DOI:
10.1039/D3TA00324H
(Paper)
J. Mater. Chem. A, 2023,
11, 11187-11201
Hybrid-biotaxonomy-like machine learning enables an anticipated surface plasmon resonance of Au/Ag nanoparticles assembled on ZnO nanorods†
Received
18th January 2023
, Accepted 3rd April 2023
First published on 5th April 2023
Abstract
Sustainable energy strategies, particularly alternatives to fossil fuels, e.g., solar-to-hydrogen production, are highly desired due to the energy crisis. Therefore, materials leading to hydrogen production by utilizing water and sunlight are extensively investigated, such as nanomaterials modified by gold nanoparticles (AuNPs) of different structures, which enable photoelectrochemical water splitting through light-to-plasmon resonance. However, light-to-plasmon resonance depends on the gold nanoparticles' properties. Therefore, an accurate projection model, which correlates the fabrication parameters and light-to-plasmon resonance, can facilitate the selection and the subsequent application of AuNPs. In this regard, we established a hybrid-biotaxonomy-like machine learning (ML) model based on genetic algorithm neural networks (GANN) to investigate the light-to-plasmon properties of a six-layer coating of noble metal nanoparticles (NMNPs) on ZnO nanorods. Meanwhile, we understood the plasmonic peak shift of every NMNP coating layer by exploiting the multivariate normal distribution method and the concept of phylogenetic nomenclature from evolutionary developmental biology.
Introduction
The worldwide reliance on fossil fuels and the consequent supply shortage have caused a severe energy crisis, leading to an urgent need for sustainable energy strategies and alternatives to fossil fuels. Therefore, numerous efforts have been dedicated to nano-energy materials, particularly for solar-to-hydrogen production.1–7 Nano-energy materials have unique reactivity to light, which can polarize electrons on their surfaces and facilitate electron excitation to the conduction band.8–15 Hence, nano-energy materials, particularly nano-metal oxides, manifest unique properties, e.g., light-to-plasmon resonance, which can be further applied to improve the performance of solar cells, optical sensors, and gas sensors.16–20 Among these nano-metal oxides, ZnO, a typical material for photocatalysts and sensors, displays promising application potential due to its high photosensitivity, low cost, and stability.6,7,9,10,21 Furthermore, vertically aligned ZnO nanorods (ZnONRs), thanks to the high length/diameter ratio, high surface area, and short electron–hole pair separation distance, are the optimum morphology to improve the light absorption ability, leading to enhanced efficiency of light-driven photoelectrochemical (PEC) water splitting.11,12,22–24 However, most PEC materials for water splitting can only utilize a limited amount of solar light (roughly 4%) due to their wide band gap (3.4 eV) and the consequent performance happening only in the ultraviolet light region.25 Numerous efforts have been made to improve the photo-response, hydrogen evolution rate, and photocatalyst activity by coating noble metal nanoparticles (NMNPs)13,14 onto metal oxide semiconductors, e.g., ZnO.26–31 But in most studies AuNPs of a single structure were coated onto metal oxides, slightly improving light utilization at a small absorption band located within a narrow range of visible light.32–35 To expand the light utilization to a wider visible light region and even the NIR region, a multilayer coating of NMNPs on metal oxides has demonstrated its feasibility.36
Nevertheless, a delicate multilayer coating design and parameter selection for fabrication are crucial to developing a material of anticipated light utilization. Therefore, it is beneficial to establish a prediction model coordinating the fabrication parameters and the yielded functions of the material. However, conventional modeling can hardly discover such coordination as the complex fabrications lead to a non-linear relation between the fabrication input and output beyond description by physical laws. Such challenges can be eradicated by applying a machine learning (ML) model based on genetic algorithm neural networks (GANN), which is a powerful tool to optimize the fabrication process and predict outcomes, while avoiding unnecessary costly experiments.37–40 Nowadays, many studies based on GANN have been conducted to study the fluid dynamics and plasmon resonances of NPs.40–45 To employ GANN for process optimization and outcome prediction, a careful parameter setting is necessary to construct the model accurately. In this regard, we propose a unique concept, hybrid biotaxonomy inspired by recombinant DNA technology, to select parameters for the multilayer coating of NMNPs in order to train the GANN model.
