binding strength of peptide–NP conjugates should be reliably described. Several force fields used in MD simulations have been compared in their performances to describe the pHLIP adsorption on gold nanosurfaces. The CHARMM36 and OPLS-AA are good choices that are able to correctly describe the conformation and folding of pHLIP upon interacting with Au nanosurfaces.155
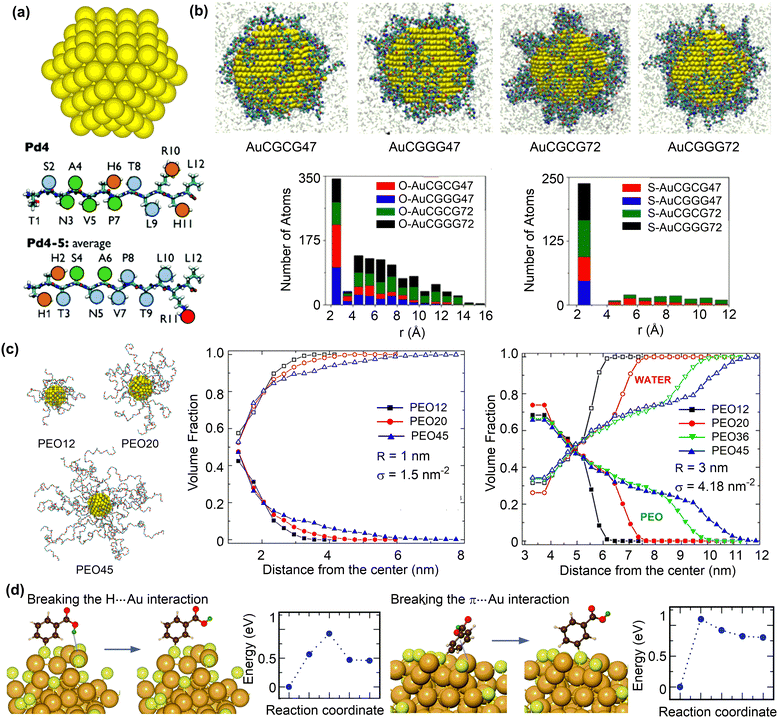 |
| Fig. 9 Structures of ligand functionalised nanosurfaces. (a) Au nanoparticle (AuNP) and representative peptide ligands. Reproduced with permission from ref. 154. Copyright 2017, the Royal Society of Chemistry. (b) Configurations of peptide-functionalised Au nanoparticles and histograms of the distance of oxygen/sulfur atoms to AuNP surfaces with different ligand sequences and surface coverage. Reproduced with permission from ref. 156. Copyright 2019 American Chemical Society. (c) Snapshots of 1 nm AuNP grafted with PEO chains of 12, 20, and 45 repeating units. Volume fractions of PEO (solid symbols) and water (open symbols) as a function of the radial distance from the centre of the nanoparticle for PEO chains of different lengths. r and σ represent nanoparticle diameter and ligand coverage, respectively. Reproduced with permission from ref. 157. Copyright 2020 American Chemical Society. (d) Structure and energy changes for ligand reorientation on the Au68 surface. Reproduced with permission from ref. 158. Copyright 2020, CC BY 3.0. | |
The adsorption strength and distribution of peptides on nanosurface can be influenced by the grafting density. The reactive force field MD simulations were conducted to study the interaction between gold nanoparticles (AuNPs) and short-chain peptides (CGCG and CGGG) at different grafting densities (Fig. 9b).156 The short-chain peptides can be tightly adsorbed onto gold nanoparticles in a slightly facet-dependent manner. The strong binding interaction between these peptides and AuNPs can be attributed to both the Au–S bonds and the polarizable oxygen and nitrogen atoms that strongly interact with the gold surface (Fig. 9b). Importantly, a strong binding was found at a low grafting density, because the normalized surface coverage of peptide atoms interacting with AuNP surface is larger at a low grafting density than at a higher density. These structural features suggest that the orientation of adsorbed peptides can be rationally designed by changing the grafting density.
Polymeric ligands can also be applied to protect nanoparticles, for which the chain length and grafting density may influence the adsorption structures and properties. The structural properties of gold nanoparticles grafted with different lengths and grafting densities of polyethylene oxide (PEO) ligands were investigated using MD simulations.157 At a low grafting density, both the PEO chains and water molecules reside in the vicinity of the gold nanosurface (Fig. 9c). Conversely, a dense and partially dehydrated PEO brush is formed at a high grafting density. The formation of dehydrated PEO brush is essential to protect the inner core of nanoparticles from protein adsorption, which is also affected by the tail flexibility. In this regard, long PEO chains with a grafting density larger than the critical value, which depends on the curvature of nanoparticles and the radius of gyration of a polymer chain in solution, can be favourable in preventing protein adsorption.
In addition to peptide and polymeric ligands, organic ligands, such as thiols, phosphines, and alkynyls, have been reported to protect metal clusters with ten to a few hundred atoms. The atom-precise structure of the monolayer protected clusters (MPCs) is crucial to understand structure–property relationships and tune the structure for a desired function.159 For example, MD simulations and DFT calculations were conducted to investigate the structures of meso-mercaptobenzoic acid (m-MBA) protected gold nanoclusters using.158 The protection arose from the inter-ligand hydrogen bonding, H-bond-like Au⋯H bonding, and π⋯Au bonding interactions between m-MBA ligands and gold nanoclusters in addition to the strong Au–S bonds. Meanwhile, breaking the Au⋯H and π⋯Au bonding interactions are not very difficult, demonstrating the dynamic nature of the metal sites that are either protected or available.160 Given the importance of structure prediction of MPCs, a number of methods have been proposed,159 such as the numerically effective method based on a set of reference structures.161 This method can accurately predict the interface structures of Au/Ag nanoclusters protected by thiols, phosphines or even mixed ligands.
Ligand functionalisation of other nanosurfaces, such as metal oxides162,163 and carbon nanomaterials,164,165 can likewise improve their biocompatibility and targeting ability. Classical MD simulations were conducted to investigate the adsorption of collagen tripeptides (CTPs) on TiO2 nanosurfaces, which were synergistically affected by the surface topographies, hydroxylation and bioactive ions.162 The free energy changes and radial distribution functions demonstrated that hydroxylated TiO2 with grooves or ridges and aggregated bioactive ions can reduce the adsorption energy barrier of CTPs on TiO2. Additionally, the formation of numerous hydrogen bonds between CTPs and hydroxyls on TiO2, along with the bioactive ions, synergistically improve the stability of the CTPs on TiO2. However, too dense packing of targeting ligands on nanosurfaces should be avoided to maintain the specific binding with targeted receptors. Co-adsorption of other biocompatible ligands such as PEG is a useful strategy. For example, folic acid can be strongly adsorbed with little changes in adsorption energies until reaching the full coverage on the TiO2 surface.163 By introducing the methylated PEG ligands, the NP–folic acid interaction can be reduced and folic acid ligands are well separated with optimal targeting activity.
3.3.2. Effects on drug adsorption.
The functional ligands can affect the drug loading or release of nanosurfaces by directly interacting with drug molecules or indirectly altering the surface properties. An enhanced drug adsorption behaviour with more negative adsorption energies was observed for folic acid-modified carbon nitride compared to the pristine carbon nitride nanosheet. This was attributed to the intermolecular interactions between drug molecules and folic acid ligands.166 Similar results were found for the adsorption of doxorubicin onto peptide-coated gold nanoparticles. The “sandwich” configuration of AuNP–doxorubicin–peptide stabilises doxorubicin adsorption even in the physiological conditions. Such an adsorption stability is maintained because the tryptophan in the drug-binding peptide clip the drug molecule.167 However, the spontaneous dissociation of one doxorubicin molecule from the carrier would occur when increasing the doxorubicin
:
peptide ratio to 2
:
1 (Fig. 10a).168 Therefore, the ratio of drug molecules to functional ligands should be well tuned to balance the competitive drug adsorption on the limited number of sites.
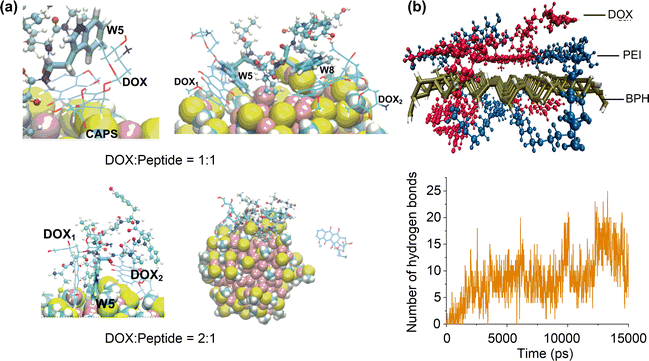 |
| Fig. 10 Effects of ligand functionalisation on drug adsorption. (a) Adsorption structures of doxorubicin (DOX) on peptide-functionalised Au nanoparticles. Reproduced with permission from ref. 167 and 168. Copyright 2020 Elsevier, Copyright 2022, CC BY-NC 3.0. (b) Adsorption structure of doxorubicin on polyethylenimine-functionalised phosphorus nanosheet and time evolution of the number of hydrogen bonds between doxorubicin and polyethylenimine. Reproduced with permission from ref. 169. Copyright 2021 Wiley-VCH. | |
Varying the ratio of drug molecules to functional ligands may cause the multi-layer adsorption of drug molecules on functionalised nanosurfaces because of the different drug–ligand and drug–nanosurface interactions. Simulations on a drug delivery system consisting of chitosan-gentamicin andcitrate-coated gold nanoparticles showed that the chitosan-gentamicin can partially replace the original citrate.170 Meanwhile, outer layers of adsorbed chitosan-gentamicin can be formed by changing the chitosan/getamicin weight ratio. The resulting conjugated nanoparticle system can effectively control and sustain the gentamicin release. A similar sustainable drug release mechanism was found in oleic acid modified ZnO nanoparticles,171 where the carfilzomib molecules can be adsorbed by entrapment among the oleic acids or by directly interacting with the reachable portions of the ZnO surface. It is anticipated that adsorbing different functional ligands on the nanosurface could modulate the binding modes with drug molecules,172 which may promote the formation of multilayer ligand–drug assemblies for a more controllable drug release.
Functional ligands not only provide additional interaction sites with drug molecules but also induce stimuli-responsive characteristics for drug adsorption and release on nanosurfaces.173 For instance, doxorubicin adsorption is enhanced on the poly(L-histidine) grafted carbon nanotube. In addition, both doxorubicin and poly(L-histidine) have different protonation states depending on the pH values.174 MD simulations indicated that the stably adsorbed doxorubicin molecules under neutral conditions can be released in response to acidic conditions, due to the increased electrostatic repulsion between protonated doxorubicin and poly(L-histidine). This pH-responsive drug adsorption and release behaviour was also found for doxorubicin adsorption on polyethyleneimine (PEI) functionalised black phosphorus (BPH) nanosheets.169 The doxorubicin molecules can be spontaneously adsorbed on the nanosurface (Fig. 10b), mainly due to the formation of π–π stacking and H-bond interactions between doxorubicin and PEI-BPH. These intermolecular interactions significantly decreases under acidic conditions, thereby promoting the release of DOX.
3.3.3. Effects on protein adsorption.
The presence of functional ligands on nanosurfaces may alter their adsorption affinities to proteins, thereby affecting the blood circulation and therapeutic efficacy of nanodrugs. Both experimental and computational studies have been conducted to understand the influence of ligand functionalisation on protein adsorption behaviour of nanoparticles. The weakly adsorbed ligands such as citrates can be replaced by proteins, exposing the native surface of NPs for protein adsorption. Conversely, protein cannot directly interact with nanosurfaces functionalised by strongly adsorbed ligands. Recent MD simulations have demonstrated such phenomena, where proteins binding to the physisorbed citrate gold surface showed more contact sites, higher interfacial energy, and greater conformational changes than to the chemisorbed GSH surface.175 Due to the little influence of weakly adsorbed ligands on protein adsorption, introducing functional groups such as –SH is often necessary to regulate protein adsorption behaviour.
As introduced in Section 3.2.4, PEGylation is an effective strategy to suppress the formation of “protein corona” on nanoparticle surfaces.149,176 However, the coating of PEG ligands cannot completely prevent protein adsorption.177 A mixing ligand strategy has been proposed for nanosurface functionalisation that can mimic the charge composition of proteins in the bloodstream, such as serum albumin (HSA) and transferrin, to prevent protein adsorption. Compared to conventional PEG coatings with negatively charged (–COOH), positively charged (–NH2), or neutral (–OCH3) terminal groups, mixed coatings can significantly reduce the binding affinity of nanosurface to proteins and achieve the anti-biofouling function. Such mixed coatings can be achieved by simultaneously introducing neutral, negatively and positively charged PEG chains based on the charge distribution of proteins.178 Similar strategies can be applied for the surface functionalisation of nanoparticles by other ligands such as zwitterionic polymers and peptides.179 However, completely eliminating protein corona formation may reduce the cellular uptake of nanodrugs, making it crucial to prevent non-specific protein adsorption. This will ultimately require simple but general criteria to describe protein adsorption on functionalised nanosurface without comprehensive experiments and MD simulations. To this end, an in silico screening method has been proposed on the basis of ligand hydration and dynamics.180 By considering systems consisting of hydrophilic or hydrophobic chains or varying length and mixtures, it has been shown that the ligand hydration and dynamics can synergistically prevent protein adsorption on silica surface. Due to the generality and transferability to other ligands and surfaces, this method is quite promising for future tailoring nanoparticle surfaces for controlling protein adsorption.
3.3.4. Summary.
In summary, functional ligands anchored on nanosurfaces are of critical importance in enhancing the therapeutic efficacy of nanodrugs. Ligands, such as peptides and polymer chains, have the potential to alter surface characteristics, which in turn affects the adsorption of drugs and proteins, the catalytic performance, and the biocompatibility. Extensive multi-scale computational studies have been conducted to elucidate important features governing the adsorption of functional ligands on nanosurfaces. These processes are affected by the intrinsic properties of the nanoparticles, such as their size and curvature, as well as the characteristics of the ligands, such as their type, length, and anchoring density. Functional ligands can affect the loading and release of drugs by interacting with drug molecules or modifying surface properties. For example, folic acid-modified carbon nitride exhibits enhanced drug adsorption as a result of intermolecular interactions. The presence of functional ligands on nanosurfaces can alter protein adsorption affinities, which in turn can influence the circulation and efficacy of nanodrugs. Strategies such as PEGylation are employed to regulate protein adsorption, but a balance is essential to prevent non-specific adsorption while maintaining efficient cellular uptake. Since surface functionalisation is typically necessary, it is of crucial importance to precisely engineer ligand properties in accordance with the nanoparticle. It is therefore essential to develop an efficient in silico screening method to facilitate the optimisation of these properties in order to control the interfacial interactions between functionalised nanoparticles with biological entities. However, given the diverse optimisation space, comprising combinations of ligands and nanoparticles properties, such a method has yet to be developed.
3.4. Other medical functions enabled by surface adsorption
3.4.1. Biosensor.
Adsorption of molecules on nanosurfaces may alter the electronic structures of nanomaterials, which can be converted into electrical signals, enabling their medical applications as biosensors for the detection biomarkers of diseases, such as uremia and cancer. The detection limit and sensitivity depend on the binding affinity of biomarkers to nanosurfaces and the change in electronic structures of nanomaterials upon biomarker adsorption. These properties can be readily accessed by DFT calculations. For example, protein tyrosine/tryptophan nitration can alter protein function and properties, playing significant roles in the onset and progress of various chronic disorders. DFT calculations revealed that ZnO nanoparticle ([ZnO]12) can stably adsorb both of the pristine and nitrated tyrosine/tryptophan with binding energies ranging from −19.5 to −29.1 kcal mol−1.181 Interestingly, the electronic structure of ZnO nanoparticle changed little upon adsorption of pristine tyrosine/tryptophan. Conversely, the adsorption of nitrated tyrosine/tryptophan significantly altered the electronic structure of ZnO nanoparticle with the HOMO–LUMO gap decreased by approximately 1 eV (∼50%). Such a high affinity and large change in electronic structure of ZnO nanoparticle in response to nitrated tyrosine/tryptophan demonstrate its potential for the early diagnosis of different chronic disorders related to protein tyrosine/tryptophan nitration. Similarly, DFT calculations have demonstrated the potential application of Si nanowires (SiNW) for uremia diagnosis.182 The pristine SiNW showed a large change in the band gap upon adsorption of urea, a biomarker of uremia. However, the binding energy between SiNW and uremia is too weak (−6.0 kcal mol−1), which would lead to a high detection limit. Introducing metal atoms can enhance the binding affinity of SiNW to urea but the change in band gap upon urea adsorption is too small (<0.08 eV), resulting in a low detection sensitivity.
To achieve reasonable detection limit and sensitivity of biosensors for disease diagnosis, it is crucial to rationally design nanostructures that influence their binding affinity to biomarkers and electronic structures. Heteroatom doping and defect engineering are common methodologies to tune the electronic structures of nanomaterials. For instance, Ti-doping can significantly enhance the adsorption affinity of dopamine to pristine boron nitride and the change in energy gap upon dopamine adsorption, thereby improving the performance for dopamine sensing.183 Similarly, introducing structural defects is useful to enhance the adsorption of gaseous lung cancer biomarkers in exhaled breath to armchair stanene nanoribbons (ASnNR).184
3.4.2. Toxin removal.
In addition to the delivery of therapeutic molecules, nanoparticles can be useful for the removal of toxins from the body through surface adsorption. For example, hemoperfusion, which is based on the adsorbent–toxin interactions, has been widely employed to purify blood in patients suffering from acute liver and renal failure. The purification performance is expected to be significantly influenced by the intermolecular interactions between adsorbents and toxins, such as bilirubin, creatinine, and uric acid, despite that other factors including surface area, stability, and biocompatibility should also be considered for practical clinical applications. A composite adsorbent consisting of cellulose acetate and graphene oxide was designed for the effective removal of creatinine, uric acid, and bilirubin.185 MD simulations demonstrated that the adsorption free energies of creatinine and uric acid on this composite were −3.2 and −7.8 kcal mol−1, respectively, indicating their preferential adsorption on the composite surface.
The introduction of additional interaction sites is an effective strategy for enhancing the trapping ability of nanomaterials for blood toxins. In this regard, MOFs are highly promising due to their porous structures, which provide versatile interaction sites and exclude proteins from entering the pores. A recent study on the bilirubin removal ability of PCN-333 and MOF-808 suggested that PCN-333 exhibited pronounced performance with high adsorption capacities for bilirubin.186 This is attributed to the strong adsorption energy of bilirubin into these MOFs, which arises from the π–π interactions between the aromatic rings of bilirubin and organic ligands in these MOFs. Similarly, the π–π, hydrogen-bonding and electrostatic interactions are crucial for stabilising p-cresyl sulfate (a uremic toxin) adsorption in NU-1000.187 These results demonstrate the crucial role of understanding the adsorbent–toxin interactions in the design of blood purification nanomaterials for clinical hemoperfusion therapy.188–190
4. Supramolecular interaction-based nanodrugs
In addition to surface adsorption, nanomaterials can form supramolecular interactions with biological entities, such as cell membranes and cellular receptors. These interactions enable various medical functions of nanomaterials, which are termed supramolecular interaction-based nanodrugs. For example, the cellular uptake of nanomaterials usually occurs through a passive or receptor-mediated internalisation process,191,192 wherein the supramolecular interaction plays a vital role. In addition, the metal–peptide complex or nanomaterial-based artificial antibody193 can be rationally designed for precise supramolecular recognition, enabling the realisation of their medical functions. In order to further advance the design of supramolecular interaction-based nanodrugs, it is necessary to have a clear understanding of such supramolecular interactions through computational studies,39,194 which are often time-consuming.
4.1. Passive supramolecular interaction
Previous simulation studies have revealed that the translocation of nanoparticles (NPs) through lipid bilayer membranes is critically determined by intrinsic properties of NPs, including size, shape, surface charge, and surface modification.195–197 Most of the previous simulation studies used membrane models composed by no more than three lipid species, which are simple compared to the real plasma membranes. To consider the effect of membrane complexity, the uptake pathways of AuNPs were comparatively studied using two membrane models, the simple negatively charged lipid bilayer model and the plasma membrane model.198,199 The simple model consists of a 1
:
3 ratio of self-assembled distearoyl phosphatidyl glycerol and distearoyl phosphatidyl choline lipids. In contrast, the plasma membrane model contains 30 mol% cholesterol.200
MD simulations suggested that AuNPs have markedly different transmembrane kinetics.199 The neutral 2 nm AuNPs have a free energy barrier of 43.47 ± 4.70 and 260.85 ± 6.04 kJ mol−1 when translocating through the simple membrane and the plasma membrane, respectively. Furthermore, the locations of AuNPs at energy minima differ a lot in the two membranes (Fig. 11a–c). The different transmembrane kinetics can be mainly attributed to the variations in hydrophilicity, thickness, and rigidity between the membrane models. The plasma membrane, which contains a substantial cholesterol content, exhibits a higher degree of hydrophobicity, thickness, and rigidity, hindering the translocation of AuNPs.
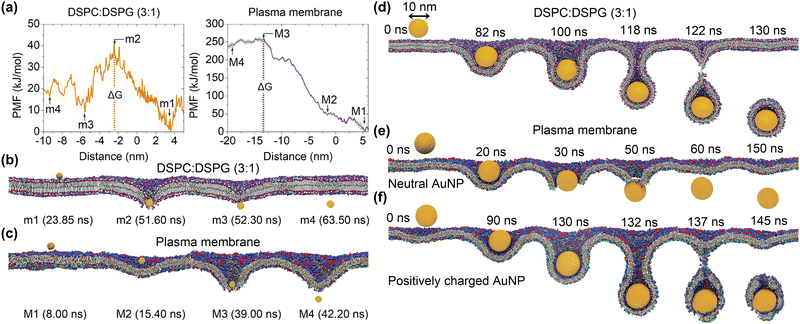 |
| Fig. 11 Cellular uptake of Au nanoparticles using different membrane models. (a) PMFs of a 2 nm AuNP in two lipid bilayer models. (b) and (c) Representative snapshots of the 2 nm AuNPs translocating through the DSPC/DSPG and the plasma membrane models. (d) and (e) Representative snapshots of internalisations of a neutral 10 nm AuNP in the DSPC/DSPG bilayer and the plasma membrane models. (f) Uptake of a positively charged AuNP with the surface charge density (σ = +0.4). Reproduced with permission from ref. 198. Copyright 2019 American Chemical Society. | |
The transmembrane pathways of NPs can be altered by the membrane complexity. Steered MD simulations using simple membrane models suggested that nanoparticles with diameters larger than 10 nm usually transfer the membranes via the endocytosis mechanism.197 As shown in Fig. 11d, the neutral 10 nm AuNP is internalised via the endocytic pathway in the case of simple bilayer membrane models. However, the direct translocation of the neutral 10 nm AuNP was observed in the case of the plasma membrane model, in which the AuNP is not fully wrapped by the membrane (Fig. 11e). Interestingly, when the AuNP is positively charged, the endocytic pathway was observed in the case of the plasma membrane model (Fig. 11f). These different membrane uptake pathways can be understood in terms of the Helfrich theory of membrane elasticity,
|  | (1) |
where
Rmin is the lower limit of NP radius below which the NP cannot be endocytosed,
kc is the bending rigidity of the membrane, and
w is the attractive adhesion energy per unit area between the membrane and the NP.
The role of surface charge on the internalisation of AuNPs through an idealized plasma membrane was further studied through CGMD simulations.198 The results suggested that all the three surface-modified 2 nm AuNPs can directly cross the membrane without forming vesicles as their hydrodynamic sizes are less than the minimum size (Rmin ≈ 5 nm) required for endosome formation. The free energy barriers for the internalisation of these NPs through the plasma membrane are 279.63 ± 3.70, 388.41 ± 2.29 kJ mol−1, and 416.86 ± 5.93 kJ mol−1, respectively. For all three NPs, the highest energies were observed when the NPs are passing through and about to leave the membrane, in contrast to the internalisation dynamics observed for bare AuNPs. Due to the weakest electrostatic attraction between the negatively charged NPs and the negatively charged membrane, the anionic AuNPs exhibit the lowest energy barrier.
The impacts of lipid charge and temperature on the intake/uptake of cationic AuNPs into lipid bilayers were investigated using AAMD/CGMD simulations.201 The positively charged AuNPs are unable to adhere spontaneously to a pure DSPC lipid membrane due to the existence of a certain barrier, which is not very large (32.4 kJ mol−1) that can be overcome by increasing temperature. The binding interaction between cationic AuNPs and the lipid membranes can be enhanced when negatively charged lipid components (DSPG) are introduced to pure lipid membranes (DSPG
:
DSPC = 1
:
3). This may accelerate the incorporation of AuNPs into the membranes and lead to lipid extraction. These results demonstrate that temperature and lipid charge can influence the stability of the membrane, which in turn affects the pathway of AuNPs incorporation into the membrane. The interaction between AuNPs with different surface chemistry and charge density and negatively charged lipid vesicles was also investigated.202 While anionic AuNPs adhere to the membrane surface, hydrophobic and cationic AuNPs tend to enter the vesicle bilayer (Fig. 12a). Cationic AuNPs with a low charge density can enter lipid bilayers to form a stable “snorkeling” configuration, whereas those with high charge density will eventually penetrate the membrane and get into the vesicle core. The energy profiles demonstrated that the interactions between cationic/hydrophobic AuNPs and lipid vesicles became stronger with a decrease in distance from the membrane centre or an increase in charge density of cationic AuNPs (Fig. 12b). These results suggest that hydrophobic and electrostatic interactions are important driving forces for their interaction with membranes.202,203
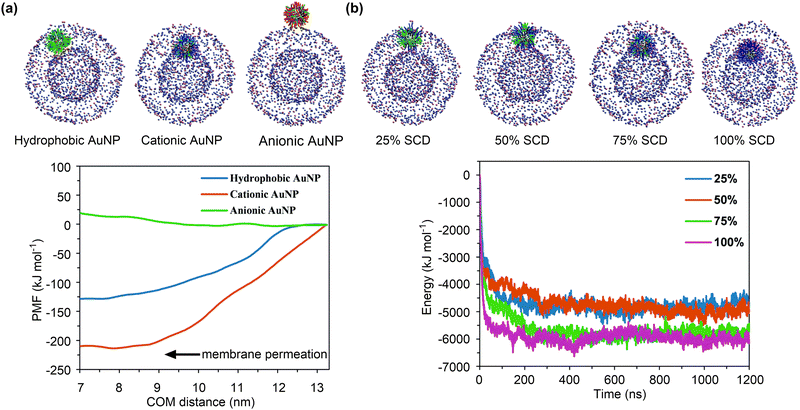 |
| Fig. 12 Effects of surface charge on cellular uptake of Au nanoparticles. (a) Equilibrium configurations of different AuNPs interacting with the vesicle and the PMF profiles of AuNPs entering into the vesicle. (b) Equilibrium configurations of the cationic AuNPs with different surface charge densities interacting with the vesicle and their interaction energy profiles. Reproduced with permission from ref. 202. Copyright 2021 the Owner Societies. | |
In addition to the charge, the size and surface modification of nanoparticles can also affect their internalisation into membranes. MD simulations demonstrated a three-stage adsorption process of the SiO2 nanoparticles into DPPC lipid membranes: SiO2 nanoparticles approach to the surface, adjust the angle of interaction with the DPPC bilayer, and reach a stable adsorption configuration. In comparison to the surface modification of SiO2 nanoparticles, which results in a slight increase in their interactions with DPPC bilayers, the size effect is more pronounced in enhancing the interactions between SiO2 nanoparticles and DPPC bilayers. Larger SiO2 nanoparticles exhibited stronger interaction energies and more pronounced effects on the arrangement of phospholipid molecules and the integrity of the membrane.204 The ligand density is another factor that affects the internalisation process of nanoparticles. Dissipative particle dynamics (DPD) simulations demonstrated that the PEGylated AuNPs with a high grafting density can be more easily encapsulated by the zero-tension and receptor-rich (50%) lipid bilayer than those with a low grafting density. It was also observed that increasing the polymerisation degree of grafted PEG results in a larger nanoparticle, which in turn makes the internalisation process less efficient due to the increased steric repulsion energy.205
Nanoparticles may undergo structural rearrangement, altering their sizes and/or interactions with membranes during the internalisation process. The structural rearrangement of nanoparticles mainly depends on their stiffness. DPD simulations suggested that only rigid nanoparticles can completely enter the cell, whereas the internalisation soft nanoparticles can be inhibited or frustrated due to wrapping-induced shape deformation and non-uniform ligand distribution.206 Soft nanoparticles may also become more compact during the membrane penetration process, making the internalisation of soft nanoparticles easier.207 These results demonstrate the complex influence of nanoparticle rigidity on membrane interactions, where slight variations in rigidity may alter cellular internalisation behaviour.208 Given the advantages of rigid and soft nanoparticles in cell internalisation, an efficient approach to regulate the cell entry was proposed based on MD simulations by tuning the mechanical properties of nanoparticles with the introduction of local rigid components into soft nanoparticles.209
4.2. Receptor-mediated supramolecular interaction
The lack of specificity in the passive interactions between nanoparticles (NPs) and biological systems may hinder their practical applicability of medical applications. To this end, various targeting ligands, such as small molecules, peptides, and proteins, have been introduced to functionalise NPs. These ligand-functionalised NPs can specifically bind to receptors overexpressed on tumour cells, thereby enabling the targeted drug delivery function of NPs via receptor-mediated endocytosis (RME). The kinetics and pathways of this process are significantly influenced by the properties of NPs, ligands, and receptors. In addition, several antibody mimics based on NPs (referred to as artificial antibodies) have also been developed for the rapid and selective detection of pathogenic bacteria.
4.2.1. Interaction of folic acid-functionalised nanoparticles with receptors.
It has been reported that the folic acid (FA)-functionalised PEGylated AuNPs can specifically recognise the folate receptors (FRs) overexpressed on HeLa cells. Furthermore, the adsorbed serum proteins do not abolish the targeting ability of AuNPs because of the highly dynamic nature of protein adsorption.210 However, the cellular uptake of AuNPs in the presence of adsorbed proteins can be affected by NP sizes. For example, protein adsorption would result in a reduction in the targeting ability of small NPs (5 nm) but an enhancement in that of large NPs (40 nm) relative to bare NPs. These observations were consistent with the DPD simulations, which demonstrated that large NPs loaded with proteins can be gradually engulfed and internalised by the membrane, whereas small NPs loaded with proteins can only adhere to the membrane surface even on a long time scale of 8000 ns (Fig. 13a). This is likely because large NPs with a small surface curvature bind to FRs through multivalent interactions, whereas small NPs with a large surface curvature recognise FRs mainly through one-on-one binding interactions. Therefore, protein adsorption would enhance cell targeting when NPs are large but interfere with cell targeting when NPs are small.
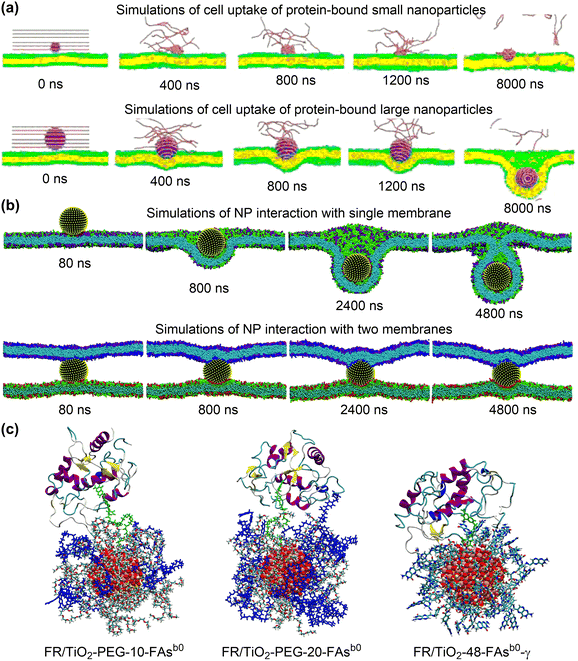 |
| Fig. 13 Interactions of folic acid-functionalised nanoparticles with folate receptors. (a) Snapshots of simulated cell uptake of protein-bound nanoparticles with different sizes. Reproduced with permission from ref. 210. Copyright 2016 American Chemical Society. (b) Snapshots of simulated nanoparticle interactions with single and two membranes. Reproduced with permission from ref. 211. Copyright 2019 the Royal Society of Chemistry. (c) configurations of folic acid functionalised TiO2 nanoparticles binding to folate receptors. Reproduced with permission from ref. 212. Copyright 2023 CC-BY 4.0. | |
The cell density of HeLa cells also has a significant effect on the cellular uptake of PEGylated Au NPs decorated with FA molecules. It was found that NPs were readily internalised by cells via endocytosis in dilute cell cultures, but were trapped at the cell junctions in high density cell cultures.211 DPD simulations (Fig. 13b) indicated that an adhesive nanoparticle placed above a single membrane can be fully wrapped by the membrane to achieve the endocytosis. However, when the nanoparticle was positioned between two membranes, the wrapping process was strikingly hindered and no internalisation occurs. Further free energy analysis suggested that adhesion, bending, and protrusion of two membranes result in complicated energy contributions that cause the nanoparticle to be trapped at cell junctions.
Classical MD simulations were conducted to investigate the dynamics of FA-functionalised TiO2 NPs in binding with FR, specifically, FRα, which is overexpressed on tumour cells.212 As shown in Fig. 13c, FR/TiO2-PEG-10-FAsb0 and FR/TiO2-PEG-20-FAsb0 represent the PEGylated TiO2 NPs functionalised by 10 and 20 FA molecules, respectively. The comparison between these two systems indicated that an increased FAsb0 density led to a stronger interaction with the FR due to a more pronounced polar environment surrounding the PEGylated NPs. For the non-PEGylated system (FR/TiO2-48-FAsb0-γ), an excessive interaction between the nanodevice and the FR was observed, leading to a larger deformation of the protein secondary structure. In addition, the contact surface area between the functionalised NPs and the FR was increased when the deprotonated FA molecules are employed. This was particularly evident for the PEGylated system with a higher FAsb0 density. As a result, the PEG spacer, FA density, and FA protonation state are important parameters that can be tuned for the optimal design of functionalised nanodevices.
4.2.2. Interaction of peptide-functionalised nanoaprticles with receptors.
Peptide is another important targeting ligand for the functionalisation of nanoparticles. For example, the peptide-functionalised Au nanoparticles (Au10Peptide5) could specifically target to the epidermal growth factor receptor (EGFR) on the membrane of HeLa cells.213 Molecular docking and MD simulations were performed to understand such specific ligand–receptor interaction. It was found that the Au10Peptide5 could specifically and stably bind to the natural active sites between domain I and domain III of EGFRs in both active (see A-EGFR) and inactive (see I-EGFR) states (Fig. 14a). Nevertheless, the binding of Au10Peptide5 to EGFR in the active state is stronger than in the inactive state. Importantly, the binding of Au10Peptide5 has a minor influence on the conformations of EGFRs, which does not affect their activity in either the A-EGFR or I-EGFR states.
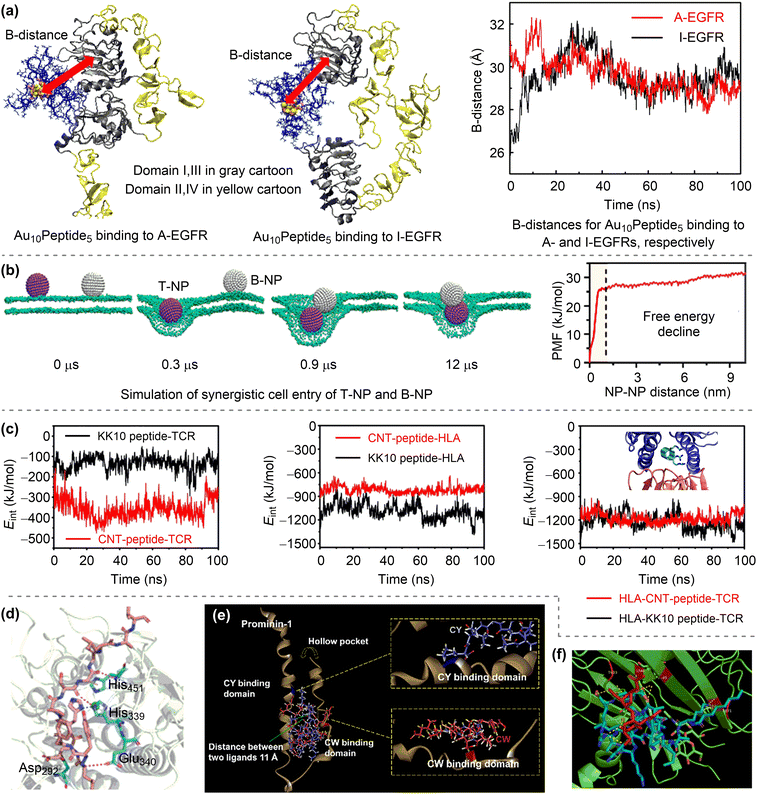 |
| Fig. 14 Interactions of peptide-functionalised nanoparticles with receptors. (a) Binding of Au10Peptide5 to A- and I-EGFRs. Reproduced with permission from ref. 213. Copyright 2018 Elsevier. (b) Simulation of synergistic cell entry of T-NP and B-NP and the corresponding potential of mean force profile. Reproduced with permission from ref. 214. Copyright 2022 American Chemical Society. (c) Time evolution of interaction energies (Eint) between CNT–peptide (or KK10 peptide) and HLA-TCR receptor. Reproduced with permission from ref. 215. Copyright 2017 AIP Publishing. (d) Binding mode of HCC167 peptide (ligand functionalising polymer self-assembled nanoparticle) and ALPPL2 receptor. Reproduced with permission from ref. 216. Copyright 2022 Wiley-VCH. (e) Molecular docking patterns of CW and CY peptides with prominin-1 receptor. Reproduced with permission from ref. 217. Copyright 2019 American Chemical Society. (f) Molecular docking pattern of P17 lipopeptide and αvβ3 integrin receptor. Reproduced with permission from ref. 218. Copyright 2023 Elsevier. | |
It is also possible that the functionalised nanoparticles may stimulate the cellular uptake of unfunctionalised bystander nanoparticles (B-NPs). For example, the transactivator of transcription (TAT) can bind with the heparan sulfate proteoglycans (HSPGs) on the cell surface, thereby inducing the receptor-mediated endocytosis.214 In the presence of TAT-functionalised NPs (T-NPs), such as T-Ag or T-Au, the B-NPs can spontaneously move towards T-NPs and eventually enter the same endocytic vesicle (Fig. 14b). The decline in free energy may be the thermodynamic driver for the T-NPs-induced cellular uptake of B-NPs. The bystander activity depends on the sizes of B-NPs, which is further rationalised through free energy calculations.
