DOI:
10.1039/D2VA00200K
(Critical Review)
Environ. Sci.: Adv., 2023,
2, 11-38
Application of neural network in metal adsorption using biomaterials (BMs): a review†
Received
23rd August 2022
, Accepted 24th October 2022
First published on 2nd November 2022
Abstract
With growing environmental consciousness, biomaterials (BMs) have garnered attention as sustainable materials for the adsorption of hazardous water contaminants. These BMs are engineered using surface treatments or physical alterations to enhance their adsorptive properties. The lab-scale methods generally employ a One Variable at a Time (OVAT) approach to analyze the impact of biomaterial modifications, their characteristics and other process variables such as pH, temperature, dosage, etc., on the removal of metals via adsorption. Although implementing the adsorption procedure using BMs seems simple, the conjugate effects of adsorbent properties and process attributes implicate complex nonlinear interactions. As a result, artificial neural networks (ANN) have gained traction in the quest to understand the complex metal adsorption processes on biomaterials, with applications in environmental remediation and water reuse. This review discusses recent progress using ANN frameworks for metal adsorption using modified biomaterials. Subsequently, the paper comprehensively evaluates the development of a hybrid-ANN system to estimate isothermal, kinetic and thermodynamic parameters in multicomponent adsorption systems.
Environmental significance
With growing environmental consciousness, biomaterial systems (BMS) have garnered attention as a sustainable material for the adsorption of hazardous water contaminants. These BMSs are engineered using surface treatments or physical alterations to enhance their adsorptive properties. The lab-scale methods generally employ a One Variable At a Time (OVAT) approach to analyze the impact of biomaterial modifications, their characteristics and other process variables such as pH, temperature, dosage, etc., on the removal of metals via adsorption. Although the adsorption procedure using BMSs seems simple in implementation, the conjugate effects of adsorbent properties and process attributes implicate complex nonlinear interactions. As a result, Artificial Neural Network (ANN) have gained traction on the quest to understand the complex metal adsorption processes on biomaterials, with applications in environmental remediation and water reuse.
|
1. Introduction
Growing population, urbanization, and changing climate cycles stress water resources and public water supplies. The United Nations reported that 2.3 billion people live in water-scarce zones worldwide.1 Public health and economic tensions from water scarcity will be exacerbated in regions with water tainted with metal contaminants. Such water-scarce areas deserve and need solutions to remove metal contaminants and enable water remediation and reuse.2
The literature has proposed physicochemical technologies like reverse osmosis, ion exchange, electrodialysis and adsorption to eliminate metallic ions from water resources and industrial effluents.3–14 Among these technologies, adsorption is the best-suited method for treating water and wastewater due to its high efficiency with low economics.15–23 The selection and fabrication of sustainable adsorbents for the eradication of water pollutants include the following criteria: (1) it should be inexpensive and (2) simple to synthesize in large quantities, (3) it should demonstrate high adsorption capacity and most importantly, (4) it should cause no harm to the environment. Nature-inspired biomaterials have gained attention as decontamination media owing to their non-toxic, biodegradable, and inexpensive features.24–30 Further, many biomaterials exhibit chemical stability and structural integrity throughout repeated adsorption and desorption cycles, enabling their use for heavy metal ions removal.31 The porous texture of biomaterials speeds up the transport of metal ions, while the presence of phenolic, carbonyl, amide and amine-containing functional groups in biomaterials facilitate metal ion adsorption through surface complexation.32,33 In addition, biomaterial systems have been engineered using various surface functionalization and physical alterations to tailor their surface chemistry and enhance their adsorptive capacity. For instance, our research team has prepared eco-friendly cellulose beads impregnated with nano iron oxide for thorium and arsenic retrieval;34,35 synthesized cost-effective cellulose nanofibers and functionalized with camphor soot carbon nanoparticles for uranium extraction;36 crosslinked starch with polyvinyl alcohol for oil–water separation;37 electrospun nano fibres for adsorption of metal ions;38–41 developed composites from agro-wastes for removal of metal and dyes.42 The details of distinct physical and chemical modifications of biomaterials are illustrated in Fig. S1.†
The BMs' performance is greatly affected by environmental conditions (pH, temperature), initial metal concentration, and the structure of the biomaterial. Laboratory experiments have been used to discover high-performing BMs; however, these studies are costly for time and resources. Accordingly, modelling techniques could offer time- and resource-saving efficiency in predicting the performance of BMs for full-scale application.43,44 Mathematical models based on multiple linear or nonlinear regression (MLR, MNLR) and response surface method (RSM) have been proposed to assess the removal potential of metallic contaminants using experimental data from isotherm and kinetic studies.45–48 The joint role of spectroscopic analysis and RSM has been applied to enumerate the adsorptive removal of zinc (Zn(II)), cobalt (Co(II)) and nickel (Ni(II)).49 Since these adsorption systems exhibit nonlinear adsorptive behaviours, it would be inappropriate to characterize them using conventional statistical models. In this sense, the ANN learning principles are potent means to optimize the metal adsorption process on biomaterials by establishing a nonlinear relationship between independent (pH, temperature, dose, time of contact, the concentration of metal ions, biomaterial characteristics, etc.) and dependent variables (metal uptake capacity, % adsorption efficiency, etc.) for single and multi-metallic wastewater systems.50–53
Studies in the past stipulated the removal efficiency of metals using various artificial intelligence (AI) models. For example, Bhagat et al., 2020 examined the application of distinct AI models like kernel, evolutionary, black box, fuzzy and hybrid models for optimizing heavy metal removal.206 Rather than illustrating the mathematical concepts necessary for automation, the author highlighted treatment procedures such as flocculation, coagulation, membrane filtration, biosorption, proposed prediction models, input and output variables, and distinct metrics for comparing model performance. Alam et al., 2022; Reynel-Ávila et al., 2022 categorized AI technologies and reported their use in the remediation of organic and inorganic contaminants.207,208 Whereas Yaseen et al., 2021 discussed the utility of AI in simulating soil and water bodies contaminated with metals.209 The utilization of classical adsorption models, multicomponent adsorption, sensitivity analysis, and progression in ANN frameworks were not discussed earlier in detail for evaluating kinetics, isotherms, and thermodynamic parameters of adsorption.
This review briefly explains the main biomaterial alteration processes and discusses conventional adsorption investigations in conjunction with ANN modelling. Then, we address the pre- and post-processing methods involved in constructing ANN models and show statistics of datasets considered for optimizing metal adsorption on biomaterial systems. We describe current advancements in the ANN framework for single and multi-metal adsorption process optimization and related improvements in hybridizing isotherm, kinetics, and breakthrough curves. Next, advances in thermodynamic parameter estimations are reported to understand the nature of the adsorption process. The sensitivity analysis of ANN has also been described to comprehend the relative influence of individual and group of adsorption factors on anticipated efficiency. Towards the end, the review highlights significant challenges and advancements in the field of ANN technology for metal remediation.
2. Experimental studies and dataset of metals adsorption on BMs
A given biomaterial's interaction with metal adsorbates depends on its functional groups, the engineered surface of biomaterials, pH and temperature conditions, and physical or chemical changes in the configuration that influence biomaterials' morphology, pore size distribution, and elemental compositions. However, biomaterials without modification have constraints regarding recovery and recycling. Because of their poor porosity and limited adsorption sites, pristine biomaterials exhibit low adsorption efficacy.54
Thus, the engineered modifications and surface alterations of the biomaterial matrix become necessary to achieve greater adsorption capacities. Research reports the conversion of natural biomaterials into beads, foams, and nanofibers to attain better pore size distribution and durability. The surface area of biomaterials has also been enhanced via their conversion to nanoscale structures and activated carbon.55–62 The surface treatment of biomaterials with acids and alkalis improved their interactions with metals, which resulted in faster adsorption rates and increased uptake capacity.63–66 The reutilization of saturated biomaterials into value-added products has been reported in detail by authors in their earlier research.7 The experimental adsorption studies that depict various aspects of metals adsorption relevant for ANN modelling are given below:
2.1 Adsorption kinetics
Adsorption kinetics gives vital information about the associated mechanism and equilibrium time required to sequester maximum metal impurities from an aqueous solution. The period of contact (CT) between the biomaterial and metal adsorbate and equilibrium time (te) corresponding to specific metal concentrations (Co) are listed in Table 1. The kinetics adsorption data using modified biomaterials fitted best with the pseudo-second-order equation, where the values of kinetic model parameters differ regarding when the equilibrium time is reached. The experimentally obtained details (initial metals concentration, contact time and equilibrium time) will aid in developing ANN-driven kinetic models for predicting the significant kinetic parameters and uptake capacity detailed further in Section 5.
Table 1 Time required (te) to reach equilibrium for the metal adsorption process using biomaterialsa
Metal adsorbed |
Biomaterials |
CT (min) |
t
e (min) |
C
o (mg L−1) |
Reference |
CT = contact time, te = equilibrium time, Co = initial concentration of metals.
|
As(V) |
Iron oxide permeated mesoporous rice-husk |
0–1440 |
1440 |
60 |
63
|
Cd(II) |
Alkali-modified oak waste residues |
0–240 |
60 |
25–100 |
64
|
Co(II) |
Alginate-SBA-15 nanocomposite |
0–300 |
300 |
100 |
58
|
Cr(VI) |
Nanocomposite from sawdust biochar |
0–200 |
120 |
50 |
67
|
Cr(VI) |
Acidically prepared rice husk carbon |
0–180 |
120 |
60 |
68
|
Cr(VI) |
Activated carbon from medlar seed |
0–130 |
60 |
200 |
69
|
Cr(III), Cr(VI) |
Nanocrystalline cellulose |
0–60 |
40 |
25 |
70
|
Cr(VI) |
Iron ions doped on the rice husk |
0–240 |
240 |
50 |
71
|
Cu(II) |
Banana floret |
0–60 |
60 |
50 |
72
|
Cu(II) |
Orthophosphoric acid–modified biochar |
0–60 |
60 |
10 |
73
|
Pb(II) |
Rice husk carbon (RHC) |
0–120 |
120 |
60 |
74
|
Pb(II) |
Carboxylate-functionalized walnut shell |
0–15 |
15 |
100–220 |
75
|
Pb(II) |
Rice husks digested with nitric acid |
0–180 |
120 |
20–80 |
76
|
Hg(II) |
Activated carbon derived from local walnut shell |
0–120 |
60 |
50 |
77
|
Ni(II) |
Alginate-based composite bead (AB) |
0–100 |
80–100 |
100 |
59
|
Th(iv) |
Chitosan/TiO2 nanocomposite |
0–70 |
70 |
200 |
78
|
U(VI) |
Polyacrylonitrile grafted potato starch-based resin |
0–120 |
120 |
20 |
79
|
U(VI) |
KMnO4-modified hazelnut shell activated carbon |
0–200 |
160 |
100 |
66
|
Zn(II) |
Rice husks digested with nitric acid |
0–120 |
15–90 |
25 |
65
|
Co(II), Ni(II) |
Alginate nanoparticles |
0–80 |
80 |
80.115, 10.523 |
55
|
Cr(VI), Cu(II) |
Wheat straw |
0–60 |
15, 30 |
20 |
80
|
Cd(II), Pb(II), Ni(II) |
Functionalized wood pulp |
0–40 |
40 |
25 |
81
|
2.2 Adsorption isotherms
Adsorption isotherm models describe the maximum adsorption capacity at equilibrium adsorption conditions. Table 2 displays the adsorption isotherm data for modified biomaterials for different metal ions. The Langmuir isotherm best characterized the adsorbent–adsorbate interactions for biomaterials, suggesting monolayer adsorption of metal ions on the biomaterial surface. In general, the use of linear empirical models to evaluate the isotherm parameters of the nonlinear adsorption process is becoming obsolete as it does not explicitly describe the simultaneous adsorption pattern over a wide range of operating temperatures and metal ion concentration.82 Furthermore, because most industrial wastewaters contain numerous metal pollutants, single-species models do not adequately reflect the complex propensity of multicomponent adsorption. For this purpose, the isotherm parameters evaluation using hybridizing isotherms with ANN, as discussed in Section 5, is gaining popularity.
Table 2 Model parameters of the adsorption isotherm used in the adsorption of specified metal contaminants onto the listed biomaterials adsorbents
Metals adsorbed |
Biomaterials |
Langmuir |
Freundlich |
Redlich–Peterson |
Dubin–Redushkevich |
References |
Q
max (Mg g−1) |
K
L (L mg−1) |
K
f (mg g−1) (mg L−1)a |
1/n |
K
r (L g−1) |
α (Lθ mgθ) |
θ |
Q
m (mg g−1) |
K
dr (mol2 kJ−2) |
Cd(II) |
Alkali-modified oak waste residues |
771.4 |
0.006 |
— |
— |
— |
— |
— |
— |
— |
64
|
Co(II) |
Alginate-SBA-15 nano composite |
78.1 |
1.04 |
— |
— |
— |
— |
— |
— |
— |
58
|
Cr(III) |
Nanocrystalline cellulose |
2.88, 2.77 |
1.49, 0.48 |
— |
— |
— |
— |
— |
— |
— |
70
|
Cr(VI) |
Acidically prepared rice husk carbon (APRHC) |
47.62 |
0.018 |
— |
— |
— |
— |
— |
— |
— |
68
|
Cr(VI) |
Activated carbon from medlar seed |
212.76 |
0.072 |
— |
— |
— |
— |
— |
— |
— |
69
|
Cr(VI) |
Iron ions doped on the rice husk |
— |
— |
0.63 |
0.28 |
— |
— |
— |
— |
— |
71
|
Cr(VI) |
Chitosan foamed structure |
10.08 |
— |
— |
— |
— |
— |
— |
— |
— |
61
|
Cu(II) |
Orthophosphoric acid-modified biochar derived from coconut (cocos nucifera) husk |
175.438 |
0.372 |
— |
— |
— |
— |
— |
— |
— |
73
|
Pb(II) |
Rice husk carbon (RHC) |
62.5 |
0.23 |
— |
— |
— |
— |
— |
— |
— |
74
|
Pb(II) |
Carboxylate-functionalized walnut shell |
192.31 |
0.36 |
15.27 |
0.43 |
— |
— |
— |
— |
— |
75
|
Hg(II) |
Activated carbon derived from local walnut shell |
80 |
0.0088 |
— |
— |
— |
— |
— |
— |
— |
77
|
Ni(II) |
Alginate-based composite bead (AB) |
— |
— |
— |
— |
76.45 |
7.98 |
0.82 |
1.539 |
1.262 × 10−8 |
59
|
Th(IV) |
Chitosan/TiO2 nanocomposite |
455 |
— |
— |
— |
— |
— |
— |
— |
— |
78
|
U(VI) |
KMnO4−modified hazelnut shell activated carbon |
22.27 |
0.041 |
— |
— |
— |
— |
— |
— |
— |
66
|
Co(II), Ni(II) |
Alginate nanoparticles |
21.46, 6.199 |
0.062, 0.026 |
— |
— |
— |
— |
— |
— |
— |
55
|
Cr(VI), Cu(II) |
Wheat straw |
09.19, 5.38 |
0.4598, 2.57 |
— |
— |
— |
— |
— |
— |
— |
80
|
Zn(II), Cu(II), Cr(VI) |
Chitosan foamed structure |
5.675, 17.77, 10.8 |
— |
— |
— |
— |
— |
— |
— |
— |
61
|
2.3 Thermodynamics
Thermodynamic parameters offer insights into the impact of changing environmental conditions (i.e. temperature) on the nature of the adsorption process using modified biomaterials. Table 3 details the adsorption process's temperature range, feasibility, and nature. The data will aid in modelling thermodynamic conditions for predicting thermodynamic parameters (e.g., Gibbs free energy changes) across a wide range of adsorption conditions, as discussed in Section 5.
Table 3 Details of nature of adsorption process at different temperature ranges
Metal adsorbed |
Biomaterials |
Temperature range (K) |
Adsorption process |
References |
Cd(II) |
Alkali-modified oak waste residues |
298–313 |
Exothermic |
64
|
Co(II) |
Alginate-SBA-15 nanocomposite |
308–328 |
Endothermic |
58
|
Cr(VI) |
Acidically prepared rice husk carbon |
298–333 |
Endothermic |
68
|
Cr(VI) |
Activated carbon from medlar seed |
298–318 |
Exothermic |
69
|
Cr(VI) |
Iron ions doped on the rice husk |
293–323 |
Endothermic |
71
|
Pb(II) |
Rice husk carbon |
298–333 |
Endothermic |
76
|
Ni(II) |
Alginate-based composite bead (AB) |
298–358 |
Exothermic |
59
|
Cu(II), Cr(VI) |
Wheat straw |
298–318 |
Exothermic |
80
|
Cd(II), Pb(II), Ni(II) |
Functionalized wood pulp |
298–318 |
Exothermic |
81
|
Th(IV) |
Chitosan/TiO2 nanocomposite |
298–318 |
Endothermic |
78
|
U(VI) |
KMnO4− modified Hazelnut shell-activated carbon |
298–318 |
Exothermic |
66
|
2.4 Adsorption data set based on BMs and pre-processing
The biomaterials in the current review included cellulosic, alginate and chitosan-based biomaterials. Compared to other polysaccharides, plant and agriculture-based biomaterials have been extensively modelled for metal remediation purposes (refer to ESI, S2†). The standards for modifying the biomaterials mentioned above are found in the literature.55,59–61,70,71,80,81,83,84 The physicochemical features of the reviewed modified biomaterial systems were determined by different analytical techniques such as BET, FTIR and SEM-EDS. The morphology of some of the modified BMSs under a scanning electron microscope is shown in Fig. S2.†
Experimental data is an essential prerequisite in processing the ANN framework. The details of the diverse dataset, including the characteristics of biomaterials, environmental conditions (temperature, pH) and process variables, are given in Section S2.† The experimental dataset contained 15 variables, including initial concentration (C0), pH solution (pH), temperature (T), biomaterial characteristics (surface area, particle size), contact time (CT), bed depth (BD), flow rate (FR), agitation speed (AS), the volume of solution (V), pyrolysis temperature (PT), effluent concentration (EC), medium of solution (MS), bias (B), metal pollutant efficiency (MPE%) and adsorption capacity (AC). MPE % and AC were the output variables; the remaining variables were applied as input variables. A few studies used final concentration (FC), final pH (FpH), and change in Gibbs free energy (dG) as the output variable in conjunction with MAE% and AC. The frequency of individual variables used by researchers is illustrated in Fig. S3.†
Pre-processing experimental data using Pearson's correlation matrix is carried out to analyze the relationship among adsorption variables. In the cellulose-based biomaterials, the correlation matrix showed a complex correlation between process variables.85 However, in the case of carbon-enriched BMSs, it was worth mentioning that the oxygen to carbon ratio (O
:
C) and the sum of oxygen and nitrogen to carbon content (O + N
:
C) showed an absolute correlation.86 Hence, one of these two variables was eliminated as they represented the same data from the database. The (O + N
:
C) variable had a better connection with the output variable over O
:
C; thus, it becomes a strong contender to increase the precision of the models and explain the characteristics of the dataset employed. As illustrated in Fig. 2, the boxplot shows the range of key variables considered for modelling metal adsorption on biomaterial systems.
