DOI:
10.1039/D4AY01030B
(Critical Review)
Anal. Methods, 2024, Advance Article
A review: early detection of oral cancer biomarkers using microfluidic colorimetric point-of-care devices†
Received
2nd June 2024
, Accepted 2nd August 2024
First published on 5th August 2024
Abstract
Oral squamous cell carcinoma (OSCC) is the most common type of head and neck cancers. OSCC constitutes 90% of the head and neck malignancies. The delayed identification of oral cancer is the primary cause of ineffective medical treatment. To address this issue, low-cost, reliable point-of-care devices that can be utilized for large-scale screening, even in low-resource settings, including rural areas and primary healthcare centers, are of great interest. Herein, a comprehensive analysis of numerous salivary biomarkers that exhibit significant variations in concentration between individuals with oral cancer and those without is given. Furthermore, the article explores several point-of-care devices that exhibit potential in the realm of oral cancer detection. The biomarkers are discussed with a focus on their structural characteristics and role in oral cancer progression. The devices based on colorimetry and microfluidics are discussed in detail, considering their compliance with the ‘REASSURED’ criteria given by the World Health Organization (WHO) and suitability for mass screening in low-resource settings. Finally, the discourse revolves around the fundamental aspects pertaining to the advancement of multiplex, cost-effective point-of-care devices designed for widespread screening purposes.
1. Introduction
TNM classification, devised by the Union for International Cancer Control, identifies oral cancer as a condition marked by the uncontrolled growth of malignant cells in different areas, including the lip as well as the oral cavity.1 Oral squamous cell carcinoma (OSCC) represents a significant proportion of head and neck cancers, accounting for about 90% of such instances.2 The high global prevalence of oral cancer has led to its designation as a major global health problem. Several strategies have been developed for the detection of different types of cancer.3,4 Late detection of the disease is a significant contributing factor to the ineffectiveness of treatment strategies. Conventional diagnostic methods, including biopsy, cytopathology, and imaging adjuncts, are currently unable to detect the disease at its early stage.5
Visual examination of the patient cannot be used to identify disease stages I through IV. Thus, it is imperative to develop a screening system for accurate staging and early diagnosis that is ideal for mass screening in low-resource settings.6 Liquid biopsies have garnered significant interest due to their potential for early diagnosis and the discomfort associated with the current tissue biopsy procedures.7
Oral cancer has been recognized as the sixth leading form of cancer worldwide, with India ranking second in the highest incidence of oral cancer cases. Fig. 1 depicts the spatial distribution of oral cancer throughout several regions of India.8
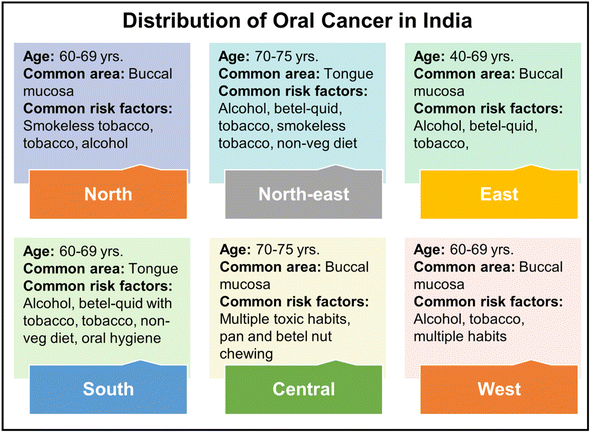 |
| Fig. 1 The geographical distribution of oral cancer across India, with common affected areas and risk factors.8 | |
Thus, detecting oral cancer in the initial stage is of utmost importance to increase the individual's life expectancy. The predominant method employed for identifying oral cancer involves utilizing enzyme-linked immunosorbent assay (ELISA) to detect various biomarkers.9,10 Various attempts have been made to develop ELISA.11 However, it is constrained by the expensive cost of test kits and equipment and the long measurement times. Thus, there exists a necessity for affordable, easily operable, expeditious detection systems that are appropriate for large-scale screening and may be implemented in resource-constrained environments. Microfluidic devices are ideal for point-of-care applications owing to their low cost, simple operation, and suitability for limited resource settings.
‘Microfluidics’ refers to developing miniaturized devices and manipulating fluids within channels on the micrometer scale, with volumes ranging from 10−9 to 10−18 liters. Microfluidics technology was introduced in 1990, with the term “miniaturized total chemical analysis systems”—μTAS in the context that a single device can perform various laboratory steps.12–14 Popular low-cost microfluidic devices include paper-based analytical devices (μPADs), digital microfluidics (DMF), lateral flow assays (LFAs), and wearable sensors. These are usually deployed for point-of-care testing (POCT) devices owing to their simplicity and rapid detection mechanism.15–18 Microfluidics enables the detection of analytes from small volumes of the samples with great sensitivity, owing to the enhanced interaction kinetics between target molecules and surface biorecognition components facilitated by the high surface area to volume ratio. This facilitates a reduced assay time and lower cost in comparison to alternative protein quantification approaches.19
The study focuses on salivary biomarkers for detecting oral cancer, especially OSCC. It details the biomarkers' increased concentrations in oral cancer cases, their structure, and their role in detection and progression. Additionally, it examines low-cost microfluidic devices for oral cancer detection, with a significant focus on LFAs and paper-based colorimetric detection systems, highlighting their simplicity, affordability, and speed. In this study, the recently reported biomarker-based oral cancer detection was discussed in detail. The literature discussing biomarker concentration in clinical trials in oral cancer cases has been included, and an extensive list of the biomarkers is given along with several study details. Regarding the discussion on point-of-care devices, the literature discusses in detail several types of devices, such as colorimetric paper-based sensors, lateral flow assays (LFAs) with different detection systems, and gold and silver nanoparticle-based sensors, strictly adhering to REASSURED criteria and ideal for low resource settings have been included.
2. Samples for oral cancer detection
Invasive oral cancer detection and analysis samples include tumor tissue and peripheral blood cells.20 Liquid biopsy is a commonly deployed technique for cancer detection. Various fluids, such as blood, pleural effusion, saliva, and urine, can be used to actively monitor oral cancer, including treatment response and patient conditions. In liquid biopsy, the detection objects are circulating tumor DNA, circulating tumor cells, and exosomes.21 The mentioned samples are invasive, except for saliva and urine, and may distress patients. Non-invasive samples are vital for consistent patient biomarker monitoring.
Thus, sweat, saliva, tears, and urine are essential biological fluids for disease diagnostics and biomedical investigation. Among these biofluids, saliva is the most preferred sample owing to its accessible collection, transport, and low cost of storage. Moreover, saliva constitutes various hormonal, toxicological, immunological, and infectious disease biomarkers; thus, it has been used for oral health monitoring and cancer diagnosis.22,23
3. Oral cancer and related biomarkers
Treatment for advanced-stage disease frequently calls for complex multimodal treatment options linked to morbidity and subpar patient outcomes. OSCC has a high frequency of advanced stage upon diagnosis and is asymptomatic in the early stages of the disease.24 In recent times, surgical resection has been the primary way of OSCC treatment, depending upon the lesion's size. However, this procedure causes facial abnormalities and severe physiological dysfunction and impairs the food intake and linguistic capabilities of an individual.25 The detection of biomarkers in various forms, including metabolites, miRNA, overexpressed proteins, etc. is the most efficacious approach for early diagnosis of OSCC.26
The structure, occurrence, and tandem of several reported oral cancer biomarkers are briefly discussed in the following section.
3.1. Reactive nitrogen species (RNS)
Some studies have demonstrated that premalignant lesion leukoplakia leads to the development of oral cancer through oxidative and nitrative stress. Reactive oxygen species (ROS) and other free radicals, after reacting with nitric oxide (NO) generate RNS. A transient species NO˙ free radical, when generated at low concentration, plays a vital role in host defense and homeostasis. However, when generated in higher concentration and for a relatively longer time, it becomes mutagenic and genotoxic. NO˙ may act as a mediator of DNA damage by producing RNS, inhibiting DNA damage-repair pathways, or forming carcinogenic nitrosamines. Salivary nitrates are important, which, upon conversion to nitrites (NO2), induce carcinogenesis and act as a carcinogenesis promoter as they form carcinogenic nitrosamines, often reacting with amines and amides. Sometimes, these changes may occur because of old age due to a reduction in the protective salivary antioxidant mechanisms.27
3.2. Lactate dehydrogenase (LDH)
LDH is responsible for catalyzing the final step of the metabolic chain of anaerobic glycolysis and is present in almost all body cells. Oral cancer and several other potentially cancerous lesions and diseases have both been linked to elevated LDH levels.28 Increasing LDH levels provide a highly acidic milieu that easily encourages metastasis and proliferation of tumor cells. Thus, elevated LDH levels are highly helpful in predicting metastasis of the neck lymph node. Moreover, Wanyong Jin et al. showed that an elevated LDH level can also be linked with the possibility of lymph node metastasis in the initial stages of OSCC.29 Carcinogenesis has been observed to facilitate a shift in the energy transformation pathway, transitioning from oxidative phosphorylation to anaerobic glycolysis. The aforementioned phenomenon is frequently denoted as the Warburg effect. In this process, it is found that the LDH level increases and catalyzes pyruvate in lactic acid in the presence of electron donor NADH2. Thus, LDH is associated with the identification of malignant transformation. Another aspect that makes LDH a potential biomarker for oral cancer is that it can detect cellular damage or death, localization, and the surveillance of systemic changes and remains unaltered under normal conditions. Its presence in the saliva and serum makes it easily accessible for monitoring its levels.30
3.3. Cyclin D1(CycD1)
The 295 amino acid long CycD1 protein was initially discovered as the PRAD-1 putative oncogene. Multiple studies have shown a robust correlation between the activation of the CCND1 gene and/or the high expression of CycD1 and the occurrence of diverse malignant tumors. Furthermore, it has been discovered that the amplification of CCND1 has an impact on the regulation of CycD1, resulting in growth promotion, disruption, and carcinogenesis. The amplification of the CyclinD1 gene has been observed in around 22–58% of various human malignancies, and its prognostic usefulness in cancer patients is demonstrated by its clenched relationship to overall survival.31 E2F1 and CycD1 are also involved in the cell cycle progression mediated by ribosomal protein L3 (uL3).32 Moreover, several studies have confirmed the overexpression of CycD1 in oral cancer, and it is is a potential biomarker for early detection.33
3.4. Cytokines: IL-6 and IL-8
Cytokines are employed in the identification of cancer as well. T helper (Th) cells are known for their ability to produce interleukins and exert their effects on leukocytes. IL-6 is classified as a cytokine belonging to the interleukin family. It is important in several host-defense processes, encompassing hematopoiesis, immunological responses, and the activation of acute-phase reactions. Because of this, IL-6 is linked to the pathology of various cancers as well as immune-mediated inflammatory disorders (IMIDs).
