Deprivation based inequality in NOx emissions in England†
Received
6th March 2023
, Accepted 12th July 2023
First published on 28th July 2023
Abstract
Inequality in air pollution exposure is an established problem globally; this study evaluates inequality in NOx emissions in England. NOx is a class of air pollutants with a detrimental impact on human health. Emissions of NOx in 2019 from the major source sectors across England were linked to deprivation data from the English Indices of Multiple Deprivation (IMD) using Lower layer Super Output Areas (LSOAs). The median NOx emissions in the most deprived decile of LSOAs was 16 tonnes per km2 per year compared with 7.0 in the least deprived. A linear regression model to account for the whole dataset showed higher inequality, with emissions of 19 tonnes per km2 per year NOx for the most deprived decile and 7.3 for the least deprived. All major emission sources, such as transport, domestic combustion, point sources and industry showed deprivation-based inequality. Geographic classifications such as region, city and Rural Urban Classification were shown to have inequalities within them, and the differences between them were shown to drive national inequality. Inequalities in the distribution of NOx emissions persisted at all levels of population density. Less densely populated, typically rural, areas had lower absolute emissions, but the highest emissions-based inequality due to point sources disproportionately affecting more deprived areas.
Environmental significance
Inequality in exposure to air pollution is a globally recognised phenomena. This work examines deprivation-based inequality in NOx emissions in England by source sector. There is a deprivation-based inequality in emissions from all major polluting sectors including road transport, domestic combustion, off-road machinery, industry and point sources. Below national scale, inequalities in NOx emissions are observed in different regions and cities in England and across land-use classifications. This shows environmental inequality and may help guide policy decisions aimed at reducing it.
|
1. Introduction
Air pollution is an established danger to human health1–7 and is associated with a wide range of poor health outcomes. Furthermore, inequality in air pollution exposure based on demographic factors is a global issue, with evidence from Europe,8 Korea,9 Australia,10 Ethiopia,11 Latin America12 and China13 showing that disadvantaged populations have higher ambient concentrations of a range of air pollutants.
Within the UK, a variety of analyses of this inequality have been undertaken. NOx is the combined measure of nitric oxide (NO) and nitrogen dioxide (NO2) produced during high temperature combustion, either from nitrogen present in the fuel or nitrogen in the air which reacts due to the high temperatures. NOx concentrations have been shown to have a strong correlation with the percentage of households in poverty14 and area deprivation15 in England and Wales. The link between households in poverty and NOx concentrations was shown to have strengthened since a similar study from 2003 (ref. 16) which showed a link between ambient NOx concentrations and the percentage of households in poverty. Additionally, linking MOT data and car ownership14 showed that areas with a lower percentage of households in poverty were likely to be responsible for higher vehicle based emissions of NO2 despite their low residential concentrations, with the emissions occurring in poorer areas. London has also been investigated on it's own, with the London Travel Demand Survey used to compare residential and personal exposure to PM2.5 and NO2, derived through modelled concentrations17 with household income. This showed decreasing levels of air pollution at place of residence as income increased, but the inverse applied when calculating personal exposure, with the increased use of car, train and underground travel by higher income individuals being a driving factor in this trend.
The indices of multiple deprivation (IMD) is an index used by the UK Government to quantify deprivation in England. It ranks deprivation using a range of measures and then combines these into a unified metric and has previously been used to examine socioeconomic inequalities in ozone and particulate matter concentrations in England.18 The IMD score is determined by combining individual indices of deprivation with the following weightings:19 income deprivation (22.5%), employment deprivation (22.5%), education skills and training deprivation (13.5%), health deprivation and disability (13.5%), crime (9.3%), barriers to housing and services (9.3%), living environment deprivation (9.3%). Use of a combined metric allows for deprivation that wouldn't be visible through simply looking at income, to be observed.
A variety of methods have been used to determine ambient concentrations of NOx in previous inequality studies. The most commonly used are air pollution models, ground level monitoring stations and satellite observations. Air pollution models have a variety of forms, and land use regression (LUR) is the most prevalent for determining ground level NOx for this application. In an LUR model, factors such as the population density, amount of traffic and presence of industry in an area are used to estimate pollutant concentrations. This allows the coverage of a wider area than direct monitoring as the required information is more routinely collected and doesn't require specialist monitoring equipment. LUR modelling has been used in a range of inequality studies investigating both NOx concentrations and exposure.8,11,20 Satellite observations have also been used to investigate inequality in NOx concentrations and exposure.21,22 These use light scattering across different wavelengths to detect compounds such as NOx, although they are limited to areas observed by satellites with appropriate equipment, and are less accurate than ground level observations. Monitoring stations provide the most accurate measurement of ground level NOx, but their coverage across the UK is limited by the cost and practicality of deployment.9 As al these methods have advantages and drawbacks, some studies use a combination.4,10
Emissions inventories provide greater discrimination of specific sources and sectors contributing to pollution when compared to the use of ambient measurements and LUR models, which reflect the accumulated effects of all sources. Emissions estimates are generated for all locations within a given country, in this study at 1 km × 1 km resolution, and hence the geographic coverage and resolution of inventories generally far exceeds observational data at this time. This study takes a novel approach, using the UK National Atmospheric Emissions Inventory to examine how each emission sector contributes to inequality in NOx emissions. NOx is a relatively short-lived air pollutant with an atmospheric lifetime of the order of a few hours which contrasts with other pollutants such as PM 2.5, ozone or carbon monoxide that have lifetimes of many days to several months. A consequence of a short atmospheric lifetime is that the pollutant is concentrated in air close to its point of emission and as a result emissions and concentrations follow one another reasonably closely. Focusing on emissions allows a determination of the source sectors that give rise to local inequalities.
Due to the significant rate of change in total air pollution, and contributing sources, continually updated research and informed adaptation of the measures used to combat it is necessary. Examples of this include the frequent updating of the European emission standards for vehicles which have been through 7 stages since 1992, with the most recent standard, Euro 7 due to be implemented in 2025. There has been an almost complete elimination of coal fired power in the UK over the last 20 years, another example of a major change in emissions. This evolving landscape of emissions has the potential to change the prevailing drivers and locations of air pollution inequality. In this study we combine the latest UK emissions estimates of NOx (with sectoral resolution) with 2019 Index of Multiple Deprivation (IMD) metrics for the UK population. A range of factors not previously considered in the literature are also used. By using existing geographical distinctions such as county/unitary authority and city, the localisation of effects and their consistency across the country is investigated. The Rural Urban Classification (RUC) of areas is also utilised, enabling comparison between eight land-type categories that range from sparsely populated rural areas to urban population centres. Inequality within each RUC is also investigated. Finally, the links between population density, deprivation and NOx emissions are investigated.
2. Method
2.1. Information sources
Annual NOx emissions data for the year 2019 at a 1 km × 1 km resolution was obtained from the UK National Atmospheric Emissions Inventory (NAEI).23 Emissions data was used as it has national spatial coverage at a resolution that was appropriate for comparison with population deprivation data and it is differentiated by source, allowing exploration of drivers of any trends identified. The emissions within each grid box are calculated by multiplying an emission factor by activity data for each contributing source type. This is calculated by either an empirical (based on observed/reported emissions from the activity) or on a stochiometric (e.g., an amount of pollutant will be released per tonne of fuel burnt) basis. The total emission values for NOx have an uncertainty score of 2, which is classed as24 “Use [of] grids which is based on good, relevant, data at high level of definition but with maybe some minor shortcomings (e.g., road transport & population emissions)”. There is an uncertainty of 9.2% associated with the average NOx emissions in this data-set.25
Emissions data from 2019 was used as this was the most recent dataset available that was not impacted by the response to the Covid 19 pandemic, which lead to national and localised ‘lock-downs’ resulting in greatly reduced road transport26–28 and potential impacts on other source sectors. An analysis using 2020 data was fully consistent with all general trends and conclusions of this work, however, the absolute emissions, particularly from transport, were significantly lower.