Post–pre-processing, the complete dataset is taken and randomly divided at 70
:
30.58 70% of the data is used for training the ANN model, while 30% is used for validating and testing the performance efficiency of ANN. The distribution of the attributes (ESI, S2†) shows a skewness in data distribution which can significantly affect the stability and accuracy of the ANN predictive models. Therefore, the features of the dataset are normalized using the Minmax function.
3. ANN for modeling for metal adsorption
3.1 Layman's guide to ANN
Artificial Neural Networks are inspired from a biological brain conceptual model to solve complex problems.87 Consider how a human brain distinguishes between different people: every human has a similar overall structure (e.g., two eyes, two ears, one nose, etc.), yet we can recognize people easily because learnings in the brain are intuitive. Instead of learning the face structure to identify people, it discovers the deviation of the face from a reference face, for example, ‘how different one's nose is from the generic nose, which is then quantified as a signal with a specific strength’. Likewise, it learns the deviations from all parts of the face from a reference base case, combines these variations into a new dimension, and finally gives an output, which is the recalled identity of the good-looking person in front of you. All these steps in the brain occur in a fraction of a second. A neural network uses a similar algorithm, but the artificial neurons process the information using a mathematical approach (Fig.S4†).
The ANN architecture is organized into 3 layers: (1) an input, (2) a hidden or intermediate layer, and (3) an output layer. The information is first received by neurons in the input layer, then passed on to a set of neurons associated with single or multiple hidden layers. The job of a hidden layer is to process the information coming from input neurons using weighted connection and activation functions to calculate the output of a neuron. The data is processed from one neuron to the other, similar to the deviations learned by the human brain. The greater the outcome of a neuron, the greater would be the influence of that input dimension. These attributes are combined in the next layer using mathematical formulations to form additional new details. When multiplied several times, this procedure develops a complex network with several connections.
The neural network learns through intuitive wisdom with the help of a learning or training mechanism. For a given set of input data, the output layer makes predictions by applying a matrix multiplication series that could be either accurate or inaccurate. Based on the output, the learning mechanism gives feedback for improving the prediction efficiency of the network. The system uses a backpropagation algorithm as a feedback mechanism to incrementally update the randomly initialized weights applied to the input data for correct predictions.88
3.2 Generation of the ANN model
Fig. 2 illustrates the flow chart for modelling the metal adsorption process via ANNs. Initially, the experimental adsorption variables containing independent and dependent parameters are collected from lab experiments compiled from the literature. The acquired database is generally divided into training, validation, and test sets. Since the adsorption data of metal ions constitute many features (as shown in Fig. 1), the associated hyperparameters of neural network function also increase, raising the model's complexity. In such scenarios, a larger proportion of data (∼70%) is kept in the training subset to make the model learn the patterns of the data, while the rest 30% of data is used for validating and testing the model performance. The network is trained to find the optimal combinations of intermediate neurons and interior layers that minimize the prediction error or loss. The neural network is trained using backpropagation (BP) algorithms which consist of the following steps, corresponding to the steps listed in Fig. 2:
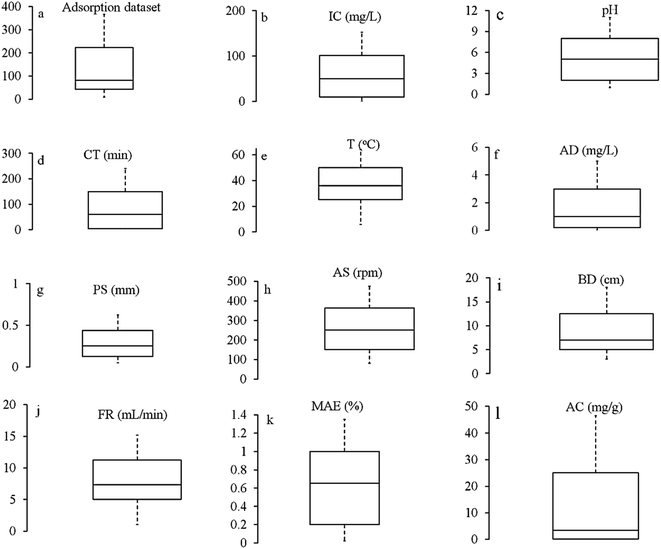 |
| Fig. 1 The dataset before analyzing and processing (the box plots generated using data listed in ESI, Tables S1 and S2†) *AD = adsorbent dose, IC = initial concentration, CT = contact time, T = temperature, PS = particle size, BD = bed depth, FR = flow rate, AS = agitation speed, MPE = metal pollutant efficiency, AC = adsorption capacity. *The middle line in the box represents the median, the center line represents the mean, while the bottom and top lines of the box represent the 1st and the 3rd quartiles, respectively. | |
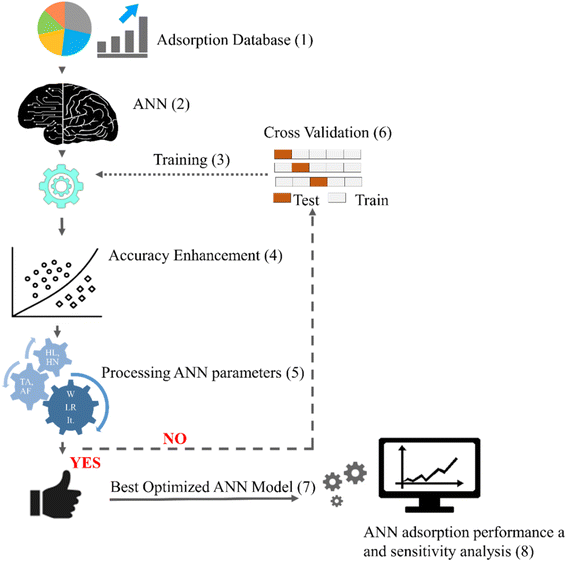 |
| Fig. 2 Standalone ANN-framework for predicting metal adsorption onto biomaterials. | |
(1) Selection of training data from the experimental data set.
(2) Identification and division of input and output variables.
(3) Forward propagation: it takes a weighted sum of inputs (by multiplying each input variable with an assigned weight) and bias. This weighted output is then passed through an activation or transfer function, which introduces nonlinearity into the result. (For mathematical interpretation, refer to ESI, S.2.2†). Depending on the activation function used, the outputs are normalized between either 0 and 1, −1 to +1, or 0.1 to 0.9. Table S3† lists activation functions applied for the nonlinear transformation of adsorption data.
(4) Backpropagation: the ANN calculates the difference in error between the experimental observation and the expected model output using the gradient descent method. When the error exceeds the acceptable value, the weights are adjusted by multiplying the error by the input and the transfer function's gradient (e.g., tansig function, which is the most common) (ESI, S.2.2†).
(5) Optimization is achieved by reducing the error between observed and model-predicted responses by varying neurons in hidden layers, transfer functions, training algorithms and iterative modification of weights assigned to links emerging from the input layer.87,89 Steps 5 and 6 (of Fig. 2) are repeated until further weight changes do not reduce errors (refer to S5.2†).
(6) The performance of the ANN for modelling adsorption is evaluated using different statistical indices (Fig. 2 step 7, 8). The researchers most commonly used the coefficient of determination (R2) and correlation coefficient (R) as efficiency evaluators, whereas Root Mean Square Error (RMSE) and Mean Square Error (MSE) are used for evaluating modelling error. The mathematical formulations of these four statistical parameters are given in S5.3.†
The illustration of the feedforward neural network (FFNN) that modelled the adsorption of the metal on biomaterials is presented in Fig. S5.†
4. Progressions in ANN frameworks for optimizing metal adsorption process using BMs
4.1 Standalone ANN frameworks
The purpose of the optimization is to achieve the maximum metal removal efficiency and uptake capacity of biomaterials used to recover metals and other contaminants from industrial wastewater for environmental protection and water purification. The optimization pathways reported in the literature are a compilation of variables influencing the design of adsorption systems and the adsorption process.90 The variables affecting the adsorbent modification or preparation conditions and the metal adsorption efficacy include biomaterial dose, surface type and thermal treatments. Under the batch adsorption systems, the adsorption attributes that affect the process behaviour include the initial concentration of metal pollutants, pH of the aqueous solution, volume and medium of adsorbate solution, agitation or shaker speed, temperature and contact time. In continuous column set-up, metal concentrations, bed depth and flow rate are of primary concern (Table S4†). Some studies also included the influence of lingo-cellulosic functional groups, particle size, and calcination temperature used to optimise biomaterial adsorbents' fabrication.52,65,66,91,92Table 4 illustrates recent developments of ANN-based optimization methods for modelling biomaterial adsorption systems.
Table 4 Recently applied optimization methods in adsorptive removal of metals using biomaterials
Metal Pollutant |
Biomaterial |
Method of optimization |
Model error (RMSE) |
Reference |
Least error from ANN-GA.
|
Cd(
II
),Al(
III
), Co(
II
), Cu(
II
), Fe(
III
), Pb(
II
)
|
Chitosan and chitosan-montmorillonite nanocomposite |
ANN-SOS |
R = 0.96 |
83
|
Pb(II) |
Hydroxyapatite/chitosan nanocomposite |
ANFIS |
R = 0.98 |
60
|
Pb(II), Co(II) |
Rafsanjan pistachio shell |
ANN-GWO |
1.12 |
101
|
As(V) |
ZnCl2 activated carbon enriched biomaterial from Opuntia ficus indica biomass |
Hybrid-ANN isotherms and kinetics |
R
2 = 0.99 |
102
|
Pb(II) |
Iron oxide nanocomposites from bio-waste mass |
ANN |
0.000076 |
85
|
Cr(VI) |
Alginate immobilized Sargassum sp. |
ANN-GAa, ANN-SA, Taguchi design |
0.07a |
93
|
Cu(
II
), Pb(
II
), Zn(
II
), As(
III
), Cd(
II
), and Ni(
II
)
|
Carbon enriched biomaterial |
ANN-QSS |
0.074 |
103
|
s described in Section 3.2, the ANN framework has been extensively implemented to optimize the variables mentioned above to attain conditions for the maximum metal adsorption efficiency or metal adsorption capacity. The details of optimal ANN architecture, activation function and modelling error corresponding to each study are depicted in Table S5.† It is to be noted that the ANN training parameters such as epoch size, learning rate, momentum and gradient influence the optimal feedforward architecture. The data division in training, validation and testing subsets and information on maximum epochs (iterations) used is illustrated in Fig. 3 (taken from Table S5–S7†).
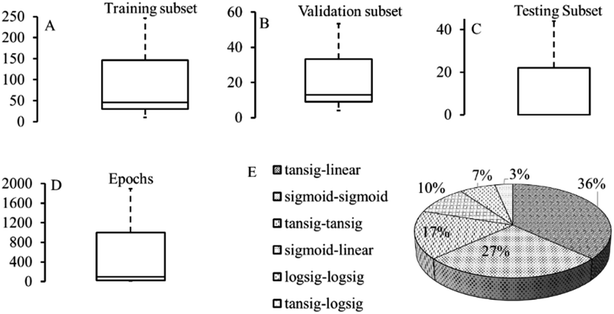 |
| Fig. 3 Dataset, maximum epoch size and activation functions considered for ANN model development with step size = 0.01, momentum = 0.7–1, minimum gradient = 0.062. (The graphs were generated using data from ESI, Section S5†). | |
Table 4 lists the recent developments in ANN frameworks for simulating metal adsorption on biomaterials. While backpropagation (BP) algorithms are prominently reported in the literature for the optimization of various adsorption variables, algorithms based on experimental designs such as the Taguchi method which use orthogonal arrays to identify critical variables that can affect the adsorption process.93 Algorithms based on 2-level factorial design (FFD) have also been employed with ANN to reduce the burden of laboratory experiments and determine the optimum process variables that can maximize metal adsorption on the biomaterials. For example, Popoola, 2019 used 2k FFD to evaluate the optimal preparation condition of carbon-enriched biomaterial that can boost cadmium removal efficiency.94 The authors used magnetite loading, walnut shell: rice husk ratio, calcination temperature and time as variables to conduct 24 = 16 i.e. 16 sets of experiments. It was observed that a small set of experiments were sufficient to predict the optimal adsorbent conditions (i.e. carbonaceous biomaterial calcinated at 1000 °C for 5 hours and loaded with 10 wt% magnetite coating) that will yield maximum cadmium removal (∼97%) from aqueous solution.
The RSM has been employed to conceptualize the interaction effects among independent adsorption variables.95 RSM uses the Box–Behnken Design (BBD) to investigate the interactions between different adsorption variables, for example, initial metal concentration, pH and biomaterial dose. The BBD works as a second-order polynomial equation. Following the solution of the BBD model, the analysis of variance (ANOVA) and regression coefficients of the whole polynomial model is used to evaluate the contribution of each process variable individually and collectively.47,63,96–98
The RSM approach is only confined to a quadratic equation; thus ANN-based model offers broader competence to capture the complex and nonlinear behaviour of the metal adsorption process from effluents with a wide spectrum of dependent factors.57,75,86,99,100 While ANN models for metal remediation call for advanced computing abilities, Narayana et al., 2021 proposed an ANN-based graphical user interface (GUI) for experimentalists or researchers unfamiliar with computation to extract adsorption data for a particular dataset.85
4.2 Ensemble ANN frameworks
The standalone ANN framework trains the adsorption data using the backpropagation (BP) training algorithm. Given that BP uses the gradient descent approach to update the network's link weights, the network may converge prematurely or get stuck in local minima.104,105 Because of such drawbacks, ANNs can fall short of expected performance. To overcome such problems, ANN is bagged with metaheuristic algorithms for efficient optimization.106–109 The flow chart illustrating any metaheuristic system's general framework is given in Fig. 4.
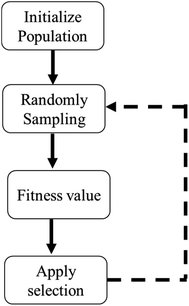 |
| Fig. 4 A general working scheme of a metaheuristic algorithm. | |
Hamidian et al., 2019 used the Symbiotic Organisms Search (SOS) algorithm in conjunction with ANN to optimize heavy metals removal (Fe(II)), Cu(II), Co(II), Cd(II) and Al(III)) using chitosan-based nanomaterials.83 The authors applied adsorbent dose, pH and initial concentration of metals as input parameters to the network. The symbiotic search algorithm (SOS) is a recently developed algorithm based on SI (Swarm Intelligence) that simulates the cooperative behaviour observed in nature among individuals. It generates a new solution using three coexisting behaviour types between paired organisms in an ecosystem: mutualism, commensalism, and parasitism. Mutualism is an interaction between two species with mutual benefit, meaning both benefit from the relationship. Commensalism occurs when one species forms a bond with another, and one species benefits while the other is unaffected. When two species form a relationship in which one benefits and the other hurts, it is referred to as parasitism. Both mutualism and commensalism focus on creating new species for the next generation. The parasitic phase prevents the search process from escaping the local minima. The ANN-SOS framework is illustrated in Fig.S6.†). The integration of ANN with SOS predicted metal removal efficiencies with R2 > 0.9 in short computation time (50 runs) and fast convergence (<20). The SOS has drawn considerable attention in several optimization fields as compared to differential evolution (DE) and particle swarm optimization (PSO) due to its simple procedure and consistency in accurate predictions.110–113
Moradi et al., 2020 used a hybrid of Bayesian regularization (BR) and Grey wolf optimizer (GWO) with ANN to model Pb(II) and Co(II) adsorption on pistachio shells.101 The ANN space was initially optimized using the BR algorithm, using principles of probability distributions to prevent overfitting of the ANN. The three input parameters, i.e. temperature, adsorbent dose and initial concentration of metals, were then further optimized by applying GWO to the space of BR-ANN for maximum metal ions removal. The GWO is a new global optimization approach that simulates grey wolf leadership and natural hunting.114 In GWO's hierarchy, the alpha is considered the group's dominant agent (best solution). The next subordinate to alpha includes beta (second fittest solution) and delta (third fittest solution), and omega wolf denotes the weakest solution. Additionally, three main phases of hunting, i.e. search for prey, encircle the prey and attack’, have been implemented for optimization. The framework of BR-ANN-GWO is shown in Fig. S7.† The ANN-BR-GWO framework predicted the metal adsorption with considerably improved accuracy (R2 = 0.99, RMSE = 1.1) against ANN-GWO (R2 = 0.96, RMSE ∼2.2. GWO's robust search capability prevents the algorithm from getting trapped in a local optimum. In GWO, achieving the right balance between the ability to explore and exploit is easy, so it can effectively solve many complex problems when coupled with ANN.115 ANN-GWO has also been successfully used to predict the performance of desalination plants and crop yields,116 to globally predict COVID-19 disease,117 measure pan evaporation to compare irrigation water needs118 and to prevent cyberattacks in our networks.119
Prabhu et al., 2021 successfully used a Genetic algorithm (GA) to determine the recovery of chromium from alginate immobilized Sargassum in a fixed bed column. GA works on the principles of Darwinian biological evolution from natural selection, where individuals are chosen from the population to serve as parents, after which they are employed to bear the future generation's offspring.93 The population “evolves” to the best option through future generations. In Fig. S9,† the ANN-GA framework is displayed. In their study, the authors tuned the chromium concentration, bed length and flow rate to maximize the adsorption of chromium metal. ANN-GA showed better productivity (RMSE = 0.07) against Boltzmann statistical thermodynamics (Simulating Annealing (SA), RMSE = 0.8) (van Laarhoven & Aarts, 1987). The development response of ANN-GA for predicting metals adsorption showed a better statistical quality compared to traditional statistical models.45,78,210 The ability of evolutionary GA to address any optimization problems by tuning the selection mechanism and varying the values of genetic operators as per the problem makes these techniques superior to classic numerical optimizations. GA-ANN has attracted significant attention due to its multiple advantages (i.e. simple method, robust response to changing conditions and flexibility etc.) in solving real-world problems. These include predicting energy consumption in buildings,120 detecting fatal heart disease,121 solving hydrogeology problems122 and optimizing machine parameters to reduce surface roughness123etc. The ability of GA to incorporate domain-specific knowledge into the algorithm results in a more efficient exploration of the state space of possible solutions.
