A universal and accurate LPMI method for calculating mismatch in heterogeneous ice nucleation†
Received
21st February 2024
, Accepted 20th May 2024
First published on 4th June 2024
Abstract
The interfacial correlation factor f(m,x), where m refers to the interaction among ice, water and the substrate and x refers to the ratio of the critical nucleation size to the surface topography characteristic size of the substrate, plays a crucial role in the classical theory of heterogeneous ice nucleation as it significantly impacts the energy of nucleation. Generally, a smaller value of f(m,x) indicates a higher propensity for ice nucleation. The degree of structural compatibility between ice and the substrate greatly influences f(m,x), particularly on specific substrates. Several approaches have been proposed to calculate the lattice matching based on this idea, which allows whether a surface is favorable for nucleation to be determined. However, none of these methods adequately correlates the mismatch index with ice growth phenomena. In this paper, we embarked on a new attempt to calculate the mismatch index by combining the lattice parameter and Miller index (LPMI). Droplet freezing experiments have been carried out on α-Al2O3 and silicon surfaces with different Miller indices to verify the rationality of the LPMI method. Furthermore, we validated the LPMI method extensively against other works and further demonstrated its readiness, accuracy and universality for freezing problems. The results consistently show that δd = 2|di − ds|/(di + ds) with interplanar spacing more accurately predicts heterogeneous ice nucleation rates across a wide range of substrates than δ1 = (ai − as)/ai with the lattice parameter of ice and the substrate and is more generally applicable than δ2D = (di − di)/di with the distances between two adjacent and congener atoms on the same plane. We believe that the proposed approach will aid in the selection of substrates for promoting or inhibiting heterogeneous nucleation on a specific substrate.
1. Introduction
Water freezing is a ubiquitous natural phenomenon and a critical process in research and industry, ranging from meteorology1 to cryopreservation.2 Pure water can remain liquid at −38 °C.3 Usually, ice crystallization is triggered at a closer theoretical freezing temperature when nucleating surfaces are present, so-called heterogeneous nucleation.4 It is a fact that water freezing on Earth is almost a heterogeneous nucleation process involving foreign particles. Besides the above-mentioned factors, a clear understanding of heterogeneous nucleation is essential for predicting the nucleation ability of the materials5 and designing rational materials to promote6 or restrain7 nucleation. However, a clear and unified picture has not been drawn from these investigations because heterogeneous nucleation is an intricate interplay of the water, ice embryo, and substrate. Fortunately, the free energy of heterogeneous ice nucleation can be expressed as ΔGheter = f(m, x)ΔGhomo based on the classical nucleation theory (CNT), and the value of ΔGheter can indicate whether nucleation is likely to occur or not. When ΔGhomo is determined under given conditions, f(m,x) is the key parameter for predicting nucleation. The interfacial correlation factor f(m,x)8 in classical nucleation theory is a comprehensive parameter, where x is associated with the surface morphology and corrugation of the substrate.9 The parameter m = (γsw−γsi)/γiw is associated with the interfacial free energy among water, ice and the substrate (the subscripts s, i, and w are the substrate, ice and water, respectively).8,10 For the liquid phase, γsw depends on the binding affinity, which is expressed as the intermolecular force between water and the substrate from a microscopic perspective. Stronger binding affinity leads to better wettability at the macroscopic level.11 For the ice phase, the interfacial free energy γsi is associated with the binding affinity of the substrate and the structural match between the substrate and nucleating phase.8,12 According to Aleksandrov,8 the interfacial free energy γsi can be estimated as a function of the binding affinity and the structural match,
, where γsi(α0) is the minimum specific interfacial free energy at a given substrate, ε, b and ν are the elastic modulus, Burgers vector, and Poisson constant, respectively, and δ describes the structural match. For a given material, γsi heavily depends on the structural match between ice and the substrate. A better structural match results in a smaller f(m,x) and thus a lower barrier for heterogeneous ice nucleation.13 Therefore, the structural match plays a vital role in heterogeneous nucleation on the specific material substrate. The most widely studied approach in structural matching is to compare the mismatch of the lattice parameters between ice and the substrate. The lattice parameter difference between ice and the substrate is commonly employed to characterize the degree of conformity between them, which is typically defined as the level of mismatch.14 In heterogeneous ice nucleation, δ denotes the difference in lattice constants of ice and the substrate. According to the consensus of existing studies,8,15,16 a smaller mismatch between the substrate and ice corresponds to enhanced structural matching, thereby favoring the growth of ice nuclei on the substrate. In the atmospheric and environmental science context, various substances have been identified in experiments that work as very effective ice nuclei agents, such as silver iodide,17 kaolinite,18 and corundum (α-Al2O3).19 The crystal structure of these substances matches well with ice, namely, the degree of mismatch is relatively small and is favorable for ice growth. Therefore, a general calculation of the ice–substrate mismatch helps to understand icing.