Leukocyte activation and migration are predominantly mediated by chemokines, and are involved in a variety of immunological responses. A chemokine called IL-8 predominantly stimulates neutrophils for migration to the site of infection. The molecular weight of the glycosylated protein IL-6 is between 21 and 28 kDa. IL-6 comprises 212 amino acids with the four-helix bundle structure arranged in an up-up-down-down topology and an N-terminal signal peptide of 29 amino acids. IL-8 is a soluble, small peptide that has an 8–10 kDa molecular weight. IL-8 is an essential mediator in the regulation of inflammatory processes. Moreover, it also plays an essential role in triggering angiogenesis. The advancement and metastasis of cancer are particularly relevant to the pro-angiogenic characteristics of IL-8. On cancer cells, endothelial cells, tumor-associated macrophages, and infiltrating neutrophils, the IL-8 receptors are widely expressed. This proves that IL-8 has a substantial regulatory role in the tumor microenvironment. The development and viability of cancer cells are facilitated by IL-8 through autocrine signaling pathways.34
3.5. Cyfra 21-1
Cyfra 21-1 is also a well-known, recently studied biomarker for the detection of various cancers. The cleavage of the CK19 protein in vitro is mediated by the intrinsic caspase 3 activity, leading to the subsequent release of Cyfra 21-1 into the cancer cell lines. Furthermore, it is also reported that there is an elevation in the level of extracellular Cyfra 21-1 and a significant elevation in the levels of intracellular Cyfra 21-1 during apoptosis. However, cell death due to caspase-independent death in the presence of the Z-VAD caspase inhibitor did not increase the Cyfra 21-1 level. Therefore, it has been postulated that the intracellular release of Cyfra 21-1 takes place during an intermediary phase of apoptosis triggered by caspase activation, subsequently leading to its release into the extracellular milieu.35
The concentration of Cyfra 21-1 can be detected from the serum or saliva. It is worth noting that the concentration of salivary Cyfra 21-1 was seen to be three times more than the concentration of serum Cyfra 21-1 in cases of oral squamous cell carcinoma (OSCC). Several other studies have also reported similar observations of higher salivary concentration of Cyfra 21-1 than that in blood serum in the case of OSCC. This makes Cyfra 21-1 a potential OSCC salivary biomarker. The importance of Cyfra 21-1 as a potential biomarker for OSCC was first revealed by Kurokawa et al. in 1997. Compared to controls and benign oral cancer patients, they found that patients with OSCC had a substantial rise in serum Cyfra 21-1. As per findings by Sugama et al., increased serum Cyfra 21-1 results from cytokeratin release due to necrosis or cell lysis. Additionally, there is an increase in the cytokeratin breakdown due to epithelial transition to malignancy, resulting in a surge in the cytokeratin fragment concentration in the local environment.36
3.6. Tissue polypeptide antigen (TPA)
Another cytokeratin evaluated for oral cancer detection is tissue polypeptide antigen (TPA). A member of the intermediate filament family of proteins, cytokeratin is a helpful tool in the diagnosis of cancers. Upon an increase in the proteolytic activity in malignant cells, CK fragments are released into the bloodstream, and can be measured using specialized serological assays that are commercially available. Various studies have confirmed that, as opposed to healthy individuals, the CK level will be significantly high in patients with carcinoma.37 It is a relatively older tumor maker in practice. It has been established that a combination of non-epidermal CK, such as CK8, 18, and 19, and TPA is immunologically linked. Through the S and G2 stages of the cell cycle, TPA is produced and released into the circulation throughout mitosis. Previous studies have provided evidence indicating that there is an elevation in the concentration of antigens in the sera and tumor tissues of individuals diagnosed with cancer, as opposed to the respective healthy controls. Nevertheless, owing to its wide-ranging specificity, the utilization of this particular marker as an indicator for tumors is infrequent.38
3.7. CA-125
CA-125 was discovered for the first time by American scientists in 1981. Known as Mucin 16 (MUC 16), the CA-125 glycoprotein is characterized by its high molecular weight, exceeding 200 kDa. It is known from the existing literature that it supports tumor growth by promoting metastatic invasion and suppression of natural killer cells. It is a long molecule consisting of three domains: the N-terminal, tandem repeat, and C-terminal. The tandem repeat domains and N-terminal are highly glycosylated and remain in the extracellular position. After proteolytic digestion, generally, the protein (extracellular portion) is released into body fluids.39 Moreover, it is found that more than 80% of individuals with epithelial ovarian cancer have elevated serum CA-125 levels.40
3.8. CA 19-9
Cancer antigen 19-9 (CA 19-9), alternatively referred to as carbohydrate antigen 19-9, is a widely utilized and established biomarker for pancreatic cancer. It was first described by Koprowski et al. in 1979; later, in 1981, it was discovered that this molecule is present in the serum in the positive cases of colon and pancreatic cancer. It was also found that CA 19-9 is a constituent of glycoproteins and mucins. Mainly healthy human pancreatic and biliary ductal cells, as well as gastric, colon, endometrial, and salivary epithelia, generate CA 19-9. CA 19-9 exhibits overexpression in several inflammatory disorders, including pancreatitis and other benign gastrointestinal conditions.41 Existing literature has established that CA 19-9 levels in the saliva of patients with OSCC were considerably altered compared to those in healthy individuals serving as controls.42
3.9. Carcinoembryonic antigen (CEA)
Carcinoembryonic antigen (CEA), one of the numerous tumor markers discussed, is a broad-spectrum serum cancer biomarker. CEA is a glycoprotein with a molecular weight of around 180 kDa. It serves as a significant cell-surface tumor marker and is present in several carcinomas. CEA is recognized as a prominent biomarker for gastrointestinal cancers, particularly in cases of colorectal malignancies.43
Numerous investigations revealed a favorable correlation between the occurrence, progression, and severity of oral cancer and the concentration of CEA in serum. However, the existing diagnostic aids are not sufficient for accurate and sensitive detection, leading to proper, early stage oral cancer prediction. Numerous interfering components in the blood hindered the accurate and precise detection of CEA. Therefore, compared to blood, saliva is an effective tool for CEA concentration monitoring, as it consists of minimal interfering substances, and the sample collection is painless and non-invasive, which is highly useful in point-of-care testing. The average concentration in healthy individuals remains 0.1–2.5 ng mL−1, which eventually increases to nearly 5 ng mL−1 in the case of early stage tumor development.44
3.10. Squamous cell carcinoma antigen (SCCA)
Squamous cell carcinoma antigen (SCCA) was first introduced by Kato and Torigoe in 1977 and found to be useful as a tumor-specific antigen for the detection of squamous cell carcinoma in the uterine cervix. However, it was found that its diagnosis efficiency for OSCC was unsatisfactory.45 SCCA has two highly homologous isoforms, SCCA1 and SCCA2, encoded by SERPINB3 and SERPINB4 genes, respectively, and is located on the long arm of chromosome 18 (18q21.3). The proteins SCCA1 and SCCA2, alternatively known as SERPINB3 and SERPINB4, are members of the serine protease inhibitor family (SERPINBs). They are characterized by an ovalbumin-like domain containing nine α-helices and three antiparallel β-sheets. Additionally, these proteins possess a reactive center loop that plays a crucial role in their ability to bind to and inhibit the target protease.46
SCCA is a 48 kDa protein that is also used for the detection of lung cancer. SCCA has also been explored for the diagnosis of OSCC, and efforts have been made to improve the detection efficiency for the diagnosis of OSCC. M. Yang et al. developed a straightforward, efficient, and scalable approach utilizing saponin treatment for the identification of intra-vesicular proteins of extracellular vesicles (EVs) without the need for additional purification procedures. This strategy works by elevating the SCCA concentration in the serum, thus resulting in improved diagnostic efficiencies of SCCA for OSCC.47
3.11. Kiel 67 (Ki-67)
The Ki-67 antibody is generally used to localize the Ki-67 protein in the tissue sections. The Ki-67 antibody was named after its characterization in Kiel, Germany. Additionally, it is worth noting that the antibody was cultivated in the 67th well of the tissue culture plate. The Ki-67 protein is located in the nucleus as detected by immunolocalization of the Ki-67 antigen, and its gene is located on chromosome 10q25-ter.48 The Ki-67 proliferation rate is an additional biological and predictive indicator. The expression of Ki-67 antigens is initiated during the S-phase and progressively increases during the S and G2 phases, ultimately peaking during mitosis. Following cell division, the cells enter the G1 phase, wherein they possess a supply of Ki-67 antigen. Throughout this phase, the level of Ki-67 antigen gradually diminishes, resulting in an extended G1 phase. Throughout the cell cycle, the Ki-67 protein is expressed in proliferating cells but not in quiescent (G0) cells.49 Multiple studies have provided evidence of the considerable prognostic importance of enhanced proliferative activity in certain forms of cancer. The Ki-67 protein is widely recognized as a proliferation marker, serving as an indicator of the overall proportion of proliferating cells in a tumor.50
3.12. Insulin-like growth factor-I (IGF-I)
Investigations have provided evidence suggesting that the IGF type I receptor (IGF-IR) and its ligands IGF-I and IGF-II have a role in the development and progression of many cancers in humans.51 The receptor tyrosine kinase family includes the insulin-like growth factor-I (IGF-I) receptor (IGF-IR). The molecular configuration comprises two α- and two β-subunits that are interconnected through disulfide bonds. The α-subunits are situated extracellularly and play a role in binding ligands, but the β-subunits span the plasma membrane and possess an intracellular kinase domain that is specifically responsible for initiating signal transduction cascades.52 One of the plausible reasons that IGF-I is associated with various types of cancers is its role in angiogenesis, apoptosis, differentiation, proliferation, and metabolism.53
3.13. Metalloproteinases MMP-2
Matrix metalloproteinases (MMPs) represent a group of proteinases that rely on zinc for their enzymatic activity. These proteinases are first secreted in an inactive form, known as proenzymes, and necessitate proteolytic cleavage to attain their active state. The association between MMP-2 and MMP-9 and the malignant traits of tumor cells has been well established. Nevertheless, a notable disparity was noted in the activation of MMP-2 compared to MMP-9 in malignant OSCC. An increased activation ratio of MMP-2 exhibits a substantial correlation with lymph node metastasis in OSCC and can serve as a potential predictor of the risk of metastasis development. Therefore, MMP-2 possesses the potential to serve as a more specific molecular target for anti-metastatic therapy for OSCC.54 It is also known from the literature that MMP-2 and MMP-9 play a significant role in type IV collagen degradation, a significant component of the basement membrane, which is a prerequisite for tumor invasion.55 Thus, based on the substantial research conducted so far, it can be inferred that MMP-2 is a noteworthy biomarker for detecting oral cancer.
3.14. Carbonyls
Carbonyls are the products of protein oxidation. The substantial increase in the salivary carbonyls can be attributed to the exposure of the epithelial cells to the substantial free radical attack. Moreover, in the malignant tissues, the extent of oxidative DNA damage was also attributed to the reduction in the total antioxidant capacity, resulting in an increase in free radical concentration.56 Thus, carbonyls are also a potential biomarker for OSCC diagnosis and monitoring.
Various reviews are dedicated to oral cancer biomarkers; the reader is advised to go through them for a thorough understanding. Moreover, there are several recently published review articles discussing the salivary biomarkers for the detection and monitoring of OSCC and other oral cancers, and readers are advised to go through an extensive list of the salivary biomarkers.57–68 Several salivary biomarkers that exhibit notable variations in concentration in cases of OSCC patients in clinical studies and other types of oral malignancies are given in Table 1. The biomarkers discussed in Table 1 are ideal for the development of point-of-care devices owing to the significant increase in concentration observed in the case of oral cancer. Apart from the discussed biomarkers, there are several other recently explored salivary biomarkers that are also linked to the detection and progression of oral cancer and tested using clinical samples, which are listed in Table 2.