Further, the two-step method for self-adapting parameters that govern evolutionary search relieves the human operator from the requirement to manually create solutions, which either consumes time or it is difficult.124 Evolutionary algorithms can be hybridized with other models to address particular real-world problems.125
More recently, Zheng and Nguyen, 2022 have implemented Queuing Search Algorithm (QSA) to update the weights of ANN using the three main activities of humans in queuing: (i) prefer following the customer queue with prompt service. (ii) Effect of customers or employees on customer service. (iii) Impact of not maintaining the queue on customer service.103 The QSA model stimulates the queening system, as described in ref. 126, to optimize the adsorption of metals, i.e. arsenic (As(II)), cadmium (Cd(II)), nickel (Ni(II)), copper (Cu(II)), lead (Pb(II)) and zinc (Zn(II)) on carbon-enriched biomaterial. The model used initial concentration, total carbon content, pH of the solution and pyrolysis temperature as input and metals adsorption efficiency as the output. The details of the ANN-QSA optimization procedure is illustrated in Fig.S10.† The adsorption efficiency predicted by the ANN-QSA model was closer to the metal adsorption efficiency of metals (i.e. RMSE = 0.051 and RMSE = 0.074 for the training and testing datasets, respectively). The standalone ANN model predicts adsorption efficiency (i.e. RMSE = 0.076 and RMSE = 0.097 for the training and testing datasets, respectively). The QSAs have been used to optimize mechanical design problems (e.g. spur gear drive systems),127,128 but its hybridization with ANN has been reported first time for wastewater treatment applications.
Besides combining metaheuristic algorithms with ANN, a fuzzy model has been coupled with ANN to capture the nonlinearity of the metal adsorption process. The ANFIS structure consists of five layers with two types of nodes: fixed and adaptable (details, refer to S5.9.3†). Nodes in the membership function layer and the next layer are tuneable, while the rest nodes are fixed. The neuro-fuzzy arrangement uses ANN learning principles and logical reasoning to map input parameters through membership functions to generate output(s). The details of ANFIS architecture can be seen in S5.9.3.1† Sadeghizadeh et al., 2019 integrated a fuzzy system with ANN to predict Pb(II) adsorption on hydroxyapatite/chitosan nanocomposite.60 The authors considered hydroxyapatite (Hap) concentration, temperature, time, pH, agitation speed, adsorbent dose and initial Pb(II) concentration as input model parameters and lead removal efficiency as model output response. ANFIS models the Pb adsorption process by combining fuzzy “if-then” logic with neural networks' superior learning capabilities (II). The anticipated model outcomes and the experimental findings were remarkably consistent, with a correlation coefficient (R) close to unity and negligible model error. The ANFIS modelling results for metals remediation using various biomaterials have outperformed results obtained using standalone ANN frameworks and conventional statistical models.52,84,91,129 Despite its acceptance in many other fields, including, e.g. medicine,130 energy,131 sports132 and passenger demand forecasting,133 ANFIS suffers from the curse of dimensionality and computational cost. The complicated structure and gradient learning in ANFIS add to the computation cost of ANFIS.
4.3 Assessment of conventional, ANN and ensemble-ANN models
ANN is a data-driven modelling approach that addresses adsorption prediction and interpretation issues by employing dataset knowledge particular to an adsorbent–adsorbate combination. The standalone ANN frameworks extensively used Levenberg–Marquardt (LM) backpropagation training algorithm and hyperbolic tangent-linear activation functions to optimize the metal adsorption process on biomaterials systems (ESI, S2†). The LM algorithm incorporates the fast convergence ability of the Gauss–Newton algorithm and inherits the steepest descent method's stability to minimize the modelling error.134 The role of activation function is critical in tuning the ANN model. The researchers have applied mainly hyperbolic tangent (tansig) activation function at the hidden layer as it centres each layer's output more or less around 0, which frequently aids in accelerating convergence.135 The current developments in the field of machine learning demonstrate the potential of scaled exponential linear unit (SELU), rectified linear unit (ReLU) and exponential linear unit (ELU) to overcome the problems of overfitting and huge training dataset.136 Since the experimental adsorption data set are not very big (<500 data points), scholars have usually selected classical activation functions for nonlinear mapping of data points. Future research can investigate the impact of varying dataset sizes, training algorithms, and activation functions on the quality of interactions and model performance.
The performance of standalone and ensembled ANN systems for simulating adsorptive eviction of metal ions using biomaterial adsorbents has also been studied along with traditional mathematical models (e.g. RSM, MLR, MNLR) as tabulated in Table 5. It was also reported that the ANN successfully optimized the metal adsorption process for datasets beyond the studied ranges.48,57,62,98,99,137,138 These results validate their true generalization capability. In the case of a smaller dataset (<50 data points), the results predicted by ANN models very well matched the actual data points, demonstrating the ANN's suitability to decrease reagent use, which would impact the economic aspect of wastewater treatment.138,139 The integration of evolutionary algorithms and fuzzy models with ANN has improved the predictive ability of ANN systems. This behaviour can be attributed to the knowledge of search algorithms to create multiple solutions to a given problem.140 Each solution holds various parameters that can aid in enhancing the ANN efficiency. Fig. 5 sums up the predictive power of ensemble ANNs over standalone frameworks and traditional statistical models.
Table 5 Comparison of different reports on standalone and ensembled ANN-based modelling methods for adsorptive eviction of distinct metal ions using biomaterial adsorbentsa
Models |
Model input |
Model output |
Performance metrics |
Comments |
Ref |
R
2: coefficient of determination, MSE: mean square error, R: correlation coefficient, RMSE: root mean square error, AARE: absolute average relative error, SD: standard deviation, MRE: mean relative error, SSE: sum of square error, MAE: mean average error, ARE: average relative error, χ2: chi-square, Fobj: objective function, ARPE: average relative percentage error, RMS: root mean square.
|
ANN |
Ion concentration, adsorbent weight, temperature, contact time and pH |
Adsorption efficiency |
MSE, R |
Based on the batch experimental data, ANN reliably predicted the adsorption efficiency of Pb(II) on Antep pistachio shells |
141
|
ANN |
Temperature, particle size, ion concentration and pH |
Adsorption efficiency |
MSE, RE |
ANN predicted the adsorptive eviction of Cu(II) using sawdust with a mean error within ±1% |
142
|
ANN |
Metal concentration, biomass dose, time and temperature |
Adsorption efficiency |
ARPE, R2 |
ANN model displayed ∼98% accuracy with the experimental As(III) adsorption on bacillus cereus biomass |
143
|
ANN |
Volume, ion concentration, adsorbent dose, contact time and pH |
Sorption efficiency |
MSE, R2, MAE |
pH of solution contributed the highest, and contact time contributed the least in describing the adsorption dynamics of lead ions on rice straw-based nanocellulose fibers |
144
|
ANN |
Contact time, initial arsenic concentration pH, and adsorbent dose |
Sorption efficiency |
MSE, R2 |
The pH and volume of solution had the most and least degree of impact, respectively, on As(III) and As(V) removal using Leucaena leucocephala seed powder |
145
|
ANN |
Bias, pH, the dose of alginate nanoparticles, and contact time |
Sorption efficiency |
SSE, RE and R2 |
ANN model showed good predictive performance of Ni(II) and Co(II) removal using alginate nanoparticles |
55
|
ANN |
Biomass dose, concentration, and time |
Sorption efficiency |
MSE |
Initial concentration of adsorbate strongly affected the Cr(III) sorption efficiency, whereas treatment time influenced the Cr(VI) sorption on nanocrystalline cellulose |
70
|
ANN |
No. of adsorbents, adsorbent weights, pH, contact time, and ion concentration |
Sorption efficiency |
AARE, SD, MSE and R |
ANN results showed better predictive performance for all the biomaterials, namely, coconut shell, neem leaves, hyacinth toots, rice husk, rice bran, rice straw, neem bark, and sawdust of teakwood |
146
|
ANN |
Residence time, ion concentration, adsorbent weight, pH and temperature |
Adsorption efficiency and final pH |
R
|
ANN response showed excellent agreement with the observed Ur (VI) adsorption results using polyacrylonitrile grafted potato starch |
79
|
ANN |
No. of adsorbents, time, flow rate, bed height, and initial concentration |
Removal efficiency |
AARE, SD, MSE and R |
ANN with a single hidden layer predicts Pb(II) sorption efficiency with reasonable accuracy on multiple biomaterials, i.e. coconut shells, neem leaves, hyacinth roots, and rice wastes |
147
|
ANN |
pH, residence time, inoculum size and initial arsenic concentration |
Removal efficiency |
R
2, MSE |
The distribution of model results closely fitted the As(III) and As(V) experimental data points using algal biomass, i.e. Botryococcus braunii |
148
|
ANN |
Adsorbent weight, pH, ion concentration, and residence time |
Removal efficiency |
R
2
|
ANN supplemented experimental results in a better explanation of Hg(II) adsorption on Sargassum bevanom biomass |
149
|
ANN |
Adsorbent weight, residence time, and ion concentration |
Adsorption efficiency |
R
2, MSE |
The excellent values of R2 (>0.99) showed the precision of ANN in predicting the adsorption of Pb(II), Cd(II) and Ni(II) on functionalized wood pulp |
81
|
ANN, RSM |
Residence time, adsorbent weight, and ion concentration |
Adsorption efficiency |
R
2, RMSE |
ANN (3-9-2) accurately predicted the removal of Cu(II) and Pb(II) using nanocomposites derived from rice straw and Fe3O4 nanoparticles (R2 = 0.95–0.99, RMSE = 0.95–1.87) with a negligible residual standard error of 0.02% as against RSM output |
57
|
ANN |
No. of adsorbents, adsorbent weight, ion concentration, pH adsorbent dose, time, initial concentration of ion and pH |
Removal efficiency |
R, AARE, SD and MSE |
ANN was proved to be the best predictive model for Cr(VI) eradication from wastewater using multiple biosorbents, i.e. coconut shell powder, bamboo leaf, garlic peel, acid-treated rubber leaf, onion peel, mango, and jackfruit leaf |
150
|
ANN |
Bed depth, effluent concentration rate, flow rate, pH, time, and initial metal concentration |
Removal efficiency |
AARE, SD, MSE, R and χ2 |
ANN response indicates its usefulness for predicting Cu(II) removal from wastewater using peanut and almond shells in a column adsorption system |
151
|
ANN |
Adsorbent weight, ion concentration, agitation speed and pH |
Removal efficiency |
R
2
|
ANN was found to predict the Cd(II) removal using Spirulina (Arthrospira) sp. with the best coefficient of determination |
152
|
ANN |
Residence time, adsorbent weight, ion concentration, particle size and pH |
Adsorption efficiency |
R
2
|
The ANN results predicted Cd(II) adsorption on gossypium barbadense waste with reasonable accuracy at epoch = 6 |
91
|
ANN |
Particle size, ion concentration, temperature, and pH |
Adsorption efficiency |
R
2
|
ANN results predicted Cd(II) adsorption on moringa oleifera seed accurately |
153
|
ANN |
Walnut shell–rice husk ratio, calcination duration and temperature |
Sorption efficiency |
R
2
|
Adsorption of Cd on nano-magnetic walnut shell–rice husk |
154
|
ANN, RSM |
Residence time, ion concentration, particle size, pH, and adsorbent weight |
Removal efficiency |
R
2, MSE, SD |
The study found an enormous potential of ANN over the quadratic mathematical model (deviation ∼16.3%) to forecast Ni(II) adsorption on potamogeton pectinatus closer to the experimental response (R2 = 0.9714, MSE = 1.4). Also, initial ion concentration has the highest and particle size has the most negligible influence on removal efficiency |
155
|
ANN, RSM |
Metal concentration, pH, adsorbent weight, and temperature |
Removal efficiency |
R
2
|
The study reported that the ANN model (4-4-1) performed well (R2 = 0.9971) for the Cr(VI) adsorption on cyanobacterial biomass with diminished error values against RSM |
48
|
ANN |
Adsorption dose, ion concentration and pH |
Removal efficiency |
R
2
|
The chromium adsorption on Borassus flabellifer coir was modelled by ANN with a high coefficient of determination |
156
|
ANN, RSM |
Adsorption dose, ion concentration and pH |
Removal efficiency |
R
2, RMSE |
The adsorptive removal of chromium using Flabellifer coir powder and Ragi husk powder was better simulated using the ANN scheme |
157
|
ANN, MLR |
Ion concentration, pH, residence time, and temperature |
Adsorption capacity |
R
2, RMSE |
The dataset of chromium removal using maize bran was better modelled using the ANN framework |
158
|
ANN |
Adsorbent dosage, contact time, temperature, pH, metal ion concentration |
Removal efficiency |
R
2, MSE |
An excellent fit between ANN output and experimental results was achieved in 188 iterations for As(III) adsorption on Bacillus thuringiensis strain WS3 |
159
|
ANN |
Temperature, ion concentration, reaction time and adsorbent weight |
Removal efficiency |
R
|
The cadmium removal efficiency using activated oak waste showed a high correlation (>0.999) for the observed data with a negligible deviation |
64
|
ANN |
Initial metal ion concentration, time, adsorbent dose, and pH |
Removal efficiency |
MRE (%), MSE and R2 |
ANN proved to be a valuable approach for modelling Cr(VI) biosorption using date palm fiber with a high coefficient of determination |
160
|
ANN, MLR |
pH, residence time, ion concentration and adsorbent weight |
Removal efficiency |
R
2
|
ANN showed better prediction potential (R2 = 0.99) for Pb(II) removal using a carboxylate-functionalized walnut shell over conventional models |
75
|
ANN |
Temperature, pH, agitation rate, adsorbent dose, and contact time |
Removal efficiency |
R
2, MSE |
The ANN architecture predicted chromium adsorption onto iron-doped rice husk more accurately than rice husk |
71
|
ANN |
pH, residence time, T-Fe3O4 nanocomposite dosage, and initial concentration |
Removal efficiency |
MAE, RMSE |
ANN satisfactorily assisted in extracting the knowledge about the adsorption of lead ions on iron nanocomposites derived from Tangerine bio-waste with a small experimental data set |
85
|
ANN |
Adsorbent dose, bed depth, flow rate, pH, initial concentration, particle size, and treatment time |
Adsorption capacity |
R
2, RMSE |
Adsorption time and bed depth were found to be the most and most minor influential parameters affecting Cu(II) adsorption on sunflower shells |
161
|
ANN |
Adsorbent dose, bed depth, flow rate, pH, initial concentration, particle size, and treatment time |
Adsorption capacity |
R
2, RMSE |
Adsorption time and pH were found to be the most and least influential parameters affecting Co(II) uptake on sunflower shells |
58
|
ANN, RSM |
Temperature, adsorbent weight, contact time and pH |
Adsorption capacity |
R
2, RMSE |
ANN proved to be a helpful tool for predicting Zn(II) adsorption from leachate (R2 = 0.99, RMSE = 0.0029) on hazelnut shell |
162
|
ANN, RSM |
pH, temperature, ion concentration, biomass weight, bed depth and flow rate |
Adsorption capacity |
R
2
|
ANN response indicated its potential to simulate arsenic ions adsorption on rice polish in batch and column mode |
138
|
ANN, RSM |
pH, adsorbent dose, and temperature |
Adsorption capacity |
R
2, RMSE |
ANN closely fitted with the arsenic adsorption results using black cumin over the RSM model |
163
|
ANN |
Ion concentration volume, residence time, pH and adsorbent weight |
Adsorption efficiency |
RMS, R2 |
ANN best predicted the effect of interactions among multiple variables on the Pb(II) adsorption capacity using bacillus subtilis beads |
164
|
ANN |
Metal ion concentration, operating temperature, pH, agitation time, particle size and contact time |
Adsorption capacity |
R
2, MSE |
The ANN displayed a compelling correlation between the predicted output and experimental response for cadmium adsorption on valonia tannin resin |
165
|
ANN, RSM |
Adsorbent weight, ion concentration and pH |
Adsorption capacity |
R
2, MSE |
A high R2 value confirmed the closeness between the predicted and observed values. The study reported the superiority of ANN over RSM in predicting Cu(II) adsorption capacity of flax meal |
47
|
ANN, RSM |
pH, temperature, residence time and ion concentration |
Adsorption capacity and removal efficiency |
R
2, SSE and ARE |
ANN topology agreed excellently with the experimental data (R2 ∼ 1) at low average relative errors of 1.032 and 0.056, corresponding to nickel removal efficiency and uptake capacity using alginate composite beads |
59
|
ANN |
pH, temperature, ion concentration and residence time |
Adsorption capacity |
R
2
|
Neural architecture predicted the cobalt adsorption capacity of alginate-based nanocomposites nearer to the experimental observation |