Artificial neural networks (ANN), a computational intelligence system simulating the behavior of the human brain and neurons, are composed of basic elements called artificial neurons, which are highly interconnected in layers. The layers contain an input layer, an output layer, and numerous hidden layers, providing the adjustment in weight for every datapoint in the receiving and filtering process, thereby enabling us to construct a non-linear relationship model. Additionally, a genetic algorithm (GA), inspired by the biological evolution process, is developed to assist the ANN system during the training of neural networks. Briefly, the basic elements of the GA are chromosomes, fitness selection, and bio-inspired operators. In chromosomes, two possible alleles (variant forms of genes), 0 and 1, are on each locus (specific position on the chromosome). The chromosomes are considered as points in the solution space, further utilizing the genetic operator through iterative reproduction and population replacement. Subsequently, all the chromosomes are assigned a value by the fitness function. Additionally, the operators have three steps to reproduce the next generation, i.e., selection, crossover, and mutation. The selection retains the optimum genes determined by the fitness values of the chromosomes, thus enabling the reproduction of the promising population in the solution space. In the crossover operator, two or more random chromosomes are selected to mate, leading to the exchange of the genes and generating the potential offspring. The mutation operator diversifies the original population, as some loci on chromosomes will be flipped or randomly alternated.
The hybrid biotaxonomy aspect plays a crucial role in biotechnology, agriculture, clinical diagnosis, veterinary medicine, etc. Taxonomy is applied in different systems, e.g., mammalian systems, insect expression systems, bacterial systems, filament fungi, yeasts, and unicellular cells.15,46–57Escherichia coli is therefore extensively used thanks to the fast growth kinetics and low production costs,58,59 consequently leading to substantial application in protein taxonomy through various plasmid expressions.59,60 Nowadays, biologists are increasingly applying evolutionary developmental biology to address environmental challenges, food crises, and health care, in which tree-shaped diagrams, namely phylogenetic trees, are often utilized to understand the evolutionary relationships between the species and populations, and to solve the respective challenges. For example, researchers in medical science have used phylogenetic trees to figure out the origin of a virus and subsequently to develop suitable treatments.61,62 In ecological studies, researchers have employed phylogenetic trees to classify the species and the related probability of occurrence based on environmental factors, like the pH value of water, insolation duration, and temperature of the growing plant, to investigate the diversity in a phylogeny.63,64 In addition, research concerning evolutionary biology has mainly investigated the similarities of the outcomes by comparing evolutionary trees through different methods to uncover the far-reaching implications and present the diversity of the evolved species.65
However, the concept of evolutionary developmental biology is rarely used to study a phenomenon or a property in the engineering field. We herein propose the utilization of phylogenetic trees to describe the property evolution of plasmonic nanoparticles (NPs), which were evaluated according to the dielectric environment, coupling effect, sizes, NP shapes, and effect of reactants. We subsequently established an evolutionary tree for plasmonic NPs by considering the branches of independent pathways, which can help to organize the results, trace the origin of NPs, and consequently create a library of NP characteristics impacted by the tunable parameters. We furthermore considered the multilayer coating of NMNPs as a sequence of protein taxonomy, namely the synergistic plasmonic bands yielded by the six-layer coating of NMNPs. Meanwhile, tuning the experimental parameters for the multilayer coating, i.e., reactant amounts, coating method, and time, is analogous to engineering plasmids, which leads to the protein taxonomy. The multilayer coating of NMNPs on ZnONRs was herein realized through the layer-by-layer coating method, which thus extended the overall light absorption ability and enabled their application in the visible light and the NIR region due to the localized surface plasmon resonance (LSPR) effect of NMNPs. Furthermore, we tuned several parameters inspired by the hybrid protein taxonomy to fabricate the spherical (S)–Ag/S–Au–Ag/S–Au–Ag (575)/urchin-like Au (ULA)/AuNRs/S–Au/ZnONRs samples, whose optical absorbance was subsequently investigated to determine the plasmonic peaks generated by the NMNPs of each layer. In this work, we successfully demonstrated a machine learning (ML) model with low data training established by utilizing the genetic algorithm-based artificial neural networks (GANN) and phylogenetic trees of plasmonic peak evolution of NPs caused by the coupling effect. This model enables prediction of plasmonic properties of the anticipated coating of AuNPs, which can be potentially applied to select optimal parameters to develop materials of desired light-to-plasmon resonance, particularly for designing photocatalysts, solar-to-hydrogen production, and gas sensors.