Table 1 List of salivary biomarkers and the percentage change observed in the OSCC in several clinical studies
Classification of chemicals |
Salivary biomarker |
% change observed |
Related disease |
Clinical/preclinical study |
References |
Carbonyl compounds |
Carbonyls |
246 |
Tongue cancer |
Clinical |
56 |
Proteins |
Kiel 67 (Ki-67) |
127 |
Clinical |
Lactate dehydrogenase (LDH) |
86 |
Oral cancer and oral submucous fibrosis |
Clinical |
Cyclin D1(CycD1) |
87 |
Tongue cancer |
Clinical |
Interleukin 6 (IL-6) |
6200 |
OSCC |
Clinical |
59 |
Interleukin 8 (IL-8) |
200 |
Clinical |
Cyfra 21-1 |
400 |
Clinical |
69 |
CA125 |
Clinical |
Tissue polypeptide antigen |
Clinical |
CA 19-9 |
∼300 |
Clinical |
CEA |
∼170 |
Clinical |
Squamous cell carcinoma antigen (SCCA) |
∼170 |
Clinical |
Insulin-like growth factor-I (IGF-I) |
117 |
Clinical |
70 |
Metalloproteinases MMP-2 |
75 |
Clinical |
Reactive nitrogen species (RNS) |
NO |
60 |
Clinical |
71 |
NO2 |
190 |
NO3 |
93 |
Table 2 A list of recently reported salivary biomarkers obtained from the clinical studies has been provided. The table also gives the detection method, sample size, and final outcomes of the study
Year |
Salivary biomarkers |
Type of cancer |
Detection method |
Number of samples/patients under investigation |
Outcomes |
References |
2024 |
miRNAs (miR-7-5p, miR-10b-5p, miR-182-5p, miR-215-5p, miR-431-5p, miR-486-3p, miR-3614-5p, and miR-4707-3p) |
Oral cancer |
Quantitative real-time polymerase chain reaction (PCR) |
Oral cancer, n = 50; oral potentially malignant disorders, n = 52; controls, n = 60 |
Salivary miRNA can be deployed in diagnosing and predicting oral cancer |
72 |
2024 |
Methylation statuses of genes TRH, RASSF1A, p16, and MGMT |
Oral tongue squamous cell carcinoma (OTSCC) |
mRNA sequencing |
OTSCC patients = 45 and healthy control = 20 |
Salivary DNA methylation as a predictive OTSCC biomarker |
73 |
2024 |
Matrix metalloproteinase-1 (MMP-1) |
OSCC |
Time-saving rapid strip test (RST) for MMP-1 |
n = 196 OSCC patients, n = 236 patients with oral potentially malignant disorders, and n = 171 healthy controls |
Salivary MMP-1 can be deployed for the detection and monitoring of OSCC |
74 |
2024 |
Human beta-defensin 3 (hBD-3)/human beta-defensin 2 (hBD-2) |
OSCC |
96-Well immune-plates |
n = 40 subjects; n = 54 for the tissue immunofluorescence microscopy study |
The beta-defensin index can be a functional biomarker for oral cancer detection |
75 |
2024 |
Cellular prion protein (PrPC) |
OSCC |
Enzyme-linked immunosorbent assay (ELISA) |
n = 76 OSCC patients, n = 30 oral potentially malignant disorders (OPMDs) patients, and n = 78 control |
PrPC in saliva and serum can be a potential biomarker for early diagnosis of OSCC |
76 |
2024 |
Alpha-2HS-glycoprotein (AHSG) |
Oropharyngeal cancer (OPC) |
High-throughput proteomics technique, tandem Mass Tag (TMT) based quantification of salivary proteins |
Discovery phase: 20 OPC cases |
AHSG levels are elevated in the saliva of OPC patients as opposed to healthy controls |
77 |
Validation phase: 41OPC cases |
2023 |
MALAT1 |
OSCC |
Quantitative real-time polymerase chain reaction (PCR) and histopathological examination |
n = 20 OSCC patients and n = 20 healthy controls |
Higher expression of MALAT1 and lower expression of miRNA-124 in OSCC |
78 |
2022 |
miRNA-1307-5p |
OSCC |
Flow cytometry |
n = 12 saliva samples |
Exclusively overexpressed in tissues and salivary exosomes in OSCC |
79 |
2022 |
Exosomal MicroRNA-486-5p and MicroRNA-10b-5p |
Oral and oropharyngeal squamous cell carcinoma |
Quantitative real-time PCR |
n = 50 |
miR-486-5p: elevated and miR-10b-5p: reduced in oral and oropharyngeal squamous cell carcinoma |
80 |
2020 |
Exosomal miR-24-3p |
OSCC |
miRNA microarray analysis and qRT-PCR |
n = 45 OSCC patients and n = 10 normal controls |
miR-24-3p expressed at a higher level in OSCC |
81 |
2020 |
Lactate dehydrogenase (LDH), C-reactive protein (CRP), and cancer antigen 125 (CA125) |
Oral lichen planus (OLP) and OSCC |
Enzyme-linked immunosorbent assay (ELISA) |
n = 15 OSCC patients, n = 20 OLP patients, and n = 20 healthy controls |
Salivary LDH, CA125, and CRP can be deployed to evaluate malignant changes |
82 |
2020 |
Matrix metalloproteinase-1 (MMP-1) |
OSCC |
Developed ELISA |
n = 269 OSCC patients, n = 578 oral potentially malignant disorder (OPMD) patients, and n = 313 healthy controls |
Developed MMP-1 ELISA can be deployed for OSCC identification and monitoring |
83 |
2019 |
Tropomyosin alpha-4 chain (TPM4) |
OSCC |
Two-dimensional polyacrylamide gel electrophoresis and mass spectrometry |
n = 30 OSCC patients and n = 30 healthy controls |
Salivary upregulation of TPM4 in the case of OSCC |
84 |
2017 |
miR-486-5p and miR-10b-5p |
Head and neck squamous cell carcinoma (HNSCC) |
Droplet digital PCR |
miRNA-seq pilot: n = 5 and n = 5 healthy controls |
miR-486-5p: Upregulated |
85 |
ddPCR assays: n = 11 and n = 9 healthy controls |
miR-10b-5p: downregulated |
|
2016 |
C-Reactive protein (CRP) |
Oral premalignant lesions and OSCC |
Immunoturbidimetry method |
n = 20 OSCC patients, n = 20 oral premalignant patients, and n = 20 healthy controls |
Higher CRP levels in OSCC and oral premalignant lesion cases |
86 |
2011 |
γ-Aminobutyric acid, phenylalanine, valine, n-eicosanoic acid and lactic acid |
OSCC |
Ultra performance liquid chromatography coupled with quadrupole/time-of-flight mass spectrometry and multivariate statistical analysis |
n = 37 OSCC patients, n = 32 oral leukoplakia (OLK) patients, and n = 34 healthy subjects |
Oral cancer can be detected using salivary metabolome diagnostics |
87 |
4. Salivary metabolites for oral cancer detection
As we have discussed so far, various salivary biomarkers are known to change significantly in oral cancer compared to healthy controls. Metabolites are the small molecules involved in metabolic reactions.88–90 Various metabolites are released into the saliva by blood.91 As a result, salivary metabolites are also an important class of small molecules that can be deployed for early oral cancer detection and can be considered powerful early screening tools.92–97 Herein, we have given a list of selected metabolites that are known to change concentration significantly in the case of OSCC. Metabolites such as 3-phenylpropionate, cytosine, hexanoate, 3-(4-hydroxyphenyl)propionate, and S-adenosylmethionine are found to increase by 627%, 604%, 467%, 395%, and 348% respectively. Various additional metabolites exhibit a substantial increase in concentration, which depends upon the timing of saliva collection. The extensive list and the change in the absolute concentration values can be found in the review by Shigeo Ishikawa et al.98 There are several other studies that have listed and discussed salivary metabolites in detail.95,99
5. The cancer treatment and the concentration of biomarkers
The detailed discussion of cancer metabolism and its significance in clinical application can be seen in the review by Honigova et al.100,101 The stage of the disease determines how oral cancer, or any other cancer in general, will be treated. Surgery can be used to treat early stage identification, but a multidisciplinary approach is needed for advanced-stage identification.102 In the case of OSCC, it is mainly managed with surgery; however, radiotherapy and chemoradiotherapy are used post-operatively for disease control.103 Immune checkpoint blockade (ICB) therapy has been used for OSCC since 2016 and has been found to have a low response rate and adverse events.104
It has been found that little attention has been given to monitoring the levels of different salivary biomarkers and metabolites throughout and after treatment, such as chemotherapy, radiation, and immunotherapy. A detailed cohort study is required for various biomarkers and their concentration during the disease and treatment progression.
6. ‘ELISA’: a conventional gold standard method for oral cancer detection
The application of immunoassays for the identification of infectious disorders can be traced back to the year 1917 when they were initially employed for the rapid identification of antigens through interaction with antibodies. Enzyme-linked immunosorbent assay (ELISA) and radioimmunoassays (RIA) represent two prominent immunoassay-based platforms that have undergone substantial investigation to develop novel, highly sensitive, and point-of-care diagnostic tools for disease detection. Currently, ELISA continues to be widely utilized as a prevalent immunoassay technique for identifying and quantifying biomarkers within laboratory settings.105–108 ELISA techniques rely on the selective binding interactions between antigens and antibodies for target proteins in body fluids. Nevertheless, the limited sensitivity of these methods restricts their utilization in modern laboratory protocols.109
Moreover, the utilization of ELISA is again constrained by the expensive test kits, equipment, long measurement time, and the challenges encountered when attempting multiplexing. Therefore, ELISA is not typically the preferred option for the implementation of point-of-care diagnostics.110
The search for innovative, cost-effective, and user-friendly point-of-care devices designed for the diagnosis of oral cancer in low-resource settings concludes with microfluidic devices which are discussed in detail in the following sections.
7. Microfluidic devices
Recently, research interest has grown in ‘micro total analysis systems’ (μ-TASs) or ‘lab-on-chip’ (LOC). There are generally two main approaches in microfluidics. The first is combining microsensors with fluidic components such as a pump and flow chips, and the other is miniaturizing various analytical chemical methods.111 Moreover, extensive research is focused on the ‘organ-on-a-chip’ (OoC) for pre-clinical and clinical translational precision. The current research is centered around streamlining the intricate nature of human organ architecture, specifically elucidating its fundamental cellular microanatomy. This simplification enables a detailed investigation into the mechanisms underlying drug action, absorption, metabolism, and other related processes.112–118
Another fascinating approach used in microfluidic systems is ‘origami,’ which is the folding of paper. Origami is used in various applications such as tissue engineering, tissue scaffolds, drug delivery, stents, catheters, etc.119 Another low-cost and effective microfluidic device for point-of-care (POC) testing is lateral flow assays (LFAs), also known as lateral flow test strips (LFTSs). Cellulosic paper and plastic supports are the first choices to maintain the low cost of the fabrication of LFAs. In addition to the low cost, the World Health Organization (WHO) insists that all the POC devices must follow the REASSURED criteria, i.e., real-time connectivity, ease of specimen collection, affordable, sensitive, specific, user-friendly, rapid and robust, equipment-free, and delivered to the end-users. Numerous LFAs reported to date for various cancer-related biomarkers can be seen in the review by Mahmoudi et al.120–122
7.1. Colorimetric paper-based sensors
Colorimetric sensors rely on the alteration in color when the analyte of interest is present, as implied by their name. The colorimetric signal can be detected with the naked eye. Paper-based sensors are successfully deployed for qualitative and semiquantitative measurements. Colorimetric paper-based analytical devices (PADs) include spot tests, LFAs, dipsticks, and 2D/3D μPADs. Dipsticks are not so popular for cancer detection owing to their requirement of high volume of samples.123
7.1.1. Lateral flow assays (LFAs). LFAs have gained recognition as prominent POC devices due to their efficacy in qualitative, semi-quantitative, or quantitative detection of several biomarkers.124–127 LFAs working with various detection systems can be categorized as colorimetric, luminescent, magnetic, electrochemical, surface-enhanced Raman spectroscopy (SERS), and thermal detection, as shown in Fig. 2.128 It is essential for the detection system to have powerful signal amplification for the precise detection of various analytes. Herein, various LFAs with different detection systems are discussed in detail. Fig. 2 shows various detection systems deployed in the LFAs.
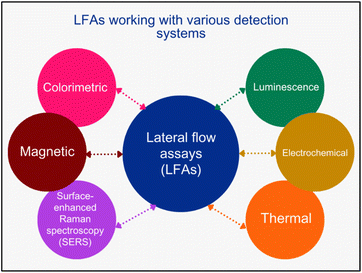 |
| Fig. 2 Schematic showing various detection systems used in LFAs. | |
7.1.2. Basic working principle of LFAs. The critical component of the LFA is a nitrocellulose (NC) membrane, which provides the platform for the reactions during the analysis. The antibodies interact with the nitrate ester through the peptide bond and strong dipoles of the ester, resulting in the electrostatic interaction. After the interaction of the antibodies on the test membrane, it is dried so that the antibodies remain fixed on the test NC. Polyvinyl alcohol is used to inhibit the activity of free active sites on the membrane. The sucrose solution forms a hydrated glaze that is quickly and efficiently dissolved when the aqueous sample is dropped and helps in the unrestricted mobility of the labeled antibodies. In this way, a test region and a control region are developed. The biorecognition elements, i.e., captured molecules, remain on the NC in the respective test or control region via hydrogen bonds, electrostatic interaction, and/or hydrophobic forces. Capillary forces drive the sample movements on the NC; the specific interaction, depending on the sample type, occurs in the test region (T-line) and the control region (C-line), giving the results of the conducted test depending on the types of the antigen to be detected and the antibodies deployed. Depending on the type of detection mechanisms deployed, it can be divided into sandwich format and competitive format LFAs. Details of this can be seen in the review by Elif Burcu Bahadır et al.129 The basic working principle of an antibody-antigen-antibody sandwich LFA is shown in the following schematic, in Fig. 3.
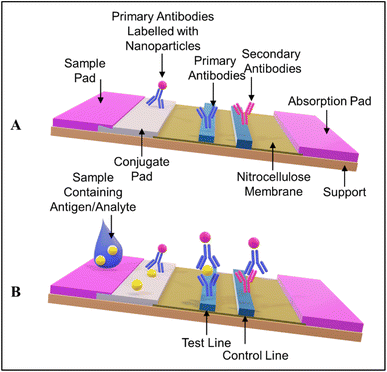 |
| Fig. 3 (A) The architecture of a standard LFA. (B) Basic working principle of an antibody-antigen-antibody sandwich LFA. | |
Here, selected recently reported LFAs, which are based on various detection mechanisms, are discussed. Several detection systems, including distance-based, lanthanide-based, cascade nucleic acid amplification, upconversion nanoparticle, and immuno-sandwich assay, are discussed in detail.