58
|
ANN |
Adsorbent weight, ion concentration and pH |
Adsorption capacity |
R
2
|
ANN gave highly consistent results with the experimental zinc adsorption capacity of peanut shells |
166
|
ANN |
Ion concentration, residence time, pH and adsorbent weight |
Adsorption capacity |
R
2, MSE |
ANN excellently estimated the potential of gundelia tournefortii to remove Pb(II) from synthetic wastewater |
167
|
ANN, MLR |
pH, nanoparticles mass, contact time, and initial concentration |
Adsorption capacity |
R
2, MSE |
ANN accurately predicted simultaneous adsorption of the nickel and cobalt from wastewater using carboxymethyl-chitosan-bounded Fe3O4 nanoparticles. In addition, the study documented the superiority of ANN over MLR in predicting adsorption with the most negligible variation in error |
99
|
ANN, MLR |
Temperature, ion concentration, adsorbent weight, contact time and pH |
Adsorption capacity |
R
2, MSE |
The ANN showed a firm fit with copper uptake capacity of raw guideline tournefortii results compared with conventional regression results |
168
|
ANN |
Equilibrium concentrations of the metal ions |
Adsorption capacity |
F
obj
|
ANN response of cadmium and zinc adsorption on sargassum filipendula fitted better than the classical adsorption models |
169
|
ANN, RSM |
Equilibrium concentrations of metal ions |
Maximum adsorption capacity |
R
2, MRE |
ANN model response closely fitted with experimental isotherm points of Cr(VI), Zn(II) and Cu(II), adsorption on chitosan foamed structure, indicating good correctness |
61
|
ANN |
Adsorbent characteristics, metal ion properties, experimental isotherm and kinetics data |
Kinetics and isotherm adsorption capacity |
R
|
ANN that included both sorbent characteristics and metal ion properties as input variables produced the best results for Pb(II), Zn(II), Ni(II), and Cd(II) adsorption on cellulosic wastes (from jacaranda, nutshell, and plum kernels) |
92
|
ANFIS |
Ion concentration, adsorbent weight, hydroxyapatite concentration, pH, temperature, shaker velocity and time |
Adsorption capacity |
R
2, MSE |
The ANFIS structure predicted the Pb(II) removal using hydroxy-apatite/chitosan composites with high accuracy |
60
|
ANFIS, MLR |
pH, initial concentration of copper, amount of date palm seeds |
Adsorption capacity |
R
2, MSE |
The results indicated a greater potential of ANFIS to deal with copper adsorption data on date palm seeds in relation to the regression model |
170
|
ANN, ANFIS |
pH, temperature ion and concentration |
Removal efficiency |
R
2
|
The high R2 (∼0.99) indicated the applicability of both the models in the prediction of the mercury removal using vibrio parahaemolyticus PG02 |
171
|
ANFIS, RSM |
Ion concentration, pH, and concentration of treatment solution |
Adsorption capacity |
R
2, SD |
ANFIS predicted the maximum Pb(II) uptake with less than a 5% error deviation from the experimental values. In contrast, FFD showed a relatively weak prediction of biosorption capacity, chemically treated olive stone |
84
|
ANFIS |
Metal ion concentrations, wheat straw granulometry, temperature, contact time |
Adsorption capacity |
R
2, RMSE |
The ANFIS architecture, after 15 iterations, showed high R2 between the predicted and experimental results of Cr(VI) and Cu(II) adsorption on the wheat straw with diminished root means square error values |
80
|
ANFIS |
Contact time, nickel and cadmium ions concentration, adsorbent dose, pH, and particle size of adsorbent |
Removal efficiency |
R
2, RMSE |
The results indicated a relatively more significant influence of pH and ion concentration on the Ni(II) and cadmium(II) removal efficiency using Typha domingensis |
172
|
ANFIS |
Adsorbent weight, residence time, pH, and ion concentration |
Adsorption efficiency |
R
2, RMSE |
The biomass dose and pH were depicted as the most influential process parameters which affected the Cr(VI) sorption efficiency for date palm leaves and broad bean shoots, respectively |
173
|
ANN, ANFIS, 23factorial design |
Initial ion concentration, adsorbent dose, and pH |
Adsorption efficiency |
R
2, RMSE |
The initial ion concentration with the least training and checking error controlled the cadmium adsorption on rice straw, followed by the pH and adsorbent dose. The full factorial design also complemented the ANFIS results |
129
|
ANN-GA, RSM |
Temperature, ion concentration, pH, and residence time |
Adsorption efficiency |
R
|
The optimization results revealed the potential of ANN-GA (99.86%) to represent and explain actual Hg(II) adsorption data using yeast against the RSM (97.75%) model |
45
|
ANN-GA |
Contact time, pH, particle size and mixing speed |
Removal efficiency |
R
2, RE |
A residual error of 1.3% was found between the observed Cu(II) adsorption on banana floret and model results, which validated the ANN-GA hybrid structure. In addition, the pH and time have the most significant influence on the removal efficiency |
72
|
ANN-GA |
Initial metal ion concentration and temperature |
Removal efficiency and change in gibbs free energy |
R, MSE |
The optimization with GA resulted in a 92.9% of Pb(II) removal using thiosemicarbazide modified chitosan and −5 kJ mol−1 of gibbs energy change at 55 °C and 10 ppm of initial ion concentration |
62
|
ANN-GA, MNLR |
pH, bed depth, number of adsorbents, time, and flow rate |
Removal efficiency |
R, AARE, MSE and SD |
The high correlation coefficient (R = 0.9965 to 0.9992) confirmed the model prediction accuracy of the chromium removal using mango, jackfruit, and rubber leaves prediction over MNLR. |
174
|
ANN-GA |
Adsorbent dose, pH, and contact time |
Removal efficiency |
R, MSE |
The statistical indices hold minor variance (<5%) between the experimental and ANN output, indicating the great potential of the hybrid model to simulate thorium adsorption on chitosan-derived TiO2 composites |
175
|
ANN-GA, Taguchi design ANN-SA |
Bed depth, flow rate, and ion concentration |
Removal efficiency |
R, RMSE |
The results indicated better productivity of GA when compared with the Taguchi design, and SA optimizer outputs to model chromium removal using alginate immobilized Sargassum sp. |
176
|
ANN-GWO, ANN-BR-GWO |
Temperature, pH, ion concentration, and adsorbent dose |
Adsorption efficiency |
R
2, RMSE |
FFNN model (trained with Bayesian-regularization (BR) algorithm) and optimized with GWO efficiently modelled the adsorption of Pb(II) and Co(II) ions on Rafsanjan pistachio shell |
101
|
ANN-COA |
Elution solvent, flow rate, the concentration of PAN [1-(2-pyridylazo)-2-naphthol], adsorbent dose, pH, and volume of elution solvent |
Adsorption efficiency |
R
2, RMSE |
The ANN-COA model predicted the output with a small residual error of 1.3% and R2 value of 0.9954 for the uranium adsorption using zinc oxide nanoparticles–chitosan |
177
|
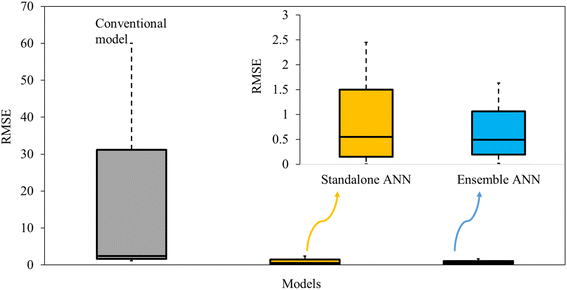 |
| Fig. 5 Modelling error occurred in conventional, standalone and ensemble models for simulating adsorption on biomaterials (figure generated using the data from Section S5.6†). | |
The authors acknowledged the capacity of the developed ANN models with metaheuristic optimizers to simulate the adsorption of metal ions on biomaterials. Yet, there is a need to explore these optimizers with a varied dataset on different metal pollutants with a clear explanation of the methodological phase for developing the research knowledge and comparing their capacity to deal with the stochastic, nonlinear complex data.
5. ANN frameworks for hybridizing classical adsorption models
5.1 Hybridized-ANN models
The process model development is an integral part of water treatment via adsorption. The classical means of modeling adsorption include calculating parameters related to isotherm, kinetics and thermodynamics through experimental values obtained at optimum conditions. However, the One Variable At a Time (OVAT) approach is applied to independently optimize the individual effect of adsorption variables such as contact time, pH, temperature, adsorbent dose and initial metals concentration.178–181 Considering the impact of individual variables, analytical error and uncertainty associated with the traditional experimental approach, different AI models are used to improve the mathematical representations of adsorption process models. In this regard, Rodríguez-Romero et al., 2020 hybridized the ANN with classical isotherm and kinetic equations to improve the arsenic adsorption capacity of carbon-enriched biomaterial.211 The authors obtained the ANN-Langmuir model from the classical Langmuir functionality using initial metal concentration, pH and temperature as input parameters with sigmoid activation functions. Likewise, the other hybridized models were also obtained. The hybridized ANNs outperformed the traditional kinetics and isotherm models as portrayed in Fig. 6 where it is clear that the hybrid ANNs are less susceptible to error.
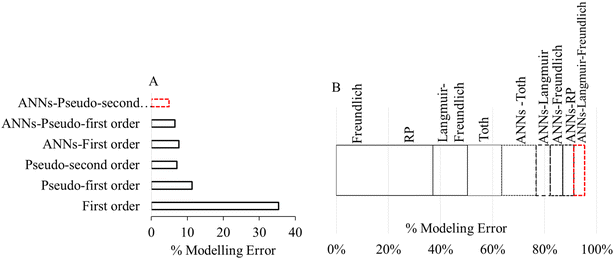 |
| Fig. 6 Percent error in hybrid-ANN and traditional adsorption models using (A) kinetics, (b) isotherms (reproduced from ref. 102 with permission from [Elsevier], copyright [2020]). | |
Only a few studies report using such a modelling strategy to remove fluoride ions, indicating a novel area of research.182–184 ANN tools are easily used because they can establish dependencies and correlations between multiple variables. ANN architectures with equilibrium concentrations of metal toxins and temperature as net entrance data and metals adsorbed as the exit variables are processed to capture the best fit for the single and multicomponent adsorption process.53 It is important to remark that similar ANN frameworks can be used to design multicomponent adsorption processes for metal removal at different operating conditions. The utility of AI has expanded with the hybridization of ANN with isotherm or kinetic equations to acquire pertinent adsorption parameters that are not possible using a standard model.185
Apart from isotherm and kinetics, estimating thermodynamic parameters of the adsorption process using ANN is an emerging field of research. Recently, Zaferani et al., 2019 implemented ANN to recognize the standard Gibbs free energy changes (ΔG) related to Pb(II) adsorption process based on the changing temperature and initial metal concentration. The authors investigated different structures of ANN for modelling ΔG and metal removal efficiency.62 The results revealed that the minimum value of ΔG (−6 kJ K−1) occurred at the highest temperature (55 °C) and the lowest initial Pb(II) concentration (10 ppm). The negative Gibbs free energy verified the spontaneity of the adsorption process. Limited studies explored the possibility of analyzing thermodynamics aspects of the adsorption process using ANN-framework. Much research is needed to create intelligent models that can predict the nature and viability of the adsorption process.
It should be emphasized that the parameters of conventional adsorption models are established by fitting specific experimental data. This indicates that the parameters of conventional adsorption models are constant for a given range of experimental conditions (Tables 1–3). On the other hand, ANN model parameters are derived through concurrent regression of all experimental observations.186 Thus, hybrid adsorption model parameters are treated as nonlinear functions of the input variables used to train the artificial neural network, enhancing their adaptability and data correlation capabilities. These results demonstrated that using ANNs to estimate analytical adsorption equations parameters significantly improved modelling results.
5.2 Multicomponent adsorption
Wastewater is a matrix of multiple pollutants; therefore, developing a single technique for concurrent extraction of coexisting metallic impurities is critical. However, fabricating an adsorbent that considers the characteristics of all contaminants whose removal is required is challenging.100,187 The process of multiple metal adsorption on biomaterials is usually studied in the column set-up in the laboratory and analyzed using breakthrough curves; but the existence of various metal pollutants in the feed makes modeling of breakthrough curves complex due to the pollutants' antagonistic, synergistic, and noninteractive tendencies.188 Advanced models that illustrate multicomponent adsorption and the associated physics are part of the evolving research.189 In this context, for the first time, Pauletto et al., 2020 implemented ANN with a Bayesian regularization algorithm to investigate the antagonistic and synergistic effects during the adsorption of dye (MB) and metals (Co(II)), Ni(II)) in single, binary and ternary mixtures on ultrasound modified chitin.212 They optimized the input parameters (i.e. temperature and initial pollutants concentration) to simultaneously forecast the uptake capacity of individual adsorbates from a multicomponent system. The ANN-based simulations indicated that the optimized network of single component systems can be applied to model the equilibrium adsorption of cobalt (Co(II)), nickel (Ni(II), and methylene blue (MB) effectively (R2 = 0.99) in multicomponent systems.
Simulating biomaterial systems for multi-metals adsorption is an emerging field of study that has the potential to computationally design cost-effective adsorbents with tailored properties to effectively treat industrial effluents without the need for multiple stages involved in adsorption system design. Other advantages of this technique include cost reduction and time savings to generate high-efficiency adsorption systems that simultaneously treat diverse water pollutants. The future studies can analyse the application of ANN integrated with nature and human-inspired metaheuristic optimization algorithms for the adsorptive eviction of multiple species from BMs.
6. Sensitivity analysis
The ANN frameworks' limitations is their inability to comprehend the physico-chemistry behind the adsorption behaviour; therefore, their uninformed application to a sorption system without paying attention to the physicochemical characteristics could result in dubious outcomes. To address this concern, researchers used a sensitivity analysis of ANN to determine the impact of experimental factors on the adsorption of metal ions. A sensitivity analysis of the developed ANN allows assessment of the input attributes as per their impact on the output response. In the literature, the Weights method has usually been employed to examine the sensitivity of the adsorption process and identify the most significant parameters that influence the adsorption performance (ANN output).190 The mathematical presentation of network weights for determining the relative weights of input parameters is given in ESI, Section S6.†
In the biomaterial adsorption system, pH, initial concentration of adsorbate, and contact time were the most critical process variables that impacted the metal adsorption on biomaterials. Since the pH alters the ionic strength and affects the ionization of metals onto biomaterials, the contact time between adsorbate and adsorbent affects the available active sites,213,214 whereas the concentration of metal affects the interaction of metal ions with the available binding sites. Furthermore, it was found that the quantity and quality of the biomaterial adsorbed significantly impacted the effectiveness of the adsorption process.73 The medium of aqueous solutions also affected the adsorptive removal of metal ions. For example, the presence of organic matter and its derivatives or salinity might change the biomaterials' surface characteristics and block the metals' adsorption on biomaterials. Considering the wastewater treatment plants, the quantity of biomaterial required for successful adsorption of metal adsorbates at a fixed initial concentration serves importance from an economic perspective. The process cost will be lowered when sufficient adsorption is attained with a small biomaterial dose. Since the selling cost of natural biomaterials is very low, but their excessive usage can increase their waste disposal costs, while inefficient use can increase the process cost. Thus, understanding how operating variables affect the adsorption using ANN approach will aid in the appropriate process design, scale-up and optimization of an industrial adsorption process. In addition, implementing neural networks will process monitoring and control, save time, and lower costs.
Besides determining the contribution of single input parameters, researchers studied the conjugate effects of multiple input parameters on ANN prediction. Fig. 7 shows the significant decrement in the prediction error (MSE) for copper and mercury removal with the increasing combinations of input parameters. Fig. 7 shows that as the number of input variables in the group rose, the values of MSE decreased because of the strong effect of all parameters on adsorption capacity. Although the influence of process variables has been explored widely, the contribution of biomaterial characteristics in terms of their lignin–cellulose–hemicellulose content, pore size distribution, particle size and surface area on the predictive ANN response has not been fully explored. The information about the influence of the above-mentioned attributes can benefit technologists and engineers in selecting and designing adsorption systems.
