DOI:
10.1039/D4AN00325J
(Paper)
Analyst, 2024, Advance Article
Multidimensional mass profiles increase confidence in bacterial identification when using low-resolution mass spectrometers
Received
28th February 2024
, Accepted 1st May 2024
First published on 2nd May 2024
Abstract
Field-forward analytical technologies, such as portable mass spectrometry (MS), enable essential capabilities for real-time monitoring and point-of-care diagnostic applications. Significant and recent investments improving the features of miniaturized mass spectrometers enable various new applications outside of small molecule detection. Most notably, the addition of tandem mass spectrometry scans (MS/MS) allows the instrument to isolate and fragment ions and increase the analytical specificity by measuring unique chemical signatures for ions of interest. Notwithstanding these technological advancements, low-cost, portable systems still struggle to confidently identify clinically significant organisms of interest, such as bacteria, viruses, and proteinaceous toxins, due to the limitations in resolving power. To overcome these limitations, we developed a novel multidimensional mass fingerprinting technique that uses tandem mass spectrometry to increase the chemical specificity for low-resolution mass spectral profiles. We demonstrated the method's capabilities for differentiating four different bacteria, including attentuated strains of Yersinia pestis. This approach allowed for the accurate (>92%) identification of each organism at the strain level using de-resolved matrix-assisted laser desorption ionization-time of flight (MALDI-TOF) data to mimic the performance characteristics of miniaturized mass spectrometers. This work demonstrates that low-resolution mass spectrometers, equipped with tandem MS acquisition modes, can accurately identify clinically relevant bacteria. These findings support the future application of these technologies for field-forward and point-of-care applications where high-performance mass spectrometers would be cost-prohibitive or otherwise impractical.
Introduction
Bacterial infections are one of the highest causes of mortality in the world, representing the second leading cause of death worldwide. In 2019, only 33 different bacterial pathogens caused 13.6% of all global deaths.1 Increasing the speed and accuracy of bacterial identification remains a high priority for clinical researchers.2–4 Timely identification of bacteria (both genus and species) is vital for selecting effective treatment options and patient outcomes.5–7 Time to answer represents one of the biggest challenges facing clinicians when identifying the source of infections. Without rapid and accurate bacterial typing, clinicians routinely prescribe broad-spectrum antibiotics to combat unclassified infections, leading to increased rates of antimicrobial resistance (AMR), hospital readmissions, and higher healthcare costs.8–10
Classical methods for bacterial identification include culture-based methods, e.g., Gram stain and biochemical testing. More recently, chemical genotyping methods, e.g., polymerase chain reaction (PCR) and 16S rRNA, have been used to identify bacteria with higher confidence.11,12 These methods have been relied upon for decades and are simple enough to be widely employed in clinical scenarios; however, classical methods have certain limitations. Next-generation sequencing and 16S sequencing methods have limited sample throughput, at times requiring as much as several days to deliver conclusive answers. Unfortunately, during an active infection, time spent culturing bacteria and acquiring sequencing results can be quite costly from a clinical treatment perspective.13 Genotyping methods, while often faster and more accurate than culture-based methods, are also impaired by the inaccessibility of specialized laboratory personnel and consumable kits. In addition to these methods, mass spectrometry (MS)-based methods have sharply increased in popularity in recent years.14,15 Identifying and differentiating bacteria using mass spectral fingerprints has proven to be a robust, high-throughput method in a clinical setting. The US Food and Drug Administration (FDA) has approved several commercial systems for clinical use, e.g., VITEK® MS and Biotyper®.16,17 When applied to bacterial co-cultures and clinical samples taken from complex sample matrices like urine and blood, these methods have limited specificity. Still, bacterial identification from clinically relevant MS samples has improved accuracy dramatically in recent years.18–20
A standard analytical method for mass spectral fingerprinting of bacteria is matrix-assisted laser desorption ionization (MALDI) coupled to a mass spectrometer, e.g., time-of-flight (TOF).21 Enriched or cultured bacteria are often lysed before analysis but can be analyzed as intact cells.22 Following lysis, biomolecules within the bacteria, such as ribosomal proteins and cell membrane lipids, are ionized by a laser firing at a sample mixed with an energy-absorbing matrix. MALDI-TOF MS is ideal for probing cellular contents for bacterial identification as it utilizes ‘soft’ ionization that incurs minimal fragmentation of the biomolecules and produces minimal charge states.23–25 These instruments can also run hundreds of bacterial extracts simultaneously on the same target plate, facilitating clinically relevant sample throughput. Bacteria growth conditions and MALDI plate preparation can substantially impact the quality of the data collected from MALDI-TOF MS experiments and how well bacteria can be identified and differentiated.26–28 Like chemical genotyping techniques, MALDI-TOF MS is often limited by the number of spectra in databases available for spectral matching.29,30 However, the output from these instruments can be acquired rapidly, and the time to answer is often much shorter (≈minutes) compared to other related techniques.31
One of the significant obstacles to overcome for broader acceptance of MS-based analysis is the need for instrumentation to be as close to the clinic as possible with the most simplified sample preparation for the user. Accurate point-of-care operation of a field-forward MALDI-MS would enable the most rapid results, potentially increase the efficacy of prescribed antibiotics, and drastically reduce the consumables required for clinical bacterial identification and differentiation.17,32 While commercial systems exist, these instruments are still bulky, require significant capital, and rely heavily upon proprietary libraries for identifications. Over the last five years, instrument vendors have made considerable investments to advance miniature, low-cost, portable mass spectrometers equipped for applications in the biological detection space. Many of these investments have been made by the United States Department of Defense and integrated into commercialized products, including the BioFlyte BioTOF™ z200 and BaySpec Continuity™ and Portability™ miniature mass spectrometers. Despite progress, these portable systems still struggle to confidently identify biological threats/pathogens of interest, such as bacteria, viruses, and proteinaceous toxins, primarily due to the lack of adequate mass resolution.
Herein, we describe a new multidimensional mass fingerprinting technique that makes bacterial differentiation possible using low-resolution scans, including MS/MS or fragment scans, for the analysis. These fragment scans allow individual unresolved features in the full scan to be isolated and fragmented. These fragments detected in the MS/MS scans provide additional molecular information previously hidden in the original low-resolution MS1 scan. In traditional MS analyses, these scans typically require a narrow isolation window for the precursor ion (≈1–2 Da) as additional information for a single peak of interest. This application aims not to identify any specific peak or peptide of interest from which to base identifications. Therefore, we utilized wide MS/MS isolation windows (≈100 Da) for fragmentation akin to data-independent acquisition (DIA) commonly used in proteomics. By concatenating MS2 scans collected from broad isolation windows with their corresponding full MS1 scan, a relatively rapid analysis can be performed that includes MS1 and MS/MS information across the entire mass range (Fig. 1). These multidimensional composite spectra facilitate bacterial identification with greater data fidelity than MS1 scans alone. To that end, we have implemented this novel multidimensional scan technique to differentiate several species and strains of bacteria, including both Gram-positive and Gram-negative strains, from monoculture to test the feasibility of this acquisition technique for use in a clinical or field-forward setting.
