DOI:
10.1039/D4AN00320A
(Paper)
Analyst, 2024,
149, 2738-2746
Conformational fingerprinting with Raman spectroscopy reveals protein structure as a translational biomarker of muscle pathology†
Received
28th February 2024
, Accepted 11th March 2024
First published on 27th March 2024
Abstract
Neuromuscular disorders are a group of conditions that can result in weakness of skeletal muscles. Examples include fatal diseases such as amyotrophic lateral sclerosis and conditions associated with high morbidity such as myopathies (muscle diseases). Many of these disorders are known to have abnormal protein folding and protein aggregates. Thus, easy to apply methods for the detection of such changes may prove useful diagnostic biomarkers. Raman spectroscopy has shown early promise in the detection of muscle pathology in neuromuscular disorders and is well suited to characterising the conformational profiles relating to protein secondary structure. In this work, we assess if Raman spectroscopy can detect differences in protein structure in muscle in the setting of neuromuscular disease. We utilise in vivo Raman spectroscopy measurements from preclinical models of amyotrophic lateral sclerosis and the myopathy Duchenne muscular dystrophy, together with ex vivo measurements of human muscle samples from individuals with and without myopathy. Using quantitative conformation profiling and matrix factorisation we demonstrate that quantitative ‘conformational fingerprinting’ can be used to identify changes in protein folding in muscle. Notably, myopathic conditions in both preclinical models and human samples manifested a significant reduction in α-helix structures, with concomitant increases in β-sheet and, to a lesser extent, nonregular configurations. Spectral patterns derived through non-negative matrix factorisation were able to identify myopathy with a high accuracy (79% in mouse, 78% in human tissue). This work demonstrates the potential of conformational fingerprinting as an interpretable biomarker for neuromuscular disorders.
1 Introduction
Neuromuscular disorders are a complex, heterogeneous group of conditions that can be difficult to diagnose and monitor. Examples include amyotrophic lateral sclerosis (ALS), in which weakness is caused by a progressive loss of the motor neurones controlling movement; mitochondrial disease, in which cellular energy production is comprised leading to multi-system pathology; and primary myopathies in which alterations to muscle proteins result in functional impairment of the muscle. Neuromuscular disorders can present to clinicians with a wide range of different symptoms and several different investigations may be used in the diagnostic work up. For example, imaging (e.g. MRI), blood tests (e.g. autoimmune antibody testing) and neurophysiology (electromyography) may be utilised in differing combinations to complement clinical acumen, while genetic testing is now often used as a frontline test when a genetic component is suspected. Regardless of the approach, significant diagnostic delays are experienced across most neuromuscular conditions.1–4 In addition, translational biomarkers of disease that can cross the divide between preclinical research and clinical trials are lacking.5
Raman spectroscopy is an emerging biomarker for neuromuscular diseases that derives a biochemical fingerprint from the sample of interest via the vibration of chemical bonds. Work from our group and others has also demonstrated the potential of Raman to identify several neurological diseases from a range of tissues, including serum,6–8 saliva9 and tears.10 We have recently developed in vivo muscle recordings for the assessment of preclinical mouse models11–13 and demonstrated efficacy in human biopsy samples.14 Spontaneous Raman spectroscopy is an attractive potential biomarker for neuromuscular disease as it is simple to implement, requiring no sample preparation or tissue labelling. Technological advancements are starting to overcome the inherently weak Raman scattering effect and make the instrumentation suitable for deployment in clinical environments.
While a range of compositional features are assessed in a Raman spectrum, Raman spectroscopy has long been used to probe protein structure. With peak fitting techniques, spectra can provide information on secondary and tertiary arrangements, which have been termed a ‘conformational fingerprint’.15 The amide I region is particularly well suited for this purpose, with protein rich tissues manifesting a strong peak between 1600–1700 cm−1.16 This region has been extensively used to study the secondary structure of proteins through the contribution of α-helical, β-sheet and non-regular components.17,18 In recent years, this has been applied to neurological conditions characterised by protein misfolding, for example in studies on the pathological protein aggregates observed in Alzheimer's disease.19
As a protein rich tissue, in which α-helices are the predominant secondary structure,20 muscle is highly suitable for protein conformation profiling. Using Raman, protein structures have been studied in the context of the handling and storage of muscle foods, using both isolated protein preparations and intact whole tissue.21 However, the study of protein conformations in human neuromuscular diseases has not yet been performed with Raman spectroscopy. Despite this, alterations to protein structure are a common pathological feature of many muscle diseases. Indeed, some can be considered conformational disorders characterised by protein unfolding, misfolding and aggregation, leading to the label ‘protein aggregate myopathies’.22,23 Specific examples include inclusion body myopathies and myofibrillar myopathies. Many myopathies outside this group also demonstrate evidence of protein misfolding, these include dystrophic24–26 and inflammatory myopathies.27
In this study we have tested the hypothesis that myopathies will result in an altered secondary protein structure or ‘conformational fingerprint’ detectable through Raman spectroscopy which can be used to identify disease. Using a combination of peak fitting and matrix factorisation techniques across preclinical models and human samples, we show that muscle disease manifests a reduction in α-helical content that can be quantified at both a group and individual sample level.
2 Methods
2.1 Fibre optic Raman spectroscopy
The fibre optic Raman system utilised a 0.5 mm probe housed within a 21-gauge hypodermic needle (Fig. 1).28 A 830 nm semiconductor laser was used (Innovative Photonics Solutions), with two low-OH fibres (cladding diameter 125 μm, core diameter 105 μm) providing the delivery and collection light paths. In-line bandpass filters were used to remove inelastically scattered light and fluorescence associated with the fibres (Semrock Inc.). The collection fibre was optically coupled to the spectrometer (Raman Explorer Spectrograph, Headwall Photonics, Inc.) and iDus 420BR-DD CCD camera (Andor Technology, Ltd). The spectral resolution across the fingerprint region was 2.3 cm−1. Laser power at the probe end was 60 mW and the acquisition time for the collection of spectra was 40 seconds for all studies. The probe was constructed such that the fibre optics were able to be deployed around 1 mm from the top of the needle.
