DOI:
10.1039/D2CS00991A
(Review Article)
Chem. Soc. Rev., 2024,
53, 6445-6510
Material-specific binding peptides empower sustainable innovations in plant health, biocatalysis, medicine and microplastic quantification
Received
5th December 2023
First published on 15th May 2024
Abstract
Material-binding peptides (MBPs) have emerged as a diverse and innovation-enabling class of peptides in applications such as plant-/human health, immobilization of catalysts, bioactive coatings, accelerated polymer degradation and analytics for micro-/nanoplastics quantification. Progress has been fuelled by recent advancements in protein engineering methodologies and advances in computational and analytical methodologies, which allow the design of, for instance, material-specific MBPs with fine-tuned binding strength for numerous demands in material science applications. A genetic or chemical conjugation of second (biological, chemical or physical property-changing) functionality to MBPs empowers the design of advanced (hybrid) materials, bioactive coatings and analytical tools. In this review, we provide a comprehensive overview comprising naturally occurring MBPs and their function in nature, binding properties of short man-made MBPs (<20 amino acids) mainly obtained from phage-display libraries, and medium-sized binding peptides (20–100 amino acids) that have been reported to bind to metals, polymers or other industrially produced materials. The goal of this review is to provide an in-depth understanding of molecular interactions between materials and material-specific binding peptides, and thereby empower the use of MBPs in material science applications. Protein engineering methodologies and selected examples to tailor MBPs toward applications in agriculture with a focus on plant health, biocatalysis, medicine and environmental monitoring serve as examples of the transformative power of MBPs for various industrial applications. An emphasis will be given to MBPs' role in detecting and quantifying microplastics in high throughput, distinguishing microplastics from other environmental particles, and thereby assisting to close an analytical gap in food safety and monitoring of environmental plastic pollution. In essence, this review aims to provide an overview among researchers from diverse disciplines in respect to material-(specific) binding of MBPs, protein engineering methodologies to tailor their properties to application demands, re-engineering for material science applications using MBPs, and thereby inspire researchers to employ MBPs in their research.
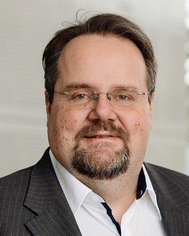
Ulrich Schwaneberg
| Ulrich Schwaneberg graduated in chemistry (in 1996) and received its PhD (in 1999; supervisor Prof. R. D. Schmid) from the University in Stuttgart. He was, after a post doc at Caltech in the lab of the Noble laureate Prof. Frances H. Arnold, appointed as Professor at the Jacobs University Bremen in 2002. In January 2009, he moved to the RWTH Aachen University as Head of the Institute of Biotechnology and is since 2010 co-appointed in the Scientific Board of Directors at the Leibniz Institute for Interactive Materials. Furthermore, he coordinates with Prof. Bergs the competence center Bio4MatPro (one of two BMBF flagship projects in the bioeconomy model region), serves in the board of directors in the Bioeconomy Science Center, and is Speaker of the RWTH profile area Molecular Science & Engineering. He cofounded the companies SeSaM Biotech & Aachen Proteineers and has a special interest is Protein engineering to understand fundamental structure–function relationships and to design with develop methodologies tailored proteins as building blocks for the biological transformation of material science and production. In 2016, he received the BMBF-Forschungspreis for the next generation of bioprocesses and has published >380 original manuscripts and is coinventor on >30 patents, mostly with industry. |
Introduction
Material-binding peptides (MBPs) are amino acid sequences that possess a unique ability for material(-specific) binding. Based on their origin, MBPs can be divided into two categories: (a) naturally occurring binding peptides (nMBPs) and (b) man-made or engineered binding peptides (eMBPs; see Fig. 1). Naturally occurring binding peptides have evolved over millions of years and are mainly designed to bind to carbohydrates, proteins and minerals. Carbohydrate-binding MBPs target natural carbohydrate polymers, and in this review, we focus on the main abundant ones, namely cellulose, chitin, xylan, starch, hyaluronic acid, polysialic acid, heparin, amylose, alginate. Discussed mineral-binding peptides target calcium carbonates, hydroxyapatite and silica, which are the most abundant biogenic minerals. Reported protein-binding peptides target silk, collagen and keratin. Naturally occurring binding protein domains, such as carbohydrate-binding modules (CBMs) have a defined 3-D structure in common, sizes that range from 30 to 200 amino acids, and their binding motifs as well as material(-specific) binding interactions are well-studied and understood on the molecular level. In the case of mineral and protein-binding peptides, shorter peptide sequences are embedded within larger proteins; if not embedded the short sequences are by themselves usually unstructured. The latter nMBPs govern essential natural processes, from biomineralization, host-pathogen interactions, self-assembly to cell adhesion, and degradation of natural polymers.
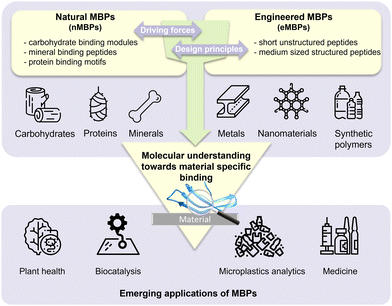 |
| Fig. 1 Overview of material-binding peptides (MBPs) divided in natural MBPs (nMBPs), engineering MBPs (eMBPs) and emerging applications in agriculture (plant health, biocatalysis, medicine and microplastic analytics). An understanding of molecular interactions governing material-specific binding is crucial for innovating applications of material-binding peptides, particularly in the context of microplastics analytics. | |
Man-made binding peptides can be divided into two classes depending on their size. Class I are short peptides (≤20 amino acids), usually derived from phage display libraries or designed rationally, and often do not have a stable secondary structure. Class II eMBPs are very diverse depending on the addressed materials (metals, polymers, metal oxides or nanomaterials) and sizes range often between 20 and 100 amino acids. Class II eMBPs have, similarly to natural binding domains, and in contrast to most class I eMBPs, often a defined 3-D structure, especially after material binding. In this review, we refer to peptides with amino acid sequences <100 amino acids, and to proteins (including binding domains) according to a size of >100 amino acids.
Binding strength and material-specific binding to the targeted material surfaces are achieved through a precise arrangement of amino acid side chains in MBPs, and are usually governed by non-covalent interactions comprising hydrogen bonding, electrostatic interactions, van der Waals forces and/or hydrophobic interactions. Design of material-specific binding proteins/peptides requires a comprehensive understanding of interactions that govern material-specific binding, and takes into account differences in recognition and structures. Interestingly, recurring binding motifs in nMBPs and eMBPs have been reported. Binding strength and material-specific binding can be altered by protein engineering methodologies to tailor MBP properties to different application conditions (see Section “Engineering of binding peptides and proteins”).1–3
MBPs show great promise as a technology platform for microplastics (MP) analytics due to their inherent ability to material-specifically bind different types of common polymers and distinguish microplastics from other particles in complex environmental samples, and thereby enabling accurate quantification and characterization of microplastics (see Section “Advances in nano- and microplastics analytics”).4 This comprehensive review aims to provide a thorough understanding of MBPs by focusing on the molecular interactions responsible for their material-specific binding properties, and emerging applications of MBPs in plant health, biocatalysis and medicine (see Section “Emerging applications of material-binding peptides”) will provide examples of MBPs offering sustainable solutions for a circular bioeconomy and healthcare.
A discussion on lessons learned in respect to molecular interactions, that govern binding strength and material-specific binding of nMBPs and eMBPs, will provide a condensed summary of knowledge, advancements, and limitations/challenges. The discussion part focuses on forces and structural features that govern the initial and/or material-specific binding of MBPs, especially to man-made polymers, including challenges in discriminating chemically similar polymers and challenges that arise from different polymer morphologies.
A gain in fundamental molecular understanding and transferable knowledge enables in part, already today, a time-efficient design of MBPs. Emerging applications of MBPs in plant health, biocatalysis, medicine and microplastic quantification, as well as challenges that impede their widespread adoption, illustrate how MBPs can be used in sustainable materials and can contribute to climate-neutral circular economy.
Furthermore, we propose a roadmap that combines experimental and computational methods (including AI/machine learning) to overcome the combinatorial complexity of the protein sequence space, and ultimately achieve a computational supremacy in which material-specific MBPs can fully be designed in silico.
In summary, we intent that this review provides a comprehensive overview on MBPs, their application potential and challenges, and that interdisciplinarity inspires researches and spreads their use; the latter especially in monitoring, as well as in removing MP-particles from the environment, food and drinking water, and thereby mitigate the risks to human, animal and environmental health.
Material-binding peptides, their material-specific interactions and protein engineering campaigns to tailor binding properties
Naturally occurring binding peptides (nMBPs) that bind to natural polymers and naturally occurring inorganic materials
Natural material-binding peptides (nMBPs) play a crucial role in nature, where nature has developed for a few compound classes, namely carbohydrates, proteins and minerals, a broad array of structurally and functionally diverse proteins for material-specific binding (Fig. 2).5 In the following sections, the nMBPs for the most abundant carbohydrates (alginate, cellulose, chitin, chitosan, heparin, hyaluronic acid, polysialic acid, starch, xylan), proteins (collagen, keratin, silk) and minerals (calcium carbonate, hydroxyapatite, silica) are introduced. The structures of nMBPs, as well as reported binding motifs and interactions, are summarized. nMBPs, as CBMs, have a defined 3-D structure and common secondary structure elements. Mineral- and protein-binding proteins contain binding motifs ranging from 3 to 12 amino acids.6 Electrostatic interactions are driven by amino acids with charged side chains (Asp, Glu, Lys, Arg and His). Amino acids, such as Ser, Asn, Thr and Glu, provide hydrogen bonds. Amino acids with nonpolar side chains govern van der Waals interactions, and can provide hydrophobic interactions. In addition, amino acids containing aromatic groups can engage in π–π interactions or stacking interactions. The binding of peptides to a solid material surface usually involves an initial binding event through an initial contact interface mediated by a few amino acids, followed by a main binding event over a larger contact, involving a combination of diverse molecular interactions. Understanding these interactions is essential for designing and engineering peptides with tailored binding properties that match diverse application demands. General design trends and common interaction motifs are summarized at the end of the section to provide a basis for the comparison with phage display-derived peptides.
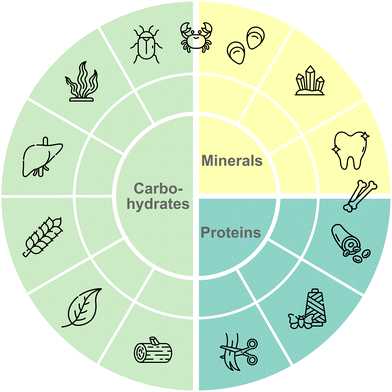 |
| Fig. 2 Overview of most common materials in nature. Natural materials can be divided into three main types: carbohydrates, minerals, and proteins. All materials have further subtypes ranging from cellulose to chitin for carbohydrates, from calcium carbonate to hydroxyapatite for minerals and from collagen to keratin for proteins. Nature evolved structurally diverse nMBPs to perform complex tasks in recognition and material(-specific) binding. All listed natural polymers plus polysialic and hyaluronic acid are introduced and interactions with their corresponding nMBPs and eMBPs are discussed. | |
nMBP for carbohydrates
Naturally occurring carbohydrate polymers are abundant in plants, animals, bacteria, and algae.7 Polysaccharides, the most predominant form of carbohydrates, account for 90% of all sugars and contain functional groups such as hydroxyl-, carboxyl-, aldehyde- or ketone-groups, with the general formula Cx(H2O)n.8,9 Mono- or disaccharide units are connected through α- or β-glycosidic bonds, which determine the linear and branched forms of polysaccharides.10 Regular repeating units in polysaccharides often have highly ordered helical and ribbon-like secondary structures.11 Functional groups on monosaccharide rings, such as hydroxyl-, carboxyl-, amine-, sulphate- and acetyl-moieties, contribute to material-specific interactions and/or polysaccharide recognition.12–14 The latter variability in interactions results in a broad use of carbohydrates as structural support, for energy storage, immune system recognition and several other biological functions.15–17 Polysaccharides can structurally be linear/non-charged (cellulose and chitin), branched/non-charged (starch and xylan) or linear/charged (chitosan, positively charged; alginate, hyaluronic acid, heparin and polysialic acid, negatively charged).
Their huge abundance in nature has made carbohydrates industrially attractive for the synthesis of platform chemicals such as polymer building blocks,18 biobased polymers19 or bioethanol production.20 Nature has evolved specific binding proteins (carbohydrate-binding modules; CBMs), which are usually through a flexible linker connected to the hydrolytic enzymes on one polypeptide chain.21 CBMs are grouped into 100 families,22 of which can diversly bind to carbohydrate polymers. CBMs are, except the carbohydrate-activated enzymes (CAZymes;23 chitin-binding protein CBP21 of Serratia marcescens from CBM33 family24) binding domains, without a catalytic function.25 The catalytic activity of CAZymes can be enhanced on the polysaccharide surface through direct binding of CBMs or disruption of the crystalline polysaccharide form by CBMs.26
CBMs typically have a β-sandwich fold, in which two overlapping β-sheets are composed of six to twelve antiparallel β-strands.27 Depending on the binding mode toward carbohydrate moieties, three main types of CBMs are designed by nature: type A, B and C. Type A CBMs bind in a ‘flat’ binding mode to planar crystalline cellulose, chitin and xylan surfaces via a planar platform composed of amino acids involved in binding. Type B CBMs have an ‘internal’ binding mode, in which binding sites that are shaped like extended grooves or clefts, accommodating longer sugar chains. Type C CBMs employ a ‘termini’ binding mode with small binding pockets to identify moieties composed of one to three monosaccharide units from the reducing/non-reducing ends of the polysaccharide.21
Cellulose
Cellulose is a main component of plant cell walls and the most abundant renewable resource on earth, with an annual production of over 70 billion tons.28 Cellulose is a linear and non-charged polysaccharide consisting of β-D-glucopyranose units (C6H10O5)n linked through β(1 → 4) glycosidic bonds with a non-reducing hydroxyl group and a reducing hemiacetal group at both ends (Fig. 3b).29–31 Notably, each glucose unit contains three hydroxyl groups, which leads to the formation of extensive intra- and interchain hydrogen bonds.32,33 Cellulosic materials contain highly ordered long-chain regions (crystalline) that are interrupted by disordered short-chain regions (amorphous). Crystalline cellulose has a stacked arrangement, while short chains of amorphous cellulose form random coils. Amorphous cellulose chains, consisting of at least one cellulosic unit, are linked by hydroxyl groups at the C-2 and C-3 positions to form isotropic intermolecular hydrogen bonds.33–38 The water solubility of polysaccharides depends, apart from their chemical composition, on the number of repeating monomeric or dimeric units. Cellodextrins with up to 6 repeating units are highly soluble whereas those with 7–8 become less soluble.39 Crystalline and amorphous cellulose are markedly recalcitrant to dissolve in water, which can be attributed to the presence of numerous hydrogen bonds.40 Members of CBM superfamily have in general a β-sandwich fold structure.41 Depending on the conformation of cellulose chains, all three types of CBMs bind to the cellulose surface (Fig. 3b). CH–π interactions between CH moiety from sugar ring and aromatic ring from CBM42 as well as polar hydrogen-bonding are the main driving forces for binding.
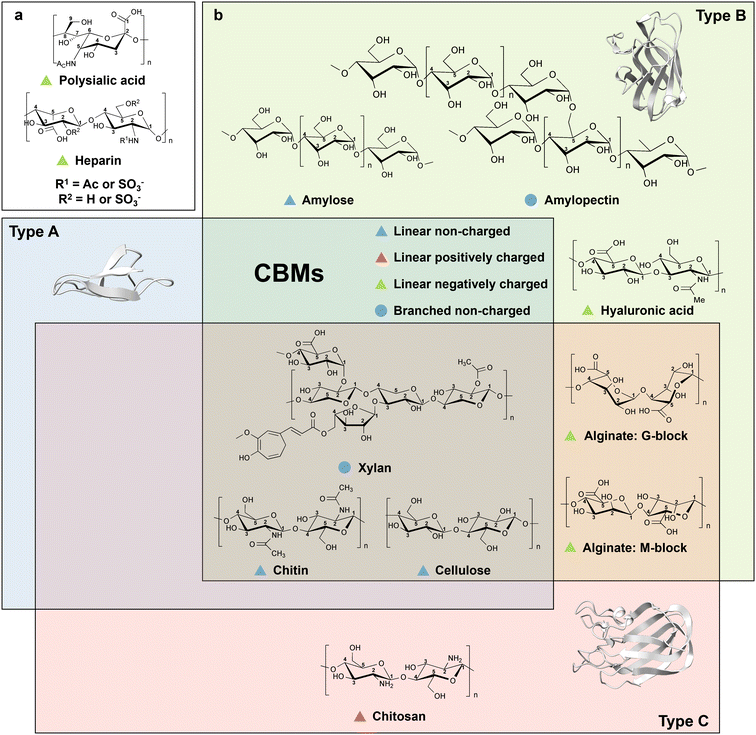 |
| Fig. 3 Overview of CBM types and corresponding carbohydrate moieties. (a) Polysaccharides that do not have a defined type of CBM binding domains. (b) Polysaccharides with reported CBM domains. Polysaccharide are depending on structure and charge properties divided into four groups, linear non-charged (blue triangle), linear positively charged (green triangle), linear negatively charged (red triangle), and branched non-charged (blue circle). Depending on the binding modes, CBMs are divided into three main types, type A, B and C. Polysaccharides with identical binding modes of CBMs are grouped into corresponding squares. Representative structures are shown for each CBM type: for type A the CBM1 from Richoderma reesei cellobiohydrolase I (PDB: 1CBH)43 with a planar binding mode, for type B the CBM6 from Clostridium thermocellum xylanase 11A (PDB: 1GMM)44 with an internal binding mode harbouring a binding site that is shaped like a groove or a cleft, and for type C the CBM32 from Clostridium perfringens N-acetyl-β-hexosaminidas (PDB: 2J1E)45 that with termini binding mode harbouring a small binding pocket. Protein models are visualized and coloured by ChimeraX 1.4.46 | |
Type A cellulose-binding domains have a planar binding mode generated by two to four aromatic amino acid residues (e.g. Trp and Tyr) that interact with the pyranose ring of crystalline cellulose through face-to-face stacking, while the polar amino acids on the flat surface form hydrogen bonds with hydroxyl groups on the ligand to reinforce the binding.47,48 Owing to the release of constrained water molecules from CBM and water-layer around the cellulose surfaces, the binding of type A CBMs on crystalline cellulose is supposed to be entropically driven.49,50 For example, CBM1 from Trichoderma reesei cellobiohydrolase I contains two conserved Tyr residues that interact with pyranose ring structure through CH/π interactions as well as Gln and Asn forming hydrogen bonds with the carbohydrate hydroxyl groups. The variants from Ala scanning, in which Tyr was substituted by Ala in position 5 (Y5A), showed reduced binding affinity towards crystalline cellulose, for instance at positions Y32A, Y31A, Q34A, and N29A (Fig. 4a).51 Interestingly, EXLX1, a bacterial expansin from Bacillus subtilis, which shows strong binding to crystalline cellulose, possesses a CBM63 binding domain with type A CBM characteristics, in which a flat contact surface is composed of three linearly arranged aromatic amino acids (Trp in position 125 (Trp125), Trp126, and Trp157) that interacts with cellohexaose (Fig. 4a).50,52,53 Interestingly, the reduced binding affinity in the Y157A variant is less than that in W125A and W126A variants.50,52 The latter demonstrates the importance of matching distances between aromatic rings in the side chains to bridge the 5.5-Å distance gap to pyranose moieties of cellohexaose.
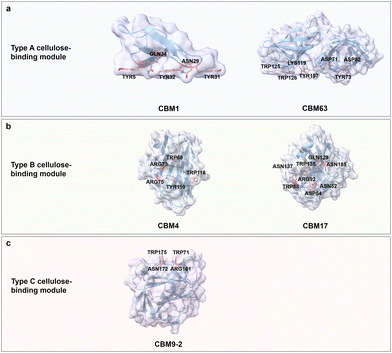 |
| Fig. 4 Structures and key amino acids in cellulose binding in the three main cellulose-binding modules (type A–C). (a) Type A cellulose-binding modules that bind to cellohexaose. Representative CBMs are CBM1 from Trichoderma reesei cellobiohydrolase I (PDB: 1CBH)51,54 and CBM63 from Bacillus subtilis expasin,50 which have a planar-shaped binding site. (b) Type B cellulose-binding modules that bind to cellodextrin and cellotetraose respectively. Representative CBMs are CBM4 from Clostridium thermocellum CbhA (PDB: 3K4Z)55 and CBM17 from Clostridium cellulovorans Cel5A (PDB: 1J84),56 which have a cleft- or groove-shaped binding site. (c) Type C cellulose-binding module that binds to cellobiose. Representative CBM is CBM9-2 from Thermotoga maritima xylanase 10 A (PDB: 1I82),57 with a narrow binding pocket. Key residues for cellulose binding interactions are highlighted in red. CBM structures without specified PDB IDs are predicted with AlphaFold2.58 Protein models are visualized and coloured by ChimeraX 1.4.46 | |
Type B cellulose-binding domain employs a binding site shaped like a cleft, an open groove or an open tunnel to accommodate individual amorphous cellulose chains. A common feature is that aromatic residues located in the central zone of the binding site with polar residues in close proximity jointly interact with the amorphous cellulose.59 CBM4 from Clostridium thermocellum CbhA has three aromatic residues (Trp68, Tyr110 and Trp118) surrounding the deep groove, which, together with polar amino acids (Arg73 and Arg75) specifically recognize single chains of amorphous cellulose. The considerably decreased binding affinity of variants W68A, W110A, R73A and R75A confirms the importance of aromatic and polar residues for cellodextrins binding (Fig. 4b).55 Similarly, alanine scanning in polar amino acids (Asn52, Asp54, Arg92, Gln129, Asn185 and Asn137) and aromatic amino acids (Trp88 and Trp135) located on the shallow binding cleft of CBM17 from Clostridium cellulovorans Cel5A resulted in a reduced binding affinity towards non-crystalline cellulose, indicating the importance of hydrogen bond formation between polar amino acids and cellulose as well as stacking interactions between aromatic amino acids and pyranose moieties of cellulose (Fig. 4b).56
Type C cellulose-binding domains possess a small binding pocket to accommodate the termini of cellulose polymer chains through interactions with aromatic amino acids (e.g. Trp and Tyr) at the entrance of the pocket for interactions with pyranose moieties of cellulose and polar amino acids at the end of the pocket that form hydrogen bonds with cellulose.60 CBM9-2 from Thermotoga maritima xylanase 10A adopting a β-barrel fold binds to crystalline and amorphous cellulose at termini of polymer chain moieties of cellulose. The Trp residues at Trp71 and Trp175 provide with the polar amino acids Arg161 and Asn172 the required interacts, as described for type A and B, for cello-oligosaccharide binding (Fig. 4c).57,60
Chitin
Chitin is a carbohydrate primarily found in the exoskeleton of crustaceans, insects, and fungi cell walls, with a worldwide production of 8–10 billion tons year−1.61,62 It is an unbranched and non-charged amino-polysaccharide composed of repeating N-acetyl D-glucosamine (GlcNAc) units with a general formula (C8H13O5N)n linked through β(1 → 4) glycosidic bonds; each repeating GlcNAc unit contains four hydroxyl groups and two acetamide groups (Fig. 3b).63,64 Chitin adopts a semi-crystalline structure, similar to cellulose, which is comprised of crystalline and amorphous regions.65,66 Interestingly, the crystalline conformation is predominant in chitin with usually more than 90% degree of acetylation. Acetamide residues near the hydroxyl groups in the trans conformation drive the formation of a large number of hydrogen bonds within and between linear chitin chains.67 According to the orientation of the crystalline form, chitin is classified into α-, β-, and γ-types. α-chitin is the most abundant and stable form with anti-parallel conformation, β-chitin has parallel sugar strand conformation which induces a high flexibility, and γ-chitin is a mixed form of α- and β-chitin.68,69 Regarding water solubility, N-acetyl chitooligosaccharides are well soluble with a range of 1–5 repeating units, while larger oligosaccharides from hexamer to octamer become less and less soluble.70,71 Based on the excellent biocompatibility, biodegradability, and antimicrobial activity of chitin, it is widely used for food and biomedical applications, like controlled drug release and wound dressing.72,73
Chitin-binding domains have similar driving forces for chitin binding compared to CBM domains for cellulose; again, aromatic and polar amino acids are the key binding forces. Interestingly, some of the type A CBMs have binding promiscuity to crystalline chitin and cellulose, due to structural similarities. For instance, the chitin-binding domain from Clostridium paraputrificum chitinase can bind cellulose, while the cellulose-binding domain CbpA (CBM3) from Clostridium cellulovorans also binds to chitin.74,75 ChBD2 (CBM2) from Pyrococcus furiosus binds only to chitin but not cellulose, because the recognition of cellobiose units is blocked by negatively charged amino acids, Glu279 and Asp281 (Fig. 5a).76 Interestingly, nature has designed some chitin-binding proteins that bind material-specifically to a chitin allomorphs; for instance, CHB1 from Streptomyces olivaceoviridis binds only to α-chitin and neither to β-chitin, nor to cellulose.77,78 First reports suggest that W57 and two pairs of disulfide bonds are important for specific-binding to a chitin allomorph.78,79
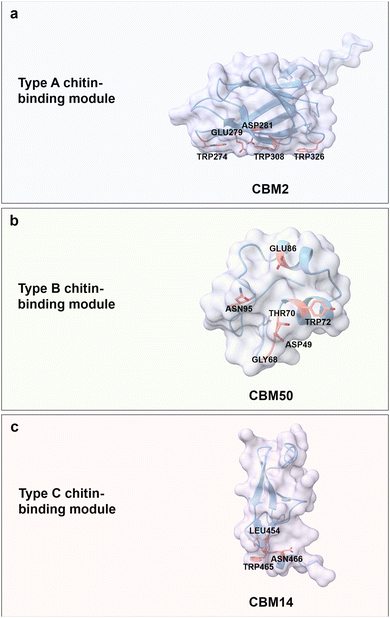 |
| Fig. 5 Structures and key amino acids in chitin-binding modules. (a) Type A chitin-binding module that bind to crystalline chitin. Representative CBM is ChBD2 (CBM2) from Pyrococcus furiosus,76 which has a planar-shaped binding site. (b) Type B chitin-binding module that binds to (GlcNAc)5. Representative CBM is LysM (CBM50) from Pteris ryukyuensis chitinase-A,80 which has a groove-shaped binding site. (c) Type C chitin-binding module that bind to (GlcNAc)3 and β-chitin. Representative CBM is CBM14 from Homo sapiens chitotriosidase-1,81 which has a small binding pocket. Key residues in CBMs for chitin binding interactions are highlighted in red. The CBM structures are predicted with AlphaFold2.58 Protein models are visualized and coloured by ChimeraX 1.4.46 | |
Chitin-binding domain CBM50, also known as LysM, possesses CBM type B binding characteristics with a big groove binding site.82 It typically has a βααβ fold, which is composed of two antiparallel β-strands. The LysM domain from Pteris ryukyuensis chitinase-A binds to (GlcNAc)5 through the key aromatic amino acid Tyr72; the latter is substantiated by a Y72A substitution which disrupts chitin-binding properties (Fig. 5b).80 The interactions between (GlcNAc)5 and LysM from Arabidopsis chitin receptor kinase 1 suggest that the N-acetyl moieties can be specifically recognized, for example, by the formation of hydrogen bonds between N-acetyl moiety and Glu110/Ile141.83 Type C chitin-binding domain CBM14, with a small binding pocket to accommodate the termini of chitin moieties, has a hevein-like fold consisting of three antiparallel β-strands linked to two small antiparallel β-strands.84 CBM14 from Homo sapiens chitotriosidase-1 binds to (GlcNAc)3 and β-chitin with a flat interaction surface composed of Trp465 and Asn466 via face-to-face stacking and hydrogen bonding, respectively. Interesting, L454A impairs the binding interactions through an indirect alteration of Trp465 conformation that results in a less productive orientation (Fig. 5c).81
Starch
Starch is the energy storage polysaccharide compound in plants. It is produced in plastids of leaves, seeds, and storage organs and contributes to 80% of the world's caloric intake.85,86 It is a semi-crystalline and granular polysaccharide consisting of linear amylose and branched amylopectin made of D-glucosyl units (C6H10O5)n.87 Amylose with a chain length of approximately 100–10
000 glucosyl units is by weight present in up to 28% in starch. Amylose is primarily composed of monomeric glucose unit that linked by α(1 → 4) glycosidic bonds, interspersed by a few branches of α(1 → 6) glycosidic linkages.88,89 With increased numbers of α(1 → 6) linkages and long chain length of ranging from 10
000–100
000 glucosyl units, the glucan is referred to as amylopectin (Fig. 3b).88 The branch-chain length and molecular arrangement of amylopectin is related to the granule size of strach.90 Starch is classified into three types according to the crystal form, packing density of its helical structures, and water content. A-type allomorph (e.g. from maize), which is composed of compactly packed left-handed helices, is a large disk-shaped granule with a diameter of 10 to 35 μm. It crystallizes in an orthogonal unit cell with eight water molecules.91–94 B-type allomorph (e.g. from potato), which is 1 to 10 μm in diameter, is spherical and less compact. It is hexagonal and contains 36 water molecules per unit cell.92–95 C-type allomorph (e.g. from legume) is the mixture of type A and type B starch with a size of 3.1 to 50 μm and has an oval to irregular shape.96 Maltodextrins linked by α(1 → 4) glycosidic bonds with less than 20 D-glucosyl units are well water soluble.97 Starch is the most common carbohydrate polymer in the human diet and is widely used in industry. It is employed for the production of biohydrogen,98 ethanol,99 as well as for the synthesis of bioplastics100 and many other products.
The starch-binding domain from the CBMs superfamily has a β-sandwich fold with characteristics of B-type CBMs; i.e. at least one carbohydrate-binding site has a cleft- or groove-like shape, in which aromatic amino acids are located on either side of the binding pocket. The convex angles formed between aromatic residues match the helical spacing of the amylose and amylopectin chains.101 CBM41 from Eubacterium rectale α-amylase has a single carbohydrate-binding site where the pairs of Trp469/Trp418 and Trp416/Lys460/Asp477 contribute to CH–π interactions and formation of hydrogen bonds, respectively (Fig. 6).102 CBM20 from Aspergillus niger GH15 glucoamylase has two binding sites that can work cooperatively to bind on β-cyclodextrin and maltoheptose.101,103 In detail, site 1 has a shallow and solvent-exposed binding area composed of Trp543 and Trp590 for stacking interaction, as well as Lys578 for potential hydrogen bonding.104 Interestingly, Lys578 undergoes a slight structural change during the binding process and might have an essential role in the initial recognition of maltooligosaccharides moieties.105 Binding site 2 consists of three important aromatic residues, in which Trp527 and Tyr556 are key for stacking interactions with glucose moieties of starch, and buried Trp563 is involved in making contact of the neighbouring residues Thr526 and Ile531 with starch moieties (Fig. 6).104 A large conformational rearrangement occurs upon binding and may guide maltodextrin molecules to the active site.105 Regarding the binding of CBM20 to maltoheptaose, the mutational analysis resulted in W590K (binding site 1 variant) with a dissociation constant (Kd) of 0.95 μM and W563K (binding site 2 variant) with a Kd of 17 μM, compared to the wild type with a Kd of 23 μM.106,107 The binding affinity of site 2 is around 18-fold stronger than that of site 1. In addition, the binding affinity of each binding site alone is stronger than that of the two binding sites acting together. Interestingly, only when both binding sites are involved in the binding, the binding of CBM20 to amylose can form a novel structure of a two-turn amylose complex, which can accelerate the enzymatic degradation of crystalline starch.106,108
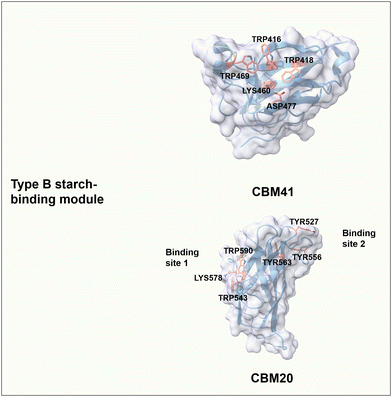 |
| Fig. 6 Structure and key amino acids of type B starch-binding modules. Representative CBMs are CBM41 from Eubacterium rectale α-amylase (PDB: 6AZ5)102 and CBM20 from Aspergillus niger GH15 glucoamylase (PDB: 1AC0),104 which have one or two binding sites respectively. Key residues of CBMs for starch binding are highlighted in red. Protein models are visualized and coloured by ChimeraX 1.4.46 | |
Xylan
Xylan is the major hemicellulose component of plant cell walls and is found in grasses, grains, and trees. Xylan is the third most abundant biopolymer on earth after cellulose and chitin.109 Xylan is a diverse group of polysaccharides consisting of a linear backbone of xylose residues connected by β(1 → 4) glycosidic bonds with various branching residues attached to the backbone depending on tissues and species.110 Grass xylan (GAX) usually contains arabinofuranose (Araf) monomeric units linked by α(1 → 2) and α(1 → 3) glycosidic bonds with a low number of glucuronosyl (GlcA) and (4-O-methyl)-glucuronosyl (MeGlcA) building blocks, which are connected through α(1 → 2) glycosidic bonds.111,112 Xylan is acetylated at the O-2 and O-3 positions on the backbone or at the branch of the arabinosyl residue at the O-2 position and esterified with hydroxycinnamic acid (i.e., ferulic acid and p-coumaric acid) at the O-5 or O-3 position of arabinosyl branches (Fig. 3b).113,114 Xylan exists in mainly two conformations. The first conformation consists of a two-fold screw with a flat-ribbon-shaped even pattern, in which the branching residues are located on the same side of the backbone, or a three-fold screw with a helical shape.115 Most xylans adopt a three-fold screw, which shifts to a two-fold screw when tightly bound to cellulose microfibrils.116 Xylo-oligosaccharides shorter chains up to DP10 are well soluble in water, while those of DP11-20 are becoming less and less soluble in water.117 Xylan is used in various applications, such as food products,118 packaging,119 and drug formulations.120
Xylan-binding domains from the CBMs superfamily have type A, B, and C binding modes, in which the main driving forces are, as reported for the other carbohydrates before, CH/π interactions between aromatic residues and C–H in xylose residues as well as the hydrogen bonds between the polar residues and hydroxyl groups in xylose residues. Type A xylan-binding modules bind to xylo-oligosaccharide via exposed flat binding sites, where CH/π interactions contribute stronger to the binding specificity and affinity than hydrogen bonds.121 CBM2b-1 from Cellulomonas fimi xylanase 11 A adopts a β-sandwich fold with a flat binding site composed of two aromatic (Trp259 and Trp291) and six polar residues (Glu257, Asp261, Asn265, Gln288, Asn292 and Thr316) (Fig. 7a).122 Alanine-substitutions at hydrogen bonding residues Asn292, Gln288 and Glu257 have only a slight effect on the binding strength (binding energy of ≤0.3 kcal mol−1). The loss of at least 2.5 kcal mol−1 in binding energy is observed when either Trp259 or Trp291 is mutated to the corresponding Ala variants.121
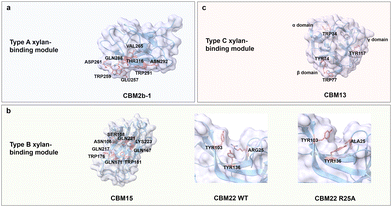 |
| Fig. 7 Structure and key amino acids of xylan-binding modules. (a) Type A xylan-binding module that binds to xylohexaose. A representative CBM is CBM2b-1 from Cellulomonas fimi xylanase 11 A (PDB: 2XBD),122 which has a planar-shaped binding site. (b) Type B xylan-binding modules that bind to Xylopentaose and xylotetraose respectively. Representative CBMs are CBM15 from Pseudomonas cellulosa xylanase Xyn10C123 and CBM22 from Clostridium thermocellum xylanase Xyn10B (PDB: 1H6XY),121,124 which have groove-shaped binding sites. Variant R25A changes the orientation of aromatic residues. (c) Type C xylan-binding module that binds to xylobiose. Representative CBM is CBM13 from Streptomyces lividanse endo-β-1,4-xylanase 10A,125 which has three binding pockets. Key residues of CBMs for xylan binding interactions are highlighted in red. The CBM structures without specified PDB IDs are predicted with AlphaFold2.58 Protein models are visualized and coloured by ChimeraX 1.4.46 | |
Xylan-binding domain CBM15 from Pseudomonas cellulosa xylanase Xyn10C employs a classic β-jelly roll fold with an extended groove as binding pocket. The concave surface contains two Trp residues (Trp181 and Trp176) at an orientation angle of 240°, complementing the three-fold helical conformation of xylan moieties which rotate at 120°. The other polar residues, Asn106, Ser108, Gln167, Gln171, Gln217, Gln221 and Lys223, are responsible for the formation of a hydrogen bond network (Fig. 7b).123 Variant R25A of CBM22 from Clostridium thermocellum xylanase Xyn10B suggests the importance of a productive orientation of aromatic residues, in which Arg25 plays a decisive structural role in Tyr103 and Tyr136 orientation (Fig. 7b).124 Interestingly, the distance between two Trp residues for face-to-face stacking interactions with the rings of xylan moieties determines the minimum distance required for binding, i.e., CBM15 requires a minimum of three xylan building units, whereas CBM22 with adjacent stacking residues requires two.123,126
Type C xylan-binding domain CBM13 from Streptomyces lividanse endo-β-1,4-xylanase 10 A has a β-trefoil fold with three subdomains (α, β, and γ) to accommodate short xylooligosaccharides. Aromatic residues Trp34 from α-site, Tyr74 and Trp77 from β-site as well as Tyr117 from γ-site are key for the stacking interactions (Fig. 7c). Polar residues Asp19, Asp22, Gln32, His37, Asn41 and Gln42 in the α-site, Asp61, Gln72, Asn81 and Gln82 in the β-site and Asp102, Asn106, Gln115, Ser120, Asn124 and Gln125 in the γ-site are responsible for binding through a hydrogen bond network. Each site involved in binding accommodates four xylose units.125
Chitosan
Chitosan is the only bulk polycationic carbohydrate in nature; it is found for instance in the cell walls of filamentous fungi and the exoskeleton of crustaceans.127 Chitosan is a linear and positively charged (amine group) polysaccharide derived from the partial deacetylation of chitin by D-glucosamine (GlcN) units linked to N-acetyl D-glucosamine building blocks via β(1 → 4) glycosidic bonds (Fig. 3b).64 Chitooligosaccharides (COS) with a DP value of less than 10 are typically well water soluble; chitosan water solubility with DP values above 10 can be increased with increased acetylation and at decreased pH.128 Deacetylation of chitin by chemical methods employs alkali treatment and results in a random acetylation pattern; recently developed enzymatic methods (chitin deacetylases (CDAs)) in chitin treatment yielded defined acetylation degrees with defined patterns.129,130 The enzymatic methods for controlled deacetylation are important since the physicochemical properties and biological activities of chitosans are affected by the DP, degree of acetylation, and its acetylation patterns.131 The cationic charge and the presence of a reactive functional group make chitosan a versatile biopolymer. Chitosan products are used in many biomedical applications like controlled drug delivery,132 wound healing,133 tissue regeneration,134 or in wastewater treatment,135 food packaging,136 cosmetics,137 and agriculture.138
Chitosan-binding domains from the CBMs superfamily adopt a canonical β-sandwich fold. For instance, CBM32 from Paenibacillus sp. IK-5 chitosanase is regarded as a member of type C CBM with a small binding pocket to accommodate the non-reducing end of a (GlcN)3 molecule.139 The two CBMs discoidin domains 1 and 2 (DD1 and DD2; 70% amino acid sequence identity), which belong to the CBM32 family are highly specific in binding to chitosan.140 Aromatic residues like Tyr119 from DD1 as well as Tyr36 and Tyr120 from DD2 contribute to CH/π stacking interaction with (GlcN)3 moieties. Conserved negatively charged amino acids (Glu14, Glu36 and Glu61 from DD1, Glu14 and Glu61 from DD2) are involved in the interactions with the positively charged amino groups in (GlcN)3 molecules through electrostatic interactions and a hydrogen bond network (Fig. 8a).13,141 Notably, DD1 with three negatively charged Glu residues exhibits a 1.5-fold higher binding affinity towards (GlcN)4 than DD2 with only two Glu residues.139
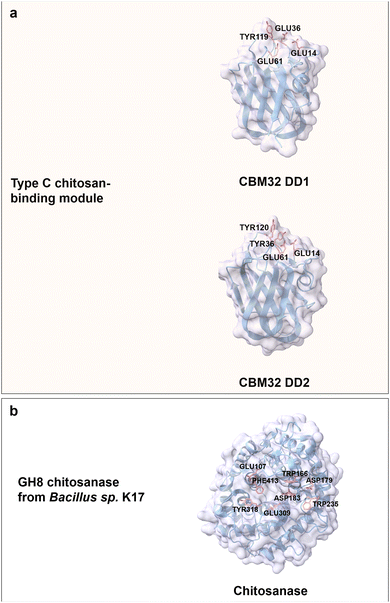 |
| Fig. 8 Structures and key amino acids of chitosan-binding proteins. (a) Type C chitosan-binding modules that bind to (GlcN)3. Representative CBMs are two domains of CBM32 from Paenibacillus sp. IK-5 chitosanase (PDB: 4ZY9 for DD1, and PDB: 4ZZ8 for DD2),140 which have a narrow binding pocket. (b) Chitosanase. A representative chitosanase is GH8 chitosanase from Bacillus sp. K17 (PDB: 1V5C),142 which harbours a groove-shaped catalytic binding pocket. Key residues for CBM binding interactions to chitosan moieties are highlighted in red (see main text). Protein models are visualized and coloured by ChimeraX 1.4.46 | |
Interestingly, most of the chitosanases with efficient catalytical properties lack a chitosan-binding domain in contrast to cellulases. The extended catalytic grooves with both ends open take over the role of binding to long COS.143 A typical example is GH8 chitosanase from Bacillus sp. K17 with an (α/α)6 fold formed by six repeating helix-loop-helix motifs. Like the CBMs binding driving forces, aromatic residues Trp235, Trp166, Phe413 and Tyr318 located in the binding groove interact through face-to-face stacking. Negatively charged residues Asp179, Glu309, Asp183 and Glu107 are through electrostatic interactions part of chitosan recognition (Fig. 8b).142
Alginic acid
Alginic acid is a naturally occurring anionic polysaccharide mainly found in algae and bacteria.144,145 Alginic acid is a linear and negatively charged (carboxyl group) polysaccharide composed of β-D-mannuronic acid (M block) and α-L-guluronic acid (G block) connected by (1 → 4) glycosidic bonds. The building blocks of alginic acid can be either homogeneous or heterogeneous. The M block features a flexible chain with a 4C1 (equatorial chair) conformation, while the G block adopts a rigid 1C4 (axial chair) conformation, attributed to steric hindrance around the carboxyl groups (Fig. 3b).64 Physical properties of alginate vary considerably depending on the composition ratio, polymer length, and connection mode between M- and G-blocks.146 Besides, the G block is reported to chelate with Ca2+, which results in gelation.147 Alginate oligosaccharides with DP of 2 to 25 are well water-soluble oligomers/products generated through enzymatic hydrolysis.148 As a general trend, the water solubility increases with higher GG building block content.149 Alginate has broad applications in tissue engineering,150 wound dressing,151 and drug formulations152,153 due to its biocompatibility, low toxicity, and mild gelation process.