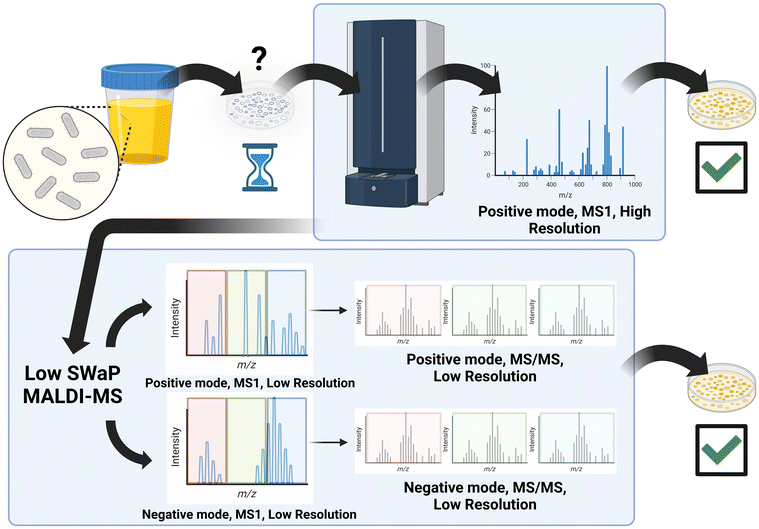 |
| Fig. 1 Workflow for traditional MALDI-TOF bacterial identification (top), and similar workflow for the multidimensional scan technique with MS1 and MS/MS data (bottom). Image created in Biorender. | |
Materials and methods
Bacterial growth and lysis for MALDI-TOF MS analysis
All organisms for this study (Table 1) were grown on the same lot of tryptic soy agar plates with sheep blood (Fisher Cat#221239). Each plate was split into three zones, and generous streaks of each bacterial strain were made on one plate. Plates were incubated at 37 °C overnight under aerobic conditions. An inoculating loop was used to scrap colonies from the plate in two passes, and these colonies were resuspended in 1 mL of ice-cold Optima™ LC/MS-grade methanol (Sigma Aldrich, St Louis, MO, USA) in a 2 mL Eppendorf™ LoBind microcentrifuge tube (Sigma Aldrich, St Louis, MO, USA). Each tube was vortexed for thirty seconds and placed in a freezer at −80 °C overnight to lyse. In addition, each tube was passed through an 18G needle ten times to ensure lysis. These tubes were then spun at 10
000g for 10 min in a cold centrifuge, and the supernatant was removed to a fresh 2 mL tube. A small aliquot was taken for BCA protein quantification and to test for bacterial viability. This protocol was repeated for ten replicates. Clarified lysed samples in methanol (≈400 μL) were used for spotting onto MALDI plates.
Table 1 All organisms and strains utilized for multidimensional MALDI-TOF analysis
Species |
Strain |
Source |
Escherichia coli |
DH5α |
Invitrogen |
PUTI |
Clinical Laboratory, VU |
CFT073 |
BEI Resources NR-2654 |
UTI89 |
Clinical laboratory, VU |
|
Enterococcus faecalis |
CH19 |
BEI Resources NR-31990 |
Portland |
ATCC 29212 |
SF24413 |
BEI Resources NR-31971 |
|
Staphylococcus aureus |
USA300 |
BEI Resources NR-46070 |
NRS39 |
BEI Resources 45883 |
HT 20020396 |
BEI Resources 46054 |
|
Yersinia pestis |
A1122 |
BEI Resources NR-15 |
KIM 10+ |
BEI Resources NR-642 |
K25 |
BEI Resources NR-4703 |
MALDI-TOF-TOF MS analysis
Spotting was performed with 0.5 μL of Optima™ LC/MS-grade water (Sigma Aldrich, St Louis, MO, USA) followed by 1.5 μL of lysed sample and finally 0.5 μL of 4-(dimethylamino)cinnamic acid (DMACA) MALDI matrix onto a stainless-steel target. MALDI-TOF-TOF analysis was performed on an AB Sciex 4800 MALDI TOF/TOF mass spectrometer. The instrument has a 355 nm wavelength Nd:YAG laser operating at a rate of 200 Hz. MS1 and MS/MS data were collected in positive and negative polarity. The instrument was operated in linear mode for all data acquisition. For all MS1 data acquisition, the mass range was set to m/z 200–1200, a focus mass of m/z 350, a laser intensity of 2800 arbitrary units (a.u.), and a total number of shots/spectrum of 400. MS/MS data were acquired in a data-independent acquisition fashion with a lower isolation width of 100 Daltons (Da). The precursor values isolated were m/z 300, 400, 500, 600, 700, 800, 900, 1000, 1100, and 1200. For example, the isolation width for m/z 300 was m/z 200–300. High-energy collision-induced dissociation (HE-CID) MS/MS data for positive and negative polarity were collected at an acceleration energy of 1 keV, a laser intensity of 3800 a.u., and a total number of shots/spectrum of 1000. After data acquisition, all data were exported as .txt files for further processing. All strains were run in three technical replicates with ten biological replicates.
QA/QC and data analysis
For spectra QA/QC, peaks within two standard deviations of mean blank signal in individual spectra were removed for denoising. Mass to charge values were de-resolved to 0.1 Da via rounding to make peaks coherent at reasonable instrument resolution, and peaks observed in matrix background samples were removed from representation in all bacterial samples. The resulting data were binned at m/z by summing the density of binned peaks to simulate low-resolution data, which would be achievable using a miniaturized, low-cost mass spectrometry platform. Additionally, peak features not observed in at least three samples were removed to increase confidence and enhance statistical analysis of features. Given the dimensionality of the data being collated together, each mass feature was independently tagged not to confuse equal masses obtained from different scans, e.g., negative mode, positive mode, MS1, or MS/MS scans. For all subsequent statistical analysis and model development, three composite datasets were examined independently: (1) MS1-MS/MS composite negative mode, (2) MS1-MS/MS composite positive mode, and (3) all MS positive and negative mode composite data.
Data visualization and analysis were performed in R.33 Ordination data was visualized using the plotly version 4.10.1 package in R.34,35 MANOVA-type analyses PERMANOVA and ANOSIM were performed using the vegan package version 2.6.4 in R.36 Non-metric multidimensional scaling (NMDS) was run using the vegan package in R. EnvFit ordination-based models were generated using the vegan package in R. NMDS ordinations for MS1 and MS/MS comparisons as well as composite datasets were performed using the Procrustes and Protest functions in the vegan package in R. Optimal feature binning associated with metadata was performed using the opticut package version 0.1.2 in R.37 Opticut has a 3-tier ranking for the strength of association; only the strongest tier of association (“+++”) was considered in this analysis. Spectral fingerprint figures were generated using the scatterplot3d package in R.38 Heatmap fingerprints were generated using the pheatmap package in R.39 Bacterial strain fingerprint plots were built using the scatterplot3d package in R.38
Results and discussion
Choice of bacterial organisms
For this study, four strains of E. coli, three strains of E. faecalis, three strains of S. aureus, and three strains of Y. pestis were grown from culture and analyzed via MALDI-TOF MS (Table 1). These bacteria represent Gram-positive and Gram-negative strains and one BSL-3 surrogate. Several of these bacteria, such as E. coli and E. faecalis, are some of the most common causes of urinary tract infections (UTI), representing most hospital-acquired infections in the United States and a significant public health burden.40 Infections caused by S. aureus are also a source of UTIs, and this pathogen is also a growing concern for hospital-acquired infections and antibiotic resistance.41 Although Y. pestis infections are rare, this pathogen represents a significant biothreat; humans can become vectors for transmission (pneumonic plague), it is endemic to the Southwest region of the United States, mortality is significantly increased only 24 hours after infection, and there are currently no approved vaccines for treatment.42,43 These bacterial strains can also possess varying antibiotic resistances and represent pathogens very likely to be found in a clinical or bioterrorism scenario in which field-forward detection and identification are critical to patient outcomes.