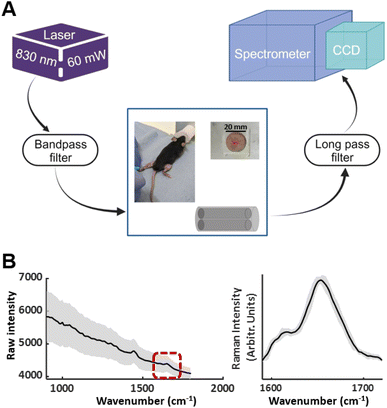 |
| Fig. 1 Schematic of the experimental set up. (A) An 830 nm semiconductor laser and two low-OH fibres (shown in the cylinder) were used, with filters to remove inelastically scattered light and fluorescence. Preclinical recordings were made in vivo under anaesthesia, while human muscle biopsy measurements were made on calcium fluoride slides. The collection fibre was optically couple to the spectrometer and CCD. Created with BioRender.com. (B) Raw spectra (mean, standard deviation shaded) within the fingerprint region are shown with the amide I region highlighted (red box). This region was selected, spectra from each sample averaged and background subtracted (Rubber band algorithm), followed by smoothing (second order Savitzky–Golay filter, 5 data point window width) and vector normalisation. The resulting mean (±standard deviation) of the amide I region is shown to the right. | |
2.2 Preclinical recordings
Procedures were undertaken with University of Sheffield Ethical Review Sub-Committee and UK Home Office approval (licence number 70/8587), as per the Animal (Scientific Procedures) Act 1986. Experiments were performed following the ARRIVE guidelines.29 Breeding was undertaken in a specified pathogen-free environment and experimental work was undertaken in a standard preclinical facility (12-hour light/dark cycle and room temperature 21 °C). As a model of muscle disease, the mdx model of Duchenne muscular dystrophy was utilised. Mice were aged between 30–90 days, during which time pathological changes are evident (total n = 32).30 Corresponding wild-type healthy control mice (C57BL/10ScSnOlaHsd) were used with the same age range (total n = 32). The SOD1G93A model of motor neurone disease was used as a model of ‘neurogenic’ disease (disease relating to nerve or motor neurone pathology) at 90 days, when pathological changes are clearly evident (n = 16),11 together with non-transgenic healthy littermate control mice (n = 16). For analysis, spectra from the two colonies of healthy mice were combined into one ‘healthy’ group. A total of 96 mice were used, all of which were female. This was done as female SOD1G93A mice display less variability in their phenotype than male mice31 and female mdx mice manifest prominent pathological changes (as unlike in the human disease – Duchenne muscular dystrophy – the mutation in mdx is not sex-linked).32 By using female mice from both colonies, we were therefore able to avoid any confounding effects relating to gender.
Recordings were undertaken as detailed previously.11 Briefly, mice were anaesthetised using 2% isoflurane and hindlimb fur removed. The needle probe was then inserted through the skin and into the gastrocnemius muscles and the optical fibres were deployed. Two insertions were made in each muscle (the medial and lateral heads of the gastrocnemius muscle) and both legs were studied (recordings undertaken by JJPA, MP and JCD).
2.3 Human muscle sample recordings
Samples from 54 participants were studied. Briefly, these comprised 10 healthy volunteers with no neurological disease and 17 patients investigated for myopathy but found to have alternative conditions. These were combined to form a ‘not myopathy’ group. The age range of this group was 23–80 years, details regarding diagnoses can be found in ESI Table 1.† 27 patients with a final diagnosis of myopathy (age range of 22–80 years) contributed samples. The most common form of myopathy was mitochondrial myopathy (n = 15), other diagnoses included dystrophic and inflammatory conditions. Further details are in ESI Table 1.† Biopsies were collected either during surgery for a joint injury (healthy volunteers), or via conchotome needle or open biopsy (in patients investigated for/diagnosed with muscle disease at Newcastle and Sheffield, respectively). Samples were snap frozen and stored at −80 °C until use. In addition, three samples from boys with DMD were also obtained. The study was approved by NHS Research Ethics committees (references 16/YH/0261 and 09/H0906/75) and Good Clinical Practice (GCP) guidelines were followed. All participants provided informed consent. For the acquisition of spectra, the fibre optics were pressed gently against the muscle sample. A total of 2–6 sites were studied, depending upon the size of the sample.
2.4 Data analysis
Spectral pre-processing, matrix factorisation and classification were undertaken using custom code in MATLAB (R2023a). Spectra were collected between 900–1800 cm−1, with the lower bound set at 900 cm−1 to avoid the silica-related background from the fibre optics. Spectra were interpolated to even wavenumber spacings and then windowed in the amide I region 1590–1720 cm−1.17 Averaging (mean) was then performed such that one mouse/human muscle sample presented one spectrum to the subsequent analysis. Background removal was undertaken using the Rubber band algorithm,33 followed by smoothing (second order Savitzky–Golay filter, 5 data point window width) and vector normalisation, with a minimum arbitrary intensity of zero for each spectrum.
Peak fitting was undertaken with Origin (2023). First, group means were generated and scaled (0–1). A mixed Lorentz/Gaussian (Voigt) function was used. For preclinical data, six peaks centred on 1601 and 1615 cm−1 (aromatic amino side chains), 1635 cm−1 (nonregular), 1652 cm−1 (α-helix), 1663 cm−1 (β-sheet) and 1677 cm−1 (nonregular) were used.15,17,19 For human sample analysis, an additional peak at 1705 cm−1 (nonregular) was included.34 In both preclinical and clinical analyses, the starting height for each peak was the amide I spectral intensity at that wavenumber. Full width at half maximum was enabled to an upper limit of 30 cm−1. The percentage of aromatic amino acids and secondary structure components were reported as the percentage of a given peak relative to all peaks utilised in the fitting. Secondary structure ratios were calculated using the percentage integrated area under the peaks of interest (as a proportion of all peaks).