In earlier studies, a parameter characterizing δ was widely expressed as20
| 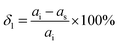 | (1) |
where
ai and
as are the lattice parameters of ice and the substrate, respectively. To further characterize the quantitative relationship between ice and substrate lattice matching, Pruppacher
et al. proposed a new function.
21 | 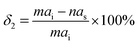 | (2) |
where
m and
n are integers that were selected to minimize
δ. When using the above two methods to calculate the mismatch between crystal planes, such as the primary prism plane (10
![[1 with combining macron]](https://www.rsc.org/images/entities/char_0031_0304.gif)
0) of ice and the substrate, the lattice parameters
a and
c need to be calculated separately. However, the atoms are not exactly in the same plane in the basal plane (0001) and the primary prism plane (10
![[1 with combining macron]](https://www.rsc.org/images/entities/char_0031_0304.gif)
0) of the ice hexagonal (I
h). Therefore, G. N. Patey
et al. came up with a new parameter to describe the mismatch.
15 | 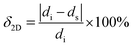 | (3) |
where
di and
ds are the distances between two adjacent and congener atoms on the same plane for ice and the substrate, respectively, as shown in Table S1 (ESI
†). They are analogous lattice distances, not necessarily lattice parameters. The distances
di and
ds are selected depending on the location of the potential or known plane “binding site,” usually interatomic distance.
15 This method is ingenious and takes into account the atomistic morphological features of the plane, which allows us to see how the inner and outer atoms on the same crystal plane of the substrate interact with ice. However,
δ2D requires accurate knowledge of the crystal structure and the positions of the corresponding atoms. Meanwhile,
δ2D is not applicable (NA) in a complex crystal structure, such as the basal plane of boehmite. More details about the mismatch are listed in Table S1 (ESI
†). In summary, the existing methods for characterizing the degree of mismatch have certain limitations. For
δ1 and
δ2, the mismatch would be calculated separately if the lattice parameters
a,
b, and
c are not equal. Moreover, both mismatches for basal and prism planes are relatively small in
δ1 and
δ2, but ice nucleation is observed at the basal plane, not at the prism plane.
15 For
δ2D,
di and
ds require a detailed and accurate crystal structure. Furthermore,
δ2D is not applicable when the crystal structure is complicated, such as mica, boehmite,
etc. To address these shortcomings comprehensively, we aim to propose a more effective approach to quantify the mismatch between ice and the substrate by utilizing interplanar spacing (which combines lattice parameters with Miller indices). Furthermore, experimental validation was conducted to support this expression of mismatch while comparing it with molecular dynamics simulations carried out by other researchers. The comparison demonstrates that our proposed
δd aligns well with both experimental observations and numerical simulation results. We firmly believe that this methodology will greatly contribute to the analysis of heterogeneous ice nucleation.
2. Definition and method
The mismatch denoted by interplanar spacing can be expressed as22 | δd = 2|di − ds|/(di + ds) | (4) |
where di and ds are the interplanar spacing of the crystal panel in ice and the substrate, respectively. Interplanar spacing dhkl between adjacent planes with Miller indices (hkl) is defined as the distance between the first plane and a parallel plane passing through the origin. Interplanar spacing can be visualized in the examples shown in Fig. 1, an example of a simple cubic lattice (a = b = c, α = β = γ = 90°) and a hexagonal lattice (a = b, α = β = 90°, γ = 120°). The formulas of the interplanar spacing for different crystal systems are listed in Table S1 (ESI†) for reference.
