DOI:
10.1039/D3CB00200D
(Review Article)
RSC Chem. Biol., 2024,
5, 483-499
Recent advances in chemical biology tools for protein and RNA profiling of extracellular vesicles
Received
17th October 2023
, Accepted 25th April 2024
First published on 30th April 2024
Abstract
Extracellular vesicles (EVs) are nano-sized vesicles secreted by cells that contain various cellular components such as proteins, nucleic acids, and lipids from the parent cell. EVs are abundant in body fluids and can serve as circulating biomarkers for a variety of diseases or as a regulator of various biological processes. Considering these characteristics of EVs, analysis of the EV cargo has been spotlighted for disease diagnosis or to understand biological processes in biomedical research. Over the past decade, technologies for rapid and sensitive analysis of EVs in biofluids have evolved, but detection and isolation of targeted EVs in complex body fluids is still challenging due to the unique physical and biological properties of EVs. Recent advances in chemical biology provide new opportunities for efficient profiling of the molecular contents of EVs. A myriad of chemical biology tools have been harnessed to enhance the analytical performance of conventional assays for better understanding of EV biology. In this review, we will discuss the improvements that have been achieved using chemical biology tools.
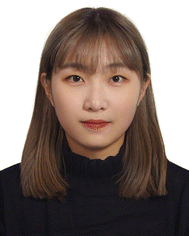
Woojeong Lim
| Woojeong Lim received her BS (2020) and MS (2022) from the Department of Chemistry at Kangwon National University. Her research interest is focused on the extracellular vesicle analysis using electrochemical technologies for disease diagnosis. |
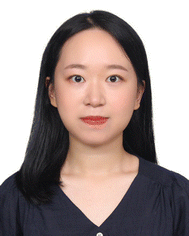
Soyeon Lee
| Soyeon Lee received her BS (2022) from the Department of Chemistry at Kangwon National University. Her research interest is focused on the extracellular vesicle analysis using radical reactions for disease diagnosis. |
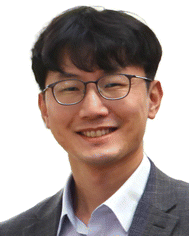
Minseob Koh
| Minseob Koh completed his BS (2007) and PhD (2013) in the Department of Chemistry at Seoul National University. After postdoctoral work at Scripps Research, he began his academic career in 2020 at the Department of Chemistry at Pusan National University, Korea. His research is primarily focused on the design of genetic circuits for biological logic gates, expanding the genetic code, and engineering proteins for use in biomedical applications. |
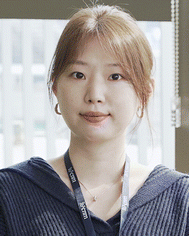
Ala Jo
| Ala Jo received her BS (2011) and PhD (2017) from the Department of Chemistry at Seoul National University. After postdoctoral work at Harvard Medical School, she is working at the Center for Nanomedicine of Institute for Basic Science at Yonsei University, Korea. Her research interest is focused on the extracellular vesicle analysis for disease diagnosis. |
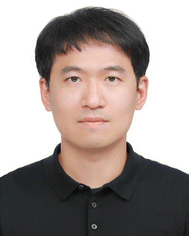
Jongmin Park
| Jongmin Park received his BS (2005) and PhD (2012) from the Department of Chemistry at Seoul National University. After postdoctoral work at Harvard Medical School, he began his academic career in 2018 in the Department of Chemistry at Kangwon National University, Korea. His research interests are focused on the development of novel bioprobes, small molecule-target protein identification and extracellular vesicle analysis for disease diagnosis. |
1. Introduction
Extracellular vesicles (EVs) are membrane-bound nanoscale vesicles secreted by all types of cells in our body.1–4 According to their size and subcellular origin, EVs can be further classified into exosomes, ectosomes, microvesicles (MVs) and apoptotic bodies.3–11 EVs function as mediators of intercellular communications within the body.12,13 They contain various molecular cargos of parent cells, including proteins,14,15 nucleic acids (NAs),16–18 lipids19 and metabolites.20,21 By shuttling their molecular contents between distant cells, EVs regulate a variety of physiological processes and disease progression such as cellular homeostasis,22,23 angiogenesis,24,25 tumor development,26,27 and inflammation.28,29 Thus, the monitoring of the molecular contents of EVs offer new opportunities to unravel their biogenesis pathways and biological functions. Furthermore, EVs serve as promising circulating biomarkers for various diseases.30–35 Under different physiological conditions, distinct cellular contents are packaged into EVs and then secreted into systemic circulation.36,37 Thus, the molecular contents of EVs often reflect the presence, stage, and progression of disease. EVs are also abundant in many biological fluids including blood, saliva, urine, ascites, and cerebrospinal fluids. In this context, the detection of EV biomarkers in complex biological fluids (e.g., liquid biopsy) has emerged as a new avenue for disease diagnosis and prognosis (Fig. 1(A)). Among the biological fluids, EVs in plasma or urine from patients are easily accessible and have been well-studied recently for various biomedical research.38 The comprehensive analysis of EV biomarkers in plasma and urine has immense potential in enabling the diagnosis of various medical conditions, including cancers,17,30,32 kidney disease,39 and neurodegenerative diseases.40,41 Along with diagnosis, treatment monitoring and prognosis by EV analysis have been also recently been in the spotlight.42 Plasma or urinal EV analysis is now expected to suggest a fast and personalized disease treatment strategy.
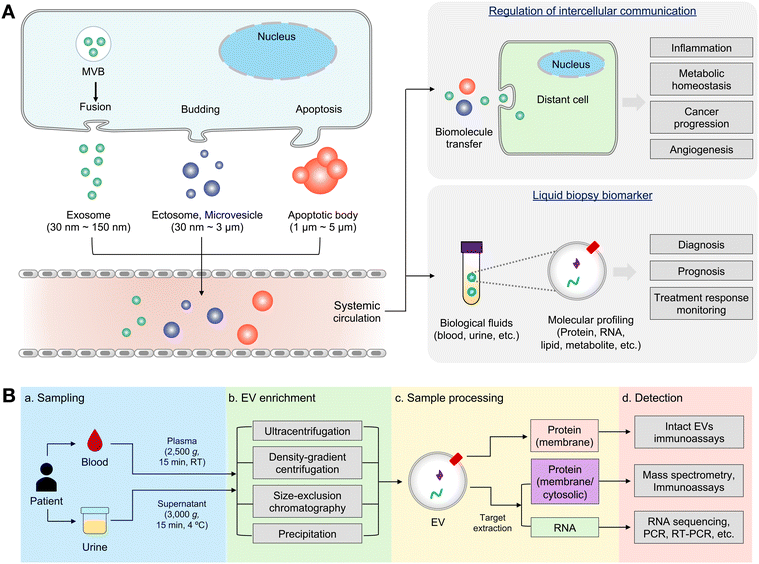 |
| Fig. 1 (A) Intracellular pathways of EV biogenesis, biological functions of EVs, and their analysis. EVs are generated either through the outward budding of plasma membrane (forming ectosomes, microvesicles and apoptotic bodies) or through the inward budding of the endosomal membrane (forming exosomes). Once secreted into the systemic circulation, EVs mediate intercellular communication by transferring their biomolecules between distant cells. Analyzing EVs in biological fluids can help to determine the stage of disease by profiling various EV biomarkers, including proteins, nucleic acids, metabolites, and lipids. (B) The experimental process of EV profiling. Biological fluids are obtained from patients, and processed prior to the EV enrichment step. EVs can be enriched from the similarly sized non-EV particles by various methods include ultracentrifugation, density-gradient centrifugation, size-exclusion chromatography and precipitation. The isolated EVs may require additional sample processing steps to extract analytical targets depending on the downstream detection method. Finally, intact EVs or extracted target analytes are analyzed by various analytical methods for protein and RNA detection. | |
Over the past decade, there have been significant advancements in analytical technologies for molecular profiling of EVs in the field of biomedical research.30,43,44 Analytical technologies such as proteomics,45 sequencing,46 flow cytometry,47 and microfluidics48 have been adapted to analyze EVs with high precision and efficiency. In particular, assay techniques for liquid biopsy have become more widespread to enable the rapid and efficient detection of EVs in biofluids.30 Although tissue biopsies are a gold standard in disease diagnostics, they are highly invasive and show limited capacity in repeated sampling to capture spatiotemporal dynamics of disease biology.49 On the other hand, EVs offer a minimally invasive sampling capacity for both disease diagnosis and treatment monitoring. More importantly, EVs show strong potential in early diagnosis as the alterations in the EV molecular cargos may occur prior to changes in traditional serum markers or the size of the lesion reaches the detection limit of imaging modalities.50 In addition, since EVs contain diverse cellular contents such as proteins and NAs, molecular profiling of EVs can thus provide various pathophysiological information of parent cells.51 This stands in contrast to other circulating biomarkers such as circulating tumor cells,52 cell-free NAs,53,54 and proteins.55 Such appealing features render EV profiling with significant advantages over traditional diagnostic methods.
Despite their clinical potential, the unique physical and biological properties of EVs present challenges in their detection within body fluids (Fig. 1(B)). Firstly, the nanoscale of EV dimensions (typically 50–200 nm) is a major hurdle for their isolation from biofluids, due to the presence of similarly sized non-EV particles (e.g., lipoproteins, exomeres, soluble proteins, and RNA complexes).56,57 Secondly, conventional assays such as enzyme-linked immunosorbent assay (ELISA), western blotting and polymerase chain reaction (PCR) often show limited sensitivity and require laborious sample processing to detect traces of target EVs.58 Notably, EV proteins and NAs are mostly membrane-bound or intravesicular, requiring complex sampling processes for their detection using conventional assays.59 In addition, EVs display significant heterogeneity in their molecular constituents, and only a few EVs carry disease-specific information such as protein mutations.10 As a result, bulk analysis of EVs may overlook crucial details about subcellular origin, dynamics of EV biogenesis, and rare disease-specific markers.
Recent advances in chemical biology provide new opportunities for efficient profiling of the molecular contents of EVs. In the field of EV analysis, a myriad of chemical biology tools have been harnessed to enhance the analytical performance of conventional assays.30 Immunoassays and NA detection methods have gained significant attention, given that proteins and NAs are the primary molecular targets of EV detection. These chemical biology strategies mostly improve the sensitivity of traditional bioassays through signal amplification, enhance specificity by enriching targets in biofluids, and enable multiplexed detection of biomarkers, which are challenging in conventional EV bioassays (Table 1). In this review, we explore pivotal assay technologies advancing molecular profiling of EVs. Our primary emphasis is on protein and NA detection, and we highlight how chemical biology tools are contributing to the development of robust EV assays.