Deprivation statistics from the 2019 census19 were used as this was the most up to date available and coincided with the year used for emissions. Emissions and population data were mapped on to geographic areas defined as Lower Layer Super Output Areas (LSOA). These are a geographic hierarchy designed to improve the reporting of small area statistics in England and Wales. Each LSOA is shaped so it represents approximately 1600 people, and they are typically between 0.1 and 2 km2 in area. There were 32844 LSOAs defined in the 2011 census areas, which are used here with 2019 deprivation data. LSOA geometries were obtained from the national archives;29 city30 and county31 designation and the Rural Urban Classification (RUC)32 for each LSOA were obtained from the office for national statistics.
NOx emissions were used an indicator for NO2 pollution to which people who live in a particular area may be exposed to. Unlike concentration measurements, emissions data is reported on a regular grid that covers the whole of England and can be directly related to deprivation data using LSOAs. Additionally, as many government policies focus on reducing emissions from particular source sectors, actions that seek to reduce inequalities in pollution exposure are largely focused on this measure of pollution.
2.2. Linking NOx emissions to population data
To investigate links between IMD and NOx emissions, the first step was to use a common feature that could be used to connect them. This was done by using pre-defined LSOA geographical areas associated with population census data24 that could be mapped on to the emissions inventory as they shared the same coordinate system (OSGB 1936), in turn allowing for the annual emissions within that LSOA to be calculated.
The programming language R33 was the chosen statistical analysis tool, drawing on a range of pre-existing packages that expand the relevant functionality. The package exactectractr34 was used to calculate the mean emissions for each LSOA. This was matched to the IMD decile and other associated statistics then passed through automated processing and the package ggplot2 (ref. 35) to produce graphs. A flow-chart of this process is included in the ESI: Fig. 1.†
The first step of data processing was reading the .asc files from the inventory as raster objects using the rast function from the package terra.36 The shapefile describing the LSOAs was then read as a series of vectors describing the shape of each LSOA using the function vect, from the package terra.36 The mean value of NOx emissions within each LSOA was calculated using the function exact_extract from the package exactextractr.34 Further data processing used a variety of functions from the tidyverse37 collection of packages. Graphs were created using ggplot2 (ref. 35) with extended functionality from ggpubr,38 given accessible colour scales using viridis39 and saved using the ragg40 package.
2.3. PRAWNS package
To make the analysis reproducible, and ensure the method is accessible for anyone wishing to perform similar analysis, the code used was compiled in a package for Pollution Raster Analysis With National Statistics (PRAWNS) which is now publicly available.41 This package contains vignettes explaining the uses of the existing functions and an explanation of the methods used to create each graph. The packages sf42 and stars43 were also used to enable flexibility in the input data format. Compilation of PRAWNS used roxygen2 (ref. 44) and devtools.45
2.4. Treatment of NA values
Cells containing NA values, present only in the energy production (8% missing values) and industrial combustion (0.3% missing values) categories were treated as not existing in this step, meaning that the value returned is the mean across all non NA area within each LSOA. This leads to a discrepancy between the total and apparent sum of sources in Fig. 8 due to the propagation of NA values present in industrial sources: when calculating the average emissions for each source, cells with NA values are ignored; whereas the total emissions value in the source data-set treats these values as 0 when calculating the sum of all emission sources. As altering the treatment has negligible effect on all other findings in this paper, NA values have been excluded.
2.5. Land-based classifications used
For analysis, four different land-based classifications were applied to the data. The first two were geographic: the city and county/unitary authority that each LSOA fell within. This allowed the localisation of inequalities to be evaluated at a level of granularity broadly comparable with regional and local government. The third grouping variable applied was the Rural Urban Classification (RUC)32 of each LSOA, as this allowed for a national comparison of inequalities effects between cities and rural areas or towns. The fourth classification was the physical area extent of the LSOA (km2), which serves as a proxy for population density as LSOAs are designed to have the same total population (LSOA size ∝ 1/population density). As population density is easier to understand intuitively, this term will be used in the discussion, but LSOA size is used in the graphs to remove the need for a conversion factor. Within the land-based classifications, links between deprivation and NOx were investigated. This involved use of linear models where appropriate, and the creation of graphs to better visualise the information gained.
2.6. Evaluation of linear models
A linear model was fitted to the values of NOx emission in every LSOA which are associated with each IMD decile. The relationship between IMD and NOx emissions is shown in Fig. 3 using a linear regression line derived from this model. However, this fails to convey the large variance from this line, so a box and whisker plot was added to show the distribution of values. Due to the large spread of emissions and the long tail in the distribution as discussed in Section 3.1, the standard method of plotting whiskers using a function of the interquartile range was rejected in favour of extending the top and bottom whiskers to the 90th and 10th percentiles respectively.
To assess whether the correlations observed at the national level were the result of the high number of observations, the dataset was randomly split into smaller chunks of 73 observations per chunk and regression analysis was performed on each of these. The resulting vector of p values was adjusted to account for false discovery rate using Benjamini and Hochberg's method46 to account for multiple testing effects. A significance rate of 0.05 was used. To ensure these results were not coincidence based on the seed used for the random assignment, 10 different seeds for the random number generator were used and the trends described were consistent across all of them.
Inequalities in the emissions concentrated in each geographic area are quantified here as the difference between the average absolute NOx emissions experienced in LSOAs in the highest decile for IMD and those experienced by LSOAs in the lowest decile by IMD. This difference is calculated in several different ways, firstly as a simple difference between the average emissions estimate for LSOAs in the highest decile by IMD and the lowest decile by IMD. This quantification may not fully represent the trend in emissions change moving from highest to lowest deprivation decile. So the inequality is also represented by fitting a linear model to the average emission estimates for each deprivation decile and using that to calculate the difference between the most and least deprived deciles, and using linear regression of the entire data set.
3. Results and discussion
3.1. NOx emissions in England
The distribution of mean annual NOx emissions by LSOA is right skewed, and there are two peaks in the frequency distribution (Fig. 1). This clear skewing of the data is important to consider in later analysis, as neither measure of central tendency (mean or median) is fully representative of the spread of the data. This has implications for later interpretation of the scale of inequality in the geographical distribution of emissions as the average values will be considerably lower than the highest emissions experienced.
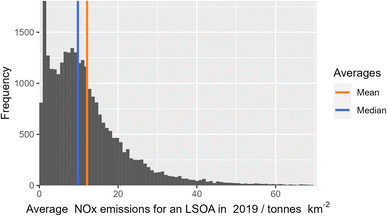 |
| Fig. 1 The frequency distribution of mean total annual NOx emissions across all LSOAs in England based on 2019 data from the UK National Atmospheric Emissions Inventory, cropped to the 99th percentile. | |
3.2. Inequalities in emissions sectors across England
3.2.1. Establishing links to IMD.
The mean and median emissions for each major NOx emission sector show the national scale connections between sectoral emissions on a per km2 basis and IMD when aggregating data from all LSOAs in England (Fig. 2). There are many LSOAs in London due to the high population density and the capital also experiences high levels of pollution emissions, meaning it has a significant influence on the national picture. The results were plotted both with and without London to demonstrate that the trend observed was not driven solely by the situation in London itself, and to isolate the effects of London from the rest of the data. With London included, the inequality slope goes from 22–9 tonnes NOx km−2 whereas without it the slope goes from 19–7 tonnes NOx km−2. This is a decrease in absolute inequality but an increase in relative inequality from 59% to 62%.