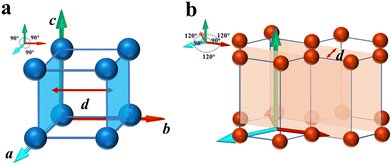 |
| Fig. 1 Examples of some interplanar spacings: (a) (100) plane in a cubic lattice and (b) (10 0) plane in a hexagonal lattice. | |
Each crystal has a set of interplanar spacings of different sizes, which are a function of the lattice parameter and Miller indices. The interplanar spacing is calculated as
| 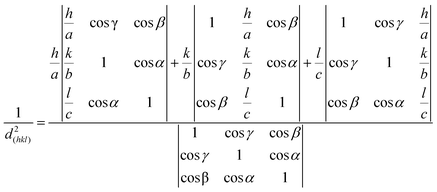 | (5) |
where
a,
b,
c,
α,
β, and
γ are the lattice parameters and (
h,
k,
l) are the Miller indices of the crystal plane. The increase in Miller indices would result in a decrease in the interplanar spacing.
23 To verify the LPMI method, we performed droplet freezing experiments on the surfaces of monocrystalline silicon (cubic structure) and α-Al
2O
3 (hexagonal structure) with different Miller indices. The experimental details are elaborated in the ESI.
† Moreover, we compared the different mismatch expressions with other results of heterogeneous ice nucleation in molecular dynamics. Unit cell parameters for crystal structures in this paper are shown in Table S2 (ESI
†). The results are discussed in the following section.
3. Results and discussion
3.1. Validation of the mismatch by experiments
The freezing process of droplets on monocrystalline silicon wafers and α-Al2O3 flakes is depicted in Fig. 2(a). Upon freezing, the ice–water interface progresses from the bottom to the top of the droplet. The impact of substrate mismatch on droplet freezing was analyzed in terms of the freezing delay time, which is a parameter conventionally considered as the reciprocal of the nucleation rate.8 The freezing delay time refers to the duration required for the droplet to freeze after reaching the target temperature on the substrate. The freezing delay time τc for each substrate is the mean of the measured value from 15 experiments. Fig. 2(b) illustrates that the freezing delay time is shorter on the monocrystalline silicon substrate (110) compared to (100) and (111) surfaces. Concerning α-Al2O3, the freezing delay time is longer on the C-plane (0001) in comparison to the M-plane (10
0) and A-plane (11
0). Table 1 presents the mismatch between the substrate and ice. The crystalline structure of ice was assumed to be hexagonal since the minimum temperature in the experiments was −15 °C, and cubic ice remains stable under extreme conditions such as low temperatures (<190 K)24 and under the influence of a strong external electric field.25
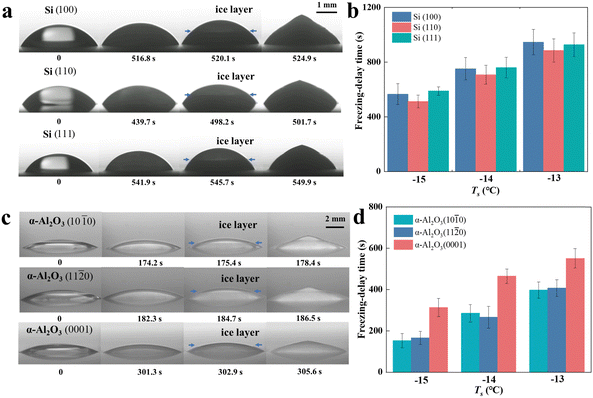 |
| Fig. 2 (a) A droplet with a volume of 10 μL freezes on a silicon substrate at Ts = −15 °C. (b) The freezing delay time of the droplet on silicon substrates. (c) A droplet with a volume of 10 μL freezes on α-Al2O3 substrates at Ts = −15 °C. (d) The freezing delay time of the droplet on α-Al2O3 substrates. The smaller the δd, the shorter the freezing delay time. The δd values for Si (100), α-Al2O3 (10 0) and α-Al2O3 (11 0) are 1.9%, 5.2% and 5.2%, which are smaller than other crystal planes. Thus, freezing occurs easily on these substrates above. The error bar is the error of the mean. | |
Table 1 Mismatch δd between the substrate and ice
Crystal |
Silicon (100) (%) |
Silicon (110) (%) |
Silicon (111) (%) |
α-Al2O3 (10 0) (%) |
α-Al2O3 (11 0) (%) |
α-Al2O3 (0001) (%) |
Ice (Ih) (10 0) |
32.5 |
1.9
|
26.1 |
5.2
|
48.7 |
30.3 |
Ice (Ih) (11 0) |
82.5 |
51.8 |
32.4 |
58.4 |
5.2
|
62.9 |
Ice (Ih) (0001) |
30.1 |
62.8 |
80.4 |
56.3 |
102.2 |
51.7 |
The minimum δd of 1.9% observed between silicon (110) and the primary prism plane of Ih corresponds to the minimum freezing delay time observed on silicon (110). On α-alumina, the freezing delay time on the (0001) plane is longer compared to the (1
0) and (11
0) planes. The minimum δd between ice and α-Al2O3 was 5.2%. A smaller mismatch leads to a lower interfacial correlation factor f(m,x), thereby reducing the barrier for heterogeneous ice nucleation. Consequently, the freezing delay time is shorter under consistent conditions. In other words, the freezing delay time on different Miller indices confirms the accuracy of the mismatch (determined by the interplanar spacing). Molecular dynamics (MD) studies on the first hydration layer13,26,27 reveal that when the lattice mismatch is small, the water dipole distribution closely resembles that of bulk ice, resulting in stronger water–water interactions. Conversely, larger deviations caused by a larger lattice mismatch accelerate the decay of dipole orientation. This leads to higher potential energy and a less stable hydrogen bond network.13 Therefore, a smaller δd value correlates with a shorter freezing delay time. Furthermore, the findings of other MD simulations provide additional evidence to support the significance of the mismatch δd.