Spectral patterns were derived through a hierarchical alternating least squares non-negative matrix factorisation (NMF) algorithm optimised for low rank solutions.35 Briefly, non-negative matrix factorisation approximates the original data (A, an n × m matrix where n is the number of samples and m is the matrix length or number of observations per sample) as the product of two lower rank matrices, A = WH, where W represents the derived non-negative spectral patterns (modes) and the matrix H represents the relative importance (the weights, or coefficients) of those patterns to each sample. The number of selected spectral patterns (rank) was determined by calculating the root mean square residual of randomly divided healthy samples in both the preclinical and human datasets, since the difference between two such matrices can be considered to represent biological noise.13 To estimate the relative contributions of different secondary structures within each spectral pattern (mode), two approaches were employed, First, the second derivative of each mode was calculated and subjected to a Savitzky–Golay smooth (second order, 5 data points). Peaks were then identified using a 20% threshold, which excluded minor peaks, and a Voigt fitting function utilised. Peaks were allocated to α-helix (1650–1658 cm−1), β-sheet (1664–1673 cm−1) and nonregular (1630–1640, 1674–1689 and 1700–1710 cm−1) structures.15,17,19,34 A simpler approach was also used in which the area under those wavenumber regions was integrated. Lastly, principal component analysis over the amide I region was also performed for comparison with the NMF approach. Mode coefficients (weights) were compared using unpaired t-tests (GraphPad Prism, version 9).
For classification, the NMF weights were fed into a linear discriminant model and performance statistics derived through a 10-fold cross validation with stratification, which preserves the balance of classes across the folds (MATLAB, 2023a). For the three-group mouse analysis, the area under the receiver operating characteristic curves (AUROCs) was computed using a one-versus-all approach in which the multiclass classification is reduced to a set of binary classifications.
3 Results and discussion
3.1
In vivo preclinical data
In vivo Raman spectra were collected from the mdx model of Duchenne muscular dystrophy (a form of primary muscle disease), the SOD1G93A model of ALS (a ‘neurogenic’ condition arising due to the loss of motor neurones) and age-matched non-transgenic/wild type healthy mice. Peak fitting of the amide I region was performed to explore secondary protein structure through four mixed Gaussian/Lorentz profiles, representing α-helical, β-sheet and non-regular (NR) structures (Fig. 2). In addition, two further curves representing aromatic amino acids were included. Detailed peak characteristics are shown in supplemental Table 2; of note, full width at half maximum was within the resolution of the system. Utilising the percentage area under each curve, a reduction in the α-helical content in the mdx model was seen (48.3%for mdx versus 73.2% for healthy muscle). A smaller reduction in α-helical was observed for SOD1G93A muscle (68%; Fig. 1). There was a corresponding increase in β-sheet content in mdx (19.3% in mdx versus 4.6% for healthy muscle and 5.6% for SOD1G93A muscle). Nonregular content was increased in mdx (20.3% in mdx versus 10.8% in healthy muscle) and to a lesser degree in SOD1G93A (14.2%). These changes can also be appreciated through α-helix: β-sheet and β-sheet: NR ratios (Fig. 1E).
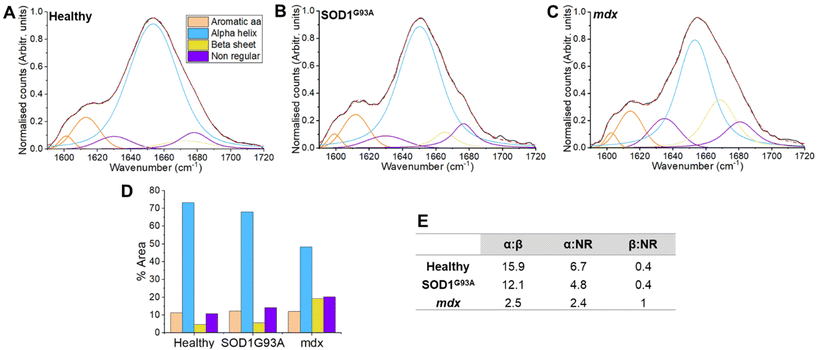 |
| Fig. 2 Preclinical peak fitting of healthy, neurogenic and myopathic muscle shows a reduction in α-helix conformation in myopathy. (A–C) Amide I fitting using standard peaks centred on 1601, 1615, 1635, 1652, 1663 and 1677 cm−1. For the analysis, each mouse presented one spectrum to the analysis (healthy n = 48, mdx n = 32 and SOD1G93An = 16). A group average was created which was used in the fitting process. (D) Each of the peaks resolved as a percentage of the total area. A reduction of α-helix and concomitant increase in β-sheet can be seen in the model of myopathy (mdx). (E) Ratios of different protein conformations. The reduced α-helix/increased β-sheet is evident in the mdx model of myopathy, which also manifests an increase in the β-sheet/nonregular ratio. | |
Of relevance to the dystrophic myopathy model employed herein (mdx), protein studies have demonstrated significant misfolding in Duchenne muscular dystrophy, with reduced α-helical content and aggregation of intermolecular β structures of the dystrophin protein and associated protein complex.24,36,37 The dystrophic associated complex interacts with many important proteins (e.g. structural, scaffolding proteins) either directly or indirectly and, as a result, a deficiency of dystrophin leads to a loss of the structural integrity of the muscle cells.38 By taking measures from intact muscle, we are not able to say whether our results stem directly from alterations to the dystrophin-associated protein complex or follow-on effects upon other muscle cell proteins. Notwithstanding this uncertainty, in the closely related Becker form of muscular dystrophy, more profound structural modifications in the mutated dystrophic protein are associated with a more severe form of the disease,39 raising the possibility that conformational fingerprinting may be useful for the monitoring of disease state. Our observations are also in agreement with the preclinical Raman data of Gautam et al., who observed a reduction in α-helical content across a several fly models of human myopathy.40
In our analysis, the changes to protein folding appear different in myopathy and neurogenic disease models. In mdx, a large increase in both β-sheet and nonregular structures was observed. By contrast, SOD1G93A largely manifested a more selective increase in nonregular structures. Protein misfolding is a feature of SOD1 ALS, with accumulations particularly prominent within the spinal cord in both human patients and rodent models.41–43 Increases in misfolded proteins have also been reported in the context of denervation due to nerve ligation.44 Thus, it is possible that the more modest structural alterations we observed in SOD1G93A mice are a general phenomenon relating to denervation, rather than specific to ALS. In future work it will be worthwhile to ascertain if this difference is present in animal and human tissues, as the myopathy changes appear to be (see section 3.2). In both myopathic and neurogenic conditions it will be of interest to ascertain whether the structural modifications change with progression of the underlying disease.