Table 1 Protein and RNA profiling of EVs: challenges and overcoming chemical biology strategies
Challenges |
Conventional approaches |
Novel approaches |
Strategies |
Limitations |
Strategies |
Chemical biology tools |
Key features |
EV enrichment |
|
Ultracentrifugation |
Purity |
Affinity-based enrichment |
Immunoaffinity isolation |
Sensitive |
Density gradient centrifugation |
Low throughput |
Specific |
Size-exclusion chromatography |
Time-consuming |
Simple procedure |
Precipitation |
|
|
Analysis of EV RNA and EV protein |
Protein |
ELISA |
Low sensitivity |
Signal amplification |
Enzyme reaction |
Sensitive |
Western blotting, |
Complex |
HCR, RCA |
Rapid |
Mass spectrometry |
Time-consuming |
DNA nanostructure, EV–liposome fusion, stimuli-responsive hydrogel |
Simple procedure |
RNA |
PCR |
|
Multiplexed analysis |
Cyclic imaging |
Sensitive |
RNA sequencing |
|
Immuno-sequencing |
EV heterogeneity |
EV glycoprotein analysis |
|
Fluorescent protein reporters |
Signaling perturbation |
Metabolic labeling |
Metabolic glycoengineering (via bioorthogonal chemistry) |
Specific |
Covalent modification, |
EV dysfunction |
Mild conditions |
Lipid dyes |
Dye aggregation |
|
Treatment response monitoring |
|
Protein and RNA profiling |
Limited biomarker |
Competitive target protein binding |
Bioorthogonal chemistry |
Direct drug–target interaction monitoring, |
Specific |
2. EV enrichment
2.1 Immunoaffinity isolation
The primary challenge of liquid biopsy is detecting specific biomarkers in the complex biofluids that contain various background biomolecules. Therefore, enrichment of target biomarkers is crucial to elevate the assay sensitivity and specificity.60–62 For enriching EVs, conventional isolation methods such as ultracentrifugation, size exclusion chromatography, and precipitation are widely used in the field.63 These methods rely on the biophysical properties of EVs, such as size, density and solubility. However, conventional isolation methods often struggle to distinguish EVs from other non-EV biomolecules with similar physical properties of EVs.64–67 As a result, downstream immunoassays may exhibit low sensitivity. Due to these challenges, there is a growing interest in immunoaffinity isolation methods.68,69 Immunoaffinity isolations utilize affinity ligands, such as antibodies or aptamers to enrich target proteins. Typically, these affinity ligands are functionalized on the surface of solid substrates such as microbeads,32,70 nanoparticles,71 glasses72 or PDMS devices,73 and are used to capture the target biomarker selectively from various background biomolecules. Immunoaffinity-based EV isolation can efficiently separate EVs by recognizing and capturing proteins on the EV's surface, including tetraspanins (e.g., CD63, CD9, and CD81) and tumor-specific markers.
Recently, click chemistry has significantly empowered immunoaffinity-based EV isolation.14,74–76 In this approach, solid substrates are functionalized with one component of the click reaction, while antibodies are modified with the other part of the reaction. First, surface protein markers on EVs are targeted with specific antibodies conjugated with a click handle. By leveraging the fast kinetics and orthogonality of click chemistry, antibody-targeted EVs are rapidly and selectively captured on the solid substrates, followed by downstream molecular characterization to profile marker expression. Moreover, through surface functionalization chemistry, various materials can be functionalized with the click reaction components and implemented for immunoaffinity-based EV isolation (Fig. 2).
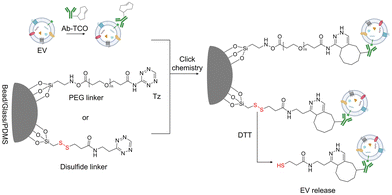 |
| Fig. 2 Schematic diagram of immunoaffinity isolation based on tetrazine (Tz)/trans-cyclooctene (TCO) click chemistry. Solid substrates are functionalized with Tz either through non-cleavable or cleavable linkers. EVs are recognized using TCO-conjugated antibodies and captured to solid substrates through click chemistry. Under mild reduction conditions (e.g., DTT), EVs are released without dysfunction, allowing for subsequent downstream bioassays. | |
For example, Shao et al. developed a highly sensitive and rapid EV analytical technique based on immunoaffinity isolation of MVs from glioblastoma (GBM) patient samples.14 In this approach, surface protein markers on MVs are primarily targeted using antibodies modified with trans-cyclooctene (TCO). These MVs are then captured by magnetic nanoparticles (MNPs) functionalized with 1,2,4,5-tetrazine (Tz). The fast click reaction between Tz and TCO enables the rapid capture of MVs that are expressing target protein markers. In addition, by using miniaturized micronuclear magnetic resonance (μNMR), the decay rate (R2) which is proportional to the MNP concentration was directly measured, allowing the quantification of protein markers on MVs without the need for further downstream bioassays. The two-step immunoaffinity capture approach offers higher capture efficiency compared to direct MNP–antibody conjugation.77
Integrating immunoaffinity isolation with downstream RNA profiling further expands the diagnostic potential of EVs. Following the immunoaffinity-based EV isolation, the EVs are lysed to extract their RNAs, which are then subjected to profiling such as reverse-transcription PCR (RT-PCR) or sequencing. With this approach, Sun et al. successfully purified plasma EVs from pancreatic cancer patients, and identified oncogenic gene alterations by the profiling of EV RNAs with digital PCR (dPCR) analysis.74 Alternatively, hiring a cleavable disulfide cross-linker facilitates a rapid EV purification platform for downstream EV profiling. Compared to other bond cleavage methods, disulfide bonds are easily and specifically cleaved under mild reducing conditions such as 1,4-dithiothreitol (DTT). Therefore, after EV capture mediated by click chemistry, exposure to DTT leads to the rapid release of EVs without affecting their biomolecular composition. For example, Sun et al. developed an EV purification system aiming for early detection of hepatocellular carcinoma (HCC).75 In this study, densely packed silicon nanowire substrates (SiNWS) were functionalized with Tz using a disulfide-containing linker to capture EVs via Tz-TCO click chemistry. An anti-HCC marker (e.g., EpCAM, ASGPR1 and CD147) antibody cocktail modified with TCO was then introduced to isolate HCC-derived EVs. After capturing HCC-derived EVs, they were released with DTT treatment, and then lysed for RNA extraction and profiling. By employing a digital droplet PCR (ddPCR) assay, 10 HCC-specific mRNA transcripts were quantified from HCC patient samples for early detection.
3. Analysis of EV RNA and protein
Signal amplification is the most important strategy in biomarker detection, enabling enhanced sensitivity and improved accuracy.78 This is especially critical for detecting EV biomarkers given the challenges associated with their low abundancy. To detect EV protein biomarkers, various chemical biology tools have been incorporated into the immunoassays to amplify the analytical signal. The most common signal amplifiers include enzyme reactions79 and nucleic-acid based isothermal signal amplification, such as hybridization chain reaction (HCR)80 and rolling circle amplification (RCA).81 This section explores the recent advances in signal amplification strategies tailored for EV profiling.
3.1 Enzyme reaction
With a focus on high-grade serous ovarian carcinoma (HGSOC), SAViA (Signal Amplifying Vesicles in Array) was developed as an extracellular vesicle (EV)-based test for early diagnosis of ovarian cancer (Fig. 3(A)).82 The SAViA assay, integrating EV physisorption and tyramide-assisted signal amplification, increased analytical sensitivity over conventional immunoassays by more than 10
000-fold, enabling the detection of a small amount of EVs (limit of detection (LOD): 600 vesicles) within a practical microwell-plate format (384 wells). Notably, this SAViA assay represents the first reported approach for targeting EVs derived from fallopian tubes. Ongoing evaluation of SAViA in the tumor mouse model and HGSOC patient samples supports the assay's potential for the early detection of ovarian cancer.
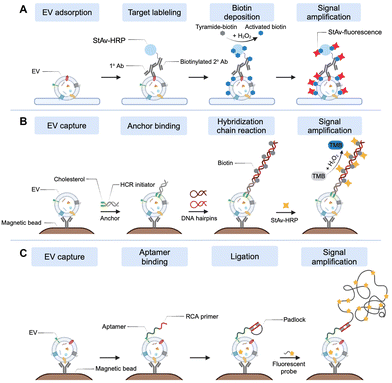 |
| Fig. 3 Signal amplification strategies for EV protein profiling. (A) Schematic illustration of SAViA (signal amplifying vesicles in array). EV surface proteins are detected by the antibodies and the signals are amplified through a tyramide-assisted reaction. (B) Signal amplification using HCR. Bivalent cholesterol (BChol)-labeled HCR initiators are inserted into the EV membrane, and the signal is amplified using horseradish peroxidase (HRP)-linked HCR. (C) Schematic of RCA (rolling circle amplification)-assisted FCA (flow cytometry approach). RCA primer-conjugate aptamers recognize EV surface proteins on the magnetic beads, and the RCA process amplifies the fluorescent signal. The beads are analyzed by flow cytometry. | |
DEST (digital extracellular vesicle screening technique) is another EV analysis method harnessing tyramide-assisted signal amplification for reliably distinguishing high-risk intraductal papillary mucinous neoplasms (IPMN) from low-risk IPMN.70 In DEST, EVs are immuno-captured onto microbeads via capture antibodies and subsequently detected using biotinylated detection antibodies. This is followed by a tyramide signal amplification for the fluorescent bead labeling. The DEST method enables rapid and sensitive detection of both surface and intravesicular EV proteins. Moreover, the DEST assay allows for high-throughput digital analysis of clinical samples and has a broad dynamic range of EV detection.
3.2 Hybridization chain reaction
He et al. developed a signal amplification strategy for the efficient detection of EV protein markers using hybridization chain reaction (HCR) (Fig. 3(B)).83 In this technique, immunomagnetic beads are employed to capture surface proteins of EVs. Subsequently, bivalent cholesterol (BChol)-labeled DNA anchors are inserted into the EV membrane. These anchors are conjugated to HCR initiator sequences and the HCR was initiated by introducing biotin-conjugated hairpin probes. Following this, binding with horseradish peroxidase (HRP)-linked streptavidin and enzyme-mediated oxidation leads to a color change. This method allows for highly sensitive, accurate, and reproducible detection of EVs with a LOD of 2.2 × 103 EVs per μl, outperforming conventional ELISA by a factor of a hundred.
Xing et al. developed the apta-HCR-CRISPR assay, a novel method that synergizes HCR and CRISPR-Cas12a-based signal amplification for the direct and highly sensitive detection of tumor-derived EVs.84 In this study, aptamers undergo HCR amplification to generate long sequences filled with repeated CRISPR RNA (crRNA) target sequences. These sequences are bound to CRISPR-Cas12a, undergo concomitant cleavage, resulting in a significant amplification of the fluorescent signal. Employing this strategy, the team successfully detected nucleolin and programmed death ligand 1 (PD-L1) proteins in tumor-derived EVs. Impressively, this method achieved a LOD of 102 EVs per μl, which is at least 1000 times lower than aptamer-based ELISA. When applied directly to clinical samples, the assay demonstrated its potential in identifying nucleolin- and PD-L1-positive EVs, showing promise for enhanced cancer management.
3.3 Rolling circle amplification
Gao et al. developed an RCA-assisted flow cytometry approach (FCA) to profile surface proteins and quantify EVs simultaneously (Fig. 3(C)).85 In this method, EVs are first captured by CD63 antibody-conjugated magnetic beads. Following this, aptamers coupled with DNA primers recognize and bind to the surface proteins of EVs. The subsequent RCA process generated repetitive DNA sequences, which facilitate the binding of fluorescent probes. Using a standard flow cytometer, the research team analyzed various EV protein markers. Notably, this RCA-assisted FCA technique demonstrated an impressive detection limit of 1.3 × 105 EVs per mL.
Zhang et al. developed a local fluorescence imaging technique called Digital Profiling of Proteins in Individual EVs (DPPIE) to analyze multiple proteins in individual EVs.86 This assay captured EVs from patient plasma samples using an CD9 antibody-engineered biochip. The captured EVs are further labeled with anti-CD63/EpCAM/MUC1 DNA aptamers, and then followed by rolling circle amplification (RCA) to amplify local fluorescence signals. By analyzing the heterogeneity of these individual EVs, the high-dimensional data collected from each EV provides more comprehensive information than bulk analysis like ELISA. Intriguingly, a notably higher proportion of CD63/EpCAM/MUC1 triple-positive EVs was found in breast cancer patients compared to healthy donors. The method impressively achieved an overall accuracy of 91% for breast cancer diagnosis. Furthermore, DPPIE has the ability to discriminate between EVs derived from patients with lung squamous and lung squamous cell carcinoma. This innovative approach to analyzing individual EV heterogeneity presents promising avenues for uncovering extensive information of EVs, advancing multi-cancer diagnosis and classification.
3.4 DNA nanostructure
DNA nanostructures are artificial nanoscale constructs created by self-assembly of DNA molecules as building blocks.87,88 These structures can be precisely engineered for a specific size, structure and functionality for application across various research fields including biosensors,89 nanodevices,90 bioimaging,91 and drug delivery.92 The ease of functionalization and high programmability make DNA nanostructures an appealing platform to improve assay sensitivity and specificity. In this section, we explore the versatile applications of DNA nanostructures in the profiling of protein and RNA biomarkers of EVs.