Alginate-binding domains from the CBMs superfamily generally adopt type B and C binding modes, in which aromatic residues are as for other carbohydrates involved in CH/π interactions, polar residues take part in the formation of a hydrogen bond network, and additionally positively charged residues provide electrostatic interactions with carboxylate groups in alginate. CBM96 from Defluviitalea phaphyphila alginate lyase, a recent member of carbohydrate-binding modules, has type B CBM characteristics with a β-sandwich fold; it binds to alginate through a shallow and wide binding cavity/site, in which positively charged residues (Lys10, Lys22, Lys25, Lys27, Lys31 and Arg36) and an aromatic residue (Tyr159) mediate CBM-ligand recognition (Fig. 9a). The key roles of these residues in alginate recognition/binding has been verified by site-directed mutagenesis studies.154
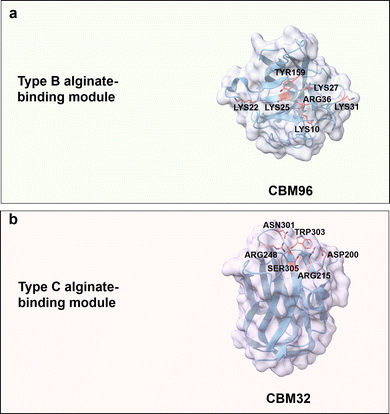 |
| Fig. 9 Structures and key amino acids for alginate-binding modules. (a) Type B alginate-binding module that binds to alginate pentasaccharide. Representative CBM is CBM96 from Defluviitalea phaphyphila alginate lyase (PDB: 7VBO),154 which has a groove-shaped binding site. (b) Type C alginate-binding module that binds to alginate trisaccharide. Representative CBM is CBM32 AlyQB from Persicobacter sp. CCB-QB2 alginate lyase (PDB: 7D2A),14,155 which has a narrow binding pocket. Key residues for alginate binding interactions are highlighted in red. Protein models are visualized and coloured by ChimeraX 1.4.46 | |
CBM32s with a typical β-sandwich fold and a highly flexible pocket have been reported for binding to the non-reducing ends of oligosaccharides ranging from simple galactose to complex N-acetylgalactosamine.155,156 CBM32 AlyQB from Persicobacter sp. CCB-QB2 alginate lyase, with a type C binding mode, specifically binds to 4,5-unsaturated mannuronic acid. Aromatic residue Trp303 plays again a role for stacking interaction with the unsaturated mannuronic acid ring. Polar residues Asn301 and Ser305 interact with the carboxyl group of 4,5-unsaturated mannuronic acid, Asp200 and Arg215 are reported to contribute by hydrogen bond formation with the C3–OH group, and finally the positively charged residue Arg248 forms a salt bridge with the carboxylate group (Fig. 9b).14
Hyaluronic acid
Hyaluronic acid (HA) is an anionic polysaccharide that forms water-flexible hydrogels and is found throughout the human body from the skin to the extracellular matrix (ECM) of connective and epithelial tissues.64,157 HA is a linear, anionic (carboxyl group) and water-soluble polysaccharide composed of repeating disaccharide units of D-glucuronic acid (GlcA) and GlcNAc linked by alternating β(1 → 4) and β(1 → 3) glycosidic bonds (Fig. 3b).158 The H-bonds formation between water molecules and functional groups of HA (carboxyl and acetamido group) stabilizes the secondary structure and enhances water solubility.159 Based on its biodegradability, biocompatibility, non-immunoactivity, and moisture-retaining properties, HA is broadly used in wound dressing,157 tissue engineering,160 drug delivery161 and cosmetics.162
Up to date, CBM70 is the only reported CBM from the superfamily that specifically binds to HA. CBM70 from Streptococcus pneumoniae hyaluronate lyase has a β-sandwich fold with type B binding mode. The binding cavity harbours with several positively charged amino acids Arg112, Arg145, Lys143, Lys185 and His116, and a highly conserved aromatic residue Trp82 (Fig. 10a). The critical role of these residues is confirmed by alanine-substitution. For instance, in H116A and K143A, the binding constant was reduced by around 10-fold, and in W82A and K185A by 50-fold, while the variants R112A and R145 lost the ability to bind to a hyaluronan 7-mer.163 The main driving forces are again CH/π interactions, hydrogen bonds, and electrostatic interactions. On top of CBM70, cell surface receptors composed of glycoproteins also show specific binding to HA. HA can interact with inflammation- and cancer-associated receptors (CD44 and the receptor for hyaluronic acid-mediated motility (RHAMM)) on cell surfaces and trigger signalling processes.164,165 The specific recognition of HA is as for the previously discussed carbohydrates achieved by CH/π interactions between aromatic residues and both GlcA and GlcNAc rings, electrostatic interactions, and formation of a hydrogen bond network. In detail, positively charged amino acids (Lys38, Arg41, Lys68 and Arg78), aromatic amino acids (Tyr42, Tyr79 and Tyr105), and the polar amino acids (Asn100 and Asn101) of the human cell surface receptor CD44 are considered to be the key residues for HA-specific binding (Fig. 10b).164,166,167 Interestingly, the RHAMM receptor protein has a different BX7B binding motif, in which B stands for either Lys or Arg and X contains no negatively charged residues and at least one positively charged residue.168 The reduced binding affinity of the variants K785N and R793S for HA indicates the importance of these two positively residues on either side of the binding cavity.169
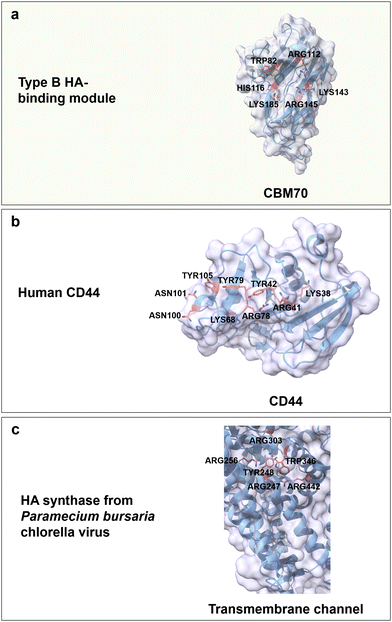 |
| Fig. 10 Structure and key amino acids for HA-binding proteins. (a) Type B HA-binding module that binds to hyaluronan 7-mer. Representative CBM is CBM70 from Streptococcus pneumoniae hyaluronate lyase (PDB: 4D0Q),75,163 which has a grooved-shaped binding site. (b) Sugar receptor human is of human origin (PDB: 1UUH),166 which specifically recognizes HA moieties. (c) HA synthase from the Paramecium bursaria chlorella virus,170 in which the transmembrane channel synthesizes and translocates poly-HA through the transmembrane channel protein. Key residues for HA binding interactions are highlighted in red. The CBM structures without specified PDB IDs are predicted with AlphaFold2.58 Protein models are visualized and coloured by ChimeraX 1.4.46 | |
HA synthases are a family of membrane-associated glycosyltransferases or transmembrane enzyme complexes that catalyse the synthesis of HA from uridine diphosphate-activated precursors and translocation through the membrane.171 HA synthases have no corresponding CBMs and specifically recognize HA moieties through a transmembrane channel by face-to-face stacking, electrostatic interactions and a hydrogen bond network. For HA synthase from Paramecium bursaria chlorella virus, positively charged residues Arg303, Arg256, Arg247 and Arg442 interact with the negatively charged carboxylates of GlcA. Aromatic residues Tyr248 and Trp346 coordinate the ring of GlcNAc, but not GlcA (Fig. 14c). Variants R247A/K, R256K or Y346L confirm their functional importance by reduced HA synthease activity; interestingly, the variant W248A causes a 80% activity drop when compared to the wild type.170
Heparin
Heparin is the most negatively charged polysaccharide since it is highly sulphated. It can be found for instance in the liver, intestine, lungs, and mast cells.172,173 Heparin is a linear and water-soluble polysaccharide consisting of repeating disaccharide units of uronic acid (10% β-GlcA and 90% α-L-iduronic acid (IdoA)) and GlcN/GlcNAc linked by α,β(1 → 4) glycosidic bonds.64 Sulphated positions are O-2 of either IdoA or GlcA, O-3 and O-6 of N-sulphated GlcN (GlcNS), as well as O-6 of GlcNAc (Fig. 3a). The one negative charge per sulphate molecule and the carboxyl group of uronic acid make heparin a highly negatively charged polymer (max. 4 neg. charges per repeating disaccharide unit).172,174 Heparin has been used for antithrombotic and anticoagulant medication, specifically to treat heart attacks and angina pectoris.175
There are no CBMs from any superfamily reported for the specific binding to heparin. Activity of heparin is mediated through direct interactions with various proteins involving characteristic motifs responsible for heparin–protein binding, that have been excellently summarized in previous reviews.176,177 The reported general principles involve electrostatic interactions between basic residues and negatively charged sulphate/carboxyl groups, formation of hydrogen bonds between polar residues and heparin disaccharide side chains (sulphate, carboxyl and hydroxyl groups) and the sulfation patterns (2-O-sulphate, 6-O-sulphate and N-sulphate). Understood highlights comprise interactions between 8-mer heparin and thrombin, as well as the 5-mer heparin and antithrombin. In detail, thrombin is a protease that can break down soluble fibrinogen finally resulting in the formation of the fibrin polymer. Specific recognition between thrombin and 8-mer heparin is achieved through ionic interactions between positively charged residues (Arg93, Lys236, Lys240, Arg101, His91, Arg126 and Arg165) and carboxylate/sulphate groups, hydrogen bonding formation between polar residues (Arg233, His230 and Trp237) and sugar moieties with an important role of water molecules (Fig. 11a).178 Antithrombin is a 464 amino acids protein that acts as serum protein protease inhibitor (binding protein) and plays a major role as physiological regulator of vertebrate blood coagulation proteases. Positively charged residues (Lys114, Lys125 and Arg129) mediate the binding between antithrombin and 5-mer heparin via electrostatic interaction and hydrogen bonding formation (Fig. 11b).179 The specific recognition between Lys114 residue and the 3-O-sulphate group contributes around 60% of the binding free energy. The deletion of the sulphate group at the 3-O position reduces the binding affinity by 104 to 105-fold.12
Polysialic acid
Polysialic acid (PSA) is a nine-carbon sugar homopolymer widely present in bacteria and vertebrates. PSA is an important posttranslational modification to cell adhesion molecules, such as neural cell adhesion molecule (NCAM), and has important roles in intercellular adhesion and cell migration.180–182 PSA is a linear, water-soluble and negatively charged polysaccharide consisting of at least eight units of 5-N-acetylneuraminic acid (Neu5Ac), 5-N-glycolylneuraminic acid (Neu5Gc) or deaminated-neuraminic acid (KDN) residues linked by α(2 → 8) (predominantly), α(2 → 9) or α(2 → 8)/α(2 → 9) alternating glycosidic bonds depending on the origin (Fig. 3a).183,184 For instance, the human PSA is α(2 → 8) linked Neu5Ac that is mainly found in embryos and infants.185 It is often used in drug delivery systems due to its non-immunogenicity, biodegradation, hydrophilicity, softness, and properties similar to poly(ethylene glycol).186,187
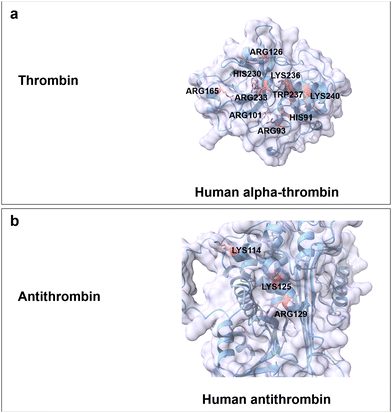 |
| Fig. 11 Structure and key amino acids for heparin-binding proteins. (a) Thrombin. Representative is α-thrombin from human.178 (b) Antithrombin. Representative is antithrombin from human.179 Key residues for heparin binding interactions are highlighted in red. All the protein structures are predicted by AlphaFold2.58 Protein models are visualized and coloured by ChimeraX 1.4.46 | |
Regarding carbohydrate-binding protein from the CBMs superfamily, CBM40 from Ruminococcus gnavus trans-sialidase is reported to bind α(2 → 3)- or (2 → 6)-sialyllactose with high affinity; again hydrophobic, H-bonds, and electrostatic interactions are the main driving forces. Specifically, Tyr210 and Ile95 form a hydrophobic pocket that interacts with the N-acetyl group. Positively charged residues Arg204 and Arg128 interact electrostatically with the carboxyl group of Neu5Ac. Polar residues Lys135 and Glu126 form hydrogen bonds with the C4 hydroxyl group, and Glu126 as well as Tyr210 interact with the nitrogen of the N-acetyl group (Fig. 12a). Notably, no CBMs have yet been reported to bind to PSA linked by α(2 → 8) glycosidic bonds.188
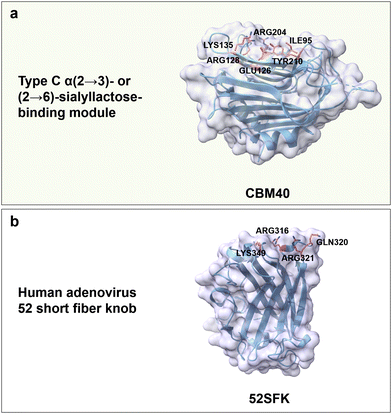 |
| Fig. 12 Structure and key amino acids of sialic acid oligosaccharide-binding proteins. (a) Type C α(2 → 3)- or (2 → 6)-sialyllactose-binding modules that bind to Neu5Ac. Representative CBM is CBM40 from Ruminococcus gnavus trans-sialidase (PDB: 6ER2),188 which has a narrow binding pocket to accommodate α(2 → 3)- or (2 → 6) linked Neu5Ac. (b) A representative adenovirus for HA binding is 52SFK from human (PDB: 4XL8).189 Key residues for sialic acid–oligosaccharide binding interactions are highlighted in red. Protein models are visualized and coloured by ChimeraX 1.4.46 | |
Human adenoviruses are nonenveloped viruses associated with gastrointestinal, ocular, and respiratory infections. Interestingly, the knob domain from the short fibre of adenovirus type 52 (52SFK) has a specific binding towards PSA, with a binding pocket that can accommodate only one sialic acid moiety and has a positively charged rim (Gln320, Arg321, Arg316 and Lys349) around its binding site. The complete loss of cell binding affinity for R316A, R321Q and K349A variants indicate the importance of these basic residues (Fig. 12b); the binding affinity in the variants R321Q/E348Q, compared to the variant R321Q, is somehow restored, due to an overall reduction of negative charges in the binding site.189
In summary, nMBPs for polysaccharides can be divided into three categories, based on the polysaccharide properties. In non-charged polysaccharides (cellulose, chitin, starch and xylan), the main binding driving forces are CH/π interactions and hydrogen bonding. Aromatic amino acids (Tyr, Trp and Phe) and polar amino acids (Glu, Asn, Arg, Asp, Glu, Tyr and Lys) are important for carbohydrate-specific recognition. Cellulose-, chitin- and xylan-binding modules from the CBMs superfamily adopt type A, B, and C binding modes, while starch-binding modules only adopt the type B binding mode. The alignment of aromatic amino acids and spatial control of distance between pyranose rings in oligosaccharides is key for carbohydrate-specific binding; especially in the case of crystalline cellulose and xylan.50,126 In general, a productive conformation and precise positioning of aromatic residues that align with the conformation of carbohydrate moieties (cellulose and chitin moieties are planar, and three-fold screw xylan moieties rotate 120°) is crucial for face-to-face stacking interactions.81,123,124,190 Starch-binding domains utilize a cooperative mechanism involving two binding sites—one for initial recognition and the second one for tight/precise binding.103
In the case of the positively charged chitosan, and the negatively charged polysaccharides HA, alginate, PSA, and heparin, CH/π interactions, hydrogen bonding, and additionally electrostatic interactions are involved in carbohydrate-specific binding. In case of chitosan, the positive charges generally contribute to the binding interactions between chitosan CBMs and COS moieties. CBM32 (type B binding mode) is the only binding module identified from CBMs superfamily for chitosan-binding up to date.139 The binding affinity increases with additional negatively charged residues involved in binding.
In case of HA, alginate, PSA, and heparin, the negative charges range from 1 to 4 per repeating unit. In general, the negative charges are mainly used to electrostatically interact with the positively charged amino acid residues. CBM96 (type B binding mode) and CBM32 (type C binding mode) from CBMs superfamily are reported binding modules for alginate.14,154 CBM70 (type B binding B) is the only CBM from the superfamily reported to bind HA up to date.163 Heparin and PSA do not employ CBM binding module. For the binding proteins other than CBMs, such as RHAMM for HA, thrombin for heparin and 52SFK for PSA, aromatic residues are not essential for carbohydrate specific recognition.168,189 In summary, the CBM superfamily is the most successful found in nature for binding of cellulose, chitin, starch, xylan, chitosan, alginate and HA. Coevolution occurred and has yielded, as reported for chitosan and HA, enzymes such as chitosanases and HA synthases.142,170 Interestingly, the binding sites of chitosanases and HA synthases, found similar molecular solutions for specific carbohydrate-binding. For example, chitosanases adopt type B-like catalytic binding grooves, in which aromatic residues interact with GlcN or N-acetyl GlcN ring through face-to-face stacking and negatively charged residues interact with positively charged amino groups of chitosan moieties via electrostatic interaction.142
Binding promiscuity is common for CBMs. Crystalline cellulose, chitin, and xylan have type A binding mode with a planar binding interface in common. In detail, the promiscuous CBM3 binds to cellulose and chitin.74 The promiscuous CBM29, which is not mentioned in detail above, has a type B binding mode with a groove-shaped binding site and binds to soluble glucomannan, galactomannan, β-glucan, hydroxyethylcellulose, as well as insoluble forms of cellulose and mannan. CBM32 has a type C binding mode with a narrow and flexible binding pocket and promiscuously binds to various oligosaccharides including galactose and N-acetylgalactosamine that are for instance found in chitosan and alginate.14,139
Interestingly, material-specific binding to allomorphs (e.g. α- and β-chitin) as well as crystalline and amorphous cellulose could be achieved in the CBM superfamily evolved by nature.59,78
nMBP for minerals
Calcium carbonate-, calcium phosphate- and silica-based composites are the main minerals found in nature often with structural functions to support and/or protect biological tissues. Invertebrates use calcium carbonate (CC) to assemble shells, while vertebrates employ hydroxyapatite (HAP) to form teeth and bones.191 Silica-based composite materials are part of the skeleton in diatoms and sponges. Biomineralization follows a general principle starting with the accumulation of precursor ions, which are deposited in a highly organized manner through sophisticated biomineralization processes. Examples of biomineralization processes are summarized in several well-written reviews192–196 and will not be further discussed in this review. Impressively, organisms can in general control the composition and morphology from the nanometre scale to the macroscopic level, while fine-tuning properties of the produced materials with exceptional precision. All these are achieved under mild conditions in terms of temperature, pH, and pressure.197–199 Mineral-binding proteins serve in several functions to direct the nucleation and growth of minerals, controlling the formation of minerals into specific shapes. The focuses in the following paragraphs are some prominent biomineralization composites and their interactions with mineral-binding peptides. In general, electrostatic interactions are often the main driving force for protein binding to minerals that occur mainly as calcium carbonate-, calcium phosphate- and silica-based composites with different morphologies.
Calcium carbonate
Calcium carbonates (CCs) are one of the most abundant minerals in biological systems. CCs can be found as mineral components in the shells of invertebrates as well as in avian eggshells; these structures can consist of up to 95% inorganic minerals with a few percentage of organic components.200 Calcium carbonates can be categorized into three crystalline and amorphous forms.197,201,202 Amorphous calcium carbonate (ACC) plays a key role during the early stage of biomineral formation as a transient phase and precursor towards the more stable calcium carbonates.203 The crystal structures of different polymorphs are depicted in Fig. 13. Following Ostwald's rule,204 which states that the less stable polymorph crystallizes first, vaterite is formed followed by a transformation into more stable polymorphs over time (first aragonite followed by calcite, the most stable polymorph).
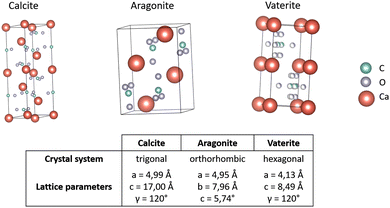 |
| Fig. 13 Crystal structure of calcium carbonate polymorphs. Coordination of atoms within one crystal cell of the CC polymorphs calcite,205 aragonite,206 and vaterite.207 Carbon (C) shown in green, xxygen (O) in grey and calcium (Ca) in red. Structures were generated with VESTA.208 | |
CC polymorph formation is influenced by abiotic factors like temperature or the ratio of Mg-/Ca-ions.209 Polymorph specific calcium carbonate structures can be stabilized independent of the surrounding conditions with the help of binding proteins.209 Calcium carbonate biominerals are composed of three main components, being CC as the mineral component, chitin as a scaffold for the formation of the mineral, and proteins functioning as binding crosslinkers for the framework and mineral respectively.210 Calcium carbonate binding domains, nMNPs, have highly conserved binding regions, to construct organized and complex composite structures.211 Looking at the shells of molluscs, two different layers can be differentiated, the prismatic or outer shell, in which the polymorph calcite is predominant and the nacreous or inner shell, which is composed of the polymorph aragonite. A comparison of the terminal sequences of associated proteins found in the prismatic and the nacre layer showed some significant differences.212 Nacre associated proteins are characterized by clusters of anionic, cationic, and hydrogen bond forming residues, which results in a heterogeneous surface. Within this group of proteins associated with the inner shell the amino acid composition can vary as net charges range from −7 to +6. Defined spatial distribution of charged and hydrogen bond forming residues is critical for function within the biomineralization process.212 Interestingly, terminal sequences of proteins associated with the prismatic layer of shells have a high content of anionic residues, which can vary from 10% to 80% within the approximately 50 amino acid-long binding sequences, generally resulting in a net negative charge in CCs binding proteins. Notably, Asp is up to 6 times more common than Glu in nMNPs.212 Positively charged amino acids are only found rarely in prismatic binding sequences. Interestingly, they are separated from anionic residues, or occur as single positively charged residues close to an anionic residue, which has been reported as a unique trait of prismatic-associated CCs binding proteins.212
Eggshell is another material that utilizes CC in the form of the polymorph calcite, which functions as a protective layer.203 Eggshells consist of around 95% of the mineral with 3.5% of organic components, which include hundreds of proteins that play a certain role in the formation of the mineral.213 A family of eggshell matrix proteins that bind to the surface of mineral crystals have been identified in avian species.214 In general, electrostatic interactions are the main driving force of binding CC in eggshells. Interestingly several solutions have been found by nature to interact with the mineral CC during eggshell formation; some proteins rely on acidic residues Glu and Asp, similar to the calcite-binding proteins described above, while others have a higher content of the basic amino acid residues Arg and Lys.214,215
Hydroxyapatite
Hydroxyapatite (HAP) belongs to the group of calcium orthophosphates and is the main mineral in teeth and bones of vertebrates consisting of calcium–phosphate and calcium–hydroxide, with the general formula Ca5(PO4)3(OH). Properties, formation processes, and occurrences of calcium orthophosphates have been reviewed in several excellent reports.216–219 HAP is produced from amorphous calcium phosphate (ACP) precursor which forms first,220 and which is subsequently converted into the crystalline form HAP.221 In non-biological processes, the precipitation of the mineral occurs within hours at optimal temperature and pH.222 Biological HAP formation processes that utilize nMBPs generally take longer as, developed structures have to be highly organized in order to ensure the structural integrity of the biomineral.223
Depending on the biological tissue, the mass percentage of the mineral component can vary from 60% to 95%.224,225 In bones and dentin, the mass percentage of the mineral component reaches often 65% to 70%, respectively;226 the most prevalent protein in HAP-composite materials is collagen, which functions as a scaffold for the mineralization. HAP is also the main mineral component of dental enamel; here, the proteins amelogenin and enamelin act as scaffolds for mineralization. The inorganic phase in enamel is with 95% significantly higher than in other HAP-containing materials in the human body. The latter is the hardest human composite material and functions as a protection against mechanical, chemical, and thermal forces.225
The mineralization process in bone, dentin, and enamel is further assisted by various non-collagenous proteins, which modulate the growth of HAP crystals. Osteocalcin (OC) is after collagen the most abundant protein in the organic phase of bones. OC's structure is highly conserved and contains three γ-carboxylated Glu residues. The interaction of OC and calcium ions was investigated by NMR analysis and molecular docking simulations, revealing that the negatively charged protein surface coordinates calcium ions complementary to the position of calcium within a hydroxyapatite crystal.227
Another group of non-collagenous proteins are phosphoproteins from the small integrin-binding ligand, N-linked glycoprotein family (SIBLING),228 namely osteopontin (OPN), bone sialoprotein (BSP), dentin matrix protein 1 (DMP1), dentin sialophosphoprotein (DSPP), and matrix extracellular phosphoglycoprotein (MEPE). The latter proteins are essential in the modulation of the HAP mineralization process and have been extensively reviewed.219,228,229 All SIBLING binding proteins bind to HAP through negatively charged amino acids, namely Glu and Asp. The acidic, Ser- and Asp-rich motif, referred to as ASARM-peptide, is a fingerprint sequence (RDDSSESSDSGSSSESDGD), that is commonly found within proteins of the SIBLING family and facilitates binding on HAP. Common and repetitive sequences found in these proteins are DSS or ESS in which serin residues are often phosphorylated.229 A common feature of proteins of the SIBLING family is the transition from random coils to structured forms in the presence of HAP.230,231 Posttranslational modifications in the form of phosphorylation are a vital part in tuning the binding affinity towards HAP, as additionally introduced negative charges can strengthen the interaction with the mineral surface.229 A study from Addison et al.232 examined the binding of the phosphorylated and non-phosphorylated MEPE-ASARM motif on HAP. The binding on the mineral was greatly enhanced when the Ser residues 12, 14, and 16 were phosphorylated, proving that posttranslational modifications and negatively charged residues are essential for binding on the HAP mineral.
Statherin is another notable protein associated with the growth and regulation of HAP biomineralization, especially in tooth enamel.233 Statherin contains the acidic motif (DSSEE) at its N-terminus, which is remarkably similar to those found in SIBLING proteins. While statherin is disordered in aqueous solutions,234 it adopts, after binding to HAP, an alpha helix structure in its N-terminal HAP-binding domain as proven by solid-state NMR.234,235
Silica
Silica or silicon dioxide (SiO2) is a biomineral produced through the polymerization of orthosilicates (Si(OH)4) and can be found in the cell walls of diatoms, sponges, and plants.236 The yearly production of biogenic silica has been estimated to be in the range of 10–40 Gt.237 Enrichment, formation, and deposition of silica for different organisms has been described in several reviews.236,238,239 In contrast to carbonate and apatite, biomineralized silica occurs only in its amorphous form. In aqueous solutions silica is hydrated, which results in the formation of silanol groups. The surface of the silica is negatively charged under neutral or basic conditions, due to the deprotonation of the silanol groups.240 Crystalline silica can be found for example as quartz in rocks or sand. The following paragraphs summarize key proteins and molecular principles that govern the silification process. In diatoms silaffins play a key role in the biomineralization of silica and are characterized by highly repetitive sequences, rich in Ser, Tyr, and basic amino acid residues.241 Silaffin-1 from Cylindrotheca fusiformis was the first isolated protein associated with the mineralization of silica; it consists of seven highly homologous repeats (R1–R7) at its C-terminus with Lys–Lys and Arg–Arg clusters, as well as Thr and Ser residues.241 The latter repeats are proteolytically cleaved resulting in the release of seven individual peptides (R1–7).
Peptide R1 consists of 33 amino acids and includes the sequence of silaffin-1B (SSKKSGSYYSYGT). The sequence of silaffin-1A (SSKKSGSYSGS) is identical to the first 11 amino acids of the repeats R2 and R3–7, which are 22 and 19 amino acids long, respectively. The latter peptides undergo posttranslational modifications, and every Ser residue is phosphorylated. In addition, Lys residues in silaffins are widely modified with long chain polyamines (LCPA), which consist of linear oligopropyleneimide chains connected with propylenediamine, putrescine or spermidine.242 These modifications enable a combination of cationic and hydrogen bonding interactions to silica particles. In combination with the negatively charged phosphate residues, these proteins exhibit a zwitterionic character, which has been deemed to be essential for the self-assembly process during silica formation.243 A fully modified peptide from the silaffin-1A fraction contains 8 phosphate groups and a high number of positive charges, which are highly dependent on the specific LCPA that are added.244 The effects of these modifications on the silification process were further investigated through simulations of the R5 peptide245 and precipitation experiments246 leading to the conclusion that the main driving forces of biosilification are electrostatic interactions.243 Another group of proteins associated with the formation of silica in diatoms are silacidins, which are in contrast to the rather positively charged silaffins, rich in Ser and negatively charged amino acids. Silacidins also contain R–X–L motifs indicating endoproteolytic cleavage, as well as a high degree of phosphorylation at Ser residues of more than 60%.247 Comparing silaffin-like proteins from different diatom species showed no sequence homology, but it could be observed that Lys and Ser residues are highly abundant.241,248
The biosilification in sponges is catalysed by the enzyme silicatein, which forms proteinaceous filaments.249 Silicatein is the mayor silicifying enzyme in sponges with a catalytic triad consisting of Ser, His, and Asn. The proposed mechanism relies on hydrogen bonding between the imidazole of the His residue and the Ser in the active site.250 Silicatein is hypothesized to use orthosilicic acid as a substrate to form cyclic silicic acid species which are more reactive and, therefore, greatly accelerating the process of silica polycondensation.251
Aside from silicateins, sponges also possess other proteins involved in biomineralization processes. The protein glassin from the marine sponge Euplectella aspergillum, is another example of a silica-binding nMBP. Glassin consists of three distinct domains and has a high His content of about 30%. The first domain is rich in His and Asp residues (HD-domain), the second is characterized by Pro residues (P-domain), while the third is again rich in His and Thr residues (HT-domain).252 In contrast to other biosilica associated proteins, it was observed that glassin is not dependent on posttranslational modifications to facilitate its silica-forming activity.253 Further research by Arima et al.252 showed that the HD-domain is the primary compound in facilitating the silica formation. It was hypothesized that the proximity of His and Asp residues results in the constant protonation of the imidazole groups through a charge relay effect.254 The condensation of silica is then accelerated through the formation of hydrogen bonds and electrostatic interactions between the mineral and protein.255
While diatoms and sponges are the main sources of biogenic silica, other organisms also use silica biomineralization processes to facilitate specific tasks. One example is the spores of the bacterium Bacillus cereus, where the protein CotB1 was identified to be responsible for the interaction with silica. The protein contains a silacidin-like sequence in the C-terminal region followed by 14 amino acid sequence rich in Arg, which results in a protein with a zwitterionic character.256
In addition, a silica precipitating protein called siliplant1 was also reported in plants (Sorghum bicolour).257 Siliplant1 contains seven repeating units, which can be divided into the domains A, B, and C. Domain B contains Pro, Lys, and Glu residues, which results in the formation of a zwitterionic structure. Domains A and C flank the domain B in certain repeats of the protein. Domain A is rich in His and Asp residues resulting in a negatively charge at physiological pH. High occurrences of Pro, Thr and Tyr are a characteristic of domain C. At the end of the repeats 1, 3, and 5 R–X–L motifs can be found, which indicate cleavage. Kumar et al.257 suggested, based on Raman and NMR spectroscopy results, that the interactions occur between the carboxyl groups of the protein and the hydroxyl groups of the mineral.
nMBP protein-based materials
Proteins are diverse macromolecules with extraordinary properties including catalytical and structural functions. The latter can be attributed to ∼20 genetic encoded amino acid building blocks, the corresponding tremendous protein sequence space (20n; n number of amino acids; for n = 100 ∼ 1.268 × 10130) and two defined secondary structure elements (helices, β-strand), which jointly enable to generate a defined three-dimensional structure with diverse interaction possibilities.258,259
In the following paragraphs, three abundant and broadly used protein-based materials (collagen, keratin and silk) that form strong fibre-type structures based on self-assembly will be presented. Emphasis will be given on specific MBPs and hydrophobic, H-bond and charged interactions that drive the self-assembly process. Properties of collagen and their application in food, medicine and skin care are summarized in several reviews.260–264 Collagen showed a worldwide market value of 720 million US$ in 2017, and it is expected to rise to 1.453 billion US$ in 2025. Properties of keratin and their biomedical applications are summarized in several other reviews.265–269 The market size value of keratin was shown to be 1.47 billion US$ in 2022, and it is estimated to be 2.4 billion US$ in 2023. Properties of silk and its application in common and specialized textiles, such as biomedical and healthcare textiles, are summarized in additional other reviews.270–272 Silk possessed a market valued of 15.6 billion US$ in 2021, with a projection of 34.1 billion US$ by 2031.
Collagen
Collagen can be divided into 20 collagen subtypes.260 In general, collagen proteins structurally form triple helices, in which three helices intertwine (Fig. 14a). Collagen can be found in all types of connective tissues as skin, bones, and cartilage. It can either be fibril forming or network forming. While one tropocollagen protein (basic unit) is 3000 Å long and 13–15 Å wide (∼300 kDa),273–275 the higher formed fibrils can range from 10 to 100 μm in length.276 On a molecular level, the two most abundant motifs are G–G–X and G–X–hydroxyproline (HyP), whereby X could be any amino acid.277 Found binding peptides often mimic a collagen motif with the above-mentioned sequences to achieve the same molecular interactions as self-assembly. Self-assembly refers to the spontaneous and non-covalent assembly of protein molecules into higher-order structures, and it is used in synthesized cyclic and branched peptides for collagen detection.278 Gly usually introduces flexibility, and HyP with its hydrophobic character above neutral pH-values supports through hydrophobic interaction a triple helix formation. Kar et al. reported that aromatic amino acids and their interactions with Pro and HyP promote the self-assembly of collagen.279
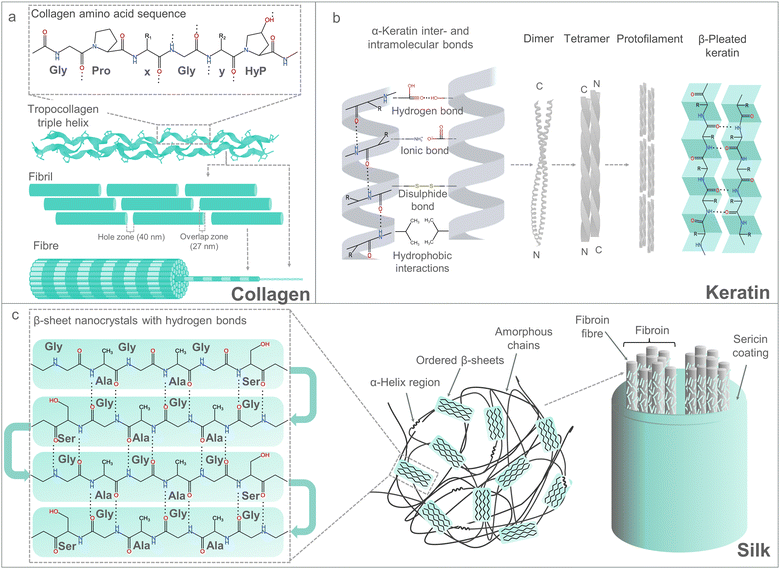 |
| Fig. 14 Overview of natural protein materials, such as collagen (a), keratin (b) and silk (c). Material composition and molecular organization are depicted starting from amino acid composition to filament or fibre assembly. Hydrogen bonds are displayed as dotted lines.280,281 | |
In addition to the self-assembly motifs (G–G–X, G–X–HyP), sequence motifs have been reported from different collagen-binding proteins as fibronectin282 or the glycoprotein “bone sialoprotein” (BSP),283 which do not show any similarity to the G–G–X motif; identified binding sequences are e.g. “CQDSETRTFY” or “NGVFKYRPRYYLYKHAYFYPHLKRFPVQ”, respectively. The latter 28 amino acids peptide sequence has a high content of aromatic amino acids including Tyr (6x Tyr), Phe (3x Phe), and Pro (3x Pro), as well as positively charged Arg (3x Arg). BSP binds to collagen with a dissociation constant of 5 × 10−7 M. The main binding interactions were reported to be with sialic acids or N-acetylneuraminic acids (Neu5Ac), which are added to collagen in post-translational steps. In one study, Neu5Ac was removed by treatment with neuraminidases, which decreased the binding affinity of BSP.284 Different studies discussed the binding of acidic non-collagenous proteins to the 40 nm hole zones of native type I collagen (Fig. 14a).284–287 Therefore, it can be concluded that the hole zones in collagen type I are potential target interfaces with acidic or negatively charged amino acids. Interestingly, the latter charged interactions in collagen hole zones differ from the self-assembly interactions in the G–G–X and G–X–HyP motifs of helices, which are driven by hydrophobic interactions and hydrogen bonds.
Keratin
Keratin is another important structural protein that can be found in nails, feathers, reptile scales, epidermis and in any form of animal hair. Keratin derived from human hair naturally consists of 7.6% Cys.269 The high Cys content leads the formation of many intra- and intermolecular disulphide bonds. Keratin forms fibres by self-assembly (see collagen) that often range in length between 100 μm and a few cm (Fig. 14b).267,288 Keratin can be grouped into hard and soft keratin, whereby hard keratin has more Cys, and therefore, also disulphide bonds between the helices. The latest studies suggest that hard α-keratin forms coil–coil helices, which are further assembled into oligomers (tetrameric oligomers) (Fig. 14b).289 A high Cys content could also be found in their corresponding binding peptides. In the case of keratin, no consensus binding motif has to the best of our knowledge been reported. All reported peptides, which were found to bind to keratin have a 20–45% content of Cys.290 In a study investigating the use of keratin-binding peptides as biological hair straightening modulators, eight keratin-binding peptides have been reported.291 The three peptides PepE, PepG and KP (CLPCLPAASC, CQCSCCKPYCS, GGVCGPSPPCITT) were found to straighten the hair by binding to keratin as chemical hair modulators. PepG was found in MD simulations to have a predisposition to form intramolecular disulphide bonds because of its five Cys. Binding of PepE, PepG and KP to a hair model until 120 °C confirmed disulphide bond formation, or rearrangements during the process.290 In summary, disulphide bonds are part of the self-assembly process of keratin substructures, which can also be supported by designed keratin-binding peptides.
Silk
Silk fibres obtained from silkworms have been used since ancient times for manufacturing fabrics due to beneficial properties as high strength and durability, without compromising shine and lustre. The latter resulted in its use as surgical meshes, biosensors, and even in drug delivery.292–294 The unique strength properties of the natural silk fibre from silkworms can be explained by its structure and composition with two main protein components: fibroin and sericin (Fig. 14c).
Fibroin forms the core of silk fibres and consists of three subunits: a heavy chain (H-fibroin), a light chain (L-fibroin) and the glycoprotein fibrohexamerin (Fhx, previously known as P25). H- and L-Fibroin are connected through covalent disulphide bonds, while Fhx interacts with H- and L-fibroin through non-covalent interactions.295 H-Fibroin is mainly made of the repeating motif G–A–G–A–G–X, in which X can be Ser, Tyr or Ala. The repeating motifs interact with each other through hydrophobic interactions and hydrogen bonds, consequently enabling the self-assembly of the protein through a hydrophobic core with a β-sheet structure (Fig. 14).296 Similar to collagen, described peptides that bind to silk fibres comprise the basic fibroin repeating motif G–A–G–A–G–X.297 L-Fibroin represents the hydrophilic part of fibroin with a heterogeneous amino acid composition and no repetitive sequences;298 binding of L-fibroin to H-fibroin is achieved through disulphide bonds. In addition, Fhx has a bridging function that connects H/L-fibroin dimers through hydrophobic interactions, although, until now, modes of binding have not been elucidated.
Sericin is a water-soluble glycoprotein with a molecular weight ranging from 20 to 400
kDa, and it acts as an adhesive coat for fibroin chains. In the textile industry, it is estimated that more than 400
000 tons of dry cocoons are harvested worldwide annually, from which 50
000 tons of sericin are discarded after a degumming process.299 Degumming is the process of removing sericin from the silk fibres by incubating them in hot water (50–60 °C) or in an alkaline solution, in which sericin is degraded and separated from the hydrophobic fibroin fibres.300 Sericin is mainly composed of Ser (>30 mol%), Gly (∼15 mol%) and Asp (∼13 mol%), depending on the origin organism and the analysed sericin fraction.301 The main conformation of sericin is a random coil, ensured through the high number of Gly residues, while charged residues such as Asp contribute to the hydrophilicity of the protein. The gluing properties of sericin have been explained by an interplay between Gly of fibroin and Ser of sericin.300 In the interaction, mainly driven by hydrogen bonding, Gly and Ser form a highly dynamic fibroin–sericin complex.302 Still, macromolecular protein conformations that provide a comprehensive understanding of interactions between fibroin and sericin have not been reported yet.
Table 1 Sequences that bind to naturally occurring polymer materials. Sequence properties are based on amino acid characteristics like charge, hydrophobicity, and aromatic structures
Material |
Sequence |
Charge |
Hydrophobicity |
Aromatic |
Ref. |
Silica |
SSKKSGSYSGYSTKKSGS |
4 |
−26.5 |
2 |
242
|
Silica |
SGRARAQRQSSRGR |
5 |
−29.1 |
0 |
256
|
Hydroxyapatite |
DSSEE |
−3 |
−12.1 |
0 |
303
|
Hydroxyapatite |
ESQES |
−2 |
−12.1 |
0 |
304
|
Hydroxyapatite |
QESQSEQDS |
−3 |
−23.4 |
0 |
304
|
Hydroxyapatite |
PLEPRRREVCELNP |
0 |
−18.0 |
0 |
227
|
Hydroxyapatite |
DDEDDDD |
−7 |
−24.5 |
0 |
219
|
Hydroxyapatite |
DDDDDDDDD |
−9 |
−31.5 |
0 |
219
|
Hydroxyapatite |
EETEEE |
−5 |
−18.2 |
0 |
305
|
Hydroxyapatite |
EEEGEEEE |
−7 |
−24.9 |
0 |
306
|
Hydroxyapatite |
EEEEEEEEEE |
−10 |
−35.0 |
0 |
306
|
Hydroxyapatite |
DDSSESSDSGSSSESDGD |
−7 |
−32.5 |
0 |
307
|
Hydroxyapatite |
DDSWDTNDANVVCRQLGA |
−3 |
−13.5 |
1 |
308
|
Calcite |
EEAQTELPQAR |
−2 |
−16.9 |
0 |
309
|
Calcite |
YRGPIARPRSSRYLAKYLKQGRSGKRLQKP |
10 |
−42.4 |
3 |
310
|
Calcite |
GKGASYDTDADSGSCNRSPGYLPG |
−1 |
−24.2 |
2 |
310
|
Aragonite |
DEADEADADEADADEAD |
−11 |
−27.7 |
0 |
311
|
Aragonite |
DDEDDDDDDDD |
−11 |
−38.5 |
0 |
312
|
Collagen |
CQDSETRTFY |
−1 |
−13.2 |
1 |
282
|
Collagen |
NGVFKYRPRYYLYKHAYFYPHLKRFPVQ |
8 |
−25.4 |
6 |
283
|
Collagen |
LRELHLNNN |
1 |
−10.3 |
0 |
313
|
Keratin |
CCQSSCCKPSC |
1 |
1.1 |
0 |
290
|
Keratin |
CVSSCCKPSCC |
1 |
8.8 |
0 |
290
|
Keratin |
PIVCRRTCYH |
3 |
−2.1 |
1 |
290
|
Keratin |
DCKLPCNPCA |
0 |
−1.0 |
0 |
290
|
Keratin |
CLPCLPAASC |
0 |
14.7 |
0 |
290
|
Keratin |
CEPAICEPSC |
−2 |
2.8 |
0 |
290
|
Keratin |
CQCSCCKPYCS |
1 |
0.6 |
1 |
290
|
Keratin |
GGVCGPSPPCITT |
0 |
5.5 |
0 |
290
|
Binding promiscuity of naturally occurring binding peptides
Synthetic polymers have been introduced into our daily lives since the second half of the 19th century, with larger scale production starting in the 1950s. Today, the renouncement of plastics would not be devisable, since it is found in many daily life goods and consumables as for packaging or consumer electronics. Six polymers account for the vast majority (95%) of all plastics (polyethylene (PE), polypropylene (PP), polyvinyl chloride (PVC), polystyrene (PS), polyethylene terephthalate (PET) and polyurethane (PUR)). Approximately 77% of all produced plastics are non-functional polymers, that present a pure carbon backbone (PP, PP, PVC, PS). Polymers with functional bonds such as in PET or PUR constitute for ∼18% of global production, and over 400 additional man-made polymers account for ∼5% of the total plastics production.314,315 ‘Fibre plastics’ are mainly based on polyester polymers, like PET.314
In contrast to the numerous examples, that were presented above, nature does not provide peptides to interact with synthetic polymers. This is mainly caused by the fact, that nature has not been exposed to these polymers long enough to develop binders. Additionally, man-made polymers do not present easily utilizable growth substrates, since non-functional polymers can hardly be broken enzymatically. The absence of proprietary binders for man-made polymers, however, does not imply that there are no proteins which bind to these polymers. In this section, we summarize the research on binding peptides for man-made polymers with a focus on the most abundant ones.
Polypropylene and Polyethylene
Polypropylene (PP) and polyethylene (PE) are hydrocarbon polymers with excellent chemical resistance, stiffness and processability.316 PP is therefore vastly used including automotive parts317 and textiles as carpets or clothing.318 The polymer was first identified in 1954 and accounted for 16% of the worldwide plastic market in 2020. Rübsam et al. identified the peptide LCI (47 amino acids) as a PP-binding peptide that forms a dense and stable monolayer at ambient temperature from an aqueous solution with prior PP surface activation and 1 g was reported to be sufficient to cover a surface of >600 m2.319 The LCI peptide originates from Bacillus subtilis, and it is composed of four antiparallel β-strands with a hand-shaped structure.320 Only the hydrogen bonds between the β-strands are responsible for the LCI structures, which enables to flexibly engineer the interface above and below the β-stands. The antimicrobial peptide Cecropin A was identified as a PP binder in the same study. Cecropin A (37 amino acids) originates from Hyalophora cecropia and has a random coil structure in solutions, which changes into a defined helical structure in bacterial membranes.321 More recently, the peptide Tachystatin A2 (44 amino acids) was reported to efficiently bind to PP.322 The peptide originated from Limulus polyphemus.323 It is structurally composed of three antiparallel β-strands and stabilized by intramolecular disulphide bonds.324 In addition, adsorption of proteins like hexokinases and lipases to PP was previously reported,325,326 however, the region that governs the enzyme surface interactions were not described. Besides, all the reported enzymes do not have a natural binding domain for PP. In case of PE, no interacting peptides or domains were identified so far.
Polystyrene
Polystyrene (PS) is a non-functional carbon-backbone polymer with aromatic sidechains. The orientation of the rings determines the tacticity of the polymer. For isotactic PS, all aromatic rings are oriented to the same direction, while syndiotactic PS shows an alternating orientation of aromatic rings along the chain. Atactic PS does not feature defined repetition schemes with the rings being oriented randomly between two sides. This is the standard tacticity found in PS tacticity influences the melting temperature of the polymer as well as the possible crystallinity.327 In 2018, the annual PS production was estimated at 35 million tons with a growth rate of 5% per year.328 The peptides Cecropin A, LCI, and Tachystatin A2 bind also to PS surfaces.329 Binding was achieved in fusion proteins which will be further discussed in the Section “Emerging applications of material-binding peptides”.
Tachystatin A2 showed a preferential binding in C-terminal fusion while Cecropin A and LCI bound more efficiently in N-terminal fusion. The two adhesive surface proteins RspA and RspB from Erysipelothrix rhusiopathiae were found to bind PS surfaces (83–92 amino acids). RspA and RspB proteins have a key role in biofilm formation due to their binding to proteins of the extracellular matrix. A C-terminal binding motive (LPXTG) could be identified for both peptides, which is preceded by a repeating consensus sequence.
AltE was identified as a prerequisite for biofilm formation of Staphylococcus epidermis.330 Two domains from AltE, R2ab domain and amidase domain were further analysed. It was found that both domains were capable of binding PS nanoparticles, as well as macromolecular PS surfaces. Furthermore, circular dichroism analysis showed that the binding process induced a partial unfolding of the protein.331 Like for PP, the attachment of several proteins to PS was observed. However, this attachment can again be classified as unspecific, since no exact domains for binding could be identified.332
Polyethylene terephthalate
Polyethylene terephthalate (PET) presents a more complex structure than the previously mentioned hydrocarbons (PP and PS). The polymer is formed by the esterification of ethylene glycol and terephthalic acid, or by transesterification of ethylene glycol and dimethyl terephthalate.333 PET may occur in two states: amorphous and semi-crystalline. The crystallinity alters transparency, as well as thermal and mechanical properties of the polymer.334 PET is the most commonly used polymer in the polyester family and it is mainly applied in the textile and food industry.333 56 million tons of PET were produced yearly by 2013, leading to extensive disposal of the polymer into the environment, and an ever-increasing production of monomers from raw petroleum.335 Since PET is very common in daily life, and the included bonds can be effectively broken enzymatically, substantial research has been performed regarding the degradation and binding to PET, using nMBPs with promiscuous binding to this polymer as binding domain in protein fusion constructs with PET depolymerases. Ribitisch et al. fused a cutinase from Thermomyces cellullosyliticia to two binding domains, originating from cellobiohydrolase I from Hypocrea jecorina (CBM), and polyhydroxyalkanoate depolymerase from Alcaligenes faecalis (PBM). This cutinase represents one example of an enzyme capable of degrading PET. The amount of cutinase adsorbed to the PET surface was increased by 51% for the CBM fusion, and almost doubled for the PBM fusion when measured using quartz crystal microbalance coupled to dissipation monitoring (QCM-D). The results were confirmed by a chemiluminescent reaction using horseradish peroxidase. Finally, the fusion of the enzyme to the binding domains resulted in enhanced polymer hydrolysis.336 In a similar approach, the chitin-binding domain from Chitinolyticbacter meiyuanensis was fused to leaf-branch compost cutinase. The fusion resulted in increase in degradation by 19.6%. After fusion to the chitin-binding domain, PET depolymerization was even possible for highly crystalline PET of 40% crystallinity.337 Besides these larger domains derived from hydrolytic proteins, smaller peptides that bind PET were reported. Within the study by Dedisch et al., which was mentioned above, the peptide Tachystatin A2 was identified as a potent binder to PET. The amount of immobilized protein on the PET surface, as well as the activity of proteins fused to TA2, could be enhanced for three different fusion constructs. This increase was reported higher as compared to the binding peptides LCI and Cecropin A.338 Binding of smaller peptides to PET was used in a study by Büscher et al. to facilitate the immobilization of phenolic acid decarboxylase, which is described in more detail in Section “Emerging applications of material-binding peptides”. The peptide Dermaseptin S1 showed the best binding performance in this investigation, increasing the ferulic acid conversion by more than 100%. Dermaseptin S1 is an antimicrobial peptide, originally identified from amphibian skin. It consists of 34 amino acids and it is proposed to have a 80% helical secondary structure, in the form of one amphiphatic helix when exposed to a hydrophobic milieu.339 Dermaseptin S1 was also used to immobilize cutinase Tfuc2 on PET, as it was performed before with CBM and PBM domains. This application resulted in a 22.7-fold increase in degradation, as compared to the Tfuc2 itself.
Polylactic acid
Polylactic acid (PLA) is another thermoplastic polyester, which is obtained by the condensation of lactic acid monomers. The morphology of PLA can range from amorphous to highly crystalline polymers, with basic physical properties similar to PS or PET. Since lactic acid is a chiral molecule, a number of distinct polymers can be formed including poly-L-lactide (PLLA), poly-D-lactide (PDLA) and poly-DL-lactide (PDLLA). PDLLA results from the polymerization of racemic mixtures of lactic acid monomers. PDLA typically occurs in amorphous form, but the mixing ratio of D- and L-monomers can be used to determine the degree of crystallinity. The mixture of PDLA and PLLA results in a complex with high crystallinity.340 In contrast to the polymers that were previously mentioned, PLA is a bio-based, as well as, a biologically degradable polymer. It already takes up the biggest market share in biodegradable plastics and it is expected to show further growth.341 Besides many applications in medicine as the fabrication of scaffolds for tissue engineering,342 PLA is commonly used for packaging of food and other daily-life goods.343 There is not much data on peptides or proteins that bind to PLA. Lu et al. identified the material-binding peptide Cg-Def to efficiently bind PLA, and performed protein engineering to increase the peptides specificity to the polymer.344 More details on the engineering campaign are given in the next section. Cg-Def is a defensin derived from Crassostrea gigas. The secondary structure was reported to present a Cys-stabilized α-β motif, and the protein weight was determined to be at 4.6 kDa. Investigation by Gueguen et al. suggested that Cg-Def is crucial for the initial repelling of pathogens, and that it is therefore continuously expressed in the oyster mantle.345 Using computational analysis, the interaction between Cg-Def and the PLA polymer was proposed to rely on non-charged amino acids in two turns of the peptide structure.344 PUR and PVC represent additional polymers, that are commonly used and produced in high quantities. While PVC shows simple and similar structures, the structure of PUR features organic units, that are joined by urethane links.346 To the best of our knowledge there are no reports on natural binding domains, that show binding promiscuity to these three polymers.
In summary, no material-specific binding peptides for the most common man-made polymers have been reported or found in nature yet. Identified binding peptides show promiscuous binding to chemically similar polymers, however, a first encouraging study on Cg-Def for improved material-specific binding to PLA, and improvements in binding strength of the LCI peptide for PP,347 demonstrated the potential to develop the material-specific binding peptides by protein engineering.
Engineering of binding peptides and proteins
Protein engineering is a valuable tool that offers the possibility to further evolve functions of proteins. In the realm of eMBPs, this entails the binding strength or the material specificity. eMBPs can be divided into class I (<20 amino acids) and class II (20–100 amino acids). Furthermore, class II eMBPs often possess, in contrast to eMBPs class I, a defined 3-D structure. Depending on the class of eMBP, different engineering approaches can be applied to tailor the properties of eMBPs (Fig. 15). Phage display is the preferred method to obtain eMBPs of class I. The phage display of peptides and antibodies was awarded the Nobel Prize for chemistry in 2018, as it represented a breakthrough that allowed for the directed evolution of very short binding proteins via expression and selection on the surface of phages, thereby achieving a physical coupling between a phenotype and the respective genotype.348 One limitation here is that the size of the displayed peptides is often limited to <16 amino acids.