7.1.3. Distanced-based colorimetric detection. Recently, a distance-based paper sensor (dPADs) was developed by Kawin Khachornsakkul et al. The paper-based analytical device could quantitatively detect interleukin-6 (IL-6) from human saliva and urine samples. They proposed a hydrophilic bridge valve coupled with a dPAD (B-dPAD) for distance-based colorimetric immunoassay. This approach helps in the reduction of washing steps, the time required, and the complexity involved, and it improves the analytical performance. The measuring step entails methylene blue (MB) decomposition utilizing hydrogen peroxide (H2O2). The developed B-dPADs comprise three distinct regions: a sample zone, a capture zone, and a detection zone. Two hydrophilic bridge valves separate these regions. The workflow is shown in Fig. 4.
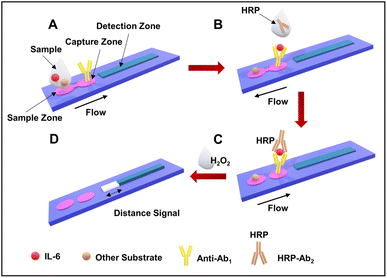 |
| Fig. 4 Assay workflow of B-dPADs for IL-6 detection. (A) Sample addition and the binding of antibodies. (B) The addition of the secondary HRP-labeled antibodies. (C) The flow of liquid in the capture zone and then in the detection channel. (D) The quantification of the bleaching observed in the detection channel. Reproduced/re-drawn with permission from ref. 130. Copyright 2022 American Chemical Society. | |
The fabricated B-dPADs were evaluated for IL-6 quantification in the range of 0.05–25.0 pg mL−1 (see Fig. 5). The measurement of the detection zone was conducted using a conventional ruler and subsequently graphed. The bleaching length was linear in the tested range and was suitable for the clinical range of 5.0–15.0 pg mL−1, with an R2 value of 0.9992.130
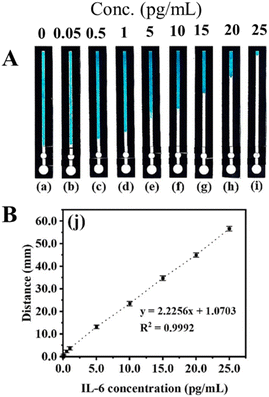 |
| Fig. 5 (A) Photographs of devices with the IL-6 assay performed at various concentrations. (B) The calibration curve. Reproduced with permission from ref. 130. Copyright 2022 American Chemical Society. | |
7.1.4. A lanthanide-based fluorescence detection system. Lanthanide-based LFAs are extensively employed techniques, with particular emphasis on the utilization of Eu(III) chelates. These chelates exhibit notable and appealing properties, including time-resolved fluorescence and a significant Stokes shift. A novel mini-emulsion polymerization technique was employed by Zhenhua Li et al. to produce carboxyl-modified fluorescent microspheres (referred to as OS-EuCM) achieved through a one-step process. The OS-EuCM microspheres exhibited favorable attributes such as high stability, specific adhesion, and uniform distribution of particle sizes. These characteristics were superior to those of conventional microspheres obtained using the swelling method.The developed LFAs were used for the detection of alpha-fetoprotein, a tumor marker. The increased sensitivity and specificity exhibited by the developed LFA make it highly suitable for diagnostics in clinical settings. LFAs could detect a higher concentration of 320 ng mL−1, with a detection limit of 0.683 ng mL−1. A schematic representation of the structure of the OS-EuCM, biconjugation process, and fabrication of the LFA is shown in Fig. 6.
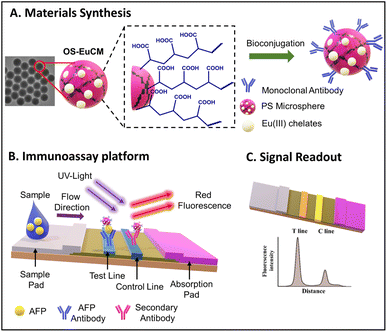 |
| Fig. 6 A schematic representation of the structure and bioconjugation process of OS-EuCM, as well as the corresponding fabrication of lateral flow immunoassays (LFIAs) for detecting AFP. Reproduced/re-drawn with permission from ref. 131. Copyright 2021 Chinese Society of Rare Earths. | |
OS-EuCM shows better fluorescence intensity under ultraviolet (UV) light as opposed to conventional microspheres synthesized using the swelling method shown in Fig. 7(A). OS-EuCM was used for the fabrication of test strips, as shown in Fig. 7. Succinctly, following the pre-mixing of the serum sample with the OS-EuCM labeled AFP-specific antibody, the resulting complexes were introduced onto the sample pad and subsequently passed over the nitrocellulose membrane. The concentration of AFP in the sample exhibited a direct relationship with the ratio of HT/HC. HT and HC are the fluorescence peak heights for the test and control lines, respectively. The various concentrations tested and the optical images of the strips are shown in Fig. 7(B).131
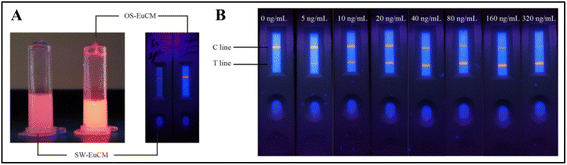 |
| Fig. 7 (A) The photographs depict two distinct types of microsphere solutions and strips after film drawing, each containing identical solid contents, which were subjected to irradiation at a wavelength of 365 nm. (B) Optical images of tested strips exhibiting various alpha-fetoprotein (AFP) concentrations under irradiation at a wavelength of 365 nm. Reproduced with permission from ref. 131. Copyright 2021 Chinese Society of Rare Earths. | |
The fabricated strips were tested for AFP concentration in the range of 0–320 ng mL−1 under irradiation at a wavelength of 365 nm, as shown in Fig. 7(B).
7.1.5. Cascade nucleic acid amplification (HRCA)-based colorimetric detection. Another LFA with the HRCA mechanism was developed and deployed for the detection of miRNA 31 for OSCC diagnosis by Wenna Li et al. The miRNA under investigation demonstrated complementary sequences with both biotin-modified probes (referred to as b-Lcp) that were affixed to Streptavidin-modified magnetic beads, as well as with initiator strand probes (referred to as Dp). With target miRNA, it initiates hybridization with b-Lcp and Dp, resulting in the formation of the sandwich structure.They have employed two specific probes acting as the primer for the rolling circle amplification (RCA) reaction. After various series of subsequent reactions, the final blue color signal was observed on the streptavidin-labeled strip.
The detailed schematic of the working principle is given in the following Fig. 8.132
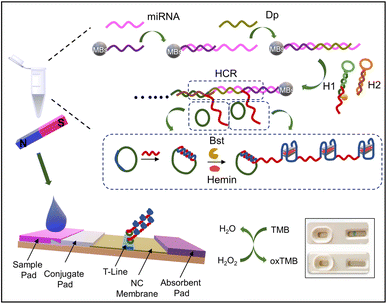 |
| Fig. 8 LFA: based on the cascade nucleic acid amplification technology for miRNA 31 for OSCC diagnosis. Reproduced/re-drawn with permission from ref. 132. Copyright 2022 Elsevier B.V. | |
7.1.6. Upconversion nanoparticle-based luminescence detection system. Upconversion nanoparticles (UCNPs) belong to a distinctive category of optical nanomaterials and are usually obtained by lanthanide ion doping. These nanoparticles exhibit a wide range of electronic transitions within their 4f electron shells and can undergo up-conversion, a process when two or more photons with lower energy levels are converted into a single photon with higher energy levels.133 Wanghong He et al. developed an LFA by deploying core–shell upconversion nanoparticles (G-UCNPs) as a luminescent probe. The developed multiplex LFA was used for the detection of three periodontitis biomarkers in gingival crevicular fluid (GCF), matrix metalloproteinases-8 (MMP-8), interleukin-1 beta (IL-1β) and tumor necrosis factor-alpha (TNF-α).The working principle is shown in Fig. 9. A sterile Whatman strip was used for GCF sample collection, and the strip was transferred to a sterile Eppendorf tube and eluted (as shown in Fig. 9(A) and (B)). The amount of the sample was also taken into consideration for accurate measurements. The sample was introduced to a disk-like platform, where there will be an interaction with the three kinds of pre-added probes for the detection of MMP-8, IL-1β, and TNF-α, T, and C lines (Fig. 9(C)). The immune-sandwich assay was the basis of the detection mechanism. The general detection mechanism can be discussed by using the example of MMP-8. MMP-8 antigens from the samples bind specifically with detection antibodies on G-UCNPs, forming G-UCNPs-MMP-8 conjugates on the conjugation pad (Fig. 9(D)). The migration of conjugates through the nitrocellulose (NC) membrane is facilitated by capillary force, leading to their capture by the MMP-8 capture antibodies (T line). This results in an antibody-antigen-antibody sandwich structure at the T line (Fig. 9(E)). The detection antibodies used in this study are generated from mice; it is expected that any surplus G-UCNP probes that fail to bind to the analytes will be captured by the goat anti-mouse IgG located on the C line. In the absence of an analyte within the sample, the probes will only bind to the goat anti-mouse IgG located on the C line. The luminescence signals on the G-UCNPs-LFIS are captured using a camera and afterward processed using Image J software for the concentration measurements.134
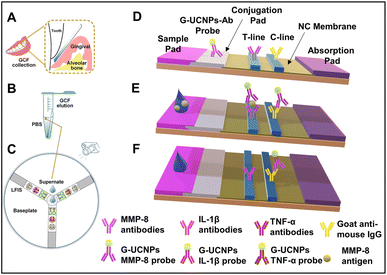 |
| Fig. 9 Working principle of the developed upconversion nanoparticle based LFA. (A) Sample collection, (B) sample elution, (C) disk-like detection platform, and (D–F) schematic showing the working principle. Reproduced/re-drawn with permission from ref. 134. Copyright 2021 Elsevier B.V. | |
A summary of recently reported LFAs with the colorimetric and fluorescence-based detection systems is given in Table 3. Such detection systems are suitable for low-resource settings and ideal for mass screening.
Table 3 A summary of recently reported LFAs with colorimetric and fluorescence-based detection systems
Biomarker/bioanalyte |
Detection mechanism |
Tag/signal amplification |
Type of sample |
Sample volume |
Detection time |
Linear range |
LOD |
Ref. |
Helicobacter pylori |
Fluorescence |
Time-resolved fluorescent microspheres |
Saliva |
60 μL |
8 min |
101–105 CFU mL−1 |
102 CFU mL−1 |
135 |
Human osteopontin (OPN) protein |
Colorimetric |
Streptavidin-modified gold nanoparticles |
Serum |
20 μL |
5 min |
10–500 ng mL−1 |
0.1 ng mL−1 |
136 |
Human epidermal growth factor receptor 2 (HER2) |
Colorimetric |
Biotin-modified aptamer modified gold nanoparticles |
Serum |
Not given |
30 min |
0–99 nM |
24 nM |
137 |
Prostate-specific antigen |
Colorimetric |
Platinum nanoparticles |
Serum |
Not given |
30 min |
0–12 ng mL−1 |
0.54 ng mL−1 |
138 |
Alpha-fetoprotein |
Fluorescence |
Lanthanide-based carboxyl-modified fluorescent microspheres |
NA |
110 μL |
Not given |
0–320 ng mL−1 |
0.683 ng mL−1 |
131 |
Human papillomavirus (HPV) type 16 DNA |
Colorimetric |
Copper oxide nanoparticles |
NA |
30 μL |
20 min |
5–100 nM |
1.0 nM |
139 |
Helicobacter pylori with cytotoxin-associated protein (CagA) |
Fluorescence |
CdS quantum dots |
Serum |
Not given |
Not given |
Not given |
20 pg mL−1 |
140 |
Human papilloma virus (HPV): HPV16 and HPV18 |
Colorimetric |
Gold nanoparticles and CIALFB (CRISPR/Cas-isothermal amplification based LFB) |
Cervical exfoliated cell samples |
2–4 μL |
<60 min |
Not given |
3.1 attomoles (∼1.8 copies) |
141 |
β-Galactosidase |
Colorimetric and fluorescence |
The recombinant spores and gold nanoparticles |
NA |
100 μL |
15–20 min |
Not given |
10−15 mol |
142 |
Apart from LFAs, colorimetric detection is an alternative for low-cost detection of oral cancer. As discussed earlier, colorimetric detection of oral cancer using ELISA was the most popular and widely used technique. However, low detection sensitivity, high costs, high-end laboratory settings, and longer experimental time are the limitations of the use of ELISA. However, gold and silver nanoparticles are highly explored for the development of various colorimetry-based assays for the detection of various biomarkers due to their surface plasmon resonance, high sensitivity, and ease of surface modification. The results can be interpreted using the basic principle of colorimetry. Thus, the following section discusses various gold and silver nanoparticle-based microfluidic detection systems.