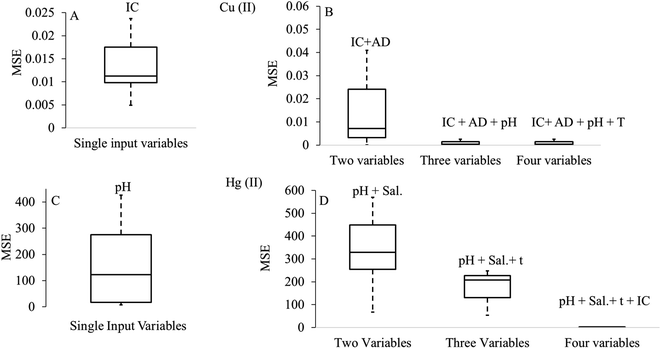 |
| Fig. 7 Influence of single and combinations of input variables on the ANN response. *AD = adsorbent dose, IC = initial metal ion concentration, T = temperature, CT = contact time and Sal. = salinity of aqueous medium. (A and B) were generated using the data obtained from ref. 168 with permission from [Elsevier], copyright [2019], while (C and D) were generated using the data obtained from ref. 77 with permission from [Springer Nature], copyright [2018]. | |
The sensitivity analysis was primarily reported in studies focusing on standalone ANN frameworks, which followed a gradient-based optimization scheme (details of studies in Table 5). Thus a comparative analysis of metaheuristic-based ensemble models and ANN can be carried out in future to predict the relative contribution of adsorption variables on the metal adsorption efficacy of BMs. Apart from Weights methods, there are other methods of calculating sensitivity and feature importance, e.g., partial derivate algorithm,191 input perturbation algorithm,192 profile193 and stepwise method.194 There is a need to test the sensitivity of ANN response using different approaches to determine the optimal sensitivity criteria, which could be the focus of future studies.
7. Challenges and advancements in ANN technology for the removal of metals
The main disadvantage of ANN is its black box and empirical character. The connection link between neurons is denoted by weights, which are difficult to represent mathematically. The contribution of a specific input variable to the outcome of ANN is identified based on its numeric value and sign of the associated weight. The higher the weight value, the greater the independent variable's contribution to the expected response. The effects of negative weights on neurons are conflicting. Thus, positive outcomes have a synergistic impact on the neurons, boosting the value of the response, whereas negative outcomes have the opposite effect and decrease the expected value of the output (Section 6).
Although the above-discussed sections demonstrate ANN as a superior approach to the traditional models due to less formal statistical training requirements, detection of complex nonlinear relationships and all possible interactions between the variables, there is a need to develop novel strategies that can provide in-depth analysis of adsorption phenomena in terms of surface interactions and associated adsorption energy. Recent research by Fagundez et al., 2021 offered novel hybrid ANN-isotherms for simultaneous estimation of isotherm and thermodynamics parameters to predict metal uptake capacity and associated thermodynamic parameters of various zeolites under different temperature conditions.215 The utility of molecular simulations combined with ANN has recently been reported as a valuable modelling strategy to gain insights into the mechanism of heavy metal adsorption on graphene nanocomposites with high accuracy.195 The computation models based on density functional theory (DFT) have been employed to determine the adsorption energies on solid surfaces. However, developing a neural network-based scheme that can predict surface energies on sorbents can assist in optimizing the relative stability of adsorbent surfaces for spontaneous adsorption of targeted metal pollutants on available active sites.196–199 In addition to developing new computational strategies, the researchers emphasize on meaningful experimental results to understand the equilibrium, saturation and regenerative potential of adsorption systems from a chemical science point of view.56,200
The possibility of overfitting is the second major issue that needs to be addressed by researchers while applying ANN algorithms to predict biomaterial systems' efficacy for wastewater treatments.91,92 To prevent the over-parameterization and over-training of the ANN system, researchers have advocated the implementation of emerging activation functions (e.g., SeLU, ReLU).201,202 Using simple models (e.g., AdaBoost) ensures the generalizability of output in the small dataset.203
It is commonly recognized that a smaller error value is better for optimum network learning; however, it may be possible that the network training stops due to getting stuck in local minima. Such situations call for hybridizing the ANN framework with novel evolutionary algorithms. Recently, Ke et al., 2021 proposed novel ANN-based-surrogate models (i.e., BA-ANN, SVM-ANN, RF-ANN, M5Tree-GP, M5Tree-ANN and GP-ANN) for predicting metal removal efficiency based on characteristics of carbonaceous materials, source of metal and environmental conditions.107 The particle swarm optimizer (PSO) has been coupled with ANN to predict the dye and copper removal efficiency using graphene oxide-based nanocomposites and pomelo-peel-based carbonaceous material.204,205
8. Prospective scope
❖ Based on the reviewed literature on metal ion modelling using ANN, estimating the absorption capability of biomaterials other than polysaccharide-derived materials requires additional research.
❖ Models discussed were prepared and assessed based on metals present in synthetic solutions. The natural wastewater or water system is a complex matrix where various pollutants co-exist. The influence of co-existing metal pollutants in natural systems on the adsorption behaviour via ANN has not been fully explored. Thus, there is a greater scope of research in this domain.
❖ Although ANN-based models provided a better fit than static adsorption models, the literature lacked sufficient research on the development of ANN systems that can best describe the breakthrough curves against dynamic adsorption models such as Thomas, Bohart–Adams and Yoon–Nelson models. Future research should demonstrate the performance of neural networks against dynamic adsorption models to forecast breakthroughs.
❖ As per the principles of circular economy, the reuse and recyclability of biomaterial systems are essential. A vast development of ANN models has predicted pollutant removal efficiency; however, little attention has yet been given to developing intelligent systems that can optimize the dose of eluting agents for maximum regeneration of adsorbent materials. Modelling desorption processes can allow further utilization and effective management of spent adsorbent materials.
❖ ANNs have been chiefly applied to model the removal of heavy metals and dyes. But the potential of bioadsorbents to treat emerging contaminants such as per- and polyfluoroalkyl substances (PFAS) and rare earth metals (e.g., neodymium, cerium, and lanthanum) via adsorption needs attention for advancements in adsorption systems design.
❖ Environmental remediation occurs in different climate conditions. Also, the wastewater being processed for reuse can be at varying temperatures. In such situations, modelling thermodynamic aspects of the adsorption process can give crucial information related to the efficacy of biomaterials and the feasibility of adsorption phenomena for a broad spectrum of temperatures.
❖ ANN results need to be compared with the latest machine learning models, which are not yet explored for simulating adsorption processes such as super learning, decision tree, deep learning, and data mining. These models have provided promising solutions for various problems related to environmental engineering; however, they are yet to be assessed for wastewater treatment applications.
9. Conclusion
Metal pollution treatment is essential to prevent waste metals' bioaccumulation, environmental pollution, and soil degradation. Biomaterials act as a versatile and cost-effective system for removing metals from wastewater. Different chemical and physical modification methods of natural biomaterials can improve their metal adsorption efficiency, but conventional laboratory-based research has yet to describe multicomponent adsorption systems comprehensively. An artificial neural network can automate the adsorption process to optimize process variables and adsorbent fabrication pathways for increasing metal removal efficiency. Furthermore, ANN frameworks can generate hybrid isotherm and kinetic models that minimize error and accurately model an efficient, rapid, and cost-effective multicomponent system for metal removal. The application of ANN-based models leads to a better understanding of biomaterials' efficiency, energy, time and economic benefits. The thermodynamic aspects of metal adsorption to improve environmental water quality and applications in water reuse is a burgeoning field of research. The challenges of ANN can be briefly summarized as a) collecting experimentally characterized data to filter and identify specific metal contaminants in the complex matrix of water; and b) establishing ensemble models to assist in solving local minima problems, thereby improving the prediction efficiency of ANN. The intervention of ANN demonstrated robustness and rigour in simulating adsorptive eviction of metal ions using BMs, which could be expanded for other organic and emergent pollutants.
Disclaimer
The research presented was not performed or funded by EPA and was not subject to EPA's quality system requirements. The views expressed in this article are those of the author(s) and do not necessarily represent the views or the policies of the US Environmental Protection Agency.
Author contributions
Amrita Nighojkar: conceptualization, investigation, data analysis, visualization, writing – original draft; Karl Zimmermann: data analysis, writing – review & editing; Mohamed Ateia: visualization, writing – review & editing; Benoit Barbeau: writing – review & editing; Madjid Mohseni: writing – review & editing; Satheesh Krishnamurthy: writing – review & editing; Fuhar Dixit: investigation, data analysis, visualization, writing – original draft; Balasubramanian Kandasubramanian: conceptualization, supervision, project administration.
Conflicts of interest
The authors declare that they have no known competing financial interests or personal relationships that could have appeared to influence the work reported in this paper.
Abbreviations
AARE | Absolute average relative error |
AD | Adsorbent dose |
AI | Artificial intelligence |
ANFIS | Adaptive neuro-fuzzy inference system |
ANN | Artificial neural networks |
ANN-COA | Cuckoo optimized hybridized neural network |
ANN-GA | Genetic algorithm hybridized neural network |
ANN-GWOA | Wolf optimized hybridized neural network |
ANN-SA | Simulated annealing hybridized neural network |
ARPE | Average relative percentage error |
BD | Bed depth |
BR | Bayesian regularization |
C
e
| Equilibrium concentration |
COA | Cuckoo optimization algorithm |
CT | Contact time |
DA | DIRECT algorithm |
FFNN | Feed forward neural network |
FIS | Fuzzy inference system |
FR | Flow rate |
GA | Genetic algorithm |
GD | Gradient descent |
GWO | Grey Wolf optimizer |
GWOA | Grey Wolf optimization algorithm |
IC | Initial metal ion concentration |
LM | Levenberg-Marquardt |
MAE | Mean average error |
MAPE | Mean absolute percentage error |
MNLR | Nonlinear multiple linear regression |
MRE | Mean relative error |
MSE | Mean square error |
NLR | Nonlinear regression |
PS | Particle size |
R
| Correlation coefficient |
R
2
| Coefficient of determination |
r% Ads | Correlation coefficient for % adsorption |
R
2
| Coefficient of determination |
RMSE | Root mean square error |
Rprop | Resilient backpropagation |
RSM | Response surface methodology |
SA | SIMPLEX algorithm |
SCG | Scaled conjugate gradient |
SD | Standard deviation |
SDR | Standard deviation ratio |
SSE | Sum of square error |
T
| Temperature |
TAAE% | Total mean of absolute error% |
VS | Volume of solution |
y
m
| Predicted value |
y
e
| Experimental value |
Acknowledgements
Fuhar Dixit and Karl Zimmermann are Natural Sciences and Engineering Council of Canada's Vanier Scholars. The authors would further like to acknowledge Sharan Vasvani (Faculty of Computing Science, Simon Fraser University, Canada) for reviewing the manuscript and providing valuable feedback.
References
-
UN-Water, Scarcity, UN-Water, https://www.unwater.org/water-facts/scarcity/, accessed July 22, 2022 Search PubMed.
- S. Bolisetty, M. Peydayesh and R. Mezzenga, Sustainable technologies for water purification from heavy metals: review and analysis, Chem. Soc. Rev., 2019, 48, 463–487 RSC.
- R. Chakraborty, A. Asthana, A. K. Singh, B. Jain and A. B. H. Susan, Adsorption of heavy metal ions by various low-cost adsorbents: a review, Int. J. Environ. Anal. Chem., 2022, 102, 342–379 CrossRef CAS.
- R. Rashid, I. Shafiq, P. Akhter, M. J. Iqbal and M. Hussain, A state-of-the-art review on wastewater treatment techniques: the effectiveness of adsorption method, Environ. Sci. Pollut. Res., 2021, 28, 9050–9066, DOI:10.1007/s11356-021-12395-x.
- A. Zehra, M. Meena, P. Swapnil, N. A. Raytekar and R. S. Upadhyay, Sustainable approaches to remove heavy metals from water, Microb. Biotechnol. Basic Res. Appl., 2020, 127–146 Search PubMed.
- N. L. Torad, A. Takahashi, M. Kawakami, T. Kawamoto and H. Tanaka, Decontamination of very dilute Cs in seawater by a coagulation–precipitation method using a nanoparticle slurry of copper hexacyanoferrate, Environ. Sci. Water Res. Technol., 2019, 5, 1328–1338, 10.1039/C9EW00259F.
- A. Nighojkar, V. K. Sangal, F. Dixit and K. Balasubramanian, Sustainable conversion of saturated adsorbents (SAs) from wastewater into value-added products: future prospects and challenges with toxic per- and polyfluoroalkyl substances (PFAS), Environ. Sci. Pollut. Res., 2022, 29, 78207–78227, DOI:10.1007/s11356-022-23166-7.
- V. U. Kavitha and B. Kandasubramanian, Tannins for wastewater treatment, SN Appl. Sci., 2020, 2, 1–21, DOI:10.1007/s42452-020-2879-9.
- F. Dixit, K. Zimmermann, R. Dutta, N. J. Prakash, B. Barbeau, M. Mohseni and B. Kandasubramanian, Application of MXenes for water treatment and energy-efficient desalination: a review, J. Hazard. Mater., 2022, 423, 127050 CrossRef CAS PubMed.
- A. Purabgola, N. Mayilswamy and B. Kandasubramanian, Graphene-based TiO2 composites for photocatalysis & environmental remediation: synthesis and progress, Environ. Sci. Pollut. Res., 2022, 1–21 Search PubMed.
- K. V. Udayakumar, P. M. Gore and B. Kandasubramanian, Foamed materials for oil-water separation, Chem. Eng. J. Adv., 2021, 5, 100076 CrossRef CAS.
- M. N. Issac and B. Kandasubramanian, Review of manufacturing three-dimensional-printed membranes for water treatment, Environ. Sci. Pollut. Res., 2020, 27, 36091–36108 CrossRef CAS PubMed.
- P. M. Gore, P. Gawali, M. Naebe, X. Wang and B. Kandasubramanian, Polycarbonate and activated charcoal-engineered electrospun nanofibers for selective recovery of oil/solvent from oily wastewater, SN Appl. Sci., 2020, 2, 1–13 Search PubMed.
- A. K. Nighojkar, A. Vijay, A. Kumavat, S. Gupta, R. K. Satankar and A. Plappally, Use of marble and iron waste additives for enhancing arsenic and E. coli contaminant removal capacity and strength of porous clay ceramic materials for point of use drinking water treatment, Desalination Water Treat., 2019, 157, 290–302 CrossRef CAS.
- C. Shen, Y. Zhao, W. Li, Y. Yang, R. Liu and D. Morgen, Global profile of heavy metals and semimetals adsorption using drinking water treatment residual, Chem. Eng. J., 2019, 372, 1019–1027 CrossRef CAS.
- J. Febrianto, A. N. Kosasih, J. Sunarso, Y.-H. Ju, N. Indraswati and S. Ismadji, Equilibrium and kinetic studies in adsorption of heavy metals using biosorbent: a summary of recent studies, J. Hazard. Mater., 2009, 162, 616–645 CrossRef CAS PubMed.
- S. Rastogi and B. Kandasubramanian, Progressive trends in heavy metal ions and dyes adsorption using silk fibroin composites, Environ. Sci. Pollut. Res., 2020, 27, 210–237 CrossRef CAS PubMed.
- M. I. U. Hoque, Y. Yamauchi, R. Naidu, R. Holze, R. Saidur, Q. Qu, M. M. Rahman, N. L. Torad, M. S. A. Hossain and M. Kim, A facile synthesis of hematite nanorods from rice starch and their application to Pb (II) ions removal, ChemistrySelect, 2019, 4, 3730–3736 CrossRef CAS.
- N. L. Torad, R. Kanai, K. Ishikawa, R. Kamimura, T. Kawamoto and H. Tanaka, The development of a rapid monitoring method for radiocesium in seawater in the Fukushima region, Environ. Sci. Water Res. Technol., 2022, 8, 1547–1560, 10.1039/D2EW00211F.
- N. L. Torad, M. Hu, S. Ishihara, H. Sukegawa, A. A. Belik, M. Imura, K. Ariga, Y. Sakka and Y. Yamauchi, Direct Synthesis of MOF-Derived Nanoporous Carbon with Magnetic Co Nanoparticles toward Efficient Water Treatment, Small, 2014, 10, 2096–2107, DOI:10.1002/smll.201302910.
- P. Cheng, M. Kim, H. Lim, J. Lin, N. L. Torad, X. Zhang, Md. S. A. Hossain, C.-W. Wu, C. Wang, J. Na and Y. Yamauchi, A General Approach to Shaped MOF-Containing Aerogels toward Practical Water Treatment Application, Adv. Sustain. Syst., 2020, 4, 2000060, DOI:10.1002/adsu.202000060.
- A. Pillai and B. Kandasubramanian, Carbon xerogels for effluent treatment, J. Chem. Eng. Data, 2020, 65, 2255–2270 CrossRef CAS.
- A. K. Nighojkara, A. K. Agrawalb, B. Singhc, S. Guptaa, R. K. Satankara, J. M. Oommena, L. Davea, M. Sharifd, A. B. O. Soboyejoe and A. Plappallya, Establishing correlations among pore structure, surface roughness, compressive strength, and fracture toughness of ceramic water filters local to Rajasthan, India, Water Treat., 2019, 157, 332–341 Search PubMed.
- M. Abali, A. Ait Ichou, A. Zaghloul, M. Chiban, F. Sinan and M. Zerbet, Evaluation and improvement of the WWTP performance of an agricultural cooperative by adsorption on inert biomaterial: case of orthophosphate, nitrate and sulfate ions, Appl. Water Sci., 2022, 12, 1–9 CrossRef.
- A. Subash, M. Naebe, X. Wang and B. Kandasubramanian, Biopolymer – a sustainable and efficacious material system for effluent removal, J. Hazard. Mater., 2023, 443, 130168, DOI:10.1016/j.jhazmat.2022.130168.
- P. M. Gore, M. Naebe, X. Wang and B. Kandasubramanian, Nano-fluoro dispersion functionalized superhydrophobic degummed & waste silk fabric for sustained recovery of petroleum oils & organic solvents from wastewater, J. Hazard. Mater., 2022, 426, 127822 CrossRef CAS PubMed.
- N. Mayilswamy and B. Kandasubramanian, Green composites prepared from soy protein, polylactic acid (PLA), starch, cellulose, chitin: a review, Emerg. Mater., 2022, 5, 727–753, DOI:10.1007/s42247-022-00354-2.