3.2. Validation of the mismatch by molecular dynamics
In this section, we present the results of heterogeneous nucleation on β-AgI,15,16 kaolinite,15,28 mica,15,29 gibbsite,15,30 and hematite15,31 in existing studies. Complete information about the substrates can be found in Table S2 (ESI†). Furthermore, we compared the values of δd with δ1, δ2, and δ2D as summarized in Table 2. The results further support the generality of δd, which considers Miller indices and lattice parameters. As shown in Table 2, nucleation occurred on β-AgI, kaolinite (prime plane), mica (basal plane), and PbI2 (basal plane) substrates, but not on gibbsite, hematite, and boehmite substrates within the same simulated time scale. A smaller δ value indicates a higher similarity between the crystal structure of the substrate and that of ice, indicating a greater likelihood of ice formation. Ice nucleation was observed on both the basal and prism planes of β-AgI due to their good lattice matching with ice (Ih).15,16 By comparing the different δ values, we find that δd (by the interplanar spacing) is in good agreement with the MD simulation results. δ1 (by lattice parameter) and δ2 (by lattice parameter) partially align with the MD simulation results. However, there are discrepancies in the case of kaolinite nucleating the (10
0) prism plane of ice (Ih).15,32 The δ1 values for both the basal (14.29%, 98.4%) and prism (14.29%, 2.23%) faces are relatively large. Additionally, for δ2, the match between gibbsite and ice in the prism plane (3.6%, 3.69%) is better than that of kaolinite and ice in the prism plane (5.16%, 0.8%), yet no nucleation of gibbsite is observed in simulations.19 Both δ2D (by analogous lattice distance) and δd are consistent with the observations of kaolinite and gibbsite, but δ2D is not applicable in situations involving crystals with complex structures such as mica, where defining the analogous lattice distance in the prism plane is challenging.
Table 2
δ
1, δ2, δ2D, and δd lattice mismatches of ice (Ih) for the surface considered in the molecular dynamics simulation
Surface label |
|δ1| (%) |
|δ2| (%) |
|δ2D| (%) |
|δd| (%) |
Nucleation |
Basal |
Prism |
Basal |
Prism |
Basal |
Prism |
Basal |
Prism |
β-AgI15,16 |
2.08 |
(2.08, 2.23). |
2.04 |
(2.04, 2.23) |
2.04 |
(2.04, 2.23) |
1.87
|
1.37 |
Y |
Kaolinite15,28 |
(14.29, 98.4) |
(14.29, 2.23) |
(14.29, 0.8) |
(5.16, 0.8) |
14.6 |
(0.78, 1.85) |
11.01 |
5.65
|
Y (prism) |
Mica15,29 |
(15.2, 100.3) |
(15.2, 173.7) |
(15.2, 0.19) |
(6.16, 0.19) |
(0.2, 5.93) |
NA |
4.53
|
21.74 |
Y (basal) |
PbI215 |
1.13 |
(1.13, 6.95) |
1.13 |
(1.13, 6.95) |
1.13 |
NA |
2.52
|
55.47 |
Y (basal) |
Gibbsite15,30 |
(92.8, 12.7) |
(92.8, 32.5) |
(3.6, 12.7) |
(3.6, 3.69) |
14.1 |
(6.2, 0.59) |
27.94 |
16.68 |
N |
Hematite15,31 |
11.6 |
(11.6, 87.17) |
11.6 |
(11.6, 2.71) |
11.6 |
(3.3, 0.58) |
124.67 |
11.01 |
N |
Boehmite15 |
(26.5, 17) |
(26.5, 1.84) |
(26.5, 17) |
(26.5, 1.84) |
NA |
(17.09, 15.48) |
116.6 |
33.78 |
N |
AsI15 |
0 |
51.7 |
0 |
(0, 8) |
0 |
(13.4, 10.94) |
0 |
51.7 |
Y (basal) |
AsH15 |
11.6 |
87.17 |
11.6 |
(11.6, 2.71) |
11.6 |
(3.3, 0.58) |
124.6 |