A HIV-1 immunodominant peptide KK10 was predicted to be a suitable candidate for covalent conjugation to CNT via the arginine residues.215 As shown in Fig. 14c, the interaction of the CNT–peptide with the T cell receptor (TCR) is stronger than that of KK10, but the interaction of the former with the human leukocyte antigen (HLA) is weaker than that of the latter. Structural analysis revealed that the CNT–peptide binds to the TCR with more salt bridges than KK10, but with fewer hydrogen bonds and hydrophobic interactions when binding to the HLA. However, the CNT–peptide and KK10 exhibit strong and comparable binding interactions with the HLA–TCR complex (Fig. 14c), demonstrating that the CNT–peptide complex can take advantage of both stability and targeting ability. In addition to being anchored on rigid inorganic nanoparticles, the peptide can be loaded into the soft nanoparticles via co-assembly. For example, the HCC167 peptide-loaded polymer nanoparticle can specifically recognise the ALPPL2 protein on the HepG2 cell membrane, exhibiting a potent active targeting strategy for mRNA-based hepatocellular carcinoma (HCC) therapy.216 The molecular docking simulation showed that the binding affinity between the HCC167 ligand and the ALPPL2 receptor mainly came from the hydrogen-bonding interactions and π–H interactions (Fig. 14d).
The functionalisation of nanoparticles with a single peptide may not achieve the desired targeting efficiency. An example was found in the PEGylated-liposome functionalised with the activated endothelium targeting peptide (AETP).219 The off-targeting of the AETP is likely due to its restricted availability to target receptors, resulting from the steric hindrance induced by the PEG polymer and the affinity for bloodstream proteins, especially human serum albumin (HSA). MD simulations and protein–ligand docking calculations demonstrated that the AETP is located deeper inside the PEG layer and that the affinities of AETP and PEG for HSA are similar.220 To improve the targeting efficacy, a dual-peptides functionalisation strategy was proposed. Molecular docking simulations demonstrated that a CW/CY-peptides-targeting (CW = CWRLRWHSPLK and CY = CYIVFYDSPLE) liposomal probe exhibits a strong affinity towards the prominin-1 receptor in both cellular and in vivo levels.217 As shown in Fig. 14e, CW and CY can both insert into the hollow pocket of prominin-1 and bind to the two inner sides of the protein. These studies suggest that a precise control of the surface density and systematic optimisation of the surface spatial distance of the ligand peptides could significantly enhance the targeting efficiency of the peptide-coated nanoparticles.
Peptides can also self-assemble into nanoparticles for medical applications. An amphiphilic P17 lipopeptide (C8H15O-KALKALKKALKALKDGR) was fabricated through the conjugation of the RGD peptide with the KLA peptide and the modification by n-octanoic acid.218 The self-assembled P17 lipopeptide can encapsulate anticancer drugs such as doxorubicin to form P17@doxorubicin. In addition, it can recognise the αvβ3 receptor of the integrin protein overexpressed on the tumour surface. Subsequent molecular docking simulations indicated that the RGD of P17 could successfully dock with the extracellular fragment of the αvβ3 receptor through 12 hydrogen-bonding interactions (Fig. 14f). Similarly, a biomimetic peptide nanoparticle (BP-KFFVLK-WYKDGD) was reported to demonstrate a specific targeting ability to the lysophosphatidylcholine (LPC) receptor. This was attributed to the stronger interaction between the peptide nanoparticle and the LPC receptor in atherosclerotic plaques than that with the human umbilical vein endothelial cell.221 The all-atom MD simulations showed that, the YKDG amino acids are the main fragment responsible for the specific binding between the peptide and the LPC receptor under neutral conditions.
4.2.3. Interaction of protein-functionalised nanoparticles with receptors.
Inspired by exosomes, a natural nanocarriers, the mucin-domain-containing molecule-4 (TIM4 protein)-decorated chitosan-based NPs were synthesized and showed a higher cellular uptake than unfunctionalised NPs. The cellular uptake was tested in cancer cells such as glioblastoma U-87 MG, which overexpressed phosphatidylserine (PS).222 The higher cellular uptake of TIM4-decorated NPs was rationalised by all-atom MD simulations, which demonstrated that the binding free energy between the TIM4 ligand and PS receptor is more negative (−17.5 kcal mol−1) than that between chitosan and PS (−9.1 kcal mol−1). This can be attributed to the stronger electrostatic and van der Waals interactions between the TIM4 ligand and PS receptor. In addition, it was found that 10 residues of TIM4, including ASN39, SER40, LYS41, ARG91, GLU93, VAL94, GLY96, PHE98, ASN99 and ASP100, played crucial roles in the binding interaction with the PS receptor (Fig. 15a).
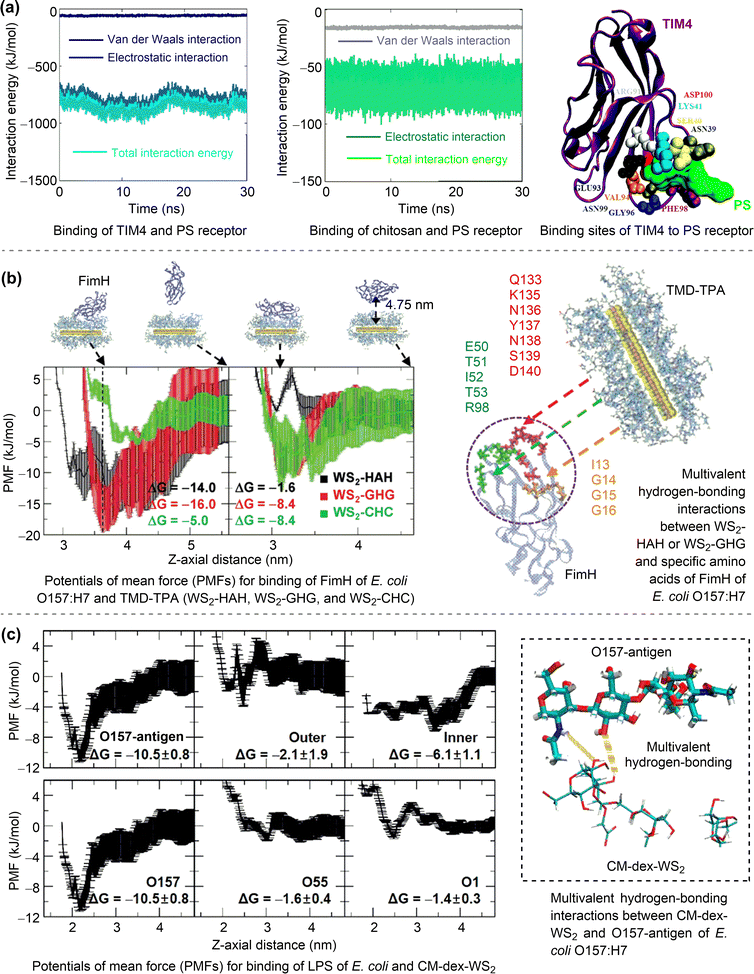 |
| Fig. 15 Interactions of protein-functionalised nanoparticles with receptors and artificial antibodies. (a) Binding of TIM4 (protein ligand functionalising chitosan-based NPs) and PS receptor. Reproduced with permission from ref. 222. Copyright 2016 the Royal Society of Chemistry. (b) Binding of TMD-TPA artificial antibodies and FimH of E. coli O157:H7. Reproduced with permission from ref. 223. Copyright 2021 Wiley-VCH. (c) Binding of CM-dex-WS2 artificial antibody and O157–antigen of E. coli O157:H7. Reproduced with permission from ref. 224. Copyright 2021 American Chemical Society. | |
4.2.4. Artificial antibodies.
The supramolecular interactions between antigen and antibody are crucial in the immune response to eliminate pathogens, such as viruses and bacteria. By carefully designing the anchoring peptides from natural antibodies, the resulting peptides-functionalised nanoparticles (artificial antibodies) can achieve the functionality of specific antigen recognition.225 For example, a series of luminescent antibody mimics was designed using transition metal dichalcogenide (TMD) nanosheets as the rigid scaffolds and multivalent tripeptide assembly (TPA) as the flexible recognition sites.223 Among the TMD-TPA antibody mimics, WS2-HAH, WS2-HHH, and WS2-GHG were E. coli O157:H7-specific, recognising the type 1 fimbrial adhesin (FimH, a membrane protein); WS2-CHC and MoSe2-KSC were S. typhimurium-specific, recognising the FimH and lipopolysaccharides (LPS), respectively; and MoSe2-KSI was S. aureus-specific, recognising the peptidoglycan (PepG).
To understand the selective recognition of these TMD-TPA antibody mimics, their binding free energies with bacterial membrane components (i.e., FimH, LPS, and PepG) were calculated using all-atom MD simulations. As shown in Fig. 15b, the calculated binding free energies of WS2-HAH (−14.0 kJ mol−1) and WS2-GHG (−16.0 kJ mol−1) are more negative than that of WS2-CHC (−8.4 kJ mol−1) for binding with the FimH of E. coli O157:H7. Likewise, the binding free energy of MoSe2-KSI (−7.7 kJ mol−1) is more negative than that of WS2-GHG (−3.1 kJ mol−1) for binding with the PepG of S. aureus. Additionally, the binding free energy of MoSe2-KSC (−22.5 kJ mol−1) is more negative than that of WS2-CHC (−10.8 kJ mol−1) for binding with the LPS of S. typhimurium. These results are consistent with above experimentally observed specific recognition of bacteria by these antibody mimics. It was also noted that multivalent hydrogen bonding interactions exist between these antibody mimics and bacteria proteins. Therefore, the dynamic and multivalent tripeptide spaces of TMD-TPA antibody mimics played important roles for their selective recognition of pathogenic bacteria.223 In addition to TPA peptides, the nonbiological multivalent polymeric ligands can be used in the design of antibody mimics.224 The CM-dex-WS2 was E. coli O157:H7-specific, recognizing the O-antigen (O157) residue of the bacteria LPS, while dex-WS2 was S. aureus-specific, recognizing the Staphylococcal protein A (SpA) of the bacteria. The CM-dex and dex represent carboxymethylated dextran and neutral dextran, respectively. These specific bacteria recognitions were consistent with the strong binding interaction between CM-dex-WS2 and the O157-antigen of E. coli O157:H7 LPS via multivalent hydrogen-bonding interaction (Fig. 15c).
4.2.5. Factors influencing the kinetics and pathways of receptor-mediated endocytosis.
Nanoparticle properties.
The influence of NP properties (size, shape, stiffness, etc.) on RME has been theoretically investigated by using CGMD or DPD simulations. For the size effect, it was found that the endocytosis of larger NPs can be more readily achieved than that of smaller ones, due to a more favourable compromise between bending rigidity and surface adhesive energy,226 which was also observed for other NPs, such as the icosahedral NPs with different diameters (5, 8, and 12 nm) and the graphene nanosheets with sizes ranging from 3.5–14.0 nm.227,228 An optimal size was suggested for spherical NPs, at which endocytosis would occur in the shortest time. As shown in Fig. 16a, the simulations demonstrated that NPs with a small size (NP radius R = 5.0σ, σ ≈ 2 nm) could only be partially wrapped. NPs with an intermediate size (R = 7.5σ) exhibited the fastest endocytosis process, while further increasing the NP sizes (R = 10.0σ and 12.5σ) resulted in a monotonic slowing of the endocytosis process.229 These results seemingly suggest that a size of approximately 15 nm is optimal for the endocytosis of NPs, which requires further investigation.
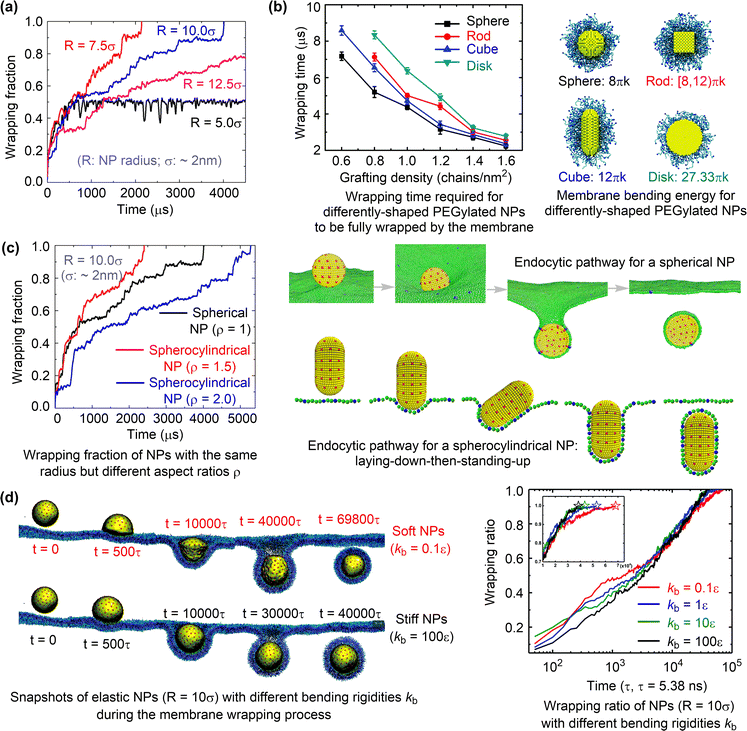 |
| Fig. 16 Effects of nanoparticle properties on receptor-mediated endocytosis. (a) Size effects. Reproduced with permission from ref. 229. Copyright 2013 American Chemical Society. (b) and (c) Shape effects. Reproduced with permission from ref. 230. Copyright 2015 the Royal Society of Chemistry. (d) Stiffness effects. Reproduced with permission from ref. 231. Copyright 2018 the Owner Societies. | |
For the shape effect on RME, it was found that spherical NPs exhibited the fastest endocytosis rate, followed by cubic NPs, then rod-like NPs, and finally disk-like NPs (Fig. 16b).230 Free energy calculations showed that the difference in membrane bending energy, increasing in the order of sphere < cube < rod < disk, is the main reason for the observed NP shape effect (Fig. 16b). Similarly, the spherical NPs can be fully endocytosed more rapidly than the spherocylindrical NPs with an aspect ratio of 2, but less rapidly than those with an aspect ratio of 1.5 (Fig. 16c).229 In addition, the spherocylindrical nanoparticles exhibited an endocytic pathway consisting of a sequence of laying-down and standing-up events, different from that observed for spherical NPs (Fig. 16c). These findings suggest that the shape of NPs plays complex roles on RME kinetics, which is significantly influenced by the rotation and initial orientation of differently shaped NPs.232,233
For the stiffness effect on RME, it was found that nanoparticles with a bending rigidity (kb) of 100ε were the first to be fully wrapped, followed by those with kb values of 10, 1, and 0.1ε (Fig. 16d).231 Further free energy analysis revealed that the energy barriers for soft nanoparticles (kb = 0.1, 1ε) were lower than those for stiff nanoparticles (kb = 10, 100ε) at the wrapping ratio less than 0.5. However, the former exhibited a sharp increase and became much higher than the latter at the wrapping ratio larger than 0.5. The overall energy barriers for the stiff nanoparticles are lower than those for the soft ones, resulting in the faster endocytosis of the stiff nanoparticles.
Ligand properties.
MD simulations have also been employed to elucidate the influence of functional ligands on the RME of nanoparticles.227,234,235 For example, the strength of the receptor–ligand interaction as well as the density, rigidity, and length of ligands, are all important factors influencing the RME.234 As shown in Fig. 17a, an increase in the strength of the receptor–ligand interaction, ligand density and ligand rigidity would result in a larger engulfment degree and a higher probability of complement engulfment of NPs. For the effect of ligand length on RME (Fig. 17a), it was found that, when the receptor–ligand interaction strength is low, the engulfment degree of NPs increases with the increasing ligand length. However, when the receptor–ligand interaction strength is high, the short ligand is good for the engulfment. Similarly, NPs with a high ligand density can be spontaneously wrapped, whereas NPs with a low ligand density cannot.227 However, for NPs with a medium number of ligands, the ligand distribution becomes the most important factor affecting the RME kinetics rather than the ligand density; the most homogeneous distributions with ligands evenly spread are the most efficient for the uptake of NPs.
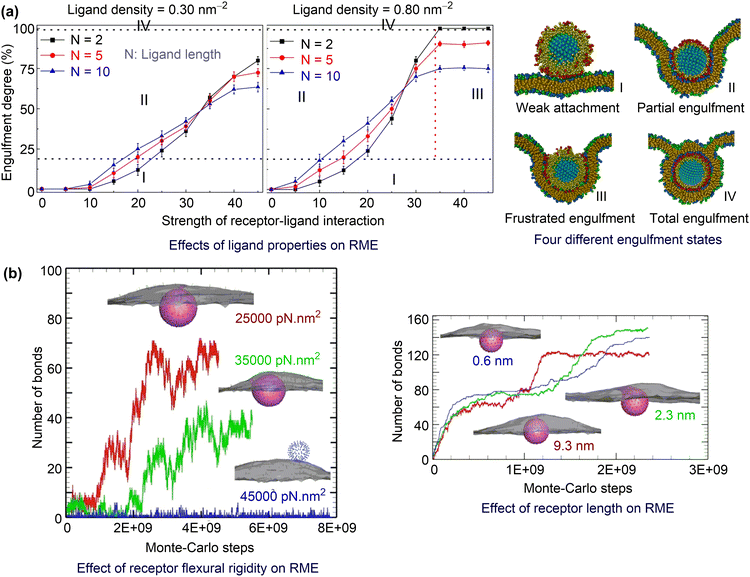 |
| Fig. 17 Effects of ligand and receptor properties on receptor-mediated endocytosis. (a) Effects of ligand properties. Reproduced with permission from ref. 234. Copyright 2012 Elsevier. (b) Effects of receptor properties. Reproduced with permission from ref. 236. Copyright 2023 the Royal Society of Chemistry. | |
Receptor properties.
MC simulations were performed to systematically investigate the effects of mechanical (receptor flexural rigidity), geometrical (receptor length), and biochemical (ligand–receptor reaction cutoff) properties of receptors, on RME with and without the existence of clathrin.236 As shown in Fig. 17b, in the presence of clathrin, a smaller receptor flexural rigidity and a shorter receptor length facilitates the formation of more ligand–receptor bonds and promotes the internalisation of nanoparticles. On the contrary, the ligand–receptor bonds formed for RME without clathrin are much less, suggesting that the clathrin is crucial for nanoparticle internalisation.
4.3. Summary
In summary, nanomaterials can interact with biological entities like cell membranes and receptors through supramolecular interactions, which include the passive and receptor-mediated internalisation processes of nanoparticles. All-atom and coarse-grained MD simulations are the main computational methods to investigate these supramolecular interactions. MD simulations have been used to investigate the translocation of nanoparticles through lipid bilayer membranes. The study reveals that the intrinsic properties of nanoparticles, such as size, shape, surface charge, and modifications, significantly affect their interaction with the membrane. The simulations also consider the impact of membrane complexity on the uptake pathways and energy barriers for nanoparticle internalisation. MD simulations have also been employed to study the binding dynamics and the influence of nanoparticle properties, ligand properties, and receptor properties on the receptor-mediated endocytosis process. The research emphasizes the importance of functionalising nanoparticles with targeting ligands to improve their specificity in medical applications. The simulations play a critical role in elucidating the kinetics and pathways of cell-nanoparticle interactions. They provide insights into how different surface charges, sizes, and surface modifications of nanoparticles affect their internalisation. Furthermore, simulations help in understanding the role of ligand density, rigidity, and length, as well as the properties of receptors, in the receptor-mediated endocytosis process. The study suggests that a clear understanding of supramolecular interactions, facilitated by MD simulations, is necessary for the advancement of nanodrug research.
Although MD simulations play a crucial role in understanding supramolecular interactions. However, leveraging these techniques to computationally design novel nanodrugs with tailored membrane translocation characteristics remains a significant challenge. Additional experimental research is essential to elucidate the key determinants of nanomaterial transmembrane activity. Concurrently, computational studies should concentrate on these pivotal factors to establish robust quantitative structure–activity relationships, facilitating the de-novo design process.
5. Surface catalysis-based nanodrugs
The catalytic ability of nanomaterials is an important property that enables their various medical functions. In recent years, numerous reports have shown that nanomaterials can serve as efficient biocatalysts to regulate cellular reactive oxygen species,13,237,238 to cleave phosphoester and other P-containing bonds present in organophosphates,239 and to catalyse other medically relevant biochemical reactions, such as the constant generation of gaseous signalling molecule NO240,241 and lactate dehydrogenation.242,243 The catalytic properties of nanomaterials have made them promising nanodrugs (termed surface catalysis-enabled nanodrugs) for the treatment of cancer, bacterial infection, oxidative stress-related diseases, nerve agent poisoning, cardiovascular diseases, and other diseases.13,237–240,244–247 Apparently, the catalytic activity plays an important role in the therapeutic efficacy of surface catalysis-enabled nanodrugs. Therefore, it is of great importance to comprehensively understand the underlying mechanisms and kinetics of biochemically relevant reactions catalysed by nanomaterials. Such knowledge is useful for the development of predictive models for catalytic activity, which will facilitate the screening and rational design of high-performance nanodrugs.
5.1. Targeted biochemical reactions and relevant medical functions
5.1.1. Nanodrugs regulating reactive oxygen species (ROS).
Nanomaterials (NMs) that can regulate the intracellular level of ROS such as O2˙−, H2O2, 1O2, and ˙OH, are representative surface catalysis-based nanodrugs.237 There are two main approaches for nanomaterials regulating ROS: one is driven by exogenous light, electricity, ultrasound, X-ray, etc; the other is based on endogenous chemical stimuli, such as the enzyme-like catalysis.237,246,247 Herein, we will focus on the ROS regulation reactions driven by enzyme-like catalysis, including peroxidase-, oxidase-, superoxide dismutase-, and catalase-like catalysis.
Peroxidase-like nanocatalysis.
Nanomaterials with peroxidase-like activity can catalyse the reduction of hydrogen peroxide (H2O2) with organics, such as amines, which act as electron donors, (Fig. 18a). One of the most commonly used chromogenic substrates is 3,3′,5,5′-tetramethylbenzidine (TMB), which can be oxidised to TMB+ in solution, resulting in a colour change from colourless to blue.20 NMs possessing the peroxidase-like activities can convert H2O2 into highly cytotoxic ROS such as hydroxyl radicals (˙OH). The high concentration of ˙OH produced in bacteria would induce the oxidative stress, cause damage to the bacterial membrane, and eventually lead to bacteria death, making those NMs promising nanodrugs for antibacterial applications.237,247 In addition, the ˙OH species generated from the overexpressed H2O2 in the tumour microenvironment (TME) can elevate the oxidative damage of tumour cells, inhibit the tumour growth, and induce the apoptosis and necrosis of tumour cells, making those NMs promising nanodrugs for tumour chemodynamic therapy.237,247
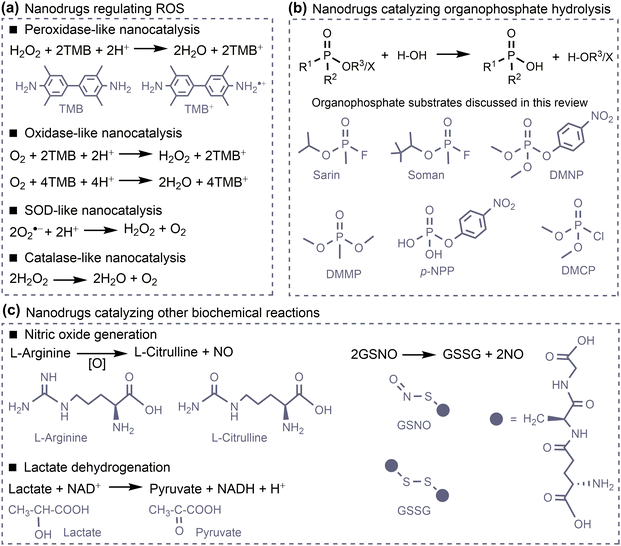 |
| Fig. 18 Targeted biochemical reactions for nanodrugs based on surface catalysis. (a) Nanodrugs regulating reactive oxygen species (ROS). (b) Nanodrugs catalysing organophosphate hydrolysis. (c) Nanodrugs catalysing other biochemical reactions. | |
Oxidase-like nanocatalysis.
Nanomaterials with oxidase-like activity can catalyse the oxidation of organic substrates such as TMB by O2 molecules (Fig. 18a). In this reaction, O2 can undergo either a two-electron or a four-electron reduction, being reduced to H2O2 and H2O, respectively.20 Similar to peroxidase-like nanocatalysis, NMs with oxidase-like activity can also exhibit excellent antibacterial applications because they can promote the formation of highly cytotoxic ROS such as O2˙−.13,237,238,247
Superoxide dismutase-like nanocatalysis.
Nanomaterials with superoxide dismutase-like (SOD-like) activity can catalyse the disproportionation of superoxide radical (O2˙−) (Fig. 18a). In this reaction, one O2˙− transfers an electron to the other with the assistance of two protons, generating one H2O2 molecule and one O2 molecule.20 In contrast to the peroxidase- and oxidase-like nanocatalysis, which facilitate the generation of ROS, the SOD-like nanocatalysis can catalyse the scavenging of ROS (O2˙−), thereby possessing therapeutic potential for the treatment of oxidative damage-related diseases, such as neurodegenerative diseases (e.g., Alzheimer's disease and Parkinson's disease) and ischemic stroke-reperfusion injury.13,237,238,247
Catalase-like nanocatalysis.
Nanomaterials with catalase-like activity can catalyse the disproportionation of H2O2 (Fig. 18a). In this reaction, one H2O2 molecule transfers two hydrogen atoms to the other, generating one O2 molecule and two H2O molecules.20 Similar to SOD-like catalysis, NMs possessing the catalase-like activities can catalyse the scavenging of H2O2 and hold great potential for clinical therapeutics associated with oxidative stress.13,237,238,247 In addition, they can also be used to alleviate tumour hypoxia by catalysing the overexpressed H2O2 into O2, and subsequently enhancing the tumour sonodynamic/photodynamic therapy by the production of singlet oxygen (1O2) from the generated O2 in TME under ultrasound/light irradiation.13,237,238,247
5.1.2. Nanodrugs catalysing organophosphate hydrolysis.
The NM-catalysed organophosphate hydrolysis (Fig. 18b) has received increasing attention because of its promising biomedical applications in the prevention, diagnostics, and treatment of diseases such as nerve agent poisoning, bacterial infection, and even cancer.239,248–250 For example, it has been reported that a series of NMs such as noble metal Ru, metal oxides CeO2 and ZrO2, metal organic frameworks UiO-66, NU-1000, and MOF-808, polyoxometalates, and their relevant composites can effectively catalyse the detoxification of organophosphate nerve agent simulants (e.g., methylparaoxon and dimethyl methylphosphonate) and real nerve agents (e.g., sarin, soman, and VX) by hydrolytically cleaving the phosphoester bond and other related P–X bonds (X = F, S, etc).239,251–253 Furthermore, some of those NMs, particularly those based on Ce(IV), have been demonstrated to be effective biocatalysts for the hydrolysis of extracellular DNA, making them promising candidates for the treatment of drug-resistant bacterial biofilm infections.249
5.1.3. Nanodrugs catalysing other biochemical reactions.
Nitric oxide generation.
The nitric oxide (NO)-generating nanodrugs play an important role in tumour therapy, antibacterial/biofilm, and the treatment of cardiovascular disease, eye disease, wound healing, due to the crucial biomedical functions of the signalling molecule NO in regulating vascular relaxation, immune response, neurotransmission, and so on.240 The NM-catalysed NO generation (Fig. 18c) has been reported to be an effective approach to release NO in a controlled and sustained manner for in vivo applications, in which L-arginine and S-nitrosothiols such as S-nitrosoglutathione (GSNO) are widely used as NO donors.241
Lactate dehydrogenation.
The excessive lactate produced by the rapid glycolysis of tumour cells can trigger the acidification of TME, leading to the formation of an immunosuppressive TME and the dysfunction of tumour-associated macrophages.242,254 The lactate dehydrogenase (LDH)-like nanocatalysis (Fig. 18c) can serve as a useful tool to deplete lactate and alleviate the acidic TME, thereby promoting tumour-associated macrophage reprogramming and enhancing tumour immunotherapy.242,243
5.1.4. pH-dependent activities of catalytic nanodrugs.
It has been well demonstrated that the activities of catalytic nanodrugs are usually pH-dependent. An acidic pH is optimal for peroxidase-mimicking nanodrugs, whereas neutral and alkaline pH conditions are better for nanodrugs that mimic superoxide dismutase and catalase.13 Most of the current computational studies on the catalysis-based nanodrugs have been conducted under neutral conditions, largely due to the inherent difficulty in directly simulating the actual pH conditions. However, several strategies have been suggested to incorporate the influence of pH on the activities of catalytic nanodrugs. For example, an H or OH group can be pre-adsorbed on the nanosurface to simulate the acidic or alkaline conditions, respectively, in order to understand the reaction mechanisms of catalytic nanodrugs.255 In the case of nanodrugs catalysing the hydrolysis of organophosphates such as methylparaoxon, an alkaline buffer solution is usually required, which can be considered by using an OH− anion as the nucleophile in theoretical calculations.256
5.2. Mechanistic insight into activities of catalytic nanodrugs
5.2.1. Catalytic mechanisms for nanodrugs mimicking peroxidase.
Metals.
A base-like H2O2 decomposition mechanism was proposed for noble metals (e.g. Au, Ag, Pd, and Pt) mimicking peroxidase, in acidic conditions.255 As shown in Fig. 19a, the H2O2 molecule is firstly adsorbed on the nanosurface (A → B), followed by the O–O bond cleavage to yield two HO* (B → C). Subsequently, the rearrangement of the two HO* occurs to generate H2O* and O* (C → D), in which the generated O* would further oxidise organic substrates such as TMB to complete the catalytic cycle. In this mechanism, the step of B → C is the rate-determining step (RDS). The calculated energy barriers of this step for the four noble metals are moderate or small, with the order of Au(111) > Ag(111) > Pt(111) > Pd(111), which is consistent with their increased peroxidase-like activities observed in experiments.255 Based on the base-like H2O2 decomposition mechanism, the energy barrier of the RDS for Hg@Au(111) (0.26 eV) is lower than that for Au(111) (0.57 eV),257 consistent with the better peroxidase-like activity of Hg@Au amalgam than Au. A similar mechanism was proposed for 2D titanium carbide-supported RhRu alloy nanoclusters (RhRu/Ti3C2Tx). The energy barrier for this composite is significantly lower than that for Ti3C2Tx (Fig. 19b), indicating that the peroxidase-like activity of this composite mainly arises from the RhRu alloy.258
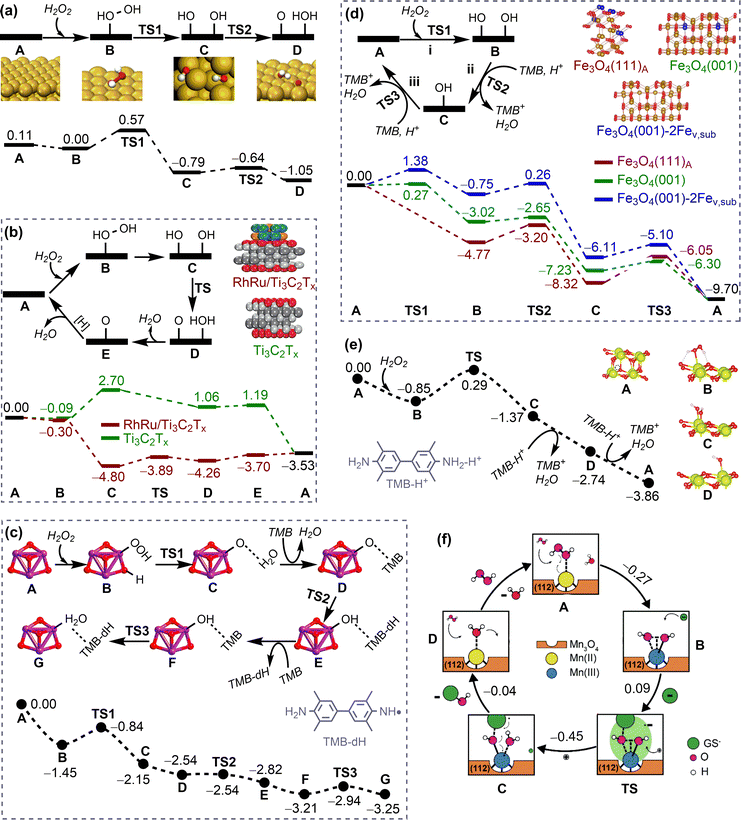 |
| Fig. 19 Mechanisms and energy profiles (in eV) for nanomaterials mimicking peroxidase. (a) Au(111). Reproduced with permission from ref. 255. Copyright 2015 Elsevier. (b) Comparison between RhRu/Ti3C2Tx and Ti3C2Tx. Reproduced with permission from ref. 258. Copyright 2023 Wiley-VCH. (c) Fe3O4 cluster. Reproduced with permission from ref. 259. Copyright 2019 American Chemical Society. (d) Periodic models of iron oxides. Reproduced with permission from ref. 260. Copyright 2020 American Chemical Society. (e) CeO2-O3c. Reproduced with permission from ref. 261. Copyright 2021 American Chemical Society. (f) Citrate functionalised Mn3O4. Reproduced with permission from ref. 262. Copyright 2021 Wiley-VCH. | |
Metal oxides.
The mechanisms for iron oxides mimicking peroxidase were investigated by using both cluster and periodic models.259,260 It was found that the H2O2 molecule would undergo the dissociative adsorption on the surface in a heterolytic manner (Fig. 19c). Subsequently, a reactive oxygen adsorbate is formed (B → C, RDS), followed by the one-by-one oxidation of two TMB molecules. The highly negative reaction energy and the moderate energy barrier for the overall reaction suggest that this reaction is both thermodynamically and kinetically favourable. A similar mechanism was proposed by using a series of periodic models of iron oxides.260 As shown in Fig. 19d, the homolytic dissociative adsorption of a H2O2 molecule firstly occurs on the surface, resulting in the formation of two HO* (i, H2O2 + 2* → 2HO*). The subsequent main reactions are the consecutive reduction of these two OH* by TMB (ii and iii, OH* + TMB + H+ → H2O + TMB+ + *). Each reduction reaction proceeds via the protonation-coupled transfer of a hydrogen atom from the amino group of TMB to OH*. The RDS can be step i or step iii for different iron-oxide slabs, depending on the affinity of nanosurface to the hydroxyl group. This characteristic feature is of great importance in establishing an activity prediction model for NMs mimicking peroxidase. A similar catalytic mechanism was found for nanoceria mimicking peroxidase.261 The dissociation of H2O2* to two OH* (B → C, Fig. 19e) is the RDS with a moderate energy barrier of 1.14 eV, consistent with the experimental finding that nanoceria exhibited a peroxidase-like activity under acidic conditions.263 Furthermore, the calculated energy barriers for the decomposition of H2O2 on different facets of nanoceria increase in the order of (110) < (100) < (111), which is consistent with their decreasing peroxidase-like activities observed in experiments.264 The catalytic mechanism for metal oxides mimicking peroxidase with other organic donors, such as glutathione, is similar to that with TMB as the electron donor.262 As shown in Fig. 19f, the overall reaction process is almost barrierless (0.09 eV), demonstrating their high glutathione peroxidase-like activities.262
Carbon nanomaterials.
In order to gain insight into the active centre and the catalytic mechanism for graphene oxide (GO) mimicking peroxidase, systematic theoretical calculations have been performed, considering various GO models with different oxygen-containing functional groups.265 The carbonyl group can serve as the active centre, undergoing three key elementary steps: the protonation of the carbonyl, the activation of the C
O bond by the addition reaction of H2O2 (RDS), and the oxidation of TMB molecules. The energy barrier of the RDS is not very large (1.02 eV), agreeing with the experimentally observed peroxidase-like activity of GO in acidic conditions.266
Heteroatom doping is a common method for improving the catalytic activity of carbon nanomaterials. A detailed mechanistic investigation on N-doped rGO was conducted to elucidate reasons for the enhanced peroxidase-like activity of rGO by N-doping.267 The N-doped rGO firstly captures one O atom of H2O2 to generate an epoxy O and a water molecule. Subsequently, the epoxy O moves closer to the N atom and transforms into a radical O*, which further abstracts two H atoms to from two TMB-dH molecules. Conversely, the radical O* cannot be formed in the absence of the doped N atom, suggesting that the formation of the radical O* is crucial to the enhanced peroxidase-like activity of N-rGO. This mechanism is applicable to other carbon nanomaterials that mimic peroxidase when different kinds of heteroatoms are doped.245,268
Single-atom catalysts.