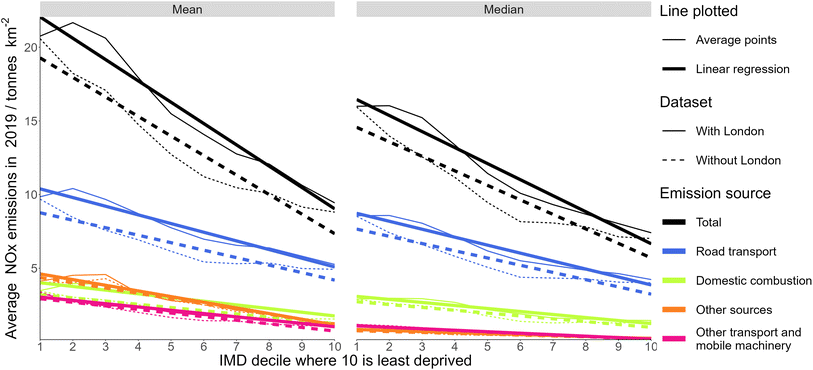 |
| Fig. 2 The mean and median emissions of NOx by LSOA across England for major source sectors, the average without London is also shown. The category “Other sources” is the combination of the source sectors: agricultural; energy production; industrial combustion; industrial combustion; solvents; natural; and point sources. | |
Using the total emissions estimates averaged across all LSOAs in England by deprivation decile, geographies representing the least deprived decile experienced on average 44% less median annual NOx emissions compared to the most deprived decile, 55% less when the difference is calculated using a linear fit through the median points, and 57% less when calculated using linear regression of the entire data set. This demonstrates that at a national level there is a high degree of inequality in the distribution of NOx emissions.
Almost all sectoral emission sources showed lower NOx emissions in less deprived deciles. This highlights that the inequality is driven by multiple different sources. The exceptions to this are agricultural and natural emissions, which are both very minor emission sectors in terms of tonnes per year. Each source sector with annual emissions above 0.1 tonnes per km2 has a reduction of at least 25% in emissions magnitude on a regression line plotted when going from the most to least deprived decile.
Since the emissions are skewed, the LSOAs with the highest 10% of emissions in each decile were compared (ESI: Fig. 2†). This showed more extreme inequality, with a percentage difference of 60%, and an absolute difference of 37 tonnes per km2 when looking at the values for total emissions on a regression line at the most and least deprived deciles, more than triple the absolute difference when looking at the whole population. There was a higher increase in the mean compared to the median for more deprived deciles, showing that more deprived areas experience more high emitting outliers. Inequality associated with road transport decreased when compared with the entire population. The absolute inequality in all other major sources increased when compared with the entire population. This effect grew larger if a lower percentage of the highest emissions (e.g., the highest 5% in each decile) was selected, with inequalities associated with all sources but road transport becoming more extreme.
Many previous studies on pollution inequalities have focused on the role played by proximity to road transport emissions;14,20,26,47–49 while this is well justified as this is currently the largest single NOx source for most areas, our analysis shows that other sources also contribute to inequalities in emissions of NOx. This has important implications for future inequality. While NOx emissions from road transport are likely to reduce over time assuming, electric vehicles become a larger proportion of the vehicle fleet, inequalities will continue to occur, driven by the uneven distribution of emissions from sectors such as domestic combustion and industrial sources. Future policies for domestic heating and industry will need to consider whether they have the potential to widen those inequalities, or to reduce them.
3.2.2. Strength of the relationship between IMD and emissions.
In the underlying data each IMD decile is associated with many LSOAs which have significant differences in NOx emission. This results in a high root mean squared error (RMSE) of 18 tonnes per km2, which reduces to 7.2 tonnes per km2 when points above the 95th percentile are removed from the RMSE calculation as compensation for the high skew in the data. Whilst this value is still of the same order of magnitude as all points on the line, it is lower than the difference between the maximum and minimum values. Further evaluating the error, the residuals were plotted on a histogram, showing a skewed Gaussian distribution of residual errors (ESI: Fig. 3†), the skew reflects that of the data, and the Gaussian distribution validates the use of a linear model. The exceptions to this were agriculture, waste treatment and disposal, and energy production. These sectors only formed a very small portion of the NOx total this was not investigated further. Plotting the residuals against IMD decile also showed nothing indicating that a linear model was inappropriate (ESI: Fig. 4†). R2 values for the fitted regression lines are low (R2t; 0.1), as a model that predicts a particular NOx emission for each IMD cannot explain the variability seen in the real data; R2 is therefore a poor statistic to use in this situation.50–54
To assess whether the correlation was simply the result of the high volume of data, regression analysis was performed on random samples (n = 73) and p-values were calculated, accounting for false discovery rate using FDR (ESI: Fig. 5†). For the sectors energy production, industrial production and point sources no samples had a significant link, reflecting that the linear correlation observed in these sectors is weak. For total emissions, and the sectors road transport, domestic combustion, waste treatment and disposal, and solvents, the majority of p-values were below 0.05. This shows that even on a small sample of the data, using a straight line with a non-zero gradient is a significantly better fit than a flat line with a gradient of 0. The sectors industrial production and other transport and mobile machinery had some p-values below 0.05, and skewed towards lower p values. Doubling the sample size resulted in the majority of p-values for these sources falling below 0.05, showing that a linear model is somewhat appropriate, reflected in how the linear regression line for these sources, whilst not truly descriptive, shows reasonable alignment with the 75th percentile of emissions. The high RMSE and low r2 values are to be expected for data summarising the wide variety of regions in England. However, the Gaussian distribution of residuals and consistently low p-values show that a linear model is valid and not the result of coincidence.
Fig. 3 shows the difference between the median and mean emissions for each source sector. The mean is higher than the median for all source sectors except waste treatment and disposal, which reflects that there are more extreme cases above the median than below it. Emissions sectors where the median and mean have a similar gradient but with an offset show that these high emission cases are equally distributed indicating that there is a similar chance of high emissions above the average for each IMD decile.
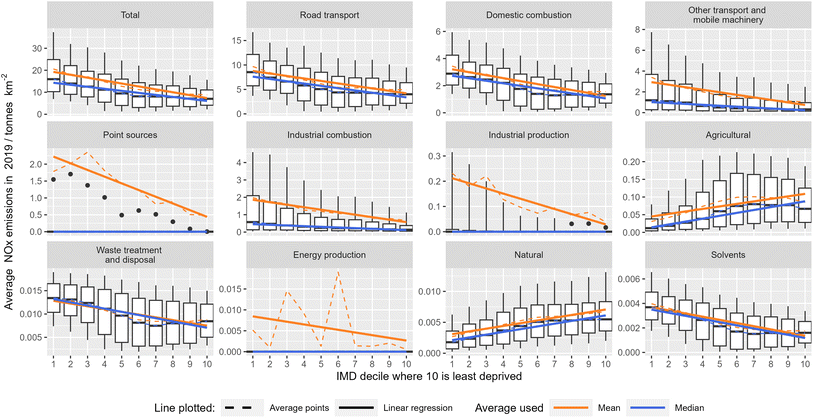 |
| Fig. 3 NOx emissions for all source sectors except offshore emissions for LSOAs in England with London excluded. The top and bottom whiskers extend to the 90th and 10th percentiles respectively, and dots represent the 90th percentile in cases where the 75th percentile is equal to 0. | |
Source sectors such as industrial combustion, industrial production and other transport and mobile machinery show a noticeable increase in gradient when using a linear regression line rather than quantile regression through the median emissions. This indicates that the high emission cases not reflected in the median are more frequent or of higher magnitude for the more deprived deciles. This is also reflected in the position of the 75th and 90th percentiles for these emission sectors, which decrease at a faster rate than the median, showing that the more deprived deciles skew towards higher NOx emissions. The values quoted so far have been for average values, but this analysis indicates that the difference between the highest emissions experienced by those in lower IMD deciles and the lowest emissions in the higher deciles would be far greater. By only considering average values those who experience the greatest inequality are effectively excluded from the analysis.