12.01 |
N |
HsI15 |
0 |
(0, 87.17) |
0 |
(0, 8) |
0 |
(13.4, 10.94) |
0 |
51.7 |
Y (basal) |
We further compared the mismatch among artificial crystals based on the previous comparisons of actual crystal structures. Scaling α-Al2O3 eliminates the mismatch for the basal plane, resulting in ice nucleation on the surface. The four measures of δ align well with the observations. Conversely, scaling α-Al2O3 to hematite (referred to as AsH) increases the mismatch for the basal plane, leading to the absence of ice nucleation in both the basal and prism planes. In this case, δ1, δ2, and δd consistently correspond to the observations, except for a relatively small δ2D value (3.3%, 0.58%) for the prism plane. Furthermore, scaling hematite to ice (referred to as HsI) eliminates the mismatch at the basal plane, resulting in ice nucleation under specific force fields. All measures of δ align well with the simulation results. By comparing different mismatches on real and fictional crystals, we find that δ1, δ2, δ2D, and δd are to some extent consistent with the observations. However, δ2D requires precise knowledge of the crystal structure and the positions of the corresponding atoms, making it inapplicable for certain complex crystal structures. Additionally, for different water-potentials (TIP4P/Ice and six-site) and the different water-substrate interactions (CLAFF and LJ), the result of ice nucleation in the same time interval is the same, which means that the potential and the water-substrate interaction have little influence on ice nucleation. Therefore, δd, which considers crystal parameters and Miller indices, provides a better description of the mismatch between ice and crystals in heterogeneous nucleation.
3.3. Regression analyses of mismatch
We conducted a regression analysis on various methods for calculating mismatches. According to Classical Nucleation Theory, the freezing delay time can be calculated by eqn (6),33 | 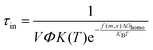 | (6) |
where V represents the droplet volume, Φ is the fraction area of the water and substrate, K(T) denotes a factor of the diffusion flux of water molecules through the ice–water interface, usually its value is 1025 cm−2 s−1, KB denotes the Boltzmann constant, and ΔGhomo is the nucleation barrier, which can be expressed as | 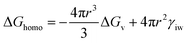 | (7) |
where ΔGv = ΔHm,f(Tf−T)/Tf, ΔHm,f and Tf denote melting enthalpy and melting temperature, respectively. f(m,x) denotes the interfacial correlation factor, which can be expressed as | 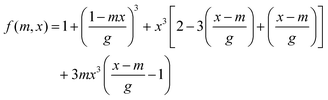 | (8) |
| x = R/rc, m = (γsw − γsi)/γiw, g = (1 + x2 − 2mx)½ | (9) |
where R and rc are the mean surface roughness and the radius of the critical nucleus, respectively. Additionally, the minimum specific interfacial free energy γsi(α0) of a given substrate is expressed as34where ε0 is the interaction between ice and a solid, and n is the number of neighboring molecules. Thus, the regression analyses of experimental τc, simulated τc and predicted τc are displayed in Fig. 3. Since δ1 and δ2 are similar, we take δ1 as an example for analysis.