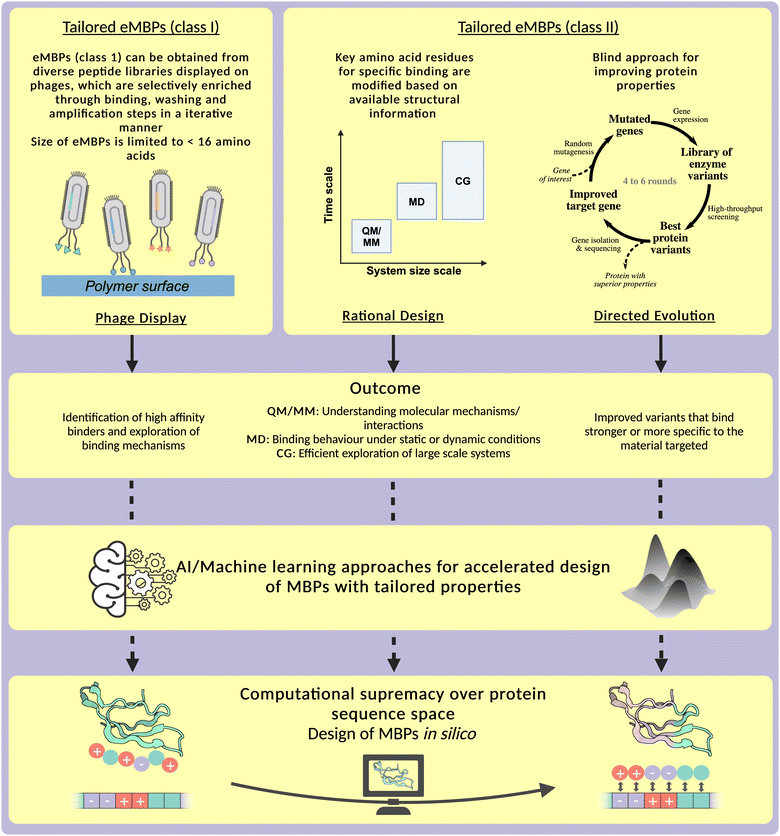 |
| Fig. 15 Overview on research fields and methodologies of protein engineering comprising phage display, rational design, and directed evolution that have been employed in the engineering efforts of eMBPs, including outcomes and contributions to provide the experimental data and biophysical knowledge to efficiently explore the gigantic protein sequence space. Phage display methodologies are till today mainly used to identify short peptides for diagnostic applications and are the method of choice for engineering eMBPs of class I. Here, a genotype phenotype-linkage between the displayed peptide (phenotype) and the corresponding genetic information (genotype) is achieved by fusing libraries, containing vast amounts of different DNA-sequences, to the capsule proteins of phages. Afterwards, the phages can be employed in a high-throughput screening procedure referred to as biopanning. During biopanning the phages are first applied on the surface of interest, followed by several washing steps to wash away weak and unspecific binders. Phages that are still attached on the surface display strong binding peptides and are subsequently recovered and amplified in a bacterial host. The cycle can then be repeated several times leading to an enrichment of the strongest binding sequences. eMBPs of class II can be engineered by rational design and directed evolution or combined methods such as KnowVolution. Rational design of eMBPs aims to simulate surface interactions based on structural data. Commonly used simulation methodologies can be performed on different time and system size scales; for instance, quantum mechanics and molecular mechanics (QM/MM), which are typically used to model protein–protein interactions or reaction mechanisms, molecular dynamics (MD) for the in depth analysis of specific residues and their interactions, and coarse-grained (CG) modeling, to generate a more in depth understanding of larger scale systems and of the biophysical properties during the binding process. All rational design methodologies contribute, in combination with directed evolution methodologies, to elucidate binding interactions between eMBPs and material surfaces. Directed evolution as a ‘blind’ approach does not require any structural information nor a molecular understanding or a hypothesis in contrast to computational methodologies. Key steps in directed evolution are random mutagenesis of the gene of interest (gene level), high-throughput screening of encoded variants (one the protein level; usually 1000–3000 variants) to identify beneficial variants, which are subsequently sequenced to determine beneficial amino acid substitutions. In traditional directed evolutions experiments the best variant of each round is used as input for the next round of directed evolution; traditionally 4 to 6 rounds have been used to generate efficient enzymes. | |
The key steps of phage display comprise the generation of peptide libraries, the cloning of libraries into the phage genome, and finally the selection of binding sequences through biopanning. Libraries that are generated for phage display experiments can reach a diversity of up to 10−11 different sequences, which are then fused with coat proteins of phages, which enables the display on the surface.349,350 Due to these large library sizes, it is for instance possible to find antibody variants with dissociation constants in the low nM range.351 The next step, after the generation of a phage display library is the iterative process of biopanning. Here, the phages are incubated on the material of interest, followed by several washing steps to get rid of unspecifically or weakly bound clones. The phages that are still bound on the material are eluted and amplified in Escherichia coli, which marks the starting point for the next round of biopanning. After several rounds, the best variants can be isolated and sequenced, as well as further analysed, leading to the identification of high-affinity binding motifs and the exploration of the underlying binding mechanisms.352 Protein engineering methodologies can also be used for the tailoring of eMBPs class II. Here, rational design, directed evolution, as well as combined approaches can be applied.
Rational design methodologies can be categorized in protein sequenced based alignments/analysis, which are computationally not demanding, or computationally more demanding structure-based modelling approaches.353 Approaches, which align or analyse sequences can help to find proteins with related structure or function. A method called consensus design can be used in this context to generate stable protein variants from evolutionary related proteins by combining the most frequent residues at every position. On the other hand, structure based computational design evaluates protein properties and functionality based on structural traits. Structural motifs can be retrieved in unrelated proteins and indicate the protein functionality. QM/MM methods are typically used to model protein–protein interactions or reaction mechanisms, since they cover the smallest time and size scale.354 An application of QM/MM methods for the engineering of material-binding peptides has not been reported so far. In-depth, analysis of specific residues and their interactions is possible by applying substrate docking or molecular dynamics simulation. This elucidates the interactions with binding partners or other interacting molecules. A more detailed explanation in the context of binding peptides can be found in the Section “Discussion and general lessons learned from nMBPs and eMBPs” on computationally assisted design. Furthermore, coarse-grained modelling can be used to explore large scale system more in depth, and analyse their behaviour over long-time spans. Through the rational design of material-binding peptides and proteins, it is possible to generate a more in depth understanding of the biophysical properties during the binding process.
Protein engineering by directed evolution has advanced into a standard industrial “tool” to tailor naturally occurring proteins to a variety of biotechnological applications. This includes the sustainable enzymatic production of chemicals, pharmaceuticals, or tailored enzymes for food, feed, and laundry industries (see Noble Prize Chemistry 2018).348,355 Directed evolution comprises iterative rounds of diversity generation (e.g. by random mutagenesis methods such as epPCR, on the gene level) and screening the generated library on the protein level to identify improved variants against the applied selection pressure (e.g. improved specific activity, solvent or temperature tolerance). The main challenge in directed evolution is to efficiently explore the natural diversity of the theoretical protein sequence, which has been addressed with smart protein engineering strategies, such as KnowVolution,356 which combines computational analysis and experimental directed evolution results in order to minimize experimental workloads and maximize property improvements. Sequence space, which is an array of possible amino acid sequences, offers enormous diversity of functionalities and tailored design possibility. For 50 amino acid peptides, the number of potential peptide sequences would be 2050 which is a huge number (1.63 × 1065). Large sequence space results in exploration complexity with resource intensive laboratory work. A technical challenge for directed evolution of peptides in the eMBP size range of 30 to 120 amino acids, which require a mutation frequency of 20 to 50 mutations per 1000 bp instead of the usually employed mutation frequency of 3 to 5 mutation per 1000 bp. This was solved by developing the cepPCR357 and PepEvo1 mutagenesis protocols in the group of Prof. Schwaneberg. In general, after directed evolution improved variants of eMBPs class II are obtained which bind stronger or more specific to the material of interest and support the unravelling of the underlying interactions.
Combining the knowledge gained from the engineering efforts for eMBPs of class I and II (Fig. 15) enables the application of machine learning or AI-based algorithms to maximise improvements.358 A more detailed overview about the potential of AI/machine learning approaches within the design of specific binding peptides can be found in Section “Computationally assisted design of polymer-binding peptides”.
The final goal within the field of engineering material-binding peptides is the computational supremacy over protein sequence space (Fig. 15), which would enable the design of MBPs in silico. However, there are still many challenges left that, which will be addressed in Section “Discussion and outlook”.
In the following paragaphs engineering approaches to alter natural binding proteins towards carbohydrate-, mineral- and protein-materials, as well as synthethic polymers are presented with a description of the engineering campaign that was performed. Since these peptides (termed eMBPs, class II) are structurally highly diverse, mostly non-systematic approaches for engineering are reported. The application of these peptides is further described in the Section “Emerging applications of material-binding peptides.” Furthermore, advances in designing and identifying man-made phage-display binding proteins (termed eMBPs, class I) for the discussed carbohydrates, protein materials, minerals as well as metals and synthetic polymer will be presented and discussed in respect to their properties.
eMBP (class II) for carbohydrates
In the “Material-binding peptides, their material-specific interactions, and protein engineering campaigns to tailor binding properties” section, we introduced the main carbohydrates found in nature (cellulose, chitin, xylan, starch, chitosan, alginic acid, hyaluronic acid, heparin and polysialic acid) and natural occurring MBPs. Protein engineering campaigns, such as computational design combined with site-directed mutagenesis and directed evolution combined with phage display, have been performed to shift the binding specificity of CBMs from one carbohydrate moiety to another. The driving forces of binding affinity and specificity between CBMs and naturally occurring polymers have been further discussed.
The binding specificity shifting from chitin to cellulose was achieved by Nakamura et al. via combination of sequence alignment analysis and site-directed mutagenesis. As mentioned previously, ChBD2 from Pyrococcus furiosus shows specific binding to chitin but not to cellulose. Based on sequence alignment between ChBD2 and CBDCex from Cellulomonas fimi, the ChBD2E279T:D281N variant introduces polar residues Thr and Asn in place of the negatively charged Glu279 and Asp281 around the solvent-exposed aromatic side chain. These substitutions alter the chemical nature of the residues in the specified positions and increase the binding affinity to cellulose without affecting chitin binding. Intriguingly, the other variants ChBD2E279A:D281A and ChBD2E279T:D281S show significantly lower binding affinity to cellulose than variant ChBD2E279T:D281N, indicating specific interactions between Thr/Asn residues and cellulose ligand.76
Simpson et al. reported a transition in binding specificity from xylan to cellulose by structural comparison of binding sites from relevant CBMs and site-directed mutagenesis. CBM2b-1 from Cellulomonas fimi xylanase 11A binds specifically to xylan in a three-fold helical conformation with a rotation angle of approximately 120° between adjacent monosaccharides. The pivotal aromatic residue Trp259, crucial for stacking interactions, aligns with the three-fold helical conformation of xylan moieties. The introduction of the R262G mutation induces a Trp259 rotation of around 90°, causing a shift in binding preference from xylan to cellulose. The substituted Gly residue corresponds to G20 in CBM2a from Pseudomonas fluorescens Xyn10A and serves to maintain the orientation of the cellulose-binding aromatic residue Trp17.190 The xylan-to-cellulose binding shift emphasizes the importance of aromatic residues orientation for xylan specific binding.
Strobel et al. combined rational design and site-directed mutagenesis to investigate the binding specificity of cellulose-binding domain to lignin. Polar, hydrophobic, positively charged and negatively charged residues are introduced to cellulose-binding module, CBM1 from Trichoderma reesei Cel7A, respectively. It was found that the introduced hydrophobic residues (Q2L, H4V and Y5W) or positively charged residues (V18R, L28K and P30K) increased the binding specificity for lignin compared to cellulose.359 Lignin-binding driving forces are concluded as potential hydrophobic interactions and electrostatic interactions.
Except for site-directed mutagenesis, directed evolution combined with phage display also contributes to binding specificity transition. Smith et al. established a combinatorial phage display library for cellulose-binding module CBM1 from Trichoderma reesei cellobiohydrolase I Cel7A. The variant AP1 (H4L, Y5G, N29R, Y31W, Y32F, and Q34M) shows binding to glycoprotein bovine alkaline phosphatase, while maintaining unaltered enzyme activity and abolishing its original affinity towards cellulose. ELISA-based binding experiments with both native and denatured glycoproteins reveal that the ligands are proteins but not carbohydrate polymers. In addition, the variant AP1 exhibits no binding affinity to alkaline phosphatase when the residues Gln7, Arg29 and Trp31 are mutated to Ala, suggesting the importance of these three amino acids for the binding.360
The binding specificity of CBM1 shifting from cellulose to porcine pancreatic α-amylase (PPA) was reported by Lehtiö et al. Two variants (CBDPPA1:4 and CBDPPA1:5) are screened from the CBM1 library mentioned above, which are able to bind PPA, but not cellulose. The variant CBDPPA1:4 (I11M, S14P, P16E, T17L, V18R, N29H, L28S, V27K, P30G, Y31L, and Y32V) competes with inhibitor acarbose and binds to the active site.361 The screening outcomes of the CBM1 library, including AP1 and CBDPPA1:4, contain substitutions at residues Asn29, Tyr31, Tyr32, and Gln34. The elimination of cellulose binding resulting from these substitutions underscores the importance of aromatic and polar residues for cellulose binding.
CBM4-2 from Rhodothermus marinus xylanase Xyn10A has also attracted significant attention for CBM engineering combined with phage display and directed evolution. Gunnarsson et al. discovered multiple CBM4-2 variants with binding specificity towards birchwood xylan, Avicel, ivory nut mannan and human monoclonal antibody IgG4, respectively.362,363 Through the incorporation of mutants Q108R, D136G and E138A/G, the variant can specifically recognize IgG4 without binding to xylan and other carbohydrates. The unaltered binding affinity of the single mutant variants for xylan and IgG4 compared to the wild type indicates the importance of the assembly of these single mutations for developing binding specificity to IgG4.364 The variant XG-34 (W69Y, F110H, E72H, F76L, Q111D, E112D, and Y149F) was identified through screening the same combinatorial library of CBM4-2 for its specific binding to non-glycosylated xylan (XG) rather than xylan and β-glucan. The presence of soluble xylan as a selection pressure is essential to ensure successful screening of XG-specific binding variant.365 From the crystal structure analysis, the variant XG-34 maintains its original folding and binding site. A notable reduction in the distance between W69Y and F110H by 5.5 Å results in a significantly narrower binding cleft, thereby restricting xylan binding.366 It suggests that the steric hindrance within the binding pocket can influence the specific recognition of xylan moiety.
Gunnarsson et al. described an evolved CBM4-2 module, X-2, (W69F, D70N, E72Q, F76L, W91R, F110L, Q111D and E118H), with high binding specificity for xylans and no affinity for glucan-containing polysaccharides.367 Hydrogen bonds between E72 and E118 in wild-type CBM4-2 and xylan are disrupted by mutations, potentially altering the protein–ligand interactions.368 The mutation of Phe110, one of the two aromatic binding residues, results in the complete loss of wild-type binding to β-glucan, potentially impacting X-2 variant similarly.367 Interestingly, the X-2 variant, with an L110F mutation, regained the binding capacity to xyloglucan and β-glucan.369 Additionally, the Trp69 residue is deemed crucial for xylan ligand recognition, suggesting the important role of Phe69 in the binding of X-2.367
In addition to CBM1 and 4, the phage library was also established for RtCBM11 from Ruminoclostridium thermocellum CelH to screen out a variant with binding specificity shifting from linear β-glucan to branched xyloglucan. MD simulations reveal that the H102L/Y152F variant generated a xylosyl binding pocket within the binding cleft to accommodate polysaccharide branches. The hydrogen bond network between the protein and the ligand is altered with less stability, resulting in a shift in binding affinity towards xyloglucan.370
eMBP (class II) for minerals (calcium carbonate, hydroxyapatite, silica)
To our knowledge there are no protein engineering campaigns by directed evolution focusing on improving already existing binding peptides for binding on mineral. However, there are some interesting approaches utilizing biomimetic molecules. For example, non-natural polymers, called peptoids, can interact with calcite more efficiently than natural binding peptides. They have been engineered to include amphiphilic and anionic parts which have been shown to be critical to produce calcite in vivo.371
eMBP (class II) for protein-based materials
For protein-based based materials, such as collagen, keratin and silk, no protein engineering campaigns by computational methodologies and directed evolution have been performed to improve binding strength or material-specific binding. Most efforts are devoted to mimetically design and synthesize chimeric proteins, in which two different binding modules are fused to give new binding properties to different materials. For example, mimetic design and synthesis were performed for collagen-like peptide to bind collagen.278 In the case of silk, protein chimeras of silk consensus motif (GAGAGS), and bacterial collagen VCL, were generated to functionalize with collagen a silk surface. Here, based on the self-assembly process of silk (described in Section “Naturally occurring binding peptides (nMBPs) that bind to natural polymers and naturally occurring inorganic materials”), the GAGAGS sequence was used as a binding motif for silk functionalization.372 In general, studies use the self-assembly properties of protein-based materials, as described for collagen, keratin and silk to design new binding modules and functionalise surfaces.
eMBP (class II) for synthetic polymers
Even though nature has not been exposed to man-made plastics for a long time, there are still some natural proteins that show promiscuous binding affinities to synthetic polymers. Engineered nMBPs capable of binding towards synthetic plastics in a material-specific manner hold significant promise for material-specific detection, and enhanced degradation of target plastics in the plastics mixture. For synthetic polymers, such as PET, PP, PS and PLA, protein engineering campaigns by computational analysis and KnowVolution have been performed to enhance the binding strength or shift the binding specificity of nMBPs.
CBMs are fused to several PET-hydrolases (EC 3.1.1.101), to increase the binding affinity towards PET substrates.336,373–376 Rennison et al. conducted alanine scanning to unravel the driving forces contributing to the robust binding affinity of CBM2 from Bacillus anthracis to PET. Aromatic triplets (Trp9, Trp44 and Trp63) and polar residues (Ser7 and Asn14), which were previously recognized for their significance in chitin binding, also play key roles in PET binding. Additionally, Gly12 is recognized for regulating the planar conformation of the aromatic residue Trp9. Mutant G12R abolishes CBM2 binding affinity for PET, suggesting that a planar binding site is required for PET recognition.76,376
Material-binding peptides LCI and Tachystatin A2 (TA2) show binding towards polymers.319 To improve binding properties of such peptides for the functionalization of polymers, the peptide–polymer evolution protocol (PePevo) was established. It enabled the directed evolution of surface-binding peptides on different polymers,1 which led to an improved PP-binding variant of LCI, as well as a variant of the peptide TA2 exhibiting stronger binding to PS. To discover the mechanism of how these peptides interact with polymers, the binding of LCI towards PP was further improved with a KnowVolution campaign,356 where the binding strength in presence of the non-ionic surfactant linear alkylbenzene sulfonate was enhanced.329 Eight positions that influenced the binding towards PP were identified. It was observed that predominantly residues with negatively charged amino acids Glu and Asp were substituted. In addition, a trend of substitutions towards hydrophobic amino acids was described, indicating that hydrophobic interactions play an important role in the binding to PP. The peptide variant that showed the highest improvement in its binding capabilities was LCI Y29R/G35R, in which two residues were substituted with the positively charged amino acid Arg. In another approach, the binding of LCI to PP was improved using cell surface display in Escherichia coli.2 Here, the best binding variant (LCI E42V/D45H) had two negatively charged residues substituted with a hydrophobic and a positively charged residue, respectively.
KnowVolution campaign has also been used to engineer the material-binding peptide Cg-Def, to improve its binding specificity towards PLA over PS/PP. Cg-Def was chosen as the engineering model peptide, since Cg-Def shows the highest binding affinity to the PLA surface and the lowest binding affinity to the PS/PP surface, compared to LCI and Spinigerin.344,377 In detail, a Cg-Def random mutagenesis library was generated by Lu et al. to screen PLA specific binding peptide over PP. In total, 8 positions were identified with potential to improve PLA binding over PP. After site-saturated mutagenesis and positions combination, top three Cg-Def variants with the highest binding specificity to PLA over PP were screened out. Interestingly, the substitution positions among these three variants (Lys10, Asn13, Lys16, Ser19, Cys25 and Cys36) are mainly located in the α-helix of Cg-Def, and show mutation bias to hydrophobic or positively charged amino acids. The binding affinity of the best variant Cg-Def V2 (S19K/K10L/N13H) was further characterized by water contact angle measurement and SPR, resulting in a smaller water contact angle (more hydrophilic surface) and a higher surface coverage (1.3-fold), respectively, compared to the wild type.377 On the other hand, Cg-Def with improved binding specificity to PLA over PS was reported by Lu et al. to enhance PLA degradation in PLA/PS mixture in a specific manner. In detail, 4 positions (Leu9, Ser19, Cys34 and Cys36) were identified by KnowVolution campaign for the contribution of binding specificity to PLA over PS. They are mainly substituted with hydrophobic or positively charged amino acids, which is also observed when Cg-Def is engineered with enhanced binding specificity to PLA over PP. It indicates the importance of hydrophobic and electrostatic interactions for PLA binding. The binding specificity of the best variant Cg-Def YH (L9Y/S19H) to PLA was also checked over the other three plastics, PP, LDPE and PET. It showed different binding specificity between hydrophobic and hydrophilic plastics, with 2-, 2.1- and 0.8-fold improvement to PLA/PP, PLA/LDPE and PLA/PET, respectively. When it was fused with ICCG for PLA degradation, ICCG-Cg-Def YH had a lower KD value (191 nM) compared to the wild type of ICCG-Cg-Def (399 nM). ICCG-Cg-Def YH outperforming ICCG WT by 1.4-fold suggests the potential of material-specific binding for plastics specific degradation.344
Recently, immune response and generation of antibodies against synthetic plastic, such as PET, was detected in rats with polymer prosthesis segments implanted.378 Moreover, production of polyclonal polystyrene-binding antibodies after immunization of rabbits with polystyrene was reported.379 Generated anti-PS antibodies did not bind to polypropylene, providing confirmation of their specificity. Such antibodies were applied for immunoassay-based analysis of microplastics. Both studies demonstrated the feasibility of in vivo generation of plastic-specific antibodies.
Affibodies and nanobodies
Nature offers a wide range of protein scaffolds with a rigid core structure for developing new affinity ligands that allow the design of high affinity binding sites in a predictable, ‘stable’ and controllable manner with highly diverse arrangement of amino acids.380,381 As a result, dozens of binding protein scaffolds have been developed in recent years and are described in multiple reviews dedicated to development and applications of these molecules.382–389 Prominent protein scaffolds are DARPin,390 ANTICALIN®,391 adnectin,392 affibody,393,394 nanobody,395 Kunitz domain,396 lipocalin,397 avimer398 and knottin.399 Binding peptides based on binding protein scaffolds overcome the production and cost challenges of antibodies and offer advantages in applications where smaller size of binding molecules is required, i.e., for penetration into tissue. Two promising binding protein families, affibodies and nanobodies, are highlighted in the next two paragraphs due to their broad application potential, easy and cost-effective production, and rapid engineering possibilities.
Affibodies are based on the B-domain of the immunoglobulin-binding region of the staphylococcal protein A and composed of 58 amino acids (MW 6.5 kDa) forming three-helix structures. The mutated version of B-domain showing higher stability and selectivity to Fc-region of antibody is called Z-domain.393
Within affibodies, two helices form the binding surface and are responsible for recognizing specific epitopes on target molecules. To generate a library of potential binders with desired specificity and affinity, 13 amino acids located in these helices are randomized (Fig. 16). Affibody libraries are traditionally based on phage display libraries that encode a large variety (more than 109 variants) of affibody molecules likely with unique specificities, expanding the repertoire of potential targets that can be recognized. Bacterial,400 mRNA401 and ribosome402 display libraries are used as well for affinity maturation of affibodies.403 The binding of affibodies to target molecules is achieved through combination of molecular forces (van der Waals, electrostatic, hydrophobic interactions and hydrogen bonding) between amino acids in the affibody binding site and specific motifs (epitope) of the target. Diversified amino acids play an important role in binding, account for 80% of the buried surface area in the affibody and involve 62% of all residues that interact with the target.404 The binding interface of affibody-target complexes is comparable in size to binding interface of the antigen–antibody. Compared to antibodies with flexible complementarity-determining regions (CDR) loops, the binding region of affibodies is relatively flat and tends to recognize planar epitopes on their targets.405 Mutations introduced to enhance affinity for target compounds can impact the structural integrity or stability of affibodies in various environmental conditions.406 Therefore, diverse protein engineering strategies were proposed to achieve high-affinity affibodies without compromising structural stability including directed evolution, the protein repair one-stop shop (PROSS) in silico algorithm,407 gradient sitewise diversity generation and screening,408 and the introduction of intramolecular disulphide bonds.409 In recent decades, affibodies for over 40 targets, including amyloid β peptide, epidermal growth factor receptor, fibrinogen, interleukins, tumour necrosis factor, or insulin, were already generated for the applications in biomedicine for both in vitro (bioimaging, diagnostics) and in vivo (therapeutics) use.410,411 Nanobodies (VHH or single-domain antibody, 15 kDa) are binding proteins that are based on the variable domain of heavy chain-only antibodies found in camelids.412 The single-domain structure of nanobodies is stabilized by a beta-sheet core formed by several beta strands. The beta-sheet core provides structural rigidity and stability to the nanobody domain. Nanobodies possess specialized regions known as complementarity-determining regions (CDR1, CDR2 and CDR3) that play a crucial role in recognizing and binding antigens. CDRs are forming the antigen-binding site (paratope) of the nanobody, allowing direct interaction with the target molecule. Nanobody regions, located between the CDRs, named framework regions (FR), maintain the overall stability of the domain by providing structural support. These regions are generally way more conserved compared to the highly variable CDRs responsible for specificity.413 Through distinct amino acid sequences, the CDRs facilitate specificity by engaging in precise interactions with the target. These interactions include combinations of hydrogen bonds, van der Waals forces, and electrostatic attractions and are highly dependent on the target or epitope structure. For example, interaction between nonpolar molecule Ochratoxin A and specific nanobody Nb28 is ensured by amino acids Gly53, Met79, Ser102, Leu149 involved in formation of hydrophobic and hydrogen bonds. Importance of those residues was shown by alanine scanning. Affinity to Ochratoxin A was completely lost in case of Nb M79A.413 Interaction of polar Reactive Red 1 (RR1) dye with specific nanobodies occurs through hydrogen bonds between sulpho-groups of RR1 and OH-groups of Tyr56 and Thr50, NH3 group of Lys95, Gly100 main chain NH group and guanidinium groups of Arg58 and Arg52. In contrast to affibodies, the recognition site of nanobodies (600–800 Å2) is relatively flexible and can adopt to epitopes of different shapes, i.e. flat or concave. Moreover, convex paratopes of nanobodies are well-suited for recognition of cavities often inaccessible for conventional antibodies.414–416 Small molecules (mycotoxins, haptens), however, can be bound in the centre of a cavity formed by the three CDRs, in a groove formed between CDR2 and CDR3 or in the tunnel formed by CDR1 and one of the FR.417 Although production costs of nanobodies are relatively low, the development costs remain high compared to antibodies.418 To generate target-specific nanobodies, three types of libraries are used: immune, naive and synthetic. Phage display is most often used for selection of specific variants, but other display systems (bacterial, yeast, ribosomal) are employed as well. Nanobody databases include more than 700 sequences of nanobodies specific to over 50 targets. The targets include viral (HIV419 and COVID-19420) and bacterial (Salmonella sp.,421Escherichia coli, Staphylococcus aureus422) pathogens, cancer or inflammation related targets,423,424 toxins,425 hormones,426etc. Similar to affibodies, nanobodies are used in imaging, therapeutics and medical diagnostics. Dozens of nanobodies are investigated in clinical trials as therapeutics for inflammatory, infectious diseases, cancer, etc.
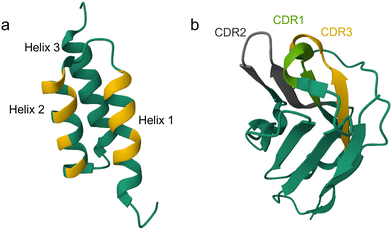 |
| Fig. 16 Structure of affibody and nanobody binding protein scaffolds. (a) 3-Helices structure of Affibody ZTaq (PDB ID: 2B88).427 Helices 1 and 2 contain amino acid positions (highlighted in yellow) that are randomized to generate affibody library. (b) Structure of nanobody (PDB ID: 5IVO)428 highlighting in green, grey and yellow complementarity-determining regions CDR1, CDR2 and CDR3, respectively. Highlighted areas in affibody and nanobody structures are primarily responsible for target recognition and binding to target. While the affibody binding region represents relatively planar surface and can interact with the epitopes on the surface of target molecules, CDR loops of nanobodies offer more flexibility and structural diversity for possible epitopes with convex type of binding.429 | |
Thus, successful engineering of affibodies and nanobodies as highly specific binding peptides for soluble targets has positioned them as promising candidates for applications that necessitate substrate recognition. Moreover, first attempts to generate nanobodies specifically binding solid surfaces (ZnO, gold, Al2O3 and CoO) by grafting ZnO and gold-binding peptides into CDR loops, are reported.430,431 The Belgian company Biotalys (formerly Agrosavfe) developed nanobodies that bind specifically to plant surfaces and were used in crop protection formulations for longer retention time that allowed reduction of chemicals sprayed on the plants.432 Similar systems employing insect-binding peptides were developed to target insect pests.433 In addition, nanobodies binding to polysaccharides were applied in seed protection formulations to improve the agricultural production yields.434 While the research on protein scaffolds for solid surface binding applications is relatively limited and soluble targets dominate the field, advancements in protein design and optimization may enable their wider applications in material-specific binding in the future. This will facilitate wider implementation of biobased sustainable solutions employing affibodies and nanobodies in agriculture and industry, particularly in applications, where material-specific binding is crucial.
Phage-display selected peptides (eMBPs, class I) and comparison to naturally occurring peptides
Phage display is a powerful biological selection method that has been widely used to identify new peptides that bind with high affinity and specificity to naturally occurring and synthetic polymers (see Section “Engineering of binding peptides and proteins”). Phage display methodologies have been awarded with the Noble Prize in chemistry in 2018 since they allowed to design antibodies. Phage display is limited in its selection to short peptides, ranging in general to up to 16 amino acids. In this section, applications of these man-made peptides (eMBP, class I) that bind to carbohydrate polymers, minerals, proteins, and synthetic polymers will be presented and their modes of binding will be discussed. Table 2 at the end of this section summarizes for carbohydrate polymers, minerals, proteins the cases, in which nMBPs and eMBPs (class I) found chemical comparable to different mode of interactions.
Table 2 Sequences and properties of eMBP (class I) obtained from phage display libraries. Described sequences bind to natural and man-made polymers. The short peptide sequences (usually <14 amino acids) form often not binding domains with cavities and can usually not distinguish between allomorphs
Material |
Sequence |
Charge |
Hydrophobicity |
Aromatic |
Ref. |
Silica |
MSPHPHPRHHHT |
6 |
−24.9 |
0 |
435
|
Silica |
RGRRRRLSCRLL |
6 |
−14.3 |
0 |
435
|
Silica |
APPGHHHWHIHH |
6 |
−17.4 |
1 |
435
|
Silica |
MSASSYASFSWS |
0 |
1.3 |
2 |
435
|
Silica |
MSPHHMHHSHGH |
6 |
−19.0 |
0 |
435
|
Silica |
KPSHHHHHTGAN |
6 |
−25.1 |
0 |
435
|
Silica |
LPHHHHLHTKLP |
6 |
−12.4 |
0 |
435
|
Silica |
APHHHHPHHLSR |
7 |
−22.1 |
0 |
435
|
Silica |
HPPMNASHPHMH |
4 |
−16.3 |
0 |
436
|
Silica |
HTKHSHTSPPPL |
4 |
−17.5 |
0 |
436
|
Silica |
HVSHFHASRHER |
5 |
−18.1 |
0 |
436
|
Silica |
HKKPSKS |
4 |
−18.1 |
0 |
437
|
Quartz |
RLNPPSQMDPPF |
0 |
−13.7 |
0 |
438
|
Quartz |
PPPWLPYMPPWS |
0 |
−7.8 |
3 |
439
|
Hydroxyapatite |
NPYHPTIPQSVH |
2 |
−12.3 |
1 |
440
|
Hydroxyapatite |
CMLPHHGAC |
2 |
4.1 |
0 |
441
|
Hydroxyapatite |
QHTNIVNTQSRV |
2 |
−11.0 |
0 |
442
|
Hydroxyapatite |
KLPPINLHPHRL |
4 |
−7.2 |
0 |
442
|
Hydroxyapatite |
TAPASMSDDRAS |
−1 |
−8.9 |
0 |
442
|
Hydroxyapatite |
SILSTMSDDRAS |
−1 |
−3.4 |
0 |
442
|
Hydroxyapatite |
SSPDRALAATPF |
0 |
−1.5 |
0 |
442
|
Hydroxyapatite |
LLADTTHHRPWT |
2 |
−9.6 |
1 |
442
|
Hydroxyapatite |
SVSVGMKPSPRP |
2 |
−5.7 |
0 |
442
|
Hydroxyapatite |
SVSVGMNPSPRP |
1 |
−5.3 |
0 |
442
|
Hydroxyapatite |
SAHGTSTGVPWP |
1 |
−5.1 |
1 |
442
|
Hydroxyapatite |
FPWLPRDNHTLN |
1 |
−12.6 |
1 |
442
|
Hydroxyapatite |
AVSSLSSTNYSI |
0 |
4.8 |
1 |
442
|
Hydroxyapatite |
TMGPTAPRFPHY |
2 |
−9.1 |
1 |
442
|
Hydroxyapatite |
QSHTRHISPAQV |
3 |
−11.3 |
0 |
442
|
Hydroxyapatite |
SAKTLSNSPLSN |
1 |
−7.0 |
0 |
442
|
Hydroxyapatite |
DAQQITLSHWRC |
1 |
−8.0 |
1 |
442
|
Hydroxyapatite |
STLPIPHEFSRE |
0 |
−9.1 |
0 |
443
|
Hydroxyapatite |
VTKHLNQISQSY |
2 |
−8.7 |
1 |
443
|
Hydroxyapatite |
QPYHPTIPQSVH |
2 |
−12.3 |
1 |
440
|
Aragonite |
HTQNMRMYEPWF |
1 |
−16.1 |
2 |
444
|
Vaterite |
ASTQPLR |
1 |
−5.5 |
0 |
445
|
Vaterite |
TTDRPKY |
1 |
−16.2 |
1 |
445
|
Vaterite |
SVPQRTP |
1 |
−8.5 |
0 |
445
|
Vaterite |
VQTPARM |
1 |
−2.4 |
0 |
445
|
Vaterite |
QPPRSTS |
1 |
−13.5 |
0 |
445
|
Vaterite |
VQTSSSY |
0 |
−3.7 |
1 |
445
|
Vaterite |
RCAPPCN |
1 |
−4.4 |
0 |
445
|
Vaterite |
HAPARVP |
2 |
−3.1 |
0 |
445
|
Cellulose |
HAIYPRH |
3 |
−7.5 |
1 |
446
|
Cellulose |
SHTLSAK |
2 |
−3.8 |
0 |
446
|
Cellulose |
TQMTSPR |
1 |
−9.9 |
0 |
446
|
Cellulose |
YAGPYQH |
1 |
−9.5 |
2 |
446
|
Cellulose |
LPSQTAP |
0 |
−2.6 |
0 |
446
|
Cellulose |
GQTRAPL |
1 |
−5.1 |
0 |
446
|
Cellulose |
QLKTGPA |
1 |
−4.5 |
0 |
446
|
Cellulose |
FQVPRSQ |
1 |
−6.9 |
0 |
446
|
Cellulose |
LRLPPAP |
1 |
0.1 |
0 |
446
|
Cellulose |
THKTSTQRLLAA |
3 |
−6.8 |
0 |
447
|
Cellulose |
WHWTYYW |
1 |
−9.2 |
5 |
448
|
Cellulose |
WHWRAWY |
2 |
−9.9 |
4 |
448
|
Chitin |
GEVGEQEKARVG |
−1 |
−13.4 |
0 |
449
|
Chitin |
EGKGVEAVGDGR |
−1 |
−10.3 |
0 |
449
|
Chitosan |
ADGVGDAESRTR |
−1 |
−14.0 |
0 |
450
|
Hyaluronan |
CKRDLSRRC |
3 |
−12.9 |
0 |
451
|
Hyaluronan |
STMMSRSHKTRSHHV |
6 |
−19.1 |
0 |
452
|
Hyaluronan |
GAHWQFNALTVR |
2 |
−2.3 |
1 |
453
|
Hyaluronan |
RYPISRPRKR |
5 |
−22.7 |
1 |
454
|
Polysialic acid |
TLPAILQSSGTRGGGS |
1 |
−1.1 |
0 |
455
|
Heparin |
CRGWRGEKIGNC |
2 |
−12.5 |
1 |
456
|
Heparin |
RTRGSTREFRTG |
3 |
−22.4 |
0 |
457
|
Collagen |
WYRGRL |
2 |
−7.8 |
2 |
458,459
|
Collagen |
KLWVLPK |
2 |
1.5 |
1 |
459,460
|
Collagen |
DAYWHPVWVHDP |
0 |
−9.5 |
3 |
461
|
Collagen |
DLQYWYPIWDTH |
−1 |
−12.1 |
4 |
461
|
Silk fibroin |
SYTFHWHQSWSS |
2 |
−14.1 |
3 |
462
|
Silk fibroin |
QSWSWHWTSHVT |
2 |
−12.2 |
3 |
462
|
Silk sericin |
HSIKMSVMQLRP |
3 |
−2.0 |
0 |
463
|
Silk sericin |
RLMYSELPQLPR |
1 |
−8.0 |
1 |
463
|
Silk sericin |
TLHMNWWQFTNW |
1 |
−9.3 |
3 |
463
|
PET |
DEYCCNN |
−2 |
−10.3 |
1 |
464
|
PET |
NALVQIS |
0 |
6.5 |
0 |
464
|
PET |
AIVGTPF |
0 |
10.6 |
0 |
464
|
Polylactic acid |
QLMHDYR |
1 |
−10.3 |
1 |
465
|
PMMA |
ELWRPTR |
1 |
−11.9 |
1 |
466
|
PUR |
VHWDFROWWOPS |
1 |
−16.3 |
3 |
467
|
Polystyrene |
ADYLSRWGSIRN |
1 |
−10.1 |
2 |
468
|
Polystyrene |
AGAGGGNVPVCS |
0 |
7.0 |
0 |
468
|
Polystyrene |
AGLRLKKAAIHR |
5 |
−2.9 |
0 |
468
|
Polystyrene |
RAFIASRRIKRP |
5 |
−8.9 |
0 |
468
|
Polystyrene |
VHWDFRQWWQPS |
1 |
−16.3 |
3 |
469
|
Polystyrene |
ETYVFDNHFHAP |
0 |
−8.9 |
1 |
468
|
Polystyrene |
EWDITTECTVTF |
−3 |
−0.2 |
1 |
468
|
Polystyrene |
EIHGNLYNWSPLLGYSYF |
0 |
−3.8 |
4 |
470
|
Polystyrene |
DSWPLRIYSGLSNYYHYF |
1 |
−10.3 |
5 |
470
|
Polystyrene |
FKFWLYEHVIRG |
2 |
0.4 |
2 |
471
|
Polypropylene |
LYARDVSRYWHV |
2 |
−6.0 |
3 |
472
|
Polypropylene |
HVSTTDLLGPRR |
2 |
−8.1 |
0 |
472
|
Polypropylene |
GNNPLHVHHDKR |
4 |
−22.5 |
0 |
472
|
Polypropylene |
NFLGAVAKGAIH |
2 |
9.3 |
0 |
472
|
eMBP (class I) for carbohydrates
Cellulose.
Phage displayed peptides for cellulose binding were first reported by Serizawa et al., where two groups of heptapeptides applied to microcrystalline cellulose particles were identified. Among them, the peptides HAIYPRH, SHTLSAK, TQMTSPR, and YAGPYQH, rich in hydroxyl and amino groups, interact with the ligand through hydrogen bonding. Subsequently, the peptides LPSQTAP, GQTRAPL, QLKTGPA, FQVPRSQ, and LRLPPAP, were reported and are rich in aliphatic amino acids, interact with the ligand through hydrophobic interactions. It has been speculated that the two groups of peptides with different binding interaction modes may have bound to the crystalline and amorphous parts of cellulose, respectively.446 Another heptapeptide, WHWTYYW, identified by Guo et al. efficiently binds to the cellulose nanowhiskers through an employed YYW motif that is present in several natural CBMs. In detail, Tyr5 is responsible for CH/π stacking interaction with the glucose ring of cellulose and the formation of hydrogen bonds.448 In addition, Qi et al. reported a 12-polymer peptide, THKTSTQRLLAA, which has a strong binding affinity for long fibrous cellulose. Alanine scanning revealed that Lys3, Gln7, Arg8, Leu9, and Leu10 have the dominating role in binding, with three polar amino acids involved in hydrogen bond formation.447
Chitin.
For chitin, Khoushab et al. reported a 12-mer chitin-binding peptide, EGKGVEAVGDGR (identified via phage ELISA), in which the middle part of the peptide mainly consists of hydrophobic residues, suggesting possible hydrophobic interactions between the chitin moiety and the peptide.449
Chitosan.
When it comes to positively charged chitosan, Tang et al. found a 12-mer peptide, ADGVGDAESRTR, that binds to chitosan-coated nanoparticles in which significant hydrogen bonding occurred between the amine group of the chitosan and the carboxyl group of the peptide, the amide group of the peptide backbone and the hydroxyl group of the chitosan, and the two hydroxyl groups on the peptide and the chitosan moiety. Besides, negatively charged amino acids (Asp2, Asp6, and Glu8) were also reported to contribute to the binding through electrostatic interactions with the positively charged chitosan building blocks.450
Hyaluronic acid.
Regarding negatively charged HA, the first eMBP for HA was reported by Mummert et al., GAHWQFNALTVR, which exhibited specific binding to HA. Alanine scattering implies a binding mode through hydrophobic–hydrophobic interactions, as hydrophobic residues Trp3, Gln4, Phe5, Leu9, Thr10 and Val11 are verified as key amino acids for binding.453 However, in most identified eMBPs, electrostatic interactions are the main driving forces for HA-binding. Examples comprise a 10-mer peptide, RYPISRPRKR, reported by Amemiya et al.454 and a 15-mer peptide, STMMSRSHKTRSHHV, reported by Tolg et al.452 adopted a B-X7-B (B: Lys or Arg; X: non-acidic amino acid) binding motif, as well as a 9-mer, CKRDLSRRC, reported by Ikemoto et al.473 harbouring the B-X5-B and dibasic R–R motif.
Heparin.
For negatively charged heparin, Yabe et al.456 identified a 12-mer peptide, RTRGSTREFRTG, containing four Arg residues. Gesteira et al.457 reported another 12-mer peptide, CRGWRGEKIGNC, presenting a chemokine-like repeat BXX motif, in which both binding modes are driven by electrostatic interactions.
Polysialic acid.
Positively charged amino acids also contribute to binding affinity and selectivity of the PSA-binding peptide. Shastry et al. reported a 16-mer peptide, TLPAILQSSGTRGGGS, in which the substitutions T1R, Q7R or Q7K resulted in strong binding affinity and selectivity in the presence of the competing glycan chondroitin sulphate A. However, increases in affinity through introduction of more basic residues resulted in decreased selectivity.455
Overall, phage display has been applied to discover eMBPs for cellulose, chitin, chitosan, HA, heparin and PSA, while eMBPs for xylan, starch, and alginate are yet to be exploited. The interactions between carbohydrate polymers and phage-displayed peptides are similar to those of the corresponding naturally occurring binding domains, relying mainly on the formation of hydrogen bonds (eMBPs for cellulose and chitosan), CH/π stacking (eMBPs for cellulose), electrostatic interactions (eMBPS for chitosan, HA, heparin and PSA) and hydrophobic interactions (eMBPs for cellulose, chitin, HA and PSA) between the protein and carbohydrate moiety.
eMBP (class I) for minerals
Hydroxyapatite.
Phage display has been used to identify HAP-binding peptides; however, no common motifs could be identified. As general trend, it could be observed that hydroxylated and basic amino acids as well as Pro occur preferentially in strong binding eMBPs.
The 12-mer peptide HA6-1 (SVSVGMKPSPRP) showed a specific binding towards HAP with alternating hydrophilic and hydrophobic residues as a common motif.474 Due to several hydroxylated residues and a net charge of +2, the primary interaction modes are hydrogen bonding and ionic interactions. Comparison of the sequence of HA6-1 with natural occurring sequences revealed similarities with domains associated with phosphate binding.474 The binding mechanism of the peptide was further examined by SPR, showing that the motif SVSV is directly binding onto HAP crystals.475 Additionally, an alanine scanning and analysis of the changes in binding HAP by QCM-D measurements, revealed that the Lys residue at position 7 has the strongest effect on HAP binding.476 Therefore, it was concluded that the electrostatic interactions governed by the cationic residue are the main driving forces in the adhesion of the peptide HA6-1 on HAP.
Chung et al. used a different approach with the goal of identifying eMBPs that promote the growth of individual HAP crystals. The best binding sequence, QPYHPTIPQSVH, displayed repeats of Pro and hydroxylated residues. Structural modeling of the peptide CLP12 using molecular mechanics calculations showed that the distance between hydroxylated residues resembles the size of single crystal HAP. Moreover, the identified consensus sequence showed remarkable similarities to the tripeptide repeat (G-P-Hyp) of the protein collagen.440 HABP1 (CMLPHHGAC) is another eMBP binding HAP and was obtained by screening a 7-polymer phage display library. Interactions with HAP are achieved through hydrogen bonds and ionic interactions of His residues with phosphate ions in the media.441 The eMBP VTKHLNQISQSY (VTK peptide) was identified as a good binding peptide on HAP. Interestingly, its composition is similar to certain regions found in the proteins fibromodulin, lumican, and decorin. The peptide is rich in polar residues, while harbouring no acidic amino acids.477 Addison et al. investigated the effect of phosphorylation on the VTK peptide, which changes the net charge of the peptide from +1 to −3.478 In natural binding peptides, phosphorylation plays an important role for many biomineralization processes. However, during phage display, it is generally not possible to introduce modifications.479 Phosphorylation of the VTK peptide did not improve the adsorption on HAP, but a 10-fold increase towards bone-like-mineral (BLM), a synthetic material mimicking the structure of natural bone, was observed.478 Here it was shown that through the introduction of phosphorylated residues, the specificity of the peptide can be tailored. With the aim of identifying HAP-binding domains on bone proteins, eMBPs displayed by phage display were selected by using HAP powder.480 In addition to confirming known HAP-binding domains from the nMBPs, statherin, DMP-1, OCN and others, new potential binding domains on these nMBPs were also identified. These sequences were, similar to the already known sequences, rich in acidic, as well as Ser and Thr residues as potential sites for phosphorylation. The authors note as main challenge, that mimics to nMBPs are difficult to obtain with phage display, since displayed peptides lack secondary structures, which was reported to be important for HAP-binding domains from DMP-1.304 Interestingly, a sequence matching the eight consecutive Glu residues in the HAP domain of BSP could not be identified, which Li et al. attributed to the fact that consecutive charged sequences are difficult to identify.480
Calcium carbonate.
Since calcium carbonate can occur in different polymorphs, phage display studies have focused on finding binding peptides that bind to each of these forms being calcite, aragonite, and vaterite. Vaterite, being the least stable form of calcium carbonate, is an especially interesting target, since it only rarely occurs in nature.481 The heptamer ASTQPLR was identified as eMBP and has a strong specificity to the polymorph vaterite. One general characteristic observed in vaterite eMBPs was that they possess positively charged amino acids, as well as Pro residues, indicating possible β-turn structures. Schüler et al.445 also showed that the peptide ASTQPLR was polymorph specific as it specifically binds to vaterite in the presence of aragonite. Interestingly, replacing the Pro residue with Gly resulted in a shift of the specificity towards the precipitation of the polymorph calcite.445 The authors hypothesized that the Pro residue plays a critical role in facilitating the polymorph specificity but gave no further conclusion on how the binding interactions to the mineral change in response to the introduction of the Gly residue.
Calcite-binding peptides found by phage display have been reported to be enriched in Asp, Glu, Ser and Thr. Commonly found motifs in the identified peptides are [DEST]–X–X–[DEST], S–[DEST] and S–X–X–S. The preferred enrichment of acidic and hydroxyl containing residues when binding calcite crystals, was unsurprisingly similar to the composition of nMBPs binding calcium containing minerals. Li et al.482 further stated the occurrence of hydroxyl containing amino acids supports the importance of phosphorylated residues in the interaction with the mineral.482 The eMBP AragBasic 10 (HTQNMRMYEPWF) was identified as a binding peptide to the polymorph aragonite and its binding behaviour was further evaluated to understand it on a molecular level.483 The amino acids His1, Arg6, Met7, and Trp11 were identified to have strong interfacial Ca–O bonding and form hydrogen bonds with the mineral. Despite the initial expectations from the authors acidic amino acids were not overly enriched in CC-binding peptides.483
Silica.
Silica has been used as a substrate to identify binding peptides in its crystalline438 and amorphous435 form, as well as thermally grown silicon dioxide.436 In nature only binding peptides towards amorphous silica can be found, which makes it interesting to compare binding modes of eMBPs to these three silica forms. Binding of phage displayed peptides to a quartz substrate (crystalline silica) led to the identification of the peptide DS202 (RLNPPSQMDPPF).438 Based on these experiments, the eMBP S1 (PPPWLPYMPPWS) was designed by a knowledge-based approach. Oren et al. employed bioinformatic tools to compare and classify eMBPs from phage display selection to discover the key characteristics of quartz peptide interactions. Strong quartz binding peptides harbour as a general trend many aromatic and hydrophobic residues.439 Naik et al. identified several eMBPs from phage display against amorphous silica.435 A striking similarity between all identified peptides was their high content of positively charged amino acids as well as a high number of His, often ranging between 5 and 6 residues within a 12-mer sequence. When evaluating the ability of these peptides to precipitate silica in vitro, the peptide Si4-1 (MSPHHMHHSHGH) showed the highest precipitation effect. Another strong binding eMBP Si4-10 (RGRRRRLSCRLL) was only able to precipitate small amounts of silica. As lessons learned the authors concluded that three characteristics are required for efficient silica precipitation. These are the presence of hydroxy- and imidazole-containing amino acids, as well as a high cationic charge. Thermally grown silicon dioxide is a typical surface in semiconductor fabrication, which makes eMBPs highly interesting for manufacturing processes. Thermally grown silicon dioxide is in contrast to amorphous silica not porous and less hydroxylated. eMBP for this material show high number of His residues, which is topped by hydrophobic and aromatic residues (examples of common binding sequences are HPPMNASHPHMH, HTKHSHTSPPPL and HVSHFHASRHER).436
eMBP (class I) for protein-based materials
Collagen.