Emission sectors where the median line tends to zero, such as point sources, energy production and industrial production reflect that these are sources where more than half of LSOAs have no associated emissions, resulting in a median value of 0. In the more extreme cases, such as point sources and industrial production, the 75th percentile is also 0 for some IMD deciles. However, in these cases the 90th percentile being at a non 0 value and of the same order of magnitude as the regression line for the entire dataset shows that the mean is still driven by an appreciable fraction of the data rather than a tiny number of edge cases so is still a relevant metric. Energy production is an exception to this as the mean values are much larger than the 90th percentile, indicating that the observed trend is driven by less than 10% of the data. This means that to remedy inequality in energy production emissions it would be necessary to look at the section of the population affected by emissions from energy production and tailor measures to them rather than addressing the population as a whole.
3.3. Regional and city-based trends
The inequality gradient of NOx emissions against IMD decile produced by a linear model for each county or Unitary Authority (UA) was calculated. These values were plotted on a histogram (ESI: Fig. 6†). The p-values for each county/UA were calculated to determine if a straight line with a gradient of 0 was more appropriate than a straight line of best fit with the associated gradient. A p-value of less than 0.05 was treated as meaning the points had sufficient linear correlation for the gradient to provide information on deprivation based inequality in the area. Overall, 66% of all counties/Unitary Authorities had both negative inequality gradient and a p-value of less than 0.05 indicating a significant correlation between emissions and deprivation for these areas. For the remaining regions with a p-value of above 0.05 this does not indicate that there is no inequality, but rather that a straight line is inappropriate to describe any observed pattern and individual assessment is required to determine its extent, nature and cause. This shows that at least two in three counties and unitary authorities in England have significant deprivation-based inequality in NOx emissions, and the true number is likely higher.
Having established inequality within many regions, the next step was to determine if there is inequality between regions. Fig. 4 shows the average total NOx emissions for each county and unitary authority, as well as the average IMD decile for LSOAs contained within it. This reveals a general downwards trend, meaning that more deprived regions are more likely to have higher emissions. It also shows that there is significant variation between regions and looking at them individually (ESI: Fig. 7†) further emphasises this.
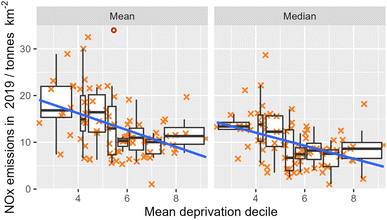 |
| Fig. 4 Scatter plot linking the average total NOx emission and mean IMD decile for all counties and unitary authorities in England. Counties and unitary authorities within London are omitted. The blue line is the line of best fit for linear regression using all points plotted. Each cross in orange represents a single county or unitary authority. The boxplot is split into 8 bins containing equal numbers of counties/unitary authorities and uses standard procedures for plotting the whiskers. The circular point in pink has an emission of 42 tonnes per km2. | |
Cities were investigated using the same procedures as used for regions. Plotting the average deprivation for each city against its emissions (ESI: Fig. 8†) showed similar results to counties and unitary authorities, with more deprived cities tending towards higher emissions. The inequality gradient linking NOx emissions and IMD decile was calculated (ESI: Fig. 9†) showing that a lower proportion of cities showed statistically significant gradients to counties and unitary authorities. This was likely because the smaller size of cities leads to a greater variation in their emissions (ESI: Fig. 10†). Many cities have large peaks in NOx emissions centred between the 2nd and 4th decile, and as there is a good chance these are centred on one geographic area, this suggests that targeted measures could be effective at addressing emissions inequality within individual cities.
3.4. Is inequality driven by the difference between rural and urban areas?
Rural and urban areas typically experience different levels of pollution55–57 and often have different levels of deprivation on a national level, although whether rural or urban areas are more deprived varies by country.58 Separating LSOAs by the Rural Urban Classification (RUC) allowed for the exploration of the contribution to national inequalities from inherent differences between rural and urban areas irrespective of IMD. Total NOx emissions were plotted against deprivation (Fig. 5) for each RUC, allowing a comparison between them and the observation of inequalities within each RUC. The deprivation and number of LSOAs in each RUC was determined (ESI: Fig. 11†), and this was used to determine how the differences between RUCs contribute to national inequality.
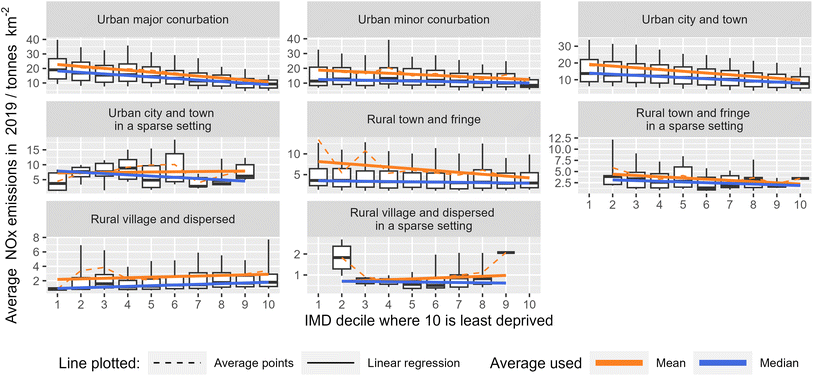 |
| Fig. 5 The relationship between IMD decile and average NOx emissions for each rural urban classification (RUC) in England. | |
The results showed that all of the classifications that represent the majority of the population have a negative inequality gradient (Fig. 5), showing that more deprived areas are likely to experience higher levels of NOx emissions. For the classifications urban city and town in a sparse setting; rural village and dispersed; and rural village and dispersed in a sparse setting where the gradient is not negative there is no linear relationship between deprivation decile and NOx emissions.
The contrast between the RUCs urban major conurbation and urban minor conurbation, which have high emissions and substantial areas of high deprivation, and rural town and fringe/rural village and dispersed, which have low emissions and deprivation, contributes to national inequality. RUCs in a sparse setting run counter to this, with low emissions and a tendency towards moderate (deciles 3–5) levels of deprivation, however they account for a small portion of the population so aren't major contributors to the national picture. Urban city and town on the other hand contains a large number of LSOAs spread fairly evenly across deprivation deciles and features a peak in population at the least deprived decile. However, as this is a single peak rather than a skew the effect on the national picture is muted. Overall, the difference between rural and urban areas in terms of deprivation and emissions is shown as a driver for national inequality.
3.5. London
London shows trends similar to the rest of England but at higher base levels of NOx emissions (Fig. 6). The median values for the rest of England fall below the 10th percentile of London's emissions for the majority of deprivation deciles, and the mean values fall below the 1st quartile in most cases. It had higher absolute inequality than the rest of England, with a difference of 13 tonnes per year between the most and least deprived deciles on the regression line, as opposed to 12 tonnes per year for the rest of England. However, due to the higher average NOx emissions this resulted in relative inequality of 38%, lower than the 62% in the rest of England. The gradient being identical for the mean and median values within London shows that the distribution of outliers is largely consistent across all deciles within London. This contrasts with the rest of England, where the median regression line has a steeper gradient than the mean. This demonstrates that high NOx emission outliers are more extreme for lower deciles in the rest of England, reflecting that large NOx point sources are less likely to be located in London.