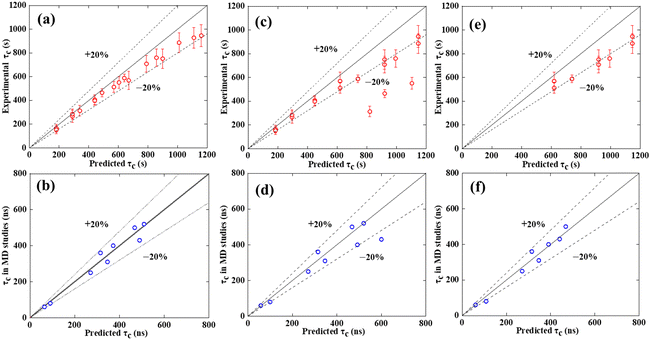 |
| Fig. 3 Regression analyses. (a), (c) and (e) Show regression analyses based on experimental data. (b), (d) and (f) Show regression analyses based on simulated data. The data from the simulation is listed in Table S4 (ESI†). (a) and (b) Show regression analyses based on δd. (c) and (d) Show regression analysis based on δ1. (e) and (f) show regression analyses based on δ2D. The mean freezing delay time is more accurately predicted across a wide range of substrates by δd than δ1 or δ2D. The red points represent the mean values of experimental measurements, with error bars indicating the standard error of the mean; the blue points derive from the freezing delay time of MD simulations15,16 in existing studies. | |
In all cases, δ describes the mismatch between the substrates and the ice (Ih). We report only absolute values (|δ|) of the mismatch. Only one value in the table indicates that the lattice parameters of the corresponding crystal planes are equal. For example, a and b of the basal plane in β-AgI are equal. Y means that ice nucleation occurred spontaneously on the substrate, and the nucleation plane is given. N means that no ice nucleation was observed within the simulation time scale. Nucleation occurs at both the basal and prism planes of β-AgI.
Fig. 3 shows the regression analyses of δd than δ1 or δ2D. In panels (a), (c), and (e), the y-axis signifies times ascertained through experimental measurements, whereas in panels (b), (d), and (f), it delineates the freezing delay time derived from MD simulations15,16 as reported in publications. The x-axis embodies the theoretical nucleation time, which is deduced by integrating nucleation temperatures and specific surface characteristics into eqn (6). The line y = x symbolizes instances where experimental or simulation values equal theoretical predictions, while the positions of points within the figure elucidate deviations between experimental or simulated outcomes and their corresponding theoretical expectations. Fig. 3 demonstrates that the predicted freezing delay time (τc) based on δd has an error of less than 20% when compared to experimental and simulated τc. However, when using δ1 for prediction, the error exceeds 20% in several trials, indicating poor accuracy. Although the predicted τc based on δ2D is within the 20% error range, determining the analogous lattice distance proves challenging, especially for complex crystal structures like α-Al2O3. Consequently, τc cannot be reliably predicted using δ2D. Hence, the mismatch measure δd is more accurate in predicting the freezing delay time and exhibits good universality.
In summary, the analysis establishes the relationship between different representations of δ and nucleation from a crystal structure perspective. The extension of the mismatch measure δd from one-dimensional lattice parameters (a, b, and c) to crystal planes with various Miller indices has demonstrated its superior accuracy in both experimental and numerical simulations. This approach only requires basic information such as crystal type, lattice parameters, and Miller indices of the crystal plane to obtain interplanar spacings. By comparing the mismatch between the substrate and ice, it becomes possible to rapidly select and design structures for promoting or inhibiting ice nucleation in specific materials. However, heterogeneous ice nucleation is a complex interplay between ice and the substrate, involving atomic-level insights. Achieving a more accurate description of heterogeneous ice nucleation would require further systematic and microscopic analysis.
4. Conclusions
In this study, we performed a comparison of δ1 (based on lattice parameters), δ2 (based on lattice parameters), δ2D (based on analogous lattice distances), and δd (based on interplanar spacings) through experiments and simulations. Our results demonstrate that δd, which takes into account the lattice parameter and Miller index, is more accurate than δ1 and δ2, as well as more universal than δ2D. δd provides a more comprehensive description of the mismatch in heterogeneous ice nucleation. It is crucial to have a proper match between the crystal structure and ice for successful ice nucleation. However, it should be noted that heterogeneous ice nucleation is a complex phenomenon requiring further systematic and microscopic analysis. The methods utilized in this paper are expected to contribute to a broader understanding of heterogeneous nucleation.
Conflicts of interest
There are no conflicts to declare.
Acknowledgements
The authors would like to acknowledge the research grant from the National Natural Science Foundation of China (No. 52176056), the Innovative research group project of the National Natural Science Foundation of China (No. 52021004), and the Open Fund of Key Laboratory of Icing and Anti/De-icing (Grant No. IADL20200303).