Sample preparation and choice of MALDI matrix
Sample preparation for MALDI-TOF MS experiments begins with choosing the correct extraction technique for the analyte(s) of choice, e.g., proteins or lipids, within a bacterial sample. To test the initial viability of our multidimensional scan technique for bacterial differentiation, we utilized bacteria grown from culture; this represents similar conditions used to prepare samples for other commercial MALDI-TOF MS systems such as the Biotyper.44 Although it is possible to transfer a direct smear of bacteria from culture onto the MALDI plate, this technique generally does not perform as well as lysing the bacteria beforehand, especially for Gram-positive bacteria.45,46 For most lysing protocols, optimization requires multiple isolation and purification steps, and some of these, such as biphasic extractions, may be too complicated and less reproducible in a point-of-care setting. Therefore, we performed a simple, methanol-based extraction utilizing as few steps as possible to determine the feasibility of a ‘universal’ sample extraction along with the multidimensional scan technique.47 Using a simplified methanol extraction is simpler, cheaper, provides a desalting step, removes proteins, and allows the lysate to contain the most diverse biomolecular constituents, ultimately generating a rich molecular fingerprint that is not solely dependent upon one analyte class. Methanol is also less toxic than many biphasic reagents and represents a ‘greener’ solvent choice. In addition, the matrix chosen for this study, DMACA, is known to perform well in both positive and negative ionization modes; this matrix property is critical to simplifying data collection for both ionization modes from the same sample. DMACA is also vacuum-stable, non-toxic, and relatively inexpensive.48 Some limitations for our sample preparation include the necessity for −80 °C overnight lysis and reliance on some centrifugation that may not be available in many smaller clinics. However, as a proof-of-principle these represent possibilities for future improvements to the current methods.
Generation of the multidimensional mass fingerprint
One of the major limitations of almost all MALDI-MS analyses for bacterial identification and differentiation is the reliance solely on MS1 scans.49 This limitation is especially important for mass spectral fingerprinting applications, as the specificity of chemical information correlates to how accurately bacteria can be identified and distinguished within complex backgrounds such as biofluids. Accurate identification from these data requires sufficient resolution and sensitivity across a broad mass range to identify and differentiate bacteria based on a full scan alone. However, these broadband MS1 scans typically are biased by highly conserved protein sequences for similar strains and only detect approximately 30–50 unique features that can be assigned to proteins per spectrum, limiting the technique to only the differentiation of similar strains.50 Although commercially available, high-resolution MALDI-MS systems currently enable high-accuracy bacterial identifications, these systems still require sample culture as part of their sample preparation, which requires at least four hours for the most rapid culturing.51 Furthermore, adding MS/MS scans increases the number of features available for bacterial identification and differentiation.52 However, performing fragment scans for an entire full scan is normally impractical as MS/MS scans across the entire mass range of an MS1 scan produce highly convoluted data. This dearth of scan information becomes especially important as real-world clinical samples involve significant hurdles for sensitivity and specificity, including increased background signal, the presence of multiple bacteria within a sample, and reliance on a proprietary, well-curated database of spectra for comparisons.
Fig. 1 diagrams the workflow of the newly developed multidimensional mass fingerprinting method for including MS/MS data along with MS1 scan data. First, a sample is interrogated using a full MS1 scan across the entire mass range (200–1200 Da). For each subsequent fragment ion scan, this mass range is split into 100 Da isolation windows (200–300 Da, 300–400 Da, etc.) for a single MS/MS scan in which the entire window undergoes fragmentation. During post-processing, the MS/MS scans are combined into a single dataset along with the original MS1 scan, associating the original isolation window from the MS1 scan with each discrete MS/MS scan. Because of the relative speed at which these eleven scans (full MS1 scan with ten fragment ion scans) are collected, samples can be processed rapidly, and technical replicates can be taken for both MS1 and MS/MS scans in each sample. Determining what data is required to achieve strain-level differentiation is critical, so we have performed proof-of-concept MALDI analyses from monocultures in both positive and negative modes with MS1 and MS/MS scans.
Data post-processing and quality control
During preliminary data visualization in NMDS, two negative mode samples, one from E. coli PUTI and one from E. faecalis SF24413, were observed to be clear outliers and removed from all data sets for the downstream analysis. In total, 372 samples were used for the analysis as these samples coherently possessed all four data types, including positive mode, negative mode, MS1, and MS/MS. A total of 72
318 reliable m/z features remained across 372 samples post-processing.
Strain-level differentiation
Heat map features from the multidimensional MS1 and MS/MS data analysis at both the species (Fig. 2A) and strain (Fig. 2B) levels indicate clear distinctions between the cultured bacteria. The strength of the association of these features is seen from the optimal binning analysis (Fig. 2C and D). The LogLR scale for these features, in which any values ≥2 indicate a strong association, reaches maximum values of approximately 200 and 600 for the most distinctive features of species and strain, respectively. Incorporated within these plots at the species level, 19
282 significant features were found statistically, including 7690 positive mode and 11
599 negative mode features. These consisted of 6288 MS1 features and 13
001 MS/MS features. At the strain level, 11
856 features were found, including 7121 positive mode and 4735 negative mode features. These consisted of 1740 MS1 and 10
116 MS/MS features. There were approximately two to six-fold as many MS/MS features per identification level; the Procrustes analysis from Table 2 indicates that as much as 69% of new relational information may come from MS/MS in positive mode and as much as 35% of new relational information from MS/MS in negative mode. Procrustes analysis considers the similarity of two data sets, with values indicating a percentile of similarity which can indicate how much new information is gained by adding the second data set. Taken together, these data indicate that although the positive mode contains less information than the negative mode for the mass range in the current study, including both scan modes with multidimensional scan data can provide significant enhancements in the encoded chemical information that can be used to build classification models. As the applications of this approach move from well-defined culture conditions to more clinically relevant samples with more complex biofluids, including these data will be crucial to maintaining the statistical power of this approach at lower resolving power.