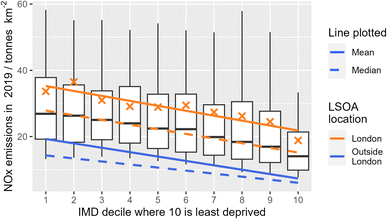 |
| Fig. 6 The mean and median total emissions of NOx by LSOA for London and the rest of England with London excluded. The boxplot represents LSOAs within London, and whiskers are calculated as the 10th and 90th percentile. The orange crosses represent the mean emissions for each deprivation decile. | |
3.6. The effect of population density on emission inequality
Population density has been shown to influence levels of air pollution,59,60 and this is also true for NOx emissions (ESI: Fig. 12†). Since more deprived areas are more likely to have a higher population density (ESI: Fig. 13†), this drives inequality. To determine if inequality is driven only by population density, LSOAs were grouped by size, and the trend in total emissions by deprivation decile plotted (Fig. 7). This shows that inequalities persisted across all sizes of LSOA, and that there was a greater deprivation-based emission inequality between larger LSOAs than between smaller ones. Most pronounced is the drop in emissions from the 3rd to 4th decile for the largest 20% of LSOAs, which was significant enough to warrant further investigation. This revealed that point sources produced the bulk of emissions for the more deprived deciles but had negligible effect on any beyond the 4th decile in the largest LSOAs (ESI: Fig. 14†). Additionally, the most deprived LSOAs between 0.42 and 2.9 km2 had higher average total emissions than their equivalents in smaller, more densely populated LSOAs. This shows that population density, and therefore activities associated with permanent residents of the area, is not the sole driving factor behind NOx emissions.
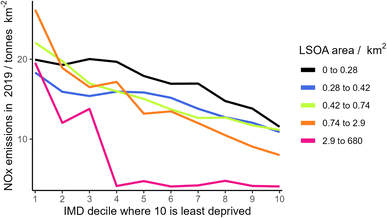 |
| Fig. 7 Inequality in mean NOx emissions for different sizes of LSOA. The division was made purely on the expanse of each LSOA so each size bracket does not contain an even distribution of deprivation deciles. | |
To better understand why emissions were relatively static regardless of population density for the most deprived LSOAs, emission sources were aggregated into road transport; industrial sources; and other sources, then plotted against LSOA area, with only the most deprived 20% of LSOAs selected (Fig. 8). The emissions from road transport, and sources associated with population density (listed as other sources) remained largely constant for the smallest 70% of LSOAs before dropping, which is to be expected due to the link between LSOA size and population density. In contrast to this, emissions associated with industry began to increase after the smallest 40% of LSOAs. This shows that industry is responsible for the extreme inequality in NOx emissions for the largest LSOAs shown in Fig. 8.
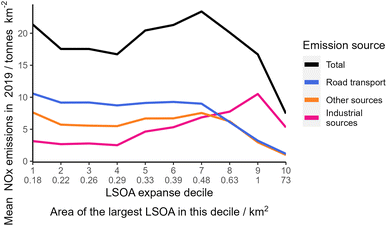 |
| Fig. 8 The change in contributions to total NOx emissions for the most deprived 20% of LSOAs. Other transport is the combination of natural sources; agricultural sources; other transport and mobile machinery; and domestic combustion. Industrial sources is a combination of solvents; energy production; waste treatment and disposal; industrial production; industrial combustion; and point sources. | |
Whilst road transport is the single largest source of deprivation-based inequality in NOx emissions on a national level, industrial sources drive extreme levels of inequality in less densely populated areas.
3.7. Limitations
Whilst the short lifetime of NOx leads to a strong connection between local emissions and local ambient concentrations, they aren't equivalent, there will be some movement of NOx between LSOA influencing the gradients observed. Personal exposure is also not represented, for example individuals from higher IMD deciles may travel through or work in LSOA with high emissions and a low IMD score. The inverse may also be true, but accounting for transport and workpattern, rather than simply place of residence, whilst possible17,61 is challenging to achieve for the population as a whole since at a national level no databases are compiled linking factors such as place of work or education to transport method and place of residence. The elevation of some point sources using chimneys or stacks changes how their emissions affect the local population, and this is not considered.
Air quality is a component of the IMD, although it is small, as demonstrated below. The living environment domain forms 9.3% of the IMD, and a third of this is allocated to the outdoor environment, which is split in half and attributed to road traffic accidents and air quality. NO2 is one of four pollutants with equal weighting included in the air quality metric in the IMD. This leads to NO2 forming only 0.4% of the total IMD ranking, which, when combined with the loss of resolution inherent to sorting by deciles, means that the impact of NO2 in the calculation of the IMD is insufficient to drive any appreciable numeric circularity.
4. Conclusions
NOx emissions in England have a significant association with the IMD composite measure of deprivation, with more deprived areas experiencing higher average NOx emissions. This persists across all emission sectors with the exceptions of agricultural and natural emissions, which are both small; and offshore emissions which were excluded as irrelevant to land based populations. The observations are consistent with analysis of inequality in NOx concentrations in England,14,15 and with international findings about socioeconomic inequality in NOx exposure. However, the use of emissions instead of concentrations, and the high resolution of the geographic groupings compared mean there are no comparisons that can be made to previous literature.
Regional inequalities exist, with more deprived regions having greater overall NOx emissions, but inequalities also exist within regions. Two thirds of the counties and unitary authorities in England show significant deprivation-based inequality in NOx emissions. While there is a high degree of variability in the trends seen for individual cities, the same overall patterns are observed: emissions increase with increasing deprivation, and this inequality exists both within individual cities and between them.
The differences between average emissions for LSOAs within each rural urban classification (RUC) were identified as a driver of national inequality, as RUCs with more deprived LSOAs tended to have higher emissions and vice versa. Each RUC that was not sparsely populated contained deprivation-based inequality in NOx emissions. This shows that whilst the difference between cities and the countryside does drive inequality, those in more deprived areas will likely have worse air quality regardless of whether they are surrounded by trees or traffic lights.
Population density was shown to contribute to inequality as less densely populated areas were likely to be less deprived and have lower average NOx emissions. However, inequality was shown to persist across different population densities, and became larger in less densely populated areas. This was a result of point sources and industry having disproportionate impact on the most deprived large area LSOAs. Overall, this study demonstrates that deprivation-based inequality in NOx emissions is widely experienced and exists both within and between most geographical groupings of data. It also shows that factors beyond road transport, which is often the focus of air pollution inequality studies, are important drivers of NOx air pollution inequality. Many non-road emission sectors cause inequality in the distribution of NOx emissions, and in specific situations, like point sources in rural areas, this inequality is extreme. The analysis speaks to the need for city and regionally appropriate emissions reduction strategies if the reduction in inequalities is a major policy motivation. It is important to note that the trend and scale of inequality are determined using average values, and that higher inequality is observed between the highest emitting areas in each deprivation decile.
Conflicts of interest
There are no conflicts of interest to declare.
Acknowledgements
This work was funded by the Department For Environment, Food and Rural Affairs (Defra). The authors would like to thank Dr Katherine Manfred and Dr Jesmond Zahra for their support during the research process. This research was made possible by publicly available emissions data from the UK National Atmospheric Emissions Inventory and census data from the UK National Archives.