References
- I. A. Renfrew, C. Barrell, A. Elvidge, J. Brooke, C. Duscha, J. King, J. Kristiansen, T. L. Cope, G. W. K. Moore and R. S. Pickart, An evaluation of surface meteorology and fluxes over the Iceland and Greenland Seas in ERA5 reanalysis: The impact of sea ice distribution, Q. J. R. Meteorol. Soc., 2021, 147(734), 691–712 CrossRef.
- Q. Fan, M. Dou, J. Mao, Y. Hou, S. Liu, L. Zhao, J. Lv, Z. Liu, Y. Wang and W. Rao, Strong Hydration Ability of Silk Fibroin Suppresses Formation and Recrystallization of Ice Crystals During Cryopreservation, Biomacromolecules, 2021, 23(2), 478–486 CrossRef.
- B. Murray, D. O'sullivan, J. Atkinson and M. Webb, Ice nucleation by particles immersed in supercooled cloud droplets, Chem. Soc. Rev., 2012, 41(19), 6519–6554 RSC.
- D. Turnbull, Kinetics of heterogeneous nucleation, J. Chem. Phys., 1950, 18(2), 198–203 CrossRef CAS.
- N. Hiranuma, O. Möhler, K. Yamashita, T. Tajiri, A. Saito, A. Kiselev, N. Hoffmann, C. Hoose, E. Jantsch, T. Koop and M. Murakami, Ice nucleation by cellulose and its potential contribution to ice formation in clouds, Nat. Geosci., 2015, 8(4), 273–277 CrossRef CAS.
- I. Massie, C. Selden, H. Hodgson and B. Fuller, Cryopreservation of encapsulated liver spheroids for a bioartificial liver: reducing latent cryoinjury using an ice nucleating agent, Tissue Eng., Part C, 2011, 17(7), 765–774 CrossRef CAS PubMed.
- M. J. Kreder, J. Alvarenga, P. Kim and J. Aizenberg, Design of anti-icing surfaces: smooth, textured or slippery?, Nat. Rev. Mater., 2016, 1(1), 15003 CrossRef CAS.
- Z. Zhang and X.-Y. Liu, Control of ice nucleation: freezing and antifreeze strategies, Chem. Soc. Rev., 2018, 47(18), 7116–7139 RSC.
- V. J. Rico, C. López-Santos, M. N. Villagrá, J. P. Espinós, G. F. de la Fuente, L. A. Angurel, A. Borrás and A. N. R. González-Elipe, Hydrophobicity, freezing delay, and morphology of laser-treated aluminum surfaces, Langmuir, 2019, 35(19), 6483–6491 CrossRef CAS PubMed.
- L. Boinovich, A. M. Emelyanenko, V. V. Korolev and A. S. Pashinin, Effect of wettability on sessile drop freezing: when superhydrophobicity stimulates an extreme freezing delay, Langmuir, 2014, 30(6), 1659–1668 CrossRef CAS.
- W. Abdallah, J. S. Buckley, A. Carnegie, J. Edwards, B. Herold, E. Fordham, A. Graue, T. Habashy, N. Seleznev and C. Signer, Fundamentals of wettability, Technology, 1986, 38(1125–1144), 268 Search PubMed.
-
R. F. Sekerka, Advances in Crystal Growth Research, Fundamentals of phase field theory. 2001, p. 21–41 Search PubMed.
- Z. Liu, C. Li, E. C. Goonetilleke, Y. Cui and X. Huang, Role of Surface Templating on Ice Nucleation Efficiency on a Silver Iodide Surface, J. Phys. Chem. C, 2021, 125(34), 18857–18865 CrossRef CAS.
- M. A. Olmstead and R. Bringans, Role of lattice mismatch and surface chemistry in the formation of epitaxial semiconductor-insulator interfaces, Phys. Rev. B: Condens. Matter Mater. Phys., 1990, 41(12), 8420 CrossRef CAS.
- A. Soni and G. N. Patey, How Microscopic Features of Mineral Surfaces Critically Influence Heterogeneous Ice Nucleation, J. Phys. Chem. C, 2021, 125(19), 10723–10737 CrossRef CAS.
- A. Soni and G. N. Patey, Ice Nucleation by the Primary Prism Face of Silver Iodide, J. Phys. Chem. C, 2022, 126(15), 6716–6723 CrossRef CAS.
- P. DeMott, Quantitative descriptions of ice formation mechanisms of silver iodide-type aerosols, Atmos. Res., 1995, 38(1–4), 63–99 CrossRef CAS.