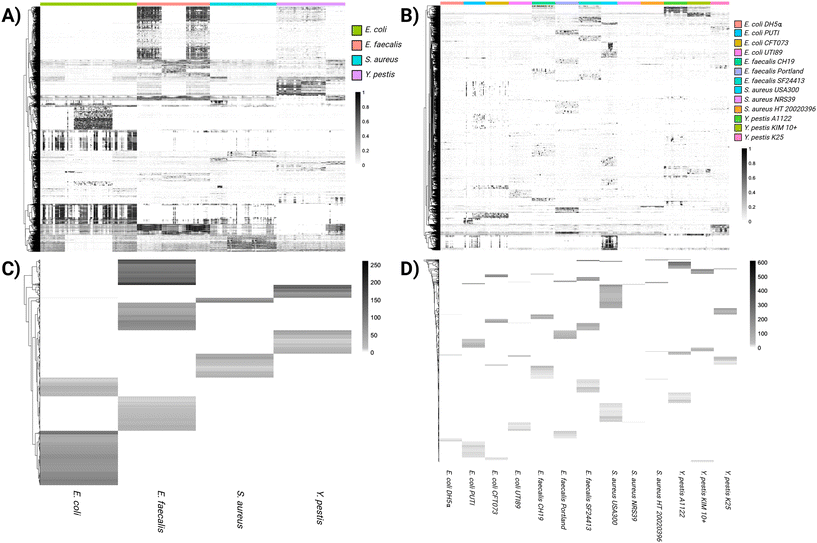 |
| Fig. 2 Heat maps for features unique to species (A) and strain (B), with corresponding LogLR plots for species (C) and strain (D). | |
Table 2 Summary of statistical analysis performed based upon data-source level
Ionization mode(s) |
Test statistic |
ID level |
Statistical result (0.1 Da, (p ≥ 0.001)) |
Positive mode |
ANOSIM |
Species |
R = 0.429 |
Strain |
R = 0.682 |
PERMANOVA |
Species |
F = 35.94 |
Strain |
F = 34.25 |
EnvFit |
Species |
r2 = 0.293 |
Strain |
r2 = 0.699 |
Procrustes |
MS vs. MS/MS |
0.310 |
|
Negative mode |
ANOSIM |
Species |
R = 0.649 |
Strain |
R = 0.749 |
PERMANOVA |
Species |
F = 131.44 |
Strain |
F = 108.71 |
EnvFit |
Species |
r2 = 0.692 |
Strain |
r2 = 0.960 |
Procrustes |
MS vs. MS/MS |
0.652 |
|
Positive and negative modes |
ANOSIM |
Species |
R = 0.720 |
Strain |
R = 0.938 |
PERMANOVA |
Species |
F = 92.87 |
Strain |
F = 82.03 |
EnvFit |
Species |
r2 = 0.750 |
Strain |
r2 = 0.954 |
Procrustes |
MS vs. MS/MS |
0.539 |
MANOVA-type analysis using PERMANOVA and ANOSIM showed significant differences at both the species and strain levels for positive and negative mode data, with test statistics generally improving for the composite data set (Table 2). Discrete EnvFit models were also significant for all data sets and data resolutions, with the models for species and strain on the composite data set having r2 values of 0.75 and 0.95, respectively (Table 2). It is interesting to note that the analysis and modeling both point to a more robust delineation of features at the strain level than at the species level; this is atypical of taxonomic identification results in biological studies, where it is usually easier to identify organisms at higher taxonomic levels. This finding suggests that agnostic features detected in multidimensional scans are highly strain-specific, with hypervariability observed between strains of the same species (Fig. 2). This hypervariability could be of benefit in the future to this multidimensional technique for matrices such as urine that can dominate the MS1 feature space.
To better visualize the differences between the ionization modes, non-metric multidimensional scaling (NMDS) plots were constructed (Fig. 3). All ordinations were run at k = 3 with acceptable stress levels ranging from 0.02 to 0.10. It can be observed visually that negative mode-only (Fig. 3B) provides better separation amongst the strains than for positive-mode only (Fig. 3A), consistent with the findings of Table 2. However, even for negative mode-only data, there is still significant overlap between strains, particularly for the E. coli and S. aureus strains. Once both ionization modes are combined (Fig. 3C), the separation between strains becomes stronger, and pairwise ANOSIM values drastically improve (Table 2).
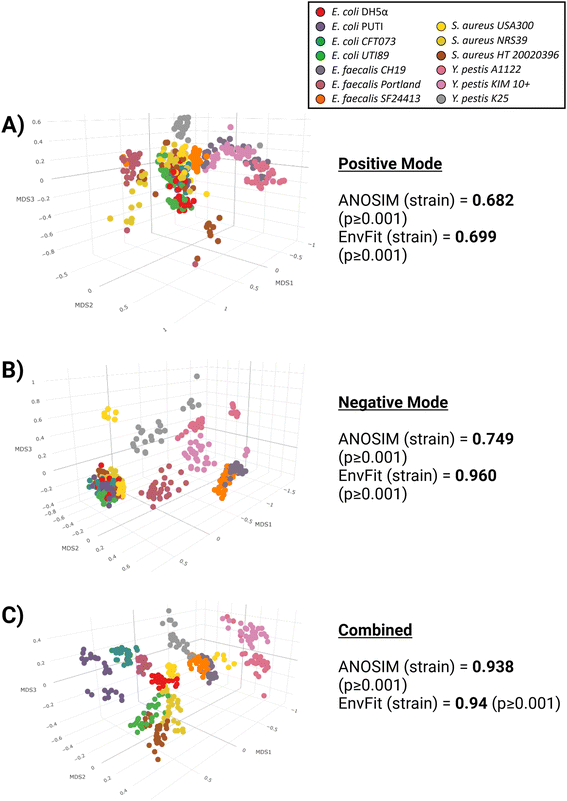 |
| Fig. 3 Non-metric multidimensional scaling (NMDS) plots and ANOSIM/EnvFit values for positive (A) and negative (B) ionization modes, along with both modes combined (C). | |
To visualize the sum of these data three-dimensionally as a multidimensional ‘fingerprint’, the multidimensional scans have been combined with their associated LogLR values (Fig. 4). This graphical scheme depicts the MS1 full scan on the x-axis, as well as the ten MS/MS windows on the z-axis. The strength of association for these features for each strain is indicated by the magnitude of the LogLR values on the y-axis. This graph also displays both positive and negative mode data together as separate colors. Fig. 4 shows how easily these strains can be distinguished from one another by visual inspection of all the included data for three strains of Y. pestis. It should be noted that the features shown are those that are deemed meaningful based on the optimal binning analysis rather than all of the peaks associated with each organism. Therefore, when moving from the MS1 axis to the MS/MS axis, there are some features that appear in multiple strains that are statistically removed based upon being common amongst the strains. This can give the appearance of MS/MS features with no corresponding MS1 counterpart when MS/MS peaks are meaningfully unique to an organism while the related MS1 peak is not. There are far fewer positive mode features compared with negative mode for the entire dataset; however, based on the LogLR values from Fig. 4, the unique positive peaks associate quite strongly with their designated strain. This also explains the Procrustes values from Table 2 in which the correlation between MS1, MS/MS, positive, and negative mode data sets range between 0.2576 and 0.6245; each data set contributes a meaningful portion of new information to the final composite data set for bacterial differentiation. Negative mode data contributes the most information to the final composite dataset, with 87% of the relational information in the final dataset able to be captured in the negative information alone (protest correlation = 0.873), while positive mode data captures just 55% of the relational information in the final dataset (protest correlation = 0.558). The negative MS/MS dataset is the most important individual contributor, with a protest correlation of 0.808.