Rothenfluh et al. identified by phage display a 5-mer eMBP, WYRGRL that binds to type II collagen α1 chain. The 5-mer eMBP sequence is similar in its composition to the naturally occurring peptides, and consists of the aromatic amino acids Trp and Tyr, and the positively charged amino acid Arg.458,459 Juliana et al. reported a 6-mer eMBP, KLWVLPK, which shows specificity to bind the human type IV collagen.459,460 Similarly, it takes the aromatic residue Trp and the positively charged residue Lys for binding. In addition, Lin et al. identified two eMBPs, DAYWHPVWVHDP and DLQYWYPIWDTH, which bind specifically to the α1 chain of type XII collagen (human cartilage lysate and cartilage pieces used for screening of the phage display library). Computational molecular docking studies identified WXPXW as the binding motif,461 which it is not the same with commonly reported binding motif G–G–X and G–X–HyP in nMBPs.
Silk.
eMBPs that bind to fibroin and sericin silk proteins have also been identified in phage display experiments. Nomura et al. used degummed silk fibroin fibres to identify silk-binding peptides. The identified sequences had a high frequency of Trp and the motif QSWS was found repeatedly. To elucidate the mode of binding, the QSWS motif was modified by replacing the Trp with an Ala, obtaining the QSAS motif. As a result, the modified motif showed a decrease in binding competence of more than 90%, highlighting the influence of hydrophobic interactions. Although the motifs found are not similar to those reported in nature, which are rich in Gly and Ala (GAGAGX), phage display also shows that similarly to the natural self-assembly process, hydrophobic interactions guide the binding to fibroin fibres.462 In another study, a phage display library was used to identify silk sericin binding peptides. The sequences identified were mostly positively charged under the conditions screened, and proposed a binding mechanism via hydrogen bonds between the polar residues of sericin and the charged peptide.463 These studies demonstrated that the interaction of binding peptides with silk depends on the type of silk protein targeted. Hydrophobic interactions drive the interaction with fibroin, whereas polar interactions drive the interaction with sericin. It suggests that the identified binding forces are similar between nMBPs and eMBPs, even though binding motifs were different.
eMBP (class I) for synthetic polymers
A huge challenge in medicine and material/polymer science is a target- or material-specific binding. The latter is especially challenging for polymers, since a large variety of man-made polymers with subtle differences in chemical properties is available.435,484,485 This section focuses on binding peptides for the most common polymers previously described in the Section “Naturally occurring binding peptides (nMBPs) that bind to natural polymers and naturally occurring inorganic materials”.
Plastic-binding sequences derived from phage display experiments can sometimes be identified unintentionally, as target molecules are often presented on polymer beads. Adey et al. identified 17 plastic-binding phage sequences (P-bΦ), which bind the synthetic polymers PS and PVC. The peptides showed an enrichment of the aromatic amino acids Tyr and Trp, predominantly in the N-terminus of the peptides. The authors have attributed the interaction of PS with the peptides to π–π stacking of aromatic residues of the polymer with Trp and Tyr. Common binding motifs identified for peptides binding to PS include WXXW,470 FHXXW486 and WXXWXXXW.487 For the interaction of peptides with the polymer PVC hydrophobic interactions are expected to be the main driving force, but the binding mechanism was not further evaluated.470
Polyurethane binding peptides have been identified with the goal of achieving bioadhesive-related fouling resistance. Four peptides (P1–4) showed strong binding towards the polymer. When evaluating the frequency of amino acids present in the isolated peptides, it became evident that over 50% of all residues could be accounted to the amino acids Ser, Arg and Thr, while Ser had the highest occurrence. Based on these results, the authors suggested that the interaction between the polymer and the peptides is achieved through hydrogen bond interactions with the carbonyl groups of the polymer.467
Juds et al. combined phage display technology and next-generation sequencing to report a polypeptide (LYARDVSRYWHV) that binds to polypropylene (PP) foils. Within the peptide sequences, which were the most prevalent, a trend towards positive net charges and a low hydrophobicity was observed. Ser scanning showed the importance of Arg4, Arg8 and His11 residues, due to the reduced binding affinity of the variant to the PP surface. Herein, the guanidino moiety of Arg plays a crucial role in ligand recognition, as the exchange of Arg to Lys inhibits the binding affinity, which might be related to the formation of hydrogen bonds with C–O groups on the PP surface. In contrast, the binding affinity of the variant D5S was enhanced, suggesting that the acidic amino acids Asp/Glu negatively affects the binding affinity. Additionally, variants with three positions mutated to Ala (L1A, V6A and V12A) did not bind to PP, indicating that hydrophobic interaction was part of the driving force. The binding affinity decreased by more than 50%, when the amino acids Arg8-Tyr9 were split by a Ser, or when the Arg–Tyr position was shifted. The authors concluded that hydrophobic interactions do not dominate the PP binding, as the presence of cationic residues, especially Arg, was relevant.472
To functionalize PET surfaces, Swaminathan et al. identified several binding peptides. The sequences were characterized by a low net charge ranging from +2 to −2 at neutral pH, and in general high values of pI. DEYCCNN showed the best result in binding PET surfaces, and it was further combined with a silver synthesis motif in a bifunctional peptide to assemble metallic materials on PET. The mechanism of how the peptide interacts with the polymer surface was not further elucidated.464
Studies from Serizawa et al.488 led to the discovery of peptides specific to syndiotactic-PS (st-PS) with enrichment of hydrophobic amino acids as Gly, Ala and Phe. Material-binding specificity towards isotactic- (it-PMMA) and syndiotactic-PMMA (st-PMMA) could also be observed. It-PMMA binding peptides are generally more enriched in Ser, Thr, Tyr and Arg residues, suggesting that hydrogen bonding between the hydroxy groups of the amino acids with the ester groups of the polymer explains the interactions. In contrast, peptides binding specific to st-PMMA showed an enrichment in amine-containing residues (His, Lys, Arg and Trp). Interestingly, strong binding peptide sequences included motifs with amine-containing residues adjacent to another study of Serizawa et al.489 It suggested that the amine-containing residues act as proton donors to the ester groups of st-PMMA, which in turn form hydrogen bonds. Furthermore, the peptide c22 (QLMHDYR) showed specificity towards crystalline PLLA in comparison to amorphous and atactic PLLA reference surfaces. Here, an enrichment of hydrogen bond promoting amino acids (His, Lys, Arg, Asp), as well as non-polar Leu and Ala residues was demonstrated, which suggests that hydrophobic interactions also improve the binding ability of the peptides.465
Computationally assisted design of polymer-binding peptides
As outlined in Section “Naturally occurring binding peptides (nMBPs) that bind to natural polymers and naturally occurring inorganic materials,” peptides have undergone evolutionary processes to material-specifically recognize carbohydrates, minerals and proteins. In addition, nature managed with a relatively small subset of materials to generate a huge functional and structural diversity, whereas mankind developed a huge number of polymers that are often used in complex blends. Material-specific binding domains are missing for man-made materials including the six abundant man-made polymers (PE, PP, PS, PVC, PET, PUR). Targeting these polymers specifically is promised to impact the circular economy of plastics and the management of mixed plastic waste. This section will focus on the ways in which computational design of eMBPs could promote specific polymer binding. Molecular understanding of general binding interactions is usually generated through molecular dynamic (MD) simulations. In addition, emerging machine learning (ML) and AI-approaches are being used to cluster specific binding patterns and to identify key amino acids for each material, as explained in detail in this section.
A main challenge and prerequisite for computational studies are parametrized polymer surfaces, which mimic its structural diversity (amorphous and crystallin region). Another challenge is the correlation between computational results and experimental findings, as well as data volumes required for ML-/AI-approaches. There are several parameters that can be varied, as force fields or the source of starting structures and their parametrization. Until today, several surfaces were used to investigate peptide interactions in MD simulations. The surfaces include models of minerals like gold, silver or tin oxide and silica, but also some synthetic polymers like poly(methyl methacrylate; PMMA) and more complex substrates as apple leaf wax, which are described and discussed. Besides, there are models of polymer surfaces that were not investigated in the context of peptide binding. These include crystalline and amorphous representations of polyethylene (PE) and poly(vinyl chloride) (PVC),490 as well as, crystalline models of poly(tetrafluoroethylene) (PTFE) and poly(ethylene terephthalate) (PET).491
Molecular simulations
The interaction of binding peptides to different surfaces is studied on a molecular level through molecular dynamic simulations. The first prerequisite of MD simulations is highly resolved structures or cured homology models. To tackle the challenge of the lack of crystal structures, AlphaFold2 has made significant advancements in the generation of high-quality three-dimensional (3D) structures that closely resemble solved crystal structures. Prior to the emergence of AlphaFold2, another method called PEP-FOLD 3 was used for the generation of unknown peptide structures.492,493 When investigating material-binding peptides, the second requirement is an accurate model of the studied material. To achieve an accurate model of a material, the right force field must be chosen, and the parameterization has to be approximated in iterative cycle.494 To name some specifically designed force fields for materials, there are specific force fields for crystalline polyethylene,495 titanium oxide (TiO2)496 or glycolipids.497 To study the binding process of the peptide, the forcefield should be optimized to the peptide simulation. An advanced forcefields for peptide simulations is AMBER ff14SB,498 which is based on AMBER ff99SB.499 Major changes for the forcefield included new parameters for side chain dihedrals and adjustments to the backbone energy profile. Further changes were summarized by Huang and MacKerell in 2018.500 Similarly important for reliable MD simulations is the sampling of all conformation states of peptides/proteins, which requires a sufficient amount of computing power and that will be discussed together with the limitations for simulations further below.
Machine learning
Machine learning, and in particular deep learning in the biotechnology field, became widely popular between 2010 and 2020 with published articles about computational protein function prediction,501 and protein structure prediction,502 resulting finally in AlphaFold 1,503 AlphaFold 258 and AlphaFill.504 AlphaFold is based on deep learning, one field of machine learning. Deep learning uses deep neural networks to learn features directly from raw data. This allows the discovery of complex patterns and relationships in the data.58,505 The emergence of AlphaFold2 (AF2)58 as a remarkably precise tool for protein structure prediction has spurred the development of novel applications, including a network specifically designed for predicting peptide binding.506 In their study, the structure prediction capabilities of AF2 were combined with logistic regression to build the mentioned predicting network. Using AF2, models were generated for the major histone complex (MHC) in both peptide-bound and peptide-unbound states. These models were then translated into a scoring system that discriminated between binders and non-binders. By leveraging three-dimensional structures rather than relying solely on sequences, this technique enabled a classification approach for predicting binding peptides towards MHC as case study.506
In recent years, the use of machine learning techniques to identify key characteristics in peptide sequences has been on the rise. It was well summarized by F. Arnold in 2019,358 who gave an overview about ML techniques used for protein engineering. To name them, there are linear regression models, random forest tree approaches or the support vector machines next to the more complex neural networks.
Notably, in the realm of classifying and predicting material-binding peptides, support vector machines (SVMs) have played a crucial role as two recent reports on peptides that bind to polystyrene (PS) indicate.507,508 SVM is a supervised machine learning concept employed for tasks, such as classification and regression. In the context of material-binding peptides, particularly with regards to PS binding, a prediction webserver based on an SVM framework was introduced in 2017. This web server was trained using a dataset consisting of positive and negative experimental results obtained from phage display, encompassing 104 sequences.507 In this study, a trend to the aromatic amino acids Trp, Tyr and Phe could be observed to positively affect the PS binding affinity. Later in 2020, a similar approach to identify PSBPs was published by Meng et al.508
Prerequisite for MD and ML.
It is difficult to compare the two method areas, as both have gained their own importance in biotechnology. MD simulation is used to understand the molecular processes of enzymatic catalysis and peptide binding. While molecular dynamic simulation requires high-resolution crystal structures or very good homology models, in machine learning processes, the amount of data plays a crucial role. In summary, MD simulations require little and very high-quality data, while machine learning requires high data volumes. In the latest years, these distinctions have become somewhat blurred, as scientists try to minimize the amount of data required in machine learning models, being AlphaFold an example for generating protein structures that approximate a crystal structure in their overall resolution and quality.58
The application of machine learning relies heavily on large datasets and, therefore, the generation of binding data for material-binding peptides often involves high throughput screening methods like phage display. Although phage display has a limitation in sequence size, typically smaller than 12 amino acids, it remains a versatile approach capable of producing sample data for machine learning purposes.507,509
When delving into the details of machine learning, it is also noticeable that the machine learning field can use both structural and sequence data for training. Therefore, a new field has risen, which tries to combine machine learning with MD simulation.510,511 This approach promises profound prediction of peptides through machine learning, which is not dependent on large amounts of experimental data.
Despite the power of machine learning, most of the already investigated materials and their corresponding material-binding peptides have been studied by MD simulation. Among the studied material, it can be found metals, such as gold (Au), silver (Ag), aluminium (Al), titanium oxide (TiO2) or platinum (Pt). When it comes to synthetic polymers as PP, PET, PS, or even more complex materials as apple leaf wax, only a few studies have been conducted.
MD and ML strategies to understand peptide binding to different materials
Polymers.
The study of polymers through MD simulations and machine learning is a complex endeavour. Due to this complexity, studies that have focus on the design if peptides binding to synthetic polymers are scarce. In 2005, Raut et al. studied the correlation between MD simulations of a functionalized alkanethiol self-assembled monolayer and their experimental results based on surface plasmon resonance (SPR). Their model did not directly correlate with their experimental results and demonstrated the complexity of such materials.512 In the study of synthetic polymers, rational design of MBPs based on MD lead to the design of three eMBPs with a higher affinity to poly(methyl methacrylate) (PMMA).513 This was the only found study about peptide engineering towards a synthetic polymer. Even more complex in its composition is natural apple wax. To the best of our knowledge, there is only one study investigating material-binding peptides that interact with heterogenous materials, as the apple leaf wax.514 This study included molecular dynamic simulations, alanine scanning and peptide engineering. It was already described in Section “Engineering of binding peptides and proteins” as an example of protein engineering of peptides towards a specific material, and it will also be discussed in the Section “Emerging applications of material-binding peptides.”
When generating polymer models for MD simulations, the issue of crystallinity arises. Parts or even the entire polymer can be amorphous, making it even more challenging to compute. Although there are different articles about simulation of liquid or amorphous metals like germanium,515 aluminium oxide516 or polymers like silicon,517 and polyethylene,518 there is no simulation of a material-binding peptide binding to an amorphous surface. Thus, in most cases, stiff surfaces are assumed for simulation of peptide binding.
Graphene.
In the realm of biomedicine and biosensor technology, graphene has emerged as a promising surface with significant potential. In recent years, there has been growing interest in investigating the binding of myelin basic protein (MBP) to graphene. Simulations were conducted to study the binding of all amino acids to graphene using a newly developed force field called GRAPPA,519 and findings revealed different binding preferences of each amino acid. The simulations demonstrated that Arg, Tyr, Trp and Gln exhibited strong binding affinity to graphene, as indicated by their calculated free energies. In contrast, Ile displayed a weak binding to graphene. The researchers further elucidated that compact amino acids displayed stronger binding due to their reduced interaction with the surrounding water layer. Overall, this investigation sheds light on the binding preferences of different amino acids to graphene, providing valuable insights into the interactions between biomolecules and graphene. Simulating the graphene-binding peptide P1 (HSSYWYAFNNKT) and its variant P1A3 (HSSAAAAFNNKT) in both adsorbed state and in solution revealed a helical conformation of P1. This structure affected the binding of residues Tyr4, Trp5, Phe8, Asn9 (P1) by positioning them in a defined manner. In contrast to that, the helical structure could not be formed in P1A3 trough their substitution Y4A and W5A, leading to a diminished binding to graphene. This highlights the importance of a three-dimensional structure for binding.519
Gold and silver.
Most of the studies presented in this review focused on binding of peptides to metals by engineering binding peptides, with material-specific binding towards gold and silver surfaces.520 Reports focus on three eMBPs, namely PD4,521 A3,522 and AUBP3,523 as well as four newly predicted peptides designed to bind specifically to either silver or gold. To predict new binding peptides, a combination of Bayesian optimization, MD simulations and experimental amino acid substitutions was employed. The study compared the binding affinity and specificity of the original wild-type (WT) peptides, their five corresponding variants, and four predicted peptides obtained through Bayesian optimization. The application of Bayesian optimization allows efficient maximization of an objective function since the next evaluation points are intelligently chosen. This decreases computational requirements, while low data situations can be handled. Biophysical interactions towards gold and silver were analysed using both quartz crystal microbalance (QCM) and MD simulations. The results revealed that certain amino acids, such as Tyr1, Met9 and Phe12 exhibited high preference towards silver binding. Furthermore, an eMBP variant containing a triad of Tyr, Met and Phe displayed reduced binding affinity, indicating that the type of residues present is not solely responsible for material interactions. The study concluded that not only the specific amino acid residues, but also the spatial arrangement and distance between these residues within the three-dimensional structure played a crucial role in the binding process.
This research provides valuable insights into the key amino acids and their spatial relationships that govern the binding affinity and selectivity of the peptides towards gold and silver surfaces. The combination of experimental and computational approaches contributes to the understanding of peptide–surface interactions and aids in the engineering of peptides with desired binding properties. Through this method of operation, computational models are approved in their ability to describe experimental behaviour and necessary changes can be introduced accordingly.
In another approach, Janairo J. I. B. used the experimental binding data of 1720 peptides524 towards gold for training an SVM to predict the gold binding of new peptides. In addition of an accuracy of 80.2% of the testing set, he also could find key interactions and their importance for gold binding, as hydrophobicity. Additionally, the side chain size and the extended structure preferences proved to be important. Less important seems to be the presence of helical structures, or the preference between helices or bends.525
In 2014, Palafox-Hernandez et al. compared four gold and silver-binding eMBPs, namely AgBP1 (WAGAKRLVLRRE), AgBP2 (WALRRSIRRQSY), AuBP1 (TGIFKSARAMRN) and AuBP2 (EQLGVRKELRGV), on an experimental level and in silico to elucidate the molecular differences in binding. The preferred mode of gold binding is the direct interaction with the surface, while for binding silver the interaction was based on solvent-mediated interactions.526 Here, the side-chain residues interact with local variations in solvent density at the material interface, rather than with the material itself. Furthermore, they could prove the material-specificity for AgBP1 for silver over gold. The peptides AgPB2, AuBP1 and AuBP2 showed binding to both surfaces, for both materials. Here, QCM served as experimental confirmation as well.
In addition, all amino acids and their binding to gold and silver were simulated in silico to calculate the free energy. This simulation pointed out the amino acids Arg, Trp, Cys and Tyr as good binders, and Met, Arg, Cys, Asp and Ser as not influential.527
In a separate study, researchers conducted simulations to investigate the binding process of two gold-binding eMBPs, namely 6GBP (MHGKTQATSGTIQS) and 3GBP (MHGKTQATSGTIQS), in both a vacuum environment and TIP3P water.528 Interestingly, the authors reported that the flexibility of the eMBPs only influenced their binding behaviour in the presence of water molecules. Surprisingly, even in a vacuum environment, the selected negative control peptide, 6NGBP (AIRRDVNCIGASMH), exhibited an affinity for gold binding despite its structural stability. As a result, the researchers concluded that not only specific amino acids such as Ser, Thr, Arg and Asp are important for gold binding, but also the overall flexibility of the peptide itself.
Aluminum.
One study, investigating the binding of the peptide “LWFYTMWH” to aluminum, led to the conclusion of a negative effect of the water layer on the binding. The modification (NO2, OH, COOH) of the aluminum surface could improve the binding in silico.529 This trend could also be observed for other metals, as it is explained in the following.
Titanium oxide.
Simulations of side chain analogues (SCAs) of all amino acids binding to titanium dioxide (TiO2) revealed that the bound surface solvation layer (water) could only be penetrated by the Ser and Thr side chain analogue.530 All the other side chain analogues bound to the water surface, on top of the TiO2. Besides the SCAs, the binding of a six amino acid long titanium binding peptide (RKLPDA) found by phage display, was simulated and showed two distinct binding states. The first binding state was an elongated structure, while the second state was a C-formed, compact structure, supported by a salt bridge between the Arg1 and Asp5. It is also important to say that the calculated free energy of the titanium binding peptide was lower than the sum of the individual SCAs. Similar results for titanium binding peptides were found by Sampath et al. They analyzed the binding of the three amino acids Arg, Lys and Asp towards four titanium variations with different hydroxylation states. Hereby, Arg was found as a universal titanium binder, while for Lys and Asp the hydroxylation state of titanium is of high importance.531 Another study was about the binding of the peptide RGD to two forms of TiO2 (rutile (110) and anatase (101). They could also show a negative influence of the aqueous environment on the peptide binding.531 The introduction of step edges as a binding surface further decreased the binding, due to less interacting atoms. The differences between the studies about TiO2 were reasoned by the distribution of surface hydroxyl groups in another study. Hereby, the known titanium binding peptide RKLPDA and RKLPDAPGMHTW were used on four titanium (Ti) surfaces (negative/non-hydroxylated, neutral/non-hydroxylated, negative/hydroxylated, and neutral/hydroxylated).
Platinum.
The noble metal platinum (Pt) and binding of peptides to it was less researched than other described metals. N. Katarci et al. constructed four cyclic peptides binding to platinum (1: CPTSTGQAC; 2: CQSVTSTKC; 3: CVRTSTWRCC; 4: CIMRDGPMC).532 Their binding strength was analysed by immunolabelling fluorescence microscopy, which concluded that two of them were designated as weak binders, while the residual two were designated as strong binders. All peptides had an N- and C-terminal Cys, which were used for cyclization via disulphide bonds. They focused in particular on the sequence T–S–T, which was also reported before as a good metal binding sequence.533,534 It was shown that not only the T–S–T motif was responsible for the binding, but also residues as Arg. Interestingly, they could find some hints for the reported importance of the T–S–T motif, which positively affects the flexibility of the peptide during surface binding. In addition, it was seen that the flexibility was reduced after binding, which was hypothesized to be important for the binding stability.
Silica (Quartz).
In addition to metals, silica and hydroxyapatite were also employed as materials in various simulations for different purposes. As an illustrative example, the simulation of a silica-binding peptide (S1, PPPWLPYMPPWS), binding to quartz (SiO2) was utilized as a benchmark to compare two molecular dynamics (MD) techniques. Specifically, these techniques were based on either the temperature replica-exchange molecular dynamics (T-REMD) approach or the replica exchange with solute tempering (REST) approach.535 Besides the economic advantages of REST-based approach with less needed computing power (see limitations), Wright et al. could also identify the contact motifs for peptide S1, which were mainly composed of Pro, Trp, and Leu. Besides the contact amino acids, the focus of the described study was more on the comparison of the replica-exchange techniques.
Hydroxyapatite.
Hydroxyapatite is one key component of bones and, therefore, of high interest to understand the process of biomineralization, which also included the interaction with collagen. In a study from Duanis-Assaf, T. et al., they investigated the binding process of a collagen peptide (SVSVGMKPSPRP) to hydroxyapatite systemically.536 They combined MD simulation alanine scanning and QCM-D analysis to study the contribution of every amino acid to its binding to hydroxyapatite. The alanine scanning pointed out that the variants G6A, K7A, S9A, P10A decreased the binding affinity measured by QCM-D. In particular the K7A showed the highest reduction in binding.
Limitations of computational design.
The biggest limitation of MD simulation arises from the sheer computational power, which is required by more complex simulations. This leads to a reduction in sampling, limiting the coverage of all possible conformations. Particularly complex structures such as biomolecules suffer from this limitation due to their rough energy landscape. In the past, there have been several solutions to either lower the complexity of the system by coarse graining (see below) or to achieve a higher sampling by new sampling methods with the same complexity of the system. Concepts like the metadynamics or replica-exchange molecular dynamics (REMD) to overcome the described limitations are well summarized.537
The reduction of the complexity includes, for example, grouping atoms into smaller packages to limit the degree of freedom (coarse grain), in contrast to the complex all-atom model. Furthermore, further degrees of freedom are minimized in most simulations from the outset, for example by constraining the center of mass of the molecule in the simulation cell or by periodic boundary conditions.538
In order for the simulation to mimic reality as closely as possible, force fields are used, often based on empirical data. In these force fields every unbound interaction is stored as a function. For applications such as the simulation of peptides in aqueous solution, the AMBER force fields FF99SB and FF14SB498,499 are considered as most suitable. Despite their tailored nature, these force fields remain models that do not simulate all aspects with the same quality.515–518,539
ML faces additional limitations that extend beyond peptide binding applications. ML for protein engineering is very dependent on big databases with a high data quality. This dependency introduces two limitations for machine learning. Firstly, most of the experimental results are small datasets of several variants to a few hundred, because of the laborious work required to create and screen variants in the lab.358,540 Secondly, there is no standard operating protocol for performing assays and saving the data consistently. This disparity between wet lab practices and machine learning is one of the significant gaps in the field of protein engineering.
One limitation for most of the SVM based approaches in regard to material-binding peptides is the classification into binders and non-binders. Therefore, these methods do not provide any information about the binding affinity, making it challenging to draw conclusions about the strength of the binding interactions.506
Machine learning models based on supervised learning, which for example rely on regression models, tend to overfitting. This was already discussed by Diettrich et al. in 1995541 and mentioned by Davari et al. in 2020 in the context of protein engineering.540 The general challenge of overfitting in the field of machine learning was summarized together with its solutions and alternative methods to avoid overfitting.542,543
Conclusion and lessons learned from computational analyses
As a general trend in reports, more and more deep learning approaches are used to predict peptide structures and slowly replace crystallization studies. Predicted structures can be used in MD simulations to get molecular understanding of the binding process. Either the simulation results, or the peptide sequence, or its structure, can also be used by ML again to identify binding patterns for initial binding and/or binding strength. In particular, experimental data on mMBPs can assist in amino acid pattern recognition, and three key observations can be drawn. First, the binding is not only dependent on the peptide amino acid sequence alone, but also on its structure, flexibility and the targeted surface chemistry (e.g. hydroxyl groups). Flexibility, for example, was enhanced by the motif T–S–T.532 Second, it could be observed in several studies that the hydration shell or the water solvation layer plays a key role in the binding process, and it is indispensable for any binding simulation. Amino acid analogues as Ser and Thr were found to penetrate the solvation layer, while all other amino acids bound to it.530 In one report, the solvation layer negatively influenced the binding during the simulation, in contrast to the vacuum.528 Various and different binding motifs with prominent amino acid selections have been reported, for instance in the case of Au (Arg, Met, Trp, His, (T–S–T)), TiO2 (Thr, Ser), Pt (T–S–T, Arg), graphene (Arg, Tyr, Trp, Gln) or PS (Trp, Tyr and Phe). Based on the presence of Trp, Thr and Arg in the mentioned motifs, it can be deduced that those amino acids play an important role in several binding processes.544,545 Even though important residues and motifs were highlighted, an underlying mechanism for material specificity could not be identified so far. It is barely understood how the found interactions can be transferred into specific peptide variants using protein engineering. Additional work in this field is promised to result in such variants, with a high impact on the circular economy of materials and polymers.
Discussion and general lessons learned from nMBPs and eMBPs
Nature uses two complementary binding principles to design material-specific binding domains/modules: chemical interactions and spatial-recognition of materials/polymers. Chemical interactions include usually a combination of several forces such as electrostatic, hydrophobic, and/or CH/π interactions, hydrogen bonding and/or disulphide bond formation. The second principle, using a defined spatial-distribution of chemical interactions, enables to even distinguish allomorphs, for example in carbohydrates. In the following paragraphs, we summarize the lessons learned and highlight the general binding trends of MBPs required to understand the main driving forces for material-specific binding (Table 3). Different binding modes between nMBPs and eMBPs will be analysed, and an overview of selected emerging applications will be given.
Table 3 Natural and man-made binding motifs/peptides for natural and man-made polymer materials including main chemical driving forces in material binding. N/A: not available
Material |
Main driving force of interaction nMBPs |
Main driving force of interaction eMBPs |
Functional properties/type that enables binding |
Carbohydrates |
Cellulose |
CH/π interactions |
CH/π interactions |
Type A, B and C |
Hydrogen bonds |
CH/π interactions |
Hydrophobic interactions |
Chitin |
CH/π interactions |
Hydrophobic interactions |
Type A, B and C |
Hydrogen bonds |
Starch |
CH/π interactions |
N/A |
Type B |
Hydrogen bonds |
Xylan |
CH/π interactions |
N/A |
Type A, B and C |
Hydrogen bonds |
Chitosan |
CH/π interactions |
Hydrogen bonds |
Type C |
Hydrogen bonds |
Electrostatic interactions |
Hydrogen bonds |
Alginate |
CH/π interactions |
N/A |
Type B and C |
Hydrogen bonds |
Electrostatic interactions |
HA |
CH/π interactions |
Hydrophobic interactions |
Type B |
Hydrogen bonds |
Electrostatic interactions |
Hydrophobic interactions |
Heparin |
Hydrogen bonds |
Electrostatic interactions |
N/A |
Electrostatic interactions |
PSA |
Hydrogen bonds |
Hydrophobic interactions |
N/A |
Electrostatic interactions |
Electrostatic interactions |
Minerals |
Calcium carbonate |
Electrostatic interactions |
Electrostatic interactions |
Structure changes through binding |
Hydrogen bonds |
Hydrogen bonds |
Hydroxyapatite |
Electrostatic interactions |
Electrostatic interactions |
Structure changes through binding |
Hydrogen bonds |
Hydrogen bonds |
Silica |
Electrostatic interactions |
Electrostatic interactions |
Structure changes through binding |
Hydrogen bonds |
Electrostatic interactions |
Hydrophobic interactions |
Protein-based materials |
Collagen |
Hydrophobic interaction |
Hydrophobic interaction |
Self-assembly |
Hydrogen bonds |
Hydrogen bonds |
Keratin |
Disulfide bonds |
N/A |
Self-assembly |
Silk |
Hydrophobic interaction |
Hydrophobic interaction |
Self-assembly |
Synthetic polymers |
PS |
Hydrophobic interactions |
π–π interactions |
N/A |
π–π interactions |
PE |
N/A |
N/A |
N/A |
PP |
Hydrophobic interactions |
Hydrophobic interactions |
N/A |
PU |
N/A |
Hydrogen bonds |
N/A |
PET |
π–π interactions |
N/A |
N/A |
Hydrogen bonds |
PVC |
N/A |
Hydrophobic interactions |
N/A |
PLA |
CH interactions, Electrostatic interactions |
CH interactions |
N/A |
Electrostatic interactions |
PMMA |
N/A |
Hydrogen bonds |
N/A |
Electrostatic interactions are the main driving forces in HAP and CC, but also to a lesser extent, in charged carbohydrate polymers such as chitosan, alginate, HA, heparin and PSA. Positively charged chitosan and negatively charged natural polymers (alginate, HA, heparin and PSA) interact with oppositely charged residues in proteins. Translational modifications, such as phosphorylation on Ser and Thr residues increase negative charges in the protein, which strengthen their ability to interact with positively charged materials. For carbohydrates, positively charged residues in proteins, such as Arg and Lys, interact with negatively charged carboxyl or sulphate groups of carbohydrates, which are similarly observed in amorphous silica and collagen.
CH/π interactions are relevant interactions limited to carbohydrate-binding peptides, in which CH/π stacking occurs between carbohydrate polymers and the pi system of Tyr, Trp or Phe. CH/π interactions were not yet reported for peptide binding to the carbohydrates heparin and PSA, and mineral or protein-based materials. Heparin and PSA-binding peptides were shown to predominantly bind by electrostatic interactions. HA-binding peptides were also shown to interact via CH/π, despite the high similarity of HA to heparin and PSA. One specific feature of heparin differentiating it from other described carbohydrate polymers are the sulphate groups, which increase its overall charge, and thereby increase its propensity to bind by electrostatic interactions.
Hydrogen bonding between polar amino acids (Gln, Glu and Asp, Arg, and Asn) and ligand functional groups such as –OH and –NH2 are observed in all the three classes of nMBPs, binding to carbohydrates, mineral- and protein-based materials. Hydrogen bonds can therefore be classified as a universal binding force among material-binding peptides.
Hydrophobic interactions are the predominant driving force for binding within protein-based materials and are involved in self-assembly processes of collagen, keratin and silk fibres. Main differences of the protein-based materials to minerals and carbohydrates are the highly polar characteristics of the mineral- and carbohydrate-based materials, which often do not occur in protein-based materials. In protein-based materials, usually repeating motifs (e.g. in collagen, keratin and silk) occur. They ensure that the hydrophobic interactions induce a self-assembly process for instance in form of fibres. Protein-binding MBPs do not have a separate/specific binding domain, but fully rely instead on their peptide sequence.
Disulphide bonds play a predominant role only in protein-based MBPs. For example, they strengthen material stiffness in keratin and silk fibres. Thiol groups that form disulphide bonds are to the best of our knowledge not reported in any binding peptide for minerals or carbohydrates.
Spatial recognition of specific chemical interactions between MBPs and materials is achieved by a defined structural conformation of the binding interface between the peptide and the material. In naturally occurring carbohydrate-binding modules, three main binding interfaces are flat/plane-shaped, or with narrow or wide binding pockets (see type A, B, and C CBMs, respectively). In rare cases, two binding sites occur in a nMBP. These binding interfaces assist to accommodate materials/polymers with a diverse chemical structure. For instance, with type A CBMs crystalline areas of carbohydrates such as cellulose, chitin, and xylan can be addressed material-specifically. Type B CBMs are the most abundant form of CBMs, and accommodate carbohydrate chains with four or more monosaccharide units via large binding pockets. When recognition needs to be directed to a specific site of the polymer, type C CBMs can come in handy as they recognize the reducing or non-reducing termini of glycol-oligomers through their narrow binding pocket.
In contrast to carbohydrate-binding peptides, protein- and mineral-binding peptides do not rely on spatial recognition through 3D-binding pockets, as described above.
Clustering of amino acid sequences of reported nMBPs provides a comparison of their chemical properties (Fig. 17). For a comparison, each amino acid in the respective sequence was given a hydrophobicity value based on the scale of J. Kyte and R. F. Doolittle.447 Furthermore, charges were assigned based on the side chain (pH 7). Negative values were assigned for acidic side chains (−1: D, E), while positive values were assigned for basic side chains (+1: R, H, K). Keratin-binding peptides were found to have higher overall hydrophobicity (−2.1) to (14.7) and a neutral charge (Fig. 17) confirming the main interactions of keratin-binding peptides, as described in the previous paragraphs. In contrast to keratin, hydroxyapatite has an overall negative charge (0 to (−10)) and a lower hydrophobicity ((−12.1) to (−31.5)) (Fig. 17). nMBPs for aragonite, calcite, collagen, and silica differ in their chemical composition and a trend seems to emerge, although not further analysed (less than three data points per material were reported, see Table 1). Only the chemical composition of collagen-binding peptides was contrary to their proposed binding mode, which was mostly based on the hydrophobic interactions. This is described in the Sections “Engineering of binding peptides and proteins” and “Phage-display selected peptides (eMBPs, class I) and comparison to naturally occurring peptides”.
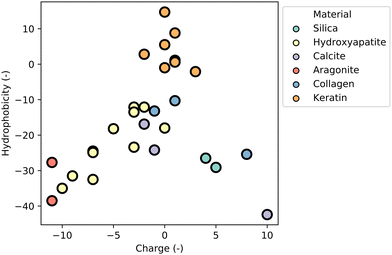 |
| Fig. 17 Clustering of nMBP sequences based on amino acid characteristics. Sequences of nMBPs were analysed regarding their amino acid content. For every amino acid, a charge (−1: D, E; 1: R, H, K) and a value of hydrophobicity447 was assigned and summed up. Hydrophobicity was plotted against charge. All sequences were clustered in colours regarding their binding preference. Hydroxyapatite-binding peptides clustered in the area of lower hydrophobicity and lower charge. Keratin-binding peptides showed a net charge close to zero and a low positive hydrophobicity. All the other peptides showed trends for clustering, but were not further analysed, due to a lack of reported sequences in literature. | |
In general, it can be stated that MBPs and their main binding driving forces correlate with their chemical composition on amino acid level, suggesting that a data driven approach for binding prediction of peptides without 3D structure is possible, and promising as an initial step.
eMBPs class I are short peptides (<20 amino acids) that often do not have defined 3D structures or binding domains. Binding motifs are primarily driven by specific chemical interactions, such as electrostatic, hydrogen bonds and/or hydrophobic interactions. Therefore, clustering of eMBPs class I based on their amino acid sequence permits to elucidate their binding properties and preferences.
eMBPs for carbohydrates bind through hydrogen bonds and electrostatic interactions. It has been found that hydrophobic interactions can also drive binding for uncharged (cellulose and chitin) and charged carbohydrates (chitosan, HA and PSA). It can be concluded that for materials like carbohydrates, driving forces of eMBP (class I) correlate with the driving forces identified in nMBPs, but lack a spatial recognition.
eMBPs for minerals, such as vaterite-binding peptides, showed a high similarity to cellulose-binding peptides regarding their charge (Fig. 18). The hydrophobicity range of vaterite-binding peptides was slightly decreased in comparison to cellulose-binding peptides. However, for vaterite binders, no crystal structures have been reported in contrast to CBMs. Sequences of silica-binding peptides could also be clustered based on their average amino acid characteristics, showing an average positive charge and a low hydrophobicity similar to that of the related nMBPs (Fig. 17 and 18). In contrast to silica binders, mineral-binding peptides for CC and HAP have neutral or rather positively charged sequences. The latter finding is contrary to what was reported for the corresponding nMBPs, indicating that their target might be the carbonate or phosphate groups in CC and HAP.
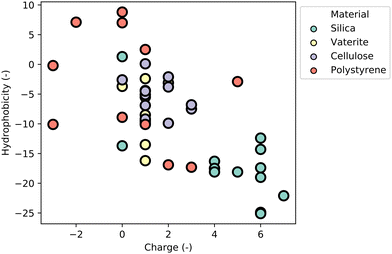 |
| Fig. 18 Clustering of eMBP sequences based on amino acid characteristics. Sequences of eMBPs were analysed regarding their amino acid content. For every amino acid a charge (−1: D, E; 1: R, H, K) and a value of hydrophobicity447 was assigned and summed up. Hydrophobicity was plotted against charge. All sequences were clustered in colours regarding their binding preference. While silica-binding peptides were clustering with a very low hydrophobicity and higher charge, cellulose- and vaterite-binding peptides had a similar charge, but differed slightly in their hydrophobicity. Polystyrene-binding peptides were not clustering at all. | |
eMBPs for protein-based materials showed the same amino acid characteristics (hydrophobicity and charge) as their nMBPs, despite of different binding motifs. The common motifs for collagen (G–G–X and G–X–HyP) and for silk (GAGAGX) have not been found/reported for eMBPs. Nevertheless, their interactions are based on aromatic amino acids (hydrophobic parts of fibres) and positive charges (hydrophilic parts).
eMBPs for polymers, as PS binders, could not be clustered based on their average amino acid characteristic (Fig. 18), indicating a more complex mechanism based on the identified sequences, as WXXW,470 FHXXW,486 and WXXWXXXW (see Section “Phage-display selected peptides (eMBPs, class I) and comparison to naturally occurring peptides”). No binding motifs identified by phage display could be found within MBPs with a defined 3D structure. Additionally, there are no nMBPs reported for synthetic polymers such as PS, PET, PE, etc. Therefore, no comparison between nMBPs and eMBPs for this category of materials can be made. Designing material-specific MBPs for man-made polymers is an emerging and enabling research field for microplastic quantification and sustainable polymer recycling processes. The de novo design of material-specific peptides with a defined 3D structure remains a great challenge to be solved, mostly due to the combinatorial complexity of the protein sequence space; even a short peptide with five amino acids generates a protein sequence space of 3.2 Mio different peptides. Measures to address the protein sequence space challenge and achieve computational supremacy over protein sequence space will be discussed with emerging applications in the Section “Discussion and outlook”.
Advances in nano- and microplastic analytics
Micro(nano)plastics (MNPs) are persistent pollutants whose increasing presence in land, water, food, and even air has generated concern about potentially harmful, but poorly understood effects on the environment and human health.546 While the initial scientific concern started many years ago,547 public attention has been captured by the growing media exposure given to microplastics in the environment and, more recently, to smaller sub-micron or nanometric plastic particles. The latter has been reported not only in the aquatic environment,548 but also in the air,549 drinking water, and food,550 possibly by release from food contact materials, such as feeding bottles157 and plastic tea bags.551 A staggering 42
000 tons of microplastics are released into the environment per year, as reported by the European Chemical Agency (ECHA).552 Potentially more alarming from a human health point of view are the reports of nanoplastics being internalized in the human body.553 Moreover, microplastics have a unique capacity to adsorb and accumulate various toxic substances, including persistent organic pollutants (POPs), heavy metals, and other harmful chemicals present in the environment.554 Unfortunately, the presence, distribution, and fate of MNPs is still poorly understood, due to the lack of methods harmonization for the larger microplastics and the near total absence of methods for the smaller sub-micron (<1 μm) nanoplastics. This problem has been highlighted by Schwarferts555 which, although published in 2019, reflects a scenario that still exists today (Fig. 19).556
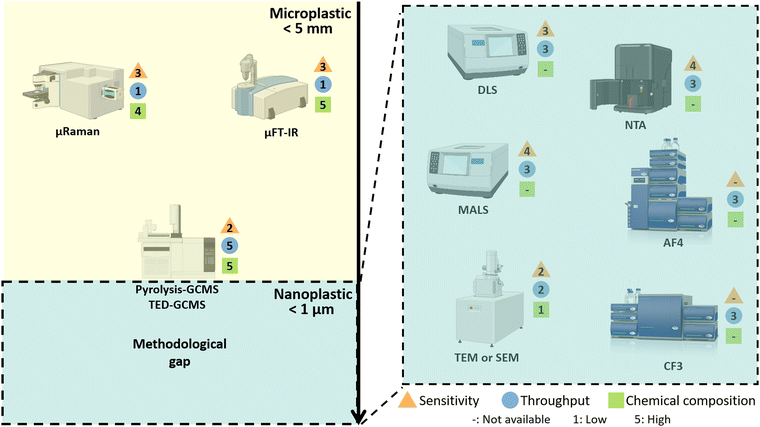 |
| Fig. 19 Established and developing techniques relevant to detection, quantification and characterization of micro- and nanoplastics. For particles less than 1 μm a methodological gap is present, which is driving the development of new or hybrid techniques. Detection techniques are rated for their sensitivity (triangles), analysis throughput (circles), and determination of chemical composition (squares), rated as 1 for low performance and 5 for high performance. No sensitivity is assigned to fractionation methods. Techniques such as HPLC, NMR, EM-EDX, CLS, TRPS and SLS were not included in the analysis, and they are thoroughly discussed by Schwaferts et al.555 and Caputo et al.556 In figure abbreviations: Fourier-transform infrared spectroscopy (FT-IR), dynamic light scattering (DLS), multi angle light scattering (MALS), nanoparticle tracking analysis (NTA), asymmetric field flow fractionation (AF4), centrifugal field flow fractionation (CF3), gas chromatography-mass spectrometry (GC-MS), thermal extraction and desorption (TED), transmission and scanning electron microscopy (TEM and SEM). Pictures for AF4 and CF3 devices were kindly provided by Postnova Analytics GmbH. | |
In the case of MNPs, identification and quantification can be undertaken using a limited number of techniques, each of which has specific limits, advantages and disadvantages. For particles with sizes of a few microns and above, the methods are established, but lack harmonization. In general, methods able to identify polymers fall into two categories: those based on optical microspectroscopy and those based on mass spectroscopy. In the first case, individual particles are visualized and identified as plastics using methods that combine light microscopy with vibrational spectroscopy, specifically IR-microscopy (μFTIR or QCL-IR) or Raman microscopy (μRAMAN). For the detection, identification, and enumeration of microplastics, the optical diffraction limit and detector sensitivity remain dominant factors in dividing the measurable from the non-measurable – μRaman may detect particles ranging from 0.5 μm to 1 μm, while μFTIR instruments with linear or focal plan array detectors can reach only around 5 μm to 6 μm. For these methods, the analysis time is a major factor when sample complexity often requires the analysis of hundreds or thousands of particles to achieve statistical relevance. For μFTIR and μRAMAN analysis, times of 0.5 to 1 days are realistic estimates for the analysis of sample derived from low solids aquatic sources. In the case of the more recently commercialised QCL-IR, this time may be reduced by a factor of 4 to 5, but compared to μFTIR, it may have more limited spatial resolution, and by acquiring spectra over a narrower spectral range may be more prone to misidentification when analysing weathered particles, as compared to μFTIR.557
Although the μFTIR/QCL-IR and μRaman techniques have become the workhorse methods for routine microplastics analysis, their lower size limits and modest throughput are pushing researchers to find new ways to improve their performance. In the case of Raman, studies indicate that the speed of analysis and spatial resolution can be improved by the use of stimulated Raman microscopy.558,559 In the case of -IR, it has been shown how improvements in performance can be achieved by substituting the conventional IR light source in a benchtop instrument with radiation from a synchrotron source.560 When applied to microplastics, the brightness and highly collimated nature of synchrotron IR light can produce higher spatial and spectra resolution, which can improve not only the lower size limit but the specificity of the spectral identification of microplastics, through improved signal-to-noise ratios.561 These variants show the potential for improvement to the family of vibrational microscopy-based methods, but cost and availability will present challenges to their more widespread application.
The second category, thermo-analytical methods (Pyrolysis-GCMS, TED-GCMS), use high temperature to decompose the polymeric materials into characteristic volatile chemical fragments, which are separated by gas chromatography (GC), identified by mass spectrometry (MS), and subsequently used to determine the polymer of origin. With appropriate calibration, the methods have the potential to identify and quantify the mass of polymers with a sensitivity of 0.1 μg to 1 μg, depending on the polymer. The main advantages of the thermo-analytical methods are the lower analysis times (0.5 h to 1 h) and the absence of any fundamental lower limit on particle size, provided the sample contains a sufficiently large number of particles as to reach the mass detection limit. In this respect, it should be noted that the mass detection limit of 0.1 μg is the equivalent of a single polymer particle with a size of 50 μm to 60 μm, and consequently, the method is much less sensitive than the μFTIR and μRaman methods (1 μm PE ≈ 0.5 pg). The key disadvantage of these methods, in addition to their limited sensitivity, is that they require time-consuming calibration for each polymer type, produce a mass-based result and cannot directly provide any information on particle size, shape or number (Fig. 19).
For the identification of individual plastic particles in the sub-micron range, the conventional vibrational spectroscopy instruments are not effective, but IR combined with atomic force microscopy (AFM) or Raman with Optical Tweezers562 can produce IR563 or Raman spectra with spatial resolutions compatible with nanoplastics. These methods, although promising, are highly time-consuming and their suitability for routine measurements has yet to be demonstrated. For ensemble measurement of particles smaller than 1 μm, the nanotechnology field offers many technical solutions for measuring size,556 and number or mass of particulates, but except for sp-ICPMS,564 all lack the ability to identify particle composition. In the absence of a revolutionary new technological breakthrough to overcome these limitations, the most promising strategy would be to look for ways to extend the capabilities of existing nanoanalytics, by providing a means to render plastic materials distinct from the natural background of organic and inorganic colloids. Since traditional analytical techniques for microplastic analysis are time-consuming, labour-intensive, and often limited by the sample throughput there is a growing need for cost-effective methodologies that enable high-throughput sampling and analysis. In this context, MBPs offer several advantages in microplastic analytics. They enable selective detection and differentiation of different types of plastics, which is crucial for understanding the composition and distribution of microplastics in environmental or food samples. Moreover, their application can be extended to different sample matrices, such as water, sediment or biological tissues. Thus, by using these peptides, researchers can develop selective and efficient methods to identify and analyse microplastics in various samples.
In the following section, we present different methods that have been adapted to detect nano- and microplastics, and offer promising technological opportunities in the field.
Emerging analytical techniques for nano- and microplastic detection and characterisation
Field flow fractionation (FFF).
A prerequisite to identify and analyse nano- and microplastics is their effective separation in size and nature from other polymer-based materials. While material specific labelling is often used as methodological approach for their fluorescence-based detection, the need to separate excess free labelled molecules from the plastic particles without disrupting the binding between label and nanoparticles presents a considerable challenge that cannot be neglected. For molecular species or small particulates in the lower nanometer size, size exclusion chromatography (SEC) offers means of purification possibilities,565 but the usable size range and the levels of surfactants necessary in eluents may make it unsuitable for many samples.555,566 As an alternative, in recent years FFF has begun to emerge as a powerful separation technique, which is compatible with water-based sample dispersions and eluants. FFF has been shown to be effective in the size fractionation of polymeric nanoparticles while also allowing their separation from low-molecular-weight molecules.567 In contrast to SEC, FFF methods do not use any static phase to obtain size separation. Size fractionation by FFF is based on pumping liquid dispersed particles through a thin, ribbon-like channel (typically 50 μm to 350 μm thick), which is subjected to a separating force perpendicular to the direction of the flow (Fig. 20). Under appropriate flow rates, the liquid moves under laminar flow conditions, producing a parabolic velocity profile across the channel with a maximum value at the centre, and decreasing to zero at the channel walls. When a separating force is applied perpendicular to the direction of the flow, particles in suspension move towards one wall of the channel, while counteracting diffusive forces tend to drive the particles back towards the centre of the channel. When these forces reach equilibrium, each particle tends to move along the channel at a characteristic distance from the accumulation wall, which is dependent on its diffusion coefficient and size. In general, the separating field helps to retain larger particles closer to the channel walls where the liquid flow is slower. As a result, smaller particles travel faster and elute earlier from the channel, while larger particles elute later.568 This mode of fractionation, small particles eluting first, is known as normal or Brownian separation. The separating field, depending on the FFF technique, may be a perpendicular liquid flow (asymmetric field flow field fractionation, AF4) or by centrifugal force (centrifugal field flow fractionation, CF3). Other members of the FFF family such as gravitational sedimentation and electric FFF are not discussed here as these have limited applicability to the particular materials of this study. The general applicability of FFF is attributed to ease with which it may be coupled to a broad range of detectors for concentration measurements, such as UV-visible, fluorescence and differential refractive index detectors. Online coupling of particle-sizing instruments such as multi-angle light scattering (MALS) and dynamic light scattering (DLS) detectors are also often applied (for improved quality in size measurements), and direct or off-line coupling to ICP-MS for elemental analysis is also known.569
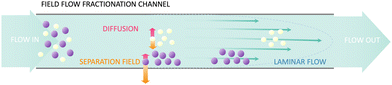 |
| Fig. 20 Working principle of field flow fractionation, smaller particles travel longer through the flow channel, while larger particles are retained. | |
AF4 has been shown to perform well in characterisation of nanomedicines,570 separating size fractions of polymeric nanoparticles, and in analysing protein–nanoparticle interactions in pre-clinical testing studies.567,571 However, comparing to other potential nanoplastic characterisation techniques, AF4 fractionation systems may not operate reliably as particle size approaches the micron size range,556 where the usual “normal” elution regime gradually transitions to “steric” elution with the larger particles eluting faster than smaller particles. In a standard AF4 channel,572 the onset of steric elution may occur as particle size increases above 0.5 μm to 0.8 μm. As an alternative or complementary fractionation method, CF3 coupled to various detectors has been successfully applied in the characterisation of nanoplastics.556,573 In the case of CF3, the sample fractionation occurs as the sample flows through a curved channel mounted on the rim of a spinning disc. To achieve the fractionation, the disc and channel are spun at high speed so generating a centrifugal field which moves larger and denser particles towards the outer wall of the channel where they elute more slowly than the smaller or less dense particles, which remain closer to centre of the channel.