- H. Wex, P. DeMott, Y. Tobo, S. Hartmann, M. Rösch, T. Clauss, L. Tomsche, D. Niedermeier and F. Stratmann, Kaolinite particles as ice nuclei: learning from the use of different kaolinite samples and different coatings, Atmos. Chem. Phys., 2014, 14(11), 5529–5546 CrossRef.
- A. Soni and G. N. Patey, Why α-Alumina Is an Effective Ice Nucleus, J. Phys. Chem. C, 2019, 123(43), 26424–26431 CrossRef CAS.
- Y. Qiu, N. Odendahl, A. Hudait, R. Mason, A. K. Bertram, F. Paesani, P. J. DeMott and V. Molinero, Ice nucleation efficiency of hydroxylated organic surfaces is controlled by their structural fluctuations and mismatch to ice, J. Am. Chem. Soc., 2017, 139(8), 3052–3064 CrossRef CAS.
-
H. R. Pruppacher, J. D. Klett and P. K. Wang, Microphysics of clouds and precipitation. Taylor & Francis, 1998 Search PubMed.
- J. Liu, L. Zhang, D. Yao and L. Meng, Microstructure evolution of Cu/Ag interface in the Cu–6 wt% Ag filamentary nanocomposite, Acta Mater., 2011, 59(3), 1191–1197 CrossRef CAS.
- Q. Fan, A new method of calculating interplanar spacing: the position-factor method, J. Appl. Crystallogr., 2012, 45(6), 1303–1308 CrossRef CAS.
- B. J. Murray, D. A. Knopf and A. K. Bertram, The formation of cubic ice under conditions relevant to Earth's atmosphere, Nature, 2005, 434(7030), 202–205 CrossRef CAS PubMed.
- J. Yan and G. Patey, Heterogeneous ice nucleation induced by electric fields, J. Phys. Chem. Lett., 2011, 2(20), 2555–2559 CrossRef CAS.
- C. Li, X. Gao and Z. Li, Roles of surface energy and temperature in heterogeneous ice nucleation, J. Phys. Chem. C, 2017, 121(21), 11552–11559 CrossRef CAS.
- C. Li, Z. Liu, E. C. Goonetilleke and X. Huang, Temperature-dependent kinetic pathways of heterogeneous ice nucleation competing between classical and non-classical nucleation, Nat. Commun., 2021, 12(1), 4954 CrossRef CAS PubMed.
- G. C. Sosso, T. Li, D. Donadio, G. A. Tribello and A. Michaelides, Microscopic Mechanism and Kinetics of Ice Formation at Complex Interfaces: Zooming in on Kaolinite. The, J. Phys. Chem. Lett., 2016, 7(13), 2350–2355 CrossRef CAS PubMed.
- A. Soni and G. N. Patey, Unraveling the Mechanism of Ice Nucleation by Mica (001) Surfaces. The, J. Phys. Chem. C, 2021, 125(48), 26927–26941 CrossRef CAS.
- E. Chong, M. King, K. E. Marak and M. A. Freedman, The Effect of Crystallinity and Crystal Structure on the Immersion Freezing of Alumina, J. Phys. Chem. A, 2019, 123(12), 2447–2456 CrossRef CAS PubMed.
- N. Hiranuma, N. Hoffmann, A. Kiselev, A. Dreyer, K. Zhang, G. Kulkarni, T. Koop and O. Möhler, Influence of surface morphology on the immersion mode ice nucleation efficiency of hematite particles, Atmos. Chem. Phys., 2014, 14(5), 2315–2324 CrossRef.
- S. A. Zielke, A. K. Bertram and G. N. Patey, Simulations of Ice Nucleation by Kaolinite (001) with Rigid and Flexible Surfaces. The, J. Phys. Chem. B, 2016, 120(8), 1726–1734 CrossRef CAS PubMed.
- R. Zhang, P. Hao, X. Zhang and F. He, Supercooled water droplet impact on superhydrophobic surfaces with various roughness and temperature, Int. J. Heat Mass Transfer, 2018, 122, 395–402 CrossRef CAS.
-
I. Z. Fisher, Statistical theory of liquids, University of Chicago Press, 1964 Search PubMed.
Footnote |
† Electronic supplementary information (ESI) available: The experimental system, experimental procedure, characterization of substrates, and detailed crystallographic parameters. See DOI: https://doi.org/10.1039/d4cp00748d |
|
This journal is © the Owner Societies 2024 |