The reaction mechanisms for single-atom catalysts (SACs) mimicking peroxidase have also been reported. The one-step decomposition of H2O2 molecule into a O* radical was found on M–N–C after H2O2 adsorption. The energy barrier of this step is much lower on Fe–N–C than that on Co–N–C, agreeing well with their peroxidase-like activities.269 The H2O2 molecule can also undergo a stepwise dissociation on SACs to form the O* intermediate. This process involves the formation of two OH* and subsequent rearrangement to form the O* and H2O*,270 which has been found on FeN3P-SAC, FeN4-SAC, and Fe3O4. However, the reduction of O* and desorption of H2O molecules are uphill in energy, determining the peroxidase-like activity of SACs. The proposed mechanism could also be employed to understand the difference in the peroxidase-like activity of Fe- or Pt-based SACs.271,272
Summary.
In conclusion, the peroxidase-like nanocatalysis typically occurs via the activation of H2O2 and the subsequent oxidation of substrates, such as TMB. The mechanism of H2O2 activation can be broadly described as follows:
(1) H2O2 is decomposed into 2OH* with iron oxide and CeO2-O3c as representative examples.
(2) H2O2 is decomposed into O* and H2O, with the generation of O*occurring with or without the formation of 2OH* as the intermediate. This reaction process has been observed on Au(111), FeN3P-SAC with 2OH* as the intermediate, and on N-rGO and Fe–N–C SAC without 2OH* as the intermediate.
The subsequent oxidation of TMB can be achieved by the reactive oxygen species. In the aforementioned theoretical studies, different forms of TMB (TMB, TMB + H+, and TMB-H+) and oxidised TMB (TMB-dH and TMB+) have been considered. Collectively, these mechanisms can provide a rationale for the catalytic activities of NMs mimicking peroxidase and are useful for the development of predictive models for their activities.
5.2.2. Catalytic mechanisms for nanodrugs mimicking oxidase.
Metals.
The O2 dissociation mechanism (Fig. 20a) was proposed for noble metals mimicking oxidase. In this mechanism, the O2 molecule is firstly adsorbed on the surface and then undergoes the homolytic cleavage to generate two adsorbed O*.273 The calculated energy profiles indicated that Pd(111) and Pt(111) are both thermodynamically and kinetically more favourable than Ag(111) and Au(111) for O2 dissociation, agreeing well with the experimental results.273 The simplicity of this mechanism has facilitated its extensive application in elucidating the differences in oxidase-like activities of various nanomaterials.274–278
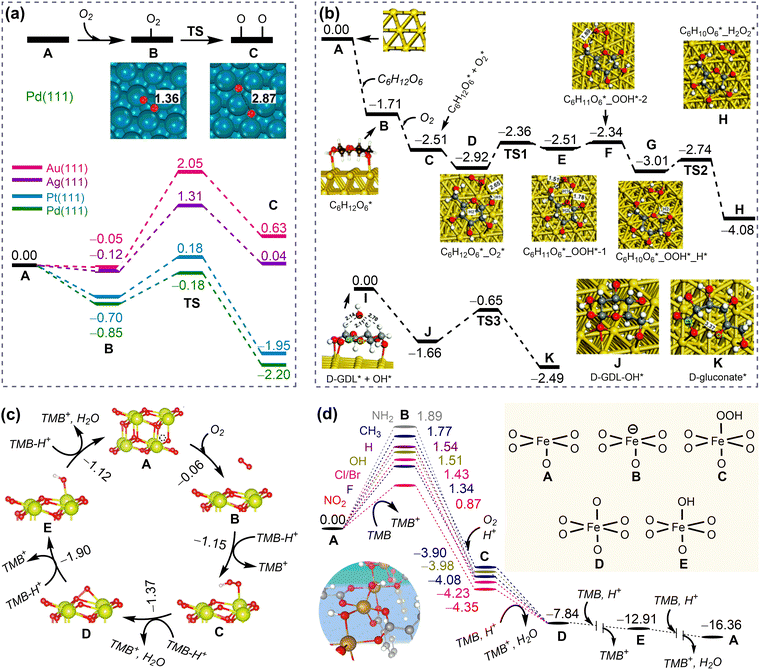 |
| Fig. 20 Mechanisms and energy profiles (in eV) for nanomaterials mimicking oxidase. (a) Noble metals. Reproduced with permission from ref. 273. Copyright 2015 American Chemical Society. (b) Glucose oxidase-like mechanism for Au(111). Reproduced with permission from ref. 279. Copyright 2020 the Royal Society of Chemistry. (c) CeO2-O3c. Reproduced with permission from ref. 261. Copyright 2021 American Chemical Society. (d) MIL-53(Fe)-X (X = NH2, CH3, H, OH, F, Cl, Br, and NO2). Reproduced with permission from ref. 280. Copyright 2021 Wiley-VCH. | |
A more detailed investigation into the glucose oxidase-like activity of Au was carried out, revealing two main half-reactions: the dehydrogenation of glucose to form glucono delta-lactone (GDL) and the hydrolysis of GDL to gluconate (Fig. 20b).279 The first half-reaction proceeds via co-adsorption of glucose and O2, followed by two consecutive hydrogen transfers from glucose* to O2*, resulting in the formation of C6H10O6* and H2O2* (A → H). The second half-reaction occurs with the assistance of a hydroxyl group, which binds to the GDL ring and subsequently breaks the ring to form the gluconate* (I → K). This step is the RDS with a moderate energy barrier of 1.02 eV, which is consistent with the experimentally observed oxidase-like activity of Au.281
Metal oxides.
A four-electron reduction mechanism of O2 by TMB on the CeO2-O3c surface261 was proposed to understand the oxidase-like activity of nanoceria in acidic conditions.282 This mechanism involves the adsorption of O2 on the surface, followed by the stepwise reduction of O2* (Fig. 20c). The energy for these processes decreases continuously, resulting in the formation of four TMB+ and two H2O molecules as products, in accordance with the experimental findings.282
Metal organic frameworks.
Similar to metal oxide, a four-electron reduction mechanism was proposed for MIL-53(Fe)-X mimicking oxidase. As shown in Fig. 20d, the initial transfer of one electron from TMB to MIL-53(Fe)-X (A → B) is followed by the proton-coupled adsorption of an O2 molecule to the Fe centre to generate HOO*, which is then reduced by three consecutive proton-coupled electron transfer steps to yield two H2O molecules.280 The calculated energy profiles indicate that the step of A → B is the RDS and that the reactivity follows the order of X = NO2 > F > Cl, Br > OH > H > CH3 > NH2, in accordance with the experimentally observed decreasing oxidase-like activity order of MIL-53(Fe)-X.280
Summary.
In conclusion, the mechanisms for oxidase-like nanocatalysis can be broadly outlined as follows:
(1) The O2 dissociation mechanism, exemplified by noble metals, such as Au, Ag, Pd, and Pt.
(2) The two-electron reduction mechanism of O2, which has been found on Au(111) mimicking the glucose oxidase.
(3) The four-electron reduction mechanism of O2, which has been found on CeO2-O3c and MIL-53(Fe).
These mechanisms provide a rational explanation for the catalytic activities observed in NMs mimicking oxidase. The direct O2 dissociation mechanism is particularly useful for understanding the difference in oxidase-like activities of various nanomaterials.
5.2.3. Catalytic mechanisms for nanodrugs mimicking SOD.
The superoxide anion radical O2˙− is a Brønsted base with pKb = 9.12, which enables it to capture a proton from water to form HO2˙ and OH−. Therefore, the HO2˙ radical has been commonly employed in computational studies of the catalytic mechanisms for SOD-like nanocatalysts.
Noble metals.
Simultaneous rearrangement of two HO2˙ to O2 and H2O2 can occur on noble metals.273 As shown in Fig. 21a, the adsorption of two HO2˙ on the Au(111) and Pt(111) surfaces is highly exothermic. Subsequently, the adsorbed two HO2˙* undergo a rearrangement to yield H2O2* and O2* with small energy barriers for both Au and Pt, in consistent with their SOD-like activities observed in the experiment.283,284
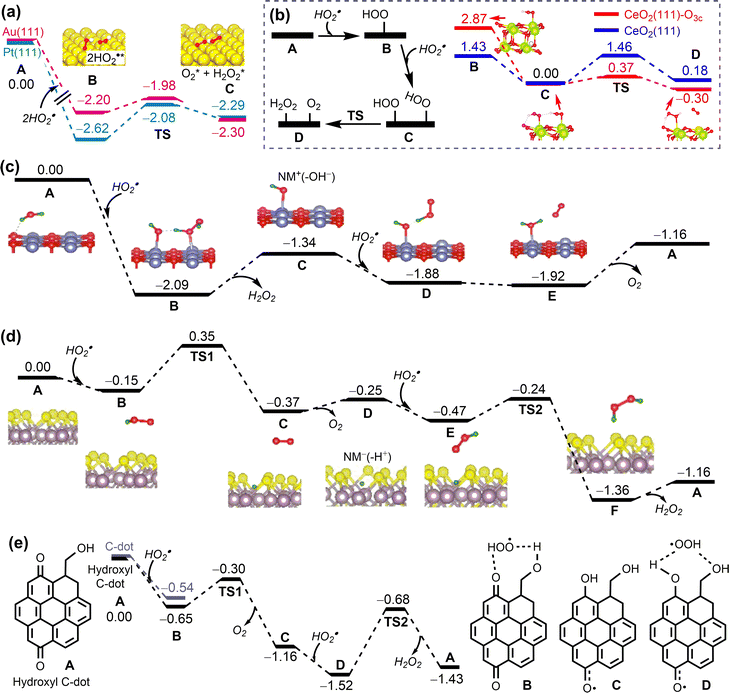 |
| Fig. 21 Mechanisms and energy profiles (in eV) for nanomaterials mimicking superoxide dismutase (SOD). (a) Au(111) and Pt(111). Reproduced with permission from ref. 273. Copyright 2015 American Chemical Society. (b) Comparison between CeO2(111)-O3c and CeO2(111). Reproduced with permission from ref. 285. Copyright 2019 the Royal Society of Chemistry. (c) NiO(100). (d) MoS2−x. Reproduced with permission from ref. 286. Copyright 2021 CC BY 4.0. (e) C-dot with hydroxyl groups. Reproduced with permission from ref. 287. Copyright 2023 CC BY 4.0. | |
Metal oxides and sulfides.
Three possible mechanisms have been found for metal oxides and sulfides, such as CeO2, NiO, and MoS2−x, which exhibit the SOD-like activities.285,286,288–290 The mechanism of the simultaneous rearrangement of two HO2˙ was proposed for nanoceria (Fig. 21b). The calculated energy barrier for Ov-containing CeO2 such as CeO2(111)-O3c is small (0.37 eV), whereas that for Ov-free CeO2(111) is large (1.46 eV). This is consistent with the experimental observation that a higher Ce3+/Ce4+ ratio in nanoceria would result in a stronger SOD-like activity.285
A HOMO (the highest occupied molecular orbital)-mediated mechanism was proposed for the SOD-like catalytic activity of NiO. In this mechanism, the NM is first oxidised by one O2˙− (O2˙− + 2H+ + NM → H2O2 + NM+) and then reduced by another O2˙− (O2˙− + NM+ → O2 + NM), with positively charged NM+ as the intermediate (Fig. 21c).286 On the contrary, a LUMO (the lowest unoccupied molecular orbital)-mediated mechanism was proposed for MoS2−x. In this mechanism, the NM is first reduced by one O2˙− (O2˙− + NM → O2 + NM−) and then oxidised by another O2˙− (O2˙− + 2H+ + NM− → H2O2 + NM) with a negatively charged NM− as the intermediate (Fig. 21d). The calculated energy profiles demonstrate that these reactions can proceed with small energy barriers.286
Carbon nanomaterials.
The LUMO-mediated mechanism was proposed for carbon nanomaterials, such as tris-malonyl C60, C60 fullerenols, and C-dots.287,291,292 Taken the C-dot as an example, calculations showed that the oxidation of the first HO2˙ and the reduction of the second HO2˙ can both readily occur with moderate energy barriers of less than 1 eV (Fig. 21e). In addition, the presence of hydroxyl groups on C-dots enhances the binding affinity to HO2˙, which may facilitate the adsorption of HO2˙, thereby enhancing the SOD-like activity of the C-dots. The role of the hydroxyl groups for enhancing the activity of SOD-mimicking carbon nanomaterials has also been found in C60 fullerenols.292
Summary.
In conclusion, the mechanisms underlying SOD-like nanocatalysis can be broadly outlined as follows:
(1) Two HO2˙, the computational model of O2˙−, undergo a simultaneous rearrangement to generate products O2 and H2O2. This mechanism has been found in the case of noble metals (Au, Pt) and CeO2(111)-O3c.
(2) The first HO2˙ or O2˙− is oxidised to O2, followed by the introduction and reduction of the second HO2˙ to H2O2. SOD-like nanocatalysis with this mechanism include MoS2−x, C60 fullerenols, and hydroxyl C-dot.
(3) The first HO2˙ was reduced to H2O2, after which the second HO2˙ was introduced and oxidised to O2. NiO(100) is a representative example of SOD-like nanocatalysis with this mechanism. These mechanisms provide a comprehensive explanation of the catalytic activities observed in NMs that mimic SOD.
5.2.4. Catalytic mechanisms for nanodrugs mimicking catalase.
Noble metals.
The HOMO- and LUMO-mediated mechanisms have also been proposed for catalase-mimicking nanocatalysts. For example, the LUMO-mediated H2O2 decomposition mechanism was proposed for noble metals, such as Au, Ag, Pd, and Pt mimicking catalase, using a pre-adsorbed OH group to simulate the basic environment.255 In this mechanism, the first H2O2* is decomposed into HOO* and H, with the resulting H automatically bonding with the pre-adsorbed OH to yield a H2O molecule (Fig. 22a). Subsequently, the HOO* transfers its H to another H2O2 molecule, releasing an O2 molecule and converting H2O2 to H2O* and HO* (C → D, RDS). The energy barriers of the RDS are higher for Au(111) and Ag(111) than for Pt(111) and Pd(111), consistent with the catalase-like activities of these noble metals in alkaline conditions.255 This mechanism allows for the understanding of the catalase-like activities of other noble metal-based NMs, such as graphdiyne-templated Pd nanoparticles and Hg@Au amalgams.257,293
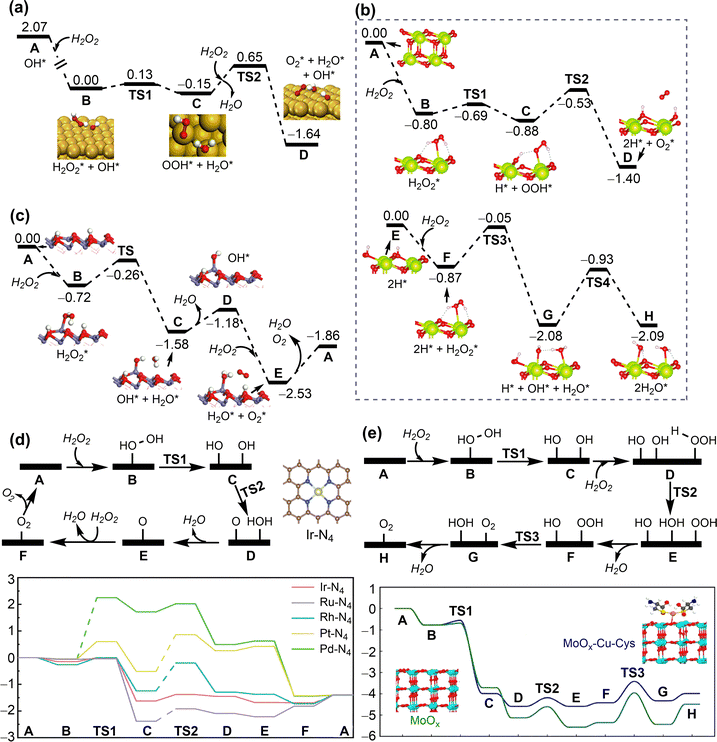 |
| Fig. 22 Mechanisms and energy profiles (in eV) for nanomaterials mimicking catalase. (a) Au(111) in alkaline conditions. Reproduced with permission from ref. 255. Copyright 2015 Elsevier. (b) CeO2(111). Reproduced with permission from ref. 285. Copyright 2019 the Royal Society of Chemistry. (c) H-terminated Fe5HO8(100) surface of ferrihydrite. Reproduced with permission from ref. 294. Copyright 2021 CC BY-NC-ND 4.0. (d) Platinum group metal-based M-NC SACs. Reproduced with permission from ref. 295. Copyright 2023 Wiley-VCH. (e) Comparison between MoOx-Cu-Cys SAC and MoOx. Reproduced with permission from ref. 296. Copyright 2023 American Chemical Society. | |
Metal oxides.
The LUMO-mediated mechanism also holds for nanoceria with catalase-like activity. As shown in Fig. 22b, the first H2O2 is oxidised by CeO2(111), resulting in the formation of an O2 molecule and H2–CeO2(111) (A → D), which is then oxidised by another H2O2 to yield two H2O molecules (E → H).285 These two processes are both thermodynamically and kinetically favourable. In comparison with the Ov-free CeO2, the reaction energy for H2O2 oxidation on Ov-containing CeO2 is less negative. Therefore, a more reduced CeO2 nanosurface has a lower ability to oxidise H2O2, consistent with the experimental observation that a larger Ce3+/Ce4+ ratio leads to a lower catalase-like activity of nanoceria.297 However, further investigation is warranted since the reaction following the HOMO-medicated mechanism may become dominant when the nanoceria surface is sufficiently reductive. Such a phenomenon has been observed in ferrihydrite with catalase-like activity.294 As shown in Fig. 22c, the H atom of the surface hydroxyl facilitates the O–O bond cleavage of the first H2O2* (HOMO-mediated mechanism), producing HO*, H2O*, and an H-vacancy (B → C). The resulting OH* and H-vacancy can readily capture H atoms of the second H2O2 to generate O2* and H2O* (D → E).294
Single-atom catalysts.
The HOMO-mediated mechanism was proposed for platinum group metal-based M-NC single-atom catalysts (SACs) with catalase-like activity. As shown in Fig. 22d, the adsorbed H2O2 molecule undergoes the homolytic cleavage into two HO* (B → C), which recombine into O* and H2O* (C → D).295 The RDS for Pd-N4 is the step of B → C, while the step of C → D is the RDS for Rh-N4, Ru-N4, and Pt-N4. For Ir-N4, all of these steps can occur with small energy barriers. Therefore, the homolytic cleavage of H2O2 and the HO recombination are two crucial steps in determining the overall catalase-like activities of platinum group M-NC SACs. The calculated energy barriers of the RDS follow the order of Ir-N4 < Ru-N4 < Rh-N4 < Pt-N4 < Pd-N4, suggesting their decreasing catalase-like activities, as validated in the experiment.295 A similar mechanism was proposed to elucidate the difference in catalase-like activity among Co-based SACs with different coordination configurations.298 In addition to the carbonaceous-supported SACs, the catalytic mechanisms for metal oxide-supported SACs with catalase-like activity have also been reported. For example, the HOMO-mediated mechanism was employed to explain the difference in the catalase-like activity of the SAC MoOx–Cu–Cys and MoOx (Fig. 22e).296
Summary.
The mechanisms underlying catalase-like nanocatalysis can be broadly outlined as follows:
(1) The first H2O2 is decomposed into OH* or O*, which subsequently react with the second H2O2 to generate O2 and H2O. This mechanism has been found in the case of MoOx–Cu–Cys and platinum group metal-based M-NC SACs.
(2) The first H2O2 is decomposed into O2 and 2H*, followed by the reaction of the second H2O2 with 2H* to generate 2H2O. CeO2(111) is a representative catalase-like nanocatalysis showing this mechanism.
(3) The pre-adsorbed OH group on nanosurfaces plays an important role in the catalase-like activities of NMs, as evidenced on Au(111). These mechanisms can rationalize well the catalytic activities for NMs mimicking catalase.
5.2.5. Mechanisms for nanodrugs catalysing organophosphate hydrolysis.
A growing number of theoretical studies have been conducted to explore the mechanisms of organophosphate hydrolysis by nanocatalysts, including noble metals, metal oxides, metal organic frameworks (MOFs), and polyoxometalates (POMs). Organophosphate substrates, such as nerve agents (sarin and soman) and their simulants (dimethyl p-nitrophenolphosphate (DMNP), dimethyl methylphosphonate (DMMP), and dimethyl chlorophosphite (DMCP)), as well as the chromogenic substrate p-nitrophenyl phosphate (p-NPP), have been considered in these calculations.
Metal- and metal oxide-catalysed organophosphate hydrolysis.
(a) Sarin hydrolysis.
The mechanism for sarin hydrolysis on ZnO299 was investigated using the hydroxylated ZnO(10
0) surface as the model system.300Fig. 23a shows the elementary steps in the proposed mechanism: (i) sarin adsorption on the surface through hydrogen bonding interactions between the phosphoryl oxygen and two surface hydroxyl groups, (ii) isomerisation of the sarin-adsorbed complex, (iii) the subsequent hydrolysis of the P–F bond, resulting in the formation of isopropyl methyl phosphonic acid (IMPA) on a Zn atom, (iv) the migration of the fluorine atom between neighbouring Zn atoms, and (v) finally the desorption of IMPA and hydrogen fluoride (HF) from surface. The calculated energy profiles show that the energy barrier for the decomposition of sarin is moderate (A → F, 0.94 eV), but the energy required for the final product desorption is considerably high (F → A, 2.88 eV). Thus, it is likely that ZnO could be employed to effectively hydrolyse sarin at room temperature under humid conditions. However, the products would remain on the surface, thereby hindering the recycling of the catalyst.
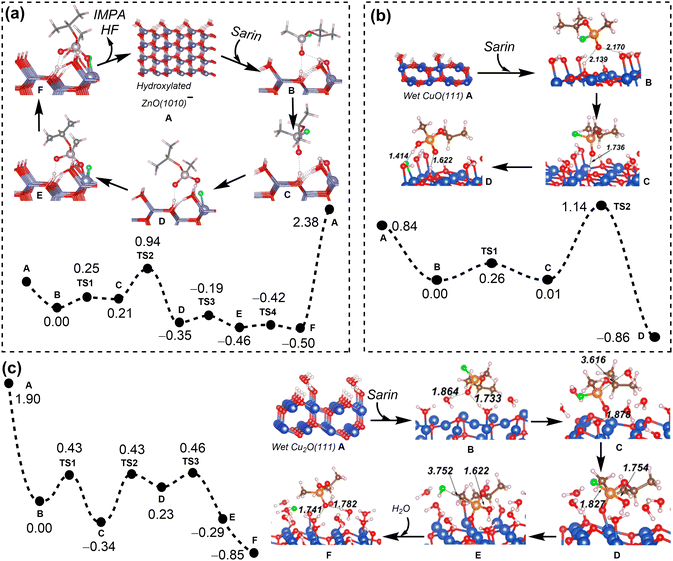 |
| Fig. 23 Mechanisms and energy profiles (in eV) for metal oxide-catalysed organophosphate (sarin) hydrolysis. (a) Hydroxylated ZnO(10 0). Reproduced with permission from ref. 299. Copyright 2019 American Chemical Society. (b) Wet CuO(111). (c) Wet Cu2O(111). Reproduced with permission from ref. 301. Copyright 2021 American Chemical Society. | |
Similar mechanisms were found for sarin hydrolysis on two copper oxides (CuO and Cu2O) under the humid conditions.301 For the wet CuO(111), the reaction process is similar to that on the hydroxylated ZnO(10
0) surface (Fig. 23b), with an overall energy barrier of 1.13 eV. Unlike the sarin degradation on wet CuO(111) initiated by the nucleophilic attack of surface hydroxyl group, the hydrolysis of sarin on wet Cu2O(111) surface arises from the nucleophilic attack of a surface water molecule (Fig. 23c). The total energy barriers for sarin hydrolysis on these two surfaces are not large, with the values of 1.14 eV for wet CuO(111) and 0.80 eV for wet Cu2O(111), suggesting that both copper oxides may be promising for the hydrolytic decontamination of sarin.301
(b) DMNP hydrolysis.
The hydrolysis of DMNP on noble metal Au and metal oxide ZrO2 followed a mechanism including the following steps: (i) DMNP and H2O adsorption on the surface, (ii) H2O decomposition, (iii) OH attack to the P centre of DMNP, (iv) P–OPhNO2 σ-bond cleavage, and (v) regeneration of the catalyst (Fig. 24a).251 The calculated overall energy barrier is moderate for m-ZrO2(
11) (0.78 eV) but extremely high for fcc-Au(111) (1.95 eV). This is consistent well with the experimental observation that zirconia exhibited superior activity in catalysing the hydrolysis of organophosphates, whereas gold did not.248,302 For fcc-Au(111), the step i is exothermic, the step iii can proceed with a small energy barrier, the step iv is nearly barrierless, and the step v is thermodynamically favourable. Nevertheless, the step ii for this NM is highly endothermic and has to overcome a very high energy barrier of 1.89 eV. This indicates that the H2O decomposition is the key elementary step that leads to the poor activity of gold. For m-ZrO2(
11), the OH attack on the P centre of DMNP is the key elementary step that determines the overall catalytic activity of this nanomaterial.
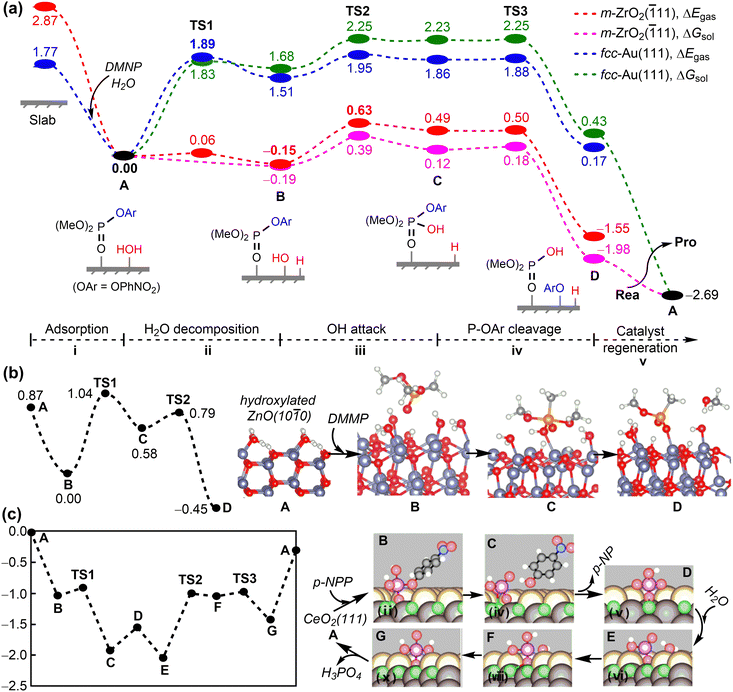 |
| Fig. 24 Mechanisms and energy profiles (in eV) for metal- and metal oxide-catalysed organophosphate (DMNP, DMMP, and p-NPP) hydrolysis. (a) Comparison between m-ZrO2( 11) and fcc-Au(111) for DMNP hydrolysis. Reproduced with permission from ref. 251. Copyright 2023 American Chemical Society. (b) Hydroxylated ZnO(10 0) for DMMP hydrolysis. Reproduced with permission from ref. 300. Copyright 2019 American Chemical Society. (c) CeO2(111) for p-NPP hydrolysis. Reproduced with permission from ref. 303. Copyright 2018 Elsevier. | |
(c) DMMP hydrolysis.
The mechanism for the hydrolysis of DMMP on metal oxides is similar to that of sarin. As shown in Fig. 24b, the surface hydroxyl serves as a nucleophile to attack on the P atom of DMMP, resulting in the formation of a 5-fold coordinated phosphorus atom (B → C). Subsequently, the P–OCH3 bond is cleaved and the methanol is formed via an intermolecular hydrogen transfer from the surface water molecule to the OCH3 group. In this reaction, the step of B → C is the RDS with an energy barrier of 1.04 eV, suggesting that the hydroxylated ZnO surface exhibits a good catalytic activity towards the hydrolysis of DMMP.300 A similar mechanism was also found for the hydrolysis of DMMP on mesoporous CeO2. The energy barrier for the degradation of DMMP into methanol on the hydroxylated CeO2(110) surface is 1.15 eV, higher than that on the pristine CeO2 surface. Therefore, the degradation of DMMP on the hydroxylated CeO2 surface may occur at higher temperatures or proceed after being dehydroxylated.304
(d) p-NPP hydrolysis.
A surface-assisted hydrolysis mechanism was proposed for CeO2(111)-catalysed p-NPP hydrolysis in the gas phase.303 As shown in Fig. 24c, this mechanism involves p-NPP adsorption on the surface via P–Olatt bonding interaction, P–O ester bond scission, the formation and desorption of p-nitrophenol (p-NP), the hydration of the remaining phosphate group (HPO3), and finally the desorption of phosphoric acid (H3PO4). In this catalytic reaction, the P–O ester bond scission (B → C) is facile due to the weakening of the P–O ester bond by the pentavalent P centre of the adsorbed p-NPP. The rate-limiting steps are the hydration of HPO3 (E → G) and the desorption of H3PO4 (G → A), with an overall energy barrier of approximately 1.1 eV. Given that the barrier obtained in gas phase would be expected to be reduced by the solvation effect, it is possible that the high catalytic activity of nanoceria for p-NPP hydrolysis can be experimentally observed in aqueous solutions.305
MOF-catalysed organophosphate hydrolysis.
(a) Sarin hydrolysis.
The hydrolysis of sarin on MOFs usually occurs with a water molecule acting as the nucleophile. This reaction mainly includes three stages: (i) the displacement of water molecule for the binding of sarin to an open Lewis acidic metal site, such as ZrIV, (ii) the nucleophilic attack of water at the phosphorus centre of sarin, and (iii) the HF elimination by P–F bond scission.306 For example, a general base catalytic mechanism was proposed for the hydrolytic degradation of sarin on defective UiO-66 under ambient conditions.307 As shown in Fig. 25a, sarin coordinates to the undercoordinated Zr atom of MOF and H2O is weakly bound to the MOF. The weakly bound water molecule undergoes dissociation to protonate the Zr-OH group of the MOF, and the nascent hydroxide adds concertedly to sarin. The –F substituent is located in an equatorial position of the resulting pentacoordinated intermediate C, which has to undergo Berry pseudorotation directing –F to an axial site for the subsequent HF elimination. The moderate energy barrier of this hydrolytic process (A → F, 0.76 eV) suggests that sarin could be readily decomposed on the defective UiO-66 via hydrolysis under ambient conditions. However, the large binding energy of IMPA with MOF (2.12 eV) makes the product inhibition inevitable without thermal treatment.
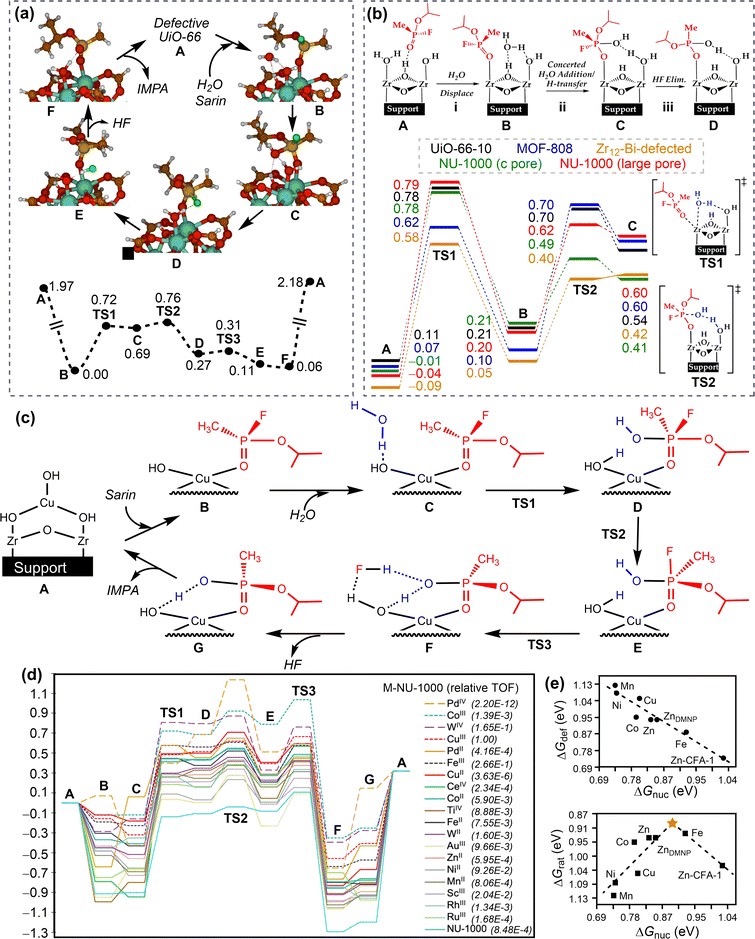 |
| Fig. 25 Mechanisms and energy profiles (in eV) for MOF-catalysed organophosphate (sarin and soman) hydrolysis. (a) Defective UiO-66 for sarin hydrolysis. Reproduced with permission from ref. 307. Copyright 2016 American Chemical Society. (b) Comparison among different Zr-MOFs for sarin hydrolysis. Reproduced with permission from ref. 306 and 308. Copyright 2018 American Chemical Society. (c) and (d) Comparison among different M-NU-1000 systems for sarin hydrolysis. Reproduced with permission from ref. 309. Copyright 2020 American Chemical Society. (e) Linear relationship of ΔGdef with ΔGnuc and volcano curve of ΔGrat as a function of ΔGnuc for M-MFU-4l-(OH) catalysed soman hydrolysis. Reproduced with permission from ref. 310. Copyright 2022 American Chemical Society. | |
Mechanistic studies were also conducted on the hydrolytic degradation of sarin on Zr-based MOFs (MOF-808 and NU-1000). The energy barrier for MOF-808 is lower than those for NU-1000 and UiO-66-10 (Fig. 25b), in agreement with the experimental observation that MOF-808 was the fastest catalyst for sarin hydrolysis in buffered aqueous solutions.311 It was also predicted that the reactivity of one double-node Zr12-bi-defective MOF for sarin hydrolysis would be greater than that of the above single-node Zr6 MOFs.308
As discussed above, the catalytic hydrolysis of sarin by Zr-based MOFs in the gas phase may be inhibited by product inhibition. In addition, the inhibition resulting from the bidentate binding of IMPA could be more pronounced than that resulting from the monodentate binding mode.307 A comprehensive screening of single-atom transition metal catalysts deposited on the nodes of MOFs such as NU-1000 (M-NU-1000 system) for the catalytic hydrolysis of sarin has been conducted to enhance the catalyst activity.309 In this catalytic reaction, the deposited M-OH is the active centre and the proposed mechanism (Fig. 25c) involves substrates (sarin and H2O) adsorption, nucleophilic attack, Berry pseudorotation, HF elimination, and IMPA desorption. Given the variability in the RDS among these catalysts (Fig. 25d), the relative turnover frequencies (TOFs) were employed to determine the efficiency of each catalyst. The CuIII-NU-1000 system was identified as the most promising candidate for the catalytic hydrolysis of sarin, showing an estimated activity that is over 1000 times greater than that of NU-1000.
(b) Soman hydrolysis.
A systematic theoretical investigation on the catalytic hydrolysis of soman was conducted on a series of single-atom MOFs, M-MFU-4l-(OH) (M = Mn, Fe, Co, Ni, Cu, and Zn).310 The proposed mechanism for the hydrolysis of soman involves the following steps: (i) the adsorption of soman on the M site of MOF and that of H2O interacting with M-bound hydroxyl group, (ii) water-triggering nucleophilic attack to form the pentacoordinated phosphorus intermediate, (iii) the elimination of HF by combining the leaving F and the hydrogen of P-OH group, and (iv) the desorption of the formed product pinacolyl methylphosphoric acid. The energy profiles indicate that the steps ii and iv are two crucial steps for this catalytic reaction; step ii is the rate-limiting step for Fe-MFU-4l-(OH) and step iv is the rate-limiting step for other M-MFU-4l-(OH). Based on these two crucial steps, the related linear relationships and the volcano curve have been established (Fig. 25e), which show that the rate-limiting energy barrier for Fe-MFU-4l-(OH) is the closest to the optimal volcano peak. Thus, the Fe-MFU-4l-(OH) should be the most promising candidate for the hydrolytic detoxification of soman among those MOF catalysts.
(c) DMNP hydrolysis.