Quantification at the individual mouse level was undertaken using non-negative matrix factorisation. As evident in Fig. 3, spectral modes which were dominant (i.e. had higher scores) in mdx, manifested patterns associated with β-sheet and nonregular conformations (e.g. modes 3 and 5; see ESI Fig. 1 for mode profiles with peak wavenumbers labelled†). By contrast, mode 2, which was more dominant in SOD1G93A had no β-sheet region. Feeding these modes into a three-group linear discriminant analysis algorithm demonstrated an average area under the receiver operating characteristic curve (AUROC) of 0.75 (Table 1), with the identification of mdx most successful. An equivalent analysis with principal component analysis (arguably the standard dimensional reduction technique in Raman spectroscopy) is shown in ESI Fig. 2,† demonstrating a lower classification performance (AUROC – 0.70).
Table 1 Three-group classification performance for in vivo preclinical data using the non-negative matrix
|
Accuracy
|
Sensitivity |
Specificity |
AUROC |
Healthy |
64% |
48% |
79% |
0.68 |
Mdx |
79% |
75% |
81% |
0.83 |
SOD1G93A |
76% |
62% |
79% |
0.74 |
Average |
73% |
62% |
80% |
0.75 |
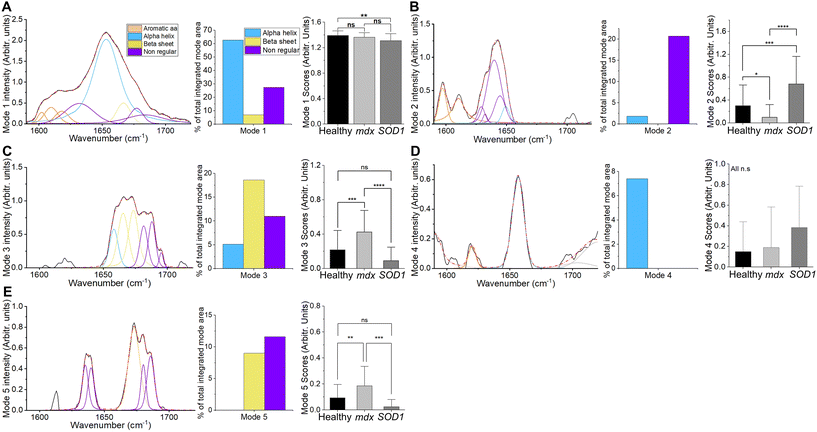 |
| Fig. 3 Non-negative matrix factorisation derived spectral patterns show conformational differences in preclinical models. Using the amide I spectra from all mice (n = 96), non-negative matrix factorisation was performed. The five unique spectral patterns (A–D, ‘modes’) output are shown. Peak fitting profiles are shown within the pattern profiles. In this analysis, the peaks for fitting were identified through examination of the second derivative (see methods). The integrated area for each protein structure is then shown as a bar chart, allowing clear differences in conformational content to be appreciated. The mode scores, representing the importance of a given mode to each sample, were then subjected to statistical testing. Each mouse presented one score for each mode (healthy n = 48, mdx n = 32 and SOD1G93An = 16). *P < 0.05, **P < 0.01, ***P < 0.001, ****P < 0.0001. | |
The matrix factorisation technique employed constrains the outputs to a non-negative distribution. The result is a more interpretable profile than would be obtained through, for example, principal component analysis.45 Thus, the spectral modes provide a profile for peak fitting (as shown in Fig. 3), or a simpler integration of the area under specific wavenumber windows (ESI Fig. 3†). Both approaches align with a simple visual inspection of the modes and their peaks, as well as the more traditional peak fitting data. A benefit of utilising the non-negative approach is that the importance of each spectral mode to each sample allows for quantitation at the level of individual samples (mice in this instance), something not possible with peak fitting, unless broad wavenumber window smoothing is employed.15
3.2
Ex vivo human muscle biopsy data
In the preclinical part of our study, we used the well characterised mdx model of DMD. As DMD is diagnosed in childhood, and often from a genetic blood test, obtaining human muscle samples can be challenging. After consultation with UK biobanks, we were able to obtain three samples. Inclusion of these alongside the mouse data showed a promising agreement with the preclinical results (ESI Fig. 4).