-
P. M. Gore, M. Naebe, X. Wang and B. Kandasubramanian, Bioinspired and Natural Materials for Oil/Water Separation, in Oil− Water Mix. Emuls. Vol. 2 Adv. Mater. Sep. Treat., ACS Publications, 2022, pp. 107–123 Search PubMed.
- C. Zinge and B. Kandasubramanian, Nanocellulose based biodegradable polymers, Eur. Polym. J., 2020, 133, 109758 CrossRef CAS.
- P. M. Gore, M. Naebe, X. Wang and B. Kandasubramanian, Silk fibres exhibiting biodegradability & superhydrophobicity for recovery of petroleum oils from oily wastewater, J. Hazard. Mater., 2020, 389, 121823, DOI:10.1016/j.jhazmat.2019.121823.
-
A. Rajeswari, E. J. S. Christy and A. Pius, Biopolymer blends and composites: processing technologies and their properties for industrial applications, in Biopolym. Their Ind. Appl., Elsevier, 2021, pp. 105–147 Search PubMed.
-
A. E. Ali, Z. Z. Chowdhury, R. F. Rafique, R. Ikram, A. N. M. Faisal, S. Shibly, A. Barua, Y. A. Wahab and B. M. Jan, Science and Technology Roadmap for Adsorption of Metallic Contaminants from Aqueous Effluents Using Biopolymers and Its' Derivatives, in Adv. Ind. Wastewater Treat. Reclam. Water, Springer, 2022, pp. 165–196 Search PubMed.
- Z. Xiang, N. Tang, X. Jin and W. Gao, Fabrications and applications of hemicellulose-based bio-adsorbents, Carbohydr. Polym., 2021, 118945 Search PubMed.
- P. D. Bhalara, D. Punetha and K. Balasubramanian, Kinetic and isotherm analysis for selective thorium (IV) retrieval from aqueous environment using eco-friendly cellulose composite, Int. J. Environ. Sci. Technol., 2015, 12, 3095–3106 CrossRef CAS.
- S. Sharma, K. Balasubramanian and R. Arora, Adsorption of arsenic (V) ions onto cellulosic-ferric oxide system: kinetics and isotherm studies, Desalination Water Treat., 2016, 57, 9420–9436 CrossRef CAS.
- N. Singh and K. Balasubramanian, An effective technique for removal and recovery of uranium (VI) from aqueous solution using cellulose–camphor soot nanofibers, RSC Adv., 2014, 4, 27691–27701 RSC.
- K. Thakur, A. Rajhans and B. Kandasubramanian, Starch/PVA hydrogels for oil/water separation, Environ. Sci. Pollut. Res., 2019, 26, 32013–32028 CrossRef CAS PubMed.
- P. D. Bhalara, K. Balasubramanian and B. S. Banerjee, Spider–web textured electrospun composite of graphene for sorption of Hg (II) ions, Mater. Focus, 2015, 4, 154–163 CrossRef CAS.
- S. Rastogi and B. Kandasubramanian, Application of electrospun materials in water treatment, Electrospun Mater. Allied Appl., 2020, 151–183 Search PubMed.
- P. M. Gore, M. Naebe, X. Wang and B. Kandasubramanian, Progress in silk materials for integrated water treatments: Fabrication, modification and applications, Chem. Eng. J., 2019, 374, 437–470 CrossRef CAS.
- A. Rajhans, P. M. Gore, S. K. Siddique and B. Kandasubramanian, Ion-imprinted nanofibers of PVDF/1-butyl-3-methylimidazolium tetrafluoroborate for dynamic recovery of europium (III) ions from mimicked effluent, J. Environ. Chem. Eng., 2019, 7, 103068 CrossRef CAS.
- R. R. Gonte, G. Shelar and K. Balasubramanian, Polymer–agro-waste composites for removal of Congo red dye from wastewater: adsorption isotherms and kinetics, Desalination Water Treat., 2014, 52, 7797–7811 CrossRef CAS.
- N. Ali, A. Khan, S. Nawaz, M. Bilal, S. Malik, S. Badshah and H. M. Iqbal, Characterization and deployment of surface-engineered chitosan-triethylenetetramine nanocomposite hybrid nano-adsorbent for divalent cations decontamination, Int. J. Biol. Macromol., 2020, 152, 663–671 CrossRef CAS PubMed.
- M. H. Dehghani, R. R. Karri, E. C. Lima, A. H. Mahvi, S. Nazmara, A. M. Ghaedi, M. Fazlzadeh and S. Gholami, Regression and mathematical modeling of fluoride ion adsorption from contaminated water using a magnetic versatile biomaterial & chelating agent: insight on production & experimental approaches, mechanism and effects of potential interferers, J. Mol. Liq., 2020, 315, 113653 CrossRef CAS.
- E. A. Dil, M. Ghaedi, G. R. Ghezelbash, A. Asfaram, A. M. Ghaedi and F. Mehrabi, Modeling and optimization of Hg2+ ion biosorption by live yeast Yarrowia lipolytica 70562 from aqueous solutions under artificial neural network-genetic algorithm and response surface methodology: kinetic and equilibrium study, RSC Adv., 2016, 6, 54149–54161 RSC.
- S. Mohan, Y. Singh, D. K. Verma and S. H. Hasan, Synthesis of CuO nanoparticles through green route using citrus limon juice and its application as nanosorbent for Cr(VI) remediation: process optimization with RSM and ANN-GA based model, Process Saf. Environ. Prot., 2015, 96, 156–166, DOI:10.1016/j.psep.2015.05.005.
- D. Podstawczyk, A. Witek-Krowiak, A. Dawiec and A. Bhatnagar, Biosorption of copper (II) ions by flax meal: empirical modeling and process optimization by response surface methodology (RSM) and artificial neural network (ANN) simulation, Ecol. Eng., 2015, 83, 364–379 CrossRef.
- S. Sen, S. Nandi and S. Dutta, Application of RSM and ANN for optimization and modeling of biosorption of chromium (VI) using cyanobacterial biomass, Appl. Water Sci., 2018, 8, 1–12 CrossRef CAS.
- A. Asfaram, M. Ghaedi and G. R. Ghezelbash, Biosorption of Zn2+, Ni2+ and Co2+ from water samples onto Yarrowia lipolytica ISF7 using a response surface methodology, and analyzed by inductively coupled plasma optical emission spectrometry (ICP-OES), RSC Adv., 2016, 6, 23599–23610 RSC.
- D. S. P. Franco, F. A. Duarte, N. P. G. Salau and G. L. Dotto, Analysis of indium (III) adsorption from leachates of LCD screens using artificial neural networks (ANN) and adaptive neuro-fuzzy inference systems (ANIFS), J. Hazard. Mater., 2020, 384, 121137, DOI:10.1016/j.jhazmat.2019.121137.
- R. Gomez-Gonzalez, F. J. Cerino-Córdova, A. M. Garcia-León, E. Soto-Regalado, N. E. Davila-Guzman and J. J. Salazar-Rabago, Lead biosorption onto coffee grounds: Comparative analysis of several optimization techniques using equilibrium adsorption models and ANN, J. Taiwan Inst. Chem. Eng., 2016, 68, 201–210, DOI:10.1016/j.jtice.2016.08.038.
- P. R. Souza, G. L. Dotto and N. P. G. Salau, Artificial neural network (ANN) and adaptive neuro-fuzzy interference system (ANFIS) modelling for nickel adsorption onto agro-wastes and commercial activated carbon, J. Environ. Chem. Eng., 2018, 6, 7152–7160 CrossRef CAS.
- P. S. Pauletto, G. L. Dotto and N. P. G. Salau, Optimal artificial neural network design for simultaneous modeling of multicomponent adsorption, J. Mol. Liq., 2020, 320, 114418, DOI:10.1016/j.molliq.2020.114418.
- S. Hokkanen, A. Bhatnagar and M. Sillanpää, A review on modification methods to cellulose-based adsorbents to improve adsorption capacity, Water Res., 2016, 91, 156–173 CrossRef CAS PubMed.
- A. Esmaeili and A. A. Beni, Novel membrane reactor design for heavy-metal removal by alginate nanoparticles, J. Ind. Eng. Chem., 2015, 26, 122–128 CrossRef CAS.
- M. Khajeh and E. Jahanbin, Application of cuckoo optimization algorithm–artificial neural network method of zinc oxide nanoparticles–chitosan for extraction of uranium from water samples, Chemom. Intell. Lab. Syst., 2014, 135, 70–75 CrossRef CAS.
- R. Khandanlou, H. R. F. Masoumi, M. B. Ahmad, K. Shameli, M. Basri and K. Kalantari, Enhancement of heavy metals sorption via nanocomposites of rice straw and Fe3O4 nanoparticles using artificial neural network (ANN), Ecol. Eng., 2016, 91, 249–256 CrossRef.
- M. R. Kowsari, H. Sepehrian, M. Mahani and J. Fasihi, Cobalt (II) adsorption from aqueous solution using alginate-SBA-15 nanocomposite: kinetic, isotherm, thermodynamic studies and neural network modeling, Mater. Focus, 2016, 5, 91–99 CrossRef CAS.
- A. A. Oladipo and M. Gazi, Nickel removal from aqueous solutions by alginate-based composite beads: central composite design and artificial neural network modeling, J. Water Process Eng., 2015, 8, e81–e91 CrossRef.
- A. Sadeghizadeh, F. Ebrahimi, M. Heydari, M. Tahmasebikohyani, F. Ebrahimi and A. Sadeghizadeh, Adsorptive removal of Pb (II) by means of hydroxyapatite/chitosan nanocomposite hybrid nanoadsorbent: ANFIS modeling and experimental study, J. Environ. Manage., 2019, 232, 342–353 CrossRef CAS PubMed.
- E. Tomczak, Application of ANN and EA for description of metal ions sorption on chitosan foamed structure—equilibrium and dynamics of packed column, Comput. Chem. Eng., 2011, 35, 226–235 CrossRef CAS.
- S. P. G. Zaferani, M. R. S. Emami, M. K. Amiri and E. Binaeian, Optimization of the removal Pb (II) and its Gibbs free energy by thiosemicarbazide modified chitosan using RSM and ANN modeling, Int. J. Biol. Macromol., 2019, 139, 307–319 CrossRef CAS PubMed.
- B. K. Nath, C. Chaliha and E. Kalita, Iron oxide permeated mesoporous rice-husk nanobiochar (IPMN) mediated removal of dissolved arsenic (As): chemometric modelling and adsorption dynamics, J. Environ. Manage., 2019, 246, 397–409 CrossRef CAS PubMed.
- A. Takdastan, S. Samarbaf, Y. Tahmasebi, N. Alavi and A. A. Babaei, Alkali modified oak waste residues as a cost-effective adsorbent for enhanced removal of cadmium from water: isotherm, kinetic, thermodynamic and artificial neural network modeling, J. Ind. Eng. Chem., 2019, 78, 352–363 CrossRef CAS.
- S. Ullah, M. A. Assiri, M. A. Bustam, A. G. Al-Sehemi, F. A. Abdul Kareem and A. Irfan, Equilibrium, kinetics and artificial intelligence characteristic analysis for Zn (II) ion adsorption on rice husks digested with nitric acid, Paddy Water Environ., 2020, 18, 455–468 CrossRef.
- M. Zhu, F. Li, W. Chen, X. Yin, Z. Yi and S. Zhang, Adsorption of U (VI) from aqueous solution by using KMnO4-modified hazelnut shell activated carbon: characterisation and artificial neural network modelling, Environ. Sci. Pollut. Res., 2021, 28, 47354–47366 CrossRef CAS PubMed.
- S. Chakrabarty and H. P. Sarma, Heavy metal contamination of drinking water in Kamrup district, Assam, India, Environ. Monit. Assess., 2011, 179, 479–486, DOI:10.1007/s10661-010-1750-7.
- T. Khan, M. H. Isa, M. R. U. Mustafa, H. Yeek-Chia, L. Baloo, T. S. B. Abd Manan and M. O. Saeed, Cr (VI) adsorption from aqueous solution by an agricultural waste based carbon, RSC Adv., 2016, 6, 56365–56374 RSC.
- M. Solgi, T. Najib, S. Ahmadnejad and B. Nasernejad, Synthesis and characterization of novel activated carbon from Medlar seed for chromium removal: experimental analysis and modeling with artificial neural network and support vector regression, Resour.-Effic. Technol., 2017, 3, 236–248 Search PubMed.
- K. Singh, J. K. Arora, T. J. M. Sinha and S. Srivastava, Functionalization of nanocrystalline cellulose for decontamination of Cr (III) and Cr (VI) from aqueous system: computational modeling approach, Clean Technol. Environ. Policy, 2014, 16, 1179–1191 CrossRef CAS.
- V. Singh, J. Singh and V. Mishra, Development of a cost-effective, recyclable and viable metal ion doped adsorbent for simultaneous adsorption and reduction of toxic Cr (VI) ions, J. Environ. Chem. Eng., 2021, 9, 105124 CrossRef CAS.
-
C. Sutherland, A. Marcano and B. Chittoo, Artificial neural network-genetic algorithm prediction of heavy metal removal using a novel plant-based biosorbent banana floret: kinetic, equilibrium, thermodynamics and desorption studies, in Desalination Water Treat., IntechOpen, 2018, pp. 385–411 Search PubMed.
- K. Yadav, M. Raphi and S. Jagadevan, Adsorption of copper (II) on chemically modified biochar: a single-stage batch adsorber design and predictive modeling through artificial neural network, Biomass Convers. Biorefinery, 2021, 1–16 Search PubMed.
- T. Khan, M. R. U. Mustafa, M. H. Isa, T. S. B. A. Manan, Y.-C. Ho, J.-W. Lim and N. Z. Yusof, Artificial neural network (ANN) for modelling adsorption of lead (Pb (II)) from aqueous solution, Water. Air. Soil Pollut., 2017, 228, 1–15 CrossRef CAS.
- M. Ashrafi, H. Borzuie, G. Bagherian, M. A. Chamjangali and H. Nikoofard, Artificial neural network and multiple linear regression for modeling sorption of Pb2+ ions from aqueous solutions onto modified walnut shell, Sep. Sci. Technol., 2020, 55, 222–233 CrossRef CAS.
- S. Ullah, M. A. Assiri, A. G. Al-Sehemi, M. A. Bustam, M. Sagir, F. A. Abdulkareem, M. R. Raza, M. Ayoub and A. Irfan, Characteristically insights, artificial neural network (ANN), equilibrium, and kinetic studies of Pb (II) ion adsorption on rice husks treated with nitric acid, Int. J. Environ. Res., 2020, 14, 43–60 CrossRef CAS.
- M. Pazouki, M. Zabihi, J. Shayegan and M. H. Fatehi, Mercury ion adsorption on AC@Fe3O4-NH2-COOH from saline solutions: experimental studies and artificial neural network modeling, Korean J. Chem. Eng., 2018, 35, 671–683 CrossRef CAS.
- B. R. Broujeni, A. Nilchi and F. Azadi, Adsorption modeling and optimization of thorium (IV) ion from aqueous solution using chitosan/TiO2 nanocomposite: application of artificial neural network and genetic algorithm, Environ. Nanotechnol. Monit. Manag., 2021, 15, 100400 Search PubMed.
- H. Heshmati, M. Torab-Mostaedi, H. Ghanadzadeh Gilani and A. Heydari, Kinetic, isotherm, and thermodynamic investigations of uranium (VI) adsorption on synthesized ion-exchange chelating resin and prediction with an artificial neural network, Desalination Water Treat., 2015, 55, 1076–1087 CrossRef CAS.
- S. Rebouh, M. Bouhedda and S. Hanini, Neuro-fuzzy modeling of Cu (II) and Cr (VI) adsorption from aqueous solution by wheat straw, Desalination Water Treat., 2016, 57, 6515–6530 CrossRef CAS.
- S. Varshney, P. Jain, J. K. Arora and S. Srivastava, Process development for the removal of toxic metals by functionalized wood pulp: kinetic, thermodynamic, and computational modeling approach, Clean Technol. Environ. Policy, 2016, 18, 2613–2623 CrossRef CAS.
- F. Brouers and T. J. Al-Musawi, On the optimal use of isotherm models for the characterization of biosorption of lead onto algae, J. Mol. Liq., 2015, 212, 46–51, DOI:10.1016/j.molliq.2015.08.054.
- A. H. Hamidian, S. Esfandeh, Y. Zhang and M. Yang, Simulation and optimization of nanomaterials application for heavy metal removal from aqueous solutions, Inorg. Nano-Met. Chem., 2019, 49, 217–230 CrossRef CAS.
- A. Ronda, M. A. Martín-Lara, A. I. Almendros, A. Pérez and G. Blázquez, Comparison of two models for the biosorption of Pb (II) using untreated and chemically treated olive stone: experimental design methodology and adaptive neural fuzzy inference system (ANFIS), J. Taiwan Inst. Chem. Eng., 2015, 54, 45–56 CrossRef.
- P. L. Narayana, A. K. Maurya, X.-S. Wang, M. R. Harsha, O. Srikanth, A. A. Alnuaim, W. A. Hatamleh, A. A. Hatamleh, K. K. Cho and U. M. Reddy, Artificial neural networks modeling for lead removal from aqueous solutions using iron oxide nanocomposites from bio-waste mass, Environ. Res., 2021, 111370 CrossRef CAS.
- Y. J. Wong, S. K. Arumugasamy, C. H. Chung, A. Selvarajoo and V. Sethu, Comparative study of artificial neural network (ANN), adaptive neuro-fuzzy inference system (ANFIS) and multiple linear regression (MLR) for modeling of Cu (II) adsorption from aqueous solution using biochar derived from rambutan (Nephelium lappaceum) peel, Environ. Monit. Assess., 2020, 192, 1–20 CrossRef.