References
- S. S. Lim, T. Vos, A. D. Flaxman, G. Danaei, K. Shibuya, H. Adair-Rohani, M. Amann, H. R. Anderson, K. G. Andrews, M. Aryee, C. Atkinson, L. J. Bacchus, A. N. Bahalim, K. Balakrishnan, J. Balmes, S. Barker-Collo, A. Baxter, M. L. Bell, J. D. Blore, F. Blyth, C. Bonner, G. Borges, R. Bourne, M. Boussinesq, M. Brauer, P. Brooks, N. G. Bruce, B. Brunekreef, C. Bryan-Hancock, C. Bucello, R. Buchbinder, F. Bull, R. T. Burnett, T. E. Byers, B. Calabria, J. Carapetis, E. Carnahan, Z. Chafe, F. Charlson, H. Chen, J. S. Chen, A. T.-A. Cheng, J. C. Child, A. Cohen, K. E. Colson, B. C. Cowie, S. Darby, S. Darling, A. Davis, L. Degenhardt, F. Dentener, D. C. Des Jarlais, K. Devries, M. Dherani, E. L. Ding, E. R. Dorsey, T. Driscoll, K. Edmond, S. E. Ali, R. E. Engell, P. J. Erwin, S. Fahimi, G. Falder, F. Farzadfar, A. Ferrari, M. M. Finucane, S. Flaxman, F. G. R. Fowkes, G. Freedman, M. K. Freeman, E. Gakidou, S. Ghosh, E. Giovannucci, G. Gmel, K. Graham, R. Grainger, B. Grant, D. Gunnell, H. R. Gutierrez, W. Hall, H. W. Hoek, A. Hogan, H. D. Hosgood, D. Hoy, H. Hu, B. J. Hubbell, S. J. Hutchings, S. E. Ibeanusi, G. L. Jacklyn, R. Jasrasaria, J. B. Jonas, H. Kan, J. A. Kanis, N. Kassebaum, N. Kawakami, Y.-H. Khang, S. Khatibzadeh, J.-P. Khoo, C. Kok, F. Laden, R. Lalloo, Q. Lan, T. Lathlean, J. L. Leasher, J. Leigh, Y. Li, J. K. Lin, S. E. Lipshultz, S. London, R. Lozano, Y. Lu, J. Mak, R. Malekzadeh, L. Mallinger, W. Marcenes, L. March, R. Marks, R. Martin, P. McGale, J. McGrath, S. Mehta, G. A. Mensah, T. R. Merriman, R. Micha, C. Michaud, V. Mishra, K. M. Hanafiah, A. A. Mokdad, L. Morawska, D. Mozaffarian, T. Murphy, M. Naghavi, B. Neal, P. K. Nelson, J. Miquel Nolla, R. Norman, C. Olives, S. B. Omer, J. Orchard, R. Osborne, B. Ostro, A. Page, K. D. Pandey, C. D. H. Parry, E. Passmore, J. Patra, N. Pearce, P. M. Pelizzari, M. Petzold, M. R. Phillips, D. Pope, C. A. Pope, J. Powles, M. Rao, H. Razavi, E. A. Rehfuess, J. T. Rehm, B. Ritz, F. P. Rivara, T. Roberts, C. Robinson, J. A. Rodriguez-Portales, I. Romieu, R. Room, L. C. Rosenfeld, A. Roy, L. Rushton, J. A. Salomon, U. Sampson, L. Sanchez-Riera, E. Sanman, A. Sapkota, S. Seedat, P. Shi, K. Shield, R. Shivakoti, G. M. Singh, D. A. Sleet, E. Smith, K. R. Smith, N. J. C. Stapelberg, K. Steenland, H. Stoeckl, L. J. Stovner, K. Straif, L. Straney, G. D. Thurston, J. H. Tran, R. Van Dingenen, A. van Donkelaar, J. L. Veerman, L. Vijayakumar, R. Weintraub, M. M. Weissman, R. A. White, H. Whiteford, S. T. Wiersma, J. D. Wilkinson, H. C. Williams, W. Williams, N. Wilson, A. D. Woolf, P. Yip, J. M. Zielinski, A. D. Lopez, C. J. L. Murray and M. Ezzati, A Comparative Risk Assessment of Burden of Disease and Injury Attributable to 67 Risk Factors and Risk Factor Clusters in 21 Regions, 1990-2010: A Systematic Analysis for the Global Burden of Disease Study 2010, Lancet, 2012, 380, 2224–2260 CrossRef.
- F. Chen and Z. Chen, Cost of Economic Growth: Air Pollution and Health Expenditure, Sci. Total Environ., 2021, 755, 142543 CrossRef CAS.
- R. Beelen, O. Raaschou-Nielsen, M. Stafoggia, Z. J. Andersen, G. Weinmayr, B. Hoffmann, K. Wolf, E. Samoli, P. Fischer, M. Nieuwenhuijsen, P. Vineis, W. W. Xun, K. Katsouyanni, K. Dimakopoulou, A. Oudin, B. Forsberg, L. Modig, A. S. Havulinna, T. Lanki, A. Turunen, B. Oftedal, W. Nystad, P. Nafstad, U. De Faire, N. L. Pedersen, C.-G. Ostenson, L. Fratiglioni, J. Penell, M. Korek, G. Pershagen, K. T. Eriksen, K. Overvad, T. Ellermann, M. Eeftens, P. H. Peeters, K. Meliefste, M. Wang, B. Bueno-de-Mesquita, D. Sugiri, U. Kraemer, J. Heinrich, K. de Hoogh, T. Key, A. Peters, R. Hampel, H. Concin, G. Nagel, A. Ineichen, E. Schaffner, N. Probst-Hensch, N. Kuenzli, C. Schindler, T. Schikowski, M. Adam, H. Phuleria, A. Vilier, F. Clavel-Chapelon, C. Declercq, S. Grioni, V. Krogh, M.-Y. Tsai, F. Ricceri, C. Sacerdote, C. Galassi, E. Migliore, A. Ranzi, G. Cesaroni, C. Badaloni, F. Forastiere, I. Tamayo, P. Amiano, M. Dorronsoro, M. Katsoulis, A. Trichopoulou, B. Brunekreef and G. Hoek, Effects of Long- Term Exposure to Air Pollution on Natural-Cause Mortality: An Analysis of 22 European Cohorts within the Multicentre ESCAPE Project, Lancet, 2014, 383, 785–795 CrossRef CAS.
- A. J. Cohen, M. Brauer, R. Burnett, H. R. Anderson, J. Frostad, K. Estep, K. Balakrishnan, B. Brunekreef, L. Dandona, R. Dandona, V. Feigin, G. Freedman, B. Hubbell, A. Jobling, H. Kan, L. Knibbs, Y. Liu, R. Martin, L. Morawska, C. A. Pope, H. Shin, K. Straif, G. Shaddick, M. Thomas, R. van Dingenen, A. van Donkelaar, T. Vos, C. J. L. Murray and M. H. Forouzanfar, Estimates and 25-Year Trends of the Global Burden of Disease Attributable to Ambient Air Pollution: An Analysis of Data from the Global Burden of Diseases Study 2015, Lancet, 2017, 389, 1907–1918 CrossRef.
- M. Kampa and E. Castanas, Human Health Effects of Air Pollution, Environ. Pollut., 2008, 151, 362–367 CrossRef CAS PubMed.
- C. A. Pope, R. T. Burnett, M. J. Thun, E. E. Calle, D. Krewski, K. Ito and G. D. Thurston, Lung Cancer, Cardiopulmonary Mortality, and Long-Term Exposure to Fine Particulate Air Pollution, JAMA, J. Am. Med. Assoc., 2002, 287, 1132–1141 CrossRef CAS PubMed.