In order to undertake a more detailed assessment of whether changes to protein secondary structures are evident in human tissue, we studied muscle biopsies from adult patients. As patients under investigation for ‘neurogenic’ (e.g. nerve/motor neurone related) conditions such as ALS rarely undergo muscle biopsies, our human analysis focused on myopathy. Samples were divided into ‘not myopathy’ and ‘myopathy’ groups (see methods and ESI Table 2 for details†). Peak fitting once again demonstrated a reduced α-helix: β-sheet ratio in the myopathy group, together with a decrease in the in the α-helix: NR ratio (Fig. 4; see ESI Table 3 for further peak details†). On this occasion, an increase in the aromatic amino acid side chains was also evident. These residues cluster in the core of folded proteins46 and play an important role in the stability of the protein structure.47 During modifications, such as unfolding, these amino acids become exposed40 and as such may also represent a marker of muscle health.
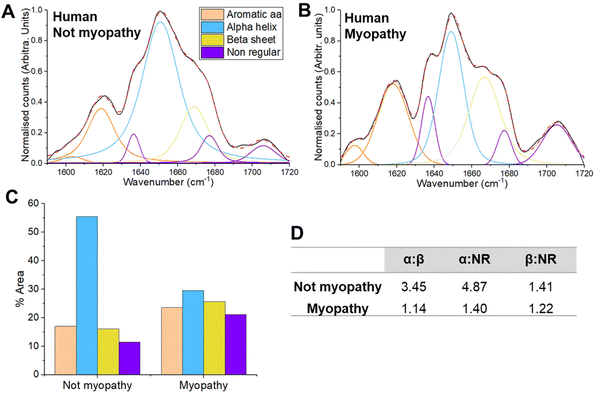 |
| Fig. 4 Peak fitting from Raman muscle spectra from human samples obtained from patients with and without myopathy. (A and B) Amide I fitting using standard peaks centred on 1601, 1615, 1635, 1652, 1663, 1677 and 1705 cm−1. For the analysis, each biopsy sample presented one spectrum to the analysis (not myopathy n = 28, myopathy = 27). A group average was created which was used in the fitting process. (C) Each of the peaks resolved as a percentage of the total area. A reduction of α-helix and increases in other conformations and aromatic amino acids can be seen in the myopathy group. (D) Ratios of different protein conformations. The reduction in α-helix and increased β-sheet/nonregular structures is evident in myopathy. | |
Non-negative matrix factorisation spectral modes were derived and the mode with the greatest dominance in the ‘not myopathy’ group demonstrated a relatively balanced conformational fingerprint (Fig. 5; see ESI Fig. 5 for peak labels and ESI Fig. 6 for the area integrated approach to conformational profiling†). Using these three spectral modes within a linear discriminant classifier demonstrated a classification accuracy of 78% (Table 2), a result comparable to use of the whole spectrum,14 with the advantage that the data are more biologically interpretable. Of note, in this instance, a PCA-LDA based analysis manifested a lower performance (classification accuracy 67%, ESI Fig. 7†). It is worth also considering that, while the amide I region appears to comprise biologically relevant information useful in identifying disease, combination with other spectral regions (e.g. amide III) may improve performance. In addition, alternative algorithms, particularly non-linear methods, may provide improved disease identification. Thus, while the data herein represent a promising start, we hypothesise that with further development our approach can be augmented and diagnostic performance improved further.
Table 2 Two-group classification performance for human ex vivo samples using the non-negative matrix factorisation modes and a linear discriminant model
|
Accuracy |
Sensitivity |
Specificity |
AUROC |
Myopathy vs. not myopathy |
78% |
92% |
64% |
0.80 |
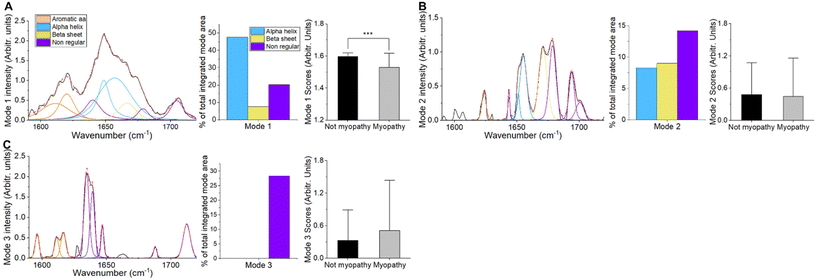 |
| Fig. 5 Non-negative matrix factorisation derived spectral patterns (modes) in human muscle. Using the amide I spectra from all sample (n = 54), non-negative matrix factorisation was performed. The three unique spectral patterns (A–C, ‘modes’) output are shown. Peak fitting profiles are shown within the pattern profiles, identified through examination of the second derivative (see Methods). The integrated area for each protein structure is then shown (bar chart), clear differences in conformational content can be appreciated. The mode scores, representing the importance of a given mode to each sample, were then subjected to statistical testing. Each sample presented one score for each mode (not myopathy n = 27, myopathy n = 27). A significant difference was observed for mode 1 (***P < 0.001). | |
Drawing a direct comparison with established investigations for muscle disease is difficult as the literature is highly variable and depends upon the patient group studied. Studies on EMG performance typically report sensitivity in the region of 0.74–0.87 and specificity in the region of 0.58–0.67.48–50 Muscle imaging results also vary (e.g. sensitivity 0.4–0.92 and specificity 0.32–0.99).51–53 Only around half of muscle biopsies may contribute to a specific diagnosis.54 As well as being broadly comparable to these reports, the results we present here provide a unique insight into the pathological changes occurring in muscle. In the UK, the Rare Diseases Framework (which many neuromuscular diseases fall within) highlights the need for better diagnostic tools.55 Similarly, outcome measures for clinical trials are also required.56 With further development, Raman spectroscopy of muscle may provide a unique means of identifying and characterising muscle disease and meeting these challenges.
It is worth noting that our human sample set comprised a range of different human myopathies, for example, mitochondrial myopathy (n = 15), muscular dystrophies (n = 5) and inflammatory myopathies (n = 3). It is possible that different myopathies may have different alterations in protein secondary structure, however, the small numbers of samples available means that we have not assessed this in the current study. If there are slightly different patterns across the groups, then pooling them as one group may make the matrix factorisation more challenging, and, by extension, the classification more difficult. For the same reason, having biopsies from the same muscle might also improve the class discrimination.