The mechanism for the hydrolysis of DMNP on Zr-based NU-1000 was investigated in both alkaline buffer solutions and the gas phase.256,312 For solution hydrolysis (Fig. 26), the OH− anion was used as the nucleophile. The reaction proceeds as follows: (i) the DMNP is adsorbed on the open coordination Zr site, (ii) the OH− anion from the solution attacks on the phosphorous atom, giving rise to the simultaneous departure of the p-nitrophenoxide from DMNP, and (iii) the formed dimethyl ester of phosphoric acid (DMPA) is desorbed from MOF and the catalyst is regenerated. The calculated energy barrier (0.45 eV) for this catalytic reaction is consistent with the remarkable catalytic activity of NU-1000 observed in alkaline buffer solutions.313 This hydrolysis mechanism in solution was employed to understand the activity order of UiO-66-NH2 > UiO-66-H > UiO-66-NO2 in the catalytic hydrolysis of DMNP.314 Similar mechanisms were found for the hydrolysis of DMNP on two Ti-based MOFs (Ti-MFU-4l and NU-1012-NDC) in basic aqueous solutions.315 The overall reaction processes are comparable, yet the nucleophile differs. In Ti- MFU-4l, the Ti(OH)3 centre is the catalytic site that can provide the HO group as the nucleophile. In contrast, in NU-1012-NDC, the Ti(IV)-OH-Ti(IV) centre is the active site, where the HO group is difficult to leave and attack on the P atom of DMNP. Therefore, the OH− anion is the nucleophile in the reaction. The calculated energy profiles suggest that the hydrolysis of DMNP is considerably more facile on Ti-MFU-4l than on NU-1012-NDC, in agreement with their overall catalytic rates for DMNP hydrolysis.315
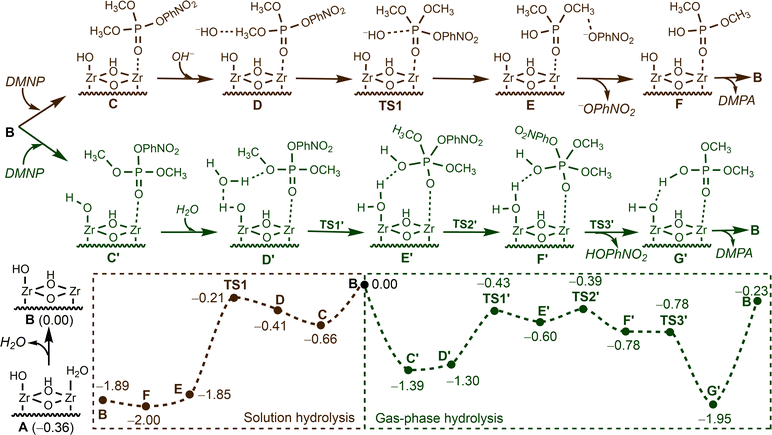 |
| Fig. 26 Mechanisms and energy profiles (in eV) for NU-1000-catalysed organophosphate (DMNP) hydrolysis in solution and gas phase. Reproduced with permission from ref. 256 and 312. Copyright 2018, 2020 American Chemical Society. | |
In the gas phase, the H2O molecule acts as the nucleophile in the hydrolysis process of DMNP by NU-1000. As shown in Fig. 26, the reaction proceeds via five key elementary steps. First, DMNP is adsorbed onto MOF. Next, The H2O molecule is introduced to attack on the phosphorus atom, with one hydrogen atom transferring to the nearby Zr-bound hydroxyl group and the remaining OH group attacking on the phosphorus centre to form a penta-coordinated phosphorus intermediate. This intermediate undergoes a Berry pseudorotation to place the p-nitrophenoxide group in the axial position. Subsequently, the product p-nitrophenol is formed and departs, resulting from the cleavage of the P–OPhNO2 bond and the binding of OPhNO2 and H in the P-OH group. Finally, the catalyst is recovered by the desorption of product DMPA. In contrast to the low energy barrier for DMNP hydrolysis in solution, the calculated energy barrier for the hydrolysis in gas phase is significantly high (1.72 eV). The desorption of the product (G′ → B) is the RDS, indicating that the efficiency of the catalyst would be significantly hindered by strong product inhibition in the gas phase.312 To reduce the product inhibition, a series of NU-1000-supported bimetallic metal–oxo systems were computationally screened. The Al2O2(OH)2@NU-1000 was identified as the most promising candidate, exhibiting the lowest product desorption energy. This suggests a promising strategy to enhance the performance of MOFs for the catalytic hydrolysis of DMNP in the gas phase.312
POM-catalysed organophosphate hydrolysis.
A general base-catalysed mechanism was proposed for the hydrolysis of DMMP on Cs8[Nb6O19]·xH2O polyoxoniobate (CsPONb).316 In this mechanism, the adsorption of H2O and DMMP on CsPONb occur first followed by a concerned water dissociation and nucleophilic addition. The dissociation of the water molecule leads to the protonation of the polyniobate oxygen and the generation of a hydroxide, which further attacks on the phosphorus atom of DMMP, leading to the formation of a pentacoordinated phosphorus intermediate. Subsequently, this intermediate undergoes a facile dissociation to produce CH3OH and methyl methylphosphonic acid (MMPA). The calculated energy profile suggests that DMMP can be readily decomposed on CsPONb with an energy barrier of 0.47 eV for the rate-limiting step. However, the catalyst reactivation is unlikely under ambient conditions because of the large desorption energy of MMPA (2.56 eV), as confirmed by in situ synchrotron-based spectroscopic measurements.316
The general base-catalysed mechanism also holds for the hydrolysis of DMCP on a single-site zirconium polytungstate (Zr-POM monomer).317 After the adsorption of DMCP to the undercoordinated Zr(IV) Lewis acid site of Zr-POM monomer, the reaction is initiated by the nucleophilic addition of a Zr hydroxo ligand to the bound DMCP. This addition process occurred through a small energy barrier of 0.46 eV, leading to the formation of a shallow pentacoordinated phosphorus intermediate. Subsequently, the reaction proceeds through chloride elimination with a proton of the aqua ligand of Zr to form the HCl and DMPA bound to Zr-POM. Finally, the catalytic cycle is completed by HCl desorption, DMPA desorption, and the binding of an ambient water molecule.
Summary.
Based on above theoretical studies, it can be concluded the nucleophilic attack on the phosphorus atom of organophosphates is an important step in the hydrolytic cleavage of phosphoesters and other P-containing σ-bonds. The nucleophiles can be a H2O molecule, a HO group on the surface or an OH− anion in the buffer solution, as outlined below:
(1) The water molecule acts as a nucleophile, which has been found in the hydrolysis of sarin on Cu2O, UiO-66, MOF-808, NU-1000, and M-NU-1000 systems; soman on M-MFU-4l-(OH); DMNP on NU-1000; and DMMP on CsPONb. In these cases, the water molecule undergoes dissociation, resulting in the protonation of the M-OH groups on nanosurfaces. Subsequently, the nascent hydroxide adds concertedly to the phosphorus atom of the organophosphate.
(2) The surface hydroxyl group acts as a nucleophile, which can be formed by the decomposition of H2O* into H* and OH* or comes from the nanomaterial itself. Examples of the former include the hydrolysis of sarin on ZnO and CuO; DMNP on Au and ZrO2; and DMMP on ZnO and CeO2. The latter case has been observed in the hydrolysis of DMNP on Ti-MFU-4l and DMCP on Zr-POM.
(3) The OH− anion in the alkaline buffer solution acts as a nucleophile, as evidenced by the hydrolysis of DMNP hydrolysis on NU-1000, UiO-66-X, and NU-1012-NDC.
In addition to the nucleophilic attack, the decomposition of a H2O molecule to generate a surface hydroxyl group was also an important step in organophosphate hydrolysis, as elucidated by the hydrolysis of DMNP on Au and ZrO2. Furthermore, the final product desorption is also a significant factor to consider, particularly in the gas phase. Overall, the mechanisms reviewed herein provide a comprehensive rationale for the NM-catalysed hydrolysis of organophosphates.
5.2.6. Mechanisms for nanodrugs catalysing other biochemical reactions.
Nitric oxide generation.
A mechanism for the generation of NO from GSNO in the presence of GSH, in which a Cu-BTTri metal–organic framework functions as the catalyst, has been proposed. As shown in Fig. 27a, the first GSNO molecule is adsorbed to the Cu site of Cu(II)-GSH (B), followed by the S–N bond cleavage to produce NO˙. Subsequently, a copper-mediated GSH-GS˙ reductive elimination reaction occurs, resulting in the formation of a Cu(I) intermediate E and GSSGH+. Upon the introduction of the second GSNO, a Cu(I) to Cu(II) oxidation-assisted S–N homolysis occurs to produce the second NO˙. While only the thermodynamic reaction processes have been investigated, the initial S–N cleavage step (C → D) could be considered as the rate-limiting step for this catalytic reaction.318
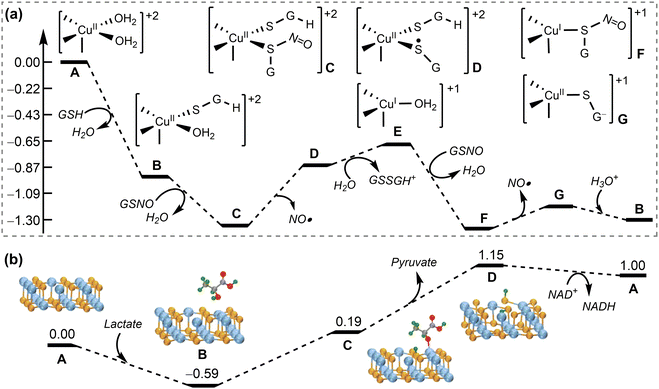 |
| Fig. 27 Mechanisms and energy profiles (in eV) for nanomaterial-catalysed other biochemical reactions. (a) Cu-BTTri MOF-catalysed NO generation from GSNO. Reproduced with permission from ref. 318. Copyright 2023 American Chemical Society. (b) SnSe-catalysed lactate dehydrogenation. Reproduced with permission from ref. 243. Copyright 2020 Wiley-VCH. | |
Lactate dehydrogenation.
A possible mechanism for SnSe mimicking lactate dehydrogenase was proposed (Fig. 27b), which involves the adsorption of lactate on the SnSe surface, the transfer of two hydrogens from the adsorbed lactate (polar hydroxyl H and α-H) to two surface Se atoms to release pyruvate, and the transfer of two chemisorbed H atoms to NAD+ (nicotinamide adenine dinucleotide), resulting in the formation of NADH. Despite the uphill energy profile calculated in a vacuum, it has been suggested that these processes could be feasible in real reaction conditions, given that the energy increase is not significant.243
5.3. Activity prediction models
5.3.1. Activity prediction models for nanomaterials mimicking peroxidase.
As shown in Fig. 28a, the eg occupancy could be an effective activity descriptor for perovskite oxides mimicking peroxidase. This is evidenced by the observation of a volcano relationship between catalytic activity and eg, with the highest activity occurring at an eg occupancy of approximately 1.2.319 This eg descriptor is also applicable to spinel oxides mimicking peroxidase, with the highest activity occurring at an eg occupancy of ∼0.6.320 These models have suggested that LaNiO3−δ with an eg of 1.22 and LiCo2O4 with an eg of 0.62 have the highest peroxidase-like activities among a series of perovskite- and spinel-oxides, respectively. Both of these predictions have been validated by experiments or DFT calculations.
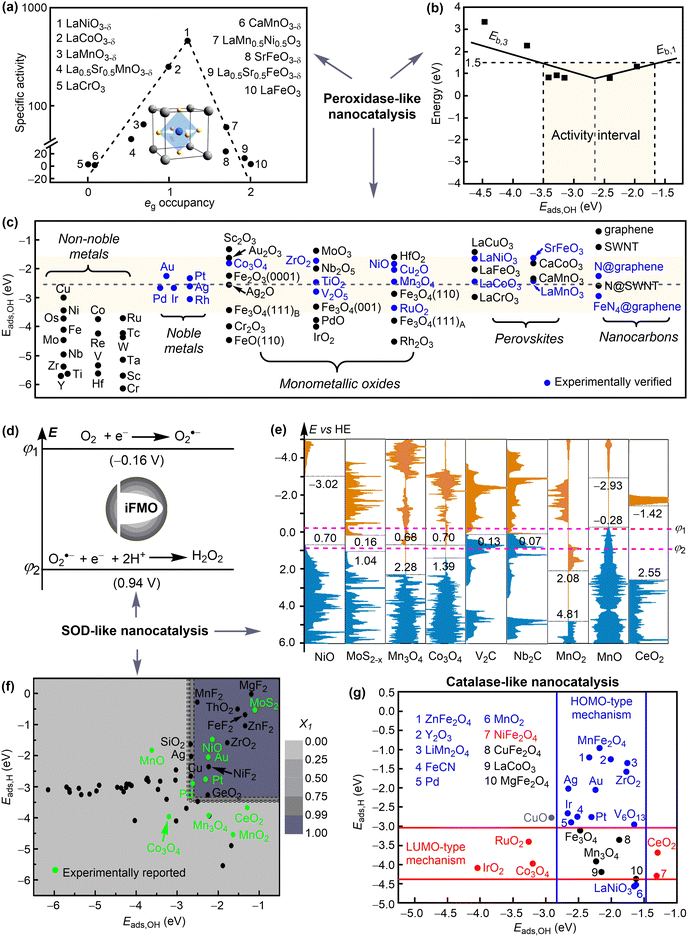 |
| Fig. 28 Activity prediction models for nanodrugs regulating ROS. (a) Peroxidase-like activities of perovskite oxides plotted as a function of eg occupancy. Reproduced with permission from ref. 319. Copyright 2019 CC BY 4.0. (b) Peroxidase-like activities of iron oxides plotted as a function of Eads,OH. (c) Overview of peroxidase-like activities of nanomaterials using the prediction model. Reproduced with permission from ref. 260. Copyright 2020 American Chemical Society. (d) and (e) Energy level principle for nanomaterials mimicking SOD and the calculated electronic density of states with the energies of FMOs marked. (f) Adsorption energy principle for nanomaterials mimicking SOD, which shows the contour plot of the partition function X1 as a function of Eads,OH and Eads,H. Reproduced with permission from ref. 286. Copyright 2021 CC BY 4.0. (g) Eads,OH- and Eads,H-based prediction model for nanomaterials mimicking catalase. Reproduced with permission from ref. 26. Copyright 2023 Wiley-VCH. | |
A more general and simple energy-based descriptor was proposed based on the DFT-calculated peroxidase-like mechanisms of iron oxides (Fig. 19d).260 By analysing the key factors that dominate the thermodynamics and kinetics of the peroxidase-like nanocatalysis, it was found that Eb,1, Er,3, and Eb,3 are all linearly dependent on Er,1; Er,1 (Er,3) and Eb,1 (Eb,3) represent the reaction energy and barrier of the RDS step i (step iii), respectively. In addition, the Er,1 exhibits an approximately linear relationship with the adsorption energy of OH on the nanosurface (Eads,OH), which is a more straightforward parameter to calculate than Er,1. Thus, the Eads,OH could be a simple and effective activity descriptor for NMs mimicking peroxidase, and a volcano relationship between catalytic activity has been observed (Fig. 28b). The activity criterion corresponding to Eb,RDS < 1.5 eV is −3.5 eV < Eads,OH < −1.6 eV, with a maximum activity when Eads,OH = −2.6 eV. This activity prediction model is applicable not only to iron oxides but also to other NMs that mimic peroxidase via a similar mechanism. For example, the peroxidase-like activities of various nanomaterials predicted using this model agree well with the available experimental results (Fig. 28c).
5.3.2. Activity prediction models for nanomaterials mimicking oxidase.
The d-band centre (εd) has been proposed as a potential descriptor for the relative activities of metals mimicking oxidase. A higher εd level is indicative of a better oxidase-like activity.273 In addition, the Hammett σm may also serve as an effective descriptor for the relative activities of metal organic frameworks mimicking oxidase. It was shown that increasing the σm value with an electron-withdrawing ligand can enhance the oxidase-like activity of MIL-53(Fe).280 Consequently, the MIL-53(Fe)-NO2 with the most electron-withdrawing NO2 substituent exhibited the highest oxidase-like activity among a series of MIL-53(Fe)-X (X = NH2, CH3, H, OH, F, Cl, Br, and NO2).
5.3.3. Activity prediction models for nanomaterials mimicking SOD.
Two principles have been proposed to predict the SOD-like activity of NMs, namely, the energy level principle and the adsorption energy principle.286 The energy level principle (Fig. 28d) suggests that NMs should possess at least one FMO with an energy level between φ1 and φ2, where φ1 and φ2 represent the reduction potentials of O2/O2˙− (−0.16 V) and O2˙−, H+/H2O2 (0.94 V), respectively. Such an FMO is named as the intermediate frontier molecular orbital (iFMO). This principle ensures that the half reactions are both thermodynamically favourable for NM-catalysed superoxide disproportionation. Actually, most of the NMs with the SOD-like activity observed in experiments have FMOs located within the desired energy window (Fig. 28e). In addition, this principle provides a systematic perspective on the catalytic mechanisms for NMs mimicking SOD, suggesting that NMs with an occupied FMO as iFMO (such as NiO) would follow a HOMO-mediated mechanism, whereas NMs with an unoccupied FMO as iFMO (such as MoS2−x) would follow a LUMO-mediated mechanism. This iFMO-dependent manner was verified by a detailed mechanism investigation (Fig. 21c and d). The adsorption energy principle suggests that the adsorption energy of some key intermediates in the reaction process should be in the range of Eads,OH > −2.7 eV and Eads,H > −3.4 eV. This principle can be used to quantitatively describe the competition between the target catalytic reaction and undesired side reactions, ensuring that the ratio for the main reaction is larger than 50%. Both of these two principles are critical criteria in the screening of NMs with the SOD-like activity (Fig. 28f).
5.3.4. Activity prediction models for nanomaterials mimicking catalase.
An adsorption energy-based activity prediction model has been proposed to predict the catalytic activity of NMs mimicking catalase based on the HOMO- and LUMO-type catalytic mechanisms.26 In this model, the Eads,OH and Eads,H are the activity descriptor for HOMO- and LUMO-type catalase-like catalysis, respectively, because the Gibbs free energy changes for the reactions can be approximately described as linear functions of Eads,OH and Eads,H, respectively. The corresponding energy windows are −2.83 eV < Eads,OH < −1.47 eV for the HOMO-type and −4.38 eV < Eads,H < −3.01 eV for LUMO-type catalase-like catalysis, respectively. The validity of this adsorption energy-based activity prediction model is supported by the observation that the vast majority of experimentally reported NMs exhibiting catalase-like activities are located within the blue or pink regions, which represent the activity criterion of HOMO- or LUMO-type catalase-like catalysis, respectively (Fig. 28g).
5.3.5. Activity prediction models for nanodrugs catalysing organophosphate hydrolysis.
As mentioned above, H2O decomposition and OH attack are the two key elementary steps that determine the overall catalytic activities of gold and zirconia, respectively, in the catalytic hydrolysis of organophosphates such as DMNP (Fig. 29a).251 The analysis of these two key elementary steps for a series of metals and metal oxides indicated that the energy barriers of both steps can be approximated by the linear functions of coadsorption energy of OH and H, Eads,OH-H, suggesting Eads,OH-H to be the effective activity descriptor. Based on the Eads,OH-H, the corresponding activity prediction models, named coadsorption energy models have been developed. For metals, the activity criterion is −7.65 eV < Eads,OH-H < −6.05 eV, with a maximum activity at Eads,OH-H = −6.68 eV. For metal oxides, the activity criterion is −6.99 eV < Eads,OH-H < −5.21 eV, with a maximum activity at Eads,OH-H = −6.31 eV (Fig. 29a). This model is capable of not only elucidating the catalytic activities of previously reported metal and metal oxides, but also identifying promising candidate, such as Ru (Fig. 29b), for the catalytic hydrolysis of phosphoester bonds.
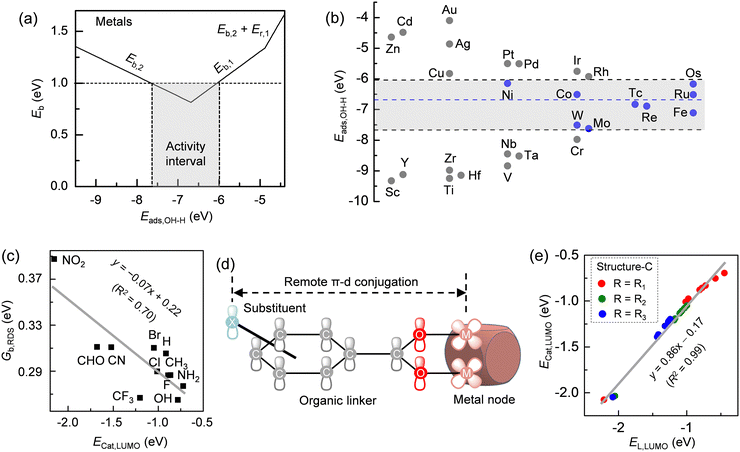 |
| Fig. 29 Activity prediction models for nanodrugs catalysing organophosphate hydrolysis. (a) Piecewise function of overall energy barrier (Eb) with coadsorption energy Eads,OH-H for metal catalysed DMNP hydrolysis. (b) Prediction of the catalytic activities for metal nanomaterials. Reproduced with permission from ref. 251. Copyright 2023 American Chemical Society. (c)–(e) Linear relationship of Gb,RDS with Ecat,LUMO and that of Ecat,LUMO with EL,LUMO for UiO-66-X catalysed DMNP hydrolysis. The remote π-d conjugation between the linker substituent and nodal metal of MOF is also presented. Reproduced with permission from ref. 314. Copyright 2023 CC BY 4.0. | |
In addition to the adsorption energy-based activity prediction model, a linker orbital energy model was proposed to understand and predict the remote substituent effects on the catalytic activities of MOFs, such as UiO-66-X for DMNP hydrolysis.314 As shown in Fig. 29c–e, the Gb,RDS (free energy barriers of the rate-determining steps) exhibits a negative correlation with the LUMO energies of the catalysts, ECat,LUMO. Moreover, the ECat,LUMO shows a strong linear relationship with the EL,LUMO (frontier orbital energies of the linkers), which originates from the remote π-d conjugation between the linker substituent and nodal metal. Therefore, the remote electronic effects of substituents on the catalytic activity of metal nodes of MOFs can be quantitatively described by eqn (2),
| 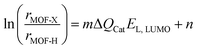 | (2) |
where
rMOF-X and
rMOF-H represent the catalytic reaction rates of substituted and unsubstituted MOFs, respectively. The Δ
QCat represents the charge variation of the catalyst. This model is applicable to MOFs with varying linkers and substituents.
5.3.6. Activity prediction models for nanodrugs catalysing other biochemical reactions.
To the best of our knowledge, the activity prediction models for NM-catalysed NO generation have not been reported. For NM-catalysed lactate dehydrogenation, it has been suggested that NMs showing a prominent lactate dehydrogenase (LDH)-like catalytic efficiency should have an appropriate hydrogen affinity (Eads,H), neither too strong nor too weak. This was evidenced by the experimentally determined LDH-like activities of a series of nanomaterials and their calculated Eads,H.243 However, the quantitative prediction models are still absent, likely due to the lack of investigation into the corresponding reaction mechanisms.
5.4. Summary
In summary, nanomaterials can catalyse the transformation of biological substances like ROS for disease prevention and therapy. The development of an activity prediction model for a specific biochemical reaction catalysed by nanomaterials is a crucial step in enabling the sufficient screening and discovery of catalysis-based nanodrugs. These models can be either experiment- or computation-driven, as outlined above. From a computational perspective, deriving such models necessitates a profound understanding of the underlying mechanisms by DFT calculations and the identification of the critical elementary steps that govern the overall catalytic activity. The energy change and barrier associated with these elementary steps should exhibit a linear dependence on a physical parameter that can be readily calculated, typically the adsorption energy of an intermediate in the reaction process. For example, the hydroxyl adsorption energy (Eads,OH) can correlate well with the reaction barriers for both H2O2 activation and subsequent TMB oxidation, which represent the key elementary steps in evaluating the activity of peroxidase-like nanocatalysts. Specifically, the physical parameter usually reversely influences the energy barriers of key elementary steps. As a result, an optimal value can be assessed to achieve the maximum catalytic activity of nanomaterials. In accordance with this principle, high-throughput DFT calculations of the aforementioned physical parameter can be conducted to identify appreciate nanomaterials with the desired catalysis-based medical functions. The activity prediction models have been derived for the prediction of the catalytic activity of nanomaterials for the regulation of ROS and organophosphate hydrolysis. Given the diverse biochemical reactions that can act as the therapeutic target, the identification of targeted reactions and the establishment of activity prediction models represent a promising avenue for the future discovery of catalysis-based nanodrugs.
6. Chemical conversion-based nanodrugs
Another important property of nanomaterials is their ability to undergo chemical conversion, which enables their applications as chemical conversion-based nanodrugs. The chemical conversions discussed here represent the structural changes of nanomaterials in response to the environment and/or external stimuli, including assembly and aggregation, deformation, ion release, disassembly and degradation. For example, metal ions released from metal or metal oxide nanoparticles could be toxic and thus are promising for antibacterial applications.321 Deformation, or disassembly or degradation of nanomaterials can result in the release of encapsulated drug molecules322 or a reduction in their toxic effects.323 In this respect, the ability of nanomaterials to undergo chemical conversion will significantly affect their therapeutic and toxic effects.
6.1. Assembly and aggregation
The aggregation and self-assembly of nanoparticles (NPs) in aqueous solution or upon contact with lipid membranes may influence their therapeutic performance, including cellular uptake and cytotoxicity. To maintain the pre-designed therapeutic functionality of nanodrugs, the aggregation of nanoparticles should be avoided or reversible in response to biological stimuli. Both experimental and theoretical efforts have been extensively devoted to investigating the aggregation or self-assembly behaviour of nanoparticles. Size, charge, morphology, and surface chemistry have been found to play virtual roles in determining the aggregation and self-assembly behaviour of nanoparticles, which can be driven by various molecular forces, such as electrostatic interactions, hydrophobic interactions, van der Waals forces, dipole interactions and steric effects.324,325
6.1.1. Nanoparticle aggregation in water.
In general, small inorganic nanoparticles tend to form aggregates in solution due to their high surface energy. Therefore, surface functionalisation is often necessary to stabilise inorganic nanoparticles. To achieve the controllable nanoparticle aggregation, it is important to well tune ligand properties, such as their type, charge, and surface coverage, to balance the interactions between nanoparticles. For example, even if all AuNPs carry the same charge, they can still form aggregates.326 To understand this phenomenon, a theoretical study was conducted considering model systems of AuNPs (3 nm) coated with thiols of various charges (neutral, cationic, or anionic) and surface coverage. Computational results showed that the neutral thiol-coated AuNPs tended to form aggregates (Fig. 30a) at all coverage densities (20%–100%).327 In contrast, for charged thiol-coated AuNPs, aggregates can be formed at low surface coverage densities (<60%), whereas the AuNPs remained dispersed in the solution at a coverage of over 80%. This behaviour can be attributed to the delicate competition between van der Waals interaction and electrostatic repulsion among charged nanoparticles.
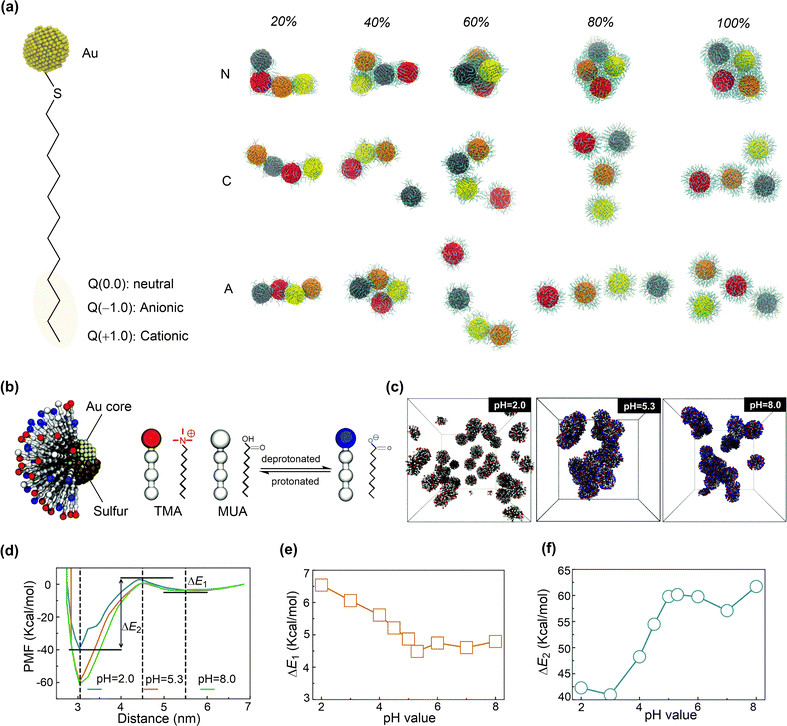 |
| Fig. 30 Simulations of nanoparticle aggregation in water. (a) Thiol-modified Au nanoparticles with different charges and aggregate morphologies of Au NPs at the end of simulations for neutral (N), cationic (C) and anionic (A) thiol coated Au NPs with 20%, 40%, 60%, 80% and 100% surface coverage. Reproduced with permission from ref. 327. Copyright 2018 the Owner Societies. (b) Computational model of N,N,N-trimethyl(11-mercaptoundecyl)ammonium ion (TMA) and 11-mercaptoundecanoic acid (MUA) ligand-modified AuNPs. (c) The equilibrated morphologies of 27 AuNPs at pH 2.0, 5.3 and 8.0. (d) Energy profiles of potential of mean force (PMF) between two AuNPs at pH 2.0, 5.3 and 8.0. (e) The energy barrier (ΔE1) of aggregation of two AuNPs varied with pH value. (f) The energy barrier (ΔE2) of dissociation of two AuNPs varied with pH value. Reproduced with permission from ref. 323. Copyright 2019 the Royal Society of Chemistry. | |
AuNPs functionalised with other ligands showed similar charge-dependent aggregation behaviour. The aggregation of monolayer-protected AuNPs, containing ligands of positively charged N,N,N-trimethyl(11-mercaptoundecyl)ammonium ion (TMA) and 11-mercaptoundecanoic acid (MUA) (pKa = 5.08), is sensitive to pH, which affects the charge states of AuNPs.323 CGMD simulations indicated that the AuNPs had an almost neutral surface and severely aggregated when the pH of the solution is close to 5.3 (Fig. 30b and c). However, AuNPs were found to be stably dispersed in solutions with both low and high pH values, resulting positively and negatively charged AuNPs, respectively. The free energy analysis revealed two energy-consuming processes in the aggregation of AuNPs (Fig. 30d). The first process (3.0 nm position) is driven by the hydrophobic interaction between the alkyl chains, and the second process (5.5 nm position) is driven by the electrostatic interaction between differently charged ligands. The energy changes for these processes depend on the charge states of AuNPs at different pH (Fig. 30e and f), leading to their different aggregation behaviour. Steric effects can also affect the behaviour of grafted nanoparticles,328 which can self-assemble into various structures, including rings, strings, dimers, coil-like aggregates, and disordered-to-ordered aggregates, depending on the graft length, density, and charge. With such knowledge on nanoparticle aggregation, various methods have been proposed to control the morphology of nanoparticle aggregates.329–331
DNA-based assemblies of AuNPs have received significant attention due to their ability to establish supramolecular assemblies, which have great potential for applications in disease diagnosis and theranostics. It was found that the length and rigidity of the grafted DNA play a crucial role in self-assembly of functionalised AuNPs.332 When shorter DNA was grafted onto AuNPs, disordered aggregates were formed, whereas longer DNA resulted in crystalline aggregates.332 In addition to the sequence of DNA, some external stimuli, such as pH, temperature and metal ions, also affect the self-assembly process. CGMD simulations were conducted to investigate how DNA–peptide interactions can be used to modulate the self-assembly and stimulus-response behaviour of AuNPs grafted with single stranded DNA (ssDNA) and poly-L-lysine (PLL) chains.333 The results showed that the self-assembly of AuNPs is driven by electrostatics between grafting chains with positive (PLL) and negative (ssDNA) charges. The morphology of the aggregates depends on the size of AuNPs and the charge of grafting chains, which can be well described by CGMD simulations, despite that many molecular forces such as hydrogen bonding and hydrophobic interactions have been neglected due to limitations of the CG model.334
6.1.2. Nanoparticle aggregation in biological media.
The aggregation behaviour of nanoparticles in biological media is more complex than that in water. In addition to physiochemical properties of nanoparticles and functional ligands, compositions of biological media can also affect nanoparticle aggregation. An investigation on the aggregation behavior of ultrasmall (1.4, 2.0 and 2.5 nm) glutathione-coated AuNPs (AuGSH) in biological media indicated that the size of nanoparticles and components of biological media jointly affect the aggregation of AuGSH.150 Nanoparticles with core diameter of 2–3 nm tended to form aggregates whereas those with smaller size (<1.4 nm) remained the colloidal stability in cell culture mediums. In addition, the aggregation of AuGSH (2.0 nm) rapidly occurred in pure cell culture medium, but was suppressed upon the addition of serum protein (fetal bovine serum, FBS). However, the presence of both FBS and PBS in the medium promoted nanoparticle aggregation. Thus, serum protein and small molecular/salts (likely Ca2+ and Mg2+) contributed to the destabilising and stabilizing forces for AuGSH aggregation, respectively. MD simulations suggested that positively charged ions (Ca2+ and Mg2+) bridged NPs through negative charge (CO2−) on their surface, thus generating attractive forces. Large sized NPs have a large surface area with a higher concentration of surface positive and negative ions, resulting in stronger NP–NP interactions, which is one of the reasons for the size-dependent aggregation behaviour. The ion-bridge can also drive nanoparticle aggregation on cell membranes.333 The unbiased MD simulations demonstrated that a short-range (1.1 nm) ion bridges can be formed between anionic ligands on the surface of two AuNPs and counterions, resulting the formation of NP–NP dimers, with the distance between two Au cores of about 7 nm. When the NPs interact with the membrane via hydrophobic contacts in the fully- or semi-snorkeled configuration, membrane-mediated aggregation forces come into play. Due to the interaction between nanoparticle and membranes, nanoparticle aggregation in the membrane also shows a size dependent behaviour. Amphiphilic AuNPs with a diameter of 2 nm formed transient dimers when embedded in the neuron plasma membrane, causing minimal perturbation of the bilayer structure. However, embedding 4 nm AuNPs resulted in the spontaneous formation of a stable dimer and protrusion from the bilayer was observed.335
The impact of aggregation on the cellular uptake of nanoparticles was investigated using model systems of AuNPs aggregates consisting of five 2 nm spherical AuNPs.198 In the pull simulations of NPs passing through the membrane, the aggregated AuNPs showed a higher free energy barrier (709.07 ± 3.12 kJ mol−1) compared to a single 4 nm nanoparticle (264.05 ± 4.59 kJ mol−1). Therefore, the aggregation of AuNPs is disadvantageous for their cellular uptake, which may also alter the cellular uptake mechanism from direct translocation to semi-endocytosis due to the increased size. It is important to note that the aggregation of NPs could also lead to cytotoxicity in cells. Carbon NPs, such as C60 and C28, can aggregate in water and cell membrane.336 C28 has a higher tendency to accumulate in the cell membrane than C60, which can reduce the thickness of the cell membrane and damage its structure, leading to stronger cytotoxicity than C60. To avoid these potential disadvantages, it is recommended to prevent nanoparticle aggregation, especially in biological media. This necessitates the optimisation of nanoparticle properties that affect the aggregation behaviour introduced above.
6.2. Deformation and disassembly
Deformation and disassembly of nanomaterials usually refer to structural changes in their shape, morphology, and phase. These structural transformations are crucial for nanodrugs, especially when they can occur in response to external stimuli, such as pH, temperature, redox, ion concentration, and ligand–receptor interaction. Stimuli-responsive nanodrugs (also known as smart nanodrugs) can alter their structures to release drug molecules and/or expose their active sites in targeted pathological conditions, which is beneficial for reducing the side effects and improving therapeutic efficacy. Representative smart nanodrugs include polypeptides, polymers and inorganic nanomaterials, etc.337–341
Polypeptides are typically composed of self-assembly motifs, fibrous motifs and targeting motifs. Fibronectin (Fn) is often selected as a receptor protein targeted by polypeptides due to its high expression level in the tumour extracellular matrix. A large number of studies have shown that the formation of nanofiber structures through ligand–receptor interactions is not only conducive to drug accumulation and release, but also has the potential to inhibit tumour metastasis.342 Polypeptides and drugs form non-covalent complex nanoparticles through electrostatic, hydrophobic and hydrogen bonding interactions. Due to the specific ligand–receptor binding, the polypeptide nanoparticles are converted into nanofibers, enabling the release of carried drugs. Three Fn-targeted drug delivery systems were designed using polypeptides possessing different motifs.343 The ligand–receptor interactions, structural changes, and release processes of the drug delivery systems were investigated through MD simulations and experiments (Fig. 31). In the absence of Fn, the peptides aggregated into clusters with the self-assembly motif as the core, due to the strong hydrophobicity of the self-assembly motif and the fibrous motif, as well as the electrostatic repulsion of the targeting motif at the end (Fig. 31a). When the peptide came into contact with Fn, the binding between the terminal targeting motif and Fn due to ligand–receptor interactions, caused the NPs to break down, resulting in the self-assembly motif and fibrous motif flattening into a nanofiber structure to release adsorbed doxorubicin molecules (Fig. 31b–d). However, when the hydrophobicity of the fibrous motif was weak, the corresponding NP could not be transformed into a nanofiber structure. Therefore, a strong hydrophobicity of the fibrous motif is necessary to realise the transformation of the NP into a nanofiber. A core–shell nanocomposites was fabricated with a photo-responsive carbonized dots as the core and a pH and thermally responsive poly(N-isopropylacrylamide) as the shell, serving as carriers of doxorubicin.340 The doxorubicin release from the core–shell nanocomposites was found to be temperature-dependent. The carrier maintained a stable coil structure above a lower critical solution temperature (LCST), but transformed into a collapsed spherical structure below the LCST, releasing doxorubicin. Acidic pH also facilitated the release of doxorubicin. MD calculations showed that the transition from a coil structure to a spheroid was promoted by strong inter-chain attractions and electrostatic repulsions between protonated N atoms of doxorubicin and O atoms of the polymer in an acidic environment (Fig. 31e). The pH-responsive deformation or disassembly of nanoparticles usually arises from the different protonation states of functional moieties, which alter inter-/intra-particle interactions. For example, the poly (lactic-co-glycolic acid)/hydroxypropylmethylcellulose phthalate (PLGA/HP55) nanoparticle is pH-sensitive.337,344 The polymer can self-assemble into a stable core–shell spherical structure with hydrophobic PLGA as the core and hydrophilic PVA as the shell. The pH-sensitive HP55 is distributed between the core and the shell with the therapeutic insulin loaded into the core. Below pH 5.5, the HP55 remains hydrophobic due to the non-ionization of its carboxyl group, maintaining the spherical core–shell structure. PVA then encapsulates the outer surface of PLGA and HP55, facilitating insulin loading (Fig. 31f). Above pH 5.5, the carboxyl group of HP55 becomes ionized, changing its hydrophobic nature to hydrophilic, allowing HP55 to move outward and creating favourable conditions for insulin release.