Notwithstanding the upper size limitations of the FFF methods, current literature data highlights the potential of FFF in size-separating very heterogenous sub-micron plastic particles from complex matrices.555,572,574,575 For example, a study of carbon-based aggregates and nanoplastics have shown that appropriate selection of loading and elution parameters can allow operators to undertake a global separation across a wide size range (1 nm to 800 nm) or selectively target narrower size populations (e.g. 100 nm to 200 nm) to obtain higher resolution data. An important practical finding was that this flexibility can be achieved without modifying the mobile phase or the channel components.572 However, despite the power of the AF4 method as a tool to isolate/fractionate nanoplastics in environmental waters and marine food products,574,575 most studies reporting analysis of samples derived from biogenic sources are still focused on the fractionation of plastic particles sized above 0.1 μm.576 A recent exception is the study by Wahl et al.577 who have used py-GCMS analysis to determine the composition of size fractionated material from contaminated soil. Using a combination of AF4 fractionation and off-line compositional analysis by py-GCMS, it was possible to show for the first time the presence of heterogeneously shaped 0.02 μm to 0.15 μm nanoplastics in soil. Moreover, considering the major advantage of being able to selectively collect nanoplastic fractions for off-line analysis by e.g. advanced high resolutions imaging techniques, it is to be expected that AF4 will play an increasingly important role in revealing the presence of real-world nanoplastics in complex environmental or biological matrices.
Although less commonly reported, the other prominent FFF technique which could be to separate nanoplastics in environmental matrices is CF3. Schwaferts et al.573 compared the use of AF4 and CF3 (each coupled to UV, MALS and Raman detectors) for the separation of particles of different sizes and densities, such as PS 0.350 μm, PS 0.500 μm and PMMA 0.500 μm. Better separation was achieved with the CF3 technique due to the separation force that depends not only on the size of the particles, but also on their density. This would make this separation technique attractive for environmental applications where various plastics with different densities could be present in the sample. Furthermore, it is expected to offer advantages in separating denser inorganic particles from lower density polymer materials. In the normal elution mode, CF3 covers a wider size range (10 nm to 20 μm, depending on material and density) than AF4, but cannot reach the same minimum size, particularly for low density materials such as polymers.
An important aspect of the elution process in FFF methods is that it can often be run in a mild, water based, close to physiological medium, often even in phosphate buffered saline. These conditions allow for biomolecules and peptides to maintain their native structure, which is highly relevant for preserving the biological or pharmaceutical functionality of emerging materials such as nanocarriers for drug delivery, including lipid, virus- and polymer-based nanoparticles.578 Similarly, maintaining these interactions is important when studying the nature of environmental nanoplastics and their association with natural organic matter and pollutants.579,580 Finally, with a view to the future, coupling FFF analytics with innovative particle labelling methods based on material-specific binding peptides could provide advances in the sizing and identification of nanoplastics derived from environmental waters or biomatrices. However, while polymer identification based on fluorescent labelling of microplastics was already demonstrated,581–584 nano-sized plastic particle identification by polymer-specific binding peptides using AF4 or CF3 are yet to be explored.
Surface affinity-based sensors
Surface affinity-based sensors can be configured to enable the detection of, e.g. nano- and microplastics, as well as the analysis of their behaviour or interactions, in a real-time manner. In this type of technique, a molecular probe is usually immobilized on a sensor chip and the sample to analyse is injected over the surface through microfluidics. The interaction of a target present in the sample and the surface generates a physical change, which is transformed into a measurable signal. Two of the main techniques used in surface-based affinity sensors are quartz crystal microbalance with dissipation monitoring (QCM-D) and surface plasmon resonance (SPR).
QCM-D measures the oscillation of a piezoelectric quartz crystal, on which a change of the absorbed mass induces a modification of the resonant frequency. Moreover, dissipation monitoring can provide information on the viscoelastic properties of the material bound to the sensor surface. In the case of rigid films, dissipation is minimal, and the frequency shift is linearly proportional to the adsorbed mass as described by Sauerbrey585 with the equation: Δm = −C × Δf/n. The resonance frequency change, Δf, which results from the binding, is used to calculate the change of mass, Δm. The constant C is related to the properties of quartz and the parameter n in the equation is the index of the quartz crystal.
QCM-D has been used to investigate the binding of nano- and microplastics to environmentally relevant surfaces in different conditions. Alginate and natural organic matter (NOM) were for instance used to study the effect of particle shape on the attachment of PS spheres of 0.200 μm.586 The deposition of PS and polyethylene (PE) on NOM and silica surfaces was compared in another study.587 In the same way, the deposition kinetics of PS (0.050 μm and 0.500 μm) was measured on silica and alumina coated crystal sensors.588 The influence of two soil components (dissolved black carbon and humic acid) was also investigated on the deposition of fresh and aged PS nanoplastics, thus aiming to provide new insights on the stability and transport of these nanoplastics in aquatic environments.589 Similarly, the sensor surface of the QCM-D was coated with different extracellular polymeric substances naturally present in biofilm from Escherichia coli in order to assess the influence of biofilm on deposition of PS nano- and microplastics (diameters of 0.02 μm, 0.20 μm, and 2.00 μm).590 The role of water chemistry and particle coating was also investigated through the deposition kinetics of PS nanoparticles (and quantum dots).591 The quartz crystal can also be modified by polymer films, as was done to study the electrostatic adsorption of PS particles by QCM-D.592,593 These studies demonstrate the potential of the technique to investigate the influence of multiple parameters on the fate of nano- and microplastics in the environment. Indeed, the sensor's surface and the experimental conditions can be adapted for environmentally relevant assays. More importantly, the main drawback of QCM-D lies in the applicability of the Sauerbrey equation, which is limited to specific conditions of low absorbed mass and dissipation energy. Thus, mass calculation of nano- and microparticles is intrinsically biased as spherical particles, when absorbed on the sensor surface and immersed in a fluid, can induce turbulence thus deviating from the ideal Sauerbrey assumptions. The variation in dissipation makes the data treatment much more complex, with the risk of overestimation of the adsorbed mass of particles.
SPR also presents potential for application in nano- and microplastics analysis. This technique is based on an optical phenomenon occurring when a p-polarized light hits a noble metal surface at the interface of media with different refractive indices. In total internal reflection conditions, the incident light photons energy is transferred to the oscillating free electrons, which generates surface plasmons inducing a dip in the reflected light spectrum. The spectral position of the dip is related to the refractive index of the layer and angle in contact with the noble metal. As the analyte binds and dissociates at the surface, the refractive index varies and modifies the resonance angle, which is monitored in real time. The linear relationship between the amount of bound material, and the spectral position of the resonant peak permits quantification of the absorbed material, with a sensing depth of around 0.300 μm from the sensor surface. So far, this technique was rarely applied to nano- and microplastics analysis: a study focused on the detection of microplastics of PE, PS and polyvinyl chloride (PVC),594 while smaller PS particles (0.100 μm to 0.460 μm diameter) were characterised by SPR using the effective refractive index of the colloids.595 SPR effect occurs also in nanostructured surfaces. Localised SPR offers several advantages over conventional SPR including higher sensitivity and miniaturisation potential. The detection of nanoplastics using localised SPR was described using gold nanoparticles coupled to a peptide probe, where the absorbance intensity and wavelength shift were measured by UV-Vis spectroscopy.596
The low number of SPR applications in the area of microplastics research can probably be explained by two factors: because of the sensing depth limited to a few hundred nanometers, the method is generally applied to the detection of smaller analytes, such as biomolecules. Moreover, nanoparticles are generally dispersed together with molecules (e.g. surfactants, NOM), and SPR is a surface sensitive technique, which can detect molecular monolayers. The signal coming from a change of refractive index can be due to the bulk effect of particles, as well as to the binding of the other components of the sample analysed. The portion of the signal attributable to the absorption of particles alone can be difficult to distinguish. Additionally, molecules diffuse more rapidly than particles and can saturate the surface signal inducing an error in the quantification of particles or in the evaluation of their binding kinetics.
Advanced electron microscopy
EM based approaches are very powerful tools for detection and characterisation of nano- and microplastics, as these provide a high resolution and can be coupled with chemical analysis. However, EM techniques might require a sample preparation that modifies the sample conditions, such as chemical fixing, deposition of a conductive coating, substrate embedding and exposure to vacuum. Thus, these are not suitable for analysis in aqueous media or real time monitoring. Optical microscopy can be easily implemented in flow conditions but, in the standard bright field configuration, the resolution is too low for nano-size materials, due to the diffraction limit. Optical microscopy in dark field (DF) configuration permits the visualisation of smaller objects due to their scattering properties. DF microscopy is based on indirect sample illumination, owing to the use of a circular opaque light stop on the condenser that filters out the direct light, resulting in bright objects on a dark background. As a result of the scattering effect, particles much smaller than the diffraction limit can be visualised. The intensity and the spectrum of the diffracted light depends mainly on the size and the refractive index of the particles. DF microscopy enables tracking the Brownian motion of the nano- and microplastics and to directly measure their binding to a surface. In this way, the affinity of the particles for a substrate can be assessed. In a similar way to QCM-D or SPR, the surface can be functionalized in a way that is tailored to the purpose of the assay. For example, classical microscope glass slides were modified with polymers and polyelectrolytes to present a range of physico-chemical properties. These surfaces were then used to characterize the hydrophobicity of nanoplastics by measuring their adsorption to the different surfaces by DF microscopy.597–599 This approach provides high versatility, since the surface can be modified with a large range of functionality (inorganic layers, polymers, biomolecules, etc.), and the measurement conditions can be tuned for environmentally relevant studies. Moreover, providing a signal for each event, this method is sensitive to single nano- or microplastic particles.
Material-binding peptides for detection of nano- and microplastics
Fluorescent labels have been reported for the detection of nano- and microplastics. The most studied dye is Nile red, as it can label a broad range of polymers, but it does not have the specificity to distinguish between the polymer types.581,600,601 The lack of specificity is particularly problematic for the detection of nanoplastics compositions, which being largely C, H, O based, cannot be reliably distinguished from other background organic material usually present in real-world sample matrices. Thus far, few studies have reported sensitive and specific detection of nano- and microplastics using peptide biosensors. Material-binding peptides present a great possibility to expand in current available methods and combine labelling of plastic particles with the above-mentioned analytical and fractionation techniques. A study by Bauten et al. demonstrated that PS nano- and microplastics can be detected and quantified in a high-throughput manner after labelling particles with a plastibody consisting of a material-binding peptide and a fluorophore. To achieve high-throughput analysis of samples, labelled particles were analysed in combination with fluorescence-activated cell sorting (FACS), which allowed the analysis of millions of particles per minute.584 Moreover, the use of material-binding peptides for polymer detection has also been reported for different types of microplastics, such as PS, PP, and PE, but on one size range (106 μm > x > 75 μm).602 Fluorescent material-binding peptides used in this study were successful in staining PS particles derived from mice intestines. A prerequisite for the application of fluorescent peptides in real environmental samples is the reduction of the organic background by reagents such as Fenton's reagent, which dissolves the organic matter.603–605 Another potential method to reduce the background is the use of washing detergents (preliminary results). In addition, the goal of material specificity of material-binding peptides through protein engineering is of great importance in order to have a targeted effect on micro- and nanoplastics. The first successes in this respect were achieved by Lu et al.344,377 Moreover, material-binding peptides LCI and Tachystatin A2 conjugated with gold nanoparticles were used to colourimetrically quantify PP and PS microplastics with high sensitivity achieving detection range of 2.5 μg mL−1 to 15 μg mL−1.606 Interestingly, high degree of specificity of LCI and Tachystatin A2 to targeted polymers was demonstrated. The developed assay was validated using PS and PP spiked fresh lake water. In addition, PS detection immunoassay was developed using PS-specific polyclonal antibodies produced in vivo following immunization of rabbits with PS particles.379 Apart from colourimetric and fluorescence-based assay, material-binding peptides were utilized in localized SPR analysis, where page display derived PS binder served as material specific probe.607
As shown in previous sections, protein engineering of material-binding peptides offers a great opportunity to modify the binding properties of different peptides in order to achieve a specific labelling of plastic particles, which will enable the differentiation of synthetic polymers and organic matter, and even the specific detection of different types of plastic. The combination of specific labelling and analytical techniques such as FFF, FACS or microscopy-based, will enable the detection, identification, and quantification of nano- and microplastics in different types of samples.
Emerging applications of material-binding peptides
MBPs enable to functionalize material surfaces in a cost- and energy-efficient way at ambient temperature. Large toolboxes of nMBPs and eMBPs exist to functionalize natural materials (e.g. plant leaves, hair, teeth) and man-made materials (e.g. inert polymers such as Teflon, PE or graphene) as shown in previous sections. MBPs are compatible with scalable production technologies including unit operations such as dip or spray coating. Therefore, many applications could be envisioned within a sustainable bioeconomy based on renewable resources since binding properties (e.g. strength, material-specific binding, resistance against pH, salt or temperature) can be reengineered by protein engineering methodologies to match applications demands. Such problem-solution-fits or need-solution-pairs are under development for various applications. To the best of our knowledge, MBPs are not yet industrially used beyond diagnostic applications, since mainly chemical immobilization methodologies are employed.
Apart from the important and prominent example on micro- and nanoplastic quantification for monitoring food quality and environmental health, three emerging fields of MBP impact (biocatalysis, plant health, and medicine) will be highlighted in the subsequent paragraphs (Fig. 21).
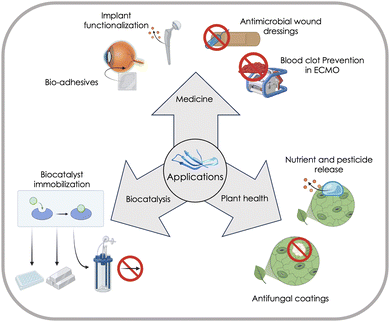 |
| Fig. 21 Highlighted applications of MBPs in biocatalysis, medicine, and plant health. In biocatalysis, MBPs enable immobilization of catalysts on surfaces like stainless steel or synthetic polymers for the construction of novel reactors. Medical applications include implant functionalization and anti-fouling effects to repel pathogens and increase implant compatibility. Applications of MBPs for plant health are focussed on nutrient release and pest control, that can enable more sustainable agriculture. Protein model was generated with ChimeraX 1.4.46 | |
All applications fields employ bifunctional MBPs. The second functionality besides material binding could be a biological functionality (e.g. an enzyme in biocatalysis application or antimicrobial feature in plant health), a chemical functionality (e.g. a chemical catalyst) or a functionality that changes physical properties (e.g. anti-fouling). Both binding domains a connected through a spacer sequence, that spatially separates both binding domains. Spatial separation of both binding domains ensures that those do not interact intermolecularly and, therefore, maintain their material-binding, catalytic or functional property. The following application areas will be discussed in this chapter:
Applications in biocatalysis comprise efficient immobilization of biocatalysts and chemocatalysts on various of material surfaces. Materials comprising polymers and stainless steel open up opportunities to design biocatalytic microfluidic and membrane reactors. Here we discuss the state of the art in immobilization matrices and enzymes.
Applications in medicine often require generation of biocompatible interfaces for implants, prostheses, and cell-growth/recruitment. Such biocompatible interfaces can be generated through MBPs. Functionalities such as anti-microbial and anti-fouling properties have been reported. Diagnostic applications are not discussed in this review since those are well summarized in several reviews.608,609
Applications in plant heath and agriculture are rapidly emerging and are considered as highly promising, especially for the reduction of pesticide use. MBPs provide a superior rainfastness compared to standard chemical pesticide formulations and can be fully biobased and degradable without accumulation in the environment.
Biocatalysis
In general, MBPs are fused through a stiff linker to an enzyme338 or a chemical catalyst,610 to enable an oriented and dense coverage of the surface material. The oriented immobilization ensures that enzymes are usually immobilized in a functional manner. A suitable linker length ensures that enzymes maintain their flexibility, which is often a prerequisite for a high activity. MBPs, referred to as MatterTaq, have been reported, in the study from Dedisch et al.338 to be generally applicable and scalable immobilisation toolbox for various enzymes on polymer surfaces.
Enzymes immobilized through MBPs have mostly been monomeric enzymes (e.g. phytase, cellulase and laccase). To the best of our knowledge, no multimeric enzymes have been reported to be immobilized through MBPs.
Polyethylene terephthalate has been reported in three studies as polymer matrix for immobilization of catalysts. The phytase enzyme YmPh and the cellulase CelA2M2 were successfully immobilized using the peptide Tachystatin A2. For both enzymes, the residual activity on the surface was improved more than 3-fold by the presence of the MBP.338 The immobilisation of phenolic acid decarboxylase (PAD) on PET was performed by Büscher et al. Functionalisation of PET with PAD using the MBP Dermaseptin S1 resulted in a substrate conversion of 88% within a reaction time of 2 h.611 This approach enabled the enzymatic decarboxylation of ferulic acid to 2-methoxy-4-vinylphenol on the additively manufacturable material. Since this product is a strong inhibitor of the enzyme, in situ product removal was needed to be applied in the form of extraction with n-heptane. PET, as an additively manufacturable material, could be used to create optimal packing structures for the in situ extraction. In a study regarding plastic degradation, immobilisation of the cutinase Tfuc2 from Thermobifida fusca was achieved using Dermaseptin S1. The immobilization resulted in a 22.7-fold increased degradation rate of PET, resulting in a degradation of 57.9% within 96 h at 70 °C. The material-binding peptide mimics the function of natural binding domains like cellulose-binding domains in cellulases by guiding the depolymerizing enzyme to the material. This functionality becomes especially important for the application within diluted suspensions of microplastic.612
Polypropylene was used as a material for immobilization of the copper efflux oxidase (CueO laccase). Immobilization of the enzyme acted as a method of semi-purification to facilitate enzyme engineering by lowering the background activity. Surface functionalization was achieved by the MBP LCI with the enzyme being attached to the bound peptide by posttranslational peptide ligation. This method, which employs the sortase A enzyme from Staphylococcus aureus enables the efficient construction of fusion proteins that cannot be expressed in their full form. The system was used for laccase and sortase engineering, enabling the identification of variants with higher specific activity.613 Immobilization of biocatalysts on polypropylene was also achieved for the phytase enzyme YmPh and the cellulase CelA2M2 by Dedisch et al. Here, the enzyme activity on the surface could be increased up to 11-fold. Additionally, the immobilization of YmPh on PP showed residual activity of the enzyme on the surface of 52%, after eight rounds of washing. Additionally, LCI was identified as the best binder for both enzymes when targeting PP.338 Additionally, a variant of the MBP LCI (LCI F16C) was used to immobilize a synthetic Grubbs–Hoveyda type catalyst on polypropylene, enabling ring-opening metathesis polymerization on the surface.610
Polystyrene was used in several studies. LCI and Tachystatin A2 were identified as effective PS-binders and two enzymes the phytase YmPh and the cellulase CelA2M2 were functionally immobilized on PS. Similar to PP, it was found that YmPh immobilized on PS showed 60% residual enzyme activity after eight cycles of catalysis and washing.338 Additionally, the application of sortase mediated protein ligation was used to enable the attachment of various functionalities to the LCI MBP. Among other surfaces, PS was reported as a suitable support here.614
Polyester–polyurethane was targeted for its biocatalytic degradation, as described for PET above. The material-binding peptide Tachystatin A2 was fused to the bacterial cutinase Tcur1278 and was shown to promote adhesion to the polymer. The degradation of the polyester–polyurethane nanoparticles was enhanced 6.6-fold and half-life of the particles was reduced 6.7-fold. More interestingly, it was shown that the anchoring effect was very prominent in highly diluted samples by actively directing the enzymes to the particles.615
Gold surfaces were chosen as another showcase of the MBP-based enzyme immobilization. Dedisch and coworkers identified LCI as a potent binder to immobilize both enzymes, while the eGFP reporter protein could also be immobilized well using Tachystatin A2. For the immobilization of YmPh phytase using LCI, the surface coverage was evaluated, identifying the coverage of YmPh-LCI to be 2.6-fold higher compared to the YmPh wildtype.338 Additionally, adsorption kinetics and Langmuir isotherms were recorded to permit a better understanding of the binding. Another application of material-binding peptides on gold surfaces were used to immobilize the P450 BM3 enzyme. Cytochrome P450 enzymes (CYPs) in general shows poor stability, often resulting in low activity on industrial substrates. Immobilizing CYPs was shown to improve stability and enable efficient separation of enzyme and products, which is especially interesting for long-term operation. The application of material-binding peptides removes the necessity to embed the protein or perform mutations across the entire protein to enable immobilization. Additionally, it enabled adjustment of the distance between the enzyme and the surface to assure a desired orientation of the enzyme, and to avoid denaturation.616
Stainless steel was reported as another surface for biocatalysis. The immobilization of YmPh phytase and CelA2M2 cellulase was characterized with the interesting observation that no phytase activity was found for the wildtype YmPh, and catalytic activity on the material could only be achieved by addition of the MBPs. Fusion of the cellulase with MBPs led to and increased activity on the stainless-steel surface, with the highest increase of 2.3-fold observed when using the MBP Cecropin A.338
Indium tin oxide served for the immobilization of P450 BM3 variant, as described above for the gold surfaces. Affinity of the P450 enzyme to the surface was increased by the fusion to the MBP HighSP-BP, which resulted in higher enzyme activity compared to the soluble form.616
The peptides Cecropin A, LCI and Tachystatin A2 were identified as potent candidates for the immobilization of enzymes on silicon wafers. The activity of both investigated enzymes (YmPh phytase and CelA2M2 cellulase) was significantly increased (up to 6-fold) by fusing the three investigated MBPs to the enzyme's C-terminus individually.338
In respect to enzyme class, no limitations seem to exist since nine enzymes of different classes were described to function in peptide-immobilized form. Moreover, the immobilization of synthetic catalysts was enabled using the same approach. Building on the immobilization of single enzymes, the immobilization of enzyme cascades can enable efficient conversion of various compounds by combining multiple enzymatic reactions in one process.617 The immobilization in flow reactors can allow for the application of enzymes or hybrid catalysts in continuous reactions, while also distributing catalysts for individual conversion steps throughout the reactor. Immobilizing enzymes in membranes offers the possibility of combining enzymatic activity with selective effects of the membrane.618
In similar approaches, biocatalysts are immobilized on microbes like Escherichia coli, to mainly facilitate enzyme engineering campaigns.619,620 Further development of this approach would open up the generation of hybrid, living and catalytic materials. However, currently, there are no MBPs directly applied in this field.
Medicine
Main application of MBPs in medicine are related to generate biocompatible interfaces for implants, prostheses, and cell-growth/recruitment and embedded additional functionalities. Here we will give emphasis on anti-microbial and anti-fouling properties due to their societal and industrial importance.
Since wound dressings are applied when critical points of entrance for pathogens are present, a killing and repelling function is highly desirable to avoid infections. Two approaches have been developed to target these aspects. Killing of the pathogens can be performed by endolysins, bactericidal enzymes, that originate from bacteriophages. Production of the endolysins at the end of their reproductive cycle results in the cleavage of the peptidoglycan layer and release of the phage. Unlike antibiotics, bacterial resistance to the endolysins is regarded as highly unlikely, presenting a great potential for the clinical treatment of infections. On the other hand, endolysins do not present any harmful effect on eukaryotic cells. An anti-fouling effect can be achieved by attaching polymer brushes to a surface. Brushes from zwitterionic carboxybetaines were shown to mediate resistance against protein adsorption and bacterial adhesion, since their jungle-like properties prevent bacteria from interacting. Additionally, the polymer brushes could render wound dressings less adhesive for mammalian cells, reducing the release of proinflammatory cytokines and minimizing tissue damage from adhesion. Unfortunately, the drafting of polymer brushes to the surface is not scalable due to required plasma treatment. Growing polymer brushes from MBPs solves the scalability challenge in an elegant manner. Since the immobilization of endolysins was shown to be possible by the application of MBPs as well (e.g. LCI-PlyGBS94), a combined solution of endolysins and polymer brushes, immobilized through MBPs could be realized. The combination of both properties by creating a certain ratio of both molecules on the surface resulted in a 93% reduction of bacterial concentration and is pivotal within the early stages of wound healing.621
Biofouling presents a major challenge for the functionality of medical devices, if they are in constant contact with a biologically active environment. One example is the construction of extracorporeal membrane oxygenations systems. These systems are utilized to treat patients with isolated or combined cardiopulmonary failure. The oxygenators consist of hollow fibres, that mediate the oxygenation and decarboxygenation of blood flowing through. Protein adsorption on the fibre surface, however, presents the risk of coagulation cascades resulting in clot formation, which drastically reduces the oxygenation efficiency and causes life-threatening complications. As a precaution measure, anticoagulation agents can be applied, which, however, drastically increase the risk of bleeding complications. The release of nitric oxide (NO) contributes to the thromboresistant properties of blood vessel epithelium and could be transferred to the oxygenation system as well. Research was performed on the immobilization of enzyme mimetic non-fouling microgels for the release of nitric oxide. To achieve immobilization, the poly(4-methyl-1-pentene) (PMP) fibre mat of the oxygenator was functionalized using an eGFP-LCI fusion protein. After washing off, the microgel containers were attached to the protein using a thiol-epoxy click reaction. Immobilization of the microcontainers was verified using X-ray photoelectron spectroscopy and the system effectively reduced blood clot formation in a 48-minute test trial.622
Introduction of implants to the human body is always linked to the risk of implant rejection due to the implant being detected as a foreign harmful object by the immune system. Furthermore, the site of implantation opens the possibility of entrance for pathogens, that can cause inflammation leading to implant rejection. To sustain implant compatibility, two strategies can be followed, either increasing positive interaction between mammalian cells and the implant or by releasing antibiotic compounds from the implant surface. Due to their great biocompatibility and mild binding conditions, the application of MBPs offers a possibility to follow these strategies.
Adhesive effects mediated by MBPs were used to increase the cell attachment to polydimethylsiloxane (PDMS) surfaces. PDMS has a number of beneficial properties for the use in biomedical applications including high flexibility, transparency and permeability for oxygen. The material is typically used for the fabrication of biocompatible devices like heart valves and microfluidic devices. These devices enable examination of cell behaviour regarding different aspects like physical stimulation, stretching or stiffness. A major drawback to this application is the limited interaction between eukaryotic cells and the PDMS surface. Large ECM proteins are typically used to render the surface more adhesive for mammalian cells, however, batch to batch variety and production cost of these proteins present some major drawbacks. A more defined approach to increase cell adhesion on PDMS surfaces was presented by fusing a cellular adhesion sequence (GRGDS) to the material-binding peptide LCI. This sequence presents the minimal recognition motif of the fibronectin ECM protein. Application of the peptide on PDMS surfaces was shown to increase cell viability and application on structured surfaces resulted in directional cell growth. Besides the presented approach, there are different cellular adhesion sequences, that could be applied in a similar way to present different signals to the adhering cells. Additionally, immobilization of growth factors might be a promising application of the material-binding peptides for cell characterization. Both approaches could be combined in the establishment of a peptide-based toolbox for cell adhesion applicable for a variety of different surfaces and cell types.623 Another application of this concept is presented by the attachment of epiretinal visual protheses, which are the most common treatment in the case of retinal degenerations. Here, a peptide-based approach for the fixation of epiretinal stimulating arrays was proposed as an alternative to the conventionally used titanium retinal tacks. These tacks are known to cause unavoidable retinal damage and removal of the prostheses is aggravated, which illustrates the need for an effective fixation method. To enable epiretinal fixation, the arrays for retinal stimulation can be coated using Poly[chloro-p-xylylene] (Parylene C). Parylene C is typically used in medical applications since it shows high biocompatibility and, moreover, presents transparency and flexibility as beneficial features for the application in retinal protheses. Parylene C could afterwards be fixed on the extracellular matrix (ECM) of the surrounding cells using material-binding peptides. The most important proteins of the ECM include collagen, laminin and fibronectin, which can be specifically targeted by binding peptides. Within the presented work, a bioadhesive peptide consisting of the material-binding peptide cecropin A, a domain Z spacer and the peptide osteopontin was used to obtain a first proof-of-concept of attaching the Parylene C material to the surrounding tissue. This enabled a non-invasive fixation of epiretinal visual prostheses with high applicability and biocompatibility.624
Antimicrobial effects by compound release were established on bare-metal alloys like stainless steel, which are commonly used in implants. These surfaces could be treated chemically or physically to create compound releasing layers before. Through the combination of two material-binding peptides, the surface can be covered with micro-containers of a different material. In this project, polycaprolactone (PCL) and stainless steel were crosslinked using the biadhesive peptide approach. Five different material-binding peptides were screened for their binding to the two chemically different materials. Dermaseptin S1 was identified as a binder for stainless steel, while LCI showed best adhesion to PCL. Both peptides were combined to a biadhesive molecule with a domain Z spacer. The immobilization of PCL particles on the stainless-steel surface was confirmed by microscopy. The particles were loaded with kanamycin and kanamycin release was detected by inhibition of cell growth after Escherichia coli cells were incubated with coated stainless steel. The presented system features wide applicability, since different surfaces could be functionalized, and a variety of compounds could be loaded to the micro-containers. General medical interest can be expected for this system since the release of compounds on a surface is widely needed.625
Plant health
The key challenges in sustainable agriculture and the production of healthy food involve minimizing pesticide use and ensuring their effective degradation. Formulations for the delivery of pesticides and nutrients must be as degradable as the agent itself and contaminations of soil with microplastic must be avoided especially. The significance of this goal was elucidated by a European Union legislative call in 2021. MBPs that decorate microgels (pesticide loaded containers) and bind to the wax layer of plant leaves in a rainfast manner are a promising solution. The underlying technology is termed greenRelease. The microgel degradation speed and compound release can be controlled through cross-linking between the polymer chain. Through control of pore size and chemical modification of the polymers, small ions (e.g. copper salts or nutrients) as well as larger pesticide molecules and proteins can be incorporated efficiently (up to 30% of the polymer dry weight).626 These microgels can further be enhanced for biodegradability by utilizing biopolymers, such as cross-linked polysaccharides. An alternative approach is presented by bifunctional peptides, in which antimicrobial peptides (AMPs) are fused to the MBPs. The advantages of AMPs are that they can target specific pathogens (e.g. against soybean rust)627 and employed AMPs are often harmless for humans. The main drawback of bifunctional peptides compared to the greenRelease technology is the amount of pesticide available; even though millions of bifunctional peptides (3 nm to 10 nm in size) are immobilized per cm2 of surface, the amount remains low compared to the contents of micrometre-sized microgels.
The main advantage of MBPs over standard formulation is that MBPs achieve an excellent and strong binding to wax layers of plant leaves and the binding strength and rainfastness can be tuneable by protein engineering.628 The strong binding is advantageous for farmers since fewer rounds of pesticide applications are necessary and standard spraying equipment can be used. MBPs are usually anchored in wax layers of plant leaves and likely move in the wax layers like proteins in biological membranes. Growth and photosynthesis of plants are not affected by the formulation, and its biodegradability can be tuned to application demands and pesticide/nutrient release (e.g. ∼50% present on plant leaves after 3 weeks).
In the following section one greenRelease and one bifunctional peptide approach are presented to illustrate the problem-solution fit that MBPs can provide to ensure healthy food production.
The controlled release of micronutrients in the form of trace elements was investigated using biohybrid microgels. The microgels were functionalized with strong chelating ligands for Fe3+. To mediate adhesion to the plant surface, different material-binding peptides were screened, resulting in the selection of Plantaricin A as a binder to plant surfaces. The immobilization of the microgel and the Fe3+ could be verified by treating iron deficient cucumber plants with the formulation. Since the introduced iron contributed to chlorophyll formation, the efficacy of the release could efficiently be monitored by regreening of the leave surface. A comparable approach could be followed using pesticides instead of nutrients in an accordingly modified microcontainer. Here, the same principles apply to achieve a rainfast application of the compounds while also facilitating release over a prolonged period, reducing the frequency and intensity of treatment, that is needed.629
A similar approach to reducing pesticide usage was presented using the direct fusion of two peptides. Active agents are not released from a container but are immobilized on the leaf surface directly. The focus of this research was on the Asian soybean rust (Phakopsora pachyrhizi). The aim was to adhere an active agent to the soybean leaf and hinder Phakopsora pachyrhizi spores from germination. This was achieved by fusing the material-binding peptide Thanatin with the antimicrobial peptide Dermaseptin 01. Thanatin was chosen based on screening of peptides, that were fused to the fluorescent reporter eGFP for detection. The peptide showed rainfast adhesion, that was also stable under elevated temperatures and sunlight over a time of 17 days. The antimicrobial effect of the bifunctional peptide was evaluated in vitro by inoculating soybean leaflets with Phakopsora pachyrhizi. A reduction in infection severity was recorded when treatment with the peptide was performed, with the reduction being more apparent when the leaf was rinsed. Direct attachment of the active compound to the leave is overall less complicated than immobilized microcontainers in terms of production of the formulation. Without the fabrication of the microgel, the application becomes even more cost effective. The direct immobilization of active compounds could, therefore, be a valuable option whenever the compound can be fused to a material-binding peptide in its active form.630
In addition to laboratory research and field trials, the interaction between material-binding peptide and leaves was investigated using computational approaches. To the best of our knowledge, this presents the only published approach in which molecular dynamics (MD) simulations were employed to engineer peptide binding to a heterogenous surface like plant wax.628 In the study conducted by Dittrich et al., the binding of MacHis peptide to the cutin matrix of apple wax was simulated. The simulation system consisted of a cellulose layer as a foundation, with a matrix of polyesters (specifically, linked 10,18-dihydroxyoctadecanoic acid) added on top. Random components of wax, which composition had been previously analysed using GC/FID and GC/MS techniques, were placed on the surface. The entire system was equilibrated through a 100 ns NVT MD simulation run.
Subsequently, the MD trajectory with the binding of MacHis peptide to the wax surface was analysed to determine the relative contact of each amino acid in MacHis with the surface. Eight amino acids exhibiting significant contact were selected for in vitro alanine scanning. MacHis variants with changes in their amino acid sequence were conjugated to enhanced green fluorescent protein (eGFP). Among the tested variants, specifically R6A, Y16A, and R6A/R10A, a highly reduced remaining fluorescence was observed after five rounds of washing, similar to the negative control (eGFP). Other variants also displayed a significant decrease in remaining fluorescence compared to the wild-type MacHis. In summary, the positions R6, R10 and Y16 are important for the rainfast binding of MacHis to the wax layer of plant leaves and these positions can be used to tune the rainfastness binding.
The potential mean force (PMF) of MacHis and several other peptides (Plantaricin A, LCI, Pleurocidin, and Magainin) for desorption from the surface was assessed using adaptive steered MD (ASMD). The simulation results were further compared to the corresponding in vitro experiments and matching results were found for Plantaricin A, Pleurocidin and Magainin. This shows that PMF and ASMD are suitable for the investigation of peptide desorption behaviour from the plant wax surface. Notably, against the predicted order, LCI exhibited higher rainfastness in vitro compared to MacHis. Even though this contradicts the expectations derived from previous simulations, the study presents a profound approach to identify key residues for wax layer binding. Understanding on a molecular level driving forces for material-specific binding to wax layers will enable the design of next generation MBPs that bind to plant leaves and not to the often more hydrophilic surface of fruits. The latter opens up possibilities to deliver pesticides to plant leaves specifically and thereby protect consumers.
Discussion and outlook
The size of the protein sequence is a curse and blessing at the same time since it is unimaginably large. Its diversity allows to design specific antibodies and material-specific binding peptides; however, the theoretical protein space of even short proteins can only be explored to a neglectable fraction by experimental means (see Section “Engineering of binding peptides and proteins”).631 Diversity supremacy of the protein sequence space is therefore, the ultimate goal for efficient design of powerful material-specific binding peptides that match application demands. In Fig. 22, we provide a roadmap composed of 18 challenges to achieve Diversity Supremacy through an understanding of biophysical principles by generating sufficient experimental data, which enables computational methodologies to explore and exploit the theoretical protein sequence for the design of powerful material-binding peptides. In addition to the general challenge of achieving computational diversity supremacy in MBP-design, specific challenges for applications in plant health, medicine, biocatalysis, and NP-/MP-management (emphasized) are discussed.
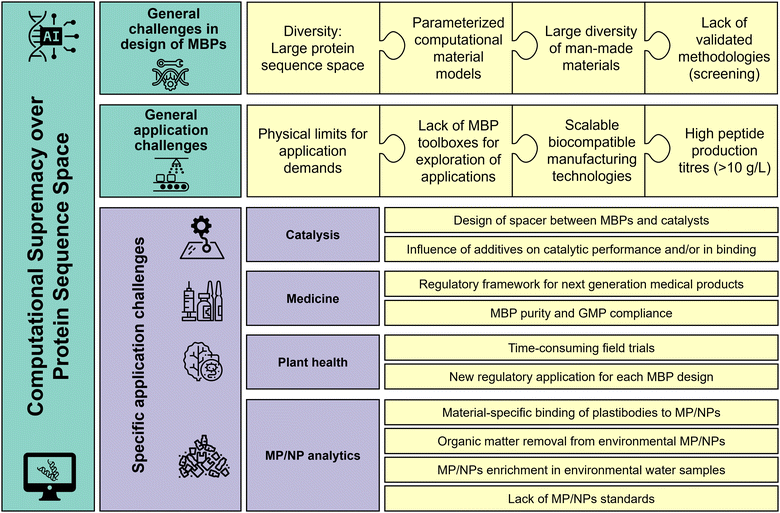 |
| Fig. 22 Challenges to achieve the ultimate goal of computational supremacy over the protein sequence space and achieve full in silico design of MBPs. The overview represents a challenge list to illustrate a roadmap to MBP innovations, reaching computational supremacy in MBP design and is accordingly divided into the “general challenges in design of MBPs”, “general application challenges” and “specific application challenges”. All challenges will be presented and discussed in the following paragraphs. | |
I. General challenges in designing material-specific and powerful MBPs are: (a) large diversity of man-made and natural materials in respect to composition and structure, (b) lack of parameterized computational material models and defined reference materials, (c) lack of systematic protein engineering strategies to understand driving forces of material-specific binding, (d) lack of validated methodologies, especially screening system for directed MBP-evolution in respect to material-specific binding and addressing the diversity challenge of the huge protein sequence space (see Section “Engineering of binding peptides and proteins”). Addressing the challenges from (a)–(d) are prerequisites to generate a molecular understanding of interactions between MBPs and material surfaces and identifying biophysical driving forces.
(a) Large diversity of man-made and natural materials in respect to composition and structure
Man-made materials and especially polymer-based products are often composed of mixed-plastics with hundreds of combinations that differ in their chemical composition, crystallinity, morphology, and contain in a low one-digit percent range additives with diverse properties and functions.484,485 In contrast, natural materials are based on a comparatively low number of materials, their properties are acquired in sophisticated mineralisation or supramolecular assembly processes.5,632–634 Therefore, changes in chemical composition, wettability, and degree of crystallinity are the main challenges to be addressed by MBPs.635,636 The example of nMBPs binding to cellulose shows, that natural cellulose-binding domains can preferentially bind to crystalline cellulose or amorphous cellulose depending on the specialized cellulase function.54 Phage display-derived peptides that bind to semiconductor materials can exhibit binding preference for the crystalline areas637 and have usually a promiscuous binding behaviour due to a lack of a defined binding domain.
Morphology is likely not a main challenge for MBPs, since MBPs have a size of 1 nm to 5 nm so that even small morphological differences e.g. in height, that often range from 1 μm to 5 μm in materials, will not influence MBP binding interactions; scaled to a 1 m MBP in size, the difference of 1 μm to 5 μm in material morphology would appear like a mountain of 1000 m, so that the direct surroundings will be ‘seen’ by an MBP as an even topology. Nevertheless, defined morphological patterns can add functionalities such as antifouling or superhydrophobic effects to products in various application fields.621,638,639
In summary, the large diversity of man-made and natural materials will likely require an array of specialized MBPs to material-specifically address difference in crystallinity and composition, especially in mixed-material products.
(b) Lack of parameterized computational material models and defined reference materials
The prerequisite to generate a molecular understanding of MBP-material surface interactions are parameterized computational models of materials, which we mostly lack (see Section “Computationally assisted design of polymer-binding peptides”). Parameterized surface models were already created for several crystalline polymer structures (e.g. PE, PTFE, PET491 or PVC640) and natural surfaces (e.g. apple leave wax628). There are some models reported for amorphous surfaces,640,641 however, the accurate representation of these surfaces is more challenging. The computational models are required to mimic material properties on a structural level, especially in respect to crystallinity and mixtures of crystalline and amorphous areas that are often important for macroscopic material properties. In addition, energetical considerations of e.g. partial charges of surface molecules must be made to most accurately model molecular interactions. Good knowledge of surface properties is needed to implement correlations between findings from computational analysis and laboratory analysis. Often employed MD simulations require substantial computational resources and time to be performed. To efficiently evaluate the different energetical states and to find the global minimum in the energy landscape of the simulation, sampling techniques can be employed, that most efficiently use the limited computational power. Examples of this approach include replica exchange molecular dynamics simulation, that runs multiple simulations in parallel and exchanges replicates repeatedly to probe the energy landscape.535 However, the application of these sampling techniques was only done in few examples for material-binding peptides. Additional work is needed for optimized implementation of these methods.
Limited amount of work has been invested into the simulation of the interaction between peptides and polymer surfaces. To build well-founded knowledge on the interaction, the complexity of the system should be increased stepwise. Identifying interactions with crystalline surfaces first, will give a basic understanding of interaction principles. Afterwards, the material complexity can be increased by including amorphous surfaces, surfaces with mixed crystallinity and mixed materials. Changes in the interaction principles, that are observed with more complex systems, can provide insights on how the peptide interaction is altered. It is needed to introduce material complexity gradually and include experimental validation to the workflow to confirm good material representation in the simulation. In combination with the constantly increasing computational power available, the computational analysis of complex systems in MD simulations will become a shrinking hurdle in the field of material sciences. In summary, this trend will, in combination with the rapid development of machine-learning approaches, lead to major advances in computational research on surfaces and surface interactions.507
(c) Lack of systematic protein engineering strategies to understand driving forces of material-specific binding peptides
In the section on lessons learned, binding motifs, molecular interactions and the role of structures of nMBPs and eMBPs are summarized in respect to material binding. Open questions concern the lack of a comprehensive protein engineering strategy to elucidate general principles for material and material-specific binding and the role of the 3D-structure of binding peptides. Experimental studies have been limited to phage display methodologies that usually employ peptides with less than 16 amino acids (limited in material-specificity) and a few directed evolution studies that are limited by the number of screened variants and biased diversity due to the application of random mutagenesis methods.258,642 Employed random mutagenesis methods have a preference to replace a large base by a large base or a small base by a small base (termed transition bias) which limits on the protein level the generated type of amino acid substitutions.643 A systematic study on the BSLA lipase and comparison of standard random mutagenesis library, obtained by error-prone PCR, to a library of the same BSLA lipase that contained all natural diversity at each amino acid positions (site saturation mutagenesis (SSM) library), concluded that general design principles cannot be deducted from epPCR libraries since only 20% to 30% beneficial positions could be identified. However, the SSM library of BSLA that contained all the natural diversity with one amino acid exchange (∼3600 variants) was successfully used to elucidate general design principles of lipases in respect to improved tolerance against organic solvents644,645 and ionic liquids.646,647 Such an SSM-library approach with MBPs, and screened against several man-made materials, with the aim to study contributions of hydrophobic, polar, and charged interactions is likely a promising and systematic strategy to generate the experimental data required for AI- and machine learning approaches to advance towards in silico designed MBPs and also to enable the de novo design of MBPs.648
(d) Lack of validated methodologies, especially screening system for directed MBP-evolution for material-specific binding
Nearly all phage-display and directed MBP evolution approaches have been performed to improve binding properties to target materials. Resulting changes in binding properties to other materials are also investigated, for instance directed evolution of the MBP LCI to improve binding to polypropylene resulted in a variant with altered binding properties to polystyrene.1,2,329 Screening concepts, in which material-binding preferences are selected in a competitive screening format are missing. Pioneering concepts are the work of Yi Lu et al. where a microtiter plate assay was developed in which PLA-binding preference over PP binding was improved 4.45-times. The screening system in 96-well plate format was separated into two screening steps; from the master mutant library plates the Cg-Def-EGFP (MBP-eGFP) variant containing supernatants are transferred into PP and PLA-coated MTPs and the remaining fluorescence ratio of the same variant in the PP and PLA plate after washing are compared and used as selection criteria (measure for the binding strength).377 A more detailed depiction of available validated methodologies for directed MBP-evolution can be found in the Section “Engineering of binding peptides and proteins”.
In summary, competitive screening concepts and systems that are close to application conditions and that employ mixed materials will in the future be required to design powerful MBPs (see specific application section).
General application challenges are (e) MBPs binding properties are often insufficient to match application demands, (f) lack of general applicable toolboxes of MBPs fused/conjugated to biological, chemical or physical functionalities to explore applications, (g) lack of validated biocompatible and scalable production technologies to functionalize materials on surfaces or to integrate bifunctionalities within materials, (h) often lack of sufficient production titres of MBPs (>10 g L−1), and MBPs as well as conjugates are often not addressed or implemented in regulatory frameworks. Regulatory challenges are addressed within the challenges of individual application fields.649–652
(e) MBPs binding properties are often insufficient to match application demands
As a protein, MBPs have physical limits in respect to their thermal resistance (in general <90 °C). Tolerance towards molar-concentrations of product/substrate, proteolytic digestion, detergents, salts, and pH need often to be improved, since industrial application conditions can differ vastly from the natural environment that enzymes are exposed to (e.g. in metabolic pathways of microorganism). Tailoring of enzyme properties to application demands by improving above mentioned properties is routinely performed by directed evolution methodologies653,654 for instance by following the KnowVolution655–658 design strategy. Improved properties in above mentioned applications have not yet been reported, but can easily be included and selected for in reported screening systems for directed evolution.1,2,329 Additional challenges, for MBPs that arise from scalable production technologies (e.g. binding within seconds for dip coating processes) have to the best of our knowledge also not yet been reported despite their importance for MBP innovations.659,660
(f) Lack of generally applicable toolboxes of MBPs fused/conjugated to biological, chemical or physical functionalities to explore applications
The general application potential of MBPs that are fused or conjugated to biological functionalities (e.g. enzymes, anti-microbial peptides, anti-freeze proteins etc.338,615,638), chemical functionalities (e.g. catalysts610), functionalities that alter physical properties (e.g. water-repellent or superhydrophobic coatings) or even particles or colloids (see microgels in plant health applications) are emerging research fields (Fig. 23). What is lacking are modular toolbox systems, in which MBPs can be combined with functional entities (biological, chemical or physical) to bifunctional units in a modular way. Processes for scaled production for bifunctional peptides with high titers up to the two-digit gram scale have to be improved individually and unexpected effects, in respect to aggregate formation or loss of functionality, need to be addressed. Nevertheless, we believe that bifunctional peptides have a huge application potential since the coating of surfaces can be achieved from aqueous solutions at ambient temperature in a sustainable an energy-efficient manner.
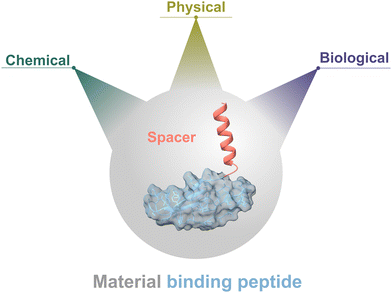 |
| Fig. 23 Universal functionalisation toolbox based on bifunctional MBPs that are fused and spatially separated through a helix to prevent intramolecular interactions that might affect their functionality. In a modular system every MBP can be equipped with functionality that could be a chemical one (e.g. a chemical catalyst or fluorophore through click-chemistry), a physical one, which alters physical properties (superhydrophobicity, water repellent), or a biological function (e.g. fluorescent eGFP reporters, enzyme or anti-microbial peptides) Protein model was generated with YASARA 20.10.4 and visualized and coloured with ChimeraX 1.7.46 | |
(g) Lack of validated biocompatible and scalable manufacturing technologies to functionalize materials on surfaces or to integrate bifunctionalities within materials
One gram of MBP is sufficient to coat >250 m2 at ambient temperature and from an aqueous solution.319 Expenses in MBP production costs will, therefore, likely not limit (bulk) industrial applications of engineered MBPs and already small amounts of MBPs in kg scale will be sufficient for bulk production processes. However, due to their limited thermal resistance of MBP as a peptide in nature, standard polymer processing technology such as injection moulding, that often require temperatures between 200 °C and 300 °C, will not be tolerated by most enzymes and MBPs. Therefore, alternative biocompatible and scale manufacturing processes must be developed to coat material surfaces and to integrate biological functionalities in materials. Promising scalable and biocompatible production technologies for MBP coatings on surfaces are spray- and dip coating processes.319 Both proved in the lab scale to be very promising since one additional washing step after spray- or dip-coating resulted usually in a dense and robust MBP-coated surfaces.338 A main limitation is, however, that a 10 min to 30 min incubation time is often required in dip coating processes to achieve high coating densities.319 The fastest reported coating with an eMBP was within 20 s on a carbon nanotube-based electrode, however, this result is highly dependent on the coated material.661 Solvent or electrospinning processes have potential to be used for integrating biofunctionalities for instance for programmed degradation or release of protein based therapeutics.662 Additive manufacturing processes can be operated at peptide compatible temperatures663 and could, as sandwich processes for multi-layered products (e.g. in cardboard production processes), be scaled and represent therefore a promising manufacturing technologies of the future for MBPs.