Human muscle diseases are relatively uncommon and muscle biopsies are invasive, making it difficult to obtain large numbers of samples from individual classes of myopathy. Progression of our in vivo preclinical protocol into human testing may help us obtain a greater number of samples/measurements in the future. In turn, this would permit a more detailed characterisation of the spectral changes across different conditions. Furthermore, human muscle pathology is not homogeneous within a sample (or muscle) and pathology can be missed.53 Thus, targeting the Raman probe to areas of interest, for example, through concomitant use of electromyography (‘optical EMG’56), may also improve detection of disease.
4 Conclusions
We have demonstrated the potential of conformational assessments of the Raman amide I region to assess disease related changes to muscle. The approach translates from an in vivo preclinical paradigm to human ex vivo tissue, using a fibre optic system with the potential for in vivo human recording. Through the application of matrix factorisation, we demonstrate that quantitative information on biologically relevant changes in protein secondary structure can be obtained and used to identify muscle pathology. We propose conformational fingerprinting as a new, translational biomarker for neuromuscular diseases and, in particular, for myopathy. Testing this approach in additional animal models and in vivo in human patients will ascertain its value as an output measure of muscle health.
Author contributions
JJPA: conceptualisation, methodology, formal analysis, investigation, data curation, writing – original draft, visualisation, supervision, project administration, funding acquisition. MP: investigation, writing – review and editing. APD: investigation, writing – review and editing. CAK: investigation, resources, writing – review and editing. CH: resources, writing – review and editing. MH: resources, writing – review and editing. GSG: resources, writing – review and editing. RWT: resources, writing – review and editing. CJM – resources, funding acquisition, writing – review and editing. PJS: resources, funding acquisition, writing – review and editing. RJM: resources, funding acquisition, investigation, writing – review and editing. JCD: resources, methodology, funding acquisition, investigation, writing – review and editing.
Conflicts of interest
J. J. P. A. and J. C. D. are the joint authors of a Patent Cooperation Treaty application concerning related technology and analyses (filed September 2023, PCT/EP2023/076380). For the remaining authors there are no conflicts of interest to declare.
Acknowledgements
This work was supported by an Academy of Medical Sciences Starter grant (JJPA, SGL015\1001) and a Medical Research Council Confidence in Concept award (J. J. P. A., J. C. D., P. J. S., MC_PC_15034). PJS is supported as a National Institute for Health Research (NIHR) Senior Investigator (NF-SI-0617-10077) and by the NIHR Sheffield Biomedical Research Centre (IS-BRC-1215-20017). RWT and GSG are supported by the Wellcome Centre for Mitochondrial Research (203105/Z/16/Z), the Mitochondrial Disease Patient Cohort (UK) (G0800674), the Lily Foundation, the NIHR Newcastle Biomedical Research Centre and the UK NHS Specialised Commissioners who fund the “Rare Mitochondrial Disorders of Adults and Children” Service in Newcastle upon Tyne. RWT receives additional support from the Medical Research Council (MRC) International Centre for Genomic Medicine in Neuromuscular Disease (MR/S005021/1), Mito Foundation and the Pathology Society. This research was funded in part, by the Wellcome Trust (203105/Z/16/Z). We also acknowledge the Oxford Brain Bank, supported by the Medical Research Council, Brains for Dementia Research (BDR) (Alzheimer Society and Alzheimer Research UK), Autistica, UK, and the NIHR Oxford Biomedical Research Centre. For the purpose of Open Access, the author has applied a CC BY public copyright licence to any Author Accepted Manuscript version arising from this submission.
The authors wish to thank the participants for contributing tissue samples for the study. We also wish to thank Lucinda Goult and Shirley Packwood (Sheffield Teaching Hospitals NHS Foundation Trust) for their valuable assistance with muscle biopsy collection. We gratefully acknowledge the support of the Newcastle Mitochondrial Research Biobank (REC reference 16NE/0267) in facilitating access to patient muscle biopsy samples.
This is independent research funded by Medical Research Council and supported by the National Institute for Health and Care Research (NIHR) Sheffield Biomedical Research Centre (BRC). The views expressed are those of the author(s) and not necessarily those of the Medical Research Council, the NIHR or the Department of Health and Social Care.
References
- G. C. Dobloug, E. A. Antal, L. Sveberg, T. Garen, H. Bitter, J. Stjärne, L. Grøvle, J. T. Gran and Ø. Molberg, Eur. J. Neurol., 2015, 22, 672-e41 CrossRef PubMed.
- S. Spuler, A. Stroux, F. Kuschel, A. Kuhlmey and F. Kendel, BMC Health Serv. Res., 2011, 11, 91 CrossRef PubMed.
- J. Grier, M. Hirano, A. Karaa, E. Shepard and J. L. P. Thompson, Neurol.: Genet., 2018, 4, e230 CAS.
- D. Richards, J. A. Morren and E. P. Pioro, J. Neurol. Sci., 2020, 417, 117054 CrossRef PubMed.
- B. S. Cowling and L. Thielemans, Dis. Models Mech., 2019, 13, dmm041434 CrossRef PubMed.
- J. J. P. Alix, N. S. Verber, C. N. Schooling, V. Kadirkamanathan, M. R. Turner, A. Malaspina, J. C. C. Day and P. J. Shaw, Analyst, 2022, 147, 5113–5120 RSC.
- Q. J. Zhang, Y. Chen, X. H. Zou, W. Hu, M. L. Ye, Q. F. Guo, X. L. Lin, S. Y. Feng and N. Wang, Ann. Clin. Transl. Neurol., 2020, 7, 2010–2018 CrossRef CAS PubMed.
- A. Huefner, W. L. Kuan, S. L. Mason, S. Mahajan and R. A. Barker, Chem. Sci., 2020, 11, 525–533 RSC.