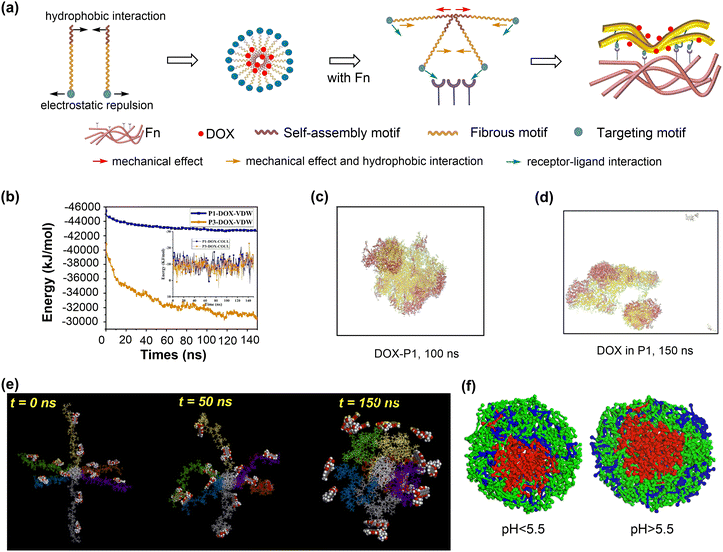 |
| Fig. 31 Simulations of nanoparticle deformation and disassembly. (a) Detailed process of deformation from NPs to nanofibers and schematic of interaction between polypeptides. (b) vdW and coulombic potential energies between polypeptides (P1 and P3) and doxorubicin (DOX). (c) Snapshots of peptide-loaded DOX of P1 at 100 ns. (d) Snapshots of drug-release processes of P1 at 150 ns, Fn was not displayed. Reproduced with permission from ref. 343. Copyright 2022 Elsevier. (e) Snapshots of CPD6 under acid condition at 310 K taken at 0 ns, 50 ns and 150 ns. Reproduced with permission from ref. 340. Copyright 2022 Elsevier. (f) Section views of polymeric NPs with different pH value. Reproduced with permission from ref. 337. Copyright 2017 Elsevier. | |
Nanoparticles with redox-responsive structural moieties can undergo deformation or disassembly. For example, a metallohydrogel, formed by the self-assembly of Cu2+ and peptide, showed redox-responsive properties. MD simulation showed that Cu2+ formed an intermolecular bridging coordination between two peptide molecules, endowing the metallohydrogel with unique characteristics, such as redox-responsiveness, antimicrobial activity, self-healing, and cytocompatibility. The addition of Cu2+ also induced the formation of nanofiber structures within the metallohydrogel. This material can serve as a wet wound dressing to promote wound healing without the need for debridement, thereby reducing the trauma and pain typically associated with traditional gauze treatments.345,346
6.3. Ion release
The release of metal ions from nanoparticles has numerous applications, including killing bacteria,347–350 treating tumuors,351–354 and promoting bone growth.355 For example, AgNPs can kill S. aureus, E. coli, fungi, and viruses by releasing Ag+ or producing ROS under photocatalysis (Fig. 32a).348,349 Ag+ can penetrate bacteria cells, bind to the –SH of proteins, and inactivate them. In addition, electrons produced upon exposure to visible light can be captured by O2 to form 1O2, which damages bacteria cell membranes. DFT calculations were conducted to investigate the ion release capabilities of AgNPs with different metal doping, which affects their antibacterial and wound healing properties.349
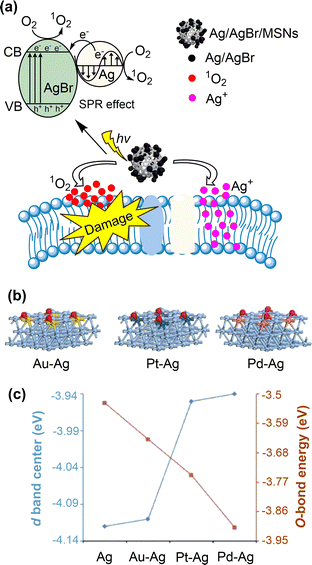 |
| Fig. 32 Simulations of ion release from nanoparticles. (a) Schematic illustration of bacteria-killing through the photodynamic effects of Ag/AgBr/MSNS under visible light and the innate antimicrobial ability of Ag+. Reproduced with permission from ref. 348. Copyright 2018 the Royal Society of Chemistry. (b) The oxygen-adsorbed slab structures of alloy Ag NPs. (c) Energy (in eV) profiles of D-band centre and Ag–O bond formation of pure and alloy Ag NPs. Reproduced with permission from ref. 349. Copyright 2019 American Chemical Society. | |
The Au–Ag, Pt–Ag, and Pd–Ag alloys exhibited antibacterial performances proportional to the upshift of the d-band centre. The calculated d-band centre energy values are −4.12, −4.11, −3.95, and −3.94 eV for Ag, Au–Ag, Pt–Ag, and Pd–Ag nanoparticles, respectively (Fig. 32b and c). Among these, Pd–Ag nanoparticles showed the strongest O-bond energy, indicating the highest electron-donating and Ag+ releasing ability. Therefore, Pd–Ag nanoparticles exhibited the best antibacterial and wound healing performance. These results demonstrate that the d-band centre is a valuable descriptor in the design of Ag-based alloys for antibacterial applications.
Ions released from nanoparticles can interact with intracellular H2O2 to produce highly toxic ˙OH via Fenton and Fenton-like reactions. For example, the Bi2S3–MnO2@BSA NPs was prepared for synergistic chemodynamic and photothermal therapy (CDT/PTT) of tumours.351 Upon entering tumour cells, MnO2 decomposes rapidly to release Mn2+ under the high GSH and H2O2 concentrations. Mn2+ further catalyses the decomposition of H2O2 to produce ˙OH via Fenton-like reactions, a process that is further enhanced by Bi2S3@BSA-induced local hyperthermia for synergistic tumour therapy.353,354 The release of Cu+ from NPs can similarly catalyse the production of ˙OH for CDT. Likewise, the release of Zn2+ from metallo-nanoassemblies can facilitate gene theranostics by supplementing DNAzyme cofactors, enhancing the catalytic efficacy of DNAzymes.352 It is noteworthy that the ion release in these cases occurs under acidic conditions, suggesting their potential safety under physiological pH.
In addition to killing bacteria and/or tumour cells, released ions can also cause the undesired death of normal cells, a phenomenon known as cytotoxicity. The quantitative structure–activity relationship (QSAR) has been used to predict the cytotoxicity of NPs induced by metal ions released from nanoparticles.321,356 The ion release ability of metal oxides was evaluated using the enthalpy of formation (ΔHMe+) of a gaseous cation with the same oxidation state as that in the nanoparticle.321 This ΔHMe+ value was then used to predict the cytotoxicity of metal oxides to E. coli according to eqn (3):
| log(1/EC50) = 2.59 − 0.50·ΔHMe+ (F = 45.4, P = 0.0001) | (3) |
where EC
50 is the effective concentration of a compound that results in a 50% reduction in cell viability. It is important to note that while this model is adoptable to predict the cytotoxicity from metal cations release, it omits other factors such as particle size and disruption of membranes or cellular substances. Nanodescriptors were proposed to analyse the impact of doping on the release of cancer-killing metal ions from metal oxides:
357 | 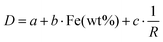 | (4) |
where
D represents the quantity related to the properties of the NP, such as lattice energy descriptors, or the average coordination number of all atoms descriptors;
a,
b and
c are equation coefficients; Fe(wt%) is the doping level (weight%) and
R is the radius of the NPs in angstroms. Although a statistically significant linear relationship was found between the experimental and computational data, it is important to note that the limited dataset may not fully reflect numerous statistical correlations.
6.4. Degradation
When considering the medical applications of nanoparticles, their biocompatibility and degradability are crucial to prevent any potential side effects on the organism. Nanoparticles typically degrade under chemical and/or biological stimuli, which can generate reactive oxygen species.358,359 For example, enzymes such as the peroxidase and lipase can catalyse the conversion of H2O2 to ˙OH, facilitating nanoparticle degradation. Nanoparticles can also undergo self-promoted degradation due to their inherent peroxidase-like catalytic activity. Theoretical and experimental studies have been conducted on the degradation of nanoparticles.
Nanoparticles such as graphene, GQDs, CNTs, and CDs, can be degraded into fragments or eventually into CO, CO2, and H2O molecules when exposed to H2O2 and neutrophil-secreted human myeloperoxidase (MPO) or eosinophil-secreted peroxidase (EPO).360,361 The interaction between nanoparticles and enzymes is a key step in degradation due to the high catalytic activity of enzymes in generating ˙OH and the non-specific cleavage ability of these radicals. The interactions between these enzymes and GQDs were investigated through MD simulations.362 Both peroxidases and GQDs undergo slight conformational changes to enhance their interaction, ensuring that the catalytic activity of peroxidases is maintained. In addition, GQDs interact with charged and aromatic amino acids through electrostatic, cation–π, and π–π stacking interactions (Fig. 33a). The strong peroxidase–GQD interaction likely accelerates the degradation of GQDs by locally generated ˙OH. Similar mechanisms are anticipated for enzymatic degradation of other carbon-based nanoparticles.
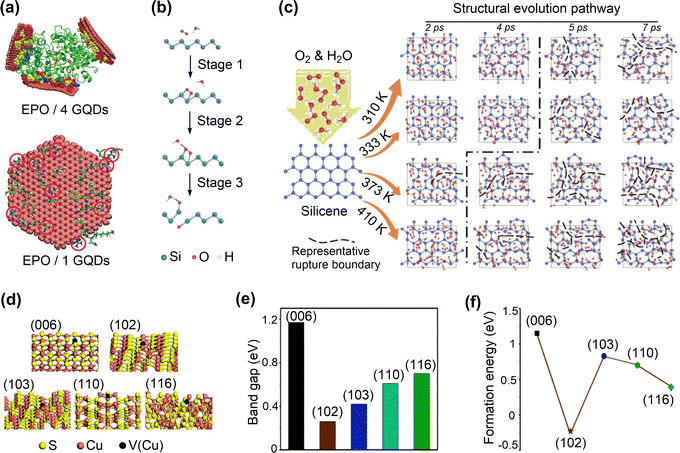 |
| Fig. 33 Simulations of nanoparticle degradation. (a) MD simulations of EPO interacting with the GQDs (van der Waals representation). Reproduced with permission from ref. 361. Copyright 2019 Wiley-VCH. (b) Oxidization and disintegration process of SNSS degradation. (c) Atomic structures of photothermally accelerated biodegradation behaviour of SNSS in the aqueous environment (H2O and O2) at different temperatures. Reproduced with permission from ref. 364. Copyright 2019 Wiley-VCH. (d) Atomic structure of different CuS surfaces. (e) and (f) Band gap and S vacancy formation energy of CuS nanocrystals. Reproduced with permission from ref. 363. Copyright 2019 the Royal Society of Chemistry. | |
A biocompatible and degradable two-dimensional silicene nanosheet (SNS) was synthesised for use in photo-triggered therapeutics and diagnostic imaging. The degradation mechanism of the SNS was studied using a combination of DFT and MD methods.364 The main degradation process of SNS was the adsorption and rearrangement reaction of O2 and H2O on the SNS surface (Fig. 33b), leading to the formation of OOH*, OH* and O*. These reactive species caused the cleavage of Si–Si bonds and the formation of Si–O bonds. The degradation behaviour under physiological conditions (pH 7.4) was found to be temperature dependent. Specifically, the SNS transformed from porous structures to nanoscale fragments as the temperature rose from 310 K to 333 K. At 363 K, the SNS was completely degraded into amorphous structures (Fig. 33c). CuS nanocrystals can degrade under acidic conditions (pH 6.0) or 808 nm laser irradiation.363 To investigate the degradation mechanism of CuS nanocrystals, DFT calculations were conducted to determine the band gaps, density of states, and the S and Cu vacancy formation energies of the main surfaces of CuS nanocrystals (Fig. 33d–f). The results indicated that the degradation of CuS nanocrystals mainly occurred mainly at the (102) surface, which has the smallest band gap, indicating superior electron transfer capacity and high photothermal conversion efficiency. Furthermore, only the S-vacancy formation energy of the CuS (102) facet was negative, suggesting a propensity for S atom release and subsequent Cu+ release, driving the degradation process.
6.5. Summary
In conclusion, the capacity of nanomaterials for chemical conversion is a pivotal factor in their application as nanodrugs, enabling structural transformations in response to environmental stimuli. These transformations include assembly, aggregation, deformation, ion release, disassembly, and degradation, which can be tailored for various medical interventions. For example, the aggregation of nanoparticles such as AuNPs can be controlled through surface functionalisation, which in turns affects cellular uptake and cytotoxicity. The pH sensitivity of AuNPs functionalised with ligands such as TMA and MUA can affect their dispersion and aggregation. Furthermore, the release of metal ions from nanoparticles has been shown to have potential applications in antibacterial and antitumour therapies. However, the possible cytotoxicity of these ions necessitates a careful balance to prevent damage to healthy cells. It is also crucial to consider the degradation of nanoparticles, which ensures the biocompatibility and minimises the potential side effects. Carbon-based nanoparticles and silicene nanosheets (SNSs) can be engineered to degrade under specific conditions, such as temperature and pH, thus facilitating their clearance from the body post-therapy. Theoretical simulations and experimental studies are essential for optimising these properties, which will facilitate the development of nanomaterials with promising therapeutic potential in medicine. It is recommended that future research focuses on optimising these properties for controlled drug delivery, enhancing therapeutic outcomes, and ensuring the safe degradation of nanomaterials within the biological environments.
7. Machine learning-assisted nanodrug discovery
The most significant challenge in the field of nanodrug discovery is the precise selection of the most appropriate nanomaterials for a specific medical application. Given the massive number of available nanomaterials, which is continuing to increase, conventional computer simulations and trial-and-error experiments are unable to fully address this challenge. Machine learning techniques have demonstrated great potential in nanodrug discovery, using datasets from the reported literature, high-throughput experiment or computation (Fig. 34). For example, it can assist in the identification of the composition and structure of protein coronas on nanomaterials,25,365 as well as in the prediction of highly bioactive catalytic nanomaterials,366–368 based on reported experimental results where the underlying molecular mechanisms remain unclear. With a sufficient understanding of the structure–activity relationship of nanodrugs, machine learning can be employed to accelerate the discovery of nanodrugs26,27,369,370 from publicly available databases of nanomaterials.
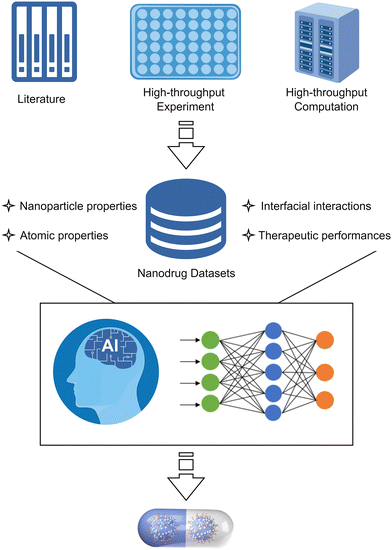 |
| Fig. 34 Schematic representation of machine learning (ML) techniques to accelerate nanodrug discovery. Literature, high-throughput experimental or computational datasets can be used to train ML models that link the atomic and/or physicochemical properties of nanoparticles to their interfacial interactions with biological entities or therapeutic performance. Drawn by figdraw (https://www.figdraw.com/#/). | |
7.1. Machine learning in surface adsorption-based nanodrugs
7.1.1. Adsorption of small molecule drugs.
The binding affinity of small molecule drugs to nanosurfaces can be well evaluated by either DFT calculations or MD simulations, as introduced in Section 3.1, which play important roles in understanding the mechanisms for nanodrug formulations. However, these computational methods are insufficient in finding appropriate drug–nanoparticle combinations in terms of time and cost. Integrating these computational methods with machine learning approaches as well as experimentation has been applied in accelerating nanodrug discovery to address issues of drug loading and drug release in surface adsorption-based nanodrugs.
It is well-known that drug loading significantly depends on the binding energies between drug molecules and nanosurfaces; the more negative binding energy, the higher drug loading efficiency. A linear or exponential relationship between reported drug loading efficiency and computed drug–nanoparticle binding energy has been uncovered for solid lipid and PLGA nanoparticles.371 These relationships enable application of the artificial neural networks in the prediction of the drug–nanoparticle binding energy and hence drug loading, based on molecular descriptors of drugs, including molecular weight, x
log
P, topological polar surface area, and fragment complexity. Because drug loading amount may also be influenced by the surface area, machine learning methods have been applied to predict the solvent accessible surface areas (SASA) of nanoparticles from short MD simulations. This integrated machine learning and molecular simulation technique can significantly reduce the computational expense for calculating SASA.372
The co-assembly of drugs and dyes (excipients) into solid nanoparticles is a promising solution to the challenges of low drug loading and solubility in nanodrug formations. An integrated framework, consisting of molecular simulation, machine learning, and high-throughput experimentation, was established to identify optimal drug–excipient combinations with desired properties.373 A random forest machine learning model trained on the datasets consisting of the co-aggregation patterns of 1440 possible drug–excipient combinations, successfully captured the co-aggregation relationships and prioritize suitable excipients for new drugs. The predicted results were further verified via experimental tests, showing that the monodisperse, nano-sized co-aggregates of drugs and excipients can be formed. In contrast, drugs without excipients self-aggregate into micrometre-sized, polydisperse structures.
Drug release is another issue to be considered in nanodrug formulations. Long-acting injectables are promising to achieve sustained or controlled release of adsorbed drugs on nanosurfaces, which is essential for the treatment of chronic diseases. Recently, machine learning methods were employed to accelerate the design of new polymeric LAIs, based on an experimental dataset.374 Among the tested ML models, the light gradient boosting machine (LGBM) showed the highest overall prediction accuracy and small mean absolute errors. Time and fractional drug release at 24 h (T = 1.0) are the most important features influencing the fractional drug release predictions. However, the drug release values at T = 1.0 are unknown prior to the design of new LAI systems. Further analysis indicated that the drug release values at T = 1.0 are closely associated with the physiochemical properties of drugs, polymers, and LAIs. By tuning these properties, two LAIs were fabricated with “fast” and “slow” drug release profiles, respectively, which were subsequently verified in experiments. These results demonstrate that integrating machine learning and high-throughput experimentation/computation can accelerate the development of safer and more efficacious nanodrug formulations in terms of drug loading and sustained release.
7.1.2. Adsorption of proteins.
Molecular simulations of protein adsorption are usually time-consuming and thus difficult to predict the binding affinities of various proteins on different nanosurfaces. Recently, machine learning methods have been applied to investigate protein adsorption, in particular protein corona formation,375,376 on nanosurfaces based on molecular simulation or experimental data. For instance, a machine learning model based on the random forest regression algorithm was trained to predict the amount of adsorbed serum protein on polymer brush films (Fig. 35a).377 Taken the physicochemical properties of polymers and structural characteristics of monomers as descriptors, the trained RF model can predict protein adsorption with high R2 (0.78) and low RMSE (0.09) values (Fig. 35a). Specially, it can distinguish polymers with protein-resistant or protein-adsorbing properties. The hydrophobicity of the monomer is the most critical descriptor, followed by the number of C–H bonds and the net charge of monomers, as well as the thickness and density of polymers. These parameters have similar impacts on protein adsorption onto self-assembly monolayers in an artificial neural network model.378
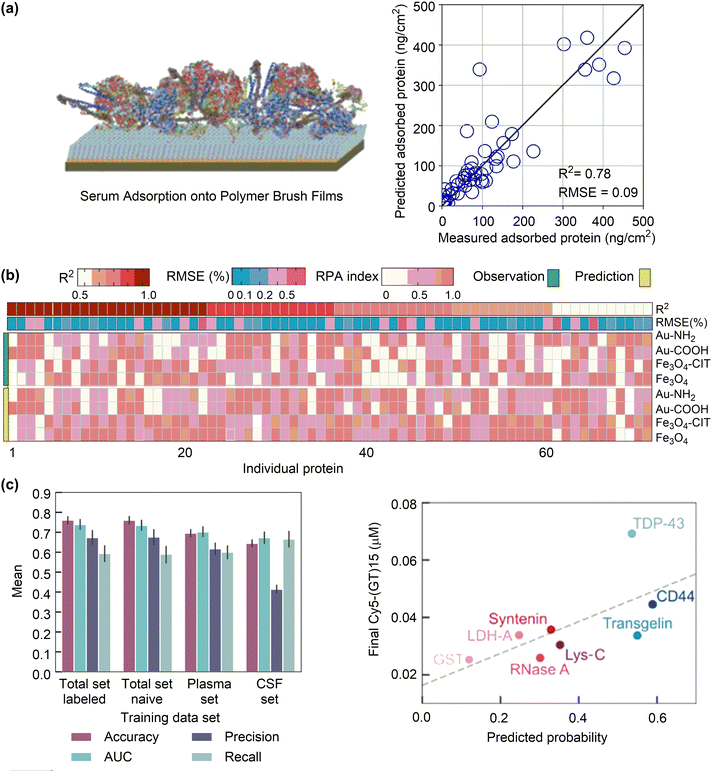 |
| Fig. 35 Performances of machine learning models for the prediction of protein corona adsorbed on nanosurfaces. (a) Predicted amounts of serum protein on polymer brush films with the trained random forest algorithm against the experimental values. Reproduced with permission from ref. 377. Copyright 2022 American Chemical Society. (b) Correlation coefficients (r2) and root mean square error (RMSE) and normalized index of the predicted and observed relative protein abundance (RPA) for 71 individuals adsorbed on selected NPs (Fe3O4, Fe3O4-Cit, Au–COOH, and Au–NH2). Reproduced with permission from 25. Copyright 2020 CC BY-NC-nd 4.0. (c) Random forest classifier trained on the full protein set or each individual biofluid (plasma or CSF) and end-state-desorbed ssDNA compared to the RFC predicted in-corona probability for (GT)15-SWCNTs. Reproduced with permission from ref. 365. Copyright 2022 CCBY 4.0. | |
In order to address the biological effects of nanoparticles due to the formation of protein corona, it is essential to identify not only the amount but also the composition of proteins adsorbed on nanosurfaces. The robust random forest (RF) models was trained based on datasets constructed from published papers, to predict the composition of protein corona, taking the experimentally measured relative protein abundance (RPA) on nanosurfaces as the endpoints.25 High prediction performances for the grouped proteins with similar physicochemical or functional properties have been achieved. The trained models also have high accuracy in predicting the RPA values of individual proteins, mostly with R2 values over 0.7 and RMSE values below 5% (Fig. 35b). These high accuracies, validated by subsequent experiments showing comparable predicted and observed RPA values, are crucial for predicting the composition of protein corona and designing nanodrugs that adsorb specific proteins to enhance the targeting ability and mediate biological recognition.
Despite these successes, the model can only make predictions for proteins and nanoparticles within the datasets, potentially limiting its broader applications. To this end, a workflow was established which is able to experimentally quantify the protein corona composition and train a random forest classifier (RFC) based only on protein sequence to predict protein adsorption on nanoparticles.365 The obtained RFC can precisely target the small number of proteins adsorbed on nanoparticles and identify the most important protein properties leading to nanoparticle binding. The developed RFC can identify adsorbed proteins with 78% accuracy and 70% precision (Fig. 35c). Because this workflow is generalisable to other nanoparticles, it is promising to include more nanoparticles with different properties. It is anticipated that the applications of this workflow will facilitate the development of a more general classifier available to predict protein adsorption on nanoparticles based on protein sequence and nanoparticle features.
Machine learning has also been integrated with conventional MD simulations to accelerate the simulation or analysis of the complex behaviour of protein adsorption on nanosurfaces. Discontinuous molecular dynamics (DMD) simulations and graph clustering analyses were employed to investigate the adsorption behaviour of lysozyme on a graphene surface.379 An autoencoder-based graph clustering model was used to segment the protein adsorption process, successfully revealing the structural evolution of lysozyme during adsorption. A similar approach was employed to examine the adsorption of ubiquitin on a graphene surface.380 The protein contact map feature extractor was trained first through contrastive learning to generate feature vectors, which can then be clustered using the k-means algorithm to identify stages of protein structural transitions during adsorption. This analysis reveals that hydrophobic interactions and π–π stacking play a pivotal role crucial to the adsorption process of proteins.
Despite the aforementioned progress, it remains challenging to fully characterise the interactions between proteins and nanosurfaces. To address this, a machine learning-based computational framework has recently been proposed for predicting how proteins interact with nanoparticles.381 A set of geometrical and graph-theoretical descriptors were developed to characterise both proteins and nanoparticles. Based on a database of protein complexes with various calculated descriptors, machine learning models were trained to predict the protein–protein and protein–nanoparticle interaction sites. In particular, the trained models can reliably predict the relative protein abundance in the protein corona around carbon nanomaterials. Although at an early stage, this work demonstrate the power of machine learning in the prediction of protein–nanosurface interactions without the need for significant computational and/or experimental efforts, by considering the three-dimensional geometry and topological features of proteins and nanoparticles.
7.1.3. Adsorption of ligands.
The functionality of ligand-engineered nanoparticles depends predominately on the binding specificity and affinity between ligands and nanosurfaces. Machine learning has recently been applied to accelerate the discovery of specific ligand–nanoparticle combinations with appropriate properties. For instance, peptides have been widely employed in the functionalisation of nanoparticles. The binding affinity of peptides to nanoparticles significantly depends on their sequences. A Bayesian Effective Search for Optimal Sequences (BESOS) approach was developed to identify peptide sequences that selectively bind to specific nanoparticles.382 This approach basically leverages three key types of information, including experimentally determined adsorption free energies of peptides on nanoparticles, simulated adsorption free energies of 20 natural amino acids on nanoparticles, and motif descriptors based on properties of amino acids such as hydrophobicity, charge, size, and mass. Using BESOS, two unknown peptide sequences were suggested that would selectively bind to Au and Ag nanoparticles, respectively. The predictive power of these motif descriptors has been validated by comparison with replica exchange with solute tempering molecular dynamics simulations. The identification of effective descriptors is crucial in this type of approach when the datasets are limited.
Alternatively, machine learning can be employed to predict nanoparticle–peptide binding when a large dataset is available. The development in high-throughput experiments has facilitated the establishment of a library of gold-binding peptides.383 Based on this library, unsupervised learning methods, such as k-means clustering and hierarchical clustering, were employed to group peptides into two clusters, which showed distinct distributions of binding intensity, isoelectric point, molecular weight, and hydrophobicity.384 Notably, the isoelectric point and hydrophobicity of peptides play a pivotal role in regulating the peptide–nanoparticle binding, whereas the molecular weight is less influential. These results demonstrate that machine learning can facilitate the rational selection and design of peptides for engineering functional nanoparticles. However, it is noteworthy that, these machine learning models are mainly developed for clustering of nanoparticle-binding peptides. Further developments are required to predict the peptide–nanoparticle binding both qualitatively (strong or weak) and quantitatively (the value of binding affinity) using sequence-based descriptors.
7.2. Machine learning in supramolecular interaction-based nanodrugs
Conventional molecular simulations of supramolecular interaction-based nanodrugs have been mainly applied to investigate the underlying mechanisms. However, the precise design of such nanodrugs, guided by mechanistic insights, remains a significant challenge. Machine learning methods, mostly based on experimental data, have thus been employed to predict the efficacy of nanodrugs associated with supramolecular interactions, such as cell–nanoparticle interactions. Appropriate cell–nanoparticle interactions are essential to ensure the effective cellular uptake and low cytotoxicity of nanodrugs. To this end, a machine learning-based graph modelling and correlation-establishing approach was proposed to quantify interactions between cells and nanoparticles, according to variations in the cell shape index (CSI) and nuclear area factors (NAFs) of cells upon interaction with nanoparticles.385 Likewise, a random forest regression model was trained to screen polymeric nanodrugs for gene delivery, taking the transfection (cellular uptake) and viability (cytotoxicity) as the endpoints.386
To identify the characteristic cellular uptake responses of nanoparticles for individual cell types, an artificial neutral network (ANN) model was trained based on a dataset consisting of various functionalised carbon nanoparticles (CNPs) and their cellular internalisation patterns in different cell lines (normal and breast cancer).387 The model can precisely predict the cellular internalisation of CNPs with a high Q2 value (0.9) and a small mean-squared error (3.3284 × 10−4), enabling the identification of the different internalisation characteristics of CNPs within various targeted cells. Subsequently, an ANN model was trained to distinguish between normal and cancerous cells, as well as to identify the stages and underlying subtypes of cancer cells, with an overall accuracy of 98.1% (Fig. 36a). Similarly, machine learning models were trained for various cell lines to predict the active cell recognition of nanoparticles via ligand–receptor supramolecular interactions, using a dataset consisting of gold nanoparticles functionalised with organic ligands and folic acids.388 The models obtained have an R2 value of over 0.7 and a standard error estimation of less than 2 × 10−11 g Au cell−1. These machine learning models show that the supramolecular cell–nanoparticle interactions can be rationally tuned by simultaneously optimising the physicochemical properties of nanoparticles to achieve efficient passive and/or active cellular uptake of nanodrugs.
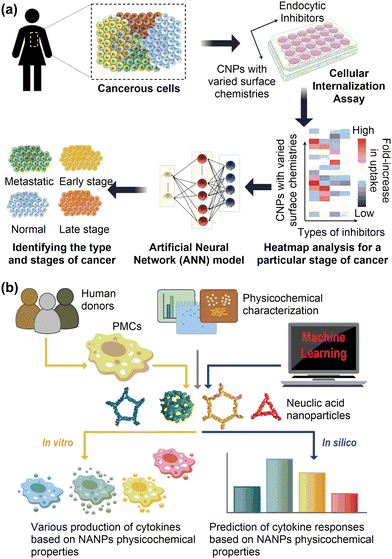 |
| Fig. 36 Machine learning models for the supramolecular interaction-based nanodrugs. (a) Machine learning assisted diagnosis of breast cancer with carbon nanoparticles-based sensor array. Reproduced with permission from ref. 387. Copyright 2020 American Chemical Society. (b) Workflow for the development of artificial immune cell. Reproduced with permission from ref. 389. Copyright 2022 CC BY 4.0. | |
Machine learning methods have also been applied to predict the supramolecular interaction-based antigen recognition. To address the complex structure-activity relationship and design rules for spherical nucleic acids (SNAs), high-throughput experiments were conducted, resulting in datasets consisting of approximately 1000 candidate SNAs with 11 design parameters and their performances in regulating the immune response.390 Although all design parameters can influence the immune activation, simply increasing or decreasing a specific parameter does not necessarily result in enhanced performance. Therefore, machine learning models were constructed, with the XGBoost demonstrating the best performance, as evidenced by the highest Q2 value of 0.83. Moreover, the structure–activity relationships for a large number of compounds (tens of thousands) can be predicted with a relatively small dataset (order of thousands), thereby significantly accelerating the design process. However, the developed models focus on one key biochemical step among many involved in immune responses, which may hinder their ability to predict the efficacy of immunotherapeutic agents. Alternatively, the “artificial immune cell” (“AI-cell”) has recently been developed to predict the immunological responses of nucleic acid nanoparticles (NANPs).389 This has enabled the establishment of NANPs structure–activity relationships and design principles (Fig. 36b). The physicochemical properties of NANPs can be simultaneously optimised, which is crucial for the development of personalised immunotherapy.
7.3. Machine learning in catalysis-based nanodrugs
The efficacy of catalysis-based nanodrugs is significantly dependent on their catalytic activities. These catalysis-based nanodrugs hold great potential in various medical applications, such as anti-cancer and antibacterial treatments. However, the discovery of catalysis-based nanodrugs is still based on the trial-and-error method. Thanks to the experimental and computational efforts in this field, machine learning has proven to be an effective tool in accelerating the discovery and design of catalysis-based nanodrugs, driven by either experimental data or physical model.22,24
Experimental data-driven machine learning models have been developed to describe the type and level of enzyme-mimicking activity of nanomaterials. An easily expandable and open-access database (https://dizyme.net) was constructed from experimental data. The database contains over 300 existing inorganic nanomaterials with enzyme-mimicking activities.367 Prior to building an ML model, 14 essential parameters were identified through feature selection, which describe the elemental composition, material physicochemical properties, shape, size, as well as the synthesis and analysis conditions. These parameters can be used to predict the catalytic activity. The random forest regression (RFR) showed the best performance among the tested ML models, exhibiting small mean absolute sample deviations from the actual values of log
Km and log
kcat. The predictive accuracy was experimentally confirmed using test samples, such as uncoated Fe3O4 and ZnFe2O4 oxides, as well as amorphous and crystalline bimetal FeCo nanomaterials. It should be noted that the current models are built on a small dataset, necessitating an expansion to incorporate additional descriptors and data points to improve the model learning ability.366
A more comprehensive database was constructed via data collection from over 300 papers, enabling the application of fully-connected deep neural network (DNN) to uncover the hidden relationship between nanomaterials features and their enzyme-mimicking activity.368 Subsequently, 14 DNN-based models were established to predict the type (classification model) and the level of enzyme-mimicking activity (quantitative model). The classification model has an accuracy of 90.6% (Fig. 37a), demonstrating its feasibility to predict enzyme-mimicking types of nanomaterials. The quantitative models exhibit reliable prediction ability for POD- and OXD-mimicking activity (R2 = 0.66 and 0.80, respectively) (Fig. 37a). Given their high accuracy, the quantitative models for predicting POD- and OXD-mimicking activity were employed to design enzyme-mimicking nanomaterials. Specifically, the predicted trend for POD-like activity of Ru, Rh, Pd and Ag nanomaterials and that for OXD-like activity of MnO2, Fe3O4, Co(OH)2, and CuO nanomaterials agree well with experimental observations.
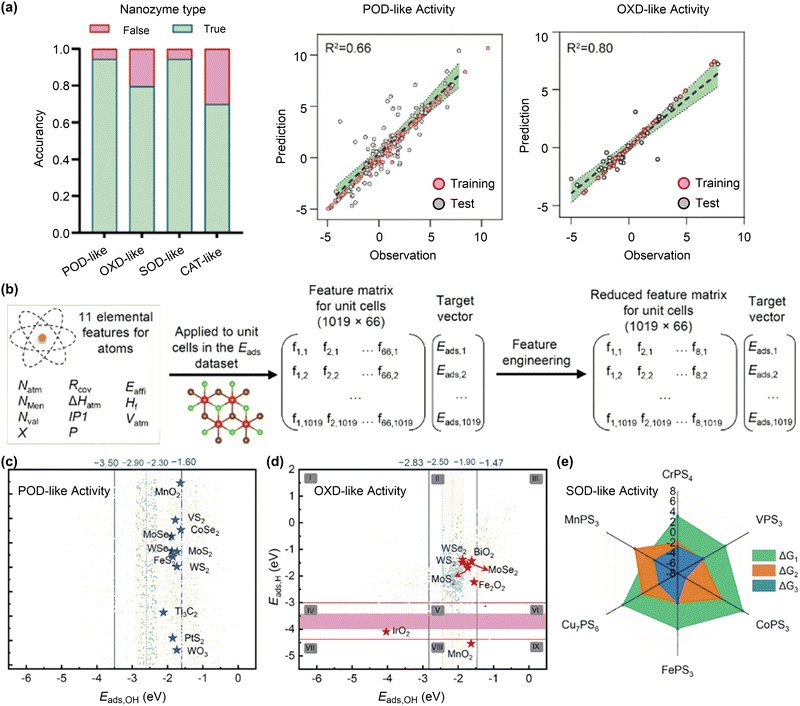 |
| Fig. 37 Machine learning models for the surface catalysis-based nanodrugs. (a) Experimental data-driven machine learning models for predictions of nanozyme type and activity. Reproduced with permission from ref. 368. Copyright 2022 Wiley-VCH. (b) Physical model-driven machine learning models for predictions of enzyme-like activity of nanomaterials. (c)–(e) Machine learning predicted nanomaterials with POD-like, OXD-like and SOD-like activities. Reproduced with permission from ref. 26 copyright 2023 Wiley-VCH and from ref. 370 Copyright 2022 American Chemical Society. | |
Although these experimental data-driven machine learning models can provide reasonably accurate predictions of enzyme-mimicking activity, the limited quality, quantity, and diversity of the dataset significantly hinder the prediction ability of ML models.368 Furthermore, it should be noted that the present models have been mainly developed for metal-containing nanomaterials, making them less effective for non-metallic nanomaterials such as carbon. This, in turn, necessitates the establishment of a standardised procedure for the characterisation of the physicochemical properties of nanomaterials and the catalytic kinetics associated with various forms of enzyme-mimicking activity. However, this is only applicable to POD-mimicking nanomaterials.391 Alternatively, the physical model-driven machine learning models, which possess universal descriptors, could be valuable supplements to these experimental data-driven models.