(h) Often lack of sufficient production titers of MBPs (>10 g L−1)
The bioethanol process demonstrates that by biotechnology means, with an engineered cocktail of cellulases, and metabolically engineered microorganisms, a bulk product such as bioethanol can be produced with costs less than $10 per kg664 without recycling of enzyme catalysts. Bacillus strains and filamentous fungi, like Trichoderma reesei, Aspergillus niger, Chrysosporium lucknowense and Aspergillus oryzae enable production titres of up to 100 g L−1 and are widely used industrially for production of enzymes like cellulase, phytase and lipase.665Escherichia coli was proven to facilitate the production of proteins at titres reaching 9.7 g L−1, as demonstrated with protein fusion construct of human growth factor.666 Yeast host, like Pichia, Yarrowia and Saccharomyces, reach production titres of 14.8 g L−1 as shown for gelatine.667 MBPs are with size range of 20 to 100 amino acids often 3 to 50 times smaller than industrial enzymes. Therefore, on a molar level, production titres of ∼10 g L−1 should already be sufficient to enable bulk applications in surface coatings since one gram is sufficient to coat >600 m2.319 Such titres are already achieved for nMBP such as cellulase binding domains in contrast to titres for eMBPs which are often below 1 g L−1;650e.g. a production titre of 0.8 g L−1 was obtained for the antimicrobial peptide plectasin in Pichia pastoris at a production scale of 5 L.668
III. Specific challenges in applications for MBP-use in (i) biocatalysis, (j) medicine, (k) plant health, and (l) nano-/microplastic.
(i) Biocatalysis
Immobilization attaches in catalysis a catalyst to an inert carrier surface, allowing for more stable, efficient, and cost-effective use of biological and chemical catalysts in industry. Catalyst can be reused and reactions can be stopped at any time through simple catalyst removal.669 eMBPs offer several advantages in enzyme immobilization over standard processes of which, an oriented and typically productive immobilization of enzymes and chemical catalysts, is an important feature.338,610 In addition, immobilizations can be performed from aqueous solutions at ambient temperature in an energy-efficient manner. Following a straightforward washing step, coating densities usually exceed 60%.319,347,377,661 eMBP-based immobilizations can be easily scaled up and used to functionalize various materials like PP, PE, Teflon, metals/metal alloys, ceramics, foams, and natural surfaces such as hair, teeth, or plant leaves that are often challenging to achieve by chemical means. The 3D-structure of eMBPs ensures that amino acid side chains can bind strongly in a defined manner to all kind of surfaces, comparable to antibodies. Methodologies such as SPR and QCM-D are routinely used to determine binding kinetics of adhesion promoting peptides and surface coating densities.338,344,377,526,661,670,671 One point to consider as a manageable challenge is that the spacer that spatially separates eMBP's binding domain and the enzyme or metal catalyst has to ensure that the two do not interact intramolecularly, which might cause a loss of catalytic and/or binding function. Moreover, in case of depolymerase-MBP fusions (Fig. 24), known for efficient depolymerisation of synthetic or natural polymers due to the presence of polymer-binding domain, that ensures proximity of catalytic domain to its solid substrate, it is crucial that the spacer allows interaction of the catalytic domain with the polymer surface. Therefore, engineering of entire fusion construct including optimization of spacer length and flexibility is important. An additional challenge arises from the presence of additives, as the majority of plastic materials originating from consumer products with a broad range of incorporated additives that may impact binding.
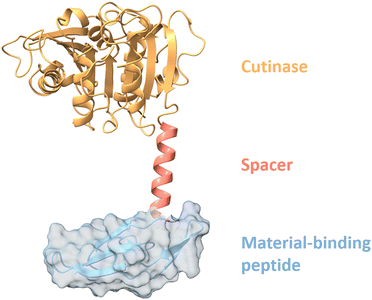 |
| Fig. 24 Exemplary construct of material-binding peptide fused to a cutinase (TfCut; 7XTR).659,660 The cutinase is fused N-terminally via a 17X linker to the material-binding peptide LCI (2B9K). LCI binds to polymers like PS and PP as well as PET as examples.338 Cutinases like TfCut or cutinase-like PET-hydrolases can be for example utilized for the enzymatic degradation of poly(butylene adipate-co-terephthalate) (PBAT) or PET, respectively. Using binding peptides in combination with polymer degrading enzymes can accelerate the polymer degradation because of the closer proximity of the biocatalyst to the polymer.615 The shown protein model was generated with YASARA 20.10.4 and visualized and coloured with ChimeraX 1.7.46 | |
(j) Medicine
The properties of MBPs reach from antimicrobial activity over support in cell adhesion to antifouling effects, which are all of high interest in different medical fields and in detailed explained in the Section “Emerging applications of material-binding peptides – Medicine.” For implants like dental implants there is an urgent need to firmly attach to the surrounding tissue and for specific antimicrobial activities after implanting to prevent inflammations by bacterial infections like from Staphylococcus aureus or different Candida spp. as summarized for AMPs.672–674 In addition, those infections need to be more and more tackled due to the increase in dental implants. In the United States (US) approximately half a million people undergo dental implantation each year with an expected grow of 150% until 2030. Using the material-binding peptides with polymer brushes on top to generate a “Kill&Repel”621 effect was published and further elaborated in Ssection “Emerging applications of material-binding peptides – medicine”. Biocompatible surface that allow differentiating human cells on PDMS or Parylene C with simple and cell-compatible removal procedures are of high interest for tissue engineering.675 Despite emerging medical applications and first proof of concept publications (technology readiness level; TRL = 3–4), there is no material-binding peptide reported in use as medical product. The advanced therapy medicinal products (ATMPs) in a european framework including the committee for advanced therapies (CAT) at the european medicines agency (EMA) has to be updated to facilitate future therapy development.676 Therapies based on tissue engineering, cell therapy and gene therapy are covered by the CAT. For the EU for example all guidelines are published by the European commission for health under the name “EudraLex”. Although the first guidelines and a regulatory framework have been generated, the approval process remains challenging and time-consuming, especially for cutting-edge innovations like material-binding peptides (not directly defined in the EU regulations so far). Challenges such as a sufficient production volume at acceptable costs and a required purity have also to be stated (see general application challenges (g) and (h)).674,677 Different plant-based production strategies like AMP expression in chloroplasts, in cereals or in callus or cell culture systems are promising and summarized with regulatory challenges by Holaskova et al.674 The summarized studies show different concepts and first promising results, but a universal expression platform system for AMPs was not found yet. Further challenges for plant-based production of AMPs like adequate regulations frameworks and their differences between for example Europe and the US are explained more in detail in the named review by Holaskova et al.674
(k) Plant health
MBPs can be used in technologies that protect plants from pathogens and, therefore, enhance plant health. These technologies consist of an MBP that binds to the surface of plant leaves (on the wax layer), which serves to immobilize through a bifunctional peptide either a microgel container that can slowly release pesticides (greenRelease) or another peptide/enzyme that possess (e.g. an antimicrobial activity).626,629 MBPs adhere in a strong and rainfast manner helping to reduce the number of required spray applications and the use of pesticides in general which aligns with the sustainable pesticide use directive (SUD) from the european commission (see “Emerging applications of material-binding peptides.”). A relevant challenge is the time-consuming field trials, in which the success and representativity of results depend on several factors, such as complex planning, implementation of statistical experimental designs, data collection and even factors that are beyond control, as weather events. Another challenge is that different design of peptides and/or containers, needed to control diverse pests, needs different regulatory applications and, therefore, different time-consuming field trials.
(l) Nano-/microplastic
In 2020, an analytical gap to monitor plastic particles smaller than 1 μm in high-throughput was identified.678 Standard analytics such as Raman, IR and GC/MS do not have a sufficient throughput due to extended sample preparation and measurement times. Additionally, these methods are not sufficiently sensitive or cannot distinguish between plastic particles and natural inorganic particles.679–682 Toxic effects of nanoplastic particles in animal experiments are reported in respect to brain inflammations, but are not well substantiated;683–686 accumulation of microplastic particles in freshwater mussel tissues are reported without detecting acute toxic effects.687 Nevertheless, high-throughput analytics to routinely monitor NP/MPs concentrations in wastewater treatment plants, drinking and ground water, as well as to monitor the accumulation in organisms that act as “accumulation biomarkers” (e.g. freshwater mussel tissues), are important to ensure human and environmental health. Notably, the shift to a circular (bio-)polymer economy might lead to a rapid increase in NP/MPs concentrations in the environment.
With fluorescent labelled eMBP (termed Plastibodies), a proof of principle to detect and sort nano- and microplastic particles in high-throughput was achieved by fluorescence-activated cell sorting (FACS) methodologies down to 500 nm.584 Field-flow fractionation is also a promising alternative to sort and analyse NP/MPs (see Section “Advanced in nano- and microplastic analytics”). It is likely, that the methodology of plastibodies can be transferred to standard and cost-effective microtiter plate readers. A main protein engineering challenge remains the design of plastibodies that bind material-specifically to NP/MPs and can distinguish between polymer particles and inorganic particles. In respect to application challenges, they grow from the application to determine the retention ability of filters (e.g. ceramic or steel filters), to isolate and quantify NP/MPs from organisms that act as NP-/MP-biomarkers, and to determine NP/MPs concentration in wastewater, ground and drinking water. In case of filters, a set of standardized NP/MPs, that are labelled with Plastibodies, can be used to determine, in combination with pyrolysis-GC/MS (polymer content) and FACS (size distribution), the ability of filters to remove NP-/MP-particles in aqueous suspension. In case of the NP-/MP-biomarkers as an additional challenge, labour-intensive preparatory steps to isolate the NP/MPs from biomarker organisms and to remove the organic matters are required prior to quantification. Determination of diluted NP/MPs in wastewater, ground and drinking water or from lakes/seas requires a concentration step of at least 1000 L. Currently, there is no technology/equipment that allows to solve this concentration challenge in a time-efficient manner to enable routine measurements.
An additional challenge that hampers research on NP-/MP-analytics that nanoplastics or microplastics standards are not available for most of the abundant synthetic polymers. Nevertheless, there are reports on simple and robust production protocols of particles for PET,688,689 PE, PP, PS, PVC690 and PLA.691,692 It is thereby important to keep in mind that laboratory produced nano- and microplastics are usually free of additives, and might differ in crystalline/amorphous content, as well as aging processes (e.g. caused by UV light in the environment).
In essence, various promising applications of MBPs in catalysis, medicine, plant health, and NP-/MP-monitoring applications have emerged due to MBPs properties and their simple, energy-efficient and scalable coating processes. In many applications in the four discussed application fields material-specific binding is a prerequisite for sustainable innovations. The efficient design of material-specific and powerful MBPs, by capitalizing on the huge protein sequence, is the holy grail for MBP-design and innovations. The latter can finally only be achieved through advanced AI and/or machine learning systems that achieve a ‘pure’ in silico design of MBPs with applications-tailored properties through supremacy over the protein sequence space. Protein engineering strategies based on SSM libraries that are combined with well-parameterized material surfaces are a straightforward roadmap to generate the experimental data and biophysical as well as molecular understanding that is required to enable in silico design of MBPs with predefined binding properties.
Author contributions
M. M., L. A., J. L., F. C., T. K., M. B., M. S. P. P., G. S., D. M., A. V., C. D., M. A. S. – writing – original draft, writing – review & editing, visualization; D. G., U. S. – conceptualization, writing – original draft, writing – review & editing, funding acquisition.
Conflicts of interest
There are no conflicts to declare.
Acknowledgements
We thank German Federal Ministry of Education and Research (BMBF) for funding of projects: Robuste und selektive lipolytische Biokatalysatoren für industrielle Anwendungen (LipoBioCat FKZ 031B0837C and LipoBioCat II FKZ 031B1342C) and Modellregion, BioRevierPLUS: Bioökonomie-REVIER Innovationscluster Biotechnologie & Kunststofftechnik (PlastiQuant and ProtLab, FKZ 031B1134C). We thank to the Centre for Advanced Studies (CAS) of the Joint Research Centre (JRC) of the European Commission for funding NANOPLASTICS project (PRJ 32529). Some of the figures were created using https://Biorender.com, https://www.Flaticon.com, RCSB.org Inkscape 1.1, YASARA 20.10.4, Microsoft PowerPoint and ChemDraw 22.0. We thank Postnova Analytics GmbH for providing the pictures of the AF4 and CF3 devices. Protein molecular graphics were performed with UCSF ChimeraX 1.4/1.7, developed by the Resource for Biocomputing, Visualization, and Informatics at the University of California, San Francisco, with support from National Institutes of Health R01-GM129325 and the Office of Cyber Infrastructure and Computational Biology, National Institute of Allergy and Infectious Diseases. Mineral structures were illustrated using Vesta 4.6.0.
Notes and references
- K. Rübsam, L. Weber, F. Jakob and U. Schwaneberg, Biotechnol. Bioeng., 2018, 115, 321–330 CrossRef PubMed.
- L. Apitius, K. Rübsam, C. Jakesch, F. Jakob and U. Schwaneberg, Biotechnol. Bioeng., 2019, 116, 1856–1867 CrossRef CAS PubMed.
- B. He, G. Chai, Y. Duan, Z. Yan, L. Qiu, H. Zhang, Z. Liu, Q. He, K. Han, B. Ru, F. B. Guo, H. Ding, H. Lin, X. Wang, N. Rao, P. Zhou and J. Huang, Nucleic Acids Res., 2016, 44, D1127–1132 CrossRef CAS PubMed.
- L. Han, J. Wang, X. Mu, T. Wu, C. Liao, N. Wu, W. Xing, L. Song, Y. Kan and Y. Hu, J. Colloid Interface Sci., 2021, 585, 596–604 CrossRef CAS PubMed.
- M. Eder, S. Amini and P. Fratzl, Science, 2018, 362, 543–547 CrossRef CAS PubMed.
- R. A. Latour, Colloids Surf., B, 2020, 191, 110992 CrossRef CAS PubMed.
- V. Vatanpour, B. Yavuzturk Gul, B. Zeytuncu, S. Korkut, G. İlyasoğlu, T. Turken, M. Badawi, I. Koyuncu and M. R. Saeb, Carbohydr. Polym., 2022, 281, 119041 CrossRef CAS PubMed.
- L. Su, Y. Feng, K. Wei, X. Xu, R. Liu and G. Chen, Chem. Rev., 2021, 121, 10950–11029 CrossRef CAS PubMed.
- C. Bertocchi, L. Navarini, A. Cesàro and M. Anastasio, Carbohydr. Polym., 1990, 12, 127–153 CrossRef CAS.
-
J. N. BeMiller, in Glycoscience: Chemistry and Chemical Biology, ed. B. O. Fraser-Reid, K. Tatsuta and J. Thiem, Springer Berlin Heidelberg, Berlin, Heidelberg, 2008, pp. 1413–1435 DOI:10.1007/978-3-540-30429-6_34.
- D. A. Rees and E. J. Welsh, Angew. Chem., Int. Ed. Engl., 1977, 16, 214–224 CrossRef.
- B. Richard, R. Swanson and S. T. Olson, J. Biol. Chem., 2009, 284, 27054–27064 CrossRef CAS PubMed.
- H. Tremblay, T. Yamaguchi, T. Fukamizo and R. Brzezinski, J. Biochem., 2001, 130, 679–686 CrossRef CAS PubMed.
- A.-H. Teh, P.-F. Sim and T. Hisano, Biochem. Biophys. Res. Commun., 2020, 533, 257–261 CrossRef CAS PubMed.
-
S. F. Kabir, A. Rahman, F. Yeasmin, S. Sultana, R. A. Masud, N. A. Kanak and P. Haque, in Radiation-Processed Polysaccharides, ed. M. Naeem, T. Aftab and M. M. A. Khan, Academic Press, 2022, pp. 1–27 Search PubMed.
- Z. Liu, Y. Jiao, Y. Wang, C. Zhou and Z. Zhang, Adv. Drug Delivery Rev., 2008, 60, 1650–1662 CrossRef CAS PubMed.
- S. Mizrahy and D. Peer, Chem. Soc. Rev., 2012, 41, 2623–2640 RSC.
- H. J. Flint, K. P. Scott, S. H. Duncan, P. Louis and E. Forano, Gut Microbes, 2012, 3, 289–306 CrossRef PubMed.
- C. Sun, S. Wei, H. Tan, Y. Huang and Y. Zhang, Chem. Eng. J., 2022, 446, 136881 CrossRef CAS.
- S. Maity and N. Mallick, J. Cleaner Prod., 2022, 345, 131153 CrossRef CAS.
- H. J. Gilbert, J. P. Knox and A. B. Boraston, Curr. Opin. Struct. Biol., 2013, 23, 669–677 CrossRef CAS PubMed.
- G. Liu, Y. Chang, X. Mei, G. Chen, Y. Zhang, X. Jiang, W. Tao and C. Xue, Int. J. Biol. Macromol., 2024, 255, 127959 CrossRef CAS PubMed.
- B. L. Cantarel, P. M. Coutinho, C. Rancurel, T. Bernard, V. Lombard and B. Henrissat, Nucleic Acids Res., 2008, 37, D233–D238 CrossRef PubMed.
- G. Vaaje-Kolstad, B. Westereng, S. J. Horn, Z. Liu, H. Zhai, M. Sørlie and V. G. H. Eijsink, Science, 2010, 330, 219–222 CrossRef CAS PubMed.
- F. Cuskin, J. E. Flint, T. M. Gloster, C. Morland, A. Baslé, B. Henrissat, P. M. Coutinho, A. Strazzulli, A. S. Solovyova, G. J. Davies and H. J. Gilbert, Proc. Natl. Acad. Sci. U. S. A., 2012, 109, 20889–20894 CrossRef CAS PubMed.
- J.-J. Deng, M.-S. Zhang, Z.-W. Li, D.-L. Lu, H.-H. Mao, M.-J. Zhu, J.-Z. Li and X.-C. Luo, Green Chem., 2020, 22, 6862–6873 RSC.
- H. Hashimoto, Cell. Mol. Life Sci., 2006, 63, 2954–2967 CrossRef CAS PubMed.
- B. Thomas, M. C. Raj, J. Joy, A. Moores, G. L. Drisko and C. Sanchez, Chem. Rev., 2018, 118, 11575–11625 CrossRef CAS PubMed.
- A. Etale, A. J. Onyianta, S. R. Turner and S. J. Eichhorn, Chem. Rev., 2023, 123, 2016–2048 CrossRef CAS PubMed.
- A. D. French, Cellulose, 2017, 24, 4605–4609 CrossRef CAS.
- Y. Habibi, Chem. Soc. Rev., 2014, 43, 1519–1542 RSC.
- S. Acharya, S. Liyanage, P. Parajuli, S. S. Rumi, J. L. Shamshina and N. Abidi, Polymers, 2021, 13, 4344 CrossRef CAS PubMed.
- C. M. Payne, B. C. Knott, H. B. Mayes, H. Hansson, M. E. Himmel, M. Sandgren, J. Ståhlberg and G. T. Beckham, Chem. Rev., 2015, 115, 1308–1448 CrossRef CAS PubMed.
- Y. Yu and H. Wu, Ind. Eng. Chem. Res., 2010, 49, 3902–3909 CrossRef CAS.
- T. Paakkari, R. Serimaa and H.-P. Fink, Acta Polym., 1989, 40, 731–734 CrossRef CAS.
- T. Kondo and C. Sawatari, Polymer, 1996, 37, 393–399 CrossRef CAS.
- Y. Nishiyama, P. Langan and H. Chanzy, J. Am. Chem. Soc., 2002, 124, 9074–9082 CrossRef CAS PubMed.
- R. H. Newman and J. A. Hemmingson, Cellulose, 1995, 2, 95–110 CrossRef CAS.
- B. Nidetzky and C. Zhong, Biotechnol. Adv., 2021, 51, 107633 CrossRef CAS PubMed.
- A. Etale, A. J. Onyianta, S. R. Turner and S. J. Eichhorn, Chem. Rev., 2023, 123, 2016–2048 CrossRef CAS PubMed.
- A. B. Boraston, D. N. Bolam, H. J. Gilbert and G. J. Davies, Biochem. J., 2004, 382, 769–781 CrossRef CAS PubMed.
- L. M. Salonen, M. Ellermann and F. Diederich, Angew. Chem., Int. Ed., 2011, 50, 4808–4842 CrossRef CAS PubMed.
- P. J. Kraulis, G. M. Clore, M. Nilges, T. A. Jones, G. Pettersson, J. Knowles and A. M. Gronenborn, Biochemistry, 1989, 28, 7241–7257 CrossRef CAS PubMed.
- M. Czjzek, D. N. Bolam, A. Mosbah, J. Allouch, C. M. G. A. Fontes, L. M. A. Ferreira, O. Bornet, V. Zamboni, H. Darbon, N. L. Smith, G. W. Black, B. Henrissat and H. J. Gilbert, J. Biol. Chem., 2001, 276, 48580–48587 CrossRef CAS PubMed.
- E. Ficko-Blean and A. B. Boraston, J. Biol. Chem., 2006, 281, 37748–37757 CrossRef CAS PubMed.
- E. C. Meng, T. D. Goddard, E. F. Pettersen, G. S. Couch, Z. J. Pearson, J. H. Morris and T. E. Ferrin, Protein Sci., 2023, 32, e4792 CrossRef CAS PubMed.
- M.-L. Mattinen, M. Linder, A. Teleman and A. Annila, FEBS Lett., 1997, 407, 291–296 CrossRef CAS PubMed.
- P. Tomme, A. L. Creagh, D. G. Kilburn and C. A. Haynes, Biochemistry, 1996, 35, 13885–13894 CrossRef CAS PubMed.
- A. L. Creagh, E. Ong, E. Jervis, D. G. Kilburn and C. A. Haynes, Proc. Natl. Acad. Sci. U. S. A., 1996, 93, 12229–12234 CrossRef CAS PubMed.
- N. Georgelis, N. H. Yennawar and D. J. Cosgrove, Proc. Natl. Acad. Sci. U. S. A., 2012, 109, 14830–14835 CrossRef CAS PubMed.
- M. Linder, M.-L. Mattinen, M. Kontteli, G. Lindeberg, J. Ståhlberg, T. Drakenberg, T. Reinikainen, G. Pettersson and A. Annila, Protein Sci., 1995, 4, 1056–1064 CrossRef CAS PubMed.
- N. Georgelis, A. Tabuchi, N. Nikolaidis and D. J. Cosgrove, J. Biol. Chem., 2011, 286, 16814–16823 CrossRef CAS PubMed.
- F. Kerff, A. Amoroso, R. Herman, E. Sauvage, S. Petrella, P. Filée, P. Charlier, B. Joris, A. Tabuchi, N. Nikolaidis and D. J. Cosgrove, Proc. Natl. Acad. Sci. U. S. A., 2008, 105, 16876–16881 CrossRef CAS PubMed.
- M. Linder, M. L. Mattinen, M. Kontteli, G. Lindeberg, J. Stahlberg, T. Drakenberg, T. Reinikainen, G. Pettersson and A. Annila, Protein Sci., 1995, 4, 1056–1064 CrossRef CAS PubMed.
- M. Alahuhta, Q. Xu, Y. J. Bomble, R. Brunecky, W. S. Adney, S.-Y. Ding, M. E. Himmel and V. V. Lunin, J. Mol. Biol., 2010, 402, 374–387 CrossRef CAS PubMed.
- V. Notenboom, A. B. Boraston, P. Chiu, A. C. J. Freelove, D. G. Kilburn and D. R. Rose, J. Mol. Biol., 2001, 314, 797–806 CrossRef CAS PubMed.
- V. Notenboom, A. B. Boraston, D. G. Kilburn and D. R. Rose, Biochemistry, 2001, 40, 6248–6256 CrossRef CAS PubMed.
- J. Jumper, R. Evans, A. Pritzel, T. Green, M. Figurnov, O. Ronneberger, K. Tunyasuvunakool, R. Bates, A. Žídek, A. Potapenko, A. Bridgland, C. Meyer, S. A. A. Kohl, A. J. Ballard, A. Cowie, B. Romera-Paredes, S. Nikolov, R. Jain, J. Adler, T. Back, S. Petersen, D. Reiman, E. Clancy, M. Zielinski, M. Steinegger, M. Pacholska, T. Berghammer, S. Bodenstein, D. Silver, O. Vinyals, A. W. Senior, K. Kavukcuoglu, P. Kohli and D. Hassabis, Nature, 2021, 596, 583–589 CrossRef CAS PubMed.
- A. A. Kognole and C. M. Payne, Biotechnol. Biofuels, 2018, 11, 319 CrossRef CAS PubMed.
- D. Krska, S. Mazurkewich, H. A. Brown, Y. Theibich, J.-C. N. Poulsen, A. L. Morris, N. M. Koropatkin, L. Lo Leggio and J. Larsbrink, Biochemistry, 2021, 60, 2206–2220 CrossRef CAS PubMed.
- N. Yan and X. Chen, Nature, 2015, 524, 155–157 CrossRef CAS PubMed.
- S. J. Horn, P. Sikorski, J. B. Cederkvist, G. Vaaje-Kolstad, M. Sørlie, B. Synstad, G. Vriend, K. M. Vårum and V. G. H. Eijsink, Proc. Natl. Acad. Sci. U. S. A., 2006, 103, 18089–18094 CrossRef CAS PubMed.
-
P. I. P. Soares, C. Echeverria, A. C. Baptista, C. F. C. João, S. N. Fernandes, A. P. C. Almeida, J. C. Silva, M. H. Godinho and J. P. Borges, in Hybrid Polymer Composite Materials, ed. V. K. Thakur, M. K. Thakur and A. Pappu, Woodhead Publishing, 2017, pp. 107–149 Search PubMed.
- L. Su, Y. Feng, K. Wei, X. Xu, R. Liu and G. Chen, Chem. Rev., 2021, 121, 10950–11029 CrossRef CAS PubMed.
- M. Rinaudo, Prog. Polym. Sci., 2006, 31, 603–632 CrossRef CAS.
- H. V. D. Nguyen, R. de Vries and S. D. Stoyanov, Green Chem., 2022, 24, 3834–3844 RSC.
- M. B. Kaczmarek, K. Struszczyk-Swita, X. Li, M. Szczęsna-Antczak and M. Daroch, Front. Bioeng. Biotechnol., 2019, 7, 243 CrossRef PubMed.
- A. Wei, J. Fu and F. Guo, J. Mater. Sci., 2021, 56, 12048–12058 CrossRef CAS.
- M. Kaya, E. Lelešius, R. Nagrockaitė, I. Sargin, G. Arslan, A. Mol, T. Baran, E. Can and B. Bitim, PLoS One, 2015, 10, e0115531 CrossRef PubMed.
- T. Usui, H. Matsui and K. Isobe, Carbohydr. Res., 1990, 203, 65–77 CrossRef CAS PubMed.
- K. Li, R. Xing, S. Liu and P. Li, Carbohydr. Polym., 2016, 139, 178–190 CrossRef CAS PubMed.
- R. Jayakumar, D. Menon, K. Manzoor, S. V. Nair and H. Tamura, Carbohydr. Polym., 2010, 82, 227–232 CrossRef CAS.
- I. Hamed, F. Özogul and J. M. Regenstein, Trends Food Sci. Technol., 2016, 48, 40–50 CrossRef CAS.
- M. A. Goldstein, M. Takagi, S. Hashida, O. Shoseyov, R. H. Doi and I. H. Segel, J. Bacteriol., 1993, 175, 5762–5768 CrossRef CAS PubMed.
- K. Morimoto, S. Karita, T. Kimura, K. Sakka and K. Ohmiya, J. Bacteriol., 1997, 179, 7306–7314 CrossRef CAS PubMed.
- T. Nakamura, S. Mine, Y. Hagihara, K. Ishikawa, T. Ikegami and K. Uegaki, J. Mol. Biol., 2008, 381, 670–680 CrossRef CAS PubMed.
- J. Schnellmann, A. Zeltins, H. Blaak and H. Schrempf, Mol. Microbiol., 1994, 13, 807–819 CrossRef CAS PubMed.
- A. Zeltins and H. Schrempp, Eur. J. Biochem., 1997, 246, 557–564 CrossRef CAS PubMed.
- D. I. Svergun, A. Bećirević, H. Schrempf, M. H. J. Koch and G. Grüber, Biochemistry, 2000, 39, 10677–10683 CrossRef CAS PubMed.
- T. Ohnuma, S. Onaga, K. Murata, T. Taira and E. Katoh, J. Biol. Chem., 2008, 283, 5178–5187 CrossRef CAS PubMed.
- E. Madland, O. Crasson, M. Vandevenne, M. Sørlie and F. L. Aachmann, ACS Omega, 2019, 4, 21975–21984 CrossRef CAS PubMed.
- S. Mesnage, M. Dellarole, N. J. Baxter, J.-B. Rouget, J. D. Dimitrov, N. Wang, Y. Fujimoto, A. M. Hounslow, S. Lacroix-Desmazes, K. Fukase, S. J. Foster and M. P. Williamson, Nat. Commun., 2014, 5, 4269 CrossRef CAS PubMed.
- T. Liu, Z. Liu, C. Song, Y. Hu, Z. Han, J. She, F. Fan, J. Wang, C. Jin, J. Chang, J.-M. Zhou and J. Chai, Science, 2012, 336, 1160–1164 CrossRef CAS PubMed.
- A. C. Kohler, L.-H. Chen, N. Hurlburt, A. Salvucci, B. Schwessinger, A. J. Fisher and I. Stergiopoulos, Plant Cell, 2016, 28, 1945–1965 CrossRef CAS PubMed.
- P. L. Keeling and A. M. Myers, Annu. Rev. Food Sci. Technol., 2010, 1, 271–303 CrossRef CAS PubMed.
- S. C. Zeeman, J. Kossmann and A. M. Smith, Annu. Rev. Plant Biol., 2010, 61, 209–234 CrossRef CAS PubMed.
- J. van Rooyen, S. Simsek, S. A. Oyeyinka and M. Manley, Compr. Rev. Food Sci. Food Saf., 2023, 22, 2292–2309 CrossRef CAS PubMed.
- P. L. Keeling and A. M. Myers, Annu. Rev. Food Sci. Technol., 2010, 1, 271–303 CrossRef CAS PubMed.
- E. Bertoft, K. Piyachomkwan, P. Chatakanonda and K. Sriroth, Carbohydr. Polym., 2008, 74, 527–543 CrossRef CAS.
- S. Singh, N. Singh, N. Isono and T. Noda, J. Agric. Food Chem., 2010, 58, 1180–1188 CrossRef CAS PubMed.
- M. Wang, M. Sun, Y. Zhang, Y. Chen, Y. Wu and J. Ouyang, Food Chem., 2019, 298, 125015 CrossRef CAS PubMed.
- K. Zhang, D. Zhao, Q. Huang, J. Huang and Q. Wen, Starch/Staerke, 2021, 73, 2100065 CrossRef CAS.
- A. Sarko and H.-C. H. Wu, Starch/Staerke, 1978, 30, 73–78 CrossRef CAS.
- A. Imberty, A. Buléon, V. Tran and S. Péerez, Starch/Staerke, 1991, 43, 375–384 CrossRef CAS.
- W. Zhang, S. Shen, T. Song, X. Chen, A. Zhang and H. Dou, Food Chem., 2021, 349, 129168 CrossRef CAS PubMed.
- Z. Guo, X. Jia, B. Zhao, S. Zeng, J. Xiao and B. Zheng, Ann. N. Y. Acad. Sci., 2017, 1398, 47–61 CrossRef CAS PubMed.
- C. Loret, V. Meunier, W. J. Frith and P. J. Fryer, Carbohydr. Polym., 2004, 57, 153–163 CrossRef CAS.
- R. Zagrodnik and M. Łaniecki, Bioresour. Technol., 2017, 224, 298–306 CrossRef CAS PubMed.
- R. A. Cripwell, L. Favaro, M. Viljoen-Bloom and W. H. van Zyl, Biotechnol. Adv., 2020, 42, 107579 CrossRef CAS PubMed.
- N. L. Tai, M. Ghasemlou, R. Adhikari and B. Adhikari, Carbohydr. Polym., 2021, 265, 118029 CrossRef CAS PubMed.
- Š. Janeček, F. Mareček, E. A. MacGregor and B. Svensson, Biotechnol. Adv., 2019, 37, 107451 CrossRef PubMed.
- D. W. Cockburn, C. Suh, K. P. Medina, R. M. Duvall, Z. Wawrzak, B. Henrissat and N. M. Koropatkin, Mol. Microbiol., 2018, 107, 249–264 CrossRef CAS PubMed.
- M. P. Williamson, M.-F. Le Gal-Coëffet, K. Sorimachi, C. S. M. Furniss, D. B. Archer and G. Williamson, Biochemistry, 1997, 36, 7535–7539 CrossRef CAS PubMed.
- K. Sorimachi, M.-F. L. Gal-Coëffet, G. Williamson, D. B. Archer and M. P. Williamson, Structure, 1997, 5, 647–661 CrossRef CAS PubMed.
- K. Sorimachi, A. J. Jacks, M.-F. Le Gal-Coëffet, G. Williamson, D. B. Archer and M. P. Williamson, J. Mol. Biol., 1996, 259, 970–987 CrossRef CAS PubMed.
- T. Giardina, A. P. Gunning, N. Juge, C. B. Faulds, C. S. M. Furniss, B. Svensson, V. J. Morris and G. Williamson, J. Mol. Biol., 2001, 313, 1149–1159 CrossRef CAS PubMed.
- C. Christiansen, M. Abou Hachem, Š. Janeček, A. Viksø-Nielsen, A. Blennow and B. Svensson, FEBS J., 2009, 276, 5006–5029 CrossRef CAS PubMed.
- J. Y. Tung, M. D. Chang, W. I. Chou, Y. Y. Liu, Y. H. Yeh, F. Y. Chang, S. C. Lin, Z. L. Qiu and Y. J. Sun, Biochem. J., 2008, 416, 27–36 CrossRef CAS PubMed.
- B. R. Urbanowicz, M. J. Peña, H. A. Moniz, K. W. Moremen and W. S. York, Plant J., 2014, 80, 197–206 CrossRef CAS PubMed.
- H. V. Scheller and P. Ulvskov, Annu. Rev. Plant Biol., 2010, 61, 263–289 CrossRef CAS PubMed.
- R. D. Hatfield, D. M. Rancour and J. M. Marita, Front. Plant Sci., 2017, 7, 204954 Search PubMed.
- A. Faik, Plant Physiol., 2010, 153, 396–402 CrossRef CAS PubMed.
- E. A. Rennie and H. V. Scheller, Curr. Opin. Biotechnol, 2014, 26, 100–107 CrossRef CAS PubMed.
- J. Vogel, Curr. Opin. Plant Biol., 2008, 11, 301–307 CrossRef CAS PubMed.
- N. J. Grantham, J. Wurman-Rodrich, O. M. Terrett, J. J. Lyczakowski, K. Stott, D. Iuga, T. J. Simmons, M. Durand-Tardif, S. P. Brown, R. Dupree, M. Busse-Wicher and P. Dupree, Nat. Plants, 2017, 3, 859–865 CrossRef CAS PubMed.
- T. J. Simmons, J. C. Mortimer, O. D. Bernardinelli, A.-C. Pöppler, S. P. Brown, E. R. deAzevedo, R. Dupree and P. Dupree, Nat. Commun., 2016, 7, 13902 CrossRef CAS PubMed.
- S. Makishima, M. Mizuno, N. Sato, K. Shinji, M. Suzuki, K. Nozaki, F. Takahashi, T. Kanda and Y. Amano, Bioresour. Technol., 2009, 100, 2842–2848 CrossRef CAS PubMed.
- X. Li, X. Shi, M. Wang and Y. Du, Food Chem., 2011, 126, 520–525 CrossRef CAS.
- D. P. Kamdem, Z. Shen, O. Nabinejad and Z. Shu, Food Packag. Shelf Life, 2019, 21, 100344 CrossRef.
- C. Gao, J. Ren, C. Zhao, W. Kong, Q. Dai, Q. Chen, C. Liu and R. Sun, Carbohydr. Polym., 2016, 151, 189–197 CrossRef CAS PubMed.
- H. Xie, D. N. Bolam, T. Nagy, L. Szabó, A. Cooper, P. J. Simpson, J. H. Lakey, M. P. Williamson and H. J. Gilbert, Biochemistry, 2001, 40, 5700–5707 CrossRef CAS PubMed.
- P. J. Simpson, D. N. Bolam, A. Cooper, A. Ciruela, G. P. Hazlewood, H. J. Gilbert and M. P. Williamson, Structure, 1999, 7, 853–864 CrossRef CAS PubMed.
- L. Szabó, S. Jamal, H. Xie, S. J. Charnock, D. N. Bolam, H. J. Gilbert and G. J. Davies, J. Biol. Chem., 2001, 276, 49061–49065 CrossRef PubMed.
- H. Xie, H. J. Gilbert, S. J. Charnock, G. J. Davies, M. P. Williamson, P. J. Simpson, S. Raghothama, C. M. G. A. Fontes, F. M. V. Dias, L. M. A. Ferreira and D. N. Bolam, Biochemistry, 2001, 40, 9167–9176 CrossRef CAS PubMed.
- M. Schärpf, G. P. Connelly, G. M. Lee, A. B. Boraston, R. A. J. Warren and L. P. McIntosh, Biochemistry, 2002, 41, 4255–4263 CrossRef PubMed.
- S. J. Charnock, D. N. Bolam, J. P. Turkenburg, H. J. Gilbert, L. M. A. Ferreira, G. J. Davies and C. M. G. A. Fontes, Biochemistry, 2000, 39, 5013–5021 CrossRef CAS PubMed.
- N. E. El Gueddari, U. Rauchhaus, B. M. Moerschbacher and H. B. Deising, New Phytol., 2002, 156, 103–112 CrossRef CAS.
- F. Liaqat and R. Eltem, Carbohydr. Polym., 2018, 184, 243–259 CrossRef CAS PubMed.
- B. Focher, P. L. Beltrame, A. Naggi and G. Torri, Carbohydr. Polym., 1990, 12, 405–418 CrossRef CAS.
- S. Sreekumar, J. Wattjes, A. Niehues, T. Mengoni, A. C. Mendes, E. R. Morris, F. M. Goycoolea and B. M. Moerschbacher, Nat. Commun., 2022, 13, 7125 CrossRef CAS PubMed.
- J. Wattjes, S. Sreekumar, C. Richter, S. Cord-Landwehr, R. Singh, N. E. El Gueddari and B. M. Moerschbacher, React. Funct. Polym., 2020, 151, 104583 CrossRef CAS.
- Z. Yu, L. Ma, S. Ye, G. Li and M. Zhang, Carbohydr. Polym., 2020, 236, 115972 CrossRef CAS PubMed.
- X. Du, L. Wu, H. Yan, Z. Jiang, S. Li, W. Li, Y. Bai, H. Wang, Z. Cheng, D. Kong, L. Wang and M. Zhu, Nat. Commun., 2021, 12, 4733 CrossRef CAS PubMed.
- Y. Feng, H.-L. Gao, D. Wu, Y.-T. Weng, Z.-Y. Wang, S.-H. Yu and Z. Wang, Adv. Funct. Mater., 2021, 31, 2105348 CrossRef CAS.
- S. Sessarego, S. C. G. Rodrigues, Y. Xiao, Q. Lu and J. M. Hill, Carbohydr. Polym., 2019, 211, 249–256 CrossRef CAS PubMed.
- S. Yadav, G. K. Mehrotra and P. K. Dutta, Food Chem., 2021, 334, 127605 CrossRef CAS PubMed.
- M. Mondéjar-López, A. J. López-Jimenez, J. C. García Martínez, O. Ahrazem, L. Gómez-Gómez and E. Niza, Int. J. Biol. Macromol., 2022, 206, 288–297 CrossRef PubMed.
- L. Zhang, S. Tang, C. Jiang, X. Jiang and Y. Guan, ACS Appl. Mater. Interfaces, 2018, 10, 43013–43030 CrossRef CAS PubMed.
- S. Shinya, S. Nishimura, Y. Kitaoku, T. Numata, H. Kimoto, H. Kusaoke, T. Ohnuma and T. Fukamizo, Biochem. J., 2016, 473, 1085–1095 CrossRef CAS PubMed.
- S. Shinya, T. Ohnuma, R. Yamashiro, H. Kimoto, H. Kusaoke, P. Anbazhagan, A. H. Juffer and T. Fukamizo, J. Biol. Chem., 2013, 288, 30042–30053 CrossRef CAS PubMed.
- T. Katsumi, M.-È. Lacombe-Harvey, H. Tremblay, R. Brzezinski and T. Fukamizo, Biochem. Biophys. Res. Commun., 2005, 338, 1839–1844 CrossRef CAS PubMed.
- W. Adachi, Y. Sakihama, S. Shimizu, T. Sunami, T. Fukazawa, M. Suzuki, R. Yatsunami, S. Nakamura and A. Takénaka, J. Mol. Biol., 2004, 343, 785–795 CrossRef CAS PubMed.
- S. Shinya and T. Fukamizo, Int. J. Biol. Macromol., 2017, 104, 1422–1435 CrossRef CAS PubMed.
- M. P. Silva, I. J. Badruddin, T. Tonon, S. Rahatekar and L. D. Gomez, Int. J. Biol. Macromol., 2023, 226, 434–442 CrossRef CAS PubMed.
- T. Y. Wong, L. A. Preston and N. L. Schiller, Annu. Rev. Microbiol., 2000, 54, 289–340 CrossRef CAS PubMed.
- S. N. Pawar and K. J. Edgar, Biomaterials, 2012, 33, 3279–3305 CrossRef CAS PubMed.
- C. Hu, W. Lu, C. Sun, Y. Zhao, Y. Zhang and Y. Fang, Carbohydr. Polym., 2022, 294, 119788 CrossRef CAS PubMed.
- X. Wang, W. Xu, Q. Dai, X. Liu, C. Guang, W. Zhang and W. Mu, Enzyme Microb. Technol., 2023, 166, 110221 CrossRef CAS PubMed.
- J. Liu, S. Yang, X. Li, Q. Yan, M. J. T. Reaney and Z. Jiang, Compr. Rev. Food Sci. Food Saf., 2019, 18, 1859–1881 CrossRef CAS PubMed.
- P. Rastogi and B. Kandasubramanian, Biofabrication, 2019, 11, 042001 CrossRef CAS PubMed.
- K. Varaprasad, T. Jayaramudu, V. Kanikireddy, C. Toro and E. R. Sadiku, Carbohydr. Polym., 2020, 236, 116025 CrossRef CAS PubMed.
- M. A. J. Shaikh, K. S. Alharbi, W. H. Almalki, S. S. Imam, M. Albratty, A. M. Meraya, S. I. Alzarea, I. Kazmi, F. A. Al-Abbasi, O. Afzal, A. S. A. Altamimi, Y. Singh, S. K. Singh, K. Dua and G. Gupta, Carbohydr. Polym., 2022, 292, 119689 CrossRef CAS PubMed.
- N.
M. Sanchez-Ballester, B. Bataille and I. Soulairol, Carbohydr. Polym., 2021, 270, 118399 CrossRef CAS PubMed.
- S. Ji, X. Tian, X. Li and Q. She, J. Biol. Chem., 2023, 299, 102854 CrossRef CAS PubMed.
- A. B. Boraston, E. Ficko-Blean and M. Healey, Biochemistry, 2007, 46, 11352–11360 CrossRef CAS PubMed.
- E. Ficko-Blean and A. B. Boraston, J. Mol. Biol., 2009, 390, 208–220 CrossRef CAS PubMed.
- M. F. P. Graça, S. P. Miguel, C. S. D. Cabral and I. J. Correia, Carbohydr. Polym., 2020, 241, 116364 CrossRef PubMed.
- J. A. Burdick and G. D. Prestwich, Adv. Mater., 2011, 23, H41–H56 CrossRef CAS PubMed.
- A. Fallacara, E. Baldini, S. Manfredini and S. Vertuani, Polymers, 2018, 10, 701 CrossRef PubMed.
- C. C. L. Schuurmans, M. Mihajlovic, C. Hiemstra, K. Ito, W. E. Hennink and T. Vermonden, Biomaterials, 2021, 268, 120602 CrossRef CAS PubMed.
- X. Zhang, D. Wei, Y. Xu and Q. Zhu, Carbohydr. Polym., 2021, 264, 118006 CrossRef CAS PubMed.
- S. N. A. Bukhari, N. L. Roswandi, M. Waqas, H. Habib, F. Hussain, S. Khan, M. Sohail, N. A. Ramli, H. E. Thu and Z. Hussain, Int. J. Biol. Macromol., 2018, 120, 1682–1695 CrossRef CAS PubMed.
- M. D. L. Suits, B. Pluvinage, A. Law, Y. Liu, A. S. Palma, W. Chai, T. Feizi and A. B. Boraston, J. Biol. Chem., 2014, 289, 27264–27277 CrossRef CAS PubMed.
- H. Ponta, L. Sherman and P. A. Herrlich, Nat. Rev. Mol. Cell Biol., 2003, 4, 33–45 CrossRef CAS PubMed.
- S. Misra, V. C. Hascall, R. R. Markwald and S. Ghatak, Front. Immunol., 2015, 6, 137534 Search PubMed.
- J. Bajorath, B. Greenfield, S. B. Munro, A. J. Day and A. Aruffo, J. Biol. Chem., 1998, 273, 338–343 CrossRef CAS PubMed.
- M. Takeda, S. Ogino, R. Umemoto, M. Sakakura, M. Kajiwara, K. N. Sugahara, H. Hayasaka, M. Miyasaka, H. Terasawa and I. Shimada, J. Biol. Chem., 2006, 281, 40089–40095 Search PubMed.
- M. K. Cowman and E. A. Turley, Proteome Res., 2023, 1, e4 Search PubMed.
- J. Zhao, M. Yoneda, M. Takeyama, O. Miyaishi, Y. Inoue, T. Kataoka, A. Ohno-Jinno, Z. Isogai, K. Kimata, M. Iwaki and M. Zako, J. Neurochem., 2008, 106, 1117–1124 CrossRef CAS PubMed.
- F. P. Maloney, J. Kuklewicz, R. A. Corey, Y. Bi, R. Ho, L. Mateusiak, E. Pardon, J. Steyaert, P. J. Stansfeld and J. Zimmer, Nature, 2022, 604, 195–201 CrossRef CAS PubMed.
- J. Mandawe, B. Infanzon, A. Eisele, H. Zaun, J. Kuballa, M. D. Davari, F. Jakob, L. Elling and U. Schwaneberg, ChemBioChem, 2018, 19, 1414–1423 CrossRef CAS PubMed.
- R. J. Linhardt, J. Med. Chem., 2003, 46, 2551–2564 CrossRef CAS PubMed.
- X. Yang, Q. Wang, A. Zhang, X. Shao, T. Liu, B. Tang and G. Fang, Carbohydr. Polym., 2022, 294, 119793 Search PubMed.
- D. L. Rabenstein, Nat. Prod. Rep., 2002, 19, 312–331 Search PubMed.
- P. Wang, L. Chi, Z. Zhang, H. Zhao, F. Zhang and R. J. Linhardt, Carbohydr. Polym., 2022, 295, 119818 CrossRef CAS PubMed.
- D. Xu and J. D. Esko, Annu. Rev. Biochem., 2014, 83, 129–157 CrossRef CAS PubMed.
- S. N. Bolten, U. Rinas and T. Scheper, Appl. Microbiol. Biotechnol., 2018, 102, 8647–8660 CrossRef CAS PubMed.
- W. J. Carter, E. Cama and J. A. Huntington, J. Biol. Chem., 2005, 280, 2745–2749 CrossRef CAS PubMed.
- E. M. Muñoz and R. J. Linhardt, Arterioscler., Thromb., Vasc. Biol., 2004, 24, 1549–1557 CrossRef PubMed.
- K. J. Colley, K. Kitajima and C. Sato, Crit. Rev. Biochem. Mol. Biol., 2014, 49, 498–532 CrossRef CAS PubMed.
- C. Sato and K. Kitajima, Mol. Aspects Med., 2021, 79, 100892 CrossRef CAS PubMed.
- R. L. Schnaar, R. Gerardy-Schahn and H. Hildebrandt, Physiol. Rev., 2014, 94(2), 461–518 Search PubMed.
- M. Mühlenhoff, M. Eckhardt and R. Gerardy-Schahn, Curr. Opin. Struct. Biol., 1998, 8, 558–564 CrossRef PubMed.
- X. Guo, S. M. Elkashef, P. M. Loadman, L. H. Patterson and R. A. Falconer, Carbohydr. Polym., 2019, 224, 115145 CrossRef CAS PubMed.
- J. Wu, X. Zhan, L. Liu and X. Xia, Appl. Microbiol. Biotechnol., 2018, 102, 9403–9409 CrossRef CAS PubMed.