-
P. J. Braspenning, F. Thuijsman and A. J. M. M. Weijters, Artificial Neural Networks: an Introduction to ANN Theory and Practice, Springer Science & Business Media, 1995 Search PubMed.
- A. T. Goh, Back-propagation neural networks for modeling complex systems, Artif. Intell. Eng., 1995, 9, 143–151 CrossRef.
- P. P. Van Der Smagt, Minimisation methods for training feedforward neural networks, Neural Network., 1994, 7, 1–11 CrossRef.
- Z. Wang, H. Zhang, J. Ren, X. Lin, T. Han, J. Liu and J. Li, Predicting adsorption ability of adsorbents at arbitrary sites for pollutants using deep transfer learning, Npj Comput. Mater., 2021, 7, 1–9, DOI:10.1038/s41524-021-00494-9.
- M. Fawzy, M. Nasr, H. Nagy and S. Helmi, Artificial intelligence and regression analysis for Cd (II) ion biosorption from aqueous solution by Gossypium barbadense waste, Environ. Sci. Pollut. Res., 2018, 25, 5875–5888 CrossRef CAS PubMed.
- D. I. Mendoza-Castillo, N. Villalobos-Ortega, A. Bonilla-Petriciolet and J. C. Tapia-Picazo, Neural network modeling of heavy metal sorption on lignocellulosic biomasses: effect of metallic ion properties and sorbent characteristics, Ind. Eng. Chem. Res., 2015, 54, 443–453 CrossRef CAS.
- A. A. Prabhu, S. Chityala, D. Jayachandran, N. N. Deshavath and V. D. Veeranki, A two step optimization approach for maximizing biosorption of hexavalent chromium ions (Cr (VI)) using alginate immobilized Sargassum sp in a packed bed column, Sep. Sci. Technol., 2021, 56, 90–106 CrossRef CAS.
- L. T. Popoola, Nano-magnetic walnut shell-rice husk for Cd (II) sorption: design and optimization using artificial intelligence and design expert, Heliyon, 2019, 5, e02381 CrossRef.
- F. Kartal and U. Özveren, An improved machine learning approach to estimate hemicellulose, cellulose, and lignin in biomass, Carbohydr. Polym. Technol. Appl., 2021, 2, 100148 CAS.
- H. A. Hamid, Y. Jenidi, W. Thielemans, C. Somerfield and R. L. Gomes, Predicting the capability of carboxylated cellulose nanowhiskers for the remediation of copper from water using response surface methodology (RSM) and artificial neural network (ANN) models, Ind. Crops Prod., 2016, 93, 108–120 CrossRef CAS.
- D. Krishna, G. S. Kumar and D. R. P. Raju, Optimization of Process Parameters for the Removal of Chromium (VI) from Waste Water Using Mixed Adsorbent, Int. J. Appl. Sci. Eng., 2019, 16, 187–200 Search PubMed.
- M. Shanmugaprakash, S. Venkatachalam, K. Rajendran and A. Pugazhendhi, Biosorptive removal of Zn (II) ions by Pongamia oil cake (Pongamia pinnata) in batch and fixed-bed column studies using response surface methodology and artificial neural network, J. Environ. Manage., 2018, 227, 216–228 CrossRef CAS.
- E. Allahkarami, A. Igder, A. Fazlavi and B. Rezai, Prediction of Co (II) and Ni (II) ions removal from wastewater using artificial neural network and multiple regression models, Physicochem. Probl. Miner. Process., 2017, 53, 1105–1118 CAS.
- M. Banerjee, N. Bar and S. K. Das, Cu (II) removal from aqueous solution using the walnut shell: adsorption study, regeneration study, plant scale-up design, economic feasibility, statistical, and GA-ANN modeling, Int. J. Environ. Res., 2021, 15, 875–891 CrossRef CAS.
- P. Moradi, S. Hayati and T. Ghahrizadeh, Modeling and optimization of lead and cobalt biosorption from water with Rafsanjan pistachio shell, using experiment based models of ANN and GP, and the grey wolf optimizer, Chemom. Intell. Lab. Syst., 2020, 202, 104041 CrossRef CAS.
- J. A. Rodríguez-Romero, D. I. Mendoza-Castillo, H. E. Reynel-Ávila, D. A. de Haro-Del Rio, L. M. González-Rodríguez, A. Bonilla-Petriciolet, C. J. Duran-Valle and K. I. Camacho-Aguilar, Preparation of a new adsorbent for the removal of arsenic and its simulation with artificial neural network-based adsorption models, J. Environ. Chem. Eng., 2020, 8, 103928 CrossRef.
- X. Zheng and H. Nguyen, A novel artificial intelligent model for predicting water treatment efficiency of various biochar systems based on artificial neural network and queuing search algorithm, Chemosphere, 2022, 287, 132251 CrossRef CAS.
- Q. Dai and N. Liu, Alleviating the problem of local minima in backpropagation through competitive learning, Neurocomputing, 2012, 94, 152–158 CrossRef.
- H.-X. Huang, J.-C. Li and C.-L. Xiao, A proposed iteration optimization approach integrating backpropagation neural network with genetic algorithm, Expert Syst. Appl., 2015, 42, 146–155 CrossRef.
- G. Dhiman, SSC: a hybrid nature-inspired meta-heuristic optimization algorithm for engineering applications, Knowl. Base Syst., 2021, 222, 106926 CrossRef.
- B. Ke, H. Nguyen, X.-N. Bui, H.-B. Bui, Y. Choi, J. Zhou, H. Moayedi, R. Costache and T. Nguyen-Trang, Predicting the sorption efficiency of heavy metal based on the biochar characteristics, metal sources, and environmental conditions using various novel hybrid machine learning models, Chemosphere, 2021, 276, 130204 CrossRef CAS.
- M. Khishe and M. R. Mosavi, Chimp optimization algorithm, Expert Syst. Appl., 2020, 149, 113338 CrossRef.
- M. Shehab, A. T. Khader, M. Laouchedi and O. A. Alomari, Hybridizing cuckoo search algorithm with bat algorithm for global numerical optimization, J. Supercomput., 2019, 75, 2395–2422 CrossRef.
- A. Ahmad, M. Sulaiman, A. Alhindi and A. J. Aljohani, Analysis of temperature profiles in longitudinal fin designs by a novel neuroevolutionary approach, IEEE Access, 2020, 8, 113285–113308 Search PubMed.
-
J. M. Chaharmahali and M. Shabanzadeh, A Machine Learning-Assisted Clustering Engine to Enhance the Accuracy of Hourly Load Forecasting, in 2020 10th Smart Grid Conf. SGC, IEEE, 2020, pp. 1–6 Search PubMed.
- M. Farzaneh-Gord, B. Mohseni-Gharyehsafa, A. Ebrahimi-Moghadam, A. Jabari-Moghadam, A. Toikka and I. Zvereva, Precise calculation of natural gas sound speed using neural networks: an application in flow meter calibration, Flow Meas. Instrum., 2018, 64, 90–103 CrossRef.
- M. S. Saad, A. Mohd Nor, I. Abd Rahim, M. A. Syahruddin and I. Z. Mat Darus, Optimization of FDM process parameters to minimize surface roughness with integrated artificial neural network model and symbiotic organism search, Neural Comput. Appl., 2022, 1–17 Search PubMed.
- S. Mirjalili, S. M. Mirjalili and A. Lewis, Grey wolf optimizer, Adv. Eng. Softw., 2014, 69, 46–61 CrossRef.
- J.-S. Wang and S.-X. Li, An Improved Grey Wolf Optimizer Based on Differential Evolution and Elimination Mechanism, Sci. Rep., 2019, 9, 7181, DOI:10.1038/s41598-019-43546-3.
-
S. Nosratabadi, K. Szell, B. Beszedes, F. Imre, S. Ardabili and A. Mosavi, Comparative Analysis of ANN-ICA and ANN-GWO for Crop Yield Prediction, in 2020 RIVF Int. Conf. Comput. Commun. Technol. RIVF, IEEE, 2020, pp. 1–5 Search PubMed.
-
S. Ardabili, A. Mosavi, S. S. Band and A. R. Varkonyi-Koczy, Coronavirus disease (COVID-19) global prediction using hybrid artificial intelligence method of ANN trained with Grey Wolf optimizer, in 2020 IEEE 3rd Int. Conf. Workshop Óbuda Electr. Power Eng. CANDO-EPE, IEEE, 2020, pp. 000251–000254 Search PubMed.
- A. Seifi and F. Soroush, Pan evaporation estimation and derivation of explicit optimized equations by novel hybrid meta-heuristic ANN based methods in different climates of Iran, Comput. Electron. Agric., 2020, 173, 105418, DOI:10.1016/j.compag.2020.105418.
-
A. Sharma and U. Tyagi, A Hybrid Approach of ANN-GWO Technique for Intrusion Detection, in 2021 Int. Conf. Recent Trends Electron. Inf. Commun. Technol. RTEICT, 2021, pp. 467–472, DOI:10.1109/RTEICT52294.2021.9573800.
- B. Yuce and Y. Rezgui, An ANN-GA semantic rule-based system to reduce the gap between predicted and actual energy consumption in buildings, IEEE Trans. Autom. Sci. Eng., 2015, 14, 1351–1363 Search PubMed.
-
M. Akgül, Ö. E. Sönmez and T. Özcan, Diagnosis of heart disease using an intelligent method: a hybrid ANN–GA approach, in Int. Conf. Intell. Fuzzy Syst., Springer, 2019, pp. 1250–1257 Search PubMed.
- S. Bahrami, F. Doulati Ardejani and E. Baafi, Application of artificial neural network coupled with genetic algorithm and simulated annealing to solve groundwater inflow problem to an advancing open pit mine, J. Hydrol., 2016, 536, 471–484, DOI:10.1016/j.jhydrol.2016.03.002.
- K. S. Sangwan, S. Saxena and G. Kant, Optimization of machining parameters to minimize surface roughness using integrated ANN-GA approach, Procedia Cirp, 2015, 29, 305–310 CrossRef.
- A. A. Kulaksız and R. Akkaya, A genetic algorithm optimized ANN-based MPPT algorithm for a stand-alone PV system with induction motor drive, Sol. Energy, 2012, 86, 2366–2375 CrossRef.
- X. Lü, Y. Wu, J. Lian, Y. Zhang, C. Chen, P. Wang and L. Meng, Energy management of hybrid electric vehicles: a review of energy optimization of fuel cell hybrid power system based on genetic algorithm, Energy Convers. Manag., 2020, 205, 112474 CrossRef.
- J. Zhang, M. Xiao, L. Gao and Q. Pan, Queuing search algorithm: a novel metaheuristic algorithm for solving engineering optimization problems, Appl. Math. Model., 2018, 63, 464–490 CrossRef.
- S. Gupta, H. Abderazek, B. S. Yıldız, A. R. Yildiz, S. Mirjalili and S. M. Sait, Comparison of metaheuristic optimization algorithms for solving constrained mechanical design optimization problems, Expert Syst. Appl., 2021, 183, 115351 CrossRef.
- J. Zhan, M. Fard and R. Jazar, A CAD-FEM-QSA integration technique for determining the time-varying meshing stiffness of gear pairs, Measurement, 2017, 100, 139–149, DOI:10.1016/j.measurement.2016.12.056.
- M. Nasr, A. E. D. Mahmoud, M. Fawzy and A. Radwan, Artificial intelligence modeling of cadmium (II) biosorption using rice straw, Appl. Water Sci., 2017, 7, 823–831 CrossRef CAS.
- I. M. El-Hasnony, S. I. Barakat and R. R. Mostafa, Optimized ANFIS model using hybrid metaheuristic algorithms for Parkinson's disease prediction in IoT environment, IEEE Access, 2020, 8, 119252–119270 Search PubMed.
- Z. Yun, Z. Quan, S. Caixin, L. Shaolan, L. Yuming and S. Yang, RBF neural network and ANFIS-based short-term load forecasting approach in real-time price environment, IEEE Trans. Power Syst., 2008, 23, 853–858 Search PubMed.
- M. Şahin and R. Erol, A comparative study of neural networks and ANFIS for forecasting attendance rate of soccer games, Math. Comput. Appl., 2017, 22, 43 Search PubMed.
- P. Srisaeng and G. Baxter, Application of an ANFIS to Estimate Kansai International Airport's International Air Passenger Demand, J. Aviat., 2022, 6, 87–92 CrossRef.
- B. M. Wilamowski, Neural network architectures and learning algorithms, IEEE Ind. Electron. Mag., 2009, 3, 56–63 Search PubMed.
-
E. Siregar, H. Mawengkang, E. B. Nababan and A. Wanto, Analysis of Backpropagation Method with Sigmoid Bipolar and Linear Function in Prediction of Population Growth, in J. Phys. Conf. Ser., IOP Publishing, 2019, p. 012023 Search PubMed.
-
M. A. Mercioni and S. Holban, The most used activation functions: Classic versus current, in 2020 Int. Conf. Dev. Appl. Syst., DAS, IEEE, 2020, pp. 141–145 Search PubMed.
- B. K. Nath, C. Chaliha and E. Kalita, Iron oxide Permeated Mesoporous rice-husk nanobiochar (IPMN) mediated removal of dissolved arsenic (As): chemometric modelling and adsorption dynamics, J. Environ. Manage., 2019, 246, 397–409 CrossRef CAS PubMed.
- D. Ranjan, D. Mishra and S. H. Hasan, Bioadsorption of arsenic: an artificial neural networks and response surface methodological approach, Ind. Eng. Chem. Res., 2011, 50, 9852–9863 CrossRef CAS.
- M. Shanmugaprakash and V. Sivakumar, Development of experimental design approach and ANN-based models for determination of Cr (VI) ions uptake rate from aqueous solution onto the solid biodiesel waste residue, Bioresour. Technol., 2013, 148, 550–559 CrossRef CAS PubMed.
- D. Whitley, S. Rana, J. Dzubera and K. E. Mathias, Evaluating evolutionary algorithms, Artif. Intell., 1996, 85, 245–276 CrossRef.
- K. Yetilmezsoy and S. Demirel, Artificial neural network (ANN) approach for modeling of Pb (II) adsorption from aqueous solution by Antep pistachio (Pistacia Vera L.) shells, J. Hazard. Mater., 2008, 153, 1288–1300 CrossRef CAS PubMed.
- N. Prakash, S. A. Manikandan, L. Govindarajan and V. Vijayagopal, Prediction of biosorption efficiency for the removal of copper (II) using artificial neural networks, J. Hazard. Mater., 2008, 152, 1268–1275 CrossRef CAS PubMed.
- A. K. Giri, R. K. Patel and S. S. Mahapatra, Artificial neural network (ANN) approach for modelling of arsenic (III) biosorption from aqueous solution by living cells of Bacillus cereus biomass, Chem. Eng. J., 2011, 178, 15–25 CrossRef CAS.
- A. Kardam, K. R. Raj, J. K. Arora and S. Srivastava, Artificial neural network modeling for biosorption of Pb (II) ions on nanocellulose fibers, Bionanoscience, 2012, 2, 153–160 CrossRef.
- K. R. Raj, A. Kardam, J. K. Arora and S. Srivastava, An application of ANN modeling on the biosorption of arsenic, Waste Biomass Valorization, 2013, 4, 401–407 CrossRef CAS.
- B. Singha, N. Bar and S. K. Das, The use of artificial neural networks (ANN) for modeling of adsorption of Cr (VI) ions, Desalination Water Treat., 2014, 52, 415–425 CrossRef CAS.
- B. Singha, N. Bar and S. K. Das, The use of artificial neural network (ANN) for modeling of Pb (II) adsorption in batch process, J. Mol. Liq., 2015, 211, 228–232 CrossRef CAS.
- M. S. Podder and C. B. Majumder, The use of artificial neural network for modelling of phycoremediation of toxic elements As (III) and As (V) from wastewater using Botryococcus braunii, Spectrochim. Acta Mol. Biomol. Spectrosc., 2016, 155, 130–145 CrossRef CAS.
- H. Esfandian, M. Parvini, B. Khoshandam and A. Samadi-Maybodi, Artificial neural network (ANN) technique for modeling the mercury adsorption from aqueous solution using Sargassum Bevanom algae, Desalination Water Treat., 2016, 57, 17206–17219 CrossRef CAS.
- S. Nag, A. Mondal, N. Bar and S. K. Das, Biosorption of chromium (VI) from aqueous solutions and ANN modelling, Environ. Sci. Pollut. Res., 2017, 24, 18817–18835 CrossRef CAS PubMed.
- M. Banerjee, N. Bar, R. K. Basu and S. K. Das, Comparative study of adsorptive removal of Cr (VI) ion from aqueous solution in fixed bed column by peanut shell and almond shell using empirical models and ANN, Environ. Sci. Pollut. Res., 2017, 24, 10604–10620 CrossRef CAS PubMed.
- R. S. Kiran, G. M. Madhu, S. V. Satyanarayana, P. Kalpana and G. S. Rangaiah, Applications of Box–Behnken experimental design coupled with artificial neural networks for biosorption of low concentrations of cadmium using Spirulina (Arthrospira) spp, Resour.-Effic. Technol., 2017, 3, 113–123 Search PubMed.
- A. Kardam, K. R. Raj, J. K. Arora, M. M. Srivastava and S. Srivastava, Artificial neural network modeling for sorption of cadmium from aqueous system by shelled Moringa oleifera seed powder as an agricultural waste, J. Water Resour. Prot., 2010, 2, 339 CrossRef CAS.
- L. T. Popoola, Nano-magnetic walnut shell-rice husk for Cd (II) sorption: design and optimization using artificial intelligence and design expert, Heliyon, 2019, 5, e02381 CrossRef PubMed.