- S. Khomenko, M. Cirach, E. Pereira-Barboza, N. Mueller, J. Barrera-Gomez, D. Rojas-Rueda, K. de Hoogh, G. Hoek and M. Nieuwen huijsen, Premature Mortality Due to Air Pollution in European Cities: A Health Impact Assessment, Lancet Planetary Health, 2021, 5, E121–E134 CrossRef.
- E. Samoli, A. Stergiopoulou, P. Santana, S. Rodopoulou, C. Mitsakou, C. Dimitroulopoulou, M. Bauwelinck, K. de Hoogh, C. Costa, M. Marí-Dell’Olmo, D. Corman, S. Vardoulakis and K. Katsouyanni, Spatial Variability in Air Pollution Exposure in Relation to Socioeconomic Indicators in Nine European Metropolitan Areas: A Study on Environmental Inequality, Environ. Pollut., 2019, 249, 345–353 CrossRef CAS PubMed.
- P. Choi and I. Min, Measuring Environmental Inequality from Air Pollution and Health Conditions, Appl. Econ. Lett., 2020, 27, 615–619 CrossRef.
- L. D. Knibbs and A. G. Barnett, Assessing Environmental Inequalities in Ambient Air Pollution across Urban Australia, Spat. Spatiotemporal Epidemiol., 2015, 13, 1–6 CrossRef PubMed ISSN: 1877-5845. https://www.webofscience.com/api/gateway?GWVersion=.
- E. Flanagan, K. Mattisson, J. Walles, A. Abera, A. Eriksson, F. Balidemaj, A. Oudin, C. Isaxon and E. Malmqvist, Air Pollution and Urban Green Space: Evidence of Environmental Injustice in Adama, Ethiopia, Front. Sustain. Cities, 2021, 3, 728384 CrossRef.
- N. Gouveia, A. D. Slovic, C. M. Kanai and L. Soriano, Air Pollution and Environmental Justice in Latin America: Where Are We and How Can We Move Forward?, Curr. Environ. Health Rep., 2022, 9, 152–164 CrossRef PubMed.
- K. Jiao, M. Xu and M. Liu, Health Status and Air Pollution Related Socioeconomic Concerns in Urban China, Int. J. Equity Health, 2018, 17, 18 CrossRef PubMed.
- J. H. Barnes, T. J. Chatterton and J. W. S. Longhurst, Emissions vs. Exposure: Increasing Injustice from Road Traffic-Related Air Pollution in the United Kingdom, Transp. Res. D: Transp. Environ., 2019, 73, 56–66 CrossRef.
- D. Fecht, P. Fischer, L. Fortunato, G. Hoek, K. de Hoogh, M. Marra, H. Kruize, D. Vienneau, R. Beelen and A. Hansell, Associations between Air Pollution and Socioeconomic Characteristics, Ethnicity and Age Profile of Neighbourhoods in England and the Netherlands, Environ. Pollut., 2015, 198, 201–210 CrossRef CAS PubMed.
- G. Mitchell and D. Dorling, An Environmental Justice Analysis of British Air Quality, Environ. Plan. A., 2003, 35, 909–929 CrossRef.
- C. Tonne, C. Mila, D. Fecht, M. Alvarez, J. Gulliver, J. Smith, S. Beevers, H. R. Anderson and F. Kelly, Socioeconomic and Ethnic Inequalities in Exposure to Air and Noise Pollution in London, Environ. Int., 2018, 115, 170–179 CrossRef CAS PubMed.
- A. Milojevic, C. L. Niedzwiedz, J. Pearce, J. Milner, I. A. MacKenzie, R. M. Doherty and P. Wilkinson, Socioeconomic and Urban-Rural Differentials in Exposure to Air Pollution and Mortality Burden in England, Environ. Health, 2017, 16, 104 CrossRef PubMed . ISSN: 1476-069X. https://ehjournal.biomedcentral.com/articles/10.1186/s12940-017-0314-5.
-
English Indices of Deprivation 2019 GOV.UK, 2022, https://www.gov.uk/government/statistics/english-indices-of-deprivation-2019.
- C. Tonne, S. Beevers, B. Armstrong, F. Kelly and P. Wilkinson, Air Pollution and Mortality Benefits of the London Congestion Charge: Spatial and Socioeconomic Inequalities, Occup Environ Med., 2008, 65, 620–627 CrossRef CAS PubMed.
- H.-J. Lee, S.-W. Kim, J. Brioude, O. R. Cooper, G. J. Frost, C.-H. Kim, R. J. Park, M. Trainer and J.-H. Woo, Transport of NOx in East Asia Identified by Satellite and in Situ Measurements and Lagrangian Particle Dispersion Model Simulations, J. Geophys. Res.: Atmos., 2014, 119, 2574–2596 CrossRef CAS.
- S. Kemball-Cook, G. Yarwood, J. Johnson, B. Dornblaser and M. Estes, Evaluating NOx Emission Inventories for Regulatory Air Quality Modeling Using Satellite and Air Quality Model Data, Atmos. Environ., 2015, 117, 1–8 CrossRef CAS.
-
Inventory, N. A. E. Emissions of Nitrogen Oxides in 2019, 2022, https://naei.beis.gov.uk/data/mapping-archive.
-
I. Tsagatakis, J. Richardson, C. Evangelides, M. Pizzolato, B. Pearson, N. Passant, M. Pommier and A. Otto, UK Spatial Emissions Methodology, 2022 Search PubMed.
-
L. Jones, L. Garland, C. Szanto and K. KingAir Pollutant Inventories for England, Scotland, Wales, and Northern Ireland: 2005–2019, 2019 Search PubMed.
- L. Brown, J. Barnes and E. Hayes, Traffic-Related Air Pollution Reduction at UK Schools during the Covid-19 Lockdown, Sci. Total Environ., 2021, 780, 146651 CrossRef CAS PubMed.
- W. Hicks, S. Beevers, A. H. Tremper, G. Stewart, M. Priestman, F. J. Kelly, M. Lanoiselle, D. Lowry and D. C. Green, Quantification of Non-Exhaust Particulate Matter Traffic Emissions and the Impact of COVID-19 Lockdown at London Marylebone Road, Atmosphere, 2021, 12, 190 CrossRef CAS.
- K. Ropkins and J. E. Tate, Early Observations on the Impact of the COVID-19 Lockdown on Air Quality Trends across the UK, Sci. Total Environ., 2021, 754, 142374 CrossRef CAS.
-
Office for National Statistics. 2011, Boundaries, 2022, https://webarchive.nationalarchives.gov.uk/ukgwa/20160110200248, http://www.ons.gov.uk/ons/guide-method/geography/products/census/spatial/2011/index.html Search PubMed.
-
Office for National Statistics. Lower Layer Super Output Area (2011) To Major Towns and Cities (December 2015), Lookup in England and Wales, 2022, https://geoportal.statistics.gov.uk/datasets/dc0b24da0880417abc979c705bce3fde_0/explore Search PubMed.
-
Office for National Statistics, Local Authority District to County (April 2019) Lookup in England, 2019, https://geoportal.statistics.gov.uk/datasets/ons::local-authority-district-to-county-april-2019-lookup-in-england/about Search PubMed.
-
Office for National Statistics, Rural Urban Classification (2011) of Lower Layer Super Output Areas in England and Wales, 2011, https://www.data.gov.uk/dataset/b1165cea-2655-4cf7-bf22-dfbd3cdeb242/rural-urban-classification-2011-of-lowerlayer-super-output-areas-in-england-and-wales Search PubMed.
-
R Core Team. R: A Language and Environment for Statistical Computing Manual. R Foundation for Statistical Computing, Vienna, Austria, 2022, https://www.R-project.org/ Search PubMed.