- T. Zhang, Z. She, Z. Huang, J. Li, X. Luo and Y. Deng, Asian J. Pharm. Sci., 2014, 9, 75–81 CrossRef.
- Q. Zhang, S. Li, L. He and X. Feng, Int. J. Biol. Macromol., 2023, 230, 123151 CrossRef CAS PubMed.
- C. D. Owen, L. E. Tailford, S. Monaco, T. Šuligoj, L. Vaux, R. Lallement, Z. Khedri, H. Yu, K. Lecointe, J. Walshaw, S. Tribolo, M. Horrex, A. Bell, X. Chen, G. L. Taylor, A. Varki, J. Angulo and N. Juge, Nat. Commun., 2017, 8, 2196 CrossRef PubMed.
- A. Lenman, A. M. Liaci, Y. Liu, L. Frängsmyr, M. Frank, B. S. Blaum, W. Chai, I. I. Podgorski, B. Harrach, M. Benkő, T. Feizi, T. Stehle and N. Arnberg, Proc. Natl. Acad. Sci. U. S. A., 2018, 115, E4264–E4273 CrossRef CAS PubMed.
- P. J. Simpson, H. Xie, D. N. Bolam, H. J. Gilbert and M. P. Williamson, J. Biol. Chem., 2000, 275, 41137–41142 CrossRef CAS PubMed.
- M. Cusack and A. Freer, Chem. Rev., 2008, 108, 4433–4454 CrossRef CAS PubMed.
- S. Yao, B. Jin, Z. Liu, C. Shao, R. Zhao, X. Wang and R. Tang, Adv. Mater., 2017, 29, 1605903 CrossRef PubMed.
- E. A. Abou Neel, A. Aljabo, A. Strange, S. Ibrahim, M. Coathup, A. M. Young, L. Bozec and V. Mudera, Int. J. Nanomed., 2016, 11, 4743–4763 CrossRef PubMed.
- P. Anbu, C.-H. Kang, Y.-J. Shin and J.-S. So, SpringerPlus, 2016, 5, 250 CrossRef PubMed.
- M. A. Icer and M. Gezmen-Karadag, Clin. Biochem., 2018, 59, 17–24 CrossRef CAS PubMed.
- M. Wysokowski, T. Jesionowski and H. Ehrlich, Am. Mineral., 2018, 103, 665–691 CrossRef.
- F. C. Meldrum and H. Cölfen, Chem. Rev., 2008, 108, 4332–4432 CrossRef CAS PubMed.
- Y. Fang, S. Lee, H. Xu and G. A. Farfan, Cryst. Growth Des., 2023, 23, 4872–4882 CrossRef CAS PubMed.
-
A. Rao and H. Cölfen, in Biomineralization and Biomaterials, ed. C. Aparicio and M.-P. Ginebra, Woodhead Publishing, Boston, 2016, pp. 51–93 Search PubMed.
- J. D. Currey, J. Exp. Biol., 1999, 202, 3285–3294 CrossRef CAS PubMed.
- T. Ogino, T. Suzuki and K. Sawada, Geochim. Cosmochim. Acta, 1987, 51, 2757–2767 CrossRef CAS.
- F. A. Andersen, L. Brečević, G. Beuter, D. B. Dell'amico, F. Calderazzo, N. J. Bjerrum and A. E. Underhill, Acta Chem. Scand., 1991, 45, 1018–1024 CrossRef CAS.
- L. Stapane, N. Le Roy, J. Ezagal, A. B. Rodriguez-Navarro, V. Labas, L. Combes-Soia, M. T. Hincke and J. Gautron, J. Biol. Chem., 2020, 295, 15853–15869 CrossRef CAS PubMed.
- R. A. Van Santen, J. Phys. Chem., 1984, 88, 5768–5769 CrossRef CAS.
- H. Chessin, W. C. Hamilton and B. Post, Acta Crystallogr., 1965, 18, 689–693 CrossRef CAS.
- B. Dickens and J. S. Bowen, J. Res. Natl. Bur. Stand., Sect. A, 1971, 75a, 27–32 CrossRef CAS PubMed.
- S. R. Kamhi, Acta Crystallogr., 1963, 16, 770–772 CrossRef CAS.
- K. Momma and F. Izumi, J. Appl. Crystallogr., 2011, 44, 1272–1276 CrossRef CAS.
- J. Huang and R. Zhang, Front. Mar. Sci., 2022, 9, 874534 CrossRef.
- D. Yang, Y. Yan, X. Yang, J. Liu, G. Zheng, L. Xie and R. Zhang, J. Biol. Chem., 2019, 294, 8371–8383 CrossRef CAS PubMed.
- M. Suzuki, Biosci., Biotechnol., Biochem., 2020, 84, 1529–1540 CrossRef CAS PubMed.
- J. S. Evans, Chem. Rev., 2008, 108, 4455–4462 CrossRef CAS PubMed.
- N. Le Roy, L. Stapane, J. Gautron and M. T. Hincke, Front. Genet., 2021, 12, 672433 CrossRef CAS PubMed.
- J. Gautron, L. Stapane, N. Le Roy, Y. Nys, A. B. Rodriguez-Navarro and M. T. Hincke, BMC Mol. Cell Biol., 2021, 22, 11 CrossRef CAS PubMed.
- L. F. Di Costanzo, Chemistry, 2022, 4, 827–847 CrossRef.
- S. V. Dorozhkin, Biomatter, 2011, 1, 121–164 CrossRef PubMed.
- S. V. Dorozhkin, Materials, 2009, 2, 399–498 CrossRef CAS.
- S. J. Omelon and M. D. Grynpas, Chem. Rev., 2008, 108, 4694–4715 CrossRef CAS PubMed.
- A. George and A. Veis, Chem. Rev., 2008, 108, 4670–4693 CrossRef CAS PubMed.
- F. Nudelman, P. H. H. Bomans, A. George, G. de With and N. A. J. M. Sommerdijk, Faraday Discuss., 2012, 159, 357–370 RSC.
- S. Weiner, J. Mahamid, Y. Politi, Y. Ma and L. Addadi, Front. Mater. Sci. China, 2009, 3, 104–108 CrossRef.
- R. I. Martin and P. W. Brown, J. Biomed. Mater. Res., 1997, 35, 299–308 CrossRef CAS PubMed.
- E. A. Wang, V. Rosen, J. S. D'Alessandro, M. Bauduy, P. Cordes, T. Harada, D. I. Israel, R. M. Hewick, K. M. Kerns and P. LaPan, Proc. Natl. Acad. Sci. U. S. A., 1990, 87, 2220–2224 CrossRef CAS PubMed.
- L. Zhang and T. J. Webster, Nano Today, 2009, 4, 66–80 CrossRef CAS.
- E. Klimuszko, K. Orywal, T. Sierpinska, J. Sidun and M. Golebiewska, Odontology, 2018, 106, 369–376 CrossRef CAS PubMed.
- X. Su, K. Sun, F. Z. Cui and W. J. Landis, Bone, 2003, 32, 150–162 CrossRef CAS PubMed.
- N. C. Collins, H. Thordal-Christensen, V. Lipka, S. Bau, E. Kombrink, J.-L. Qiu, R. Hückelhoven, M. Stein, A. Freialdenhoven, S. C. Somerville and P. Schulze-Lefert, Nature, 2003, 425, 973–977 CrossRef CAS PubMed.
- K. A. Staines, V. E. MacRae and C. Farquharson, J. Endocrinol., 2012, 214, 241–255 CAS.
- A. L. Boskey and E. Villarreal-Ramirez, Matrix Biol., 2016, 52–54, 43–59 CrossRef CAS PubMed.
- A. Gericke, C. Qin, Y. Sun, R. Redfern, D. Redfern, Y. Fujimoto, H. Taleb, W. T. Butler and A. L. Boskey, J. Dent. Res., 2010, 89, 355–359 CrossRef CAS PubMed.
- A. Gericke, C. Qin, L. Spevak, Y. Fujimoto, W. T. Butler, E. S. Sørensen and A. L. Boskey, Calcif. Tissue Int., 2005, 77, 45–54 CrossRef CAS PubMed.
- W. N. Addison, Y. Nakano, T. Loisel, P. Crine and M. D. McKee, J. Bone Miner. Res., 2008, 23, 1638–1649 CrossRef CAS PubMed.
- P. S. Stayton, G. P. Drobny, W. J. Shaw, J. R. Long and M. Gilbert, Crit. Rev. Oral Biol. Med., 2003, 14, 370–376 CrossRef PubMed.
- G. A. Naganagowda, T. L. Gururaja and M. J. Levine, J. Biomol. Struct. Dyn., 1998, 16, 91–107 CrossRef CAS PubMed.
- J. R. Long, W. J. Shaw, P. S. Stayton and G. P. Drobny, Biochemistry, 2001, 40, 15451–15455 CrossRef CAS PubMed.
- S. Kumar, F. Natalio and R. Elbaum, J. Struct. Biol., 2021, 213, 107665 CrossRef CAS PubMed.
- P. Tréguer, D. M. Nelson, A. J. Van Bennekom, D. J. DeMaster, A. Leynaert and B. Quéguiner, Science, 1995, 268, 375–379 CrossRef PubMed.
- D. Otzen, Scientifica, 2012, 2012, 867562 CrossRef PubMed.
- M. Hildebrand, Chem. Rev., 2008, 108, 4855–4874 CrossRef CAS PubMed.
- J. Nawrocki, J. Chromatogr. A, 1997, 779, 29–71 CrossRef CAS.
- N. Kröger, R. Deutzmann and M. Sumper, Science, 1999, 286, 1129–1132 CrossRef PubMed.
- I. E. Pamirsky and K. S. Golokhvast, Mar. Drugs, 2013, 11, 3155–3167 CrossRef PubMed.
- N. Kröger, S. Lorenz, E. Brunner and M. Sumper, Science, 2002, 298, 584–586 CrossRef PubMed.
- M. Sumper and N. Kröger, J. Mater. Chem., 2004, 14, 2059–2065 RSC.
- K. G. Sprenger, A. Prakash, G. Drobny and J. Pfaendtner, Langmuir, 2018, 34, 1199–1207 CrossRef CAS PubMed.
- C. C. Lechner and C. F. W. Becker, Chem. Sci., 2012, 3, 3500–3504 RSC.
- S. Wenzl, R. Hett, P. Richthammer and M. Sumper, Angew. Chem., Int. Ed., 2008, 47, 1729–1732 CrossRef CAS PubMed.
- N. Poulsen and N. Kröger, J. Biol. Chem., 2004, 279, 42993–42999 CrossRef CAS PubMed.
- X. Wang, U. Schloßmacher, M. Wiens, R. Batel, H. C. Schröder and W. E. G. Müller, FEBS J., 2012, 279, 1721–1736 CrossRef CAS PubMed.
- J. N. Cha, K. Shimizu, Y. Zhou, S. C. Christiansen, B. F. Chmelka, G. D. Stucky and D. E. Morse, Proc. Natl. Acad. Sci. U. S. A., 1999, 96, 361–365 CrossRef CAS PubMed.
- H. C. Schröder, M. Wiens, U. Schloßmacher, D. Brandt and W. E. G. Müller, Silicon, 2012, 4, 33–38 CrossRef.
- J. Arima, Y. Sakate, K. Monden, H. Kobayashi, M. Nishi and K. Shimizu, J. Biosci. Bioeng., 2022, 134, 477–483 CrossRef CAS PubMed.
- K. Shimizu, T. Amano, M. R. Bari, J. C. Weaver, J. Arima and N. Mori, Proc. Natl. Acad. Sci. U. S. A., 2015, 112, 11449–11454 CrossRef CAS PubMed.
- T. Kuno, T. Nonoyama, K. Hirao and K. Kato, Langmuir, 2011, 27, 13154–13158 CrossRef CAS PubMed.
- M.-K. Liang, S. V. Patwardhan, E. N. Danilovtseva, V. V. Annenkov and C. C. Perrya, J. Mater. Res., 2009, 24, 1700–1708 CrossRef CAS.
- K. Motomura, T. Ikeda, S. Matsuyama, M. A. A. Abdelhamid, T. Tanaka, T. Ishida, R. Hirota and A. Kuroda, J. Bacteriol., 2016, 198, 276–282 CrossRef CAS PubMed.
- S. Kumar, N. Adiram-Filiba, S. Blum, J. A. Sanchez-Lopez, O. Tzfadia, A. Omid, H. Volpin, Y. Heifetz, G. Goobes and R. Elbaum, J. Exp. Bot., 2020, 71, 6830–6843 CrossRef CAS PubMed.
- T. S. Wong, D. Roccatano, M. Zacharias and U. Schwaneberg, J. Mol. Biol., 2006, 355, 858–871 CrossRef CAS PubMed.
- A. V. Shivange, J. Marienhagen, H. Mundhada, A. Schenk and U. Schwaneberg, Curr. Opin. Chem. Biol., 2009, 13, 19–25 CrossRef CAS PubMed.
- M. G. Patino, M. E. Neiders, S. Andreana, B. Noble and R. E. Cohen, Implant Dent., 2002, 11, 280–285 CrossRef PubMed.
- M. D. Shoulders and R. T. Raines, Annu. Rev. Biochem., 2009, 78, 929–958 CrossRef CAS PubMed.
- S. Ricard-Blum, Cold Spring Harbor Perspect. Biol., 2011, 3, a004978 Search PubMed.
- D. Brett, Wounds: a compendium of clinical research and practice, 2008, 20, 347–356 Search PubMed.
- A. Sionkowska, S. Skrzyński, K. Śmiechowski and A. Kołodziejczak, Polym. Adv. Technol., 2017, 28, 4–9 CrossRef CAS.
- A. Vasconcelos and A. Cavaco-Paulo, Curr. Drug Targets, 2013, 14, 612–619 CrossRef CAS PubMed.
- S. Feroz, N. Muhammad, J. Ratnayake and G. Dias, Bioact. Mater., 2020, 5, 496–509 Search PubMed.
- J. G. Rouse and M. E. Van Dyke, Materials, 2010, 3, 999–1014 CrossRef.
- L. Wang, Y. Shang, J. Zhang, J. Yuan and J. Shen, Adv. Colloid Interface Sci., 2023, 321, 103012 CrossRef CAS PubMed.
- J. Yu, D.-W. Yu, D. M. Checkla, I. M. Freedberg and A. P. Bertolino, J. Invest. Dermatol., 1993, 101, S56–S59 CrossRef.
- T. P. Nguyen, Q. V. Nguyen, V.-H. Nguyen, T.-H. Le, V. Q. N. Huynh, D.-V. N. Vo, Q. T. Trinh, S. Y. Kim and Q. V. Le, Polymers, 2019, 11, 1933 CrossRef CAS PubMed.
- L.-D. Koh, Y. Cheng, C.-P. Teng, Y.-W. Khin, X.-J. Loh, S.-Y. Tee, M. Low, E. Ye, H.-D. Yu, Y.-W. Zhang and M.-Y. Han, Prog. Polym. Sci., 2015, 46, 86–110 CrossRef CAS.
- E. Wenk, H. P. Merkle and L. Meinel, J. Controlled Release, 2011, 150, 128–141 CrossRef CAS PubMed.
- I. Streeter and N. H. de Leeuw, Soft Matter, 2011, 7, 3373–3382 RSC.
- S. Leikin, D. C. Rau and V. A. Parsegian, Nat. Struct. Biol., 1995, 2, 205–210 CrossRef CAS PubMed.
- K. Claire and R. Pecora, J. Phys. Chem. B, 1997, 101, 746–753 CrossRef CAS.
- K. E. Kadler, D. F. Holmes, J. A. Trotter and J. A. Chapman, Biochem. J., 1996, 316, 1–11 CrossRef CAS PubMed.
- M. G. Patino, M. E. Neiders, S. Andreana, B. Noble and R. E. Cohen, Implant Dent., 2002, 11, 280–285 CrossRef PubMed.
- K. K. Takita, K. K. Fujii, T. Kadonosono, R. Masuda and T. Koide, ChemBioChem, 2018, 19, 1613–1617 CrossRef CAS PubMed.
- K. Kar, S. Ibrar, V. Nanda, T. M. Getz, S. P. Kunapuli and B. Brodsky, Biochemistry, 2009, 48, 7959–7968 CrossRef CAS PubMed.
- J. Bella, B. Brodsky and H. M. Berman, Structure, 1995, 3, 893–906 CrossRef CAS PubMed.
- C.-H. Lee, M.-S. Kim, S. Li, D. J. Leahy and P. A. Coulombe, Structure, 2020, 28, 355–362.e354 CrossRef CAS PubMed.
- R. Sistiabudi and A. Ivanisevic, Langmuir, 2008, 24, 1591–1594 CrossRef CAS PubMed.
- Y. J. Choi, J. Y. Lee, C.-P. Chung and Y. J. Park, J. Biomed. Mater. Res., Part A, 2013, 101A, 547–554 CrossRef CAS PubMed.
- R. Fujisawa, Y. Nodasaka and Y. Kuboki, Calcif. Tissue Int., 1995, 56, 140–144 CrossRef CAS PubMed.
- W. J. Landis and R. Jacquet, Calcif. Tissue Int., 2013, 93, 329–337 CrossRef CAS PubMed.
- Z. Xu, Y. Yang, W. Zhao, Z. Wang, W. J. Landis, Q. Cui and N. Sahai, Biomaterials, 2015, 39, 59–66 CrossRef CAS PubMed.
- I. Goldberga, R. Li and M. J. Duer, Acc. Chem. Res., 2018, 51, 1621–1629 CrossRef CAS PubMed.
- P. Kar and M. Misra, J. Chem. Technol. Biotechnol., 2004, 79, 1313–1319 CrossRef CAS.
- M. Er Rafik, J. Doucet and F. Briki, Biophys. J., 2004, 86, 3893–3904 CrossRef CAS PubMed.
- C. F. Cruz, M. Martins, J. Egipto, H. Osório, A. Ribeiro and A. Cavaco-Paulo, RSC Adv., 2017, 7, 51581–51592 RSC.
- C. F. Cruz, N. G. Azoia, T. Matamá and A. Cavaco-Paulo, Int. J. Biol. Macromol., 2017, 101, 805–814 CrossRef CAS PubMed.
- M. Ru, A. M. Hai, L. Wang, S. Yan and Q. Zhang, Int. J. Biol. Macromol., 2023, 224, 422–436 CrossRef CAS PubMed.
- L. Xiao, G. Lu, Q. Lu and D. L. Kaplan, ACS Biomater. Sci. Eng., 2016, 2, 2050–2057 CrossRef CAS PubMed.
- C. Holland, K. Numata, J. Rnjak-Kovacina and F. P. Seib, Adv. Healthcare Mater., 2019, 8, 1800465 CrossRef PubMed.
- S. Inoue, K. Tanaka, F. Arisaka, S. Kimura, K. Ohtomo and S. Mizuno, J. Biol. Chem., 2000, 275, 40517–40528 CrossRef CAS PubMed.
- T. Asakura, Molecules, 2021, 26, 3706 CrossRef CAS PubMed.
- C.-Z. Zhou, F. Confalonieri, M. Jacquet, R. Perasso, Z.-G. Li and J. Janin, Proteins: Struct., Funct., Bioinf., 2001, 44, 119–122 CrossRef CAS PubMed.
- K. Tanaka, S. Inoue and S. Mizuno, Insect Biochem. Mol. Biol., 1999, 29, 269–276 CrossRef CAS PubMed.
- R. I. Kunz, R. M. C. Brancalhão, L. D. F. C. Ribeiro and M. R. M. Natali, BioMed Res. Int., 2016, 2016, 8175701 Search PubMed.
- S. Du, J. Zhang, W. T. Zhou, Q. X. Li, G. W. Greene, H. J. Zhu, J. L. Li and X. G. Wang, J. Colloid Interface Sci., 2016, 478, 316–323 CrossRef CAS PubMed.
-
P. A. Narendra Reddy, Sustainable Use of Byproducts from Silk Processing, 2021, pp. 1–37 Search PubMed.
- K. H. Lee, Macromol. Rapid Commun., 2004, 25, 1792–1796 CrossRef CAS.
- M. Ndao, J. T. Ash, P. S. Stayton and G. P. Drobny, Surf. Sci., 2010, 604, L39–L42 CrossRef CAS PubMed.
- G. He, T. Dahl, A. Veis and A. George, Nat. Mater., 2003, 2, 552–558 CrossRef CAS PubMed.
- I. Matlahov, T. Iline-Vul, M. Abayev, E. M. Y. Lee, M. Nadav-Tsubery, K. Keinan-Adamsky, J. J. Gray and G. Goobes, Chem. Mater., 2015, 27, 5562–5569 CrossRef CAS.
- C. E. Tye, K. R. Rattray, K. J. Warner, J. A. R. Gordon, J. Sodek, G. K. Hunter and H. A. Goldberg, J. Biol. Chem., 2003, 278, 7949–7955 CrossRef CAS PubMed.
- V. David, A. Martin, A.-M. Hedge, M. K. Drezner and P. S. N. Rowe, Am. J. Physiol.: Renal Physiol., 2011, 300, F783–F791 CrossRef CAS PubMed.
- F. J. Bikker, N. Cukkemane, K. Nazmi and E. C. I. Veerman, Eur. J. Oral Sci., 2013, 121, 7–12 CrossRef CAS PubMed.
- V. Gerbaud, D. Pignol, E. Loret, J. A. Bertrand, Y. Berland, J.-C. Fontecilla-Camps, J.-P. Canselier, N. Gabas and J.-M. Verdier, J. Biol. Chem., 2000, 275, 1057–1064 CrossRef CAS PubMed.
- B. A. Wustman, J. C. Weaver, D. E. Morse and J. S. Evans, Connect. Tissue Res., 2003, 44, 10–15 CrossRef CAS PubMed.
- B.-A. Gotliv, N. Kessler, J. L. Sumerel, D. E. Morse, N. Tuross, L. Addadi and S. Weiner, ChemBioChem, 2005, 6, 304–314 CrossRef CAS PubMed.
- M. Suzuki, K. Saruwatari, T. Kogure, Y. Yamamoto, T. Nishimura, T. Kato and H. Nagasawa, Science, 2009, 325, 1388–1390 CrossRef CAS PubMed.
- C. F. Anderson, R. W. Chakroun, M. E. Grimmett, C. J. Domalewski, F. Wang and H. Cui, Nano Lett., 2022, 22, 4182–4191 CrossRef CAS PubMed.
- R. Geyer, J. R. Jambeck and K. L. Law, Sci. Adv., 2017, 3, e1700782 CrossRef PubMed.
- K. L. Law and R. Narayan, Nat. Rev. Mater., 2022, 7, 104–116 CrossRef CAS.
-
H. A. Maddah, 2016.
-
H. Karian, Handbook of polypropylene and polypropylene composites, revised and expanded, CRC Press, 2003 Search PubMed.
-
M. Jambrich and P. Hodul, in Polypropylene: An A–Z reference, ed. J. Karger-Kocsis, Springer Netherlands, Dordrecht, 1999, pp. 806–812 DOI:10.1007/978-94-011-4421-6_110.
- K. Rübsam, B. Stomps, A. Böker, F. Jakob and U. Schwaneberg, Polymer, 2017, 116, 124–132 CrossRef.
- W. Gong, J. Wang, Z. Chen, B. Xia and G. Lu, Biochemistry, 2011, 50, 3621–3627 CrossRef CAS PubMed.
- L. Silvestro, J. N. Weiser and P. H. Axelsen, Antimicrob. Agents Chemother., 2000, 44, 602–607 CrossRef CAS PubMed.
- S. Dedisch, A. Wiens, M. D. Davari, D. Söder, C. Rodriguez-Emmenegger, F. Jakob and U. Schwaneberg, Biotechnol. Bioeng., 2020, 117, 49–61 CrossRef CAS PubMed.
- M. M. M. Kasturi, N. A. Kamaruding and N. Ismail, Appl. Biochem. Microbiol., 2021, 57, 311–318 CrossRef CAS.
- N. Fujitani, S.-I. Kawabata, T. Osaki, Y. Kumaki, M. Demura, K. Nitta and K. Kawano, J. Biol. Chem., 2002, 277, 23651–23657 CrossRef CAS PubMed.
- T. Gitlesen, M. Bauer and P. Adlercreutz, Biochim. Biophys. Acta, Lipids Lipid Metab., 1997, 1345, 188–196 CrossRef CAS PubMed.
- P. L. Felgner and J. E. Wilson, Anal. Biochem., 1976, 74, 631–635 CrossRef CAS PubMed.
-
J. Maul, B. G. Frushour, J. R. Kontoff, H. Eichenauer, K.-H. Ott and C. Schade, Ullmann's Encyclopedia of Industrial Chemistry, 2007 Search PubMed.
-
I. Baker, in Fifty Materials That Make the World, ed. I. Baker, Springer International Publishing, Cham, 2018, pp. 175–178 DOI:10.1007/978-3-319-78766-4_33.
- K. Rubsam, L. Weber, F. Jakob and U. Schwaneberg, Biotechnol. Bioeng., 2018, 115, 321–330 CrossRef PubMed.
- C. Heilmann, M. Hussain, G. Peters and F. Götz, Mol. Microbiol., 1997, 24, 1013–1024 CrossRef CAS PubMed.
- R. P. Somarathne, E. R. Chappell, Y. R. Perera, R. Yadav, J. Y. Park and N. C. Fitzkee, Front. Microbiol., 2021, 12, 658373 CrossRef PubMed.
- L. A. Cantarero, J. E. Butler and J. W. Osborne, Anal. Biochem., 1980, 105, 375–382 CrossRef CAS PubMed.
- L. De Vos, B. Van de Voorde, L. Van Daele, P. Dubruel and S. Van Vlierberghe, Eur. Polym. J., 2021, 161, 110840 CrossRef CAS.
-
B. Demirel, A. Yaraş and H. Elçiçek, 2011.
- S. Yoshida, K. Hiraga, T. Takehana, I. Taniguchi, H. Yamaji, Y. Maeda, K. Toyohara, K. Miyamoto, Y. Kimura and K. Oda, Science, 2016, 351, 1196–1199 CrossRef CAS PubMed.
- D. Ribitsch, A. O. Yebra, S. Zitzenbacher, J. Wu, S. Nowitsch, G. Steinkellner, K. Greimel, A. Doliska, G. Oberdorfer, C. C. Gruber, K. Gruber, H. Schwab, K. Stana-Kleinschek, E. H. Acero and G. M. Guebitz, Biomacromolecules, 2013, 14, 1769–1776 CrossRef CAS PubMed.
- R. Xue, Y. Chen, H. Rong, R. Wei, Z. Cui, J. Zhou, W. Dong and M. Jiang, Front. Bioeng. Biotechnol., 2021, 9, 762854 CrossRef PubMed.
- S. Dedisch, A. Wiens, M. D. Davari, D. Soder, C. Rodriguez-Emmenegger, F. Jakob and U. Schwaneberg, Biotechnol. Bioeng., 2020, 117, 49–61 CrossRef CAS PubMed.
- A. Mor, V. H. Nguyen, A. Delfour, D. Migliore-Samour and P. Nicolas, Biochemistry, 1991, 30, 8824–8830 CrossRef CAS PubMed.
- H.-S. Park and C.-K. Hong, Polymers, 2021, 13, 1851 CrossRef CAS PubMed.
- C. Wellenreuther, A. Wolf and N. Zander, Clean. Eng. Technol., 2022, 6, 100411 CrossRef.
- E. Capuana, F. Lopresti, M. Ceraulo and V. La Carrubba, Journal, 2022, 14, 1153 CAS.
- M. Jamshidian, E. A. Tehrany, M. Imran, M. Jacquot and S. Desobry, Compr. Rev. Food Sci. Food Saf., 2010, 9, 552–571 CrossRef CAS PubMed.
- Y. Lu, K.-W. Hintzen, T. Kurkina, Y. Ji and U. Schwaneberg, ACS Catal., 2023, 13, 12746–12754 CrossRef CAS PubMed.
- Y. Gueguen, A. Herpin, A. Aumelas, J. Garnier, J. Fievet, J.-M. Escoubas, P. Bulet, M. Gonzalez, C. Lelong, P. Favrel and E. Bachère, J. Biol. Chem., 2006, 281, 313–323 CrossRef CAS PubMed.
- N. V. Gama, A. Ferreira and A. Barros-Timmons, Materials, 2018, 11, 1841 CrossRef PubMed.
- K. Rubsam, M. D. Davari, F. Jakob and U. Schwaneberg, Polymers, 2018, 10, 423 CrossRef PubMed.
- U. T. Bornscheuer, B. Hauer, K. E. Jaeger and U. Schwaneberg, Angew. Chem., Int. Ed., 2019, 58, 36–40 CrossRef CAS PubMed.
- G. Å. Løset and I. Sandlie, Methods, 2012, 58, 40–46 CrossRef PubMed.
- G. Winter, A. D. Griffiths, R. E. Hawkins and H. R. Hoogenboom, Annu. Rev. Immunol., 1994, 12, 433–455 CrossRef CAS PubMed.
- J. C. Almagro, M. Pedraza-Escalona, H. I. Arrieta and S. M. Pérez-Tapia, Antibodies, 2019, 8, 44 CrossRef CAS PubMed.
- W. Jaroszewicz, J. Morcinek-Orłowska, K. Pierzynowska, L. Gaffke and G. Węgrzyn, FEMS Microbiol. Rev., 2021, 46, 1–25 Search PubMed.
- V. Frappier and A. E. Keating, Curr. Opin. Struct. Biol., 2021, 69, 63–69 CrossRef CAS PubMed.
- C. M. Clemente, L. Capece and M. A. Martí, J. Chem. Inf. Model., 2023, 63, 2609–2627 CrossRef CAS PubMed.
- F. H. Arnold, Angew. Chem., Int. Ed., 2018, 57, 4143–4148 CrossRef CAS PubMed.
- F. Cheng, L. Zhu and U. Schwaneberg, Chem. Commun., 2015, 51, 9760–9772 RSC.
- J. Yang, A. J. Ruff, M. Arlt and U. Schwaneberg, Biotechnol. Bioeng., 2017, 114, 1921–1927 CrossRef CAS PubMed.
- K. K. Yang, Z. Wu and F. H. Arnold, Nat. Methods, 2019, 16, 687–694 CrossRef CAS PubMed.
- K. L. Strobel, K. A. Pfeiffer, H. W. Blanch and D. S. Clark, Biotechnol. Bioeng., 2016, 113, 1369–1374 CrossRef CAS PubMed.
- G. P. Smith, S. U. Patel, J. D. Windass, J. M. Thornton, G. Winter and A. D. Griffiths, J. Mol. Biol., 1998, 277, 317–332 CrossRef CAS PubMed.
- J. Lehtiö, T. T. Teeri and P.-Å. Nygren, Proteins: Struct., 2000, 41, 316–322 CrossRef.
- L. Cicortas Gunnarsson, E. Nordberg Karlsson, M. Andersson, O. Holst and M. Ohlin, Biocatal. Biotransform., 2006, 24, 31–37 CrossRef.
- L. Cicortas Gunnarsson, E. Nordberg Karlsson, A. S. Albrekt, M. Andersson, O. Holst and M. Ohlin, Protein Eng., Des. Sel., 2004, 17, 213–221 CrossRef CAS PubMed.
- L. C. Gunnarsson, L. Dexlin, E. N. Karlsson, O. Holst and M. Ohlin, Biomol. Eng., 2006, 23, 111–117 CrossRef CAS PubMed.
- L. C. Gunnarsson, Q. Zhou, C. Montanier, E. N. Karlsson, H. Brumer, III and M. Ohlin, Glycobiology, 2006, 16, 1171–1180 CrossRef CAS PubMed.
- F. Gullfot, T.-C. Tan, L. von Schantz, E. N. Karlsson, M. Ohlin, H. Brumer and C. Divne, Proteins: Struct., Funct., Bioinf., 2010, 78, 785–789 CrossRef CAS PubMed.
- L. Cicortas Gunnarsson, C. Montanier, R. B. Tunnicliffe, M. P. Williamson, H. J. Gilbert, E. Nordberg Karlsson and M. Ohlin, Biochem. J., 2007, 406, 209–214 CrossRef CAS PubMed.
- P. J. Simpson, S. J. Jamieson, M. Abou-Hachem, E. N. Karlsson, H. J. Gilbert, O. Holst and M. P. Williamson, Biochemistry, 2002, 41(18), 5712–5719 CrossRef CAS PubMed.
- L. von Schantz, M. Håkansson, D. T. Logan, B. Walse, J. Österlin, E. Nordberg-Karlsson and M. Ohlin, Glycobiology, 2012, 22, 948–961 CrossRef CAS PubMed.
- G. P. Furtado, M. R. Lourenzoni, C. A. Fuzo, R. Fonseca-Maldonado, M.-E. Guazzaroni, L. F. Ribeiro and R. J. Ward, Int. J. Biol. Macromol., 2018, 120, 2509–2516 CrossRef CAS PubMed.
- C.-L. Chen, J. Qi, R. N. Zuckermann and J. J. DeYoreo, J. Am. Chem. Soc., 2011, 133, 5214–5217 CrossRef CAS PubMed.
- B. An, T. M. DesRochers, G. Qin, X. Xia, G. Thiagarajan, B. Brodsky and D. L. Kaplan, Biomaterials, 2013, 34, 402–412 CrossRef CAS PubMed.
- Y. Zhang, L. Wang, J. Chen and J. Wu, Carbohydr. Polym., 2013, 97, 124–129 CrossRef CAS PubMed.
- J. Weber, D. Petrović, B. Strodel, S. H. J. Smits, S. Kolkenbrock, C. Leggewie and K.-E. Jaeger, Appl. Microbiol. Biotechnol., 2019, 103, 4801–4812 CrossRef CAS PubMed.
- R. Graham, E. Erickson, R. K. Brizendine, D. Salvachúa, W. E. Michener, Y. Li, Z. Tan, G. T. Beckham, J. E. McGeehan and A. R. Pickford, Chem. Catal., 2022, 2, 2644–2657 CrossRef CAS.
- A. P. Rennison, P. Westh and M. S. Møller, Sci. Total Environ., 2023, 870, 161948 CrossRef CAS PubMed.
- Y. Lu, K.-W. Hintzen, T. Kurkina, Y. Ji and U. Schwaneberg, Adv. Sci., 2023, 10, 2303195 CrossRef CAS PubMed.
- R. Köhler, C. Pohl, U. Walschus, R. Zippel, L. Wilhelm, A. Hoene, M. Patrzyk and M. Schlosser, J. Biomed. Mater. Res., Part A, 2022, 110, 52–63 CrossRef PubMed.
- L. Cao, X. Zeng and J. Lin, Chem. Eng. J., 2023, 465, 142843 CrossRef CAS.
- A. Skerra, Curr. Opin. Biotechnol, 2007, 18, 295–304 CrossRef CAS PubMed.
- L. A. Stern, B. A. Case and B. J. Hackel, Curr. Opin. Chem. Eng., 2013, 2, 425–432 CrossRef PubMed.
- R. Simeon and Z. Chen, Protein Cell, 2018, 9, 3–14 CrossRef CAS PubMed.
- Z. R. Crook, N. W. Nairn and J. M. Olson, Trends Biochem. Sci., 2020, 45, 332–346 CrossRef CAS PubMed.
- M. Gebauer and A. Skerra, Curr. Opin. Chem. Biol., 2009, 13, 245–255 CrossRef CAS PubMed.
- Y. L. Boersma and A. Plückthun, Curr. Opin. Biotechnol, 2011, 22, 849–857 CrossRef CAS PubMed.
- X. Wang, F. Li, W. Qiu, B. Xu, Y. Li, X. Lian, H. Yu, Z. Zhang, J. Wang, Z. Li, W. Xue and F. Zhu, Nucleic Acids Res., 2021, 50, D560–D570 CrossRef PubMed.
- P.-Å. Nygren and A. Skerra, J. Immunol. Methods, 2004, 290, 3–28 CrossRef CAS PubMed.
- H. K. Binz, P. Amstutz and A. Plückthun, Nat. Biotechnol., 2005, 23, 1257–1268 CrossRef CAS PubMed.
- D. Könning and H. Kolmar, Microb. Cell Fact., 2018, 17, 32 CrossRef PubMed.
- M. T. Stumpp and P. Amstutz, Curr. Opin. Drug Discovery Dev., 2007, 10, 153–159 CAS.
-
M. Gebauer and A. Skerra, in Methods in Enzymology, ed. K. D. Wittrup and G. L. Verdine, Academic Press, 2012, vol. 503, pp. 157–188 Search PubMed.
- D. Lipovšek, Protein Eng., Des. Sel., 2010, 24, 3–9 CrossRef PubMed.
- J. Löfblom, J. Feldwisch, V. Tolmachev, J. Carlsson, S. Ståhl and F. Y. Frejd, FEBS Lett., 2010, 584, 2670–2680 CrossRef PubMed.
- F. Y. Frejd and K.-T. Kim, Exp. Mol. Med., 2017, 49, e306–e306 CrossRef CAS PubMed.
- S. Muyldermans, Annu. Rev. Biochem., 2013, 82, 775–797 CrossRef CAS PubMed.
- M. Mishra, J. Mol. Evol., 2020, 88, 537–548 CrossRef CAS PubMed.
- A. Skerra, Biochim. Biophys. Acta, Protein Struct. Mol. Enzymol., 2000, 1482, 337–350 CrossRef CAS PubMed.
- J. Silverman, Q. Lu, A. Bakker, W. To, A. Duguay, B. M. Alba, R. Smith, A. Rivas, P. Li, H. Le, E. Whitehorn, K. W. Moore, C. Swimmer, V. Perlroth, M. Vogt, J. Kolkman and W. P. C. Stemmer, Nat. Biotechnol., 2005, 23, 1556–1561 CrossRef CAS PubMed.
- J. Gracy and L. Chiche, Curr. Pharm. Des., 2011, 17, 4337–4350 CrossRef CAS PubMed.
- H. Wernérus, P. Samuelson and S. Ståhl, Appl. Environ. Microbiol., 2003, 69, 5328–5335 CrossRef PubMed.
- B. J. Grindel, B. J. Engel, J. N. Ong, A. Srinivasamani, X. Liang, N. M. Zacharias, R. C. Bast, Jr., M. A. Curran, T. T. Takahashi, R. W. Roberts and S. W. Millward, ACS Chem. Biol., 2022, 17, 1543–1555 CrossRef CAS PubMed.
- S. Grimm, F. Yu and P.-Å. Nygren, Mol. Biotechnol., 2011, 48, 263–276 CrossRef CAS PubMed.
-
S. Ståhl, L. C. Hjelm, J. Löfblom and H. Lindberg, Cold Spring Harb. Protoc., 2023 Search PubMed.
- R. N. Gilbreth and S. Koide, Curr. Opin. Struct. Biol., 2012, 22, 413–420 CrossRef CAS PubMed.
- R. N. Gilbreth and S. Koide, Curr. Opin. Struct. Biol., 2012, 22, 413–420 CrossRef CAS PubMed.
- E. Wahlberg, C. Lendel, M. Helgstrand, P. Allard, V. Dincbas-Renqvist, A. Hedqvist, H. Berglund, P.-Å. Nygren and T. Härd, Proc. Natl. Acad. Sci. U. S. A., 2003, 100, 3185–3190 CrossRef CAS PubMed.
- R. Güler, S. F. Svedmark, A. Abouzayed, A. Orlova and J. Löfblom, Sci. Rep., 2020, 10, 18148 CrossRef PubMed.
- D. R. Woldring, P. V. Holec, L. A. Stern, Y. Du and B. J. Hackel, Biochemistry, 2017, 56, 1656–1671 CrossRef CAS PubMed.
- E. Wahlberg and T. Härd, J. Am. Chem. Soc., 2006, 128, 7651–7660 CrossRef CAS PubMed.
- S. Ståhl, T. Gräslund, A. Eriksson Karlström, F. Y. Frejd, P.-Å. Nygren and J. Löfblom, Trends Biotechnol., 2017, 35, 691–712 CrossRef PubMed.
- F. Behrens, P. C. Taylor, D. Wetzel, N. C. Brun, J. Brandt-Juergens, E. Drescher, E. Dokoupilova, A. Rowińska-Osuch, N. A.-K. Martin and K. D. Vlam, Ann. Rheum. Dis., 2022, 81, 170–171 CrossRef.
- B.-k Jin, S. Odongo, M. Radwanska and S. Magez, Int. J. Mol. Sci., 2023, 24, 5994 CrossRef CAS PubMed.
- X. Wang, Q. Chen, Z. Sun, Y. Wang, B. Su, C. Zhang, H. Cao and X. Liu, Int. J. Biol. Macromol., 2020, 151, 312–321 CrossRef CAS PubMed.
- D. Schumacher, J. Helma, A. F. L. Schneider, H. Leonhardt and C. P. R. Hackenberger, Angew. Chem., Int. Ed., 2018, 57, 2314–2333 CrossRef CAS PubMed.
- L. S. Mitchell and L. J. Colwell, Proteins: Struct., Funct., Bioinf., 2018, 86, 697–706 CrossRef CAS PubMed.
- T. De Meyer, S. Muyldermans and A. Depicker, Trends Biotechnol., 2014, 32, 263–270 CrossRef CAS PubMed.
- G. Gonzalez-Sapienza, M. A. Rossotti and S. Tabares-da Rosa, Front. Immunol., 2017, 8, 288027 Search PubMed.
- S. Kunz, M. Durandy, L. Seguin and C. C. Feral, Int. J. Mol. Sci., 2023, 24, 13229 CrossRef CAS PubMed.
- A. Hinz, D. Lutje Hulsik, A. Forsman, W. W.-L. Koh, H. Belrhali, A. Gorlani, H. de Haard, R. A. Weiss, T. Verrips and W. Weissenhorn, PLoS One, 2010, 5, e10482 CrossRef PubMed.
- Y. Xiang, S. Nambulli, Z. Xiao, H. Liu, Z. Sang, W. P. Duprex, D. Schneidman-Duhovny, C. Zhang and Y. Shi, Science, 2020, 370, 1479–1484 CrossRef CAS PubMed.
- Y. He, Y. Ren, B. Guo, Y. Yang, Y. Ji, D. Zhang, J. Wang, Y. Wang and H. Wang, Food Chem., 2020, 310, 125942 CrossRef CAS PubMed.
- I. A. Ehrnstorfer, E. R. Geertsma, E. Pardon, J. Steyaert and R. Dutzler, Nat. Struct. Mol. Biol., 2014, 21, 990–996 CrossRef CAS PubMed.
- E. R. Verhaar, A. W. Woodham and H. L. Ploegh, Semin. Immunol., 2021, 52, 101425 CrossRef CAS PubMed.
- S. Schoonooghe, D. Laoui, J. A. Van Ginderachter, N. Devoogdt, T. Lahoutte, P. De Baetselier and G. Raes, Immunobiology, 2012, 217, 1266–1272 CrossRef CAS PubMed.
- Z. Lu, Z. Liu, X. Li, X. Qin, H. Hong, Z. Zhou, R. J. Pieters, J. Shi and Z. Wu, ACS Infect. Dis., 2022, 8, 321–329 CrossRef CAS PubMed.
- J. Jung, Y. Jeong, Y. Xu, J. Yi, M. Kim, H.-J. Jeong, S. H. Shin, Y.-H. Yang, J. Son and C. Sung, Drug Test. Anal., 2023, 221 Search PubMed.
- C. Lendel, J. Dogan and T. Härd, J. Mol. Biol., 2006, 359, 1293–1304 CrossRef CAS PubMed.
- M. B. Braun, B. Traenkle, P. A. Koch, F. Emele, F. Weiss, O. Poetz, T. Stehle and U. Rothbauer, Sci. Rep., 2016, 6, 19211 CrossRef CAS PubMed.
- L. S. Mitchell and L. J. Colwell, Protein Eng., Des. Sel., 2018, 31, 267–275 CrossRef CAS PubMed.
- R. Saito, Y. Saito, H. Nakazawa, T. Hattori, I. Kumagai, M. Umetsu and K. Makabe, J. Biochem., 2018, 164, 21–25 CrossRef CAS PubMed.
- T. Hattori, M. Umetsu, T. Nakanishi, T. Togashi, N. Yokoo, H. Abe, S. Ohara, T. Adschiri and I. Kumagai, J. Biol. Chem., 2010, 285, 7784–7793 CrossRef CAS PubMed.
-
P. V. Erik Jongedijk, U. S. Pat. No. 8598081B2, 2013 Search PubMed.
-
P. V. Erik
Jongedijk, Eu Pat. Office No. 2609116A1, 2011 Search PubMed.
-
C. D. J. Peter Verheesen and E. Jongedijk, Eu. Pat. Office No. 2609115B1, 2011 Search PubMed.
- R. R. Naik, L. L. Brott, S. J. Clarson and M. O. Stone, J. Nanosci. Nanotechnol., 2002, 2, 95–100 CrossRef CAS PubMed.
- E. Eteshola, L. J. Brillson and S. C. Lee, Biomol. Eng., 2005, 22, 201–204 CrossRef CAS PubMed.
- H. Chen, X. Su, K.-G. Neoh and W.-S. Choe, Anal. Chem., 2006, 78, 4872–4879 CrossRef CAS PubMed.
- C. Tamerler, T. Kacar, D. Sahin, H. Fong and M. Sarikaya, Mater. Sci. Eng., C, 2007, 27, 558–564 CrossRef CAS.
- E. E. Oren, C. Tamerler, D. Sahin, M. Hnilova, U. O. S. Seker, M. Sarikaya and R. Samudrala, Bioinformatics, 2007, 23, 2816–2822 CrossRef CAS PubMed.
- W.-J. Chung, K.-Y. Kwon, J. Song and S.-W. Lee, Langmuir, 2011, 27, 7620–7628 CrossRef CAS PubMed.
- M. Gungormus, H. Fong, I. W. Kim, J. S. Evans, C. Tamerler, C. Tamerler and M. Sarikaya, Biomacromolecules, 2008, 9, 966–973 CrossRef CAS PubMed.
- M. D. Roy, S. K. Stanley, E. J. Amis and M. L. Becker, Adv. Mater., 2008, 20, 1830–1836 CrossRef CAS.
- S. Segvich, S. Biswas, U. Becker and D. H. Kohn, Cells Tissues Organs, 2008, 189, 245–251 CrossRef PubMed.
- D. J. H. Gaskin, K. Starck and E. N. Vulfson, Biotechnol. Lett., 2000, 22, 1211–1216 CrossRef CAS.
- T. Schüler, J. Renkel, S. Hobe, M. Susewind, D. E. Jacob, M. Panthöfer, A. Hoffmann-Röder, H. Paulsen and W. Tremel, J. Mater. Chem. B, 2014, 2, 3511–3518 RSC.
- S. Takeshi, I. Kyoko, M. Hisao and K. Kimio, Chem. Lett., 2007, 36, 988–989 CrossRef.
- M. Qi, J. P. O'Brien and J. Yang, Pept. Sci., 2008, 90, 28–36 CrossRef CAS PubMed.
- J. Guo, J. M. Catchmark, M. N. A. Mohamed, A. J. Benesi, M. Tien, T.-H. Kao, H. D. Watts and J. D. Kubicki, Biomacromolecules, 2013, 14, 1795–1805 CrossRef CAS PubMed.
- F. Khoushab, N. Jaruseranee, W. Tanthanuch and M. Yamabhai, Int. J. Biol. Macromol., 2012, 50, 1267–1274 CrossRef CAS PubMed.
- Y. Tang, S. Wu, J. Lin, L. Cheng, J. Zhou, J. Xie, K. Huang, X. Wang, Y. Yu, Z. Chen, G. Liao and C. Li, Nano Lett., 2018, 18, 6207–6213 CrossRef CAS PubMed.
- H. Ikemoto, P. Lingasamy, A.-M. Anton Willmore, H. Hunt, K. Kurm, O. Tammik, P. Scodeller, L. Simón-Gracia, V. R. Kotamraju, A. M. Lowy, K. N. Sugahara and T. Teesalu, Tumor Biol., 2017, 39, 1010428317701628 Search PubMed.
- C. Tolg, S. R. Hamilton, E. Zalinska, L. McCulloch, R. Amin, N. Akentieva, F. Winnik, R. Savani, D. J. Bagli, L. G. Luyt, M. K. Cowman, J. B. McCarthy and E. A. Turley, Am. J. Pathol., 2012, 181, 1250–1270 CrossRef CAS PubMed.
- X. Qin, H. Zhao, Y. Jiang, F. Yin, Y. Tian, M. Xie, X. Ye, N. Xu and Z. Li, Chin. Chem. Lett., 2018, 29, 1160–1162 CrossRef CAS.
- K. Amemiya, T. Nakatani, A. Saito, A. Suzuki and H. Munakata, Biochim. Biophys. Acta, Gen. Subj., 2005, 1724, 94–99 CrossRef CAS PubMed.
- J. Pai, J. Y. Hyun, J. Jeong, S. Loh, E.-H. Cho, Y.-S. Kang and I. Shin, Chem. Sci., 2016, 7, 2084–2093 RSC.
- T. Yabe, R. Hosoda-Yabe, Y. Kanamaru and M. Kiso, J. Biol. Chem., 2011, 286, 12397–12406 CrossRef CAS PubMed.
- T. F. Gesteira, V. J. Coulson-Thomas, A. Taunay-Rodrigues, V. Oliveira, B. E. Thacker, M. A. Juliano, R. Pasqualini, W. Arap, I. L. S. Tersariol, H. B. Nader, J. D. Esko and M. A. S. Pinhal, J. Biol. Chem., 2011, 286, 5338–5346 CrossRef CAS PubMed.
- D. A. Rothenfluh, H. Bermudez, C. P. O’Neil and J. A. Hubbell, Nat. Mater., 2008, 7, 248–254 CrossRef CAS PubMed.
- H. Wahyudi, A. A. Reynolds, Y. Li, S. C. Owen and S. M. Yu, J. Controlled Release, 2016, 240, 323–331 CrossRef CAS PubMed.