In physical model-driven machine learning methods, the prediction endpoints are not experimentally measurable kinetic parameters, but computable physical descriptors of the enzyme-mimicking activity, as introduced in Section 5.3. The datasets employed to train machine learning models are usually obtained through high-throughput DFT calculations utilising publicly available materials libraries. Recently, machine learning models have been developed for efficient in silico screening of 2D materials for tumour catalytic therapy based on the computational two-dimensional materials database.26 The peroxidase- and catalase-mimicking activities of nanomaterials were selected as target catalytic properties, which can be described by adsorption energies of hydroxyl group and hydrogen atom (Eads,OH and Eads,H) on nanosurfaces (Fig. 37b). The extreme gradient boosting regression (XGBR) algorithm was trained on the datasets derived from high-throughput DFT calculations to predict the Eads,OH and Eads,H values. This method takes advantages of the interpretability of the physical model and the efficiency of machine learning. It has identified 195 and 411 nanomaterials, which display peroxidase- and catalase-mimicking activities respectively, as potential candidates for tumour catalytic therapy (Fig. 37c and d). Similar machine learning models have been developed to predict the peroxidase-mimicking of doped graphdiyne nanomaterials.369 Such a physical model-driven machine learning method has recently been applied to assist the discovery of nanodrugs for androgenetic alopecia. A random forest (RF) model was trained using elementary and structural properties as descriptors to predict the superoxide dismutase-mimicking activity of transition-metal thiophosphates. The highly active MnPS3 (Fig. 37c), which was predicted to have a high SOD-like activity for scavenging O2˙− demonstrated effectiveness for the treatment of androgenetic alopecia in mouse models.370
7.4. Machine learning in conversion-based nanodrugs
The chemical conversion of nanomaterials in real conditions is a highly complex process to simulate, due to the computational limitations and the lack of reliable force fields. Several machine learning models have been applied to understand the structural transformation of nanomaterials and their associated medical applications. A hybrid model combining mechanistic modelling and machine learning methods was developed to predict drug release from levonorgestrel-loaded biodegradable microparticles for injectable contraception.392 Likewise, an ensemble ML model was trained to predict the dissolution behaviour of oxide glasses. The model combining random forests and additive regression, which was trained on a comprehensive database of approximately 1300 data records from original glass dissolution experiments, showed the best performance. This model can well predict the chemical degradation of bioactive glasses across a wide pH range.393
Computational data-based machine learning potentials have been trained to more efficiently understand the aggregation or degradation of nanomaterials. A deep learning framework was developed to predict the potential of mean force between polymer-grafted nanoparticles, which is crucial for understanding their self-assembly and distribution in polymer matrices. CGMD simulations were employed to generate the training data for a deep neural network. The resulting DNN can accurately predict the interaction energies, thereby allowing for the simulation of nanoparticle self-assembly into various structures. The predicted self-assembled structures match well with the actual structures formed by single-chain polymer-grafted spherical nanoparticles.394 To study the degradation of MXenes in aqueous conditions, a neural network potential (NNP) was developed. The NNP-based MD simulations revealed that the degradation of MXenes in aqueous systems was an energetically spontaneous process, driven by reaction with water molecules. However, the oxidation rate of MXenes decreased exponentially over time due to the inhibitory effects of free protons and oxides formed during the process.395 Such phenomena are difficult to capture by conventional computational methods. It is anticipated that the machine learning potentials can be applied to investigate the complicated behaviours of nanodrugs in physiological conditions, such as pH/redox-sensitive degradation.396,397
7.5. Machine learning in nanodrug pharmacokinetics
The pharmacokinetics of nanodrugs, namely the absorption, distribution, metabolism, and excretion (ADME) of nanodrugs within the body, is of great importance for achieving their therapeutic efficacy. Although the interfacial interactions between nanoparticles and biological entities can influence the pharmacokinetics of nanodrugs, there is currently no straightforward method for making reliable predictions due to the inherent complexity of these interfacial interactions and the variability of biomedical responses. Typically, the pharmacokinetics profile of a drug is obtained through laborious and time-consuming analysis. With the accumulation of such knowledge, machine learning techniques offer significant potential for the rapid and precise prediction of the pharmacokinetic behaviours of nanodrugs within the body, taking into account the biology and physiopathology details of each disease and/or patient.398
To predict the pharmacokinetics of dendrimers, a machine learning-based web tool, dendPoint, was developed, based on a manually constructed database from over 600 papers.399 DendPoint is capable of accurately predicting pharmacokinetics parameters, including half-life, clearance, distribution volume, and dose recovered in the liver and urine, through various dendrimer characteristics, such as scaffold composition, size, PEGylation, surface functionality, and drug conjugation. This tool provides a valuable platform to guide dendrimer design for nanodrug applications prior to in vivo testing. Furthermore, machine learning can be integrated with the physiologically based pharmacokinetic (PBPK) models to predict nanodrug behaviors within the body.400 For instance, a ML-assisted PBPK model has been developed to predict tumour-targeted delivery efficiency of various nanoparticles in solid tumours.401 Based on a curated Nano-Tumour Database consisting of 288 pharmacokinetic profiles of NPs in tumuors, machine learning algorithms were trained to predict critical input parameters, including the maximum uptake rate constant, release rate constant, Hill coefficient and time reaching 50% maximum uptake rate, for the PBPK model. The resulting ML-assisted PBPK model is capable of rapidly evaluating the delivery efficiency and biodistribution of nanoparticles based on their physicochemical properties, which correlate well with experimentally measured pharmacokinetic profiles (R2 up 0.70 for 133 out of 288 datasets). In order to reduce the reliance on animal testing for determining pharmacokinetic profiles of nanodrugs, the FORECAST (Fluorescence Cell Assay and Simulation Technique) has been developed. This technique integrates in vitro assays and machine learning algorithms, enabling efficient and reliable high-throughput screening to collect kinetic data of cell–nanoparticle interactions, thereby holding great potential to enhance predictions of nanoparticle distribution.402
7.6. Machine learning in nanodrug formulation
In addition to focusing on the interfacial interactions that determine the medical functions of nanodrugs, machine learning has also been widely applied to address problems in nanodrug formulation, such as drug solubility and nanoparticle formation. Similarly, both experiment- and computation-driven machine learning models have been reported.
7.6.1. Drug solubility.
Supercritical carbon dioxide (scCO2) is a promising green technology for enhancing the bioavailability of poorly water-soluble drugs. A number of machine learning models have been employed to analyse the impact of variables such as temperature and pressure on solubility.403,404 These models can accurately predict drug solubility, indicating a strong fit to the data. Notably, the ET model has emerged as a standout in several studies for its precision and ability to generalize well, even with small datasets. More comprehensive ML models have been developed to predict the solubility of drug-like compounds in scCO2.405 The obtained ML models use a variety of chemical descriptors, outperforming the DFT-based approach in terms of prediction accuracy and speed. The CatBoost model with alvaDesc descriptors406 demonstrates the most accurate predictions for the solubility of 187 drugs, with an average absolute relative deviation (AARD) of 1.8%. However, when extrapolating predictions for new compounds, the CatBoost model incorporating CDK descriptors407 and drug melting points show satisfactory accuracy (AARD = 14.3%). The success of these models suggests a promising future for the application of ML in drug solubility prediction and the other pharmaceutical development.
7.6.2. Particle size.
As discussed in above sections, the therapeutic efficacy of nanodrugs is significantly influenced by the size of nanoparticles. Therefore, it is of crucial importance to control particle sizes and their distribution in nanodrug formulations. Machine learning techniques enable the prediction of particle sizes based on synthetic conditions, which is promising to streamline the nanodrug formulation process with the desired therapeutic efficacy. A multi-layered graph convolutional network combined with a fully connected neural network has been constructed to predict the sizes of poly(methacrylates).408 The model was trained on a dataset of 3753 nanoparticle formulations based on polymer structure, degree of polymerisation, and formulation parameters (solvent, antisolvent, surfactant, as well as concentrations of polymer and surfactant). High generalizability and accuracy in predicting particle sizes can be achieved, even for polymers outside the training set. Therefore, the time-consuming trial-and-error efforts can be significantly reduced by machine learning, which is also applicable to functionalised nanoparticles. For example, an artificial neural network was employed to analyse the impact of PEG chain length and chitosan/PEG ratio on the nanoparticle size and zeta potential.409 By considering pH as a critical parameter, the ANN model can not only predict nanoparticle properties but also correlate these properties with cell–nanoparticle adhesion behaviour, providing insights into the design of nanoparticles for targeted drug delivery.410,411
Microfluidic-based preparation methods exhibit high reproducibility and the ability to scale up, making them promising for the production of nanoparticles with uniform sizes. The design of experiments (DoE) principles and machine learning techniques were used together to optimise the microfluidic production process of liposomes.412 By applying the DoE, key factors influencing liposome size and size distribution were identified, including cholesterol concentration, total flow rate, and flow rate ratio. Subsequently, an ANN model was trained to predict liposome size and its distribution, demonstrating high accuracy with minimal deviation from experimental results. The model's accuracy in predicting these parameters indicates the potential of machine learning to accelerate the development and clinical translation of precision nanodrugs.
7.6.3. Stability.
The stability of nanodrugs, both in vitro and in vivo, is a critical factor that determines their success in clinical applications. In vitro stability refers to the capacity of a nanodrug to retain its structure and functionality under laboratory conditions. It is essential that the nanoformulation should remain stable and free from degradation or aggregation throughout the preparation and storage process. In vivo stability refers to the behaviour of nanodrugs within the complex biological environment of the body. A number of factors, including enzymatic degradation, opsonization, and clearance by the immune system, can influence the in vivo stability of nanodrugs. It is therefore evident that the stability of nanodrugs is of great importance not only for their formulation and storage but also for their pharmacokinetics and biodistribution, as well as their therapeutic efficacy. However, it remains challenging to computationally predict the stability of nanodrugs, particularly for those based on polymers and biomolecules. In this regard, machine learning has shown great potential for addressing the stability of nanodrugs. As introduced above, machine learning can predict inter- and intra-particle interactions and/or interfacial interactions between nanodrugs and biological entities, as well as the pharmacokinetics to assess their in vitro/vivo stability. A recent study has also demonstrated the application of machine learning in optimising the synthetic conditions of curcumin-loaded liposomes.413 A library consisting of over 200 liposome configurations with diverse dispersity and stability is adopted to train support-vector machine models with accuracies of 94% and 92%, respectively.
7.7. FAIR principles and data management
The application of machine learning techniques in nanodrug discovery significantly depends on the quantity and quality of structured databases employed. Most of the aforementioned studies employed databases consisting of data reported by a single or a limited number of groups for a range of purposes including the development of different types of nanodrugs and formulations. In addition, while the computational data for various nanodrugs has been extensively reported, the corresponding databases remain severely limited. These databases can be highly valuable not only for gaining insight into the bio–nano interfacial interactions but also for serving as endpoints coupled with experimental data to optimise the therapeutic efficacy of nanodrugs. The lack of such databases, likely due to the absence of established guidelines on standard data sharing and management, may hinder the advancement of digital nanodrug discovery.
In order to enhance the reusability of scholarly data, a group of stakeholders, including academia, industry, funding agencies, and scholarly publisher, have designed and jointly endorsed the FAIR principles for data sharing and management, emphasising that data should be findable (F), accessible (A), interoperable (I), and reusable (R).414 In these principles, findability requires that the data consists of rich metadata. In addition, both the data and the metadata are assigned with unique and persistent identifiers, such as Digital Object Identifiers (DOIs), and are indexed in searchable resources. Accessibility emphasises the importance of data/metadata to be retrievable via standardised communication protocols, which should be open, free, and universally implementable, or allow for an authentication and authorization procedure. Interoperability refers to the ability of data to be integrated with other datasets, applications, and workflows for analysis, storage, and processing with minimal effort. Finally, reusability necessitates that data are sufficiently described, accompanied by clear usage licenses and detailed provenance. The FAIR principles have gained considerable attention across various scientific communities, including nanotechnology, as a means to address the complexity of data management and to maximize the use of data assets.
The implementation of FAIR principles in the construction of nanodrug databases is crucial, but challenging. For instance, there is currently no persistent identifiers for nanomaterials and community standards for reporting data/metadata related to the therapeutic efficacy of nanodrugs, the physicochemical properties of nanomaterials, or their interfacial interactions with biological entities. Therefore, researchers may only report and contrast databases of data/metadata that align with their specific research interests. The resulting databases are sometimes distributed across specific repositories and are not universally accessible to researchers. Despite these challenges, progress has been made in the FAIR data management of nanotechnology, such as the nanosafety data. A variety of tools and/or protocols have been developed for data FAIRification and management, including the establishment of data repositories with specialised or general-purpose, software and workflows, data management and standardisation tools, as well as community engagement and collaboration.415–419
The eNanoMapper database is a representative example of a repository that follows the FAIR principles, thereby facilitating the effective reuse of nanosafety data.420 The database incorporates community-developed standards, including community-specific ontologies and human- and machine-readable identifiers. This facilitates the pathway towards optimised data reuse and prevents the duplication of research efforts. A FAIRification workflow was developed, whereby Excel spreadsheets can be converted into a FAIR-compliant database, with the aid of the NMDataParser tool.421 This demonstrates the importance of the use of standardised templates for data entry. Likewise, the NanoCommons Knowledge Base was established to promote the implementation of FAIR data principles by sustained community cooperation, thereby ultimately supporting more effective risk assessment and governance of nanomaterials.422,423 While there are challenges for the implementation of FIAR principles in nanodrug discovery, advances in other fields, such as nanomaterials,420 proteomics,424 marine biology,425 and additive manufacturing,417 demonstrate solutions to achieve the goals of FAIR data management.
7.8. Summary
In summary, the application of machine learning techniques has enabled the addressing of inherent challenges associated with the discovery of nanodrugs. This is achieved by accelerating the identification of promising candidates and optimising their formulation. By leveraging the databases constructed from computations or experiments, machine learning models have been trained with sufficient accuracy to evaluate the interfacial interactions associated with surface adsorption, supramolecular recognition, surface catalysis, chemical conversion, which collectively determines the therapeutic efficacy, toxicity, and pharmacokinetics of nanodrugs. Furthermore, machine learning can be employed to direct the formulation of nanodrugs in terms of stability, distribution, and quality control, including the monitoring of particle size. By identifying structural features at the atomic or particle level, it is possible to guarantee the success of nanoformulations, thereby reducing the time and cost associated with nanodrug discovery. As the corpus of nanodrug data continues to grow, there is an urgent need for a standardised methodology and collaborative efforts to address the multifaceted nature of computational and digital nanodrug discovery. The introduction of the FAIR principles and data management offers a strategic framework that fosters the open science and collaborative research, ensuring that the collective pursuit of nanodrug innovation is both efficient and impactful.
8. Conclusions and prospects
Nanodrugs are continually attracting considerable interest from a broad community due to their remarkable therapeutic efficacy and safety compared to conventional therapeutics. The customizable nature of nanomaterials offers a wide range of opportunities for disease prevention and therapy, yet it also results in significant challenges in precisely optimising the physicochemical properties of nanomaterials to achieve optimal therapeutic outcomes. This review delves into the theoretical understanding of four key interfacial interactions, including surface adsorption, supramolecular recognition, surface catalysis, and chemical conversion, that significantly influence the therapeutic efficacy of nanodrugs. These interactions are crucial for aspects such as drug loading, blood circulation time, cellular uptake, selective recognition, targeted delivery, drug release, and degradation. Understanding how these interfacial interactions affect the therapeutic efficacy of nanodrugs is of invaluable for accelerating nanodrug discovery. For example, a medium adsorption energy of the drug molecule is recommended for surface adsorption-based drug delivery systems to ensure sufficient drug loading and release. Similarly, a moderate adsorption energy for key intermediates is necessary for catalysis-based nanodrugs to maximize the catalytic therapeutic efficacy. To regulate protein adsorption or supramolecular recognition of nanodrugs, surface functionalisation is necessary, for which the ligand type, charge, length, coating density play important roles. These strategies can rationalize the existing experimental results or be integrated with further experiments to discover more effective nanodrugs. In addition to the conventional physics-based computational methods, such as density functional theory calculations and molecular dynamics simulations, this review also explores the potential of machine learning in accelerating nanodrug discovery, with training data from either experiments or conventional computations.
However, due to the complex physiological and pathological conditions that vary across diseases and/or patient populations, the development of one-size-fits-all nanodrugs is unrealistic. Personalisation and precision are key goals in the further development of nanodrugs. This necessitates the simultaneous optimisation of all physicochemical properties (compositions, structures, surface functionalities and responsiveness) of nanomaterials to achieve the maximum therapeutic efficacy while minimising side effects. Despite the considerable computational advancements in nanodrugs, most of current studies have focused on individual types of interfacial interactions related to the medical functions of nanomaterials. This is mainly due to the extensive design space of nanodrugs, which is unable to be fully explored by physics-based computational methods. Therefore, computer-aided nanodrug discovery is still in its infancy compared to conventional drug development. The incorporation of machine learning into nanodrug discovery offers new opportunities, showing significant potential to more efficiently explore the design space of nanodrugs by utilising decades of published data and combining computational and experimental techniques. The integrated “computation + machine learning + experimentation” approach has a promising future for accelerating nanodrug discovery, despite several critical challenges to be addressed.
(I) Identification code for specific nanomaterials
The therapeutic efficacy of nanomaterials is significantly dependent on their properties (compositions, structures, surface functionalities and responsiveness), which must be precisely regulated in the nanodrug discovery process. However, these properties have been diversely described in the literature, which poses a significant challenge to incorporating machine learning techniques into the nanodrug discovery process. Therefore, a standardised identification code that is able to uniquely describe the features of nanomaterials becomes essential. The desired code would not only ensure that all relevant properties of nanomaterials are accounted for, but also facilitate the precise and efficient data exchange and extraction. Recently, the IUPAC International Chemical Identifier (InChI) was extended for nanomaterials (NInChI),426 which can represent the complex physicochemical properties of diverse nanomaterials. Further attempts to develop such an identification code are continuing, considering more mathematical or computable descriptors.
(II) High-quality datasets for nanodrug discovery
The high-quality datasets are of great importance for the advancement of machine learning-assisted nanodrug discovery. However, acquiring these datasets is challenging due to the complexity of nanomaterials and their interactions with biological systems. Currently, only a few datasets, such as those on nanotoxicity and enzyme-like nanocatalysts, are accessible. It is indispensable to construct robust and comprehensive databases that document a wide range of nanomaterials, including their physicochemical properties and therapeutic effects. In addition, these databases can incorporate computational endpoints of the interfacial interactions between nanomaterials and biological entities, such as protein adsorption (binding sites, structures, and strengths) and membrane penetration dynamics. The development of an identification code for nanomaterials, high-throughput experiments and computations, as well as data sharing within the scientific community, will significantly promote the construction of datasets for nanodrug discovery. These datasets should be of high-quality, accessible, up-to-date and well-maintained, thereby significantly advancing nanodrug discovery.
(III) In-depth understanding of interfacial interactions
Unlike conventional drugs, where the focus is primarily on the binding affinity between molecules and their targets, nanodrugs are influenced by a variety of complex interactions (surface adsorption, supramolecular recognition, surface catalysis, and chemical conversion) with biological systems. However, existing studies of these interfacial interactions to guide nanodrug discovery have limitations. First, the qualitative guidelines used in computational nanodrug design do not provide a clear framework for how to effectively regulate these interactions. The extent to which these interactions should be modulated to achieve optimal therapeutic outcomes remains largely unknown. In addition, most theoretical studies of interfacial interactions have been conducted under conditions that do not accurately reflect the in vivo environment. For example, in the case of surface catalysis-based nanodrugs, current theoretical studies have mostly been conducted in the absence of solvents, using clean slabs as model systems. This does not capture the influence of surface functionalisation or stimuli-responsive reconstruction on the catalytic activity and associated therapeutic efficacy. These phenomena have been observed in recent experimental studies.396,427,428 Therefore, a more in-depth understanding of these interfacial interactions, which bridge the physicochemical properties of nanomaterials and their therapeutic efficacy, is of crucial importance for the future design of precision nanodrugs with optimal properties. Not only theoretical calculations, but also advanced characterisation techniques, such as spectroscopy and microscopy, are highly warranted. The data obtained can be integrated into machine learning models, which in turn help to uncover the quantitative relationships between nanomaterials and their interfacial interactions with biological systems, as well as factors associated with their therapeutic efficacy.
(IV) Genetic map of protein–nanomaterial interactions
Protein adsorption plays a vital role in the in vivo processing of nanodrugs. The current knowledge of protein–nanomaterial interactions (PNI) is still very limited, which may hinder the efficiency of computer-aided nanodrug discovery. Therefore, it is of great significance to map the interfacial interactions between different types of nanoparticles and various proteins. However, the complexity of the proteome and the diverse nature of protein–nanomaterial interactions present significant challenges to the genetic construction of the PNI map. To address these challenges, ongoing efforts have been focused on the development of high-throughput screening methods and computational tools with the assistance of machine learning techniques. The constructed PNI genetic map may facilitate the development of more efficient and targeted nanodrugs by precisely controlling protein adsorption.429–431
(V) Machine learning-assisted computation of interfacial interactions
The interfacial interactions between nanomaterials and biological entities are too complex for traditional computational methods to describe efficiently. Machine learning has demonstrated the potential to address these challenges and to more efficiently characterise the interfacial interactions.432,433 For example, machine learning algorithms can be trained to predict the binding affinity for the adsorption of molecular species on nanosurfaces based on a small subset of computational data.26,434 Furthermore, the machine-learning potentials with DFT accuracy allow for the efficient and reliable exploration of a wider range of interaction scenarios without the need for excessive computational resources.435 Machine learning can also significantly accelerate computations of the interfacial interactions that are essential for understanding the medical functions of nanodrugs, such as protein–nanomaterial and membrane–nanomaterial interactions. By accelerating these computations, machine learning can facilitate a deeper exploration of the design space for nanodrugs, enabling the rapid evaluation of numerous candidate materials and their potential therapeutic effects.
(VI) Mechanism-incorporated machine learning models
Although machine learning models can be trained to predict the therapeutic endpoints of nanomaterials based on their physiochemical properties,436,437 their accuracy may be limited without an in-depth understanding of the interfacial interactions between nanomaterials and biological entities. Incorporating mechanistic knowledge into machine learning models may potentially improve their predictive capability and interpretability. However, the effective integration of complex mechanistic information with experimental therapeutic data of nanomaterials remains largely unexplored. As mechanistic insights into the medical functions of nanomaterials continue to expand, it becomes feasible to develop the mechanism-incorporated machine learning models for nanodrug discovery, which could lead to more accurate predictions and provide insights into the fundamental processes governing nanodrug behavior.
(VII) Comprehensive assessment platform for nanodrug efficacy
Developing a comprehensive assessment platform is of great importance for the efficient computer-aided nanodrug discovery. This platform is designed to thoroughly evaluate the nanodrug efficacy, by simultaneously optimising the physicochemical properties of nanomaterials, which would regulate their interactions with biological entities, ensuring the desired drug loading, blood circulation time, cellular uptake, selective recognition, targeted delivery, drug release, degradation, pharmacokinetics, and low toxicity. The platform would integrate various assays and analytical techniques to provide a comprehensive assessment of nanodrug candidates, integrating computational, machine learning, and experimental approaches.
Data availability
No primary research results, software or code have been included and no new data were generated or analysed as part of this review.
Conflicts of interest
There are no conflicts to declare.
Acknowledgements
This work was supported by the Basic Science Center Project of the National Natural Science Foundation of China (22388101), the National Key Research and Development Program of China (2022YFA1207300) and Natural Science Foundation of China (22203020, 22303020, 52161135107).
Notes and references
- G. Chen, I. Roy, C. Yang and P. N. Prasad, Chem. Rev., 2016, 116, 2826–2885 CrossRef CAS PubMed.
- M. A. Beach, U. Nayanathara, Y. Gao, C. Zhang, Y. Xiong, Y. Wang and G. K. Such, Chem. Rev., 2024, 124, 5505–5616 CrossRef CAS PubMed.
- Y. Zhu, Q. Li, C. Wang, Y. Hao, N. Yang, M. Chen, J. Ji, L. Feng and Z. Liu, Chem. Rev., 2023, 123, 7326–7378 CrossRef CAS PubMed.
- L. Chen, S. Zhang, Y. Duan, X. Song, M. Chang, W. Feng and Y. Chen, Chem. Soc. Rev., 2024, 53, 1167–1315 RSC.
- B. Chang, L. Zhang, S. Wu, Z. Sun and Z. Cheng, Chem. Soc. Rev., 2022, 51, 3688–3734 RSC.
- Z. Pei, H. Lei and L. Cheng, Chem. Soc. Rev., 2023, 52, 2031–2081 RSC.
- C. Altintas, I. Erucar and S. Keskin, CrystEngComm, 2022, 24, 7360–7371 RSC.
- A. K. Bindra, D. Wang and Y. Zhao, Adv. Mater., 2023, 35, e2300700 CrossRef PubMed.
- J. Kim, S. Lee, Y. Kim, M. Choi, I. Lee, E. Kim, C. G. Yoon, K. Pu, H. M. Kang and J. S. Kim, Nat. Rev. Mater., 2023, 8, 710–725 CrossRef.
- Q. Zhou, J. Xiang, N. Qiu, Y. Wang, Y. Piao, S. Shao, J. Tang, Z. Zhou and Y. Shen, Chem. Rev., 2023, 123, 10920–10989 CrossRef CAS PubMed.
- Y. Barenholz, J. Controlled Release, 2012, 160, 117–134 CrossRef CAS PubMed.
- T. Safra, F. Muggia, S. Jeffers, D. D. Tsao-Wei, S. Groshen, O. Lyass, R. Henderson, G. Berry and A. Gabizon, Ann. Oncol., 2000, 11, 1029–1034 CrossRef CAS PubMed.
- J. Wu, X. Wang, Q. Wang, Z. Lou, S. Li, Y. Zhu, L. Qin and H. Wei, Chem. Soc. Rev., 2019, 48, 1004–1076 RSC.
- B. Yang, Y. Chen and J. Shi, Adv. Mater., 2019, 31, 1901778 CrossRef PubMed.
- C. Zhang, L. Yan, X. Wang, S. Zhu, C. Chen, Z. Gu and Y. Zhao, Nano Today, 2020, 35, 101008 CrossRef CAS.
- L. M. Liz-Marzán, A. E. Nel, C. J. Brinker, W. C. W. Chan, C. Chen, X. Chen, D. Ho, T. Hu, K. Kataoka, N. A. Kotov, W. J. Parak and M. M. Stevens, ACS Nano, 2022, 16, 13257–13259 CrossRef.
- M. J. Mitchell, M. M. Billingsley, R. M. Haley, M. E. Wechsler, N. A. Peppas and R. Langer, Nat. Rev. Drug Discovery, 2021, 20, 101–124 CrossRef CAS PubMed.
- M. Shamsi, A. Mohammadi, M. K. D. Manshadi and A. Sanati-Nezhad, J. Controlled Release, 2019, 307, 150–165 CrossRef CAS PubMed.
- T. Casalini, J. Controlled Release, 2021, 332, 390–417 CrossRef CAS PubMed.
- X. Shen, Z. Wang, X. J. Gao and X. Gao, Adv. Mater., 2024, 36, 2211151 CrossRef CAS PubMed.
- X. J. Gao, Y. Zhao and X. Gao, Acc. Chem. Res., 2023, 56, 2366–2377 CrossRef CAS PubMed.
- Z. Chen, Y. Yu, Y. Gao and Z. Zhu, ACS Nano, 2023, 17, 13062–13080 CrossRef CAS PubMed.
- J.-J. Zheng, X. Wang, Z. Li, X. Shen, G. Wei, P. Xia, Y.-G. Zhou, H. Wei and X. Gao, ACS Nano, 2024, 18, 1531–1542 CrossRef CAS PubMed.
- J. Zhuang, A. C. Midgley, Y. Wei, Q. Liu, D. Kong and X. Huang, Adv. Mater., 2024, 36, 2210848 CrossRef CAS PubMed.
- Z. Ban, P. Yuan, F. Yu, T. Peng, Q. Zhou and X. Hu, Proc. Natl. Acad. Sci. U. S. A., 2020, 117, 10492–10499 CrossRef CAS PubMed.
- X. J. Gao, J. Yan, J.-J. Zheng, S. Zhong and X. Gao, Adv. Healthcare Mater., 2023, 12, 2202925 CrossRef CAS PubMed.
- Y. Jiang, Z. Chen, N. Sui and Z. Zhu, J. Am. Chem. Soc., 2024, 146, 7565–7574 CrossRef CAS PubMed.
- A. V. Singh, M. H. D. Ansari, D. Rosenkranz, R. S. Maharjan, F. L. Kriegel, K. Gandhi, A. Kanase, R. Singh, P. Laux and A. Luch, Adv. Healthcare Mater., 2020, 9, 1901862 CrossRef CAS PubMed.
- S. T. Johnston, M. Faria and E. J. Crampin, Nanoscale Adv., 2021, 3, 2139–2156 RSC.
- N. Sharma, M. Sharma, Q. M. Sajid Jamal, M. A. Kamal and S. Akhtar, Environ. Sci. Pollut. Res., 2020, 27, 19127–19141 CrossRef PubMed.
- R. Dixit, K. Khambhati, K. V. Supraja, V. Singh, F. Lederer, P.-L. Show, M. K. Awasthi, A. Sharma and R. Jain, Bioresour. Technol., 2023, 370, 128522 CrossRef CAS PubMed.
- X. Dai and Y. Chen, Adv. Mater., 2023, 35, 2204798 CrossRef CAS PubMed.
- X. Yan, T. Yue, D. A. Winkler, Y. Yin, H. Zhu, G. Jiang and B. Yan, Chem. Rev., 2023, 123, 8575–8637 CrossRef CAS PubMed.
- W. Wang, Z. Ye, H. Gao and D. Ouyang, J. Controlled Release, 2021, 338, 119–136 CrossRef CAS PubMed.
-
D. Ouyang and S. C. Smith, Computational Pharmaceutics: Application of Molecular Modeling in Drug Delivery, John Wiley & Sons Ltd, Chichester, 2015 Search PubMed.
- H. Gao, W. Wang, J. Dong, Z. Ye and D. Ouyang, Eur. J. Pharm. Biopharm., 2021, 158, 336–346 CrossRef CAS PubMed.
-
J. W. Fisher, J. M. Gearhart and Z. Lin, Physiologically Based Pharmacokinetic (PBPK) Modeling Methods and Applications in Toxicology and Risk Assessment, Academic Press, Berkeley, 2020 Search PubMed.
- L. Zhao, M.-J. Kim, L. Zhang and R. Lionberger, Clin. Pharmacol. Ther., 2019, 105, 338–349 CrossRef PubMed.
- S. H. Chen, D. R. Bell and B. Luan, Adv. Drug Delivery Rev., 2022, 186, 114336 CrossRef CAS PubMed.
- N. Tarrat, M. Benoit, M. Giraud, A. Ponchet and M. J. Casanove, Nanoscale, 2015, 7, 14515–14524 RSC.
- T. N. Kha, N. T. Si, V. M. Tran, K. Q. Vo, M. T. Nguyen and P. V. Nhat, ACS Omega, 2023, 8, 43442–43453 CrossRef CAS PubMed.
- P. Niknam, S. Jamehbozorgi, M. Rezvani and V. Izadkhah, Phys. E, 2022, 135, 114937 CrossRef CAS.
- C. Yao, F. Xiang and Z. Xu, J. Mol. Model., 2022, 28, 64 CrossRef CAS PubMed.
- F. Nattagh, S. Hosseini and M. D. Esrafili, J. Mol. Liq., 2021, 342, 117459 CrossRef CAS.
- C. G. Apebende, G. J. Ogunwale, H. Louis, I. Benjamin, M. T. Kadiri, A. E. Owen and A.-L. E. Manicum, Mater. Sci. Semicond. Process., 2023, 158, 107362 CrossRef CAS.
- V. Saravanan, A. Rajamani, M. Subramani and S. Ramasamy, Comput. Biol. Chem., 2020, 88, 107334 CrossRef CAS PubMed.
- M. Vatanparast and Z. Shariatinia, J. Mater. Chem. B, 2019, 7, 6156–6171 RSC.
- E. Shakerzadeh, J. Mol. Liq., 2021, 343, 116970 CrossRef CAS.
- X. Tu, H. Xu, C. Li, X. Liu, G. Fan and W. Sun, Comput. Theor. Chem., 2021, 1203, 113360 CrossRef CAS.
- Z. Khodadadi and L. Torkian, Mater. Res. Express, 2019, 6, 065058 CrossRef CAS.
- Z. Tabandeh and A. Reisi-Vanani, Diam. Relat. Mater., 2021, 119, 108564 CrossRef CAS.
- C. Tabtimsai and B. Wanno, J. Mol. Liq., 2021, 337, 116596 CrossRef CAS.
- N. Ershadi, R. Safaiee and M. M. Golshan, Appl. Surf. Sci., 2021, 536, 147718 CrossRef CAS.
- T. P. Alexandrovich and A. Khan, Diam. Relat. Mater., 2022, 124, 108900 CrossRef CAS.
- H. Xu, Q. Wang, G. Fan and X. Chu, Theor. Chem. Acc., 2018, 137, 104 Search PubMed.
- R. Khorram, H. Raissi and A. Morsali, J. Mol. Liq., 2017, 240, 87–97 CrossRef CAS.
- M. D. Moghaddam, S. Jamehbozorgi, M. Rezvani, V. Izadkhah and M. T. Moghim, Phys. E, 2022, 138, 115077 CrossRef CAS.
- M. M. Kadhim, S. K. Hachim, S. Alomar, T. Z. Taban, S. A. H. Abdullaha and N. Alnasoud, Silicon, 2023, 15, 4317–4323 CrossRef CAS.
- Y. Yang, A. Sun and M. Eslami, Phys. E, 2021, 125, 114411 CrossRef CAS.
- H. Karim, Shahnaz, M. Batool, M. Yaqub, M. Saleem, M. A. Gilani and S. Tabassum, Appl. Surf. Sci., 2022, 596, 153618 CrossRef CAS.
- A.-S. Hosseini nasr, H. Akbarzadeh and R. Tayebee, J. Mol. Liq., 2018, 254, 64–69 CrossRef CAS.
- R. A. Harris, J. Mol. Liq., 2019, 288, 111084 CrossRef CAS.
- K. S. Exner and A. Ivanova, J. Phys. Chem. B, 2021, 125, 2098–2104 CrossRef CAS PubMed.
- M. Shahabi and H. Raissi, Appl. Surf. Sci., 2018, 455, 32–36 CrossRef CAS.
- M. M. Mirhosseini, R. Khordad and B. Vaseghi, Chin. J. Phys., 2019, 62, 99–105 CrossRef CAS.
- S. Zheng, J. Xiong, L. Wang, D. Zhai, Y. Xu and F. Lin, Front. Chem., 2021, 9, 664355 CrossRef CAS PubMed.
- H. Qiao, X. Wang, P. Liao, C. Zhang and C. Liu, Chemosphere, 2021, 284, 131334 CrossRef CAS PubMed.
- M. Sahnoune, N. Tokhadzé, S. E. C. El Kettani, J. Devémy, F. Goujon, P. Chennell, A. Dequidt, C. Goutaudier, V. Sautou and P. Malfreyt, ACS Appl. Polym. Mater., 2022, 4, 4538–4550 CrossRef CAS.
- M. Sahnoune, N. Tokhadzé, J. Devémy, A. Dequidt, F. Goujon, P. Chennell, V. Sautou and P. Malfreyt, ACS Appl. Mater. Interfaces, 2021, 13, 18594–18603 CrossRef CAS PubMed.
- K. R. Sims, Jr., B. He, H. Koo and D. S. W. Benoit, ACS Omega, 2020, 5, 12649–12659 CrossRef PubMed.
- H. Iesavand, M. Rahmati, D. Afzali and S. Modiri, Mater. Sci. Eng., C, 2019, 105, 110010 CrossRef CAS PubMed.
- N. G. Khouri, J. O. Bahú, C. Blanco-Llamero, P. Severino, V. O. C. Concha and E. B. Souto, J. Mol. Struct., 2024, 1309, 138243 CrossRef CAS.
- A. Dobhal, A. Srivastav, P. Dandekar and R. Jain, J. Mater. Sci.: Mater. Med., 2021, 32, 126 CrossRef CAS PubMed.
- J. M. Chan, L. Zhang, K. P. Yuet, G. Liao, J.-W. Rhee, R. Langer and O. C. Farokhzad, Biomaterials, 2009, 30, 1627–1634 CrossRef CAS PubMed.
- D. Karataş, A. Tekin, F. Bahadori and M. S. Çelik, J. Mater. Chem. B, 2017, 5, 8070–8082 RSC.
- J. Wang, Y. Li and G. Nie, Nat. Rev. Mater., 2021, 6, 766–783 CrossRef CAS PubMed.
- M. Shahabi and H. Raissi, J. Mol. Liq., 2018, 268, 326–334 CrossRef CAS.
- S. Tanreh, M. Rezvani and M. Darvish Ganji, J. Phys. Chem. Solids, 2023, 174, 111171 CrossRef CAS.
- A. Singhal, J. D. Schneible, R. L. Lilova, C. K. Hall, S. Menegatti and A. Grafmüller, Soft Matter, 2020, 16, 10591–10610 RSC.
- C. Wang, Y. Yu, M. Irfan, B. Xu, J. Li, L. Zhang, Z. Qin, C. Yu, H. Liu and X. Su, Small, 2020, 16, 2002578 CrossRef CAS PubMed.
- Y. Xu, S.-W. Huang, Y.-Q. Ma and H.-M. Ding, Nanoscale Adv., 2022, 4, 754–760 RSC.
- M. Sun, X. Zhang, Z. Gao, T. Liu, C. Luo, Y. Zhao, Y. Liu, Z. He, J. Wang and J. Sun, Nanoscale, 2019, 11, 3864–3876 RSC.
- M. B. Marnani, M. Oftadeh and N. Sohrabi, J. Mol. Model., 2023, 29, 213 CrossRef CAS PubMed.
- L. Li, C. J. Fang, J. C. Ryan, E. C. Niemi, J. A. Lebrón, P. J. Björkman, H. Arase, F. M. Torti, S. V. Torti, M. C. Nakamura and W. E. Seaman, Proc. Natl. Acad. Sci. U. S. A., 2010, 107, 3505–3510 CrossRef CAS PubMed.
- S. Yin, Y. Liu, S. Dai, B. Zhang, Y. Qu, Y. Zhang, W.-S. Choe and J. Bi, Biosensors, 2021, 11, 444 CrossRef CAS PubMed.
- S. Mollazadeh, M. Yazdimamaghani, R. Yazdian-Robati and S. Pirhadi, Comput. Biol. Med., 2022, 141, 105158 CrossRef CAS PubMed.
- D. Xu, X. Chen, Z. Chen, Y. Lv, Y. Li, S. Li, W. Xu, Y. Mo, X. Wang, Z. Chen, T. Chen, T. Wang, Z. Wang, M. Wu and J. Wang, Front. Bioeng. Biotechnol., 2022, 10, 859255 CrossRef PubMed.