In the field of EV biomarker detection, DNA nanostructures have served as a signal amplifier for protein immunoassays. Recently, Guo et al. reported a novel signal amplification technique to examine individual EV surface proteins using a DNA nanostructure (Fig. 4(A)).93 To enhance the assay sensitivity, antibodies are conjugated with a circular probe for RCA and are used for targeting the EV protein markers. Once single-strand DNA (ssDNA) is elongated on the EV surface through RCA, these single-stranded RCA products spontaneously assembled into a compact DNA nanostructure, called a DNA nanoflower (DNF). By incorporating biotinylated dATP during the RCA process, the DNF can be labeled with streptavidin conjugated fluorochromes. This DNF approach not only amplifies the fluorescent signals but also enlarges the size of the EV-DNF complex, enabling easy visualization of individual EVs via confocal imaging.
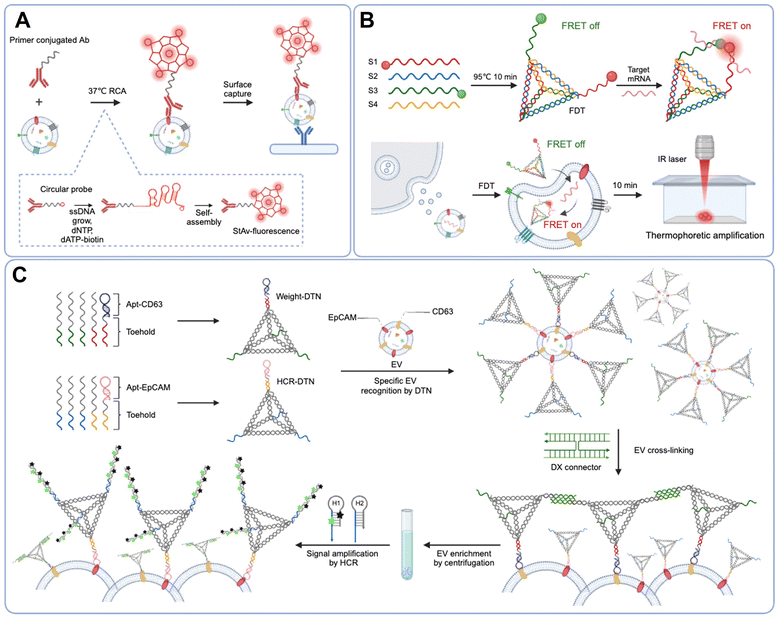 |
| Fig. 4 DNA nanostructures in EV profiling. (A) Scheme of DNA nanoflower (DNF). A long ssDNA is elongated on the EV surface via RCA and then self-assembles into a compact DNA nanostructure named DNF. (B) DNA tetrahedron-based thermophoretic assay (DTTA) for EV mRNA detection. Upon binding of the target mRNA, the FRET signal is generated within the FRET-based DNA tetrahedron (FDT). The thermophoretic effect amplifies the assay signal by accumulating the EVs into the cold-spot region. (C) DNA-nanoweight-assisted centrifugation for efficient EV enriching and profiling. EVs are enriched through weight-DTN-driven cross-linking. The HCR-DTN recognizes EV surface proteins via aptamers, and the signal is further amplified through HCR. | |
Detecting EV mRNAs remains challenging due to their low abundance and the complex detection procedures.94 Conventional methods of EV mRNA analysis mostly rely on RT-PCR.95 However, given the low abundance of EV mRNA, conventional RT-PCR approaches often require a substantial amount of samples and involve time-consuming procedures to extract intravesicular EV mRNAs. To overcome these challenges, Han et al. developed an ultrasensitive assay for in situ measurement of EV mRNA by combining DNA nanostructure and thermophoretic accumulation (Fig. 4(B)).96 They designed a Förster resonance energy transfer (FRET)-based DNA tetrahedron (FDT) probe, designed to passively penetrate EVs and to bind prostate-specific antigen (PSA) mRNAs within them. When the target mRNAs are recognized, the FRET signal is activated due to the proximity of Cy3 and Cy5 fluorophores. They further enhanced the analytical signal by combining the thermphoretic effect, initiated by a laser-induced local temperature gradient to accumulate EVs into the cold-spot region. This DNA tetrahedron-based thermophoretic assay (DTTA) allows ultrasensitive detection of PSA mRNA in serum EVs, with a detection limit of 14 aM.
The DNA nanostructure also provides a promising solution for the efficient enrichment of cancer-derived EVs from heterogeneous biofluids. Using DNA tetrahedral nanostructures (DTNs), Lu et al. developed a fast and specific EV enrichment method based on DNA-nanoweight-assisted centrifugation (Fig. 4(C)).97 In their approach, EVs are first recognized with two distinct DTNs conjugated with either a CD63 aptamer (weight-DTN) or an EpCAM aptamer (HCR-DTN). Once EVs are bound to weight-DTN, they are cross-linked by hybridizing the weight-DTN with a double crossover (DX) connector. This process facilitates the enrichment of CD63-positive EVs through a subsequent low-speed centrifugation step. After EV enrichment, an HCR is initiated by introducing hairpin probes targeting the HCR-DTN. This results in spatial separation between the fluorophore (FAM) and its quencher (BHQ), leading to fluorescence signal amplification in proportion to the level of CD63 and EpCAM dual positive EVs. Remarkably, this dual-aptamer DNA-nanoweight system achieves 1000 times enhancement of detection sensitivity compared to conventional ELISA. Moreover, the simultaneous recognition of multiple surface proteins on EVs improves assay specificity by effectively removing interference from free proteins.
3.5 EV–liposome fusion
EV–liposome fusion technologies have been developed for detecting intra-vesicular RNA cargos. These technologies incorporate RNA sensing components in liposomes (for example, lipid–polymer hybrid nanoparticles,98 fusogenic vesicles99 and lipid nanoparticles100,101) and deliver them directly to EVs through EV–liposome fusion. This strategy allows extraction-free detection of EV RNAs, without labor-intensive and time-consuming procedures of EV RNA extraction and amplification. For sensitive intra-vesicular RNA sensing, various sensing components including molecular beacons99 and catalyzed hairpin DNA circuit (CHDC)98 have been employed. Most recently, CRISPR-Cas sensing systems have been loaded into liposomes and sensitively detect RNAs in EVs. Ning et al. developed a Cas12a based assay for the detection of SARS-CoV-2 RNA-positive EVs in monkey and human plasma.100 EVs in plasma were captured with the CD81 antibody and subsequently fused with liposome containing reverse transcriptase (RT), a primer for N gene, Cas12a protein, and a fluorescence-quencher (FQ) probe. In the fused vesicles, RT mediated recombinase polymerase amplification (RPA) amplifies the target and triggers Cas12a-mediated fluorescent signal enhancement for SARS-CoV-2 diagnosis. Hong et al. showed the potential of multiplexed EV protein-miRNA analysis for more accurate cancer diagnosis (Fig. 5(A)).101 For EV miRNA detection, a liposome having crRNA, Cas13a, and a FQ probe was designed. After the fusion of EV and the liposome, hybridization of target miRNA and crRNA activates Cas13a and subsequently cleaves the FQ probe, which generates fluorescent signals. Furthermore, they integrated a gold microdisk array to the liposome-based miRNA detection system for capturing protein marker-specific EVs and detect miR-21-5p in the captured EVs (Fig. 5(B)). Using this assay, the authors not only successfully differentiated ovarian cancer patients from healthy controls, but also determined that miR-21-5p-positive and EpCAM-positive subpopulations were significantly higher than the CD63-positive subpopulation in patient samples.
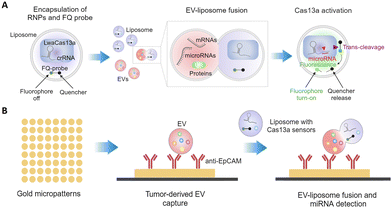 |
| Fig. 5 EV–liposome fusion-based intravesicular RNA profiling. (A) Schematic diagram for the CRISPR/Cas13a-based EV miRNA detection assay. Ribonucleoprotein (RNP) complexes (LwaCas13a and miRNA-targeting crRNA) and fluorescence-quencher (FQ) probes are encapsulated in liposomes and delivered to EVs through EV–liposome fusion. Binding of target miRNAs to crRNA activates LwaCas13a, allowing the cleavage of FQ-probe and turn-on of fluorophore by releasing a quencher. (B) Multiplexed detection of EV protein–RNA using a gold disk array. The surface of the gold disk array is functionalized with antibodies against EV surface proteins such as EpCAM. By introducing the EV–liposome fusion after the EV capture, the assay enables the detection of EV miRNA among the EpCAM-positive EV subpopulation. | |
3.6 Stimuli-responsive hydrogel
Mechanical metamaterials are artificial composites that exhibit characteristics not found in conventional materials.102 They can be precisely designed to produce unique properties suitable for various applications.103–105 Stimuli-responsive metamaterials, especially those sensitive to biological signals, are appealing for the development of biomedical sensing systems.106 Hydrogel-based mechanical metamaterials, in particular, stand out as promising candidates for such biosensing platforms.107–109 Leveraging a range of gel chemistries, hydrogels can be engineered to modulate their mechanical properties in response to external stimuli, such as pH,110,111 temperature112 and ionic strength.113,114 For biomedical applications, many of the affinity ligands (e.g., antibodies, aptamers, and enzymes) can be incorporated into gel-forming polymers. These receptors can trigger physical changes in the hydrogel, like gel formation or deformation, depending in the presence of target molecules.115
Taking those advantages of metamaterials, recent studies have pioneered hydrogel-based optical biosensors for point-of-care testing. For instance, Zhao et al. introduced an optical biosensing platform for molecular profiling of EVs utilizing hydrogel-based mechanical metamaterials.116 This technology, termed MORPH (mechanical metamaterial operating at a critical point for hyper-responsive analysis), employs a temperature and redox reaction responsive hydrogel, acting as a shape-transforming chiral interferometer (Fig. 6). The hydrogel, covalently patterned onto a gold-coated glass substrate, is incorporated with antibodies to capture target biomolecules. Light-emitting diode (LED) illumination provides plasmonic heating, tuning the metamaterial to a hyper-responsive state, often referred to as the ‘critical-point’. When EVs bind to the antibodies, they generate free radicals through horseradish peroxidase (HRP) activity to induce localized hydrogel crosslinking. This HRP mediated crosslinking induces a chiral transformation of the metamaterial, leading to mechanical deformation followed by diffraction pattern alterations. These changes can be readily detected optically, reflecting the biomarker composition. Additionally, the team developed a microfluidic system paired with a smartphone-based optical detector for the analysis of these diffraction patterns, enhancing the point-of-care applicability of MORPH. When tested with clinical samples from cancer patients, this system achieved a sensitivity surpassing conventional ELISA measurements by a thousand-fold, effectively detecting putative cancer markers (CD24, EpCAM, and MUC1) and the EV marker (CD63).
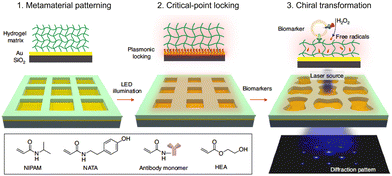 |
| Fig. 6 Schematic of a mechanical metamaterial operating at a critical point for the hyper-responsive analysis (MORPH) system. Figure reproduced from ref. 112 with permission from Springer Nature. | |
4. Analysis of EV heterogeneity
4.1 Cyclic imaging
Current EV analytical technologies for diagnosis predominantly rely on bulk EV analysis rather than single EV analysis due to their small size.117 However, bulk EV analysis necessarily loses valuable information related to EV biology, tumor heterogeneity and rare tumor subtypes.118,119 To address these issues, there is a growing interest in developing single EV analysis technologies using spectral sensing methods. These methods include optical microscopy,72,120 Raman spectroscopy,121 flow cytometry,122 and super-resolution microscopy.123
Recently, chemical biology tools for cyclic imaging have been implemented for multiplexed analyses of single EVs to unravel their heterogeneous composition. For example, Lee et al. developed a light microscopic single EV analysis (SEA) technique for multiplexed detection of protein biomarkers in individual vesicles.124 Adapting image cycling methods previously employed for multiplexed cyclic cell and tissue analyses, the authors developed a microfluidic device. This device immobilizes EVs on the chip surface through a non-covalent affinity binding between biotinylated-EVs and NeutrAvidin (Fig. 7(A)). This immobilization ensures the retention of EVs on a glass slide through multiple cycles, allowing for on-chip cyclic imaging. In each cycle, EVs are stained with three different fluorescently labeled antibodies. After acquiring multi-channel fluorescence images, the fluorochromes are quenched with hydrogen peroxide, before proceeding to the next round of cycling imaging. After completing four rounds of multi-channel fluorescence staining, twelve protein biomarkers were profiled in individual vesicles. Using this technique, the authors validated the markedly heterogeneous profiles of EV biomarkers across three isogenic glioblastoma multiforme (GBM) cell lines.