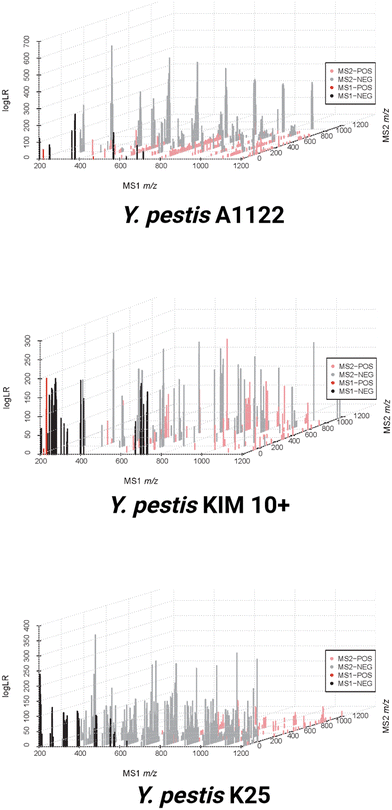 |
| Fig. 4 Multidimensional composite spectra for three strain of Y. pestis including positive MS/MS (light red, z-axis), negative MS/MS (light grey, z-axis), positive MS1 (dark red, x-axis), and negative MS1 (dark grey, x-axis) datasets. | |
As this type of work naturally progresses from monocultures to co-cultures and then environmental and clinical biofluid samples, environmental confounding effects and increased background contributions will become more pronounced. It is anticipated that the contributions of each individual dataset (positive, negative, MS1, and MS/MS) will become increasingly important for bacterial identification and classification at these stages of development. To ensure the multidimensional scan technique shown here will be a viable technique for bacterial differentiation in a point-of-care or field-forward setting, considerations must be made for technical challenges with portable MALDI instrumentation. Some commercially-available portable MALDI instruments fulfill SWaP requirements (e.g. Shimadzu MALDImini-1, MassTech MTE30) for field-forward detection but cannot provide the full functionality required for multidimensional scans (mass range >1000 Da, MS/MS, positive and negative mode ionization, large isolation windows, etc.). Modifications to both software and hardware in portable systems will be necessary to ensure reliable bacterial identification and differentiation, and these modifications will require a thorough cost–benefit analysis for manufacturers. Additionally, significant method development will also be necessary to ensure these multidimensional MALDI analyses can work in field-forward applications with different mass analyzers (e.g. time-of-flight vs. ion trap) and with reduced sample burden (simplified lysis and extraction, pre-prepared MALDI plates, etc.) Incorporating these features into a single instrument could provide the field of portable mass spectrometry with a significant leap forward for clinical and field-forward applications.
Conclusions
By utilizing a novel multidimensional scan technique, full scan MS1 data can be combined with MS/MS data for the differentiation of monocultures of several Gram-positive and Gram-negative strains of bacteria, including a BSL-3 surrogate organism. These data have been de-resolved to mimic the low resolution currently attainable on portable mass spectrometers. By utilizing this multidimensional scan technique with low-resolution data and simplified sample preparation methods, this technique could be further developed in order to be applied in a point-of-care or field-forward setting to rapidly identify bacteria of interest from complicated samples (blood, urine, peripheral fluids, etc.) utilizing a low-cost and small size, weight, and power (SWaP) instrument. Future work in this area will involve adapting this acquisition technique to portable, low-resolution instrumentation purpose-built for this application, as well as the inclusion of more clinically relevant sample types (co-cultures, cultures from biofluids, clinical samples, etc.). Our data shows greater than 92% accuracy and predictive power at the strain level for the four bacteria used in this study. These results are comparable to currently FDA-approved MALDI-MS platforms but can be achieved with low-resolution data common to portable, low SWaP instrumentation. Taken together, these advances will allow MALDI-MS to move beyond time-intensive, culture-based sample preparation towards direct biofluid or environmental sample analysis with the goal of significantly reducing time-to-answer and cost of consumables and bringing analysis closer to point-of-care and field-forward areas.
Author contributions
ZJS, formal analysis, investigation, writing – original draft; ESL, data curation, formal analysis, validation, visualization, writing – original draft; NV, investigation, methodology, resources; ARA, investigation, writing – original draft; NHP, conceptualization, project administration; MD, methodology, resources, validation, writing – review & editing; MF, methodology, resources; JLN, conceptualization, funding acquisition, project administration, writing – review & editing; RC, conceptualization, funding acquisition, project administration, supervision, writing – review & editing; PMM, conceptualization, writing – review & editing; EMM, project administration, resources, supervision, writing – original draft, writing – review & editing; TGG, conceptualization, funding acquisition, methodology, project Administration, resources, supervision, writing – original draft, writing – review & editing.
Conflicts of interest
The authors declare that they have no competing financial interests.
Acknowledgements
The U.S. Department of Energy supported this work through the Los Alamos National Laboratory. Los Alamos National Laboratory is operated by Triad National Security, LLC, for the National Nuclear Security Administration of the U.S. Department of Energy (Contract No. 89233218CNA000001). The Los Alamos National Laboratory Directed R&D Fund, LDRD, grant number 20220088ER, funded this work. The authors would also like to acknowledge collaborators at the University of Wisconsin for help with the matrix blank data acquisition. The funders had no role in the study design, data collection, analyses, data interpretation, manuscript preparation, or the decision to publish.