- M. Fawzy, M. Nasr, S. Adel and S. Helmi, Regression model, artificial neural network, and cost estimation for biosorption of Ni (II)-ions from aqueous solutions by Potamogeton pectinatus, Int. J. Phytoremediation, 2018, 20, 321–329 CrossRef CAS PubMed.
- D. Krishna and R. P. Sree, Artificial Neural Network (ANN) Approach for modeling chromium (VI) adsorption from aqueous solution using a Borasus Flabellifer coir powder, Int. J. Appl. Sci. Eng., 2014, 12, 177–192 Search PubMed.
- D. Krishna, G. S. Kumar and D. R. P. Raju, Optimization of Process Parameters for the Removal of Chromium (VI) from Waste Water Using Mixed Adsorbent, Int. J. Appl. Sci. Eng., 2019, 16, 187–200 Search PubMed.
- N. Parveen, S. Zaidi and M. Danish, Development of SVR-based model and comparative analysis with MLR and ANN models for predicting the sorption capacity of Cr (VI), Process Saf. Environ. Prot., 2017, 107, 428–437 CrossRef CAS.
- W. A. H. Altowayti, H. A. Algaifi, S. A. Bakar and S. Shahir, The adsorptive removal of As (III) using biomass of arsenic resistant Bacillus thuringiensis strain WS3: characteristics and modelling studies, Ecotoxicol. Environ. Saf., 2019, 172, 176–185 CrossRef CAS PubMed.
- R. Beigzadeh and S. O. Rastegar, Assessment of Cr (VI) Biosorption from Aqueous Solution by Artificial Intelligence, Chem. Methodol., 2020, 4, 181–190 CrossRef CAS.
- E. Oguz and M. Ersoy, Removal of Cu2+ from aqueous solution by adsorption in a fixed bed column and Neural Network Modelling, Chem. Eng. J., 2010, 164, 56–62 CrossRef CAS.
- N. G. Turan, B. Mesci and O. Ozgonenel, Artificial neural network (ANN) approach for modeling Zn (II) adsorption from leachate using a new biosorbent, Chem. Eng. J., 2011, 173, 98–105 CrossRef CAS.
- D. Bingöl, M. Hercan, S. Elevli and E. Kılıç, Comparison of the results of response surface methodology and artificial neural network for the biosorption of lead using black cumin, Bioresour. Technol., 2012, 112, 111–115 CrossRef PubMed.
- M. F. Ahmad, S. Haydar, A. A. Bhatti and A. J. Bari, Application of artificial neural network for the prediction of biosorption capacity of immobilized Bacillus subtilis for the removal of cadmium ions from aqueous solution, Biochem. Eng. J., 2014, 84, 83–90 CrossRef CAS.
- U. Yurtsever, M. Yurtsever, İ. A. Şengil and N. Kıratlı Yılmazçoban, Fast artificial neural network (FANN) modeling of Cd (II) ions removal by valonia resin, Desalination Water Treat., 2015, 56, 83–96 CrossRef CAS.
- S. Yildiz, Artificial neural network (ANN) approach for modeling Zn (II) adsorption in batch process, Korean J. Chem. Eng., 2017, 34, 2423–2434 CrossRef CAS.
- F. Rahimpour, T. Shojaeimehr and M. Sadeghi, Biosorption of Pb (II) using Gundelia tournefortii: kinetics, equilibrium, and thermodynamics, Sep. Sci. Technol., 2017, 52, 596–607 CrossRef CAS.
- S. G. Shandi, F. D. Ardejani and F. Sharifi, Assessment of Cu (II) removal from an aqueous solution by raw Gundelia tournefortii as a new low-cost biosorbent: experiments and modelling, Chin. J. Chem. Eng., 2019, 27, 1945–1955 CrossRef CAS.
- M. R. Fagundes-Klen, P. Ferri, T. D. Martins, C. R. G. Tavares and E. A. Silva, Equilibrium study of the binary mixture of cadmium–zinc ions biosorption by the Sargassum filipendula species using adsorption isotherms models and neural network, Biochem. Eng. J., 2007, 34, 136–146 CrossRef CAS.
- D. Bingöl, M. Inal and S. Çetintaş, Evaluation of copper biosorption onto date palm (Phoenix dactylifera L.) seeds with MLR and ANFIS models, Ind. Eng. Chem. Res., 2013, 52, 4429–4435 CrossRef.
- S. A. Jafari and S. Cheraghi, Mercury removal from aqueous solution by dried biomass of indigenous Vibrio parahaemolyticus PG02: kinetic, equilibrium, and thermodynamic studies, Int. Biodeterior. Biodegrad., 2014, 92, 12–19 CrossRef CAS.
- M. Fawzy, M. Nasr, S. Adel, H. Nagy and S. Helmi, Environmental approach and artificial intelligence for Ni (II) and Cd (II) biosorption from aqueous solution using Typha domingensis biomass, Ecol. Eng., 2016, 95, 743–752 CrossRef.
- M. Fawzy, M. Nasr, A. Abdel-Gaber and S. Fadly, Biosorption of Cr (VI) from aqueous solution using agricultural wastes, with artificial intelligence approach, Sep. Sci. Technol., 2016, 51, 416–426 CrossRef CAS.
- S. Nag, N. Bar and S. K. Das, Cr (VI) removal from aqueous solution using green adsorbents in continuous bed column–statistical and GA-ANN hybrid modelling, Chem. Eng. Sci., 2020, 226, 115904 CrossRef CAS.
- B. R. Broujeni, A. Nilchi and F. Azadi, Adsorption modeling and optimization of thorium (IV) ion from aqueous solution using chitosan/TiO2 nanocomposite: application of artificial neural network and genetic algorithm, Environ. Nanotechnol. Monit. Manag., 2021, 15, 100400 Search PubMed.
- A. A. Prabhu, S. Chityala, D. Jayachandran, N. N. Deshavath and V. D. Veeranki, A two step optimization approach for maximizing biosorption of hexavalent chromium ions (Cr (VI)) using alginate immobilized Sargassum sp in a packed bed column, Sep. Sci. Technol., 2021, 56, 90–106 CrossRef CAS.
- M. Khajeh and E. Jahanbin, Application of cuckoo optimization algorithm–artificial neural network method of zinc oxide nanoparticles–chitosan for extraction of uranium from water samples, Chemom. Intell. Lab. Syst., 2014, 135, 70–75 CrossRef CAS.
- A. M. Abu El-Soad, G. Lazzara, M. O. Abd El-Magied, G. Cavallaro, J. S. Al-Otaibi, M. I. Sayyed and E. G. Kovaleva, Chitosan Functionalized with Carboxyl Groups as a Recyclable Biomaterial for the Adsorption of Cu (II) and Zn (II) Ions in Aqueous Media, Int. J. Mol. Sci., 2022, 23, 2396 CrossRef CAS PubMed.
- A. Çelekli, A. I. Al-Nuaimi and H. Bozkurt, Adsorption kinetic and isotherms of Reactive Red 120 on Moringa oleifera seed as an eco-friendly process, J. Mol. Struct., 2019, 1195, 168–178 CrossRef.
- S. Narayanasamy, V. Sundaram, T. Sundaram and D.-V. N. Vo, Biosorptive ascendency of plant based biosorbents in removing hexavalent chromium from aqueous solutions–Insights into isotherm and kinetic studies, Environ. Res., 2022, 210, 112902 CrossRef CAS PubMed.
- N. A. Negm, M. G. Abd El Wahed, A. R. A. Hassan and M. T. Abou Kana, Feasibility of metal adsorption using brown algae and fungi: effect of biosorbents structure on adsorption isotherm and kinetics, J. Mol. Liq., 2018, 264, 292–305 CrossRef CAS.
- M. G. Alalm and M. Nasr, Artificial intelligence, regression model, and cost estimation for removal of chlorothalonil pesticide by activated carbon prepared from casuarina charcoal, Sustain. Environ. Res., 2018, 28, 101–110 CrossRef.
- B. G. Saucedo-Delgado, D. A. De Haro-Del Rio, L. M. González-Rodríguez, H. E. Reynel-Ávila, D. I. Mendoza-Castillo, A. Bonilla-Petriciolet and J. R. de la Rosa, Fluoride adsorption from aqueous solution using a protonated clinoptilolite and its modeling with artificial neural network-based equations, J. Fluorine Chem., 2017, 204, 98–106 CrossRef CAS.
- R. Tovar-Gómez, M. R. Moreno-Virgen, J. A. Dena-Aguilar, V. Hernández-Montoya, A. Bonilla-Petriciolet and M. A. Montes-Morán, Modeling of fixed-bed adsorption of fluoride on bone char using a hybrid neural network approach, Chem. Eng. J., 2013, 228, 1098–1109 CrossRef.
- S. Verma and A. Kuila, Bioremediation of heavy metals by microbial process, Environ. Technol. Innovat., 2019, 14, 100369 CrossRef.
- M. S. Netto, J. S. Oliveira, N. P. Salau and G. L. Dotto, Analysis of adsorption isotherms of Ag+, Co+2, and Cu+2 onto zeolites using computational intelligence models, J. Environ. Chem. Eng., 2021, 9, 104960 CrossRef CAS.
- M. Dolatabadi, M. Mehrabpour, M. Esfandyari, H. Alidadi and M. Davoudi, Modeling of simultaneous adsorption of dye and metal ion by sawdust from aqueous solution using of ANN and ANFIS, Chemom. Intell. Lab. Syst., 2018, 181, 72–78, DOI:10.1016/j.chemolab.2018.07.012.
- D. I. Mendoza-Castillo, H. E. Reynel-Ávila, F. J. Sánchez-Ruiz, R. Trejo-Valencia, J. E. Jaime-Leal and A. Bonilla-Petriciolet, Insights and pitfalls of artificial neural network modeling of competitive multi-metallic adsorption data, J. Mol. Liq., 2018, 251, 15–27 CrossRef CAS.
- A. Gopinath, B. G. Retnam, A. Muthukkumaran and K. Aravamudan, Swift, versatile and a rigorous kinetic model based artificial neural network surrogate for single and multicomponent batch adsorption processes, J. Mol. Liq., 2020, 297, 111888 CrossRef CAS.
- J. D. Olden, M. K. Joy and R. G. Death, An accurate comparison of methods for quantifying variable importance in artificial neural networks using simulated data, Ecol. Model., 2004, 178, 389–397 CrossRef.
- A. K. Abraham, W. Krzyzanski and D. E. Mager, Partial derivative—based sensitivity analysis of models describing target-mediated drug disposition, AAPS J., 2007, 9, E181–E189 CrossRef PubMed.
- M. Gevrey, I. Dimopoulos and S. Lek, Two-way interaction of input variables in the sensitivity analysis of neural network models, Ecol. Model., 2006, 195, 43–50 CrossRef.
- P. K. Srivastava, M. Gupta, U. Singh, R. Prasad, P. C. Pandey, A. S. Raghubanshi and G. P. Petropoulos, Sensitivity analysis of artificial neural network for chlorophyll prediction using hyperspectral data, Environ. Dev. Sustain., 2021, 23, 5504–5519 CrossRef.
- M. Gevrey, I. Dimopoulos and S. Lek, Review and comparison of methods to study the contribution of variables in artificial neural network models, Ecol. Model., 2003, 160, 249–264 CrossRef.
- K. Zeng, K. Hachem, M. Kuznetsova, S. Chupradit, C.-H. Su, H. C. Nguyen and A. S. El-Shafay, Molecular dynamic simulation and artificial intelligence of lead ions removal from aqueous solution using magnetic-ash-graphene oxide nanocomposite, J. Mol. Liq., 2022, 347, 118290 CrossRef CAS.
- J. H. Montoya and K. A. Persson, A high-throughput framework for determining adsorption energies on solid surfaces, Npj Comput. Mater., 2017, 3, 1–4, DOI:10.1038/s41524-017-0017-z.
- B. Li, X. Li, W. Gao and Q. Jiang, An effective scheme to determine surface energy and its relation with adsorption energy, Acta Mater., 2021, 212, 116895, DOI:10.1016/j.actamat.2021.116895.
- Y. Zhu, B. Luo, C. Sun, J. Liu, H. Sun, Y. Li and Y. Han, Density functional theory study of α-bromolauric acid adsorption on the α-quartz (1 0 1) surface, Miner. Eng., 2016, 92, 72–77 CrossRef CAS.
- V. R. Pavithra, T. Daniel Thangadurai, G. Manonmani, K. Senthilkumar, D. Nataraj, J. Jiya, K. Nandakumar and S. Thomas, Investigation on surface interaction between graphene nanobuds and cerium(III) via fluorescence excimer, theoretical, real water sample, and bioimaging studies, Mater. Chem. Phys., 2021, 264, 124453, DOI:10.1016/j.matchemphys.2021.124453.
- M. A. Hubbe, Insisting upon meaningful results from adsorption experiments, Sep. Purif. Rev., 2022, 51, 212–225 CrossRef.
- D. Q. Gbadago, J. Moon, M. Kim and S. Hwang, A unified framework for the mathematical modelling, predictive analysis, and optimization of reaction systems using computational fluid dynamics, deep neural network and genetic algorithm: a case of butadiene synthesis, Chem. Eng. J., 2021, 409, 128163 CrossRef CAS.
- L. T. Yogarathinam, K. Velswamy, A. Gangasalam, A. F. Ismail, P. S. Goh, M. N. Subramaniam, M. S. Narayana, N. Yaacob and M. S. Abdullah, Parametric analysis of lignocellulosic ultrafiltration in lab scale cross flow module using pore blocking and artificial neural network model, Chemosphere, 2022, 286, 131822 CrossRef CAS PubMed.
- L. I. Weidong, M. K. Suhayb, L. Thangavelu, H. A. Marhoon, I. Pustokhina, U. F. Alqsair, A. S. El-Shafay and M. Alashwal, Implementation of AdaBoost and genetic algorithm machine learning models in prediction of adsorption capacity of nanocomposite materials, J. Mol. Liq., 2022, 350, 118527 CrossRef.
- M. Fan, J. Hu, R. Cao, K. Xiong and X. Wei, Modeling and prediction of copper removal from aqueous solutions by nZVI/rGO magnetic nanocomposites using ANN-GA and ANN-PSO, Sci. Rep., 2017, 7, 1–14 CrossRef PubMed.
- W. Wang, X. Wu and S. Long, Optimizing the Methylene Blue Removal from Aqueous Solution Using Pomelo Peel Based Biochar Assisted by RSM and ANN-PSO, Pol. J. Environ. Stud., 2022, 31, 329–346 CrossRef CAS.
- S. K. Bhagat, T. M. Tung and Z. M. Yaseen, Development of artificial intelligence for modeling wastewater heavy metal removal: state of the art, application assessment and possible future research, J. Cleaner Prod., 2020, 250, 119473 CrossRef CAS.
- G. Alam, I. Ihsanullah, M. Naushad and M. Sillanpää, Applications of artificial intelligence in water treatment for optimization and automation of adsorption processes: recent advances and prospects, Chem. Eng. J., 2022, 427, 130011 CrossRef CAS.
- H. E. Reynel-Ávila, I. A. Aguayo-Villarreal, L. L. Diaz-Muñoz, J. Moreno-Pérez, F. J. Sánchez-Ruiz, C. K. Rojas-Mayorga, D. I. Mendoza-Castillo and A. Bonilla-Petriciolet, A Review of the Modeling of Adsorption of Organic and Inorganic Pollutants from Water Using Artificial Neural Networks, Adsorpt. Sci. Technol., 2022, 2022 Search PubMed.
- Z. M. Yaseen, An insight into machine learning models era in simulating soil, water bodies and adsorption heavy metals: review, challenges and solutions, Chemosphere, 2021, 277, 130126 CrossRef CAS PubMed.
- S. P. G. Zaferani, M. R. S. Emami, M. K. Amiri and E. Binaeian, Optimization of the removal Pb (II) and its Gibbs free energy by thiosemicarbazide modified chitosan using RSM and ANN modeling, Int. J. Biol. Macromol., 2019, 139, 307–319 CrossRef CAS PubMed.
- A. Rodríguez-Romero, D. I. Mendoza-Castillo, H. E. Reynel-Ávila, D. A. de Haro-Del Rio, L. M. González-Rodríguez, A. Bonilla-Petriciolet, C. J. Duran-Valle and K. I. Camacho-Aguilar, Preparation of a new adsorbent for the removal of arsenic and its simulation with artificial neural network-based adsorption models, J. Environ. Chem. Eng., 2020, 8, 103928 CrossRef.
- P. S. Pauletto, G. L. Dotto and N. P. Salau, Optimal artificial neural network design for simultaneous modeling of multicomponent adsorption, J. Mol. Liq., 2020, 320, 114418 CrossRef CAS.
- C. Escobar, C. Soto-Salazar and M. I. Toral, Optimization of the electrocoagulation process for the removal of copper, lead and cadmium in natural waters and simulated wastewater, J. Environ. Manage., 2006, 81, 384–391 CrossRef CAS PubMed.
- Y. Wang, S. Wang, T. Xie and J. Cao, Activated carbon derived from waste tangerine seed for the high-performance adsorption of carbamate pesticides from water and plant, Bioresour. Technol., 2020, 316, 123929 CrossRef CAS PubMed.
- J. L. Fagundez, M. S. Netto, G. L. Dotto and N. P. Salau, A new method of developing ANN-isotherm hybrid models for the determination of thermodynamic parameters in the adsorption of ions Ag+, Co2+ and Cu2+ onto zeolites ZSM-5, HY, and 4A, J. Environ. Chem. Eng., 2021, 9, 106126 CrossRef CAS.
|
This journal is © The Royal Society of Chemistry 2023 |