-
D. Baston, ISciences & L L C. Exactextractr: Fast Extraction from Raster Datasets Using Polygons version 0.9.0, 2022, https://CRAN.R-project.org/package=exactextractr Search PubMed.
-
H. Wickham, W. Chang, L. Henry, T. L. Pedersen, K. Takahashi, C. Wilke, K. Woo, H. Yutani and D. Dunnington & RStudio. Ggplot2: Create Elegant Data Visualisations Using the Grammar of Graphics version 3.4.0, https://cran.r-project.org/package=ggplot2, 2022 Search PubMed.
-
R. J. Hijmans, R. Bivand, K. Forner, J. Ooms, E. Pebesma and M. D. SumnerTerra: Spatial Data Analysis Version 1.6-17, 2022, https://CRAN.R-project.org/package=terra Search PubMed.
- H. Wickham, M. Averick, J. Bryan, W. Chang, L. McGowan, R. François, G. Grolemund, A. Hayes, L. Henry, J. Hester, M. Kuhn, T. Pedersen, E. Miller, S. Bache, K. Müller, J. Ooms, D. Robinson, D. Seidel, V. Spinu, K. Takahashi, D. Vaughan, C. Wilke, K. Woo and H. Yutani, Welcome to the Tidyverse, J. Open Source Softw., 2019, 4, 1686, DOI:10.21105/joss.01686.
-
A. Kassambara
Ggpubr:’ggplot2’ Based Publication Ready Plots Version 0.4.0, 2022, https://cran.r-project.org/package=ggpubr Search PubMed.
-
S. Garnier, N. Ross, B. Rudis, M. Sciaini, A. P. Camargo and C. Viridis Scherer: Colorblind-Friendly Color Maps for R Version 0.6.2, 2021, https://CRAN.R-project.org/package=viridis Search PubMed.
-
T. L. Pedersen, M. S. ( of AGG), T. J. ( to AGG), M. M. ( to AGG), S. G. ( to AGG) and RStudio, Ragg: Graphic Devices Based on AGG version 1.2.4, 2022, https://CRAN.R-project.org/package=ragg Search PubMed.
-
N. Gray
PRAWNS, 2023, https://github.com/Nathan-303/PRAWNS Search PubMed.
-
E. Pebesma, R. Bivand, E. Racine, M. Sumner, I. Cook, T. Keitt, R. Lovelace, H. Wickham, J. Ooms, K. Müller, T. L. Pedersen, D. Baston and D. Dunnington, Sf: Simple Features for R version 1.0-9, 2022, https://CRAN.R-project.org/package=sf Search PubMed.
-
E. Pebesma, M. Sumner, E. Racine, A. Fantini and D. Blodgett, Stars: Spatiotemporal Arrays, Raster and Vector Data Cubes Version 0.5-6, 2022, https://CRAN.R-project.org/package=stars Search PubMed.
-
H. Wickham, P. Danenberg, G. Csárdi, M. Eugster and RStudio. Roxygen2: In-Line Documentation for R Version 7.2.2, 2022, https://CRAN.R-project.org/package=roxygen2 Search PubMed.
-
H. Wickham, J. Hester, W. Chang, J. Bryan and RStudio. Devtools: Tools to Make Developing R Packages Easier Version 2.4.5, 2022, https://CRAN.R-project.org/package=devtools Search PubMed.
- Y. Benjamini and Y. Hochberg, Controlling the False Discovery Rate: A Practical and Powerful Approach to Multiple Testing, J. R. Stat. Soc., B: Stat. Methodol., 1995, 57, 289–300 Search PubMed.
- M. A. G. Demetillo, C. Harkins, B. C. McDonald, P. S. Chodrow, K. Sun and S. E. Pusede, Space-Based Observational Constraints on NO2 Air Pollution Inequality From Diesel Traffic in Major US Cities, Geophys. Res. Lett., 2021, 48, e2021GL094333 CrossRef CAS.
- S. Kingham, J. Pearce and P. Zawar-Reza, Driven to Injustice? Environmental Justice and Vehicle Pollution in Christchurch, New Zealand, Transp. Res. D: Transp. Environ., 2007, 12, 254–263 CrossRef.
- M. Habermann, M. Souza, R. Prado and N. Gouveia, Socioeconomic Inequalities and Exposure to Traffic-Related Air Pollution in the City of Sao Paulo, Brazil, Cadernos De Saude Publica, 2014, 30, 119–125 CrossRef . ISSN: 0102-311X. https://www.scielo.br/j/csp/a/.
- C. A. Onyutha, Hydrological Model Skill Score and Revised R-squared, Hydrol. Res., 2021, 53, 51–64, DOI:10.2166/nh.2021.071.
-
K. Dunn, Avoid R-Squared to Judge Regression Model Performance Medium, 2022, https://towardsdatascience.com/avoid-r-squared-to-judge-regression-model-performance-5c2bc53c8e2e Search PubMed.
-
C. Shalizi, Lecture 10: F -Tests, R2, and Other Distractions Lecture, Carnegie Mellon University, https://www.stat.cmu.edu/cshalizi/mreg/15/lectures/10/lecture-10.pdf Search PubMed.
- N. H. Anderson and J. Shanteau, Weak Inference with Linear Models, Psychol. Bull., 2022, 84, 1155 Search PubMed.
- M. H. Birnbaum, The Devil Rides Again: Correlation as an Index of Fit, Psychol. Bull., 2022, 79, 239 Search PubMed.
- R. a. O. Nunes, P. T. B. S. Branco, M. C. M. Alvim-Ferraz, F. G. Martins and S. I. V. Sousa, Gaseous Pollutants on Rural and Urban Nursery Schools in Northern Portugal, Environ. Pollut., 2016, 208, 2–15 CrossRef CAS PubMed.
- M. S. Al-Rashidi, M. F. Yassin, N. S. Alhajeri and M. J. Malek, Gaseous Air Pollution Background Estimation in Urban, Suburban, and Rural Environments, Arabian J. Geosci., 2018, 11, 59, DOI:10.1007/s12517-017-3369-2.
- E. Blaszczyk, W. Rogula-Kozlowska, K. Klejnowski, P. Kubiesa, I. Fulara and D. Mielzynska-Svach, Indoor Air Quality in Urban and Rural Kindergartens: Short-Term Studies in Silesia, Poland, Air Qual., Atmos. Health, 2017, 10, 1207–1220 CrossRef CAS PubMed . ISSN: 1873-9318. https://link.springer.com/article/10.1007/s11869-017-0505-9.
- J. Bernard, Where Have All the Rural Poor Gone? Explaining the Rural–Urban Poverty Gap in European Countries, Sociol. Rural., 2019, 59, 369–392 Search PubMed.
- R. Borck and P. P. Schrauth, Density and Urban Air Quality, Reg. Sci. Urban Econ., 2021, 86, 103596 CrossRef . ISSN: 0166-0462. https://www.sciencedirect.com/science/article/pii/S0166046220302817.
- S. Ghaedrahmati and M. Alian, Health Risk Assessment of Relationship between Air Pollutants' Density and Population Density in Tehran, Iran, Hum. Ecol. Risk Assess., 2019, 25, 1853–1869 CrossRef CAS . ISSN: 1080-7039. https://www.tandfonline.com/doi/.
- L. A. Guzman, C. Beltran, R. Morales and O. L. Sarmiento, Inequality in Personal Exposure to Air Pollution in Transport Microenvi Ronments for Commuters in Bogotá, Case Stud. Transp. Policy, 2023, 11, 100963 CrossRef.
|
This journal is © The Royal Society of Chemistry 2023 |