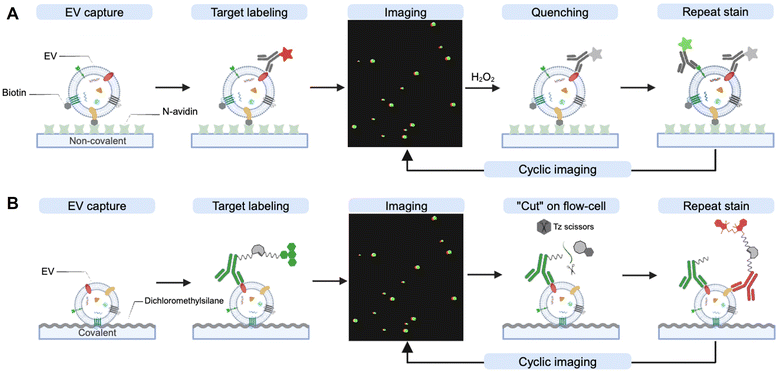 |
| Fig. 7 Cyclic imaging for multiplexed analysis of individual EVs. (A) Single EV analysis (SEA). Biotinylated EVs are immobilized on an NeutrAvidin coated microfluidic device and subsequently tagged with fluorescently labeled antibodies. After imaging, the fluorescence is quenched using hydrogen peroxide, allowing for repeated labeling with different markers. (B) Multiplexed analysis of EV technique (MASEV). EVs are captured on a dichloromethylsilane-coated flow chamber and stained using fluorescently labeled antibodies with a cleavable TCO linker. The tetrazine scissor subsequently releases the fluorochromes, enabling repetitive labeling with different markers. | |
Recent advances in bioorthogonal chemistry have further improved EV multiplexing technology. Spitzberg et al. employed an innovative bioorthogonal cleavable linker between an antibody and a fluorochrome.125 This linker contains a TCO moiety that is readily cleaved upon the addition of a functionalized tetrazine scissor (Fig. 7(B)). Its fast kinetics and orthogonality provide a quicker and gentler destaining strategy compared to the SEA protocol, which relies on fluorochrome bleaching with hydrogen peroxide. Moreover, the authors developed a simple flow chamber designed to covalently capture EVs by functionalizing the cover glass with dichlorodimethylsilane. Using this multiplexed analysis of EV technique (MASEV), they profiled 15 protein biomarkers across thousands of individual EVs.
4.2 Immuno-PCR and immuno-sequencing
Antibody-DNA based EV profiling can increase the multiplexing and throughput through sequencing (immuno-sequencing) and PCR (immuno-PCR). This approach requires the efficient conjugation of antibodies to oligonucleotides, such as DNA barcodes, to translate protein identities into DNA sequences.126 A number of chemical biology tools have been employed to produce antibody–DNA conjugates.127–129 These tools span non-covalent strategies like biotin–streptavidin coupling127 and covalent strategies such as maleimide chemistry, NHS ester chemistry and a variety of bioorthogonal chemistries.128 In addition, the incorporation of non-natural amino acids facilitates site-specific DNA conjugation, enhancing the specificity and sensitivity of antibody–DNA conjugates.129 The inclusion of cleavable disulfide crosslinker enables the release of DNA barcodes, allowing for efficient barcode detection via PCR or sequencing.130 Recently, numerous studies have adopted DNA barcode-based immunoassay technologies to explore protein profiles in EVs.131–133 Moreover, advanced assay strategies that merge immuno-sequencing with the partitioning of individual EVs allow for multiplexed profiling of proteins within individual EVs (Fig. 8).
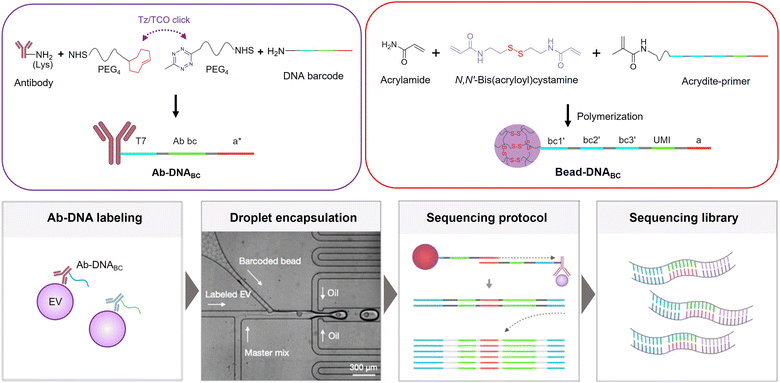 |
| Fig. 8 SeiSEQ technology for multiplexed analysis of EV proteins via immunosequencing. Ab-DNA barcodes are prepared by bioorthogonal conjugation between DNA barcode and antibody. Antibody and DNA barcodes are functionalized with Tz/TCO functional groups via NHS ester chemistry. DNA barcodes are immobilized on beads via acrylamide polymerization. A reducing agent (e.g., DTT) dissolves beads and rapidly releases barcode primers. The pipeline includes single EV labeling with Ab-DNABC, droplet encapsulation with bead-DNABC, PCR amplification, and sequencing protocol. | |
For example, Ko et al. developed a single EV immuno-sequencing method (seiSEQ) that allows multiplexed profiling of proteins within individual EVs.134 This technology labels EVs with antibody–DNA barcodes and compartmentalizes them into droplets along with barcoded beads via droplet microfluidics. Using different barcodes to define protein types (Ab-DNABC) and individual EVs (Bead-DNABC), sequencing of DNA barcodes enables the measurement of multiple proteins in individual EVs. In this approach, the authors utilized dissolvable polyacrylamide cross-linked beads for Bead-DNABC to maximize the assay efficiency. These beads, containing DNA barcodes, are constructed by crosslinking acrylamide with disulfide bonds. As a result, they rapidly dissolve when treated with DTT, releasing the DNA barcode primers. This strategy enhances the reaction efficiency of barcodes compared to conventional beads that are immobilized with DNA barcodes.
5. EV glycoprotein analysis
5.1 Metabolic glycoengineering
Metabolic glycoengineering (MGE) of cells with bioorthogonal chemical groups provides a tool for engineering the surface of EVs. The surface engineering of EVs aids in visualizing EVs, tracking their distribution both in vitro and in vivo, loading drugs in EVs, and guiding programmed EV delivery.135,136 Conventional strategies, like covalent surface modification using NHS ester, often result in protein charge shift and protein 3D structure transition, which could lead to dysfunction of EVs.137 Lipid dyes (for example, DiO/DiI, PKH67, MemGlow, ExoBrite) are also often used for visualizing EVs.138,139 The lipophilic nature of the dyes allows them to be incorporated into the lipid bilayer of the EVs. However, the dyes are easily aggregated during the labeling process and form non-EV particles, which are hard to separate from EVs, complicating the downstream applications.138,140 For universal EV labeling, fluorescent proteins (FPs) also can be genetically encoded to EV cargos such as tetraspanin family members (CD63, CD9 and CD9).141,142 This genetic labeling allows tracking and visualizing the EV's biogenesis, secretion, biodistribution and uptake in living cells and animals. However, genetic incorporation of large reporter proteins may disturb cellular signaling pathways for EV biogenesis and trafficking.
Alternatively, a bioorthogonal azide group can be incorporated into the EV surface through the MGE-mediated sialic acid metabolic pathway via incubation with tetraacetyl-N-azidoacetylmannosamine (Ac4ManNAz) without perturbation of innate protein properties.143,144 In this approach, azide groups are first introduced to the cellular surface proteins and subsequently incorporated into EVs through the endogenous EV biogenesis pathway. After the MGE, there are two main approaches for functionalizing the engineered EVs; in situ and ex situ labeling using dibenzocyclooctyne (DBCO) (Fig. 9).
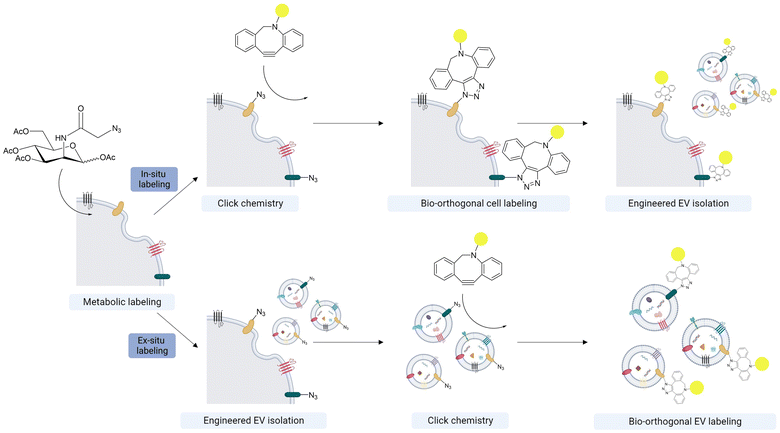 |
| Fig. 9 Metabolic labeling of EVs via glycoprotein engineering. Using Ac4ManNAz, bioorthogonal azide groups are incorporated onto the cell surface. In the in situ pathway, the cell surface is labeled with fluorochrome- or substrate-functionalized DBCO before secreting the modified EVs. The ex situ pathway first isolates engineered EVs and subsequently modifies them with DBCO-conjugated functional moieties. | |
In situ labeling involves the direct introduction of DBCO-conjugate functional moieties into cells to generate the functionalized EVs. Fluorochrome-conjugated DBCOs are widely used to fluorescently label the cell-derived EVs for both in vitro and in vivo tracking.145,146 This in situ labeling method can produce a large amount of functionalized EVs without dysfunction. In contrast, genetic engineering with fluorescent proteins or bioluminescence reporters, which is prevalent in the field, can impair biogenesis and influence gene expression in donor cells. Additionally, in situ labeling does not require a time-consuming purification process, such as ultracentrifugation and size exclusion chromatography, to remove unreacted DBCO compounds. Utilizing this approach, Song et al. generated Cy5-conjugated EVs derived from human lung adenocarcinoma (A549) cells and verified their tumor homing ability through in vivo tracking in mice bearing A549 tumors.145 Furthermore, incorporating cell-specific targeting moieties can enhance the potential of EV-based, cell-free therapeutics. You et al. reported a precise surface editing technique to deliver mesenchymal stem cell (MSC)-derived EVs for rheumatoid arthritis (RA) treatment.146 The team incorporated a macrophage-targeting moiety onto the adipose-derived stem cell (ADSC)-derived EVs via an in situ metabolic labeling strategy. These engineered MSC-derived EVs showed higher therapeutic efficacy compared to unmodified EVs, achieving a programmed EV delivery to activated macrophages in the inflamed joints of RA.
Alternatively, the ex situ labeling approach involves first isolating the metabolically engineered EVs and then labeling these EVs with DBCO-conjugated functional groups through click chemistry. While this approach requires an additional isolation step, it provides an opportunity to functionalize the EVs with a broader array of functional groups, including inorganic materials and large biomolecules.147,148 For example, Xing et al. engineered MSC-derived EVs with an azide group through MGE and then labeled these EVs with a DBCO-conjugated collagen hydrogel.148 By immobilizing MSC-derived EVs on the collagen hydrogel, prolonged in vivo retention of the EVs was achieved. This setup improved in vivo angiogenic activity of the MSC-derived EVs compared to their non-immobilized counterparts.