- J. P. M. Jämbeck, E. S. E. Eriksson, A. Laaksonen, A. P. Lyubartsev and L. A. Eriksson, J. Chem. Theory Comput., 2014, 10, 5–13 CrossRef PubMed.
- K. Islam, M. Razizadeh and Y. Liu, Phys. Chem. Chem. Phys., 2023, 25, 12308–12321 RSC.
- M. Kotzabasaki and G. E. Froudakis, Inorg. Chem. Front., 2018, 5, 1255–1272 RSC.
- L. Li, Y. Zhu, Z. Qi, X. Li, H. Pan, B. Liu and Y. Liu, Appl. Organomet. Chem., 2023, 37, e7199 CrossRef CAS.
- A. Botet-Carreras, C. Tamames-Tabar, F. Salles, S. Rojas, E. Imbuluzqueta, H. Lana, M. J. Blanco-Prieto and P. Horcajada, J. Mater. Chem. B, 2021, 9, 2233–2239 RSC.
- A. T. Sose, H. D. Cornell, B. J. Gibbons, A. A. Burris, A. J. Morris and S. A. Deshmukh, RSC Adv., 2021, 11, 17064–17071 RSC.
- R. Karimi Alavijeh and K. Akhbari, Colloids Surf., B, 2022, 212, 112340 CrossRef CAS PubMed.
- Y. Fu, Z. Kang, W. Cao, J. Yin, Y. Tu, J. Li, H. Guan, Y. Wang, Q. Wang and X. Kong, Angew. Chem., Int. Ed., 2021, 60, 7719–7727 CrossRef CAS PubMed.
- J. Liu, Z. Yang, Y. Che, Y. Zhang, Z. Zhang and C.-X. Zhao, AlChE J., 2022, 68, e17474 CrossRef CAS.
- M. Ernst and G. Gryn'ova, Helv. Chim. Acta, 2023, 106, e202300013 CrossRef CAS.
- P. J. Jodłowski, K. Dymek, G. Kurowski, J. Jaśkowska, W. Bury, M. Pander, S. Wnorowska, K. Targowska-Duda, W. Piskorz, A. Wnorowski and A. Boguszewska-Czubara, ACS Appl. Mater. Interfaces, 2022, 14, 28615–28627 CrossRef PubMed.
- D. Kim, J. M. Yoo, H. Hwang, J. Lee, S. H. Lee, S. P. Yun, M. J. Park, M. Lee, S. Choi, S. H. Kwon, S. Lee, S.-H. Kwon, S. Kim, Y. J. Park, M. Kinoshita, Y.-H. Lee, S. Shin, S. R. Paik, S. J. Lee, S. Lee, B. H. Hong and H. S. Ko, Nat. Nanotechnol., 2018, 13, 812–818 CrossRef CAS PubMed.
- Q. Xiao, C. K. McAtee and X. Su, Nat. Rev. Immunol., 2022, 22, 188–199 CrossRef CAS PubMed.
- T. John, A. Gladytz, C. Kubeil, L. L. Martin, H. J. Risselada and B. Abel, Nanoscale, 2018, 10, 20894–20913 RSC.
- M. Carballo-Pacheco and B. Strodel, J. Phys. Chem. B, 2016, 120, 2991–2999 CrossRef CAS PubMed.
- Z. Yang, C. Ge, J. Liu, Y. Chong, Z. Gu, C. A. Jimenez-Cruz, Z. Chai and R. Zhou, Nanoscale, 2015, 7, 18725–18737 RSC.
- S. Gotla and S. Matysiak, Phys. Chem. Chem. Phys., 2023, 25, 10113–10120 RSC.
- L. Zhu, G. Wang, X. Zhu, Q. Zhang and Z. Qian, ACS Appl. Nano Mater., 2023, 6, 9649–9656 CrossRef CAS.
- H. Liu, B. Ojha, C. Morris, M. Jiang, E. P. Wojcikiewicz, P. P. N. Rao and D. Du, Biomacromolecules, 2015, 16, 2363–2373 CrossRef CAS PubMed.
- G. Viola, F. Floriani, C. G. Barracchia, F. Munari, M. D’Onofrio and M. Assfalg, Chem. – Eur. J., 2023, 29, e202301274 CrossRef CAS PubMed.
- M. Ghaeidamini, D. Bernson, N. Sasanian, R. Kumar and E. K. Esbjörner, Nanoscale, 2020, 12, 19450–19460 RSC.
- M. Wang, Y. Sun, X. Cao, G. Peng, I. Javed, A. Kakinen, T. P. Davis, S. Lin, J. Liu, F. Ding and P. C. Ke, Nanoscale, 2018, 10, 19995–20006 RSC.
- J. Wang, H. Dong, T. Leng, Y. Yu and Y. Li, Phys. Chem. Chem. Phys., 2022, 24, 4270–4279 RSC.
- W. Zhao, L. Jiang, W. Wang, J. Sang, Q. Sun, Q. Dong, L. Li, F. Lu and F. Liu, J. Mater. Chem. B, 2021, 9, 6902–6914 RSC.
- C. Bai, D. Lin, Y. Mo, J. Lei, Y. Sun, L. Xie, X. Yang and G. Wei, Phys. Chem. Chem. Phys., 2019, 21, 4022–4031 RSC.
- N. Andrikopoulos, Y. Li, A. Nandakumar, J. F. Quinn, T. P. Davis, F. Ding, N. Saikia and P. C. Ke, ACS Appl. Mater. Interfaces, 2023, 15, 7777–7792 CrossRef CAS PubMed.
- L. Yao, Z. Zhou, S. Wang, Q. Zou, H.-X. Wang, L.-X. Ma, S. Wang and X. Zhang, Chem. Sci., 2022, 13, 5902–5912 RSC.
- S. Radic, T. P. Davis, P. C. Ke and F. Ding, RSC Adv., 2015, 5, 105489–105498 RSC.
- M. Jakubec, E. Bariås, S. Furse, M. L. Govasli, V. George, D. Turcu, I. A. Iashchishyn, L. A. Morozova-Roche and Ø. Halskau, FEBS J., 2021, 288, 1887–1905 CrossRef CAS PubMed.
- T. John, J. Adler, C. Elsner, J. Petzold, M. Krueger, L. L. Martin, D. Huster, H. J. Risselada and B. Abel, J. Colloid Interface Sci., 2022, 622, 804–818 CrossRef CAS PubMed.
- L. Bellucci, G. Bussi, R. Di Felice and S. Corni, Nanoscale, 2017, 9, 2279–2290 RSC.
- T. Nobeyama, K. Tataka, M. Mori, T. Murakami, Y. Yamada and K. Shiraki, Small, 2023, 19, 2300362 CrossRef CAS PubMed.
- J. Yang, Y. He, M. Zhang, C. Liang, T. Li, T. Ji, M. Zu, X. Ma, Z. Zhang, C. Liang, Q. Zhang, Y. Chen and L. Hou, Exploration, 2023, 3, 20230061 CrossRef CAS PubMed.
- B. Li, R. Zhang and X. Shi, Phys. Chem. Chem. Phys., 2019, 21, 1784–1790 RSC.
- D. Zhao and J. Zhou, Phys. Chem. Chem. Phys., 2017, 19, 986–995 RSC.
- M. Di Giosia, T. D. Marforio, A. Cantelli, F. Valle, F. Zerbetto, Q. Su, H. Wang and M. Calvaresi, J. Colloid Interface Sci., 2020, 571, 174–184 CrossRef CAS PubMed.
- Y. Shi, K. Pu, H. Yao, Y. Chen, X. Zheng, L. Zhao, X. Ma and C. Ge, ACS Appl. Mater. Interfaces, 2023, 15, 9034–9043 CrossRef CAS PubMed.
- S. Tiwari, V. Adupa, D. S. Das, K. Anki Reddy and T. V. Bharat, Langmuir, 2022, 38, 9186–9194 CrossRef CAS PubMed.
- J. Wang, Y. Yu, T. Leng, Y. Li and S.-T. Lee, ACS Appl. Mater. Interfaces, 2022, 14, 191–200 CrossRef CAS PubMed.
- S. Du, H. Wang, Y. Yang, X. Feng, X. Shao, C. Chipot and W. Cai, J. Phys. Chem. C, 2019, 123, 922–930 CrossRef CAS.
- S.-g Kang, R. Araya-Secchi, D. Wang, B. Wang, T. Huynh and R. Zhou, Sci. Rep., 2014, 4, 4775 CrossRef PubMed.
- Y. Yu, H. Sun, T. Hou, S. Wang and Y. Li, RSC Adv., 2018, 8, 13997–14008 RSC.
- Y.-B. Miao, W.-Y. Pan, K.-H. Chen, H.-J. Wei, F.-L. Mi, M.-Y. Lu, Y. Chang and H.-W. Sung, Adv. Funct. Mater., 2019, 29, 1904828 CrossRef CAS.
- Q. Zhang, J. Liang, A. Bongers, J. J. Richardson, K. Liang and Z. Gu, Adv. Sci., 2023, 10, 2206546 CrossRef CAS PubMed.
- B. Tavasoli, S. Khanmohammadi, M. Yahyaei, M. Barshan-tashnizi and F. Mehrnejad, J. Mol. Liq., 2023, 369, 120850 CrossRef CAS.
- S. Yang, D. Zhao, Z. Xu, H. Yu and J. Zhou, Phys. Chem. Chem. Phys., 2022, 24, 2866–2878 RSC.
- H. Yue, W. Wei, Z. Gu, D. Ni, N. Luo, Z. Yang, L. Zhao, J. A. Garate, R. Zhou, Z. Su and G. Ma, Nanoscale, 2015, 7, 19949–19957 RSC.
- J. G. Vilhena, A. C. Dumitru, E. T. Herruzo, J. I. Mendieta-Moreno, R. Garcia, P. A. Serena and R. Pérez, Nanoscale, 2016, 8, 13463–13475 RSC.
- A. Jain, G. F. Trindade, J. M. Hicks, J. C. Potts, R. Rahman, R. J. M. Hague, D. B. Amabilino, L. Pérez-García and F. J. Rawson, J. Colloid Interface Sci., 2021, 587, 150–161 CrossRef CAS PubMed.
- W. Lin, T. Insley, M. D. Tuttle, L. Zhu, D. A. Berthold, P. Král, C. M. Rienstra and C. J. Murphy, J. Phys. Chem. C, 2015, 119, 21035–21043 CrossRef CAS PubMed.
- J. Meissner, Y. Wu, J. Jestin, W. A. Shelton, G. H. Findenegg and B. Bharti, Soft Matter, 2019, 15, 350–354 RSC.
- D. L. Z. Caetano, R. Metzler, A. G. Cherstvy and S. J. de Carvalho, Phys. Chem. Chem. Phys., 2021, 23, 27195–27206 RSC.
- L. Cerofolini, M. Fragai, C. Luchinat and E. Ravera, Biophys. Chem., 2020, 265, 106441 CrossRef CAS PubMed.
- M. Mahmoudi, M. P. Landry, A. Moore and R. Coreas, Nat. Rev. Mater., 2023, 8, 422–438 CrossRef PubMed.
- J. Ren, N. Andrikopoulos, K. Velonia, H. Tang, R. Cai, F. Ding, P. C. Ke and C. Chen, J. Am. Chem. Soc., 2022, 144, 9184–9205 CrossRef CAS PubMed.
- J. G. Lee, K. Lannigan, W. A. Shelton, J. Meissner and B. Bharti, Langmuir, 2020, 36, 14157–14165 CrossRef CAS PubMed.
- S. Yu, A. Perálvarez-Marín, C. Minelli, J. Faraudo, A. Roig and A. Laromaine, Nanoscale, 2016, 8, 14393–14405 RSC.
- X. Wang, X. Wang, M. Wang, D. Zhang, Q. Yang, T. Liu, R. Lei, S. Zhu, Y. Zhao and C. Chen, Small, 2018, 14, 1703982 CrossRef PubMed.
- X. Lu, P. Xu, H.-M. Ding, Y.-S. Yu, D. Huo and Y.-Q. Ma, Nat. Commun., 2019, 10, 4520 CrossRef PubMed.
- S. B. Conjeevaram, R. M. Blanchard, A. Kadaba and I. M. Adjei, Nanoscale Adv., 2022, 4, 2671–2681 RSC.
- M. A. Al-Badri, P. Smith, K. T. Al-Jamal and C. D. Lorenz, Adv. Mater. Interfaces, 2022, 9, 2101236 CrossRef CAS.
- J. Grundler, K. Shin, H.-W. Suh, M. Zhong and W. M. Saltzman, ACS Nano, 2021, 15, 16118–16129 CrossRef CAS PubMed.
- A. A. Sousa, S. A. Hassan, L. L. Knittel, A. Balbo, M. A. Aronova, P. H. Brown, P. Schuck and R. D. Leapman, Nanoscale, 2016, 8, 6577–6588 RSC.
- M. S. Jahan Sajib, P. Sarker, Y. Wei, X. Tao and T. Wei, Langmuir, 2020, 36, 13356–13363 CrossRef CAS PubMed.
- F. Ramezani, M. Amanlou and H. Rafii-Tabar, J. Nanopart. Res., 2014, 16, 2512 CrossRef.
- W. Wang and H. Mattoussi, Acc. Chem. Res., 2020, 53, 1124–1138 CrossRef CAS PubMed.
- Z. E. Hughes, M. A. Nguyen, Y. Li, M. T. Swihart, T. R. Walsh and M. R. Knecht, Nanoscale, 2017, 9, 421–432 RSC.
- A. Kyrychenko, Phys. Chem. Chem. Phys., 2015, 17, 12648–12660 RSC.
- M. Samieegohar, F. Sha, A. Z. Clayborne and T. Wei, Langmuir, 2019, 35, 5029–5036 CrossRef CAS PubMed.
- U. Dahal and E. E. Dormidontova, Macromolecules, 2020, 53, 8160–8170 CrossRef CAS.
- N. Mammen, S. Malola, K. Honkala and H. Häkkinen, Nanoscale, 2020, 12, 23859–23868 RSC.
- M. F. Matus and H. Häkkinen, Nat. Rev. Mater., 2023, 8, 372–389 CrossRef CAS.
- T.-R. Tero, S. Malola, B. Koncz, E. Pohjolainen, S. Lautala, S. Mustalahti, P. Permi, G. Groenhof, M. Pettersson and H. Häkkinen, ACS Nano, 2017, 11, 11872–11879 CrossRef CAS PubMed.
- S. Malola, P. Nieminen, A. Pihlajamäki, J. Hämäläinen, T. Kärkkäinen and H. Häkkinen, Nat. Commun., 2019, 10, 3973 CrossRef PubMed.
- T. Zheng, Y. Zhang, C. Wu, L. Zhou and P. T. Cummings, Appl. Surf. Sci., 2020, 512, 145713 CrossRef CAS.
- E. Donadoni, P. Siani, G. Frigerio and C. Di Valentin, Nanoscale, 2022, 14, 12099–12116 RSC.
- A. Barzegar, A. Mansouri and J. Azamat, J. Mol. Graphics Modell., 2016, 64, 75–84 CrossRef CAS PubMed.
- A. D. Parab, A. Budi, N. Brljak, M. R. Knecht and T. R. Walsh, Adv. Mater. Interfaces, 2021, 8, 2001659 CrossRef CAS.
- A. Zaboli, H. Raissi, F. Farzad and H. Hashemzadeh, J. Mol. Liq., 2020, 301, 112435 CrossRef CAS.
- K. S. Exner and A. Ivanova, Colloids Surf., B, 2020, 194, 111155 CrossRef CAS PubMed.
- K. S. Exner and A. Ivanova, Phys. Chem. Chem. Phys., 2022, 24, 14985–14992 RSC.
- H. Hashemzadeh and H. Raissi, J. Biomed. Mater. Res. Part A, 2021, 109, 1912–1921 CrossRef CAS PubMed.
- S. Monti, J. Jose, A. Sahajan, N. Kalarikkal and S. Thomas, Phys. Chem. Chem. Phys., 2019, 21, 13099–13108 RSC.
- C. Trouki, G. Barcaro and S. Monti, Nanoscale, 2022, 14, 13123–13131 RSC.
- S. Motta, P. Siani, A. Levy and C. Di Valentin, Nanoscale, 2021, 13, 13000–13013 RSC.
- K. Ahmed, S. N. Inamdar, N. Rohman and A. A. Skelton, Phys. Chem. Chem. Phys., 2021, 23, 2015–2024 RSC.
- A. Haghi, H. Raissi, H. Hashemzadeh and F. Farzad, Comput. Biol. Med., 2022, 143, 105336 CrossRef CAS PubMed.
- X. Wang, R. Lei, L. Li, X. Fei, R. Ju, X. Sun, H. Cao, Q. Zhang, C. Chen and X. Wang, Nanoscale, 2021, 13, 20425–20436 RSC.
- J. Grundler, K. Shin, H. W. Suh, C.-H. Whang, G. Fulgoni, R. W. Pierce and W. M. Saltzman, ACS Nano, 2024, 18, 2815–2827 CrossRef CAS PubMed.
- B. Pelaz, P. del Pino, P. Maffre, R. Hartmann, M. Gallego, S. Rivera-Fernández, J. M. de la Fuente, G. U. Nienhaus and W. J. Parak, ACS Nano, 2015, 9, 6996–7008 CrossRef CAS PubMed.
- J. Toro-Mendoza, L. Maio, M. Gallego, F. Otto, F. Schulz, W. J. Parak, C. Sanchez-Cano and I. Coluzza, ACS Nano, 2023, 17, 955–965 CrossRef CAS PubMed.
- Y. Yu, B. Ghalandari, G. Shen, L. Wang, X. Liu, A. Wang, S. Li, H. Xie and X. Ding, J. Nanobiotechnol., 2021, 19, 445 CrossRef CAS PubMed.
- M. Penna and I. Yarovsky, Nanoscale, 2020, 12, 7240–7255 RSC.
- P. S. Maddahi, M. Yeganeh and F. B. Baghsiyahi, Mater. Chem. Phys., 2019, 237, 121857 CrossRef CAS.
- J. E. Santana, F. De Santiago, M. I. Iturrios, Á. Miranda, L. A. Pérez and M. Cruz-Irisson, Mater. Lett., 2021, 298, 130016 CrossRef CAS.
- K. Al-Khaza'leh, E. A. Almahmoud and J. A. Talla, Chin. J. Phys., 2020, 68, 204–213 CrossRef.
- M. Mashhadbani and E. Faizabadi, Phys. Chem. Chem. Phys., 2023, 25, 3875–3889 RSC.
- A. Tyagi, Y. W. Ng, M. Tamtaji, I. H. Abidi, J. Li, F. Rehman, M. D. Hossain, Y. Cai, Z. Liu, P. R. Galligan, S. Luo, K. Zhang and Z. Luo, ACS Appl. Mater. Interfaces, 2021, 13, 5955–5965 CrossRef CAS PubMed.
- Q. Li, W. Zhao, H. Guo, J. Yang, J. Zhang, M. Liu, T. Xu, Y. Chen and L. Zhang, ACS Appl. Mater. Interfaces, 2020, 12, 25546–25556 CrossRef CAS PubMed.
- S. Kato, K.-i Otake, H. Chen, I. Akpinar, C. T. Buru, T. Islamoglu, R. Q. Snurr and O. K. Farha, J. Am. Chem. Soc., 2019, 141, 2568–2576 CrossRef CAS PubMed.
- Y. Liu, Z. Yuan and Y. Chen, RSC Adv., 2023, 13, 35078–35087 RSC.
- X. Sun, J. Yang, D. Su, C. Wang and G. Wang, Chem. Asian J., 2021, 16, 1949–1955 CrossRef CAS PubMed.
- X. Feng, J. Li, Y. Peng, W. Guo, L. Liang, L. Zhu, S. Liu and L. Ren, Colloids Surf., A, 2024, 680, 132710 CrossRef CAS.
- M. S. de Almeida, E. Susnik, B. Drasler, P. Taladriz-Blanco, A. Petri-Fink and B. Rothen-Rutishauser, Chem. Soc. Rev., 2021, 50, 5397–5434 RSC.
- Q. Dai, N. Bertleff-Zieschang, J. A. Braunger, M. Björnmalm, C. Cortez-Jugo and F. Caruso, Adv. Healthcare Mater., 2018, 7, 1870004 CrossRef.
- G. Farahavar, S. S. Abolmaali, N. Gholijani and F. Nejatollahi, Biomater. Sci., 2019, 7, 4000–4016 RSC.
- X. Zhang, G. Ma and W. Wei, NPG Asia Mater., 2021, 13, 52 CrossRef CAS.
- Y. Li and N. Gu, J. Phys. Chem. B, 2010, 114, 2749–2754 CrossRef CAS PubMed.
- E. L. d Rocha, G. F. Caramori and C. R. Rambo, Phys. Chem. Chem. Phys., 2013, 15, 2282–2290 RSC.
- S. Zhang, H. Gao and G. Bao, ACS Nano, 2015, 9, 8655–8671 CrossRef CAS PubMed.
- T. Lunnoo, J. Assawakhajornsak and T. Puangmali, J. Phys. Chem. C, 2019, 123, 3801–3810 CrossRef CAS.
- T. Lunnoo, J. Assawakhajornsak, S. Ruangchai and T. Puangmali, J. Phys. Chem. B, 2020, 124, 1898–1908 CrossRef CAS PubMed.
- H. I. Ingólfsson, M. N. Melo, F. J. van Eerden, C. Arnarez, C. A. Lopez, T. A. Wassenaar, X. Periole, A. H. de Vries, D. P. Tieleman and S. J. Marrink, J. Am. Chem. Soc., 2014, 136, 14554–14559 CrossRef PubMed.
- F. Lolicato, L. Joly, H. Martinez-Seara, G. Fragneto, E. Scoppola, F. Baldelli Bombelli, I. Vattulainen, J. Akola and M. Maccarini, Small, 2019, 15, 1805046 CrossRef PubMed.
- X. Quan, D. Zhao and J. Zhou, Phys. Chem. Chem. Phys., 2021, 23, 23526–23536 RSC.
- X. Bai, S. M. Lam, P. Nie, M. Xu, S. Liu, G. Shui and G. Hu, Environ. Sci.: Nano, 2022, 9, 4150–4161 RSC.
- S. Yuan, H. Zhang, X. Wang, H. Zhang, Z. Zhang and S. Yuan, Colloids Surf., B, 2022, 210, 112250 CrossRef CAS PubMed.
- Y. Li, M. Kröger and W. K. Liu, Biomaterials, 2014, 35, 8467–8478 CrossRef CAS PubMed.
- Y. Li, X. Zhang and D. Cao, Nanoscale, 2015, 7, 2758–2769 RSC.
- Y. Nademi, T. Tang and H. Uludağ, Nanoscale, 2018, 10, 17671–17682 RSC.
- Z. Zhang, L. Ou, K. Yang and B. Yuan, J. Phys. Chem. B, 2024, 128, 2632–2639 CrossRef CAS PubMed.
- R. Kang, S. Li, Y. Li, X. Zhang and T. Yue, ACS Appl. Nano Mater., 2022, 5, 18337–18348 CrossRef CAS.
- G. Su, X. Zhou, H. Zhou, Y. Li, X. Zhang, Y. Liu, D. Cao and B. Yan, ACS Appl. Mater. Interfaces, 2016, 8, 30037–30047 CrossRef CAS PubMed.
- T. Yue, H. Zhou, H. Sun, S. Li, X. Zhang, D. Cao, X. Yi and B. Yan, Nanoscale, 2019, 11, 6602–6609 RSC.
- E. Donadoni, G. Frigerio, P. Siani, S. Motta, J. Vertemara, L. De Gioia, L. Bonati and C. Di Valentin, ACS Biomater. Sci. Eng., 2023, 9, 6123–6137 CrossRef CAS PubMed.
- P. Zhang, J. Zhai, X. Gao, H. Zhao, W. Su and L. Zhao, Sci. Bull., 2018, 63, 349–355 CrossRef CAS PubMed.
- Y. Wei, H. Chen, Y.-X. Li, K. He, K. Yang and H.-B. Pang, ACS Nano, 2022, 16, 5885–5897 CrossRef CAS PubMed.
- M. Feng, D. R. Bell and R. Zhou, J. Chem. Phys., 2017, 147, 225101 CrossRef PubMed.
- S. Lei, X. Chen, Y. Gao, M. Shuai, W. Zhou, J. Li, J. Wu, K. Men and X. Duan, Adv. Funct. Mater., 2022, 32, 2204342 CrossRef CAS.
- Y. Wang, F. Jia, Z. Wang, Y. Qian, L. Fan, H. Gong, A. Luo, J. Sun, Z. Hu and W. Wang, Anal. Chem., 2019, 91, 7245–7253 CrossRef CAS PubMed.
- L. Ma, M. Niu, Y. Ji, L. Liu, X. Gu, J. Luo, G. Wei and M. Yan, Colloids Surf., B, 2023, 223, 113186 CrossRef CAS PubMed.
- J. Lehtinen, A. Magarkar, M. Stepniewski, S. Hakola, M. Bergman, T. Róg, M. Yliperttula, A. Urtti and A. Bunker, Eur. J. Pharm. Sci., 2012, 46, 121–130 CrossRef CAS PubMed.
- S. Meng, B. Su, W. Li, Y. Ding, L. Tang, W. Zhou, Y. Song and Z. Caicun, Med. Oncol., 2011, 28, 1180–1187 CrossRef CAS PubMed.
- A. Wang, K. Yue, W. Zhong, G. Zhang, L. Wang, H. Wang, H. Zhang and X. Zhang, Int. J. Biol. Macromol., 2023, 227, 193–202 CrossRef CAS PubMed.
- A. Hosseini, S. Soleimani, H. P. Modarres, S. H. Emami, M. Tondar, G. Bahlakeh and M. M. Hasani-Sadrabadi, J. Mater. Chem. B, 2016, 4, 768–778 RSC.
- T. W. Kang, I.-J. Hwang, S. Lee, S.-J. Jeon, C. Choi, J. Han, Y. So, W. Son, H. Kim, C.-S. Yang, J.-H. Park, H. Lee and J.-H. Kim, Adv. Mater., 2021, 33, 2101376 CrossRef CAS PubMed.
- S. Lee, T. W. Kang, I.-J. Hwang, H.-I. Kim, S.-J. Jeon, D. Yim, C. Choi, W. Son, H. Kim, C.-S. Yang, H. Lee and J.-H. Kim, J. Am. Chem. Soc., 2021, 143, 14635–14645 CrossRef CAS PubMed.
- G.-H. Yan, K. Wang, Z. Shao, L. Luo, Z.-M. Song, J. Chen, R. Jin, X. Deng, H. Wang, Z. Cao, Y. Liu and A. Cao, Proc. Natl. Acad. Sci. U. S. A., 2018, 115, E34–E43 CAS.
- R. Vácha, F. J. Martinez-Veracoechea and D. Frenkel, Nano Lett., 2011, 11, 5391–5395 CrossRef PubMed.
- V. Schubertova, F. J. Martinez-Veracoechea and R. Vácha, Soft Matter, 2015, 11, 2726–2730 RSC.
- J. Mao, P. Chen, J. Liang, R. Guo and L.-T. Yan, ACS Nano, 2016, 10, 1493–1502 CrossRef CAS PubMed.
- C. Huang, Y. Zhang, H. Yuan, H. Gao and S. Zhang, Nano Lett., 2013, 13, 4546–4550 CrossRef CAS PubMed.
- Y. Li, M. Kroeger and W. K. Liu, Nanoscale, 2015, 7, 16631–16646 RSC.
- Z. Shen, H. Ye and Y. Li, Phys. Chem. Chem. Phys., 2018, 20, 16372–16385 RSC.
- H. Tang, H. Zhang, H. Ye and Y. Zheng, J. Phys. Chem. B, 2018, 122, 171–180 CrossRef CAS PubMed.
- H. Deng, P. Dutta and J. Liu, Soft Matter, 2019, 15, 5128–5137 RSC.
- H.-M. Ding and Y.-Q. Ma, Biomaterials, 2012, 33, 5798–5802 CrossRef CAS PubMed.
- H. Deng, P. Dutta and J. Liu, Nanoscale, 2019, 11, 11227–11235 RSC.
- M. M. Billah, H. Deng, P. Dutta and J. Liu, Soft Matter, 2023, 19, 5907–5915 RSC.
- B. Yang, Y. Chen and J. Shi, Chem. Rev., 2019, 119, 4881–4985 CrossRef CAS PubMed.
- Y. Huang, J. Ren and X. Qu, Chem. Rev., 2019, 119, 4357–4412 CrossRef CAS PubMed.
- Y. Wu, W. Chen, C. Wang and D. Xing, Biosens. Bioelectron., 2023, 237, 115470 CrossRef CAS PubMed.
- Z. Wang, A. Jin, Z. Yang and W. Huang, ACS Nano, 2023, 17, 8935–8965 CrossRef CAS PubMed.
- C. Wang, G. Tian, X. Yu and X. Zhang, Small, 2023, 19, 2207261 CrossRef CAS PubMed.
- J. Ling, Y. Chang, Z. Yuan, Q. Chen, L. He and T. Chen, ACS Appl. Mater. Interfaces, 2022, 14, 27651–27665 CrossRef CAS PubMed.
- M. Gao, Z. Wang, H. Zheng, L. Wang, S. Xu, X. Liu, W. Li, Y. Pan, W. Wang, X. Cai, R. A. Wu, X. Gao and R. Li, Angew. Chem., Int. Ed., 2020, 59, 3618–3623 CrossRef CAS PubMed.
- M. Liang and X. Yan, Acc. Chem. Res., 2019, 52, 2190–2200 CrossRef CAS PubMed.
- Z. Sun, C. Zhou, Y. Zhou, S. Su, C. Wang and M. Zhen, Adv. Healthcare Mater., 2023, 12, 2301306 CrossRef CAS PubMed.
- N. Singh, G. R. Sherin and G. Mugesh, Angew. Chem., Int. Ed., 2023, 62, e202301232 CrossRef CAS PubMed.
- C. Cao, N. Yang, X. Wang, J. Shao, X. Song, C. Liang, W. Wang and X. Dong, Coord. Chem. Rev., 2023, 491, 215245 CrossRef CAS.
- M. Gao, X. Liu, Z. Wang, H. Wang, T. Asset, D. Wu, J. Jiang, Q. Xie, S. Xu, X. Cai, J. Li, W. Wang, H. Zheng, X. Gao, N. Tarasenko, B. Rotonnelli, J.-J. Gallet, F. Jaouen and R. Li, Nano Today, 2022, 44, 101456 CrossRef CAS.
- H. Hu, X. Kang, Z. Shan, X. Yang, W. Bing, L. Wu, H. Ge and H. Ji, Nanoscale, 2022, 14, 2676–2685 RSC.
- Y. Xiong, L. Su, F. Ye and S. Zhao, Mater. Today Chem., 2022, 23, 100672 CrossRef CAS.
- Q.-Z. Li, H. Fan, Z. Wang, J.-J. Zheng, K. Fan, X. Yan and X. Gao, ACS Catal., 2023, 13, 504–514 CrossRef CAS.
- S. Li, Z. Zhou, Z. Tie, B. Wang, M. Ye, L. Du, R. Cui, W. Liu, C. Wan, Q. Liu, S. Zhao, Q. Wang, Y. Zhang, S. Zhang, H. Zhang, Y. Du and H. Wei, Nat. Commun., 2022, 13, 827 CrossRef CAS PubMed.
- T. G. Grissom, A. M. Plonka, C. H. Sharp, A. M. Ebrahim, Y. Tian, D. L. Collins-Wildman, A. L. Kaledin, H. J. Siegal, D. Troya, C. L. Hill, A. I. Frenkel, D. G. Musaev, W. O. Gordon, C. J. Karwacki, M. B. Mitchell and J. R. Morris, ACS Appl. Mater. Interfaces, 2020, 12, 14641–14661 CrossRef CAS PubMed.
- I. Vitale, G. Manic, L. M. Coussens, G. Kroemer and L. Galluzzi, Cell Metab., 2019, 30, 36–50 CrossRef CAS PubMed.
- J. Li, W. Liu, X. Wu and X. Gao, Biomaterials, 2015, 48, 37–44 CrossRef CAS PubMed.
- H. Chen, P. Liao, M. L. Mendonca and R. Q. Snurr, J. Phys. Chem. C, 2018, 122, 12362–12368 CrossRef CAS.
- Y. Chen, X. Shen, U. Carmona, F. Yang, X. Gao, M. Knez, L. Zhang and Y. Qin, Adv. Mater. Interfaces, 2021, 8, 2100086 CrossRef CAS.
- Y. Liang, C. Liao, X. Guo, G. Li, X. Yang, J. Yu, J. Zhong, Y. Xie, L. Zheng and J. Zhao, Small, 2023, 19, 2205511 CrossRef CAS PubMed.
- S. Guo and L. Guo, J. Phys. Chem. C, 2019, 123, 30318–30334 CrossRef CAS.
- X. Shen, Z. Wang, X. Gao and Y. Zhao, ACS Catal., 2020, 10, 12657–12665 CrossRef CAS.
- Z. Wang, X. Shen and X. Gao, J. Phys. Chem. C, 2021, 125, 23098–23104 CrossRef CAS.
- A. Adhikari, S. Mondal, M. Das, P. Biswas, U. Pal, S. Darbar, S. S. Bhattacharya, D. Pal, T. Saha-Dasgupta, A. K. Das, A. K. Mallick and S. K. Pal, Adv. Healthcare Mater., 2021, 10, 2001736 CrossRef CAS PubMed.
- X. Jiao, H. Song, H. Zhao, W. Bai, L. Zhang and Y. Lv, Anal. Methods, 2012, 4, 3261–3267 RSC.
- M. Chen, X. Zhou, C. Xiong, T. Yuan, W. Wang, Y. Zhao, Z. Xue, W. Guo, Q. Wang, H. Wang, Y. Li, H. Zhou and Y. Wu, ACS Appl. Mater. Interfaces, 2022, 14, 21989–21995 CrossRef CAS PubMed.
- D. Wang, X. Song, P. Li, X. J. Gao and X. Gao, J. Mater. Chem. B, 2020, 8, 9028–9034 RSC.
- H. Sun, A. Zhao, N. Gao, K. Li, J. Ren and X. Qu, Angew. Chem., Int. Ed., 2015, 54, 7176–7180 CrossRef CAS PubMed.
- Y. Hu, X. J. Gao, Y. Zhu, F. Muhammad, S. Tan, W. Cao, S. Lin, Z. Jin, X. Gao and H. Wei, Chem. Mater., 2018, 30, 6431–6439 CrossRef CAS.
- Q. Liang, J. Xi, X. J. Gao, R. Zhang, Y. Yang, X. Gao, X. Yan, L. Gao and K. Fan, Nano Today, 2020, 35, 100935 CrossRef CAS.
- X. Chen, L. Zhao, K. Wu, H. Yang, Q. Zhou, Y. Xu, Y. Zheng, Y. Shen, S. Liu and Y. Zhang, Chem. Sci., 2021, 12, 8865–8871 RSC.
- S. Ji, B. Jiang, H. Hao, Y. Chen, J. Dong, Y. Mao, Z. Zhang, R. Gao, W. Chen, R. Zhang, Q. Liang, H. Li, S. Liu, Y. Wang, Q. Zhang, L. Gu, D. Duan, M. Liang, D. Wang, X. Yan and Y. Li, Nat. Catal., 2021, 4, 407–417 CrossRef CAS.
- J. Liang, B. Johannessen, Z. Wu, R. F. Webster, J. Yong, M. Y. Bin Zulkifli, J. S. Harbort, Y. R. Cheok, H. Wen, Z. Ao, B. Kong, S. L. Y. Chang, J. Scott and K. Liang, Adv. Mater., 2022, 34, 2205674 CrossRef CAS PubMed.
- Y. Chen, P. Wang, H. Hao, J. Hong, H. Li, S. Ji, A. Li, R. Gao, J. Dong, X. Han, M. Liang, D. Wang and Y. Li, J. Am. Chem. Soc., 2021, 143, 18643–18651 CrossRef CAS PubMed.
- X. Shen, W. Liu, X. Gao, Z. Lu, X. Wu and X. Gao, J. Am. Chem. Soc., 2015, 137, 15882–15891 CrossRef CAS PubMed.
- G. Fang, W. Li, X. Shen, J. M. Perez-Aguilar, Y. Chong, X. Gao, Z. Chai, C. Chen, C. Ge and R. Zhou, Nat. Commun., 2018, 9, 129 CrossRef PubMed.
- Y. Wang, Z. Zhang, G. Jia, L. Zheng, J. Zhao and X. Cui, Chem. Commun., 2019, 55, 5271–5274 RSC.
- X. Yuan, L. Wang, M. Hu, L. Zhang, H. Chen, D. Zhang, Z. Wang, T. Li, M. Zhong, L. Xu, D. Wang, Y. Liu and W. Tan, Angew. Chem., Int. Ed., 2021, 60, 20943–20951 CrossRef CAS PubMed.
- T. Wu, J. Sun, J. Lei, Q. Fan, X. Tang, G. Zhu, Q. Yan, X. Feng and B. Shi, Nanoscale, 2021, 13, 17912–17919 RSC.
- Y. Yu, Y. Cheng, L. Tan, X. Liu, Z. Li, Y. Zheng, T. Wu, Y. Liang, Z. Cui, S. Zhu and S. Wu, Chem. Eng. J., 2022, 431, 133279 CrossRef CAS.
- P. Cheng, H. Wang and X. Shi, Nanoscale, 2020, 12, 3050–3057 RSC.
- J. Wu, Z. Wang, X. Jin, S. Zhang, T. Li, Y. Zhang, H. Xing, Y. Yu, H. Zhang, X. Gao and H. Wei, Adv. Mater., 2021, 33, 2005024 CrossRef CAS PubMed.
- M. Comotti, C. Della Pina, R. Matarrese and M. Rossi, Angew. Chem., Int. Ed., 2004, 43, 5812–5815 CrossRef CAS PubMed.