8. Gold and silver nanoparticle-based sensors
Gold and silver nanoparticles are frequently used metallic nanoparticles in microfluidic systems. Nanoparticles typically serve as elements for capturing targets and/or transmitting signals, owing to their localized surface plasmon resonance (LSPR), catalytic activity, and conductivity. These nanoparticles are generally incorporated into the microfluidic systems using in situ synthesis or simply drop casting before or after mixing the analyte. These nanoparticles are also widely used in colorimetric detection using UV-vis spectroscopy.7,143–147
8.1. Functionalization of gold and silver nanoparticles
The critical factor in the use of gold and silver nanoparticles for point-of-care devices includes functionalization. The several well-established functionalization techniques include thiol modifications, EDC-NHS coupling reactions, avidin-biotin coupling reactions, and π- π interactions, as shown in Fig. 10.148 These functionalization methods are used for the conjugation of antibodies for the respective detection of the antigens and are widely deployed strategies for specificity.
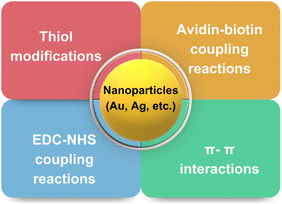 |
| Fig. 10 Various strategies deployed routinely for surface modifications of nanoparticles. | |
Here, a few gold and silver nanoparticle-based systems employed as a sensor via surface functionalization of nanoparticle surfaces are discussed. Jimenez et al. developed a gold nanoparticle-based detection system for the detection of human papillomavirus (HPV). The developed system consists of modified gold nanoparticles, with thiolated oligodeoxyribonucleotides consisting of the complementary sequence to the DNA sequence of the HPV oncogene and the modified CdTe quantum dots (QDs) for site-specific conjugation. The modified CdTe quantum dots act as a fluorescence tag in the detection system. The overall working principle is shown in Fig. 11(A).149 In another study by Kim et al., a stimuli-responsive contrast agent was developed for optical coherence tomography (OCT) using gold nanoparticles and acid-cleavable diaminoketal cross-linkers. It was shown that the developed contrast agent was able to detect early stage cancers (see Fig. 11(C)).150 Due to multiplexed capacity and high sensitivity, surface-enhanced Raman scattering (SERS) has gained a lot of attention in biosensing. Liu et al. developed an Ag nanocube-based SERS system for the detection of oral cancer DNA. The silver nanocube surface was modified with signal DNA and 4-mercaptobenzoic acid as a Raman reporter. The cubic morphology of the deployed silver nanoparticles was able to produce a strong SERS signal. A heated gold electrode was also deployed in addition to functionalized silver nanocages to achieve amplification of the targeted DNA. The schematic of the sensor development is shown in Fig. 11(B).151
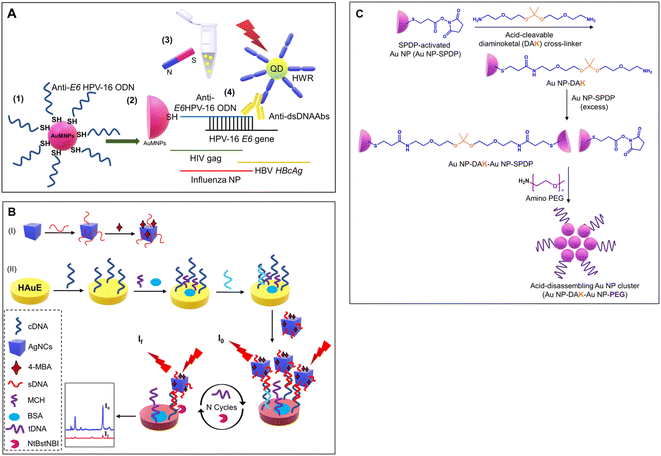 |
| Fig. 11 (A) Schematic showing specific detection of HPV using functionalized gold nanoparticles and fluorescent QDs. Reproduced/re-drawn with permission from ref. 149. Copyright 2016 Elsevier B.V. (B) Figure illustrates the fabrication of a Ag-nanocube-based SERS biosensor for DNA detection. Reproduced/re-drawn with permission from ref. 151. Copyright 2021 Elsevier B.V. (C) Fabrication of acid-sensitive gold nanoclusters as a contrast agent for optical coherence tomography (OCT). Reproduced/re-drawn from ref. 150. Creative Commons license. | |
8.2. Low-cost gold/silver nanoparticle-based detection systems
The development of multiplex systems is of great interest in increasing the confidence level of disease diagnosis. Tomas Pinheiro et al. developed a gold nanoparticle-based colorimetric, non-enzymatic multiplex microfluidic paper-based detection system. The developed device was capable of detecting uric acid, cholesterol, and glucose. The limit of detection obtained was 1.25 mM, 71 μM, and 81 μM for glucose, uric acid, and cholesterol, respectively, which were comparable those of similar reported systems. The developed device was an example of a colorimetric, microfluidic, paper-based, non-enzymatic detection system. The schematic of the device fabrication is shown in Fig. 12.152
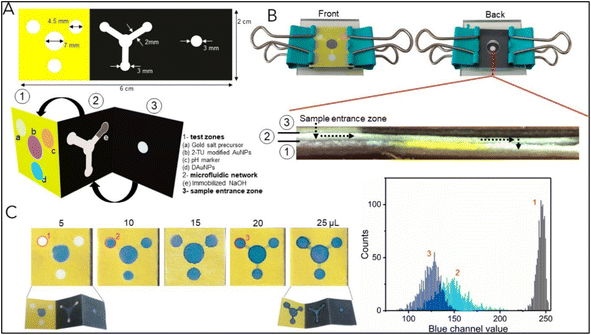 |
| Fig. 12 The schematic of the device fabrication. (A) Dimension of the wax pattern used and other components: (1) test zones, (2) microfluidic network, and (3) sample entrance zone. The device is appropriately folded into a suitable conformation for inter-component interaction (B) The device is assembled by integrating it into the acrylic sheet and springs. A cross-sectional view of the device is presented to illustrate its configuration following the assembly process (sample flow is shown by a dashed arrow). (C) Flow assay to ascertain the minimal sample volume necessary for the device to function correctly. The graph shows a color histogram obtained from three separate test zones. Reproduced with permission from ref. 152. Copyright 2021 American Chemical Society. | |
Another study using gold nanorods (GNRs) with varying aspect ratios (ARs) was performed by Debolina Chakraborty et al. for the multiplex colorimetry-based detection system of Cyfra 21-1 and CA-125. The designed assay could detect the mentioned biomarker in the salivary concentration range and was tested for artificial saliva. In this study, the antibodies targeting Cyfra 21-1 and CA-125 were utilized as probes to functionalize the gold nanorods with varying aspect ratios. The quantitative evaluation of the target analyte was assisted through antigen–antibody interactions that caused a spectrum shift of specific plasmon band maxima. The developed GNR probe could sense Cyfra 21-1 and CA-125 with a detection limit of 0.84 ng mL−1 and 1.6 U mL−1, respectively. The study introduces a revolutionary nanoscale optical transducer technology that exhibits simplicity in design and implementation.
GNRs were modified with anti-Cyfra 21-1 and anti-CA-125 using EDC-NHS chemistry to detect the respective antigen from the artificial saliva samples. GNR probes were suitable for multiplex detection owing to their different aspect ratios and peak positions. This technology holds the potential for further development into miniaturized biochips suitable for low-resource settings.153 The GNR probe development and analysis using UV-visible spectroscopy is shown in Fig. 13. In such a system, colorimetric detection can also be performed using low-cost portable optical detection systems ideal for mass screening in low-resource settings. Our group has developed several IoT-enabled portable colorimetry-based microfluidic detection systems.154,155 Such devices with slight modifications to the photodiode and a suitable light source (LED) of the required wavelength can be applied for a wide spectral range.
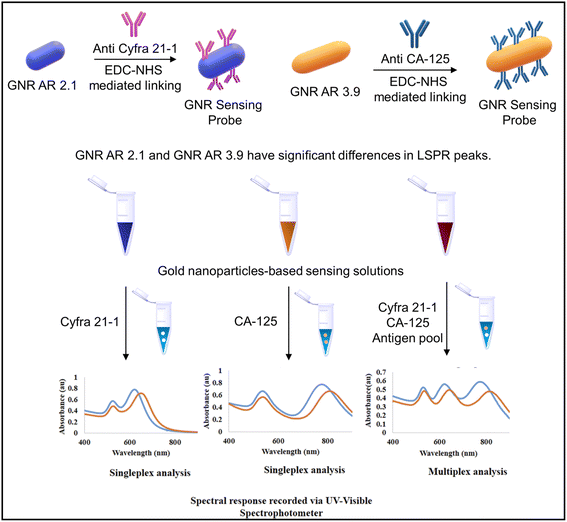 |
| Fig. 13 The schematic depicting GNR probe development and the UV-visible spectroscopy-based spectral analysis of Cyfra 21-1 and CA-125 antigens. Reproduced/re-drawn with permission from ref. 153. Copyright 2022 The Royal Society of Chemistry. | |
Dongyang Li et al. deployed gold and silver nanoparticles with different sizes. The diameters of the gold nanoparticles synthesized were 13, 25, 35, 50, and 60 nm, and for silver nanoparticles, the diameters were 25 and 31 nm to detect various proteins and bacteria without any functionalization of the nanoparticles. The developed colorimetric sensor arrays were tested for ten different proteins with 0.5, 5, and 50 μM concentrations. The proteins used in the study were cytochrome C (Cyt C), myoglobin (Mb), trypsin (Try), pepsin (Pep), lysozyme (Lys), papain (Pap), hemoglobin (Hb), casein (Cas), bovine serum albumin (BSA), and lipase (Lip). Seven types of bacteria were used as analytes, including CRPA, Acetobacter aceti, Rhodopseudomonas, Bacillus natto, Staphylococcus, E. coli, and Bacillus (see Fig. 14(B) and (C)). The types of cancer cells analyzed include OSCC cells, HeLa cells, PC3 cells, and A549 human lung adenocarcinoma cells.
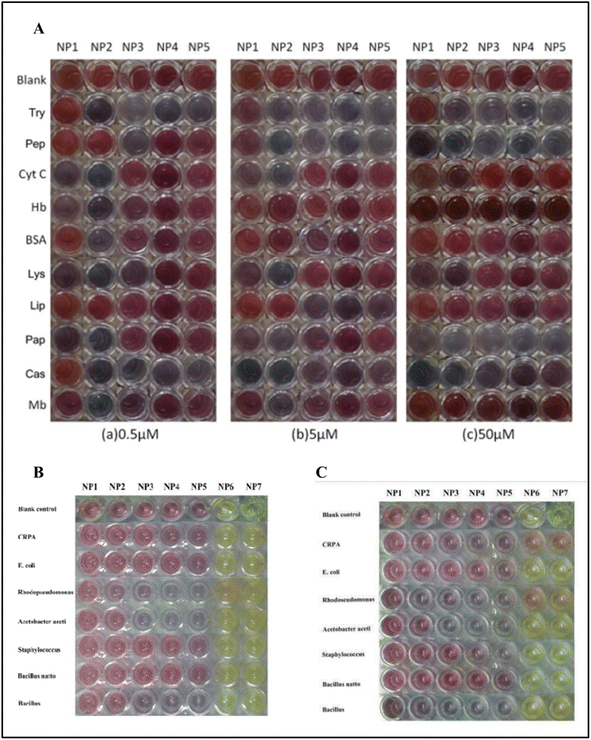 |
| Fig. 14 (A) The picture shows the color pattern developed with gold nanoparticles with varying sizes upon interaction with different proteins. (B) The interaction of the gold nanoparticles and silver nanoparticles NP6 (25 nm) and NP7 (31 nm) against bacteria after 20 min; (C) after 6 hours. Reproduced with permission from ref. 156. Copyright 2015 The Royal Society of Chemistry. | |
Depending upon the protein's interaction type and the nanoparticles' size, a unique fingerprint color was obtained, which can be detected by linear discriminant analysis (LDA). The developed detection system could detect bacteria with a concentration of 0.05 OD in 200 μL. Interestingly, the array was also capable of the detection of cancer cells with a concentration of 5000 cells in 200 μL as shown in Fig. 15.156
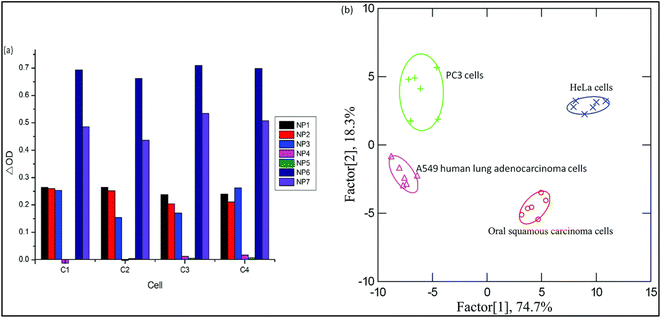 |
| Fig. 15 The identification of various cancer cells. The absorbance pattern of seven nanoparticles against cancer cells (Left (a)) and the canonical score plot (Right (b)). Reproduced with permission from ref. 156. Copyright 2015 The Royal Society of Chemistry. | |
9. Challenges and future perspectives
Colorimetry-based microfluidic devices have several benefits owing to the recent developments in the field and are an ideal solution for early oral cancer detection. However, several challenges remain, as discussed below and shown in Fig. 16.