6. Treatment response monitoring
6.1 EV drug occupancy analysis
In the past decade, EVs have gained significant attention for their potential as biomarkers for the treatment response.149 Profiling their protein and RNA contents offers insights into disease progression, treatment responses, and mechanisms of resistance.14,150,151 While the field continues to evolve, Pan et al. introduced a novel innovative strategy to monitor cancer treatment by evaluating drug-target interactions in circulating EVs.152 The authors developed a technology, named extracellular vesicle monitoring of small-molecule chemical occupancy and protein expression (ExoSCOPE). This technology utilizes bioorthogonal probe amplification and spatial patterning of molecular reactions. It measures EV drug occupancy through the competitive target protein binding of bioorthogonal click probes and their amplified detection via the deposition of insoluble optical products (Fig. 10). By assessing EV drug occupancy, ExoSCOPE evaluates the cellular treatment potency of various EGFR inhibitors, including afatinib, Osimertinib, and erlotinib. Through the monitoring of EV drug occupancy in clinical samples, ExoSCOPE successfully evaluated the treatment outcomes from lung cancer patients undergoing erlotinib treatment. Overall, this study showcases the technological advances in EV profiling for activity-based monitoring of drug–target interaction.
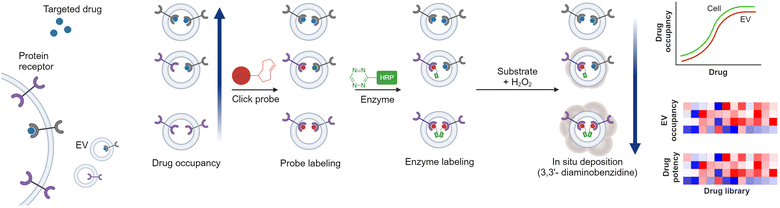 |
| Fig. 10 Schematic of ExoSCOPE for EV-drug occupancy analysis. | |
7. Conclusions and perspectives
In the past decade, extracellular vesicles (EVs) have been in the spotlight as an emerging biomarker for liquid biopsy and as a regulator of biological phenomenon. Therefore, EV profiling via the non-invasive liquid biopsy is developing very rapidly not only as an attractive diagnostic method, but also as a monitoring tool for cellular processes. Although various EV assays have been developed, isolation and sensitive analysis of EVs from biofluids remain a challenge. From the perspective of clinical diagnostics, the time-consuming process of EV purification presents obstacles to their effective utilization in robust clinical application. In addition, limited sensitivity for low abundant target EVs is one of the big hurdles for early diagnosis.153
Recently, chemical biology tools have been harnessed to increase the isolation efficiency, detection sensitivity, and multiplexing analysis capacity of EVs. Here, we review how chemical biology tools have been applied in various EV isolation and analysis platforms in order to increase their efficiency. We introduced an efficient and robust EV enrichment strategy based on immunoaffinity isolation. In particular, we highlight the recent applications that integrate click chemistry with immunoaffinity isolation, facilitating the rapid and selective EV enrichment. Next, we review various chemical biology tools for signal amplification and multiplexed analysis to enhance the assay sensitivity and capture EV heterogeneity. Finally, we explore recent advancements in the field of EV profiling, such as EV visualization, biosensing platform and treatment response monitoring. These approaches demonstrated the importance of interdisciplinary collaboration for the breakthrough in EV research. Moreover, these approaches also provide the following new insights of EV biology; (1) EVs are highly heterogenous. Each EV subpopulation has distinct molecular information. (2) In vivo destination of EVs can be decided by surface modification. (3) Drug occupied proteins are enclosed to EVs, which are related to the drug response of cancer patients.
Hierarchical step-by-step employment of chemical biology tools assisted in revealing the above mentioned EV biology as follows. For example, the Weissleder and Lee group has developed efficient isolation of biomolecules using Tz-TCO click chemistry,14,77 which is now widely used for EV isolations. They harnessed the same Tz-TCO click reaction for image cycling to understand heterogeneity of EVs.121 Smart structural modification of TCO realized chemical bond cleavage with remarkable yield for successful multiplexed EV imaging. They again utilized Tz-TCO for single EV analysis by combining droplet encapsulations and immunosequencing. Shao et al. further hired Tz-TCO click chemistry to monitor drug occupancy of target proteins in EVs for the first time.143 Another example is the utilization of enzyme based radical reactions for signal amplification. The Lee and Weissleder group developed the SAViA and DEST assay using HRP based radical reaction for tyramide polymerization mediated signal amplification.70,82 The Shao group applied HRP based radical reaction for the deposition of metal154 or insoluble optical products143 to improve the sensitivity of EV analysis. The Shao group also achieved excellent EV detection sensitivity by mechanical transition of a metamaterial using a HRP based radical reaction.112 These various smart applications of existing chemical biology tools suggest new opportunities in EV biology.
Despite the above mentioned recent advances in EV analysis, there are still multiple issues to be solved for bringing EV profiling to real clinics. (i) Discovery of disease specific EV markers is urgently required.155 EV profiling-based diagnosis has been commonly demonstrated for a specifically targeted disease instead of differentiating multiple diseases due to the lack of disease-specific EV markers. A combination of previously reported disease markers in cell lines or tissues has been widely used to increase the accuracy of EV based disease diagnosis.125 Due to this limitation, differential diagnosis of multiple diseases was barely reported. (ii) Another limitation of EV profiling-based research is the size of patient cohort for validation.156 Mostly EV analysis for disease diagnosis is validated in small patient cohorts.157–159 To claim the potential clinical significance, EV based disease diagnosis should be demonstrated in the large size of patient cohorts. (iii) Most of the current EV analysis technologies are high-cost device dependent assays, which need well-trained technicians.57 Considering the situation of real clinics, the development of easily accessible EV assays with low resource setting is highly in demand. (iv) Ethical issues of EV research should be considered during the analysis and diagnosis processes. The personal information of each patient must be handled securely, and de-identification methods need to be employed for the protection of patient information. Moreover, false positives and negatives of EV profiling-based diagnosis can contribute to unnecessary stress, or treatments of patients. Enhancing the accuracy of EV profiling based diagnosis would address this ethical issue.160 Researchers need to be aware of these ethical issues and manage those concerns in clinical applications.
Considering the above mentioned current contributions of chemical biology tools in EV research, we believe further understanding of EV biology can be achieved by accelerating the interdisciplinary collaboration between chemical biology and biomedical research. Specifically, more precise separation of EV subpopulations could provide more exact information of EV cargoes. By increasing the power of multiplexing, the heterogeneity of EV atlas would be unveiled. The increase in EV assay sensitivity could achieve very early diagnosis through the detection of a small amount of disease related EVs. However, only a limited number of chemical biology tools have been used to tackle those issues.82,124,125,134 To shed light on the unexplored territory of EV biology, we believe that the biggest challenge of chemical biology in EV research is to develop new bioorthogonal chemical reactions with superior efficiency. We anticipate that these future advancements in chemical biology tools will offer promising avenues for innovating the field of EV profiling. Chemical biology tools would be further engineered for nanoscale targets, addressing the issue of sensitivity, specificity, and EV heterogeneity. Moreover, standardizing the analytical protocol will make EV profiling robust, facilitating the translational application of EV profiling in real-world clinical diagnostics.
Author contributions
All authors contributed to writing and editing the manuscript.
Conflicts of interest
There are no conflicts to declare.
Acknowledgements
This work is supported by the Basic Science Research Program (2023R1A2C1007899 to J. P., 2022R1C1C2008563 to A. J.) and Priority Research Institute Program (RS-2023-00271205 to J. P.) through the National Research Foundation of Korea funded by the Ministry of Science, ICT & Future Planning. This work is also supported by a Korea Basic Science Institute (KBSI) National Research Facilities & Equipment Center (NFEC) grant (2019R1A6C1010006 to J. P.) and Learning & Academic research institution for Master's·PhD students, and Postdocs (LAMP) program (RS-2023-00301850 to J. P.) from the Ministry of Education, South Korea. This work is supported by a 2019 Research Grant from Kangwon National University. Figures were created with BioRender.com.
Notes and references
- C. Théry, L. Zitvogel and S. Amigorena, Nat. Rev. Immunol., 2002, 2, 569–579 CrossRef PubMed.
- C. Théry, M. Ostrowski and E. Segura, Nat. Rev. Immunol., 2009, 9, 581–593 CrossRef PubMed.
- G. Raposo and W. Stoorvogel, J. Cell Biol., 2013, 200, 373–383 CrossRef CAS PubMed.
- M. Colombo, G. Raposo and C. Théry, Annu. Rev. Cell Dev. Biol., 2014, 30, 255–289 CrossRef CAS PubMed.
- J. C. Akers, D. Gonda, R. Kim, B. S. Carter and C. C. Chen, J. Neuro-Oncol., 2013, 113, 1–11 CrossRef PubMed.
-
K. R. Feingold, in Endotext, ed. K. R. Feingold, B. Anawalt, M. R. Blackman, A. Boyce, G. Chrousos, E. Corpas, W. W. de Herder, K. Dhatariya, K. Dungan, J. Hofland, S. Kalra, G. Kaltsas, N. Kapoor, C. Koch, P. Kopp, M. Korbonits, C. S. Kovacs, W. Kuohung, B. Laferrère, M. Levy, E. A. McGee, R. McLachlan, M. New, J. Purnell, R. Sahay, A. S. Shah, F. Singer, M. A. Sperling, C. A. Stratakis, D. L. Trence and D. P. Wilson, MDText.com, Inc., SouthDartmouth (MA), January 14, 2024.
- N. S. Barteneva, E. Fasler-Kan, M. Bernimoulin, J. N. Stern, E. D. Ponomarev, L. Duckett and I. A. Vorobjev, BMC Cell Biol., 2013, 14, 23 CrossRef CAS PubMed.
- H. P. Nguyen, R. J. Simpson, L. A. Salamonsen and D. W. Greening, Biol. Reprod., 2016, 95, 109 CrossRef PubMed.
- P. Edwin van der, N. B. Anita, H. Paul, S. Augueste and N. Rienk, Pharmacol. Rev., 2012, 64, 676 CrossRef PubMed.
- E. Willms, C. Cabañas, I. Mäger, M. J. A. Wood and P. Vader, Front. Immunol., 2018, 9, 738 CrossRef PubMed.
- D. K. Jeppesen, A. M. Fenix, J. L. Franklin, J. N. Higginbotham, Q. Zhang, L. J. Zimmerman, D. C. Liebler, J. Ping, Q. Liu, R. Evans, W. H. Fissell, J. G. Patton, L. H. Rome, D. T. Burnette and R. J. Coffey, Cell, 2019, 177, 428–445 CrossRef CAS PubMed.
- G. van Niel, D. R. F. Carter, A. Clayton, D. W. Lambert, G. Raposo and P. Vader, Nat. Rev. Mol. Cell Biol., 2022, 23, 369–382 CrossRef CAS PubMed.
- C. Théry, F1000 Biol. Rep., 2011, 3, 15 Search PubMed.
- H. Shao, J. Chung, L. Balaj, A. Charest, D. D. Bigner, B. S. Carter, F. H. Hochberg, X. O. Breakefield, R. Weissleder and H. Lee, Nat. Med., 2012, 18, 1835–1840 CrossRef CAS PubMed.
- D. Choi, C. Spinelli, L. Montermini and J. Rak, Proteomics, 2019, 19, e1800169 CrossRef PubMed.
- J. Skog, T. Würdinger, S. van Rijn, D. H. Meijer, L. Gainche, W. T. Curry, B. S. Carter, A. M. Krichevsky and X. O. Breakefield, Nat. Cell Biol., 2008, 10, 1470–1476 CrossRef CAS PubMed.