References
- K. S. Ikuta, L. R. Swetschinski, G. Robles Aguilar, F. Sharara, T. Mestrovic, A. P. Gray, N. Davis Weaver, E. E. Wool, C. Han, A. Gershberg Hayoon, A. Aali, S. M. Abate, M. Abbasi-Kangevari, Z. Abbasi-Kangevari, S. Abd-Elsalam, G. Abebe, A. Abedi, A. P. Abhari, H. Abidi, R. G. Aboagye, A. Absalan, H. Abubaker Ali, J. M. Acuna, T. D. Adane, I. Y. Addo, O. A. Adegboye, M. Adnan, Q. E. S. Adnani, M. S. Afzal, S. Afzal, Z. B. Aghdam, B. O. Ahinkorah, A. Ahmad, A. R. Ahmad, R. Ahmad, S. Ahmad, S. Ahmad, S. Ahmadi, A. Ahmed, H. Ahmed, J. Q. Ahmed, T. Ahmed Rashid, M. Ajami, B. Aji, M. Akbarzadeh-Khiavi, C. J. Akunna, H. Al Hamad, F. Alahdab, Z. Al-Aly, M. A. Aldeyab, A. V. Aleman, F. A. N. Alhalaiqa, R. K. Alhassan, B. A. Ali, L. Ali, S. S. Ali, Y. Alimohamadi, V. Alipour, A. Alizadeh, S. M. Aljunid, K. Allel, S. Almustanyir, E. K. Ameyaw, A. M. L. Amit, N. Anandavelane, R. Ancuceanu, C. L. Andrei, T. Andrei, D. Anggraini, A. Ansar, A. E. Anyasodor, J. Arabloo, A. Y. Aravkin, D. Areda, T. Aripov, A. A. Artamonov, J. Arulappan, R. T. Aruleba, M. Asaduzzaman, T. Ashraf, S. S. Athari, D. Atlaw, S. Attia, M. Ausloos, T. Awoke, B. P. Ayala Quintanilla, T. M. Ayana, S. Azadnajafabad, A. Azari Jafari, D. B. B. M. Badar, A. D. Badiye, N. Baghcheghi, S. Bagherieh, A. A. Baig, I. Banerjee, A. Barac, M. Bardhan, F. Barone-Adesi, H. J. Barqawi, A. Barrow, P. Baskaran, S. Basu, A.-M. M. Batiha, N. Bedi, M. A. Belete, U. I. Belgaumi, R. G. Bender, B. Bhandari, D. Bhandari, P. Bhardwaj, S. Bhaskar, K. Bhattacharyya, S. Bhattarai, S. Bitaraf, D. Buonsenso, Z. A. Butt, F. L. Caetano dos Santos, J. Cai, D. Calina, P. Camargos, L. A. Cámera, R. Cárdenas, M. Cevik, J. Chadwick, J. Charan, A. Chaurasia, P. R. Ching, S. G. Choudhari, E. K. Chowdhury, F. R. Chowdhury, D.-T. Chu, I. S. Chukwu, O. Dadras, F. T. Dagnaw, X. Dai, S. Das, A. Dastiridou, S. A. Debela, F. W. Demisse, S. Demissie, D. Dereje, M. Derese, H. D. Desai, F. N. Dessalegn, S. A. A. Dessalegni, B. Desye, K. Dhaduk, M. Dhimal, S. Dhingra, N. Diao, D. Diaz, S. Djalalinia, M. Dodangeh, D. Dongarwar, B. T. Dora, F. Dorostkar, H. L. Dsouza, E. Dubljanin, S. J. Dunachie, O. C. Durojaiye, H. A. Edinur, H. B. Ejigu, M. Ekholuenetale, T. C. Ekundayo, H. El-Abid, M. Elhadi, M. A. Elmonem, A. Emami, L. Engelbert Bain, D. B. Enyew, R. Erkhembayar, B. Eshrati, F. Etaee, A. F. Fagbamigbe, S. Falahi, A. Fallahzadeh, E. J. A. Faraon, A. Fatehizadeh, G. Fekadu, J. C. Fernandes, A. Ferrari, G. Fetensa, I. Filip, F. Fischer, M. Foroutan, P. A. Gaal, M. A. Gadanya, A. M. Gaidhane, B. Ganesan, M. Gebrehiwot, R. Ghanbari, M. Ghasemi Nour, A. Ghashghaee, A. Gholamrezanezhad, A. Gholizadeh, M. Golechha, P. Goleij, D. Golinelli, A. Goodridge, D. A. Gunawardane, Y. Guo, R. D. Gupta, S. Gupta, V. B. Gupta, V. K. Gupta, A. Guta, P. Habibzadeh, A. Haddadi Avval, R. Halwani, A. Hanif, M. A. Hannan, H. Harapan, S. Hassan, H. Hassankhani, K. Hayat, B. Heibati, G. Heidari, M. Heidari, R. Heidari-Soureshjani, C. Herteliu, D. Z. Heyi, K. Hezam, P. Hoogar, N. Horita, M. M. Hossain, M. Hosseinzadeh, M. Hostiuc, S. Hostiuc, S. Hoveidamanesh, J. Huang, S. Hussain, N. R. Hussein, S. E. Ibitoye, O. S. Ilesanmi, I. M. Ilic, M. D. Ilic, M. T. Imam, M. Immurana, L. R. Inbaraj, A. Iradukunda, N. E. Ismail, C. C. D. Iwu, C. J. Iwu, L. M. J. M. Jakovljevic, E. Jamshidi, T. Javaheri, F. Javanmardi, J. Javidnia, S. K. Jayapal, U. Jayarajah, R. Jebai, R. P. Jha, T. Joo, N. Joseph, F. Joukar, J. J. Jozwiak, S. E. O. Kacimi, V. Kadashetti, L. R. Kalankesh, R. Kalhor, V. K. Kamal, H. Kandel, N. Kapoor, S. Karkhah, B. G. Kassa, N. J. Kassebaum, P. D. M. C. Katoto, M. Keykhaei, H. Khajuria, A. Khan, I. A. Khan, M. Khan, M. N. Khan, M. A. B. Khan, M. M. Khatatbeh, M. M. Khater, H. R. Khayat Kashani, J. Khubchandani, H. Kim, M. S. Kim, R. W. Kimokoti, N. Kissoon, S. Kochhar, F. Kompani, S. Kosen, P. A. Koul, S. L. Koulmane Laxminarayana, F. Krapp Lopez, K. Krishan, V. Krishnamoorthy, V. Kulkarni, N. Kumar, O. P. Kurmi, A. Kuttikkattu, H. H. Kyu, D. K. Lal, J. Lám, I. Landires, S. Lasrado, S.-w. Lee, J. Lenzi, S. Lewycka, S. Li, S. S. Lim, W. Liu, R. Lodha, M. J. Loftus, A. Lohiya, L. Lorenzovici, M. Lotfi, A. Mahmoodpoor, M. A. Mahmoud, R. Mahmoudi, A. Majeed, J. Majidpoor, A. Makki, G. A. Mamo, Y. Manla, M. Martorell, C. N. Matei, B. McManigal, E. Mehrabi Nasab, R. Mehrotra, A. Melese, O. Mendoza-Cano, R. G. Menezes, A.