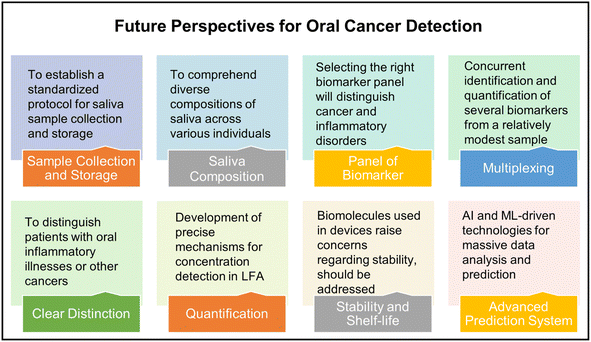 |
| Fig. 16 Schematic showing future perspectives for oral cancer detection using colorimetry-based microfluidic devices and salivary biomarkers. | |
In contemporary practice, saliva has emerged as a favored specimen for the purpose of oral cancer biomarker testing, surpassing alternative samples. This preference stems from the fact that saliva exhibits direct contact with lesions within the mouth cavity, its non-invasive nature, and its convenient collection process. Initially, it is imperative to comprehend the considerably diverse composition of saliva across various individuals. Furthermore, it is crucial to distinguish between oral inflammatory illnesses and other cancers since they may exhibit elevated levels of salivary biomarkers. This differentiation is crucial to enhance the sensitivity of salivary biomarker detection. Moreover, it is necessary to establish a standardized protocol for the saliva sample collection and storage as this will play a crucial role in the final analysis. Multiplexing is essential for accurate diagnosis. Nevertheless, the selection of an appropriate panel of biomarkers will effectively differentiate between various types of cancer and/or inflammatory disorders. This objective can be accomplished by extensively investigating a diverse range of positive samples obtained from individuals spanning different age groups, genders, and geographical locations.
The significance of paper-based microfluidic devices lies in their cost-effectiveness, portability, and simplified operation. Furthermore, the integration of diverse point-of-care devices with the Internet of Things (IoT) proves to be advantageous for conducting screenings in remote regions where the availability of advanced laboratory facilities is limited. Paper-based systems do not possess precise mechanisms for concentration detection. Multiplexing is another crucial aspect that warrants consideration. The device should be able to concurrently identify and quantify many biomarkers from a single, relatively modest sample. In addition, most paper-based devices utilize a range of antibodies and other biomolecules, raising concerns regarding their storage, transportation, and shelf-life.
Lastly, artificial intelligence (AI) and machine learning (ML) aided technologies should be extensively developed and deployed for massive data analysis and for early oral cancer diagnosis.
10. Conclusion
The present study examines a range of salivary biomarkers that are known to exhibit substantial alterations in concentration in oral malignancies. The discussion has extensively covered several salivary biomarkers, examining their structure and importance in the early identification and progression of oral cancer. As elucidated in the review above, the salivary biomarkers under consideration exhibit considerable promise in the early identification of oral cancer and monitoring disease advancement. Nevertheless, it is essential to note that the identification of oral cancer cannot be reliably achieved using a single biomarker. This is because numerous biomarkers have been observed to exhibit alterations in various types of malignancies, as well as some other medical disorders. Multiplex detection is a reliable method for early detection, offering advantages such as specificity, precision, and high levels of confidence. The conventional gold standard ELISA method has several drawbacks, such as expensive text kits requiring highly skilled technicians, laboratory settings, and high detection time. Multiplexing with the ELISA is another challenge. The incorporation of microfluidics as a platform in many biosensors has facilitated enhanced automation capabilities, simplified multiplexing, and reduced processing durations, and enabled high-throughput analysis. Utilizing paper-based microfluidics to identify salivary biomarkers effectively represents a facile, economical, and portable approach to developing new biosensors. Paper-based microfluidics are highly suitable for low-resource settings due to their adherence to the REASSURED criteria outlined by the World Health Organization. LFAs and paper-based low-cost devices working on various detection systems have been discussed in detail. Such low-cost devices with high accuracy and detection precision can be deployed for mass screening for early diagnosis.
Data availability
No primary research results, software, or code have been included, and no new data were generated or analyzed in this review.
Author contributions
Aniket Balapure: conceptualization, data curation, formal analysis, investigation, writing – original draft. Satish Kumar Dubey: funding acquisition, project administration, supervision, writing – reviewing and editing. Arshad Javed: funding acquisition, project administration, supervision, writing – reviewing and editing. Samit Chattopadhyay: funding acquisition, project administration, supervision, writing – reviewing and editing. Sanket Goel: funding acquisition, project administration, supervision, writing – reviewing and editing.
Conflicts of interest
The authors declare that they have no known competing financial interests or personal relationships that could have appeared to influence the work reported in this paper.
Acknowledgements
This work was supported by the BITS BioCyTiH Foundation, India [BBF/BITS(G)/FY2022-23/BCPS-110]. A. B. would like to thank the BITS BioCyTiH Foundation, India for fellowship support.
References
- E. Nakamichi, H. Sakakura, S. Mii, N. Yamamoto, H. Hibi, M. Asai and M. Takahashi, Oral Dis., 2021, 27, 439–447 CrossRef PubMed.
- N. J. D'Silva, C. Perez-Pacheco and L. B. Schmitd, Adv. Biol., 2023, 7 Search PubMed.
- S. Chupradit, S. A. Jasim, D. Bokov, M. Z. Mahmoud, A. B. Roomi, K. Hachem, M. Rudiansyah, W. Suksatan and R. Bidares, Anal. Methods, 2022, 14, 1301–1310 RSC.
- O. J. Old, L. M. Fullwood, R. Scott, G. R. Lloyd, L. M. Almond, N. A. Shepherd, N. Stone, H. Barr and C. Kendall, Anal. Methods, 2014, 6, 3901–3917 RSC.
- S. Verma, A. Singh, A. Shukla, J. Kaswan, K. Arora, J. Ramirez-Vick, P. Singh and S. P. Singh, ACS Appl. Mater. Interfaces, 2017, 9, 27462–27474 CrossRef CAS PubMed.
- B. L. Ziober, M. G. Mauk, E. M. Falls, Z. Chen, A. F. Ziober and H. H. Bau, Head Neck, 2008, 30, 111–121 CrossRef PubMed.
- Y. Xiang, C. Hu, G. Wu, S. Xu and Y. Li, TrAC, Trends Anal. Chem., 2023, 158, 116835 CrossRef CAS.
- V. Borse, A. N. Konwar and P. Buragohain, Sens. Int., 2020, 1, 100046 CrossRef PubMed.
- S. C. Chang, W. L. Lin, Y. F. Chang, C. T. Lee, J. S. Wu, P. H. Hsu and C. F. Chang, J. Food Drug Anal., 2019, 27, 483–493 CrossRef CAS PubMed.
- Y. W. Lin, S. T. Huang, J. C. Wu, T. H. Chu, S. C. Huang, C. C. Lee and M. H. Tai, BMC Cancer, 2019, 19, 1083 CrossRef PubMed.
- Y. T. Chang, L. J. Chu, Y. C. Liu, C. J. Chen, S. F. Wu, C. H. Chen, I. Y. F. Chang, J. S. Wang, T. Y. Wu, S. Dash, W. F. Chiang, S. F. Chiu, S. B. Gou, C. Y. Chien, K. P. Chang and J. S. Yu, Cancers, 2020, 12, 2273 CrossRef CAS PubMed.
- A. Manz, E. Verpoorte, D. E. Raymond, C. S. Effenhauser, N. Burggraf and H. M. Widmer, Micro Total Analysis Systems, ed. A. Van den Berg and P. Bergveld, Springer Netherlands, Dordrecht, 1995, pp. 5–27 Search PubMed.
- B. D. Cardoso, E. M. S. Castanheira, S. Lanceros-Mendez and V. F. Cardoso, Adv. Healthcare Mater., 2023, 2202936 CrossRef CAS PubMed.
- E. Solhi, M. Hasanzadeh and P. Babaie, Anal. Methods, 2020, 12, 1398–1414 RSC.
- H. A. Silva-Neto, I. V. S. Arantes, A. L. Ferreira, G. H. M. do Nascimento, G. N. Meloni, W. R. de Araujo, T. R. L. C. Paixao and W. K. T. Coltro, Trends Anal. Chem., 2023, 158, 116893 CrossRef CAS.
- A. Kumar, D. Jain, J. Bahuguna, M. Bhaiyya, S. K. Dubey, A. Javed and S. Goel, Biosens. Bioelectron., 2023, 238, 115582 CrossRef CAS PubMed.
- M. L. Bhaiyya, S. K. Srivastava, P. K. Pattnaik and S. Goel, IEEE Trans. Instrum. Meas., 2023, 72, 1–8 Search PubMed.
- J. M. Mohan, S. Kumar, K. Amreen, A. Javed, S. K. Dubey and S. Goel, IEEE Sens. J., 2023, 23, 16189–16196 CAS.
- M. Sharafeldin, T. Chen, G. U. Ozkaya, D. Choudhary, A. A. Molinolo, J. S. Gutkind and J. F. Rusling, Biosens. Bioelectron., 2021, 171, 112681 CrossRef CAS PubMed.
- F. Wehrhan, M. Weber, C. Baran, A. Agaimy, M. Buttner-Herold, M. Kesting and J. Ries, J. Cranio-Maxillofacial Surg., 2021, 49, 118–125 CrossRef PubMed.
- X. Zhang and B. Li, Oral Dis., 2023, 29, 51–61 CrossRef PubMed.
- M. Hasanzadeh, N. Shadjou and M. de la Guardia, Trends Anal. Chem., 2017, 91, 125–137 CrossRef CAS.
- K. E. Kaczor-Urbanowicz, F. Wei, S. L. Rao, J. Kim, H. Shin, J. Cheng, M. Tu, D. T. W. Wong and Y. Kim, Biochim. Biophys. Acta Rev. Canc., 2019, 1872, 49–59 CrossRef CAS PubMed.
- D. Kademani, Mayo Clin. Proc., 2007, 82, 878–887 CrossRef PubMed.
- M. Zhao, C. Ji, H. Dai, C. Wang, R. Liu, J. Xie, Y. Wang and Z. Gu, ACS Appl. Mater. Interfaces, 2023, 15, 4984–4995 CrossRef CAS PubMed.
- K. Mahato, A. Kumar, P. K. Maurya and P. Chandra, Biosens. Bioelectron., 2018, 100, 411–428 CrossRef CAS PubMed.
- O. Hershkovich, I. Shafat and R. M. Nagler, J. Gerontol., Ser. A, 2007, 62, 361–366 CrossRef PubMed.
- B. N. Kallalli, K. Rawson, Muzammil, A. Singh, M. A. Awati and P. Shivhare, J. Oral Pathol. Med., 2016, 45, 687–690 CrossRef CAS PubMed.
- W. Jin, M. Zhu, Y. Zheng, Y. Wu, X. Ding, H. Wu, J. Ye, Y. Wu, Z. Zhu and X. Song, Oral Dis., 2022, 28, 132–141 CrossRef PubMed.
- T. P. Mafessoni, C. E. Mazur and J. M. Amenabar, Med. Hypotheses, 2018, 119, 29–31 CrossRef CAS PubMed.
- M. Moradi Binabaj, A. Bahrami, M. Khazaei, M. Ryzhikov, G. A. Ferns, A. Avan and S. Mahdi Hassanian, Gene, 2020, 728, 144283 CrossRef CAS PubMed.
- A. Pecoraro, P. Carotenuto, G. Russo and A. Russo, Sci. Rep., 2019, 9, 15431 CrossRef PubMed.