- H. Shao, J. Chung, K. Lee, L. Balaj, C. Min, B. S. Carter, F. H. Hochberg, X. O. Breakefield, H. Lee and R. Weissleder, Nat. Commun., 2015, 6, 6999 CrossRef CAS PubMed.
- Y. Yoshioka, N. Kosaka, Y. Konishi, H. Ohta, H. Okamoto, H. Sonoda, R. Nonaka, H. Yamamoto, H. Ishii, M. Mori, K. Furuta, T. Nakajima, H. Hayashi, H. Sugisaki, H. Higashimoto, T. Kato, F. Takeshita and T. Ochiya, Nat. Commun., 2014, 5, 3591 CrossRef PubMed.
- T. Skotland, K. Sandvig and A. Llorente, Prog. Lipid Res., 2017, 66, 30–41 CrossRef CAS PubMed.
- M. Puhka, M. Takatalo, M.-E. Nordberg, S. Valkonen, J. Nandania, M. Aatonen, M. Yliperttula, S. Laitinen, V. Velagapudi, T. Mirtti, O. Kallioniemi, A. Rannikko, P. R. M. Siljander and T. M. af Hällström, Theranostics, 2017, 7, 3824–3841 CrossRef CAS PubMed.
- M. Yáñez-Mó, P. R. Siljander, Z. Andreu, A. B. Zavec, F. E. Borràs, E. I. Buzas, K. Buzas, E. Casal, F. Cappello, J. Carvalho, E. Colás, A. Cordeiro-da Silva, S. Fais, J. M. Falcon-Perez, I. M. Ghobrial, B. Giebel, M. Gimona, M. Graner, I. Gursel, M. Gursel, N. H. Heegaard, A. Hendrix, P. Kierulf, K. Kokubun, M. Kosanovic, V. Kralj-Iglic, E. M. Krämer-Albers, S. Laitinen, C. Lässer, T. Lener, E. Ligeti, A. Linē, G. Lipps, A. Llorente, J. Lötvall, M. Manček-Keber, A. Marcilla, M. Mittelbrunn, I. Nazarenko, E. N. Nolte-’t Hoen, T. A. Nyman, L. O’Driscoll, M. Olivan, C. Oliveira, É. Pállinger, H. A. Del Portillo, J. Reventós, M. Rigau, E. Rohde, M. Sammar, F. Sánchez-Madrid, N. Santarém, K. Schallmoser, M. S. Ostenfeld, W. Stoorvogel, R. Stukelj, S. G. Van der Grein, M. H. Vasconcelos, M. H. Wauben and O. De Wever, J. Extracell. Vesicles, 2015, 4, 27066 CrossRef PubMed.
- M. Eldh, K. Ekström, H. Valadi, M. Sjöstrand, B. Olsson, M. Jernås and J. Lötvall, PLoS One, 2010, 5, e15353 CrossRef PubMed.
- A. Takahashi, R. Okada, K. Nagao, Y. Kawamata, A. Hanyu, S. Yoshimoto, M. Takasugi, S. Watanabe, M. T. Kanemaki, C. Obuse and E. Hara, Nat. Commun., 2017, 8, 15287 CrossRef CAS PubMed.
- V. Cantaluppi, L. Biancone, F. Figliolini, S. Beltramo, D. Medica, M. C. Deregibus, F. Galimi, R. Romagnoli, M. Salizzoni, C. Tetta, G. P. Segoloni and G. Camussi, Cell Transplant., 2012, 21, 1305–1320 Search PubMed.
- C. A. Escudero, K. Herlitz, F. Troncoso, J. Acurio, C. Aguayo, J. M. Roberts, G. Truong, G. Duncombe, G. Rice and C. Salomon, Front. Physiol., 2016, 7, 98 Search PubMed.
- C. Marar, B. Starich and D. Wirtz, Nat. Immunol., 2021, 22, 560–570 CrossRef CAS PubMed.
- A. Becker, B. K. Thakur, J. M. Weiss, H. S. Kim, H. Peinado and D. Lyden, Cancer Cell, 2016, 30, 836–848 CrossRef CAS PubMed.
- E. N. Nolte-’t Hoen and M. H. Wauben, Curr. Pharm. Des., 2012, 18, 2357–2368 CrossRef PubMed.
- E. I. Buzas, B. György, G. Nagy, A. Falus and S. Gay, Nat. Rev. Rheumatol., 2014, 10, 356–364 CrossRef CAS PubMed.
- H. Shao, H. Im, C. M. Castro, X. Breakefield, R. Weissleder and H. Lee, Chem. Rev., 2018, 118, 1917–1950 CrossRef CAS PubMed.
- R. J. Simpson, J. W. Lim, R. L. Moritz and S. Mathivanan, Expert Rev. Proteomics, 2009, 6, 267–283 CrossRef CAS PubMed.
- J. Park, J. S. Park, C.-H. Huang, A. Jo, K. Cook, R. Wang, H.-Y. Lin, J. Van Deun, H. Li, J. Min, L. Wang, G. Yoon, B. S. Carter, L. Balaj, G.-S. Choi, C. M. Castro, R. Weissleder and H. Lee, Nat. Biomed. Eng., 2021, 5, 678–689 CrossRef CAS PubMed.
- J. Park, H.-Y. Lin, J. P. Assaker, S. Jeong, C.-H. Huang, A. Kurdi, K. Lee, K. Fraser, C. Min, S. Eskandari, S. Routray, B. Tannous, R. Abdi, L. Riella, A. Chandraker, C. M. Castro, R. Weissleder, H. Lee and J. R. Azzi, ACS Nano, 2017, 11, 11041–11046 CrossRef CAS PubMed.
- N. Younas, L. C. Fernandez Flores, F. Hopfner, G. U. Höglinger and I. Zerr, Transl. Neurodegener., 2022, 11, 28 CrossRef PubMed.
- Y. Liu and Q. Lu, Biomark. Med., 2015, 9, 791–805 CrossRef CAS PubMed.
- H. Peinado, M. Alečković, S. Lavotshkin, I. Matei, B. Costa-Silva, G. Moreno-Bueno, M. Hergueta-Redondo, C. Williams, G. García-Santos, C. M. Ghajar, A. Nitadori-Hoshino, C. Hoffman, K. Badal, B. A. Garcia, M. K. Callahan, J. Yuan, V. R. Martins, J. Skog, R. N. Kaplan, M. S. Brady, J. D. Wolchok, P. B. Chapman, Y. Kang, J. Bromberg and D. Lyden, Nat. Med., 2012, 18, 883–891 CrossRef CAS PubMed.
- E. Kilgour, D. G. Rothwell, G. Brady and C. Dive, Cancer Cell, 2020, 37, 485–495 CrossRef CAS PubMed.
- U. Erdbrügger, C. J. Blijdorp, I. V. Bijnsdorp, F. E. Borràs, D. Burger, B. Bussolati, J. B. Byrd, A. Clayton, J. W. Dear, J. M. Falcón-Pérez, C. Grange, A. F. Hill, H. Holthöfer, E. J. Hoorn, G. Jenster, C. R. Jimenez, K. Junker, J. Klein, M. A. Knepper, E. H. Koritzinsky, J. M. Luther, M. Lenassi, J. Leivo, I. Mertens, L. Musante, E. Oeyen, M. Puhka, M. E. Van Royen, C. Sánchez, C. Soekmadji, V. Thongboonkerd, V. Van Steijn, G. Verhaegh, J. P. Webber, K. Witwer, P. S. T. Yuen, L. Zheng, A. Llorente and E. S. Martens-Uzunova, J. Extracell. Vesicles, 2021, 10, e12093 CrossRef PubMed.
- C. Grange and B. Bussolati, Nat. Rev. Nephrol., 2022, 18, 499–513 CrossRef PubMed.
- R. Quiroz-Baez, K. Hernández-Ortega and E. Martínez-Martínez, Front. Neurol., 2020, 11, 580030 CrossRef PubMed.
- R. Upadhya and A. K. Shetty, Aging Dis., 2021, 12, 1438 CrossRef PubMed.
- M. Verma, T. K. Lam, E. Hebert and R. L. Divi, BMC Clin. Pathol., 2015, 15, 6 CrossRef PubMed.
- G. Huang, G. Lin, Y. Zhu, W. Duan and D. Jin, Lab Chip, 2020, 20, 2423–2437 RSC.
- P. Zhang, J. C. Yeo and C. T. Lim, SLAS Technol., 2019, 24, 477–488 CrossRef CAS PubMed.
- D. W. Greening and R. J. Simpson, Expert Rev. Proteomics, 2018, 15, 887–910 CrossRef CAS PubMed.
- A. Turchinovich, O. Drapkina and A. Tonevitsky, Front. Immunol., 2019, 10, 202 CrossRef CAS PubMed.
- J. P. Nolan and E. Duggan, Methods Mol. Biol., 2018, 1678, 79–92 CrossRef CAS PubMed.
- S. C. Guo, S. C. Tao and H. Dawn, J. Extracell. Vesicles, 2018, 7, 1508271 CrossRef CAS PubMed.
- I. Vitale, E. Shema, S. Loi and L. Galluzzi, Nat. Med., 2021, 27, 212–224 CrossRef CAS PubMed.
- R. C. Pink, E. M. Beaman, P. Samuel, S. A. Brooks and D. R. F. Carter, Br. J. Cancer, 2022, 126, 323–330 CrossRef CAS PubMed.
- A. Gyuris, J. Navarrete-Perea, A. Jo, S. Cristea, S. Zhou, K. Fraser, Z. Wei, A. M. Krichevsky, R. Weissleder, H. Lee, S. P. Gygi and A. Charest, Cell Reports, 2019, 27, 3972–3987 CrossRef CAS PubMed.
- C. Alix-Panabières and K. Pantel, Nat. Rev. Cancer, 2014, 14, 623–631 CrossRef PubMed.
- P. Song, L. R. Wu, Y. H. Yan, J. X. Zhang, T. Chu, L. N. Kwong, A. A. Patel and D. Y. Zhang, Nat. Biomed. Eng., 2022, 6, 232–245 CrossRef CAS PubMed.
- H. Schwarzenbach, D. S. B. Hoon and K. Pantel, Nat. Rev. Cancer, 2011, 11, 426–437 CrossRef CAS PubMed.
- S. M. Hanash, S. J. Pitteri and V. M. Faca, Nature, 2008, 452, 571–579 CrossRef CAS PubMed.
- L. M. Doyle and M. Z. Wang, Cells, 2019, 8, 727 CrossRef CAS PubMed.
- M. I. Ramirez, M. G. Amorim, C. Gadelha, I. Milic, J. A. Welsh, V. M. Freitas, M. Nawaz, N. Akbar, Y. Couch, L. Makin, F. Cooke, A. L. Vettore, P. X. Batista, R. Freezor, J. A. Pezuk, L. Rosa-Fernandes, A. C. O. Carreira, A. Devitt, L. Jacobs, I. T. Silva, G. Coakley, D. N. Nunes, D. Carter, G. Palmisano and E. Dias-Neto, Nanoscale, 2018, 10, 881–906 RSC.
- L. Ayers, R. Pink, D. R. F. Carter and R. Nieuwland, J. Extracell. Vesicles, 2019, 8, 1593755 CrossRef CAS PubMed.
- J. Park, H. Im, S. Hong, C. M. Castro, R. Weissleder and H. Lee, ACS Photonics, 2018, 5, 487–494 CrossRef CAS PubMed.
- M. E. Warkiani, B. L. Khoo, L. Wu, A. K. P. Tay, A. A. S. Bhagat, J. Han and C. T. Lim, Nat. Protoc., 2016, 11, 134–148 CrossRef CAS PubMed.
- Y. Chen, Q. Zhu, L. Cheng, Y. Wang, M. Li, Q. Yang, L. Hu, D. Lou, J. Li, X. Dong, L. P. Lee and F. Liu, Nat. Methods, 2021, 18, 212–218 CrossRef CAS PubMed.
- Z. Wang, H.-J. Wu, D. Fine, J. Schmulen, Y. Hu, B. Godin, J. X. J. Zhang and X. Liu, Lab Chip, 2013, 13, 2879 RSC.