-F. A. Mentis, G. Micha, I. M. Michalek, A. C. Micheletti Gomide Nogueira de Sá, N. Milevska Kostova, S. A. Mir, M. Mirghafourvand, S. Mirmoeeni, E. M. Mirrakhimov, M. Mirza-Aghazadeh-Attari, A. S. Misganaw, A. Misganaw, S. Misra, E. Mohammadi, M. Mohammadi, A. Mohammadian-Hafshejani, S. Mohammed, S. Mohan, M. Mohseni, A. H. Mokdad, S. Momtazmanesh, L. Monasta, C. E. Moore, M. Moradi, M. Moradi Sarabi, S. D. Morrison, M. Motaghinejad, H. Mousavi Isfahani, A. Mousavi Khaneghah, S. A. Mousavi-Aghdas, S. Mubarik, F. Mulita, G. B. B. Mulu, S. B. Munro, S. Muthupandian, T. S. Nair, A. A. Naqvi, H. Narang, Z. S. Natto, M. Naveed, B. P. Nayak, S. Naz, I. Negoi, S. A. Nejadghaderi, S. Neupane Kandel, C. H. Ngwa, R. K. Niazi, A. T. Nogueira de Sá, N. Noroozi, H. Nouraei, A. Nowroozi, V. Nuñez-Samudio, J. J. Nutor, C. I. Nzoputam, O. J. Nzoputam, B. Oancea, R. M. Obaidur, V. A. Ojha, A. P. Okekunle, O. C. Okonji, A. T. Olagunju, B. O. Olusanya, A. Omar Bali, E. Omer, N. Otstavnov, B. Oumer, M. P A, J. R. Padubidri, K. Pakshir, T. Palicz, A. Pana, S. Pardhan, J. L. Paredes, U. Parekh, E.-C. Park, S. Park, A. Pathak, R. Paudel, U. Paudel, S. Pawar, H. Pazoki Toroudi, M. Peng, U. Pensato, V. C. F. Pepito, M. Pereira, M. F. P. Peres, N. Perico, I.-R. Petcu, Z. Z. Piracha, I. Podder, N. Pokhrel, R. Poluru, M. J. Postma, N. Pourtaheri, A. Prashant, I. Qattea, M. Rabiee, N. Rabiee, A. Radfar, S. Raeghi, S. Rafiei, P. R. Raghav, L. Rahbarnia, V. Rahimi-Movaghar, M. Rahman, M. A. Rahman, A. M. Rahmani, V. Rahmanian, P. Ram, M. M. A. N. Ranjha, S. J. Rao, M.-M. Rashidi, A. Rasul, Z. A. Ratan, S. Rawaf, R. Rawassizadeh, M. S. Razeghinia, E. M. M. Redwan, M. T. Regasa, G. Remuzzi, M. A. Reta, N. Rezaei, A. Rezapour, A. Riad, R. K. Ripon, K. E. Rudd, B. Saddik, S. Sadeghian, U. Saeed, M. Safaei, A. Safary, S. Z. Safi, M. Sahebazzamani, A. Sahebkar, H. Sahoo, S. Salahi, S. Salahi, H. Salari, S. Salehi, H. Samadi Kafil, A. M. Samy, N. Sanadgol, S. Sankararaman, F. Sanmarchi, B. Sathian, M. Sawhney, G. K. Saya, S. Senthilkumaran, A. Seylani, P. A. Shah, M. A. Shaikh, E. Shaker, M. Z. Shakhmardanov, M. M. Sharew, A. Sharifi-Razavi, P. Sharma, R. A. Sheikhi, A. Sheikhy, P. H. Shetty, M. Shigematsu, J. I. Shin, H. Shirzad-Aski, K. M. Shivakumar, P. Shobeiri, S. A. Shorofi, S. Shrestha, M. M. Sibhat, N. B. Sidemo, M. K. Sikder, L. M. L. R. Silva, J. A. Singh, P. Singh, S. Singh, M. S. Siraj, S. S. Siwal, V. Y. Skryabin, A. A. Skryabina, B. Socea, D. D. Solomon, Y. Song, C. T. Sreeramareddy, M. Suleman, R. Suliankatchi Abdulkader, S. Sultana, M. Szócska, S.-A. Tabatabaeizadeh, M. Tabish, M. Taheri, E. Taki, K.-K. Tan, S. Tandukar, N. Y. Tat, V. Y. Tat, B. N. Tefera, Y. M. Tefera, G. Temesgen, M.-H. Temsah, S. Tharwat, A. Thiyagarajan, I. I. Tleyjeh, C. E. Troeger, K. K. Umapathi, E. Upadhyay, S. Valadan Tahbaz, P. R. Valdez, J. Van den Eynde, H. R. van Doorn, S. Vaziri, G.-I. Verras, H. Viswanathan, B. Vo, A. Waris, G. T. Wassie, N. D. Wickramasinghe, S. Yaghoubi, G. A. T. Y. Yahya, S. H. Yahyazadeh Jabbari, A. Yigit, V. Yiğit, D. K. Yon, N. Yonemoto, M. Zahir, B. A. Zaman, S. B. Zaman, M. Zangiabadian, I. Zare, M. S. Zastrozhin, Z.-J. Zhang, P. Zheng, C. Zhong, M. Zoladl, A. Zumla, S. I. Hay, C. Dolecek, B. Sartorius, C. J. L. Murray and M. Naghavi, Lancet, 2022, 400, 2221–2248 CrossRef PubMed.
- L. Váradi, J. L. Luo, D. E. Hibbs, J. D. Perry, R. J. Anderson, S. Orenga and P. W. Groundwater, Chem. Soc. Rev., 2017, 46, 4818–4832 RSC.
- C.-S. Ho, N. Jean, C. A. Hogan, L. Blackmon, S. S. Jeffrey, M. Holodniy, N. Banaei, A. A. E. Saleh, S. Ermon and J. Dionne, Nat. Commun., 2019, 10, 4927 CrossRef PubMed.
- S. Kai, Y. Matsuo, S. Nakagawa, K. Kryukov, S. Matsukawa, H. Tanaka, T. Iwai, T. Imanishi and K. Hirota, FEBS Open Bio, 2019, 9, 548–557 CrossRef CAS PubMed.
- F. P. Maurer, M. Christner, M. Hentschke and H. Rohde, Infect. Dis. Rep., 2017, 9, 6839 CrossRef PubMed.
- B. Fidalgo, L. Morata, C. Cardozo, A. del Río, J. Morales, M. Fernández-Pittol, J. A. Martínez, J. Mensa, J. Vila, A. Soriano and C. Casals-Pascual, Clin. Infect. Dis, 2023, 77, 680–686 CrossRef CAS PubMed.
- C. W. Seymour, F. Gesten, H. C. Prescott, M. E. Friedrich, T. J. Iwashyna, G. S. Phillips, S. Lemeshow, T. Osborn, K. M. Terry and M. M. Levy, N. Engl. J. Med., 2017, 376, 2235–2244 CrossRef PubMed.
- C. Giuliano, C. R. Patel and P. B. Kale-Pradhan, P T, 2019, 44, 192–200 Search PubMed.
- C. Weis, A. Cuénod, B. Rieck, O. Dubuis, S. Graf, C. Lang, M. Oberle, M. Brackmann, K. K. Søgaard, M. Osthoff, K. Borgwardt and A. Egli, Nat. Med., 2022, 28, 164–174 CrossRef CAS PubMed.
- H. Zadka, E. Raykhshtat, B. Uralev, N. Bishouty, A. Weiss-Meilik and A. Adler, Eur. J. Clin. Microbiol. Infect. Dis., 2019, 38, 2053–2059 CrossRef CAS PubMed.
- D. D. Rhoads, S. B. Cox, E. J. Rees, Y. Sun and R. D. Wolcott, BMC Infect. Dis., 2012, 12, 321 CrossRef PubMed.
- S. K. Rampini, G. V. Bloemberg, P. M. Keller, A. C. Büchler, G. Dollenmaier, R. F. Speck and E. C. Böttger, Clin. Infect. Dis, 2011, 53, 1245–1251 CrossRef CAS PubMed.
- D. L. Church, L. Cerutti, A. Gürtler, T. Griener, A. Zelazny and S. Emler, Clin. Microbiol. Rev., 2020, 33, e00053-19 CrossRef PubMed.
- J. O. Lay Jr., Mass Spectrom. Rev., 2001, 20, 172–194 CrossRef CAS PubMed.
- A. M. Hamid, A. K. Jarmusch, V. Pirro, D. H. Pincus, B. G. Clay, G. Gervasi and R. G. Cooks, Anal. Chem., 2014, 86, 7500–7507 CrossRef CAS PubMed.