- S. Manna, R. Kirtana, A. Roy, T. Baral and S. K. Patra, Arch. Biochem. Biophys., 2023, 742, 109600 CrossRef CAS PubMed.
- J. Kaur, M. Preethi, R. Srivastava and V. Borse, Biosens. Bioelectron.: X, 2022, 11, 100212 CAS.
- L. Zhong, C. Zhang, J. Zheng, J. Li, W. Chen and Z. Zhang, Arch. Oral Biol., 2007, 52, 1079–1087 CrossRef CAS PubMed.
- K. Rajkumar, R. Ramya, G. Nandhini, P. Rajashree, A. Ramesh Kumar and S. Nirmala Anandan, Oral Dis., 2015, 21, 90–96 CrossRef CAS PubMed.
- S. S. Sawant, D. A. Chaukar, S. S. Joshi, P. P. Dange, S. Kannan, S. Kane, A. K. D'Cruz and M. M. Vaidya, Oral Oncol., 2011, 47, 114–120 CrossRef CAS PubMed.
- S. S. Sawant, S. M. Zingde and M. M. Vaidya, Oral Oncol., 2008, 44, 722–732 CrossRef CAS PubMed.
- P. Panta and D. T. W. Wong, Oral Cancer Detection: Novel Strategies and Clinical Impact, ed. P. Panta, Springer International Publishing, Cham, 2019, pp. 265–295 Search PubMed.
- T. I. Goonewardene, M. R. Hall and G. J. S. Rustin, Lancet Oncol., 2007, 8, 813–821 CrossRef PubMed.
- S. Scarà, P. Bottoni and R. Scatena, Advances in Cancer Biomarkers: from Biochemistry to Clinic for a Critical Revision, ed. R. Scatena, Springer Netherlands, Dordrecht, 2015, pp. 247–260 Search PubMed.
- R. M. Nagler, Oral Oncol., 2009, 45, 1006–1010 CrossRef CAS PubMed.
- N. Shahbazi, S. Hosseinkhani and B. Ranjbar, Sens. Actuators, B, 2017, 253, 794–803 CrossRef CAS.
- Y. Li, S. Hu, C. Chen, N. Alifu, X. Zhang, J. Du, C. Li, L. Xu, L. Wang and B. Dong, Talanta, 2023, 258, 124435 CrossRef CAS PubMed.
- L. Yen, C. Kao and S. Wang, Clin. Otolaryngol. Allied Sci., 1998, 23, 82–86 CrossRef PubMed.
- H. Zhu, Diagnostics, 2022, 12, 1065 CrossRef CAS PubMed.
- M. Yang, J. Ding, Q. Luo, X. Chen and F. Chen, Clin. Chim. Acta, 2022, 525, 40–45 CrossRef CAS PubMed.
- V. Tumuluri, G. A. Thomas and I. S. Fraser, J. Oral Pathol. Med., 2002, 31, 598–604 CrossRef CAS PubMed.
- R. Patruno, N. Zizzo, A. F. Zito, V. Catalano, P. Valerio, V. Pellecchia, E. D'errico, F. Mazzone, P. D. Ribatti and G. Ranieri, Leuk. Lymphoma, 2006, 47, 1138–1143 CrossRef CAS PubMed.
- M. A. Gonzalez-Moles, I. Ruiz-Avila, J. A. Gil-Montoya, F. Esteban and M. Bravo, Oral Oncol., 2010, 46, 525–530 CrossRef CAS PubMed.
- C. J. Barnes, K. Ohshiro, S. K. Rayala, A. K. El Naggar and R. Kumar, Clin. Cancer Res., 2007, 13, 4291–4299 CrossRef CAS PubMed.
- F. Hofmann and C. Garcia-Echeverria, Drug Discov. Today, 2005, 10, 1041–1047 CrossRef CAS PubMed.
- A. Knuppel, G. K. Fensom, E. L. Watts, M. J. Gunter, N. Murphy, K. Papier, A. Perez-Cornago, J. A. Schmidt, K. Smith Byrne, R. C. Travis and T. J. Key, Cancer Res., 2020, 80, 4014–4021 CrossRef CAS PubMed.
- B. P. Patel, P. M. Shah, U. M. Rawal, A. A. Desai, S. V Shah, R. M. Rawal and P. S. Patel, J. Surg. Oncol., 2005, 90, 81–88 CrossRef CAS PubMed.
- R. D. Singh, N. Haridas, J. B. Patel, F. D. Shah, S. N. Shukla, P. M. Shah and P. S. Patel, Indian J. Clin. Biochem., 2010, 25, 250–259 CrossRef CAS PubMed.
- T. Shpitzer, Y. Hamzany, G. Bahar, R. Feinmesser, D. Savulescu, I. Borovoi, M. Gavish and R. M. Nagler, Br. J. Cancer, 2009, 101, 1194–1198 CrossRef CAS PubMed.
- J. Kaur, R. Jacobs, Y. Huang, N. Salvo and C. Politis, Clin. Oral Invest., 2018, 22, 633–640 CrossRef CAS PubMed.
- Z. Khurshid, M. S. Zafar, R. S. Khan, S. Najeeb, P. D. Slowey and I. U. Rehman, Chapter Two - Role of Salivary Biomarkers in Oral Cancer Detection, Advances in Clinical Chemistry, ed. G. S. Makowski, Elsevier, 2018, vol. 86, pp. 23–70 Search PubMed.
- Y. S. L. Cheng, T. Rees and J. Wright, Clin. Transl. Med., 2014, 3, 3 CrossRef PubMed.
- P. Kumar, S. Gupta and B. C. Das, Transl. Oncol., 2024, 40, 101827 CrossRef CAS PubMed.
- O. Barros, V. G. D'Agostino, L. Lara Santos, R. Vitorino and R. Ferreira, Expert Rev. Proteomics, 2024, 21, 149–168 CrossRef CAS PubMed.
- J. Wang, J. Jing, C. Zhou and Y. Fan, Int. J. Oral Sci., 2024, 16, 4 CrossRef CAS PubMed.
- A. Radaic, P. Kamarajan, A. Cho, S. Wang, G. C. Hung, F. Najarzadegan, D. T. Wong, H. Ton-That, C. Y. Wang and Y. L. Kapila, Periodontology, 2000, 2023, 01–31 Search PubMed.
- V. R. Umapathy, P. M. Natarajan and B. Swamikannu, Molecules, 2023, 28, 5283 CrossRef CAS PubMed.
- P. Dongiovanni, M. Meroni, S. Casati, R. Goldoni, D. V. Thomaz, N. S. Kehr, D. Galimberti, M. Del Fabbro and G. M. Tartaglia, Int. J. Oral Sci., 2023, 15, 27 CrossRef CAS PubMed.
- Y. Zhou and Z. Liu, Clin. Chim. Acta, 2023, 548, 117503 CrossRef CAS PubMed.
- R. Goldoni, A. Scolaro, E. Boccalari, C. Dolci, A. Scarano, F. Inchingolo, P. Ravazzani, P. Muti and G. Tartaglia, Biosensors, 2021, 11, 396 CrossRef CAS PubMed.
- J. Adeoye, P. A. Brennan and P. Thomson, J. Oral Pathol. Med., 2020, 49, 711–719 CrossRef PubMed.
- R. Nagler, G. Bahar, T. Shpitzer and R. Feinmesser, Clin. Cancer Res., 2006, 12, 3979–3984 CrossRef CAS PubMed.
- T. Shpitzer, G. Bahar, R. Feinmesser and R. M. Nagler, J. Cancer Res. Clin. Oncol., 2007, 133, 613–617 CrossRef CAS PubMed.
- G. Bahar, R. Feinmesser, T. Shpitzer, A. Popovtzer and R. M. Nagler, Cancer, 2007, 109, 54–59 CrossRef CAS PubMed.
- J. Balakittnen, C. Ekanayake Weeramange, D. F. Wallace, P. H. G. Duijf, A. S. Cristino, G. Hartel, R. A. Barrero, T. Taheri, L. Kenny, S. Vasani, M. Batstone, O. Breik and C. Punyadeera, Int. J. Oral Sci., 2024, 16, 14 CrossRef CAS PubMed.
- H. A. A. Alafaria and A. S. Jalal, J. Appl. Genet., 2024, 1–8 Search PubMed.
- L. J. Chu, Y. T. Chang, C. Y. Chien, H. C. Chung, S. F. Wu, C. J. Chen, Y. C. Liu, W. C. Liao, C. H. Chen, W. F. Chiang, K. P. Chang, J. S. Wang and J. S. Yu, Biomed. J., 2024, 47, 100594 CrossRef CAS PubMed.
- S. K. Ghosh, Y. Man, A. Fraiwan, C. Waters, C. McKenzie, C. Lu, D. Pfau, H. Kawsar, N. Bhaskaran, P. Pandiyan, G. Jin, F. Briggs, C. C. Zender, R. Rezaee, F. Panagakos, J. E. Thuener, J. Wasman, A. Tang, H. Qari, T. Wise-Draper, T. S. McCormick, A. Madabhushi, U. A. Gurkan and A. Weinberg, Cell Rep. Med., 2024, 5, 101447 CrossRef CAS PubMed.
- J. Zheng, K. Chen, L. Cai, Y. Pan and Y. Zeng, J. Cancer, 2024, 15, 1593 CrossRef CAS PubMed.
- A. Jain, A. A. Khan, R. Kaur, R. K. Verma, J. Bakshi, A. Chatterjee, A. Bal, S. Ghoshal and A. Pal, Oral Oncol. Rep., 2024, 10, 100478 CrossRef.
- R. Shalaby, S. Ibrahim, A. A. W. Kotb, S. Baz, L. Hafed, O. Shaker and S. Afifi, Oral Dis., 2024, 30, 2075–2083 CrossRef PubMed.
- A. Patel, S. Patel, P. Patel, D. Mandlik, K. Patel and V. Tanavde, Int. J. Mol. Sci., 2022, 23, 10639 CrossRef CAS PubMed.
- C. I. Faur, R. C. Roman, A. Jurj, L. Raduly, O. Almaşan, H. Rotaru, M. Chirila, M. A. Moldovan, M. Hedesiu and C. Dinu, Medicina, 2022, 58, 1478 CrossRef PubMed.
- L. He, F. Ping, Z. Fan, C. Zhang, M. Deng, B. Cheng and J. Xia, Biomed. Pharmacother., 2020, 121, 109553 CrossRef CAS PubMed.
- M. Honarmand, R. Saravani, L. Farhad-Mollashahi and A. Smailpoor, Int. J. Cancer Manag., 2021, 14, e108344 Search PubMed.
- Y. T. Chang, L. J. Chu, Y. C. Liu, C. J. Chen, S. F. Wu, C. H. Chen, I. Y. F. Chang, J. S. Wang, T. Y. Wu, S. Dash, W. F. Chiang, S. F. Chiu, S. B. Gou, C. Y. Chien, K. P. Chang and J. S. Yu, Cancers, 2020, 12, 2273 CrossRef CAS PubMed.
- P. C. B. Faria, A. P. Carneiro, R. Binato, R. Nascimento, P. S. Santos, D. Fagundes, S. J. da Silva, A. M. Loyola, E. Abdelhay and L. R. Goulart, Sci. Rep., 2019, 9, 18399 CrossRef CAS PubMed.
- S. Langevin, D. Kuhnell, T. Parry, J. Biesiada, S. Huang, T. Wise-Draper, K. Casper, X. Zhang, M. Medvedovic and S. Kasper, Oncotarget, 2017, 8, 82459 CrossRef PubMed.
- R. Metgud and S. Bajaj, Biotech. Histochem., 2016, 91, 96–101 CrossRef CAS PubMed.
- J. Wei, G. Xie, Z. Zhou, P. Shi, Y. Qiu, X. Zheng, T. Chen, M. Su, A. Zhao and W. Jia, Int. J. Cancer, 2011, 129, 2207–2217 CrossRef CAS PubMed.
- D. J. Patil and C. B. More, J. Oral Maxillofac. Surg. Med. Pathol., 2021, 33, 546–554 CrossRef.
- E. Hyvarinen, B. Kashyap and A. M. Kullaa, Metabolites, 2023, 13, 498 CrossRef CAS PubMed.
- S. Ishikawa, M. Sugimoto, K. Kitabatake, A. Sugano, M. Nakamura, M. Kaneko, S. Ota, K. Hiwatari, A. Enomoto, T. Soga, M. Tomita and M. Iino, Sci. Rep., 2016, 6, 31520 CrossRef CAS PubMed.