- K. Brennan, K. Martin, S. P. Fitzgerald, J. O’Sullivan, Y. Wu, A. Blanco, C. Richardson and M. M. Mc Gee, Sci. Rep., 2020, 10, 1039 CrossRef CAS PubMed.
- P. Duong, A. Chung, L. Bouchareychas and R. L. Raffai, PLoS One, 2019, 14, e0215324 CrossRef CAS PubMed.
- J. Z. Nordin, Y. Lee, P. Vader, I. Mäger, H. J. Johansson, W. Heusermann, O. P. Wiklander, M. Hällbrink, Y. Seow, J. J. Bultema, J. Gilthorpe, T. Davies, P. J. Fairchild, S. Gabrielsson, N. C. Meisner-Kober, J. Lehtiö, C. I. Smith, M. J. Wood and S. El Andaloussi, Nanomedicine, 2015, 11, 879–883 CrossRef CAS PubMed.
- J. Van Deun, A. Jo, H. Li, H. Y. Lin, R. Weissleder, H. Im and H. Lee, Adv. Biol., 2020, 4, e1900310 Search PubMed.
- Y. Tian, M. Gong, Y. Hu, H. Liu, W. Zhang, M. Zhang, X. Hu, D. Aubert, S. Zhu, L. Wu and X. Yan, J. Extracell. Vesicles, 2020, 9, 1697028 CrossRef CAS PubMed.
- P. Sharma, S. Ludwig, L. Muller, C. S. Hong, J. M. Kirkwood, S. Ferrone and T. L. Whiteside, J. Extracell. Vesicles, 2018, 7, 1435138 CrossRef PubMed.
- S. I. Brett, F. Lucien, C. Guo, K. C. Williams, Y. Kim, P. N. Durfee, C. J. Brinker, J. I. Chin, J. Yang and H. S. Leong, Prostate, 2017, 77, 1335–1343 CrossRef CAS PubMed.
- K. S. Yang, D. Ciprani, A. O’Shea, A. S. Liss, R. Yang, S. Fletcher-Mercaldo, M. Mino-Kenudson, C. Fernández-Del Castillo and R. Weissleder, Gastroenterology, 2021, 160, 1345–1358 CrossRef CAS PubMed.
- J. Ko, N. Bhagwat, S. S. Yee, N. Ortiz, A. Sahmoud, T. Black, N. M. Aiello, L. McKenzie, M. O’Hara, C. Redlinger, J. Romeo, E. L. Carpenter, B. Z. Stanger and D. Issadore, ACS Nano, 2017, 11, 11182–11193 CrossRef CAS PubMed.
- D. He, S. L. Ho, H. N. Chan, H. Wang, L. Hai, X. He, K. Wang and H. W. Li, Anal. Chem., 2019, 91, 2768–2775 CrossRef CAS PubMed.
- P. Zhang, X. Zhou, M. He, Y. Shang, A. L. Tetlow, A. K. Godwin and Y. Zeng, Nat. Biomed. Eng., 2019, 3, 438–451 CrossRef CAS PubMed.
- N. Sun, B. V. Tran, Z. Peng, J. Wang, C. Zhang, P. Yang, T. X. Zhang, J. Widjaja, R. Y. Zhang, W. Xia, A. Keir, J. W. She, H. H. Yu, J. J. Shyue, H. Zhu, V. G. Agopian, R. Pei, J. S. Tomlinson, J. A. Toretsky, S. J. Jonas, N. Federman, S. Lu, H. R. Tseng and Y. Zhu, Adv. Sci., 2022, 9, 2105853 CrossRef CAS PubMed.
- N. Sun, Y.-T. Lee, R. Y. Zhang, R. Kao, P.-C. Teng, Y. Yang, P. Yang, J. J. Wang, M. Smalley, P.-J. Chen, M. Kim, S.-J. Chou, L. Bao, J. Wang, X. Zhang, D. Qi, J. Palomique, N. Nissen, S.-H. B. Han, S. Sadeghi, R. S. Finn, S. Saab, R. W. Busuttil, D. Markovic, D. Elashoff, H.-H. Yu, H. Li, A. P. Heaney, E. Posadas, S. You, J. D. Yang, R. Pei, V. G. Agopian, H.-R. Tseng and Y. Zhu, Nat. Commun., 2020, 11, 4489 CrossRef CAS PubMed.
- Y.-T. Kang, T. Hadlock, S. Jolly and S. Nagrath, Biosens. Bioelectron., 2020, 168, 112535 CrossRef CAS PubMed.
- J. B. Haun, N. K. Devaraj, S. A. Hilderbrand, H. Lee and R. Weissleder, Nat. Nanotechnol., 2010, 5, 660–665 CrossRef CAS PubMed.
- P. Scrimin and L. J. Prins, Chem. Soc. Rev., 2011, 40, 4488 RSC.
- L. Hou, Y. Tang, M. Xu, Z. Gao and D. Tang, Anal. Chem., 2014, 86, 8352–8358 CrossRef CAS PubMed.
- S. Venkataraman, R. M. Dirks, P. W. Rothemund, E. Winfree and N. A. Pierce, Nat. Nanotechnol., 2007, 2, 490–494 CrossRef PubMed.
- T. Murakami, J. Sumaoka and M. Komiyama, Nucleic Acids Res., 2009, 37, e19 CrossRef PubMed.
- A. Jo, A. Green, J. E. Medina, S. Iyer, A. W. Ohman, E. T. McCarthy, F. Reinhardt, T. Gerton, D. Demehin, R. Mishra, D. L. Kolin, H. Zheng, J. Cheon, C. P. Crum, R. A. Weinberg, B. R. Rueda, C. M. Castro, D. M. Dinulescu and H. Lee, Adv. Sci., 2023, 10, 2301930 CrossRef CAS PubMed.
- F. He, H. Liu, X. Guo, B.-C. Yin and B.-C. Ye, Anal. Chem., 2017, 89, 12968–12975 CrossRef CAS PubMed.
- S. Xing, Z. Lu, Q. Huang, H. Li, Y. Wang, Y. Lai, Y. He, M. Deng and W. Liu, Theranostics, 2020, 10, 10262–10273 CrossRef CAS PubMed.
- X. Gao, X. Teng, Y. Dai and J. Li, ACS Sens., 2021, 6, 3611–3620 CrossRef CAS PubMed.
- J. Zhang, J. Shi, H. Zhang, Y. Zhu, W. Liu, K. Zhang and Z. Zhang, J. Extracell. Vesicles, 2020, 10, e12025 CrossRef CAS PubMed.
- N. C. Seeman and H. F. Sleiman, Nat. Rev. Mater., 2017, 3, 17068 CrossRef.
- K. E. Bujold, A. Lacroix and H. F. Sleiman, Chem, 2018, 4, 495–521 CAS.
- H. Li, M. Han, X. Weng, Y. Zhang and J. Li, ACS Nano, 2021, 15, 1710–1717 CrossRef CAS PubMed.
- M. Wang, F. He, H. Li, S. Yang, J. Zhang, P. Ghosh, H. H. Wang and Z. Nie, Nano Lett., 2019, 19, 2603–2613 CrossRef CAS PubMed.
- T. Tian, J. Li, C. Xie, Y. Sun, H. Lei, X. Liu, J. Xia, J. Shi, L. Wang, W. Lu and C. Fan, ACS Appl. Mater. Interfaces, 2018, 10, 3414–3420 CrossRef CAS PubMed.
- J. Li, C. Fan, H. Pei, J. Shi and Q. Huang, Adv. Mater., 2013, 25, 4386–4396 CrossRef CAS PubMed.
- K. Guo, Z. Li, A. Win, R. Coreas, G. B. Adkins, X. Cui, D. Yan, M. Cao, S. E. Wang and W. Zhong, Biosens. Bioelectron., 2021, 192, 113502 CrossRef CAS PubMed.
- M. Li, E. Zeringer, T. Barta, J. Schageman, A. Cheng and A. V. Vlassov, Philos. Trans. R. Soc., B, 2014, 369, 20130502 CrossRef PubMed.
- E. Lázaro-Ibáñez, T. R. Lunavat, S. C. Jang, C. Escobedo-Lucea, J. Oliver-De La Cruz, P. Siljander, J. Lötvall and M. Yliperttula, BMC Cancer, 2017, 17, 92 CrossRef PubMed.
- Z. Han, F. Wan, J. Deng, J. Zhao, Y. Li, Y. Yang, Q. Jiang, B. Ding, C. Liu, B. Dai and J. Sun, Nano Today, 2021, 38, 101203 CrossRef CAS.
- Z. Lu, Y. Shi, Y. Ma, B. Jia, X. Li, X. Guan and Z. Li, Anal. Chem., 2022, 94, 9466–9471 CrossRef CAS PubMed.
- J. Hu, Y. Sheng, K. J. Kwak, J. Shi, B. Yu and L. J. Lee, Nat. Commun., 2017, 8, 1683 CrossRef PubMed.
- X. Gao, S. Li, F. Ding, H. Fan, L. Shi, L. Zhu, J. Li, J. Feng, X. Zhu and C. Zhang, Angew. Chem., Int. Ed., 2019, 58, 8719–8723 CrossRef CAS PubMed.
- B. Ning, Z. Huang, B. M. Youngquist, J. W. Scott, A. Niu, C. M. Bojanowski, K. J. Zwezdaryk, N. S. Saba, J. Fan, X.-M. Yin, J. Cao, C. J. Lyon, C.-Z. Li, C. J. Roy and T. Y. Hu, Nat. Nanotechnol., 2021, 16, 1039–1044 CrossRef CAS PubMed.
- J. S. Hong, T. Son, C. M. Castro and H. Im, Adv. Sci., 2023, 10, e2301766 CrossRef PubMed.
- J. U. Surjadi, L. Gao, H. Du, X. Li, X. Xiong, N. X. Fang and Y. Lu, Adv. Eng. Mater., 2019, 21, 1800864 CrossRef CAS.
- T. Frenzel, M. Kadic and M. Wegener, Science, 2017, 358, 1072–1074 CrossRef CAS PubMed.
- Y. Wang, L. Li, D. Hofmann, J. E. Andrade and C. Daraio, Nature, 2021, 596, 238–243 CrossRef CAS PubMed.
- S. M. Montgomery, S. Wu, X. Kuang, C. D. Armstrong, C. Zemelka, Q. Ze, R. Zhang, R. Zhao and H. J. Qi, Adv. Funct. Mater., 2021, 31, 2005319 CrossRef CAS.
- X. Xu, B. Peng, D. Li, J. Zhang, L. M. Wong, Q. Zhang, S. Wang and Q. Xiong, Nano Lett., 2011, 11, 3232–3238 CrossRef CAS PubMed.
- J. B. Lee, S. Peng, D. Yang, Y. H. Roh, H. Funabashi, N. Park, E. J. Rice, L. Chen, R. Long, M. Wu and D. Luo, Nat. Nanotechnol., 2012, 7, 816–820 CrossRef CAS PubMed.
- M. Chen, Y. Wang, J. Zhang, Y. Peng, S. Li, D. Han, S. Ren, K. Qin, S. Li and Z. Gao, J. Nanobiotechnol., 2022, 20, 40 CrossRef CAS PubMed.
- A. S. Hoffman, Adv. Drug Delivery Rev., 2012, 64, 18–23 CrossRef.
- C. Yu, P. Yuan, E. M. Erickson, C. M. Daly, J. A. Rogers and R. G. Nuzzo, Soft Matter, 2015, 11, 7953–7959 RSC.
- W. Guo, C. H. Lu, R. Orbach, F. Wang, X. J. Qi, A. Cecconello, D. Seliktar and I. Willner, Adv. Mater., 2015, 27, 73–78 CrossRef CAS PubMed.
- O. Skarsetz, V. Slesarenko and A. Walther, Adv. Sci., 2022, 9, 2201867 CrossRef CAS PubMed.
- G. Ma, W. Lin, Z. Yuan, J. Wu, H. Qian, L. Xu and S. Chen, J. Mater. Chem. B, 2017, 5, 935–943 RSC.