- C. Carlomagno, P. I. Banfi, A. Gualerzi, S. Picciolini, E. Volpato, M. Meloni, A. Lax, E. Colombo, N. Ticozzi, F. Verde, V. Silani and M. Bedoni, Sci. Rep., 2020, 10, 10175 CrossRef CAS PubMed.
- D. Ami, A. Duse, P. Mereghetti, F. Cozza, F. Ambrosio, E. Ponzini, R. Grandori, C. Lunetta, S. Tavazzi, F. Pezzoli and A. Natalello, Anal. Chem., 2021, 93, 16995–17002 CrossRef CAS PubMed.
- M. Plesia, O. A. Stevens, G. R. Lloyd, C. A. Kendall, I. Coldicott, A. J. Kennerley, G. Miller, P. J. Shaw, R. J. Mead, J. C. C. Day and J. J. P. Alix, ACS Chem. Neurosci., 2021, 12, 1768–1776 CrossRef CAS PubMed.
- J. J. P. Alix, M. Plesia, S. A. Hool, I. Coldicott, C. A. Kendall, P. J. Shaw, R. J. Mead and J. C. Day, Muscle Nerve, 2022, 66, 362–369 CrossRef CAS PubMed.
- J. J. P. Alix, M. Plesia, C. N. Schooling, A. P. Dudgeon, C. A. Kendall, V. Kadirkamanathan, C. J. McDermott, G. S. Gorman, R. W. Taylor, R. J. Mead, P. J. Shaw and J. C. Day, J. Raman Spectrosc., 2023, 54, 258–268 CrossRef CAS PubMed.
- J. J. P. Alix, M. Plesia, G. R. Lloyd, A. P. Dudgeon, C. A. Kendall, C. Hewamadduma, M. Hadjivassiliou, C. J. McDermott, G. S. Gorman, R. W. Taylor, P. J. Shaw and J. C. C. Day, Analyst, 2022, 147, 2533–2540 RSC.
- G. Devitt, A. Crisford, W. Rice, H. A. Weismiller, Z. Fan, C. Commins, B. T. Hyman, M. Margittai, S. Mahajan and A. Mudher, RSC Adv., 2021, 11, 8899–8915 RSC.
- N. Kuhar, S. Sil and S. Umapathy, Spectrochim. Acta, Part A, 2021, 258, 119712 CrossRef CAS PubMed.
- N. C. Maiti, M. M. Apetri, M. G. Zagorski, P. R. Carey and V. E. Anderson, J. Am. Chem. Soc., 2004, 126, 2399–2408 CrossRef CAS PubMed.
- J. T. Pelton and L. R. McLean, Anal. Biochem., 2000, 277, 167–176 CrossRef CAS PubMed.
- G. Devitt, W. Rice, A. Crisford, I. Nandhakumar, A. Mudher and S. Mahajan, ACS Chem. Neurosci., 2019, 10, 4593–4611 CrossRef CAS PubMed.
- M. Pezolet, M. Pigeon, D. Menard and J. P. Caille, Biophys. J., 1988, 53, 319–325 CrossRef CAS PubMed.
- A. M. Herrero, Crit. Rev. Food Sci. Nutr., 2008, 48, 512–523 CrossRef CAS PubMed.
- V. Askanas, W. K. Engel and A. Nogalska, Brain Pathol., 2009, 19, 493–506 CrossRef CAS PubMed.
- H. H. Goebel, Brain Pathol., 2009, 19, 480–482 CrossRef PubMed.
- S. M. Singh, N. Kongari, J. Cabello-Villegas and K. M. G. Mallela, Proc. Natl. Acad. Sci. U. S. A., 2010, 107, 15069–15074 CrossRef CAS PubMed.
- D. G. Georganopoulou, V. G. Moisiadis, F. A. Malik, A. Mohajer, T. M. Dashevsky, S. T. Wuu and C. K. Hu, Protein J., 2021, 40, 466–488 CrossRef CAS PubMed.
- T. Roscioli, E. J. Kamsteeg, K. Buysse, I. Maystadt, J. van Reeuwijk, C. van den Elzen, E. van Beusekom, M. Riemersma, R. Pfundt, L. E. Vissers, M. Schraders, U. Altunoglu, M. F. Buckley, H. G. Brunner, B. Grisart, H. Zhou, J. A. Veltman, C. Gilissen, G. M. Mancini, P. Delrée, M. A. Willemsen, D. P. Ramadža, D. Chitayat, C. Bennett, E. Sheridan, E. A. Peeters, G. M. Tan-Sindhunata, C. E. de Die-Smulders, K. Devriendt, H. Kayserili, O. A. El-Hashash, D. L. Stemple, D. J. Lefeber, Y. Y. Lin and H. van Bokhoven, Nat. Genet., 2012, 44, 581–585 CrossRef CAS PubMed.
- S. Gupta, S.-M. Kim, Y. Wang, A. R. Dinasarapu and S. Subramaniam, Hum. Mol. Genet., 2014, 23, 3772–3778 CrossRef CAS PubMed.
- J. C. Day and N. Stone, Appl. Spectrosc., 2013, 67, 349–354 CrossRef CAS PubMed.
- C. Kilkenny, W. J. Browne, I. C. Cuthill, M. Emerson and D. G. Altman, PLos Biol., 2010, 8, e1000412 CrossRef PubMed.
- J. W. McGreevy, C. H. Hakim, M. A. McIntosh and D. Duan, Dis. Models Mech., 2015, 8, 195–213 CrossRef CAS PubMed.
- R. J. Mead, E. J. Bennett, A. J. Kennerley, P. Sharp, C. Sunyach, P. Kasher, J. Berwick, B. Pettmann, G. Battaglia, M. Azzouz, A. Grierson and P. J. Shaw, PLoS One, 2011, 6, e23244 CrossRef CAS PubMed.