- M. Yuvaraj, K. Udayakumar, V. Jayanth, A. Prakasa Rao, G. Bharanidharan, D. Koteeswaran, B. D. Munusamy, C. Murali krishna and S. Ganesan, J. Photochem. Photobiol., B, 2014, 130, 153–160 CrossRef CAS PubMed.
- J. Vimal, N. A. George, R. R. Kumar, J. Kattoor and S. Kannan, Arch. Oral Biol., 2023, 155, 105780 CrossRef CAS PubMed.
- K. Tzimas and E. Pappa, Metabolites, 2023, 13, 379 CrossRef CAS PubMed.
- C. Liao, X. Chen and Y. Fu, Interdiscip. Med., 2023, 1, e20230009 CrossRef.
- N. Chuchueva, F. Carta, H. N. Nguyen, J. Luevano, I. A. Lewis, I. Rios-Castillo, V. Fanos, E. King, V. Swistushkin, I. Reshetov, Y. Rusetsky, K. Shestakova, N. Moskaleva, C. Mariani, A. Castillo-Carniglia, D. Grapov, J. Fahrmann, M. R. La Frano, R. Puxeddu, S. A. Appolonova and A. Brito, Metabolomics, 2023, 19, 77 CrossRef CAS PubMed.
- S. Alapati, G. Fortuna, G. Ramage and C. Delaney, Metabolites, 2023, 13, 890 CrossRef CAS PubMed.
- G. Luo, S. Wang, W. Lu, W. Ju, J. Li, X. Tan, H. Zhao, W. Han and X. Yang, Oral Dis., 2024, 1–13 CAS.
- S. Ishikawa, M. Sugimoto, K. Kitabatake, M. Tu, A. Sugano, I. Yamamori, A. Iba, K. Yusa, M. Kaneko, S. Ota, K. Hiwatari, A. Enomoto, T. Masaru and M. Iino, Amino Acids, 2017, 49, 761–770 CrossRef CAS PubMed.
- S. Wang, M. Yang, R. Li and J. Bai, Eur. J. Med. Res., 2023, 28, 53 CrossRef PubMed.
- K. Honigova, J. Navratil, B. Peltanova, H. H. Polanska, M. Raudenska and M. Masarik, Biochim. Biophys. Acta Rev. Canc., 2022, 1877, 188705 CrossRef CAS PubMed.
- S. Jagadeeshan, O. Z. Novoplansky, O. Cohen, I. Kurth, J. Hess, A. J. Rosenberg, J. R. Grandis and M. Elkabets, Biochim. Biophys. Acta Rev. Canc., 2023, 1878, 188963 CrossRef CAS PubMed.
- K. Omura, Int. J. Clin. Oncol., 2014, 19, 423–430 CrossRef CAS PubMed.
- S. H. Huang, E. Hahn, S. I. Chiosea, Z. Y. Xu, J. S. Li, L. Shen and B. O'Sullivan, Oral Oncol., 2020, 102, 104563 CrossRef CAS PubMed.
- Y. Xiao, L. Mao, Q. C. Yang, S. Wang, Z. Z. Wu, S. C. Wan, M. J. Zhang and Z. J. Sun, Oral Oncol., 2023, 138, 106331 CrossRef CAS PubMed.
- M. Boccellino, A. De Rosa and M. Di Domenico, Diagnostics, 2023, 13, 2001 CrossRef CAS PubMed.
- J. Saini, J. Bakshi, N. K. Panda, M. Sharma, A. K. Yadav, K. Kamboj and A. K. Goyal, J. Maxillofac. Oral Surg., 2023, 1–10 Search PubMed.
- D. Chakraborty, T. S. Viveka, K. Arvind, V. Shyamsundar, M. Kanchan, S. A. Alex, N. Chandrasekaran, R. Vijayalakshmi and A. Mukherjee, Clin. Chim. Acta, 2018, 477, 166–172 CrossRef CAS PubMed.
- M. E. Arellano-Garcia, S. Hu, J. Wang, B. Henson, H. Zhou, D. Chia and D. T. Wong, Oral Dis., 2008, 14, 705–712 CrossRef CAS PubMed.
- M. S. Tabatabaei, R. Islam and M. Ahmed, Anal. Chim. Acta, 2021, 1143, 250–266 CrossRef CAS PubMed.
- Y. T. Lin, S. Darvishi, A. Preet, T. Y. Huang, S. H. Lin, H. H. Girault, L. Wang and T. E. Lin, Chemosensors, 2020, 8, 54 CrossRef CAS.
- H. Andersson and A. van den Berg, Sens. Actuators, B, 2003, 92, 315–325 CrossRef CAS.
- B. K. Nahak, A. Mishra, S. Preetam and A. Tiwari, ACS Appl. Bio Mater., 2022, 5, 3576–3607 CrossRef CAS PubMed.
- J. Shoji, R. P. Davis, C. L. Mummery and S. Krauss, Adv. Healthcare Mater., 2023, 2301067 Search PubMed.
- A. G. Monteduro, S. Rizzato, G. Caragnano, A. Trapani, G. Giannelli and G. Maruccio, Biosens. Bioelectron., 2023, 231, 115271 CrossRef CAS PubMed.
- H. Kutluk, E. E. Bastounis and I. Constantinou, Adv. Healthcare Mater., 2023, 12, 2203256 CrossRef CAS PubMed.
- L. Sun, F. Bian, D. Xu, Y. Luo, Y. Wang and Y. Zhao, Mater. Horiz., 2023, 10, 4724–4745 RSC.
- M. Keuper-Navis, M. Walles, B. Poller, A. Myszczyszyn, T. K. van der Made, J. Donkers, H. Eslami Amirabadi, M. J. Wilmer, S. Aan, B. Spee, R. Masereeuw and E. van de Steeg, Pharmacol. Res., 2023, 195, 106853 CrossRef CAS PubMed.
- S. Rahmani Dabbagh, M. Rezapour Sarabi, M. T. Birtek, N. Mustafaoglu, Y. S. Zhang and S. Tasoglu, Aggregate, 2023, 4, e197 CrossRef CAS.
- A. R. Ahmed, O. C. Gauntlett and G. Camci-Unal, ACS Omega, 2021, 6, 46–54 CrossRef CAS PubMed.
- T. Mahmoudi, M. de la Guardia and B. Baradaran, Trends Anal. Chem., 2020, 125, 115842 CrossRef CAS.
- J. A. Otoo and T. S. Schlappi, Biosensors, 2022, 12, 124 CrossRef CAS PubMed.
- K. J. Land, D. I. Boeras, X.-S. Chen, A. R. Ramsay and R. W. Peeling, Nat. Microbiol., 2019, 4, 46–54 CrossRef CAS PubMed.
- A. A. Shalaby, C. W. Tsao, A. Ishida, M. Maeki and M. Tokeshi, Sens. Actuators, B, 2023, 379, 133243 CrossRef CAS.
- M. K. Dey, M. Iftesum, R. Devireddy and M. R. Gartia, Anal. Methods, 2023, 15, 4351–4376 RSC.
- E. Gumus, H. Bingol and E. Zor, J. Pharm. Biomed. Anal., 2023, 225, 115206 CrossRef CAS PubMed.
- R. Gupta, P. Gupta, S. Wang, A. Melnykov, Q. Jiang, A. Seth, Z. Wang, J. J. Morrissey, I. George, S. Gandra, P. Sinha, G. A. Storch, B. A. Parikh, G. M. Genin and S. Singamaneni, Nat. Biomed. Eng., 2023, 7, 1556–1570 CrossRef CAS PubMed.
- W. He, M. Wang, P. Cheng, Y. Liu and M. You, Trends Anal. Chem., 2024, 176, 117735 CrossRef CAS.
- T. Mahmoudi, M. de la Guardia and B. Baradaran, Trends Anal. Chem., 2020, 125, 115842 CrossRef CAS.
- E. B. Bahadır and M. K. Sezginturk, Trends Anal. Chem., 2016, 82, 286–306 CrossRef.
- K. Khachornsakkul, W. Dungchai and N. Pamme, ACS Sens., 2022, 7, 2410–2419 CrossRef CAS PubMed.
- Z. Li, Q. Liu, Y. Li, W. Yuan and F. Y. Li, J. Rare Earths, 2021, 39, 11–18 CrossRef CAS.
- W. Li, W. Peng, Y. Zhang, P. Liu, X. Gong, H. Liu and J. Chang, Anal. Chim. Acta, 2022, 1221, 340112 CrossRef CAS PubMed.
- S. Wen, J. Zhou, K. Zheng, A. Bednarkiewicz, X. Liu and D. Jin, Nat. Commun., 2018, 9, 2415 CrossRef PubMed.
- W. He, M. You, Z. Li, L. Cao, F. Xu, F. Li and A. Li, Sens. Actuators, B, 2021, 334, 129673 CrossRef CAS.
- Y. Wang, Q. Chen, Y. Wang, F. Tu, X. Chen, J. Li and Z. Liu, Talanta, 2023, 256, 124317 CrossRef CAS PubMed.
- O. Mukama, W. Wu, J. Wu, X. Lu, Y. Liu, Y. Liu, J. Liu and L. Zeng, Talanta, 2020, 210, 120624 CrossRef CAS PubMed.
- V. Ranganathan, S. Srinivasan, A. Singh and M. C. DeRosa, Anal. Biochem., 2020, 588, 113471 CrossRef CAS PubMed.
- Z. Li, H. Chen and P. Wang, Analyst, 2019, 144, 3314–3322 RSC.
- Z. Yang, C. Yi, S. Lv, Y. Sheng, W. Wen, X. Zhang and S. Wang, Sens. Actuators, B, 2019, 285, 326–332 CrossRef CAS.
- C. Gui, K. Wang, C. Li, X. Dai and D. Cui, Nanoscale Res. Lett., 2014, 9, 57 CrossRef PubMed.
- O. Mukama, T. Yuan, Z. He, Z. Li, J. de Dieu Habimana, M. Hussain, W. Li, Z. Yi, Q. Liang and L. Zeng, Sens. Actuators, B, 2020, 316, 128119 CrossRef CAS.
- W. Z. Lin, I. C. Ma, J. P. Wang, P. C. Hsieh, C. C. Liu and S. Y. Hou, Anal. Bioanal. Chem., 2021, 413, 2235–2246 CrossRef CAS PubMed.
- Y. Hang, A. Wang and N. Wu, Chem. Soc. Rev., 2024, 53, 2932–2971 RSC.
- F. Beck, M. Loessl and A. J. Baeumner, Microchim. Acta, 2023, 190, 91 CrossRef CAS PubMed.
- N. C. Dalibera, A. F. Oliveira and A. R. Azzoni, Microfluid. Nanofluidics, 2023, 27, 56 CrossRef CAS.
- F. Hou, S. Sun, S. W. Abdullah, Y. Tang, X. Li and H. Guo, Anal. Methods, 2023, 15, 2154–2180 RSC.
- P. Nath and A. Ray, Curr. Opin. Biomed. Eng., 2023, 28, 100504 CrossRef CAS.
- R. Shandilya, A. Bhargava, N. Bunkar, R. Tiwari, I. Y. Goryacheva and P. K. Mishra, Biosens. Bioelectron., 2019, 130, 147–165 CrossRef CAS PubMed.
- A. M. Jimenez Jimenez, M. A. M. Rodrigo, V. Milosavljevic, S. Krizkova, P. Kopel, Z. Heger and V. Adam, Sens. Actuators, B, 2017, 240, 503–510 CrossRef CAS.
- C. S. Kim, D. Ingato, P. Wilder-Smith, Z. Chen and Y. J. Kwon, Nano Convergence, 2018, 5, 3 CrossRef PubMed.
- Y. Liu, S. H. Wu, X. Y. Du and J. J. Sun, Sens. Actuators, B, 2021, 338, 129854 CrossRef CAS.
- T. Pinheiro, A. C. Marques, P. Carvalho, R. Martins and E. Fortunato, ACS Appl. Mater. Interfaces, 2021, 13, 3576–3590 CrossRef CAS PubMed.
- D. Chakraborty, A. Mukherjee and K. R. Ethiraj, Anal. Methods, 2022, 14, 3614–3622 RSC.
- A. Pal, S. K. Dubey and S. Goel, Comput. Electron. Agric., 2022, 195, 106856 CrossRef.
- A. Pal, M. B. Kulkarni, H. Gupta, R. N. Ponnalagu, S. Kumar Dubey and S. Goel, Sens. Actuators, A, 2021, 330, 112896 CrossRef CAS.
- D. Li, Y. Dong, B. Li, Y. Wu, K. Wang and S. Zhang, Analyst, 2015, 140, 7672–7677 RSC.
|
This journal is © The Royal Society of Chemistry 2024 |