- K. Lee and S. A. Asher, J. Am. Chem. Soc., 2000, 122, 9534–9537 CrossRef CAS.
- R. Yin, Z. Tong, D. Yang and J. Nie, Int. J. Biol. Macromol., 2011, 49, 1137–1142 CrossRef CAS PubMed.
- H. Zhao, S. Pan, A. Natalia, X. Wu, C.-A. J. Ong, M. C. C. Teo, J. B. Y. So and H. Shao, Nat. Biomed. Eng., 2023, 7, 135–148 CrossRef CAS PubMed.
- S. Song, L. Zhu, C. Wang and Y. Yang, View, 2023, 4, 20220011 CrossRef CAS.
- J. M. Nikoloff, M. A. Saucedo-Espinosa, A. Kling and P. S. Dittrich, Proc. Natl. Acad. Sci. U. S. A., 2021, 118, e2106630118 CrossRef CAS PubMed.
- Z. J. Smith, C. Lee, T. Rojalin, R. P. Carney, S. Hazari, A. Knudson, K. Lam, H. Saari, E. L. Ibañez, T. Viitala, T. Laaksonen, M. Yliperttula and S. Wachsmann-Hogiu, J. Extracell. Vesicles, 2015, 4, 28533 CrossRef PubMed.
- G. E. Melling, R. Conlon, P. Pantazi, E. R. Dellar, P. Samuel, L. A. Baena-Lopez, J. C. Simpson and D. R. F. Carter, Sci. Rep., 2022, 12, 262 CrossRef PubMed.
- J. Penders, A. Nagelkerke, E. M. Cunnane, S. V. Pedersen, I. J. Pence, R. C. Coombes and M. M. Stevens, ACS Nano, 2021, 15, 18192–18205 CrossRef CAS PubMed.
- E. J. van der Vlist, E. N. Nolte-’t Hoen, W. Stoorvogel, G. J. Arkesteijn and M. H. Wauben, Nat. Protoc., 2012, 7, 1311–1326 CrossRef PubMed.
- G. Huang, Y. Liu, D. Wang, Y. Zhu, S. Wen, J. Ruan and D. Jin, eLight, 2022, 2, 20 CrossRef.
- K. Lee, K. Fraser, B. Ghaddar, K. Yang, E. Kim, L. Balaj, E. A. Chiocca, X. O. Breakefield, H. Lee and R. Weissleder, ACS Nano, 2018, 12, 494–503 CrossRef CAS PubMed.
- J. D. Spitzberg, S. Ferguson, K. S. Yang, H. M. Peterson, J. C. T. Carlson and R. Weissleder, Nat. Commun., 2023, 14, 1239 CrossRef CAS PubMed.
- I. Dovgan, O. Koniev, S. Kolodych and A. Wagner, Bioconjugate Chem., 2019, 30, 2483–2501 CrossRef CAS PubMed.
- C. M. Dundas, D. Demonte and S. Park, Appl. Microbiol. Biotechnol., 2013, 97, 9343–9353 CrossRef CAS PubMed.
- J. Dugal-Tessier, S. Thirumalairajan and N. Jain, J. Clin. Med., 2021, 10, 838 CrossRef CAS PubMed.
- S. A. Kazane, D. Sok, E. H. Cho, M. L. Uson, P. Kuhn, P. G. Schultz and V. V. Smider, Proc. Natl. Acad. Sci. U. S. A., 2012, 109, 3731–3736 CrossRef CAS PubMed.
- J. A. G. L. van Buggenum, J. P. Gerlach, S. Eising, L. Schoonen, R. A. P. M. van Eijl, S. E. J. Tanis, M. Hogeweg, N. C. Hubner, J. C. van Hest, K. M. Bonger and K. W. Mulder, Sci. Rep., 2016, 6, 22675 CrossRef CAS PubMed.
- M. Banijamali, P. Höjer, A. Nagy, P. Hååg, E. P. Gomero, C. Stiller, V. O. Kaminskyy, S. Ekman, R. Lewensohn, A. E. Karlström, K. Viktorsson and A. Ahmadian, J. Extracell. Vesicles, 2022, 11, 12277 CrossRef CAS PubMed.
- D. Wu, J. Yan, X. Shen, Y. Sun, M. Thulin, Y. Cai, L. Wik, Q. Shen, J. Oelrich, X. Qian, K. L. Dubois, K. G. Ronquist, M. Nilsson, U. Landegren and M. Kamali-Moghaddam, Nat. Commun., 2019, 10, 3854 CrossRef PubMed.
- R. Martel, M. L. Shen, P. DeCorwin-Martin, L. O. F. de Araujo and D. Juncker, ACS Sens., 2022, 7, 3817–3828 CrossRef CAS PubMed.
- J. Ko, Y. Wang, K. Sheng, D. A. Weitz and R. Weissleder, ACS Nano, 2021, 15, 5631–5638 CrossRef CAS PubMed.
- F. J. Verweij, L. Balaj, C. M. Boulanger, D. R. F. Carter, E. B. Compeer, G. D’Angelo, S. El Andaloussi, J. G. Goetz, J. C. Gross, V. Hyenne, E.-M. Krämer-Albers, C. P. Lai, X. Loyer, A. Marki, S. Momma, E. N. M. Nolte-’t Hoen, D. M. Pegtel, H. Peinado, G. Raposo, K. Rilla, H. Tahara, C. Théry, M. E. van Royen, R. E. Vandenbroucke, A. M. Wehman, K. Witwer, Z. Wu, R. Wubbolts and G. van Niel, Nat. Methods, 2021, 18, 1013–1026 CrossRef CAS PubMed.
- O. G. De Jong, S. A. A. Kooijmans, D. E. Murphy, L. Jiang, M. J. W. Evers, J. P. G. Sluijter, P. Vader and R. M. Schiffelers, Acc. Chem. Res., 2019, 52, 1761–1770 CrossRef CAS PubMed.
- T. Smyth, K. Petrova, N. M. Payton, I. Persaud, J. S. Redzic, M. W. Graner, P. Smith-Jones and T. J. Anchordoquy, Bioconjugate Chem., 2014, 25, 1777–1784 CrossRef CAS PubMed.
- P. Pužar Dominkuš, M. Stenovec, S. Sitar, E. Lasič, R. Zorec, A. Plemenitaš, E. Žagar, M. Kreft and M. Lenassi, Biochim. Biophys. Acta, Biomembr., 2018, 1860, 1350–1361 CrossRef PubMed.
- M. Collot, P. Ashokkumar, H. Anton, E. Boutant, O. Faklaris, T. Galli, Y. Mély, L. Danglot and A. S. Klymchenko, Cell Chem. Biol., 2019, 26, 600–614 CrossRef CAS PubMed.
- V. Hyenne, S. Ghoroghi, M. Collot, J. Bons, G. Follain, S. Harlepp, B. Mary, J. Bauer, L. Mercier, I. Busnelli, O. Lefebvre, N. Fekonja, M. J. Garcia-Leon, P. Machado, F. Delalande, A. A. López, S. G. Silva, F. J. Verweij, G. van Niel, F. Djouad, H. Peinado, C. Carapito, A. S. Klymchenko and J. G. Goetz, Dev. Cell, 2019, 48, 554–572 CrossRef CAS PubMed.
- G. Corso, W. Heusermann, D. Trojer, A. Görgens, E. Steib, J. Voshol, A. Graff, C. Genoud, Y. Lee, J. Hean, J. Z. Nordin, O. P. B. Wiklander, S. El Andaloussi and N. Meisner-Kober, J. Extracell. Vesicles, 2019, 8, 1663043 CrossRef CAS PubMed.
- M. Mittelbrunn, C. Gutiérrez-Vázquez, C. Villarroya-Beltri, S. González, F. Sánchez-Cabo, M. González, A. Bernad and F. Sánchez-Madrid, Nat. Commun., 2011, 2, 282 CrossRef PubMed.
- E. Saxon and C. R. Bertozzi, Science, 2000, 287, 2007–2010 CrossRef CAS PubMed.
- T. S. Lee, Y. Kim, W. Zhang, I. H. Song and C. H. Tung, Biochim. Biophys. Acta, Gen. Subj., 2018, 1862, 1091–1100 CrossRef CAS PubMed.
- S. Song, M. K. Shim, S. Lim, Y. Moon, S. Yang, J. Kim, Y. Hong, H. Y. Yoon, I. S. Kim, K. Y. Hwang and K. Kim, Bioconjugate Chem., 2020, 31, 1562–1574 CrossRef CAS PubMed.
- D. G. You, G. T. Lim, S. Kwon, W. Um, B. H. Oh, S. H. Song, J. Lee, D. G. Jo, Y. W. Cho and J. H. Park, Sci. Adv., 2021, 7, eabe0083 CrossRef CAS PubMed.
- J. Driscoll, A. Moirangthem, I. K. Yan and T. Patel, Front. Bioeng. Biotechnol., 2021, 9, 686510 CrossRef PubMed.
- Y. Xing, S. S. Yerneni, W. Wang, R. E. Taylor, P. G. Campbell and X. Ren, Biomaterials, 2022, 281, 121357 CrossRef CAS PubMed.
- I. Stevic, G. Buescher and F. L. Ricklefs, Cells, 2020, 9, 130 CrossRef CAS PubMed.
- J. Wang, A. Wuethrich, A. A. Sina, R. E. Lane, L. L. Lin, Y. Wang, J. Cebon, A. Behren and M. Trau, Sci. Adv., 2020, 6, eaax3223 CrossRef CAS PubMed.
- F. Tian, S. Zhang, C. Liu, Z. Han, Y. Liu, J. Deng, Y. Li, X. Wu, L. Cai, L. Qin, Q. Chen, Y. Yuan, Y. Liu, Y. Cong, B. Ding, Z. Jiang and J. Sun, Nat. Commun., 2021, 12, 2536 CrossRef CAS PubMed.
- S. Pan, Y. Zhang, A. Natalia, C. Z. J. Lim, N. R. Y. Ho, B. Chowbay, T. P. Loh, J. K. C. Tam and H. Shao, Nat. Nanotechnol., 2021, 16, 734–742 CrossRef CAS PubMed.
- S. Ferguson, K. S. Yang and R. Weissleder, Trends Mol. Med., 2022, 28, 681–692 CrossRef CAS PubMed.
- X. Wu, H. Zhao, A. Natalia, C. Z. J. Lim, N. R. Y. Ho, C.-A. J. Ong, M. C. C. Teo, J. B. Y. So and H. Shao, Sci. Adv., 2020, 6, eaba2556 CrossRef CAS PubMed.
- K. S. Yang, A. O’Shea, P. Zelga, A. S. Liss, C. F. Del Castillo and R. Weissleder, Sci. Rep., 2023, 13, 10969 CrossRef CAS PubMed.
- N. Garcia-Romero, S. Esteban-Rubio, G. Rackov, J. Carrión-Navarro, C. Belda-Iniesta and A. Ayuso-Sacido, Mol. Asp. Med., 2018, 60, 27–37 CrossRef CAS PubMed.
- S. Khan, H. F. Bennit, D. Turay, M. Perez, S. Mirshahidi, Y. Yuan and N. R. Wall, BMC Cancer, 2014, 14, 176 CrossRef PubMed.
- H. Im, H. Shao, Y. I. Park, V. M. Peterson, C. M. Castro, R. Weissleder and H. Lee, Nat. Biotechnol., 2014, 32, 490–495 CrossRef CAS PubMed.
- K. Gabriel, A. Ingram, R. Austin, A. Kapoor, D. Tang, F. Majeed, T. Qureshi and K. Al-Nedawi, PLoS One, 2013, 8, e70047 CrossRef CAS PubMed.
- S. E. F. Yong, M. L. Wong and T. C. Voo, BMC Med. Ethics, 2022, 23, 57 CrossRef PubMed.
Footnote |
† These authors contributed equally. |
|
This journal is © The Royal Society of Chemistry 2024 |
Click here to see how this site uses Cookies. View our privacy policy here.