- H. Cheng, S. Lin, F. Muhammad, Y.-W. Lin and H. Wei, ACS Sens., 2016, 1, 1336–1343 CrossRef CAS.
- W. He, Y.-T. Zhou, W. G. Wamer, X. Hu, X. Wu, Z. Zheng, M. D. Boudreau and J.-J. Yin, Biomaterials, 2013, 34, 765–773 CrossRef CAS PubMed.
- S. Shibuya, Y. Ozawa, K. Watanabe, N. Izuo, T. Toda, K. Yokote and T. Shimizu, PLoS One, 2014, 9, e109288 CrossRef PubMed.
- Z. Wang, X. Shen, X. Gao and Y. Zhao, Nanoscale, 2019, 11, 13289–13299 RSC.
- Z. Wang, J. Wu, J.-J. Zheng, X. Shen, L. Yan, H. Wei, X. Gao and Y. Zhao, Nat. Commun., 2021, 12, 6866 CrossRef CAS PubMed.
- W. Gao, J. He, L. Chen, X. Meng, Y. Ma, L. Cheng, K. Tu, X. Gao, C. Liu, M. Zhang, K. Fan, D.-W. Pang and X. Yan, Nat. Commun., 2023, 14, 160 CrossRef CAS PubMed.
- C. Korsvik, S. Patil, S. Seal and W. T. Self, Chem. Commun., 2007, 1056–1058 RSC.
- J. Mu, X. Zhao, J. Li, E.-C. Yang and X.-J. Zhao, J. Mater. Chem. B, 2016, 4, 5217–5221 RSC.
- T. Chen, H. Zou, X. Wu, C. Liu, B. Situ, L. Zheng and G. Yang, ACS Appl. Mater. Interfaces, 2018, 10, 12453–12462 CrossRef CAS PubMed.
- S. Osuna, M. Swart and M. Solà, Chem. – Eur. J., 2010, 16, 3207–3214 CrossRef CAS PubMed.
- Z. Wang, X. Gao and Y. Zhao, J. Phys. Chem. A, 2018, 122, 8183–8190 CrossRef CAS PubMed.
- J. Liu, L. Wang, X. Shen, X. Gao, Y. Chen, H. Liu, Y. Liu, D. Yin, Y. Liu, W. Xu, R. Cai, M. You, M. Guo, Y. Wang, J. Li, Y. Li and C. Chen, Nano Today, 2020, 34, 100907 CrossRef CAS.
- R. Zhang, L. Chen, Q. Liang, J. Xi, H. Zhao, Y. Jin, X. Gao, X. Yan, L. Gao and K. Fan, Nano Today, 2021, 41, 101317 CrossRef CAS.
- Z. Wang, W. Wang, J. Wang, D. Wang, M. Liu, Q. Wu and H. Hu, Adv. Funct. Mater., 2023, 33, 2209560 CrossRef CAS.
- J. Zhou, D. Xu, G. Tian, Q. He, X. Zhang, J. Liao, L. Mei, L. Chen, L. Gao, L. Zhao, G. Yang, W. Yin, G. Nie and Y. Zhao, J. Am. Chem. Soc., 2023, 145, 4279–4293 CrossRef CAS PubMed.
- T. Pirmohamed, J. M. Dowding, S. Singh, B. Wasserman, E. Heckert, A. S. Karakoti, J. E. S. King, S. Seal and W. T. Self, Chem. Commun., 2010, 46, 2736–2738 RSC.
- Y. Chen, B. Jiang, H. Hao, H. Li, C. Qiu, X. Liang, Q. Qu, Z. Zhang, R. Gao, D. Duan, S. Ji, D. Wang and M. Liang, Angew. Chem., Int. Ed., 2023, 62, e202301879 CrossRef CAS PubMed.
- R. Tsyshevsky, S. Holdren, B. W. Eichhorn, M. R. Zachariah and M. M. Kuklja, J. Phys. Chem. C, 2019, 123, 26432–26441 CrossRef CAS.
- S. Holdren, R. Tsyshevsky, K. Fears, J. Owrutsky, T. Wu, X. Wang, B. W. Eichhorn, M. M. Kuklja and M. R. Zachariah, ACS Catal., 2019, 9, 902–911 CrossRef CAS.
- D. Ma and Z. Cao, J. Phys. Chem. C, 2021, 125, 24396–24405 CrossRef CAS.
- X. Hu, T. Huang, H. Liao, L. Hu and M. Wang, J. Mater. Chem. B, 2020, 8, 4428–4433 RSC.
- C. Zhao and Y. Xu, Catal. Today, 2018, 312, 141–148 CrossRef CAS.
- T. Li, R. Tsyshevsky, L. Algrim, M. McEntee, E. M. Durke, B. Eichhorn, C. Karwacki, M. R. Zachariah, M. M. Kuklja and E. E. Rodriguez, ACS Appl. Mater. Interfaces, 2021, 13, 54597–54609 CrossRef CAS PubMed.
- M. J. Manto, P. Xie and C. Wang, ACS Catal., 2017, 7, 1931–1938 CrossRef CAS.
- M. R. Momeni and C. J. Cramer, ACS Appl. Mater. Interfaces, 2018, 10, 18435–18439 CrossRef CAS PubMed.
- D. Troya, J. Phys. Chem. C, 2016, 120, 29312–29323 CrossRef CAS.
- M. R. Momeni and C. J. Cramer, Chem. Mater., 2018, 30, 4432–4439 CrossRef CAS.
- M. L. Mendonca and R. Q. Snurr, ACS Catal., 2020, 10, 1310–1323 CrossRef CAS.
- D. Ma and Z. Cao, J. Phys. Chem. C, 2022, 126, 19159–19168 CrossRef CAS.
- S.-Y. Moon, Y. Liu, J. T. Hupp and O. K. Farha, Angew. Chem., Int. Ed., 2015, 54, 6795–6799 CrossRef CAS PubMed.
- H. Chen and R. Q. Snurr, ACS Appl. Mater. Interfaces, 2020, 12, 14631–14640 CrossRef CAS PubMed.
- J. E. Mondloch, M. J. Katz, W. C. Isley, III, P. Ghosh, P. Liao, W. Bury, G. Wagner, M. G. Hall, J. B. DeCoste, G. W. Peterson, R. Q. Snurr, C. J. Cramer, J. T. Hupp and O. K. Farha, Nat. Mater., 2015, 14, 512–516 CrossRef CAS PubMed.
- Z. Wang, H. Meng, X. J. Gao, J.-J. Zheng and X. Gao, npj Comput. Mater., 2023, 9, 59 CrossRef CAS.
- M. R. Mian, X. Wang, X. Wang, K. O. Kirlikovali, H. Xie, K. Ma, K. M. Fahy, H. Chen, T. Islamoglu, R. Q. Snurr and O. K. Farha, J. Am. Chem. Soc., 2023, 145, 7435–7445 CrossRef CAS PubMed.
- Q. Wang, R. C. Chapleski, Jr., A. M. Plonka, W. O. Gordon, W. Guo, N.-P. Thuy-Duong, C. H. Sharp, N. S. Marinkovic, S. D. Senanayake, J. R. Morris, C. L. Hill, D. Troya and A. I. Frenkel, Sci. Rep., 2017, 7, 773 CrossRef PubMed.
- Y. Tian, A. M. Plonka, A. M. Ebrahim, R. M. Palomino, S. D. Senanayake, A. Balboa, W. O. Gordon, D. Troya, D. G. Musaev, J. R. Morris, M. B. Mitchell, D. L. Collins-Wildman, C. L. Hill and A. I. Frenkel, J. Phys. Chem. Lett., 2019, 10, 2295–2299 CrossRef CAS PubMed.
- B. J. G. Rousseau, A. V. Soudackov, R. R. Tuttle, M. M. Reynolds, R. G. Finke and S. Hammes-Schiffer, J. Am. Chem. Soc., 2023, 145, 10285–10294 CrossRef CAS PubMed.
- X. Wang, X. J. Gao, L. Qin, C. Wang, L. Song, Y.-N. Zhou, G. Zhu, W. Cao, S. Lin, L. Zhou, K. Wang, H. Zhang, Z. Jin, P. Wang, X. Gao and H. Wei, Nat. Commun., 2019, 10, 704 CrossRef CAS PubMed.
- Q. Wang, C. Li, X. Wang, J. Pu, S. Zhang, L. Liang, L. Chen, R. Liu, W. Zuo, H. Zhang, Y. Tao, X. Gao and H. Wei, Nano Lett., 2022, 22, 10003–10009 CrossRef CAS PubMed.
- T. Puzyn, B. Rasulev, A. Gajewicz, X. Hu, T. P. Dasari, A. Michalkova, H.-M. Hwang, A. Toropov, D. Leszczynska and J. Leszczynski, Nat. Nanotechnol., 2011, 6, 175–178 CrossRef CAS PubMed.
- A. B. Cook and P. Decuzzi, ACS Nano, 2021, 15, 2068–2098 CrossRef CAS PubMed.
- Z. Shen, W. Baker, H. Ye and Y. Li, Nanoscale, 2019, 11, 7371–7385 RSC.
- Y. Min, M. Akbulut, K. Kristiansen, Y. Golan and J. Israelachvili, Nat. Mater., 2008, 7, 527–538 CrossRef CAS PubMed.
- D. Gentili and G. Ori, Nanoscale, 2022, 14, 14385–14432 RSC.
- G. A. DeVries, M. Brunnbauer, Y. Hu, A. M. Jackson, B. Long, B. T. Neltner, O. Uzun, B. H. Wunsch and F. Stellacci, Science, 2007, 315, 358–361 CrossRef CAS PubMed.
- D. B. Sridhar, R. Gupta and B. Rai, Phys. Chem. Chem. Phys., 2018, 20, 25883–25891 RSC.
- R. P. Pothukuchi, V. K. Prajapat and M. Radhakrishna, Langmuir, 2021, 37, 12007–12015 CrossRef CAS PubMed.
- J. Huang, D. Buratto and R. Zhou, Aggregate, 2023, 4, e324 CrossRef CAS.
- C. Lionello, C. Perego, A. Gardin, R. Klajn and G. M. Pavan, ACS Nano, 2023, 17, 275–287 CrossRef CAS PubMed.
- T. Bian, A. Gardin, J. Gemen, L. Houben, C. Perego, B. Lee, N. Elad, Z. Chu, G. M. Pavan and R. Klajn, Nat. Chem., 2021, 13, 940–949 CrossRef CAS PubMed.
- H. Xiong, D. van der Lelie and O. Gang, J. Am. Chem. Soc., 2008, 130, 2442–2443 CrossRef CAS PubMed.
- E. Lavagna, D. Bochicchio, A. L. De Marco, Z. P. Güven, F. Stellacci and G. Rossi, Nanoscale, 2022, 14, 6912–6921 RSC.
- E. Petretto, P. Campomanes and S. Vanni, Soft Matter, 2023, 19, 3290–3300 RSC.
- E. Canepa, S. Salassi, A. L. de Marco, C. Lambruschini, D. Odino, D. Bochicchio, F. Canepa, C. Canale, S. Dante, R. Brescia, F. Stellacci, G. Rossi and A. Relini, Nanoscale, 2020, 12, 19746–19759 RSC.
- B. Fang, X. Dai, B. Li, Y. Qu, Y.-Q. Li, M. Zhao, Y. Yang and W. Li, Nanoscale Adv., 2022, 4, 163–172 RSC.
- Y. Wang, B. Z. Chen, Y. J. Liu, Z. M. Wu and X. D. Guo, Colloids Surf., B, 2017, 151, 280–286 CrossRef CAS PubMed.
- S.-D. Mousavi, F. Maghsoodi, F. Panahandeh, R. Yazdian-Robati, A. Reisi-Vanani and M. Tafaghodi, Mater. Sci. Eng., C, 2018, 92, 631–643 CrossRef CAS PubMed.
- T. Tian, D. Xiao, T. Zhang, Y. Li, S. Shi, W. Zhong, P. Gong, Z. Liu, Q. Li and Y. Lin, Adv. Funct. Mater., 2021, 31, 2007342 CrossRef CAS.
- G. Forte, G. Consiglio, C. Satriano, L. Maugeri and S. Petralia, Colloids Surf., B, 2022, 217, 112628 CrossRef CAS PubMed.
- D. Liu, Z. Zhou, X. Wang, H. Deng, L. Sun, H. Lin, F. Kang, Y. Zhang, Z. Wang, W. Yang, L. Rao, K. Yang, G. Yu, J. Du, Z. Shen and X. Chen, Biomaterials, 2020, 244, 119979 CrossRef CAS PubMed.
- L. Xu, Y. Wang, C. Zhu, S. Ren, Y. Shao, L. Wu, W. Li, X. Jia, R. Hu, R. Chen and Z. Chen, Theranostics, 2020, 10, 8162–8178 CrossRef CAS PubMed.
- W. Zhong, K. Yue, A. Wang, G. Zhang, J. Wang, L. Wang, H. Wang, H. Zhang and X. Zhang, Colloids Surf., B, 2022, 219, 112836 CrossRef CAS PubMed.
- Z. M. Wu, L. Ling, L. Y. Zhou, X. D. Guo, W. Jiang, Y. Qian, K. Q. Luo and L. J. Zhang, Nanoscale Res. Lett., 2012, 7, 299 CrossRef PubMed.
- Y. Li, X. Cai, Z. Wang, Y. Han, C. Ren, L. Yang, Z. Wang, G. Mu, H. Jia, J. Liu, J. Liu and C. Yang, Chem. Eng. J., 2023, 455, 140848 CrossRef CAS.
- A. D’Souza, L. R. Marshall, J. Yoon, A. Kulesha, D. I. U. Edirisinghe, S. Chandrasekaran, P. Rathee, R. Prabhakar and O. V. Makhlynets, Nano Convergence, 2022, 9, 18 CrossRef PubMed.
- C. Ma, Z. Yang, W. Wang, M. Zhang, X. Hao, S. Zhu and S. Chen, J. Mater. Chem. C, 2020, 8, 2888–2898 RSC.
- C. Jin, X. Liu, L. Tan, Z. Cui, X. Yang, Y. Zheng, K. W. K. Yeung, P. K. Chu and S. Wu, Biomater. Sci., 2018, 6, 1735–1744 RSC.
- Y. Chang, Y. Cheng, Y. Feng, K. Li, H. Jian and H. Zhang, ACS Appl. Mater. Interfaces, 2019, 11, 12224–12231 CrossRef CAS PubMed.
- F. J. Geissel, V. Platania, N. DeBerardinis, C. Skjöldebrand, G. N. Belibasakis, C. Persson, G. Hulsart-Billström, M. Chatzinikolaidou and G. A. Sotiriou, Adv. Mater. Interfaces, 2023, 10, 2201980 CrossRef CAS.
- Z. Zheng, Q. Chen, S. Rong, R. Dai, Z. Jia, X. Peng and R. Zhang, Nanoscale, 2020, 12, 15845–15856 RSC.
- J. Hu, Z. Zou, F. Mo, X. Lin, Y. Zhao, T. Shi, F. Wang and X. Liu, Chem. Eng. J., 2023, 452, 139323 CrossRef CAS.
- W. Xie, J. Ye, Z. Guo, J. Lu, W. Xu, X. Gao, H. Huang, R. Hu, L. Mao, Y. Wei and L. Zhao, Chem. Eng. J., 2022, 438, 135372 CrossRef CAS.
- X. Qi, G. Wang, P. Wang, Y. Pei, C. Zhang, M. Yan, P. Wei, G. Tian and G. Zhang, ACS Appl. Mater. Interfaces, 2022, 14, 7659–7670 CrossRef CAS PubMed.
- X. He, X. Zhang, J. Li, R. Hang, X. Huang, X. Yao, L. Qin and B. Tang, J. Mater. Chem. B, 2018, 6, 5100–5114 RSC.
- X. Hu, S. Cook, P. Wang and H.-M. Hwang, Sci. Total Environ., 2009, 407, 3070–3072 CrossRef CAS PubMed.
- J. Burk, L. Sikk, P. Burk, B. B. Manshian, S. J. Soenen, J. J. Scott-Fordsmand, T. Tamm and K. Tämm, Nanoscale, 2018, 10, 21985–21993 RSC.
- C. Martín, K. Kostarelos, M. Prato and A. Bianco, Chem. Commun., 2019, 55, 5540–5546 RSC.
- Z. Peng, X. Liu, W. Zhang, Z. Zeng, Z. Liu, C. Zhang, Y. Liu, B. Shao, Q. Liang, W. Tang and X. Yuan, Environ. Int., 2020, 134, 105298 CrossRef CAS PubMed.
- R. Kurapati, S. P. Mukherjee, C. Martín, G. Bepete, E. Vázquez, A. Pénicaud, B. Fadeel and A. Bianco, Angew. Chem., Int. Ed., 2018, 57, 11722–11727 CrossRef CAS PubMed.
- C. Martín, G. Jun, R. Schurhammer, G. Reina, P. Chen, A. Bianco and C. Ménard-Moyon, Small, 2019, 15, 1905405 CrossRef PubMed.
- I. Srivastava, D. Sar, P. Mukherjee, A. S. Schwartz-Duval, Z. Huang, C. Jaramillo, A. Civantos, I. Tripathi, J. P. Allain, R. Bhargava and D. Pan, Nanoscale, 2019, 11, 8226–8236 RSC.
- L. Dong, K. Li, D. Wen, Y. Lu, K. Du, M. Zhang, X. Gao, J. Feng and H. Zhang, Nanoscale, 2019, 11, 12853–12857 RSC.
- H. Lin, W. Qiu, J. Liu, L. Yu, S. Gao, H. Yao, Y. Chen and J. Shi, Adv. Mater., 2019, 31, 1903013 CrossRef PubMed.
- N. Ouassil, R. L. Pinals, J. T. Del Bonis-O’Donnell, J. W. Wang and M. P. Landry, Sci. Adv., 2022, 8, eabm0898 CrossRef CAS PubMed.
- J. Razlivina, A. Dmitrenko and V. Vinogradov, J. Phys. Chem. Lett., 2024, 15, 5804–5813 CrossRef CAS PubMed.
- J. Razlivina, N. Serov, O. Shapovalova and V. Vinogradov, Small, 2022, 18, 2105673 CrossRef CAS PubMed.
- Y. Wei, J. Wu, Y. Wu, H. Liu, F. Meng, Q. Liu, A. C. Midgley, X. Zhang, T. Qi, H. Kang, R. Chen, D. Kong, J. Zhuang, X. Yan and X. Huang, Adv. Mater., 2022, 34, 2201736 CrossRef CAS PubMed.
- Y. Yu, Y. Jiang, C. Zhang, Q. Bai, F. Fu, S. Li, L. Wang, W. W. Yu, N. Sui and Z. Zhu, ACS Mater. Lett., 2022, 4, 2134–2142 CrossRef CAS.
- C. Zhang, Y. Yu, S. Shi, M. Liang, D. Yang, N. Sui, W. W. Yu, L. Wang and Z. Zhu, Nano Lett., 2022, 22, 8592–8600 CrossRef CAS PubMed.
- A. A. Metwally and R. M. Hathout, Mol. Pharmaceutics, 2015, 12, 2800–2810 CrossRef CAS PubMed.
- M. R. Kibria, R. I. Akbar, P. Nidadavolu, O. Havryliuk, S. Lafond and S. Azimi, Sci. Rep., 2023, 13, 547 CrossRef CAS PubMed.
- D. Reker, Y. Rybakova, A. R. Kirtane, R. Cao, J. W. Yang, N. Navamajiti, A. Gardner, R. M. Zhang, T. Esfandiary, J. L’Heureux, T. von Erlach, E. M. Smekalova, D. Leboeuf, K. Hess, A. Lopes, J. Rogner, J. Collins, S. M. Tamang, K. Ishida, P. Chamberlain, D. Yun, A. Lytton-Jean, C. K. Soule, J. H. Cheah, A. M. Hayward, R. Langer and G. Traverso, Nat. Nanotechnol., 2021, 16, 725–733 CrossRef CAS PubMed.
- P. Bannigan, Z. Bao, R. J. Hickman, M. Aldeghi, F. Häse, A. Aspuru-Guzik and C. Allen, Nat. Commun., 2023, 14, 35 CrossRef CAS PubMed.
- Y. Liu, D. Zhang, Y. Tang, Y. Zhang, X. Gong, S. Xie and J. Zheng, Chem. Eng. J., 2021, 420, 129872 CrossRef CAS.
- Y. Liu, D. Zhang, Y. Tang, Y. Zhang, Y. Chang and J. Zheng, ACS Appl. Mater. Interfaces, 2021, 13, 11306–11319 CrossRef CAS PubMed.
- D. Palai, H. Tahara, S. Chikami, G. V. Latag, S. Maeda, C. Komura, H. Kurioka and T. Hayashi, ACS Biomater. Sci. Eng., 2022, 8, 3765–3772 CrossRef CAS PubMed.
- R. J. Kwaria, E. A. Q. Mondarte, H. Tahara, R. Chang and T. Hayashi, ACS Biomater. Sci. Eng., 2020, 6, 4949–4956 CrossRef CAS PubMed.
- J. Chen, E. Xu, Y. Wei, M. Chen, T. Wei and S. Zheng, Langmuir, 2022, 38, 10817–10825 CrossRef CAS PubMed.
- S. Zheng, Y. Wei, Y. Lin and T. Wei, Appl. Phys. Lett., 2023, 122, 253701 CrossRef CAS.
- M. Cha, E. S. T. Emre, X. Xiao, J.-Y. Kim, P. Bogdan, J. S. VanEpps, A. Violi and N. A. Kotov, Nat. Comput. Sci., 2022, 2, 243–252 CrossRef PubMed.
- Z. E. Hughes, M. A. Nguyen, J. Wang, Y. Liu, M. T. Swihart, M. Poloczek, P. I. Frazier, M. R. Knecht and T. R. Walsh, ACS Nano, 2021, 15, 18260–18269 CrossRef CAS PubMed.
- M. Tanaka, Y. Takahashi, L. Roach, K. Critchley, S. D. Evans and M. Okochi, Nanoscale Adv., 2019, 1, 71–75 RSC.
- Kenry, Adv. Theor. Simul., 2023, 6, 2300122 CrossRef CAS.
- A. V. Singh, R.-S. Maharjan, A. Kanase, K. Siewert, D. Rosenkranz, R. Singh, P. Laux and A. Luch, ACS Appl. Mater. Interfaces, 2021, 13, 1943–1955 CrossRef CAS PubMed.
- D. Gong, E. Ben-Akiva, A. Singh, H. Yamagata, S. Est-Witte, J. K. Shade, N. A. Trayanova and J. J. Green, Acta Biomater., 2022, 154, 349–358 CrossRef CAS PubMed.
- M. Alafeef, I. Srivastava and D. Pan, ACS Sens., 2020, 5, 1689–1698 CrossRef CAS PubMed.
- T. C. Le, B. Yan and D. A. Winkler, Adv. Funct. Mater., 2015, 25, 6927–6935 CrossRef CAS.
- M. Chandler, S. Jain, J. Halman, E. Hong, M. A. Dobrovolskaia, A. V. Zakharov and K. A. Afonin, Small, 2022, 18, 2204941 CrossRef CAS PubMed.
- G. Yamankurt, E. J. Berns, A. Xue, A. Lee, N. Bagheri, M. Mrksich and C. A. Mirkin, Nat. Biomed. Eng., 2019, 3, 318–327 CrossRef CAS PubMed.
- B. Jiang, D. Duan, L. Gao, M. Zhou, K. Fan, Y. Tang, J. Xi, Y. Bi, Z. Tong, G. F. Gao, N. Xie, A. Tang, G. Nie, M. Liang and X. Yan, Nat. Protoc., 2018, 13, 1506–1520 CrossRef CAS PubMed.
- S. Sivasankaran and S. Jonnalagadda, Int. J. Pharm., 2022, 624, 121994 CrossRef CAS PubMed.
- T. Han, N. Stone-Weiss, J. Huang, A. Goel and A. Kumar, Acta Biomater., 2020, 107, 286–298 CrossRef CAS PubMed.
- S. M. B. Gautham and T. K. Patra, Soft Matter, 2022, 18, 7909–7916 RSC.
- P. Hou, Y. Tian, Y. Xie, F. Du, G. Chen, A. Vojvodic, J. Wu and X. Meng, Angew. Chem., Int. Ed., 2023, 62, e202304205 CrossRef CAS PubMed.
- X. Hu, F. Li, F. Xia, X. Guo, N. Wang, L. Liang, B. Yang, K. Fan, X. Yan and D. Ling, J. Am. Chem. Soc., 2020, 142, 1636–1644 CrossRef CAS PubMed.
- V. E. Kagan, A. A. Kapralov, C. M. St. Croix, S. C. Watkins, E. R. Kisin, G. P. Kotchey, K. Balasubramanian, I. I. Vlasova, J. Yu, K. Kim, W. Seo, R. K. Mallampalli, A. Star and A. A. Shvedova, ACS Nano, 2014, 8, 5610–5621 CrossRef CAS PubMed.
- L. Wang, S. Quine, A. N. Frickenstein, M. Lee, W. Yang, V. M. Sheth, M. D. Bourlon, Y. He, S. Lyu, L. Garcia-Contreras, Y. D. Zhao and S. Wilhelm, Adv. Funct. Mater., 2024, 34, 2308446 CrossRef CAS PubMed.
- L. M. Kaminskas, D. E. V. Pires and D. B. Ascher, Sci. Rep., 2019, 9, 15465 CrossRef PubMed.
- E. O. Kutumova, I. R. Akberdin, I. N. Kiselev, R. N. Sharipov, V. S. Egorova, A. O. Syrocheva, A. Parodi, A. A. Zamyatnin and F. A. Kolpakov, Int. J. Mol. Sci., 2022, 23, 12560 CrossRef CAS PubMed.
- W.-C. Chou, Q. Chen, L. Yuan, Y.-H. Cheng, C. He, N. A. Monteiro-Riviere, J. E. Riviere and Z. Lin, J. Controlled Release, 2023, 361, 53–63 CrossRef CAS PubMed.
- E. Price and A. J. Gesquiere, Sci. Rep., 2019, 9, 13943 CrossRef CAS PubMed.
- B. Luo, T. Yang, S. F. Jawad, H. I. Jabar, H. K. Dabis, M. Adil, A. N. Mustafa, S. K. Hadrawi, I. M. Mohammed, A. Alshetaili, N. M. Mohammed, U. Hani and A. M. Alsubaiyel, J. Mol. Liq., 2023, 377, 121517 CrossRef CAS.
- S. Xia and Y. Wang, J. Mol. Liq., 2023, 375, 121319 CrossRef CAS.
- D. M. Makarov, N. N. Kalikin and Y. A. Budkov, Ind. Eng. Chem. Res., 2024, 63, 1589–1603 CrossRef CAS.
-
A. Mauri, in Ecotoxicological QSARs, ed. K. Roy, Springer US, New York, NY, 2020, pp. 801–820 Search PubMed.
- C. Steinbeck, C. Hoppe, S. Kuhn, M. Floris, R. Guha and L. E. Willighagen, Curr. Pharm. Des., 2006, 12, 2111–2120 CrossRef CAS PubMed.
- J. Kimmig, T. Schuett, A. Vollrath, S. Zechel and U. S. Schubert, Adv. Sci., 2021, 8, 2102429 CrossRef CAS PubMed.
- U. Bozuyuk, N. O. Dogan and S. Kizilel, ACS Appl. Mater. Interfaces, 2018, 10, 33945–33955 CrossRef CAS PubMed.
- B. Baghaei, M. R. Saeb, S. H. Jafari, H. A. Khonakdar, B. Rezaee, V. Goodarzi and Y. Mohammadi, J. Appl. Polym. Sci., 2017, 134, 45145 CrossRef.
- H. Maleki, M. Naghibzadeh, A. Amani, M. Adabi and M. Khosravani, J. Pharm. Innov., 2021, 16, 11–25 CrossRef.
- R. Rebollo, F. Oyoun, Y. Corvis, M. M. El-Hammadi, B. Saubamea, K. Andrieux, N. Mignet and K. Alhareth, ACS Appl. Mater. Interfaces, 2022, 14, 39736–39745 CrossRef CAS PubMed.
- V. Di Francesco, D. P. Boso, T. L. Moore, B. A. Schrefler and P. Decuzzi, Biomed. Microdevices, 2023, 25, 29 CrossRef CAS PubMed.
- M. D. Wilkinson, M. Dumontier, I. J. Aalbersberg, G. Appleton, M. Axton, A. Baak, N. Blomberg, J.-W. Boiten, L. B. da Silva Santos, P. E. Bourne, J. Bouwman, A. J. Brookes, T. Clark, M. Crosas, I. Dillo, O. Dumon, S. Edmunds, C. T. Evelo, R. Finkers, A. Gonzalez-Beltran, A. J. G. Gray, P. Groth, C. Goble, J. S. Grethe, J. Heringa, P. A. C. ’t Hoen, R. Hooft, T. Kuhn, R. Kok, J. Kok, S. J. Lusher, M. E. Martone, A. Mons, A. L. Packer, B. Persson, P. Rocca-Serra, M. Roos, R. van Schaik, S.-A. Sansone, E. Schultes, T. Sengstag, T. Slater, G. Strawn, M. A. Swertz, M. Thompson, J. van der Lei, E. van Mulligen, J. Velterop, A. Waagmeester, P. Wittenburg, K. Wolstencroft, J. Zhao and B. Mons, Sci. Data, 2016, 3, 160018 CrossRef PubMed.
- M. Groenewold, E. A. J. Bleeker, C. W. Noorlander, A. J. A. M. Sips, M. van der Zee, R. J. Aitken, J. H. Baker, M. I. Bakker, E. A. Bouman, S. H. Doak, D. Drobne, V. I. Dumit, M.-V. Florin, W. Fransman, M. M. Gonzalez, E. Heunisch, P. Isigonis, N. Jeliazkova, K. A. Jensen, T. Kuhlbusch, I. Lynch, M. Morrison, A. Porcari, I. Rodríguez-Llopis, B. M. Pozuelo, S. Resch, A. J. Säämänen, T. Serchi, L. G. Soeteman-Hernandez, E. Willighagen, M. Dusinska and J. J. Scott-Fordsmand, NanoImpact, 2024, 35, 100513 CrossRef CAS PubMed.
- H. Gossler, J. Riedel, E. Daymo, R. Chacko, S. Angeli and O. Deutschmann, Chem. Ing. Tech., 2022, 94, 1798–1807 CrossRef CAS.
- I. M. Kusoglu, F. Huber, C. Doñate-Buendía, A. Rosa Ziefuss, B. Gökce, J. T. Sehrt, A. Kwade, M. Schmidt and S. Barcikowski, Materials, 2021, 14, 4892 CrossRef CAS PubMed.
- A. Ammar, S. Bonaretti, L. Winckers, J. Quik, M. Bakker, D. Maier, I. Lynch, J. van Rijn and E. Willighagen, Nanomaterials, 2020, 10, 2068 CrossRef CAS PubMed.
- I. Furxhi, A. J. Koivisto, F. Murphy, S. Trabucco, B. Del Secco and A. Arvanitis, Nanomaterials, 2021, 11, 1818 CrossRef CAS PubMed.
- N. Jeliazkova, M. D. Apostolova, C. Andreoli, F. Barone, A. Barrick, C. Battistelli, C. Bossa, A. Botea-Petcu, A. Châtel, I. De Angelis, M. Dusinska, N. El Yamani, D. Gheorghe, A. Giusti, P. Gómez-Fernández, R. Grafström, M. Gromelski, N. R. Jacobsen, V. Jeliazkov, K. A. Jensen, N. Kochev, P. Kohonen, N. Manier, E. Mariussen, A. Mech, J. M. Navas, V. Paskaleva, A. Precupas, T. Puzyn, K. Rasmussen, P. Ritchie, I. R. Llopis, E. Rundén-Pran, R. Sandu, N. Shandilya, S. Tanasescu, A. Haase and P. Nymark, Nat. Nanotechnol., 2021, 16, 644–654 CrossRef CAS PubMed.
- N. Kochev, N. Jeliazkova, V. Paskaleva, G. Tancheva, L. Iliev, P. Ritchie and V. Jeliazkov, Nanomaterials, 2020, 10, 1908 CrossRef CAS PubMed.
- C. Bossa, C. Andreoli, M. Bakker, F. Barone, I. De Angelis, N. Jeliazkova, P. Nymark and C. L. Battistelli, Comput. Toxicol., 2021, 20, 100190 CrossRef CAS PubMed.
- D. Maier, T. E. Exner, A. G. Papadiamantis, A. Ammar, A. Tsoumanis, P. Doganis, I. Rouse, L. T. Slater, G. V. Gkoutos, N. Jeliazkova, H. Ilgenfritz, M. Ziegler, B. Gerhard, S. Kopetsky, D. Joshi, L. Walker, C. Svendsen, H. Sarimveis, V. Lobaskin, M. Himly, J. van Rijn, L. Winckers, J. Millán Acosta, E. Willighagen, G. Melagraki, A. Afantitis and I. Lynch, Front. Phys, 2023, 11, 1271842 CrossRef.
- A. Bahl, C. Ibrahim, K. Plate, A. Haase, J. Dengjel, P. Nymark and V. I. Dumit, J. Cheminf., 2023, 15, 34 Search PubMed.
- M. Thyssen, G. Grégori, V. Créach, S. Lahbib, M. Dugenne, H. M. Aardema, L.-F. Artigas, B. Huang, A. Barani, L. Beaugeard, A. Bellaaj-Zouari, A. Beran, R. Casotti, Y. Del Amo, M. Denis, G. B. J. Dubelaar, S. Endres, L. Haraguchi, B. Karlson, C. Lambert, A. Louchart, D. Marie, G. Moncoiffé, D. Pecqueur, F. Ribalet, M. Rijkeboer, T. Silovic, R. Silva, S. Marro, H. M. Sosik, M. Sourisseau, G. Tarran, N. Van Oostende, L. Zhao and S. Zheng, Front. Mar. Sci., 2022, 9, 975877 CrossRef.
- I. Lynch, A. Afantitis, T. Exner, M. Himly, V. Lobaskin, P. Doganis, D. Maier, N. Sanabria, A. G. Papadiamantis, A. Rybinska-Fryca, M. Gromelski, T. Puzyn, E. Willighagen, B. D. Johnston, M. Gulumian, M. Matzke, A. Green Etxabe, N. Bossa, A. Serra, I. Liampa, S. Harper, K. Tämm, A. C. Jensen, P. Kohonen, L. Slater, A. Tsoumanis, D. Greco, D. A. Winkler, H. Sarimveis and G. Melagraki, Nanomaterials, 2020, 10, 2493 CrossRef CAS PubMed.
- H. Fan, J. Zheng, J. Xie, J. Liu, X. Gao, X. Yan, K. Fan and L. Gao, Adv. Mater., 2024, 36, 2300387 CrossRef CAS PubMed.
- Y. Cong, R. Qiao, X. Wang, Y. Ji, J. Yang, D. Baimanov, S. Yu, R. Cai, Y. Zhao, X. Wu, C. Chen and L. Wang, J. Am. Chem. Soc., 2024, 146, 10478–10488 CrossRef CAS PubMed.
- F. Du, C. H. Rische, Y. Li, M. P. Vincent, R. A. Krier-Burris, Y. Qian, S. A. Yuk, S. Almunif, B. S. Bochner, B. Qiao and E. A. Scott, Nat. Nanotechnol., 2024, 19, 698–704 CrossRef CAS PubMed.
- X. Lian, S. Chatterjee, Y. Sun, S. A. Dilliard, S. Moore, Y. Xiao, X. Bian, K. Yamada, Y.-C. Sung, R. M. Levine, K. Mayberry, S. John, X. Liu, C. Smith, L. T. Johnson, X. Wang, C. C. Zhang, D. R. Liu, G. A. Newby, M. J. Weiss, J. S. Yen and D. J. Siegwart, Nat. Nanotechnol., 2024 DOI:10.1038/s41565-024-01680-8.
- Q. Cheng, T. Wei, L. Farbiak, L. T. Johnson, S. A. Dilliard and D. J. Siegwart, Nat. Nanotechnol., 2020, 15, 313–320 CrossRef CAS PubMed.
- H. I. Ingólfsson, H. Bhatia, F. Aydin, T. Oppelstrup, C. A. López, L. G. Stanton, T. S. Carpenter, S. Wong, F. Di Natale, X. Zhang, J. Y. Moon, C. B. Stanley, J. R. Chavez, K. Nguyen, G. Dharuman, V. Burns, R. Shrestha, D. Goswami, G. Gulten, Q. N. Van, A. Ramanathan, B. Van Essen, N. W. Hengartner, A. G. Stephen, T. Turbyville, P.-T. Bremer, S. Gnanakaran, J. N. Glosli, F. C. Lightstone, D. V. Nissley and F. H. Streitz, J. Chem. Theory Comput., 2023, 19, 2658–2675 CrossRef PubMed.
- G. Giunta, G. Campos-Villalobos and M. Dijkstra, ACS Nano, 2023, 17, 23391–23404 CrossRef CAS PubMed.
- K. Wan, H. Wang and X. Shi, ACS Nano, 2024, 18, 12367–12376 CrossRef CAS PubMed.
- D. Xu, W. Yin, J. Zhou, L. Wu, H. Yao, M. Sun, P. Chen, X. Deng and L. Zhao, Nanoscale, 2023, 15, 6686–6695 RSC.
- S. Ekins, A. C. Puhl, K. M. Zorn, T. R. Lane, D. P. Russo, J. J. Klein, A. J. Hickey and A. M. Clark, Nat. Mater., 2019, 18, 435–441 CrossRef CAS PubMed.
- W. Poon, B. R. Kingston, B. Ouyang, W. Ngo and W. C. W. Chan, Nat. Nanotechnol., 2020, 15, 819–829 CrossRef CAS PubMed.
|
This journal is © The Royal Society of Chemistry 2024 |
Click here to see how this site uses Cookies. View our privacy policy here.