- M. D. Grounds, H. G. Radley, G. S. Lynch, K. Nagaraju and A. De Luca, Neurobiol. Dis., 2008, 31, 1–19 CrossRef CAS PubMed.
- M. Paraskevaidi, C. L. M. Morais, K. M. G. Lima, J. S. Snowden, J. A. Saxon, A. M. T. Richardson, M. Jones, D. M. A. Mann, D. Allsop, P. L. Martin-Hirsch and F. L. Martin, Proc. Natl. Acad. Sci. U. S. A., 2017, 114, E7929–E7938 CrossRef CAS PubMed.
- J. Hniopek, T. Bocklitz, M. Schmitt and J. Popp, Appl. Spectrosc., 2021, 75, 1043–1052 CrossRef CAS PubMed.
- S. M. Atif, S. Qazi and N. Gillis, Pattern Recognit. Lett., 2019, 122, 53–59 CrossRef.
- S. J. Carr, R. P. Zahedi, H. Lochmüller and A. Roos, Proteomics: Clin. Appl., 2018, 12, 1700071 Search PubMed.
- Q. Q. Gao and E. M. McNally, Compr. Physiol., 2015, 5, 1223–1239 Search PubMed.
- D. Duan, N. Goemans, S. i. Takeda, E. Mercuri and A. Aartsma-Rus, Nat. Rev. Dis. Primers, 2021, 7, 13 CrossRef PubMed.
- A. Nicolas, C. Raguénès-Nicol, R. Ben Yaou, S. Ameziane-Le Hir, A. Chéron, V. Vié, M. Claustres, F. Leturcq, O. Delalande, J.-F. Hubert, S. Tuffery-Giraud, E. Giudice, E. Le Rumeur and French Network of Clinical Reference Centres for Neuromuscular Diseases (CORNEMUS), Hum. Mol. Genet., 2014, 24, 1267–1279 CrossRef PubMed.
- R. Gautam, S. Vanga, A. Madan, N. Gayathri, U. Nongthomba and S. Umapathy, Anal. Chem., 2015, 87, 2187–2194 CrossRef CAS PubMed.
- J. P. Julien, Cell, 2001, 104, 581–591 CrossRef CAS PubMed.
- M. Maier, T. Welt, F. Wirth, F. Montrasio, D. Preisig, J. McAfoose, F. G. Vieira, L. Kulic, C. Späni, T. Stehle, S. Perrin, M. Weber, C. Hock, R. M. Nitsch and J. Grimm, Sci. Transl. Med., 2018, 10, eaah3924 CrossRef CAS PubMed.
- S. Salvany, A. Casanovas, L. Piedrafita, S. Gras, J. Calderó and J. E. Esquerda, Brain Pathol., 2022, 32, e13078 CrossRef CAS PubMed.
- J. C. Campos, L. M. Baehr, K. M. S. Gomes, L. R. G. Bechara, V. A. Voltarelli, L. H. M. Bozi, M. A. C. Ribeiro, N. D. Ferreira, J. B. N. Moreira, P. C. Brum, S. C. Bodine and J. C. B. Ferreira, Sci. Rep., 2018, 8, 11818 CrossRef PubMed.
- A. L. Blee, J. C. C. Day, P. E. J. Flewitt, A. Jeketo and D. Megson-Smith, J. Raman Spectrosc., 2021, 52, 1135–1147 CrossRef CAS.
- L. Mariño Pérez, F. S. Ielasi, L. M. Bessa, D. Maurin, J. Kragelj, M. Blackledge, N. Salvi, G. Bouvignies, A. Palencia and M. R. Jensen, Nature, 2022, 602, 695–700 CrossRef PubMed.
- H. Hong, S. Park, R. H. Jiménez, D. Rinehart and L. K. Tamm, J. Am. Chem. Soc., 2007, 129, 8320–8327 CrossRef CAS PubMed.
- C. M. Cardy and T. Potter, Rheumatology, 2007, 46, 1617–1618 CrossRef CAS PubMed.
- V. C. Constantinides, M. M. Papahatzaki, G. K. Papadimas, N. Karandreas, T. Zambelis, P. Kokotis and P. Manda, In Vivo, 2018, 32, 1647–1652 CrossRef PubMed.
- P. B. Moloney, S. Lefter, A. M. Ryan, M. Jansen, N. Bermingham and B. McNamara, Neurodiagn. J., 2021, 61, 86–94 CrossRef PubMed.
- L. ten Dam, A. J. van der Kooi, M. van Wattingen, R. J. de Haan and M. de Visser, Neurology, 2012, 79, 1716–1723 CrossRef PubMed.
- J. Verdú-Díaz, J. Alonso-Pérez, C. Nuñez-Peralta, G. Tasca, J. Vissing, V. Straub, R. Fernández-Torrón, J. Llauger, I. Illa and J. Díaz-Manera, Neurology, 2020, 94, e1094–e1102 CrossRef PubMed.
- E. Bugiardini, J. M. Morrow, S. Shah, C. L. Wood, D. S. Lynch, A. M. Pitmann, M. M. Reilly, H. Houlden, E. Matthews, M. Parton, M. G. Hanna, V. Straub and T. A. Yousry, Front. Neurol., 2018, 9, 456 CrossRef PubMed.
- C. H. Lai, G. Melli, Y. J. Chang, R. L. Skolasky, A. M. Corse, K. R. Wagner and D. R. Cornblath, Eur. J. Neurol., 2010, 17, 136–142 CrossRef PubMed.
- The UK Rare Diseases Framework, Department of Health & Social Care, UK Government, 2021.
- A. Goldstein and S. Rahman, J. Inherited Metab. Dis., 2021, 44, 343–357 CrossRef CAS PubMed.
|
This journal is © The Royal Society of Chemistry 2024 |