- J. M. Chan, L. Zhang, R. Tong, D. Ghosh, W. Gao, G. Liao, K. P. Yuet, D. Gray, J.-W. Rhee, J. Cheng, G. Golomb, P. Libby, R. Langer and O. C. Farokhzad, Proc. Natl. Acad. Sci. U. S. A., 2010, 107, 2213–2218 CrossRef CAS PubMed.
- C.-Y. Lin, Y.-L. Wang, Y.-J. Chen, C.-T. Ho, Y.-H. Chi, L. Y. Chan, G.-W. Chen, H.-C. Hsu, D. W. Hwang, H.-C. Wu and S.-C. Hung, Nat. Biomed. Eng., 2022, 6, 1105–1117 CrossRef CAS PubMed.
- Y. Nomura, V. Sharma, A. Yamamura and Y. Yokobayashi, Biotechnol. Lett., 2011, 33, 1069–1073 CrossRef CAS PubMed.
- L. Ma, T. Yang, M. Zhai, M. Yang and C. Mao, J. Mater. Chem. B, 2020, 8, 5189–5194 RSC.
- S. Swaminathan and Y. Cui, RSC Adv., 2016, 6, 14589–14592 RSC.
- H. Matsuno, J. Sekine, H. Yajima and T. Serizawa, Langmuir, 2008, 24, 6399–6403 CrossRef CAS PubMed.
- T. Serizawa, T. Sawada, H. Matsuno, T. Matsubara and T. Sato, J. Am. Chem. Soc., 2005, 127, 13780–13781 CrossRef CAS PubMed.
- Y. Wang, Y. Yu, L. Zhang, P. Qin and P. Wang, J. Biomater. Sci., Polym. Ed., 2015, 26, 459–467 CrossRef CAS PubMed.
- Y. Kumada, Y. Tokunaga, H. Imanaka, K. Imamura, T. Sakiyama, S. Katoh and K. Nakanishi, Biotechnol. Prog., 2006, 22, 401–405 CrossRef CAS PubMed.
- X. Qiang, K. Sun, L. Xing, Y. Xu, H. Wang, Z. Zhou, J. Zhang, F. Zhang, B. Caliskan, M. Wang and Z. Qiu, Sci. Rep., 2017, 7, 2673 CrossRef PubMed.
- N. B. Adey, A. H. Mataragnon, J. E. Rider, J. M. Carter and B. K. Kay, Gene, 1995, 156, 27–31 CrossRef CAS PubMed.
- B. Feng, Y. Dai, L. Wang, N. Tao, S. Huang and H. Zeng, Biologicals, 2009, 37, 48–54 CrossRef CAS PubMed.
- C. Juds, J. Schmidt, M. G. Weller, T. Lange, U. Beck, T. Conrad and H. G. Börner, J. Am. Chem. Soc., 2020, 142, 10624–10628 CrossRef CAS PubMed.
- H. Ikemoto, P. Lingasamy, A. M. Anton Willmore, H. Hunt, K. Kurm, O. Tammik, P. Scodeller, L. Simón-Gracia, V. R. Kotamraju, A. M. Lowy, K. N. Sugahara and T. Teesalu, Tumour Biol., 2017, 39, 1010428317701628 Search PubMed.
- Y. Tang, H. K. Bisoyi, X.-M. Chen, Z. Liu, X. Chen, S. Zhang and Q. Li, Adv. Mater., 2023, 35, 2300232 CrossRef CAS PubMed.
- M. C. Weiger, J. J. Park, M. D. Roy, C. M. Stafford, A. Karim and M. L. Becker, Biomaterials, 2010, 31, 2955–2963 CrossRef CAS PubMed.
- T. Duanis-Assaf, T. Hu, M. Lavie, Z. Zhang and M. Reches, Langmuir, 2022, 38, 968–978 CrossRef CAS PubMed.
- R. Plowright, D. J. Belton, D. L. Kaplan and C. C. Perry, Sci. Rep., 2017, 7, 7681 CrossRef PubMed.
- W. N. Addison, S. J. Miller, J. Ramaswamy, A. Mansouri, D. H. Kohn and M. D. McKee, Biomaterials, 2010, 31, 9422–9430 CrossRef CAS PubMed.
- A. M. Piggott and P. Karuso, Nat. Prod. Rep., 2016, 33, 626–636 RSC.
- Y. Li, B. Cao, S. Modali, E. M. Y. Lee, H. Xu, V. Petrenko, J. J. Gray, M. Yang and C. Mao, Mater. Today Adv., 2022, 15, 100263 CrossRef CAS.
- A. G. Christy, Cryst. Growth Des., 2017, 17, 3567–3578 CrossRef CAS.
- C. Li, G. D. Botsaris and D. L. Kaplan, Cryst. Growth Des., 2002, 2, 387–393 CrossRef CAS.
- A. Polasa, I. Mosleh, J. Losey, A. Abbaspourrad, R. Beitle and M. Moradi, Nanoscale Adv., 2022, 4, 3161–3171 RSC.
-
M. C. Hacker, J. Krieghoff and A. G. Mikos, in Principles of Regenerative Medicine, ed. A. Atala, R. Lanza, A. G. Mikos and R. Nerem, Academic Press, Boston, 3rd edn, 2019, pp. 559–590 Search PubMed.
- C. Englert, J. C. Brendel, T. C. Majdanski, T. Yildirim, S. Schubert, M. Gottschaldt, N. Windhab and U. S. Schubert, Prog. Polym. Sci., 2018, 87, 107–164 CrossRef CAS.
- H. Anni, O. Nikolaeva and Y. Israel, Alcohol, 2001, 25, 201–209 CrossRef CAS PubMed.
- K. Gebhardt, V. Lauvrak, E. Babaie, V. G. H. Eijsink and B. H. Lindqvist, Pept. Res., 1996, 9(6), 269–278 CAS.
- T. Serizawa, P. Techawanitchai and H. Matsuno, ChemBioChem, 2007, 8, 989–993 CrossRef CAS PubMed.
- T. Serizawa, T. Sawada and T. Kitayama, Angew. Chem., Int. Ed., 2007, 46, 723–726 CrossRef CAS PubMed.
- E. Y. Kramarenko, R. G. Winkler, P. G. Khalatur, A. R. Khokhlov and P. Reineker, J. Chem. Phys., 1996, 104, 4806–4813 CrossRef CAS.
- C. F. Fan and T. Caǧin, J. Chem. Phys., 1995, 103, 9053–9061 CrossRef CAS.
- A. Lamiable, P. Thévenet, J. Rey, M. Vavrusa, P. Derreumaux and P. Tufféry, Nucleic Acids Res., 2016, 44, W449–454 CrossRef CAS PubMed.
- J. Maupetit, P. Derreumaux and P. Tuffery, Nucleic Acids Res., 2009, 37, W498–W503 CrossRef CAS PubMed.
- A. D. Mackerell Jr., J. Comput. Chem., 2004, 25, 1584–1604 CrossRef PubMed.
- N. Karasawa, S. Dasgupta and W. A. Goddard, III, J. Phys. Chem., 1991, 95, 2260–2272 CrossRef CAS.
- A. V. Bandura and J. D. Kubicki, J. Phys. Chem. B, 2003, 107, 11072–11081 CrossRef CAS.
- C. A. López, Z. Sovova, F. J. van Eerden, A. H. de Vries and S. J. Marrink, J. Chem. Theory Comput., 2013, 9, 1694–1708 CrossRef PubMed.
- J. A. Maier, C. Martinez, K. Kasavajhala, L. Wickstrom, K. E. Hauser and C. Simmerling, J. Chem. Theory Comput., 2015, 11, 3696–3713 CrossRef CAS PubMed.
- K. Lindorff-Larsen, S. Piana, K. Palmo, P. Maragakis, J. L. Klepeis, R. O. Dror and D. E. Shaw, Proteins: Struct., Funct., Bioinf., 2010, 78, 1950–1958 CrossRef CAS PubMed.
- J. Huang and A. D. MacKerell, Curr. Opin. Struct. Biol., 2018, 48, 40–48 CrossRef CAS PubMed.
- P. Radivojac, W. T. Clark, T. R. Oron, A. M. Schnoes, T. Wittkop, A. Sokolov, K. Graim, C. Funk, K. Verspoor, A. Ben-Hur, G. Pandey, J. M. Yunes, A. S. Talwalkar, S. Repo, M. L. Souza, D. Piovesan, R. Casadio, Z. Wang, J. Cheng, H. Fang, J. Gough, P. Koskinen, P. Törönen, J. Nokso-Koivisto, L. Holm, D. Cozzetto, D. W. A. Buchan, K. Bryson, D. T. Jones, B. Limaye, H. Inamdar, A. Datta, S. K. Manjari, R. Joshi, M. Chitale, D. Kihara, A. M. Lisewski, S. Erdin, E. Venner, O. Lichtarge, R. Rentzsch, H. Yang, A. E. Romero, P. Bhat, A. Paccanaro, T. Hamp, R. Kaßner, S. Seemayer, E. Vicedo, C. Schaefer, D. Achten, F. Auer, A. Boehm, T. Braun, M. Hecht, M. Heron, P. Hönigschmid, T. A. Hopf, S. Kaufmann, M. Kiening, D. Krompass, C. Landerer, Y. Mahlich, M. Roos, J. Björne, T. Salakoski, A. Wong, H. Shatkay, F. Gatzmann, I. Sommer, M. N. Wass, M.
J. E. Sternberg, N. Škunca, F. Supek, M. Bošnjak, P. Panov, S. Džeroski, T. Šmuc, Y. A. I. Kourmpetis, A. D. J. van Dijk, C. J. F. T. Braak, Y. Zhou, Q. Gong, X. Dong, W. Tian, M. Falda, P. Fontana, E. Lavezzo, B. Di Camillo, S. Toppo, L. Lan, N. Djuric, Y. Guo, S. Vucetic, A. Bairoch, M. Linial, P. C. Babbitt, S. E. Brenner, C. Orengo, B. Rost, S. D. Mooney and I. Friedberg, Nat. Methods, 2013, 10, 221–227 CrossRef CAS PubMed.
- D. S. Marks, T. A. Hopf and C. Sander, Nat. Biotechnol., 2012, 30, 1072–1080 CrossRef CAS PubMed.
- A. W. Senior, R. Evans, J. Jumper, J. Kirkpatrick, L. Sifre, T. Green, C. Qin, A. Žídek, A. W. R. Nelson, A. Bridgland, H. Penedones, S. Petersen, K. Simonyan, S. Crossan, P. Kohli, D. T. Jones, D. Silver, K. Kavukcuoglu and D. Hassabis, Proteins: Struct., Funct., Bioinf., 2019, 87, 1141–1148 CrossRef CAS PubMed.
- M. L. Hekkelman, I. de Vries, R. P. Joosten and A. Perrakis, Nat. Methods, 2023, 20, 205–213 CrossRef CAS PubMed.
-
X. Zhang, in Encyclopedia of Machine Learning and Data Mining, ed. C. Sammut and G. I. Webb, Springer US, Boston, MA, 2017, pp. 1214–1220 DOI:10.1007/978-1-4899-7687-1_810.
- A. Motmaen, J. Dauparas, M. Baek, M. H. Abedi, D. Baker and P. Bradley, Proc. Natl. Acad. Sci. U. S. A., 2023, 120, e2216697120 CrossRef CAS PubMed.
- N. Li, J. Kang, L. Jiang, B. He, H. Lin and J. Huang, BioMed Res. Int., 2017, 2017, 5761517 Search PubMed.
- C. Meng, Y. Hu, Y. Zhang and F. Guo, Front. Bioeng. Biotechnol., 2020, 8, 245 CrossRef PubMed.
- G. P. Smith and V. A. Petrenko, Chem. Rev., 1997, 97, 391–410 CrossRef CAS PubMed.
- F. Noé, A. Tkatchenko, K.-R. Müller and C. Clementi, Annu. Rev. Phys. Chem., 2020, 71, 361–390 CrossRef PubMed.
- R. M. Cichy and D. Kaiser, Trends Cognit. Sci., 2019, 23, 305–317 CrossRef PubMed.
- V. P. Raut, M. A. Agashe, S. J. Stuart and R. A. Latour, Langmuir, 2005, 21(4), 1629–1639 CrossRef CAS PubMed.
- T. Iwasaki, M. Maruyama, T. Niwa, T. Sawada and T. Serizawa, Polym. J., 2021, 53, 1439–1449 CrossRef CAS.
- J. Dittrich, C. Brethauer, L. Goncharenko, J. Bührmann, V. Zeisler-Diehl, S. Pariyar, F. Jakob, T. Kurkina, L. Schreiber, U. Schwaneberg and H. Gohlke, ACS Appl. Mater. Interfaces, 2022, 14, 28412–28426 CrossRef CAS PubMed.
- K. Ding and H. C. Andersen, Phys. Rev. B: Condens. Matter Mater. Phys., 1986, 34, 6987–6991 CrossRef CAS PubMed.
- G. Gutiérrez and B. Johansson, Phys. Rev. B: Condens. Matter Mater. Phys., 2002, 65, 104202 CrossRef.
- W. D. Luedtke and U. Landman, Phys. Rev. B: Condens. Matter Mater. Phys., 1988, 37, 4656–4663 CrossRef CAS PubMed.
- D. Hossain, M. A. Tschopp, D. K. Ward, J. L. Bouvard, P. Wang and M. F. Horstemeyer, Polymer, 2010, 51, 6071–6083 CrossRef CAS.
- Z. E. Hughes and T. R. Walsh, J. Mater. Chem. B, 2015, 3, 3211–3221 RSC.
- Z. E. Hughes, M. A. Nguyen, J. Wang, Y. Liu, M. T. Swihart, M. Poloczek, P. I. Frazier, M. R. Knecht and T. R. Walsh, ACS Nano, 2021, 15, 18260–18269 CrossRef CAS PubMed.
- D. B. Pacardo, M. Sethi, S. E. Jones, R. R. Naik and M. R. Knecht, ACS Nano, 2009, 3, 1288–1296 CrossRef CAS PubMed.
- R. R. Naik, S. J. Stringer, G. Agarwal, S. E. Jones and M. O. Stone, Nat. Mater., 2002, 1, 169–172 CrossRef CAS PubMed.
- M. Hnilova, E. E. Oren, U. O. S. Seker, B. R. Wilson, S. Collino, J. S. Evans, C. Tamerler and M. Sarikaya, Langmuir, 2008, 24, 12440–12445 CrossRef CAS PubMed.
- M. Tanaka, S. Hikiba, K. Yamashita, M. Muto and M. Okochi, Acta Biomater., 2017, 49, 495–506 CrossRef CAS PubMed.
- J. I. B. Janairo, ACS Omega, 2022, 7, 14069–14073 CrossRef CAS PubMed.
- J. P. Palafox-Hernandez, Z. Tang, Z. E. Hughes, Y. Li, M. T. Swihart, P. N. Prasad, T. R. Walsh and M. R. Knecht, Chem. Mater., 2014, 26, 4960–4969 CrossRef CAS.
- Z. Tang, J. P. Palafox-Hernandez, W.-C. Law, Z. E. Hughes, M. T. Swihart, P. N. Prasad, M. R. Knecht and T. R. Walsh, ACS Nano, 2013, 7, 9632–9646 CrossRef CAS PubMed.
- A. V. Verde, J. M. Acres and J. K. Maranas, Biomacromolecules, 2009, 10, 2118–2128 CrossRef PubMed.
- T. Lou, X. Bai, X. He, Y. Yang and C. Yuan, Prog. Org. Coat., 2021, 157, 106310 CrossRef CAS.
- E. G. Brandt and A. P. Lyubartsev, J. Phys. Chem. C, 2015, 119, 18126–18139 CrossRef CAS.
- J. Sampath, A. Kullman, R. Gebhart, G. Drobny and J. Pfaendtner, npj Comput. Mater., 2020, 6, 34 CrossRef CAS.
- N. Kantarci, C. Tamerler, M. Sarikaya, T. Haliloglu and P. Doruker, Polymer, 2005, 46, 4307–4313 CrossRef CAS.
- T. Sakiyama, K. Tanino, M. Urakawa, K. Imamura, T. Takahashi, T. Nagai and K. Nakanishi, J. Biosci. Bioeng., 1999, 88, 536–541 CrossRef CAS PubMed.
- J. J. Gray, Curr. Opin. Struct. Biol., 2004, 14, 110–115 CrossRef CAS PubMed.
- L. B. Wright and T. R. Walsh, Phys. Chem. Chem. Phys., 2013, 15, 4715–4726 RSC.
- T. Duanis-Assaf, T. Hu, M. Lavie, Z. Zhang and M. Reches, Langmuir, 2022, 38, 968–978 CrossRef CAS PubMed.
- R. C. Bernardi, M. C. R. Melo and K. Schulten, Biochim. Biophys. Acta, Gen. Subj., 2015, 1850, 872–877 CrossRef CAS PubMed.
- R. E. Rudd and J. Q. Broughton, Phys. Rev. B: Condens. Matter Mater. Phys., 1998, 58, R5893–R5896 CrossRef CAS.
- H.-p Zhang, X. Lu, Y. Leng, F. Watari, J. Weng, B. Feng and S. Qu, J. Biomed. Mater. Res., Part A, 2011, 96A, 466–476 CrossRef CAS PubMed.
-
N. E. Siedhoff, U. Schwaneberg and M. D. Davari, in Methods in Enzymology, ed. D. S. Tawfik, Academic Press, 2020, vol. 643, pp. 281–315 Search PubMed.
- T. Dietterich, ACM Comput. Surv., 1995, 27, 326–327 CrossRef.
- X. Ying, J. Phys.: Conf. Ser., 2019, 1168, 022022 CrossRef.
-
H. K. Jabbar and R. Z. Khan, 2014.
- S. Biswas, Mesopotamian J. Comput. Sci., 2023, 2023, 8–16 Search PubMed.
- S. Mazurenko, Z. Prokop and J. Damborsky, ACS Catal., 2020, 10, 1210–1223 CrossRef CAS.
- A. Brachner, D. Fragouli, I. F. Duarte, P. M. A. Farias, S. Dembski, M. Ghosh, I. Barisic, D. Zdzieblo, J. Vanoirbeek, P. Schwabl and W. Neuhaus, Int. J. Environ. Res. Public Health, 2020, 17, 8832 CrossRef CAS PubMed.
- R. C. Thompson, Y. Olsen, R. P. Mitchell, A. Davis, S. J. Rowland, A. W. G. John, D. McGonigle and A. E. Russell, Science, 2004, 304, 838 CrossRef CAS PubMed.
- A. Ter Halle, L. Jeanneau, M. Martignac, E. Jardé, B. Pedrono, L. Brach and J. Gigault, Environ. Sci. Technol., 2017, 51, 13689–13697 CrossRef CAS PubMed.
- I. Goßmann, R. Süßmuth and B. M. Scholz-Böttcher, Sci. Total Environ., 2022, 832, 155008 CrossRef PubMed.
- C. Vitali, R. J. B. Peters, H.-G. Janssen and M. W. F. Nielen, TrAC, Trends Anal. Chem., 2023, 159, 116670 CrossRef CAS.
- S. Afrin, M. M. Rahman, M. A. Akbor, M. A. B. Siddique, M. K. Uddin and G. Malafaia, Sci. Total Environ., 2022, 837, 155833 CrossRef CAS PubMed.
-
E. C. Agency, 2020.
- H. A. Leslie, M. J. M. van Velzen, S. H. Brandsma, A. D. Vethaak, J. J. Garcia-Vallejo and M. H. Lamoree, Environ. Int., 2022, 163, 107199 CrossRef CAS PubMed.
- Y. Mato, T. Isobe, H. Takada, H. Kanehiro, C. Ohtake and T. Kaminuma, Environ. Sci. Technol., 2001, 35, 318–324 CrossRef CAS PubMed.
- C. Schwaferts, R. Niessner, M. Elsner and N. P. Ivleva, TrAC, Trends Anal. Chem., 2019, 112, 52–65 CrossRef CAS.
- F. Caputo, R. Vogel, J. Savage, G. Vella, A. Law, G. Della Camera, G. Hannon, B. Peacock, D. Mehn, J. Ponti, O. Geiss, D. Aubert, A. Prina-Mello and L. Calzolai, J. Colloid Interface Sci., 2021, 588, 401–417 CrossRef CAS PubMed.
- X. Tian, F. Beén and P. S. Bäuerlein, Environ. Res., 2022, 212, 113569 CrossRef CAS PubMed.
- J. Ao, G. Xu, H. Wu, L. Xie, J. Liu, K. Gong, X. Ruan, J. Han, K. Li, W. Wang, T. Chen, M. Ji and L. Zhang, Cell Rep. Phys. Sci., 2023, 4, 101623 CrossRef CAS.
- M. Wang, Z. Huang, C. Wu, S. Yan, H.-T. Fang, W. Pan, Q.-G. Tan, K. Pan, R. Ji, L. Yang, B. Pan, P. Wang and A.-J. Miao, Environ. Sci. Technol., 2024, 58, 2922–2930 CrossRef CAS PubMed.
- C. J. Hirschmugl and K. M. Gough, Appl. Spectrosc., 2012, 66, 475–491 CrossRef CAS PubMed.
- M. González-Pleiter, D. Velázquez, C. Edo, O. Carretero, J. Gago, Á. Barón-Sola, L. E. Hernández, I. Yousef, A. Quesada, F. Leganés, R. Rosal and F. Fernández-Piñas, Sci. Total Environ., 2020, 722, 137904 CrossRef PubMed.
- R. Gillibert, G. Balakrishnan, Q. Deshoules, M. Tardivel, A. Magazzù, M. G. Donato, O. M. Maragò, M. Lamy de La Chapelle, F. Colas, F. Lagarde and P. G. Gucciardi, Environ. Sci. Technol., 2019, 53, 9003–9013 CrossRef CAS PubMed.
- I. C. ten Have, A. J. A. Duijndam, R. Oord, H. J. M. van Berlo-van den Broek, I. Vollmer, B. M. Weckhuysen and F. Meirer, Chem.: Methods, 2021, 1, 205–209 Search PubMed.
- X. Tian, H. Jiang, L. Hu, M. Wang, W. Cui, J. Shi, G. Liu, Y. Yin, Y. Cai and G. Jiang, TrAC, Trends Anal. Chem., 2022, 157, 116746 CrossRef CAS.
- L. Pitkänen and A. M. Striegel, TrAC, Trends Anal. Chem., 2016, 80, 311–320 CrossRef PubMed.
- M. Cerasa, S. Teodori and L. Pietrelli, Polymers, 2021, 13, 3658 CrossRef CAS PubMed.
- F. Caputo, D. Mehn, J. D. Clogston, M. Rösslein, A. Prina-Mello, S. E. Borgos, S. Gioria and L. Calzolai, J. Chromatogr. A, 2021, 1635, 461767 CrossRef CAS PubMed.
-
M. E. Schimpf, K. Caldwell and J. C. Giddings, Field-flow fractionation handbook, John Wiley & Sons, 2000 Search PubMed.
- A. Barber, S. Kly, M. G. Moffitt, L. Rand and J. F. Ranville, Environ. Sci.: Nano, 2020, 7, 514–524 RSC.
- Y. Hu, R. M. Crist and J. D. Clogston, Anal. Bioanal. Chem., 2020, 412, 425–438 CrossRef CAS PubMed.
- M. Bagheri, M. H. Fens, T. G. Kleijn, R. B. Capomaccio, D. Mehn, P. M. Krawczyk, E. M. Scutigliani, A. Gurinov, M. Baldus, N. C. H. van Kronenburg, R. J. Kok, M. Heger, C. F. van Nostrum and W. E. Hennink, Mol. Pharmaceutics, 2021, 18, 1247–1263 CrossRef CAS PubMed.
- J. Gigault, H. El Hadri, S. Reynaud, E. Deniau and B. Grassl, Anal. Bioanal. Chem., 2017, 409, 6761–6769 CrossRef CAS PubMed.
- C. Schwaferts, V. Sogne, R. Welz, F. Meier, T. Klein, R. Niessner, M. Elsner and N. P. Ivleva, Anal. Chem., 2020, 92, 5813–5820 CrossRef CAS PubMed.
- M. Correia and K. Loeschner, Anal. Bioanal. Chem., 2018, 410, 5603–5615 CrossRef CAS PubMed.
- S. M. Mintenig, P. S. Bäuerlein, A. A. Koelmans, S. C. Dekker and A. P. van Wezel, Environ. Sci.:
Nano, 2018, 5, 1640–1649 RSC.
- A. Valsesia, J. Parot, J. Ponti, D. Mehn, R. Marino, D. Melillo, S. Muramoto, M. Verkouteren, V. A. Hackley and P. Colpo, Microplast. Nanoplast., 2021, 1, 5 CrossRef.
- A. Wahl, C. Le Juge, M. Davranche, H. El Hadri, B. Grassl, S. Reynaud and J. Gigault, Chemosphere, 2021, 262, 127784 CrossRef CAS PubMed.
- J. Bian, N. Gobalasingham, A. Purchel and J. Lin, Molecules, 2023, 28, 4169 CrossRef CAS PubMed.
- P. Li, Q. Li, Z. Hao, S. Yu and J. Liu, J. Environ. Sci., 2020, 94, 88–99 CrossRef CAS PubMed.
- G. Lespes and V. De Carsalade Du Pont, J. Sep. Sci., 2022, 45, 347–368 CrossRef CAS PubMed.
- L. Lv, J. Qu, Z. Yu, D. Chen, C. Zhou, P. Hong, S. Sun and C. Li, Environ. Pollut., 2019, 255, 113283 CrossRef CAS PubMed.
- M. T. Sturm, H. Horn and K. Schuhen, Anal. Bioanal. Chem., 2021, 413, 1059–1071 CrossRef CAS PubMed.
- H. Aoki, Sensors, 2022, 22, 3390 CrossRef CAS PubMed.
- W. Bauten, M. Nöth, T. Kurkina, F. Contreras, Y. Ji, C. Desmet, M.-Á. Serra, D. Gilliland and U. Schwaneberg, Sci. Total Environ., 2023, 860, 160450 CrossRef CAS PubMed.
- G. Sauerbrey, Z. Phys., 1959, 155, 206–222 CrossRef CAS.
- C. P. McNew, N. Kananizadeh, Y. Li and E. J. LeBoeuf, Chemosphere, 2017, 168, 65–79 CrossRef CAS PubMed.
- M. Shams, I. Alam and I. Chowdhury, Water Res., 2021, 197, 117066 CrossRef CAS PubMed.
- L. Liu, J. Song, M. Zhang and W. Jiang, Bull. Environ. Contam. Toxicol., 2021, 107, 741–747 CrossRef CAS PubMed.
- Y. Xu, Q. Ou, Q. He, Z. Wu, J. Ma and X. Huangfu, Water Res., 2021, 196, 117054 CrossRef CAS PubMed.
- L. He, H. Rong, D. Wu, M. Li, C. Wang and M. Tong, Water Res., 2020, 178, 115808 CrossRef CAS PubMed.
- I. R. Quevedo, A. L. J. Olsson and N. Tufenkji, Environ. Sci. Technol., 2013, 47, 2212–2220 CrossRef CAS PubMed.
- T. Serizawa, S. Kamimura and M. Akashi, Colloids Surf., A, 2000, 164, 237–245 CrossRef CAS.
- T. Serizawa, H. Takeshita and M. Akashi, Langmuir, 1998, 14, 4088–4094 CrossRef CAS.
- C.-J. Huang, G. V. Narasimha, Y.-C. Chen, J.-K. Chen and G.-C. Dong, Biosensors, 2021, 11, 219 CrossRef CAS PubMed.
- J. Tuoriniemi, B. Moreira and G. Safina, Langmuir, 2016, 32, 10632–10640 CrossRef CAS PubMed.
- S. Oh, H. Hur, Y. Kim, S. Shin, H. Woo, J. Choi and H. H. Lee, Nanomaterials, 2021, 11, 2887 CrossRef CAS PubMed.
- C. Desmet, A. Valsesia, A. Oddo, G. Ceccone, V. Spampinato, F. Rossi and P. Colpo, J. Nanopart. Res., 2017, 19, 117 CrossRef PubMed.
- C. Veclin, C. Desmet, A. Pradel, A. Valsesia, J. Ponti, H. El Hadri, T. Maupas, V. Pellerin, J. Gigault, B. Grassl and S. Reynaud, ACS ES&T Water, 2022, 2, 88–95 Search PubMed.
- A. Pradel, H. e Hadri, C. Desmet, J. Ponti, S. Reynaud, B. Grassl and J. Gigault, Chemosphere, 2020, 255, 126912 CrossRef CAS PubMed.
- T. Maes, R. Jessop, N. Wellner, K. Haupt and A. G. Mayes, Sci. Rep., 2017, 7, 44501 CrossRef CAS PubMed.
- V. C. Shruti, F. Pérez-Guevara, P. D. Roy and G. Kutralam-Muniasamy, J. Hazard. Mater., 2022, 423, 127171 CrossRef CAS PubMed.
- H. Woo, S. H. Kang, Y. Kwon, Y. Choi, J. Kim, D.-H. Ha, M. Tanaka, M. Okochi, J. S. Kim, H. K. Kim and J. Choi, RSC Adv., 2022, 12, 7680–7688 RSC.
- M. Lavoy and J. Crossman, Environ. Pollut., 2021, 286, 117357 CrossRef CAS PubMed.
- R. R. Hurley, A. L. Lusher, M. Olsen and L. Nizzetto, Environ. Sci. Technol., 2018, 52, 7409–7417 CrossRef CAS PubMed.
- M. M. Maw, N. Boontanon, S. Fujii and S. K. Boontanon, Sci. Total Environ., 2022, 853, 158642 CrossRef CAS PubMed.
- J. Zhao, Y. Ruan, Z. Zheng, Y. Li, M. Sohail, F. Hu, J. Ling and L. Zhang, iScience, 2023, 26, 106823 CrossRef CAS PubMed.
- S. Oh, H. Hur, Y. Kim, S. Shin, H. Woo, J. Choi and H. H. Lee, Nanomaterials, 2021, 11, 2887 CrossRef CAS PubMed.
-
A. Care, P. L. Bergquist and A. Sunna, in Peptides and Peptide-based Biomaterials and their Biomedical Applications, ed. A. Sunna, A. Care and P. L. Bergquist, Springer International Publishing, Cham, 2017, pp. 21–36 DOI:10.1007/978-3-319-66095-0_2.
- S. Pandey, G. Malviya and M. Chottova Dvorakova, Int. J. Mol. Sci., 2021, 22, 8828 CrossRef CAS PubMed.
- A. R. Grimm, D. F. Sauer, T. Mirzaei Garakani, K. Rubsam, T. Polen, M. D. Davari, F. Jakob, J. Schiffels, J. Okuda and U. Schwaneberg, Bioconjugate Chem., 2019, 30, 714–720 CrossRef CAS PubMed.
- N. Büscher, G. V. Sayoga, K. Rübsam, F. Jakob, U. Schwaneberg, S. Kara and A. Liese, Org. Process Res. Dev., 2019, 23, 1852–1859 CrossRef.
- Z. Liu, Y. Zhang and J. Wu, Enzyme Microb. Technol., 2022, 156, 110004 CrossRef CAS PubMed.
- Z. Zou, D. M. Mate, K. Rubsam, F. Jakob and U. Schwaneberg, ACS Comb. Sci., 2018, 20, 203–211 CrossRef CAS PubMed.
- Z. Zou, Y. Ji and U. Schwaneberg, Angew. Chem., Int. Ed., 2024, 63, e202310910 CrossRef CAS PubMed.
- S. Islam, L. Apitius, F. Jakob and U. Schwaneberg, Environ. Int., 2019, 123, 428–435 CrossRef CAS PubMed.
- S. Zernia, F. Ott, K. Bellmann-Sickert, R. Frank, M. Klenner, H.-G. Jahnke, A. Prager, B. Abel, A. Robitzki and A. G. Beck-Sickinger, Bioconjugate Chem., 2016, 27, 1090–1097 CrossRef CAS PubMed.
- D. T. Yucesoy, S. Akkineni, C. Tamerler, B. J. Hinds and M. Sarikaya, ACS Omega, 2021, 6, 27129–27139 CrossRef CAS PubMed.
- G. M. Rios, M. P. Belleville, D. Paolucci and J. Sanchez, J. Membr. Sci., 2004, 242, 189–196 CrossRef CAS.
- A. R. Grimm, D. F. Sauer, T. Polen, L. Zhu, T. Hayashi, J. Okuda and U. Schwaneberg, ACS Catal., 2018, 8, 2611–2614 CrossRef CAS.
- N. Lülsdorf, C. Pitzler, M. Biggel, R. Martinez, L. Vojcic and U. Schwaneberg, Chem. Commun., 2015, 51, 8679–8682 RSC.
- M. Garay-Sarmiento, L. Witzdam, M. Vorobii, C. Simons, N. Herrmann, A. De Los Santos Pereira, E. Heine, I. El-Awaad, R. Lütticken, F. Jakob, U. Schwaneberg and C. Rodriguez-Emmenegger, Adv. Funct. Mater., 2021, 32, 2106656 CrossRef.
- P. Winnersbach, A. Hosseinnejad, T. Breuer, T. Fechter, F. Jakob, U. Schwaneberg, R. Rossaint, C. Bleilevens and S. Singh, Membranes, 2022, 12, 73 CrossRef CAS PubMed.
- D. B. Gehlen, L. C. De Lencastre Novaes, W. Long, A. J. Ruff, F. Jakob, T. Haraszti, Y. Chandorkar, L. Yang, P. van Rijn, U. Schwaneberg and L. De Laporte, ACS Appl. Mater. Interfaces, 2019, 11, 41091–41099 CrossRef CAS PubMed.
- K. W. Hintzen, C. Simons, K. Schaffrath, G. Roessler, S. Johnen, F. Jakob, P. Walter, U. Schwaneberg and T. Lohmann, Biomater. Sci., 2022, 10, 3282–3295 RSC.
- L. Apitius, S. Buschmann, C. Bergs, D. Schönauer, F. Jakob, A. Pich and U. Schwaneberg, Macromol. Biosci., 2019, 19, 1900125 CrossRef PubMed.
- R. A. Meurer, S. Kemper, S. Knopp, T. Eichert, F. Jakob, H. E. Goldbach, U. Schwaneberg and A. Pich, Angew. Chem., Int. Ed., 2017, 56, 7380–7386 CrossRef CAS PubMed.
- U. Conrath, G. J. M. Beckers, C. J. G. Langenbach and M. R. Jaskiewicz, Annu. Rev. Phytopathol., 2015, 53, 97–119 CrossRef CAS PubMed.
- J. Dittrich, C. Brethauer, L. Goncharenko, J. Buhrmann, V. Zeisler-Diehl, S. Pariyar, F. Jakob, T. Kurkina, L. Schreiber, U. Schwaneberg and H. Gohlke, ACS Appl. Mater. Interfaces, 2022, 14, 28412–28426 CrossRef CAS PubMed.
- R. A. Meurer, S. Kemper, S. Knopp, T. Eichert, F. Jakob, H. E. Goldbach, U. Schwaneberg and A. Pich, Angew. Chem., Int. Ed., 2017, 56, 7380–7386 CrossRef CAS PubMed.
- P. Schwinges, S. Pariyar, F. Jakob, M. Rahimi, L. Apitius, M. Hunsche, L. Schmitt, G. Noga, C. Langenbach, U. Schwaneberg and U. Conrath, Green Chem., 2019, 21, 2316–2325 RSC.
- S. Pramanik, F. Contreras, M. D. Davari and U. Schwaneberg, Protein Eng., 2021, 153–176 Search PubMed.
- U. G. K. Wegst, H. Bai, E. Saiz, A. P. Tomsia and R. O. Ritchie, Nat. Mater., 2015, 14, 23–36 CrossRef CAS PubMed.
- L. A. Estroff, Chem. Rev., 2008, 108, 4329–4331 CrossRef CAS PubMed.
- B. J. G. E. Pieters, M. B. van Eldijk, R. J. M. Nolte and J. Mecinović, Chem. Soc. Rev., 2016, 45, 24–39 RSC.
- I. Firkowska-Boden, X. Zhang and K. D. Jandt, Adv. Healthcare Mater., 2018, 7, 1700995 CrossRef PubMed.
- A. A. Vertegel, R. W. Siegel and J. S. Dordick, Langmuir, 2004, 20, 6800–6807 CrossRef CAS PubMed.
- S. R. Whaley, D. S. English, E. L. Hu, P. F. Barbara and A. M. Belcher, Nature, 2000, 405, 665–668 CrossRef CAS PubMed.
- S. Dedisch, F. Obstals, A. Los Santos Pereira, M. Bruns, F. Jakob, U. Schwaneberg and C. Rodriguez-Emmenegger, Adv. Mater. Interfaces, 2019, 6, 1900847 CrossRef CAS.
- D. Soder, M. Garay-Sarmiento, K. Rahimi, F. Obstals, S. Dedisch, T. Haraszti, M. D. Davari, F. Jakob, C. Hess, U. Schwaneberg and C. Rodriguez-Emmenegger, Macromol. Biosci., 2021, 21, e2100158 CrossRef PubMed.
- J. T. Hirvi and T. A. Pakkanen, J. Chem. Phys., 2006, 125, 144712 CrossRef PubMed.
- M. T. Bergman, X. Xiao and C. K. Hall, J. Phys. Chem. B, 2023, 127, 8370–8381 CrossRef CAS PubMed.
- T. S. Wong, D. Zhurina and U. Schwaneberg, Comb. Chem. High Throughput Screening, 2006, 9, 271–288 CrossRef CAS PubMed.
- R. Verma, U. Schwaneberg and D. Roccatano, ACS Synth. Biol., 2012, 1, 139–150 CrossRef CAS PubMed.
- H. Cui, M. Vedder, L. Zhang, K.-E. Jaeger, U. Schwaneberg and M. D. Davari, ChemSusChem, 2022, 15, e202102551 CrossRef CAS PubMed.
-
H. Cui, M. Vedder, U. Schwaneberg and M. D. Davari, in Enzyme Engineering: Methods and Protocols, ed. F. Magnani, C. Marabelli and F. Paradisi, Springer US, New York, NY, 2022, pp. 179–202 DOI:10.1007/978-1-0716-1826-4_10.
- S. Pramanik, H. Cui, G. V. Dhoke, C. B. Yildiz, M. Vedder, K.-E. Jaeger, U. Schwaneberg and M. D. Davari, ACS Sustainable Chem. Eng., 2022, 10, 2689–2698 CrossRef CAS.
- S. Pramanik, G. V. Dhoke, K.-E. Jaeger, U. Schwaneberg and M. D. Davari, ACS Sustainable Chem. Eng., 2019, 7, 11293–11302 CrossRef CAS.
- J. L. Watson, D. Juergens, N. R. Bennett, B. L. Trippe, J. Yim, H. E. Eisenach, W. Ahern, A. J. Borst, R. J. Ragotte, L. F. Milles, B. I. M. Wicky, N. Hanikel, S. J. Pellock, A. Courbet, W. Sheffler, J. Wang, P. Venkatesh, I. Sappington, S. V. Torres, A. Lauko, V. De Bortoli, E. Mathieu, S. Ovchinnikov, R. Barzilay, T. S. Jaakkola, F. DiMaio, M. Baek and D. Baker, Nature, 2023, 620, 1089–1100 CrossRef CAS PubMed.
-
N. K. Arora, M. Verma, J. Prakash and J. Mishra, in Bioformulations: for Sustainable Agriculture, ed. N. K. Arora, S. Mehnaz and R. Balestrini, Springer India, New Delhi, 2016, pp. 283–299 DOI:10.1007/978-81-322-2779-3_16.
- J. Puetz and F. M. Wurm, Processes, 2019, 7, 476 CrossRef CAS.
- J. Tian, X. Song, Y. Wang, M. Cheng, S. Lu, W. Xu, G. Gao, L. Sun, Z. Tang, M. Wang and X. Zhang, Bioact. Mater., 2022, 10, 492–503 CAS.
- M. J. Paul, H. Thangaraj and J. K.-C. Ma, Plant Biotechnol. J., 2015, 13, 1209–1220 CrossRef CAS PubMed.
- Y. Wang, P. Xue, M. Cao, T. Yu, S. T. Lane and H. Zhao, Chem. Rev., 2021, 121, 12384–12444 CrossRef CAS PubMed.
- F. H. Arnold and A. A. Volkov, Curr. Opin. Chem. Biol., 1999, 3, 54–59 CrossRef CAS PubMed.
- C. Novoa, G. V. Dhoke, D. M. Mate, R. Martínez, T. Haarmann, M. Schreiter, J. Eidner, R. Schwerdtfeger, P. Lorenz, M. D. Davari, F. Jakob and U. Schwaneberg, ChemBioChem, 2019, 20, 1458–1466 CrossRef CAS PubMed.
- F. Contreras, M. J. Thiele, S. Pramanik, A. M. Rozhkova, A. S. Dotsenko, I. N. Zorov, A. P. Sinitsyn, M. D. Davari and U. Schwaneberg, ACS Sustainable Chem. Eng., 2020, 8, 12388–12399 CrossRef CAS.
- S. Brands, H. U. C. Brass, A. S. Klein, J. G. Sikkens, M. D. Davari, J. Pietruszka, A. J. Ruff and U. Schwaneberg, Catal. Sci. Technol., 2021, 11, 2805–2815 RSC.
- S. Islam, D. Laaf, B. Infanzón, H. Pelantová, M. D. Davari, F. Jakob, V. Křen, L. Elling and U. Schwaneberg, Chem. – Eur. J., 2018, 24, 17117–17124 CrossRef CAS PubMed.
- Y. Yang, J. Min, T. Xue, P. Jiang, X. Liu, R. Peng, J.-W. Huang, Y. Qu, X. Li, N. Ma, F.-C. Tsai, L. Dai, Q. Zhang, Y. Liu, C.-C. Chen and R.-T. Guo, Nat. Commun., 2023, 14, 1645 CrossRef CAS PubMed.
- B. Sui, T. Wang, J. Fang, Z. Hou, T. Shu, Z. Lu, F. Liu and Y. Zhu, Front. Microbiol., 2023, 14, 1265139 CrossRef PubMed.
- H. Cui, L. Zhang, D. Söder, X. Tang, M. D. Davari and U. Schwaneberg, ACS Catal., 2021, 11, 2445–2453 CrossRef CAS.
- J. Han, L. Xiong, X. Jiang, X. Yuan, Y. Zhao and D. Yang, Prog. Polym. Sci., 2019, 91, 1–28 CrossRef CAS.
- T. Hoang, H. Truong, J. Han, S. Lee, J. Lee, S. Parajuli, J. Lee and G. Cho, Mater. Today Bio, 2023, 23, 100838 CrossRef CAS PubMed.
- R. G. Ferreira, A. R. Azzoni and S. Freitas, Biofuels, Bioprod. Biorefin., 2021, 15, 85–99 CrossRef CAS.
- J. R. Cherry and A. L. Fidantsef, Curr. Opin. Biotechnol, 2003, 14, 438–443 CrossRef CAS PubMed.
- Y. S. Kim, H.-J. Lee, M.-H. Han, N.-K. Yoon, Y.-C. Kim and J. Ahn, Microb. Cell Fact., 2021, 20, 9 CrossRef CAS PubMed.
- W. Zhu, G. Gong, J. Pan, S. Han, W. Zhang, Y. Hu and L. Xie, Protein Expression Purif., 2018, 147, 61–68 CrossRef CAS PubMed.
- X. Cao, Y. Zhang, R. Mao, D. Teng, X. Wang and J. Wang, Appl. Microbiol. Biotechnol., 2015, 99, 2649–2662 CrossRef CAS PubMed.
- Y. R. Maghraby, R. M. El-Shabasy, A. H. Ibrahim and H. M. E.-S. Azzazy, ACS Omega, 2023, 8, 5184–5196 CrossRef CAS PubMed.
- T. R. Walsh and M. R. Knecht, Chem. Rev., 2017, 117, 12641–12704 CrossRef CAS PubMed.
- B. Hellner, S. Alamdari, H. Pyles, S. Zhang, A. Prakash, K. G. Sprenger, J. J. De Yoreo, D. Baker, J. Pfaendtner and F. Baneyx, J. Am. Chem. Soc., 2020, 142, 2355–2363 CrossRef CAS PubMed.
- A. D. Pye, D. E. A. Lockhart, M. P. Dawson, C. A. Murray and A. J. Smith, J. Hosp. Infect., 2009, 72, 104–110 CrossRef CAS PubMed.
- J. M. Schierholz and J. Beuth, J. Hosp. Infect., 2001, 49, 87–93 CrossRef CAS PubMed.
- E. Holaskova, P. Galuszka, I. Frebort and M. T. Oz, Biotechnol. Adv., 2015, 33, 1005–1023 CrossRef CAS PubMed.
- D. B. Gehlen, L. C. De Lencastre Novaes, W. Long, A. J. Ruff, F. Jakob, T. Haraszti, Y. Chandorkar, L. Yang, P. van Rijn, U. Schwaneberg and L. De Laporte, ACS Appl. Mater. Interfaces, 2019, 11, 41091–41099 CrossRef CAS PubMed.
- C. K. Schneider, P. Salmikangas, B. Jilma, B. Flamion, L. R. Todorova, A. Paphitou, I. Haunerova, T. Maimets, J.-H. Trouvin, E. Flory, A. Tsiftsoglou, B. Sarkadi, K. Gudmundsson, M. O'Donovan, G. Migliaccio, J. Ancāns, R. Mačiulaitis, J.-L. Robert, A. Samuel, J. H. Ovelgönne, M. Hystad, A. M. Fal, B. S. Lima, A. S. Moraru, P. Turčáni, R. Zorec, S. Ruiz, L. Åkerblom, G. Narayanan, A. Kent, F. Bignami, J. G. Dickson, D. Niederwieser, M.-A. Figuerola-Santos, I. G. Reischl, C. Beuneu, R. Georgiev, M. Vassiliou, A. Pychova, M. Clausen, T. Methuen, S. Lucas, M. Schüssler-Lenz, V. Kokkas, Z. Buzás, N. MacAleenan, M. C. Galli, A. Linē, J. Gulbinovic, G. Berchem, M. Frączek, M. Menezes-Ferreira, N. Vilceanu, M. Hrubiško, P. Marinko, M. Timón, W. Cheng, G. A. Crosbie, N. Meade, M. L. di Paola, T. VandenDriessche, P. Ljungman, L. D'Apote, O. Oliver-Diaz, I. Büttel and P. Celis, Nat. Rev. Drug Discovery, 2010, 9, 195–201 CrossRef CAS PubMed.
- J. P. da Costa, M. Cova, R. Ferreira and R. Vitorino, Appl. Microbiol. Biotechnol., 2015, 99, 2023–2040 CrossRef PubMed.
- H. Cai, E. G. Xu, F. Du, R. Li, J. Liu and H. Shi, Chem. Eng. J., 2021, 410, 128208 CrossRef CAS.
- A. Rafiq and J.-L. Xu, Waste Manage., 2023, 171, 54–70 CrossRef CAS PubMed.
- I. Azeem, N. Shakoor, S. Chaudhary, M. Adeel, M. Zain, M. A. Ahmad, Y. Li, G. Zhu, S. A. A. Shah, K. Khan, A. A. Khan, M. Xu and Y. Rui, Plant Physiol. Biochem., 2023, 204, 108132 CrossRef CAS PubMed.
- C. Zarfl, Anal. Bioanal. Chem., 2019, 411, 3743–3756 CrossRef CAS PubMed.
- N. P. Ivleva, Chem. Rev., 2021, 121, 11886–11936 CrossRef CAS PubMed.
- V. Kopatz, K. Wen, T. Kovács, A. S. Keimowitz, V. Pichler, J. Widder, A. D. Vethaak, O. Hollóczki and L. Kenner, Nanomaterials, 2023, 13, 1404 CrossRef CAS PubMed.
- M. Prüst, J. Meijer and R. H. S. Westerink, Part. Fibre Toxicol., 2020, 17, 24 CrossRef PubMed.
- S. Shan, Y. Zhang, H. Zhao, T. Zeng and X. Zhao, Chemosphere, 2022, 298, 134261 CrossRef CAS PubMed.
- Z. Liu, A. Sokratian, A. M. Duda, E. Xu, C. Stanhope, A. Fu, S. Strader, H. Li, Y. Yuan, B. G. Bobay, J. Sipe, K. Bai, I. Lundgaard, N. Liu, B. Hernandez, C. Bowes Rickman, S. E. Miller and A. B. West, Sci. Adv., 2023, 9, eadi8716 CrossRef CAS PubMed.
- A. Binelli, C. Della Torre, L. Nigro, N. Riccardi and S. Magni, Sci. Total Environ., 2022, 806, 150574 CrossRef CAS PubMed.
- M. Cole, Sci. Rep., 2016, 6, 34519 CrossRef CAS PubMed.
- D. Magrì, P. Sánchez-Moreno, G. Caputo, F. Gatto, M. Veronesi, G. Bardi, T. Catelani, D. Guarnieri, A. Athanassiou, P. P. Pompa and D. Fragouli, ACS Nano, 2018, 12, 7690–7700 CrossRef PubMed.
- K. Tanaka, Y. Takahashi, H. Kuramochi, M. Osako, S. Tanaka and G. Suzuki, Small, 2021, 17, 2105781 CrossRef CAS PubMed.
- M. Tamayo-Belda, C. Venâncio, F. Fernandez-Piñas, R. Rosal, I. Lopes and M. Oliveira, Sci. Total Environ., 2023, 883, 163447 CrossRef CAS PubMed.
- J. R. Hunter, Q. Qiao, Y. Zhang, Q. Shao, C. Crofcheck and J. Shi, Sci. Rep., 2023, 13, 10585 CrossRef CAS PubMed.
Footnote |
† Authors contributed equally to the work. |
|
This journal is © The Royal Society of Chemistry 2024 |
Click here to see how this site uses Cookies. View our privacy policy here.