- A. Sloan, G. Wang and K. Cheng, Clin. Chim. Acta, 2017, 473, 180–185 CrossRef CAS PubMed.
- M. C. Ge, A. J. Kuo, K. L. Liu, Y. H. Wen, J. H. Chia, P. Y. Chang, M. H. Lee, T. L. Wu, S. C. Chang and J. J. Lu, J. Microbiol., Immunol. Infect., 2017, 50, 662–668 CrossRef CAS PubMed.
- T. R. Sandrin and P. A. Demirev, Mass Spectrom. Rev., 2018, 37, 321–349 CrossRef CAS PubMed.
- A. Croxatto, G. Prod'hom and G. Greub, FEMS Microbiol. Rev., 2012, 36, 380–407 CrossRef CAS PubMed.
- Y. Yuan, J. Wang, J. Zhang, B. Ma, S. Gao, Y. Li, S. Wang, B. Wang, Q. Zhang and N. Jing, J. Clin. Lab. Anal., 2020, 34, e23119 CrossRef CAS PubMed.
- K. Sogawa, M. Watanabe, K. Sato, S. Segawa, C. Ishii, A. Miyabe, S. Murata, T. Saito and F. Nomura, Anal. Bioanal. Chem., 2011, 400, 1905–1911 CrossRef CAS PubMed.
- M. Šebela, E. Jahodářová, M. Raus, R. Lenobel and P. Hašler, PLoS One, 2018, 13, e0208275 CrossRef PubMed.
- E. Carbonnelle, J. L. Beretti, S. Cottyn, G. Quesne, P. Berche, X. Nassif and A. Ferroni, J. Clin. Microbiol., 2007, 45, 2156–2161 CrossRef CAS PubMed.
- K. Bernardo, N. Pakulat, M. Macht, O. Krut, H. Seifert, S. Fleer, F. Hünger and M. Krönke, Proteomics, 2002, 2, 747–753 CrossRef CAS PubMed.
- V. Ryzhov and C. Fenselau, Anal. Chem., 2001, 73, 746–750 CrossRef CAS PubMed.
- M. Vargha, Z. Takáts, A. Konopka and C. H. Nakatsu, J. Microbiol. Methods, 2006, 66, 399–409 CrossRef CAS PubMed.
- O. Šedo, I. Sedláček and Z. Zdráhal, Mass Spectrom. Rev., 2011, 30, 417–434 CrossRef PubMed.
- R. Chean, D. Kotsanas, M. J. Francis, E. A. Palombo, S. R. Jadhav, M. M. Awad, D. Lyras, T. M. Korman and G. A. Jenkin, Anaerobe, 2014, 30, 85–89 CrossRef CAS PubMed.
- T. R. Sandrin, J. E. Goldstein and S. Schumaker, Mass Spectrom. Rev., 2013, 32, 188–217 CrossRef CAS PubMed.
- B. La Scola, P.-E. Fournier and D. Raoult, Anaerobe, 2011, 17, 106–112 CrossRef CAS PubMed.
- E. Torres-Sangiao, C. Leal Rodriguez and C. García-Riestra, Microorganisms, 2021, 9, 1539 CrossRef CAS PubMed.
- O. Clerc, G. Prod'hom, C. Vogne, A. Bizzini, T. Calandra and G. Greub, Clin. Infect. Dis, 2012, 56, 1101–1107 CrossRef PubMed.
- R. C. Team, R: A language and environment for statistical computing, https://www.R-project.org/, 2023.
- R. A. M. Villanueva and Z. J. Chen, ggplot2: elegant graphics for data analysis, Taylor & Francis, 2019 Search PubMed.
- C. Sievert, Interactive web-based data visualization with R, plotly, and shiny, CRC Press, 2020 Search PubMed.
- J. Oksanen, F. G. Blanchet, R. Kindt, P. Legendre, P. R. Minchin, R. O’hara, G. L. Simpson, P. Solymos, M. H. H. Stevens and H. Wagner, R package version, 2013, 2, 321–326 Search PubMed.
- P. Solymos and E. Azeria, Likelihood based optimal partitioning and indicator species analysis, R package version 0.1–2, <https://CRAN.R-project.org/package=opticut>, 2023 Search PubMed.
- U. Ligges and M. Maechler, J. Stat. Softw., 2003, 8, 1–20 Search PubMed.
- R. Kolde, pheatmap: Pretty Heatmaps, https://cran.r-project.org/web/packages/pheatmap/index.html, 2023.
- A. L. Flores-Mireles, J. N. Walker, M. Caparon and S. J. Hultgren, Nat. Rev. Microbiol., 2015, 13, 269–284 CrossRef CAS PubMed.
- S. Y. C. Tong, J. S. Davis, E. Eichenberger, T. L. Holland and V. G. Fowler, Clin. Microbiol. Rev., 2015, 28, 603–661 CrossRef CAS PubMed.
- C. E. Demeure, O. Dussurget, G. Mas Fiol, A. S. Le Guern, C. Savin and J. Pizarro-Cerdá, Genes Immun., 2019, 20, 357–370 CrossRef PubMed.
- I. Ansari, G. Grier and M. Byers, J. Biosaf. Biosecur., 2020, 2, 10–22 CrossRef PubMed.
- A. A. Alatoom, S. A. Cunningham, S. M. Ihde, J. Mandrekar and R. Patel, J. Clin. Microbiol., 2020, 49, 2868–2873 CrossRef PubMed.
- S. Tsuchida and T. Nakayama, Appl. Sci., 2022, 12, 2814 CrossRef CAS.
- S. C. Smole, L. A. King, P. E. Leopold and R. D. Arbeit, J. Microbiol. Methods, 2002, 48, 107–115 CrossRef CAS PubMed.
- D. Yukihira, D. Miura, K. Saito, K. Takahashi and H. Wariishi, Anal. Chem., 2010, 82, 4278–4282 CrossRef CAS PubMed.
- A. B. Esselman, N. H. Patterson, L. G. Migas, M. Dufresne, K. V. Djambazova, M. E. Colley, R. Van de Plas and J. M. Spraggins, J. Am. Soc. Mass Spectrom., 2023, 34, 1305–1314 CrossRef CAS PubMed.
- M. J. Stump, G. Black, A. Fox, K. F. Fox, C. E. Turick and M. Matthews, J. Sep. Sci., 2005, 28, 1642–1647 CrossRef CAS PubMed.
- A. Maus, B. Bisha, C. Fagerquist and F. Basile, J. Appl. Microbiol., 2020, 128, 697–709 CrossRef CAS PubMed.
- W. Florio, S. Cappellini, C. Giordano, A. Vecchione, E. Ghelardi and A. Lupetti, BMC Microbiol., 2019, 19, 267 CrossRef CAS PubMed.
- C. K. Fagerquist, B. G. Lee, W. J. Zaragoza, J. C. Yambao and B. Quiñones, Int. J. Mass Spectrom., 2019, 438, 1–12 CrossRef CAS.
Footnote |
† Current address: Bruker Scientific, Billerica, MA 01821. |
|
This journal is © The Royal Society of Chemistry 2024 |