Results & discussion
Structural characterization
Localized surface plasmon resonance (LSPR) is a cumulative resonant oscillation of the conduction electrons induced by the interaction between plasmonic NPs and an electromagnetic field with specific light irradiation. Therefore, most plasmonic NPs cause strong absorption bands in UV-vis spectra and strong electromagnetic near-field enhancements.66–69 It is well known that the plasmonic peaks' position strongly depends on the shape, size, and surface charge. With increasing NP size or the growth of pods on NPs, LSPR can increase sharply and cause a shift of the plasmonic absorption band toward a longer wavelength, consequently exhibiting a wide light absorption band compared to spherical NPs of similar sizes.70 In our study, the UV-vis absorption spectra and the morphology of multiple species of NMNPs were consistent with other research. Noble metal nanoparticles of multiple shapes and sizes were synthesized and investigated by TEM (Fig. 1a–f & S2†). The spherical Au nanoparticles processed with photoreduction for 10 min were mostly deposited at the tip and on the side of ZnONRs, where the diameter of S–Au NPs was about 10–20 nm (Fig. 1a). The morphology of AuNRs synthesized by a seed-mediated method is displayed in Fig. 1b, revealing that the aspect ratio of AuNRs was around 3.12 (length/width = 39.14 nm/12.53 nm). The urchin-like Au nanoparticles with diameter in the 70–80 nm range were synthesized by a seed-mediated method (Fig. 1c). Two kinds of spherical Au–Ag nanoparticles of molar ratios 3 and 1/3 were respectively prepared as in Fig. 1d and e. Their diameters were respectively in the 80–90 nm and 120–130 nm ranges. Additionally, the spherical Ag nanoparticles in Fig. 1f had a diameter in the 10–20 nm range.
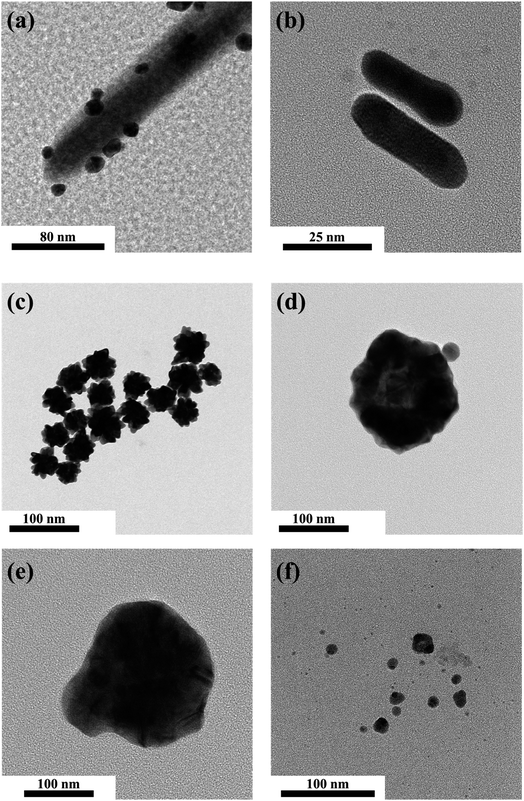 |
| Fig. 1 TEM images of (a) spherical Au/ZnONRs, (b) Au nanorods, (c) urchin-like Au, (d) spherical Au–AgNPs (molar ratio of Au/Ag = 3), (e) spherical Au–Ag (molar ratio of Au/Ag = 1/3), and (f) spherical Ag. | |
The morphology and structure of 1–6 layers of NMNPs decorated on ZnONRs were characterized by SEM in Fig. 2 & S3.† The average length and diameter of ZnONRs are 1.5 μm and 100 nm. The attachment of S–Au NPs mainly happened at the top and on the side of ZnONRs in Fig. 2a, confirming the structure of S–Au/ZnONRs. Then, the deposition of AuNRs, urchin-like Au, two S–Au–Ag NPs, and S–Ag through the layer-by-layer coating method can be observed on the previously deposited NPs and ZnONRs in Fig. 2b–f, confirming the structure of S–Ag/S–Au–Ag (448)/S–Au–Ag (575)/ULA/S–Au/ZnONRs. Furthermore, the surface coverage of the NPs on ZnONRs increased up to 60.58% in Fig. 2f, indicating a large attachment of every NP. We further performed energy-dispersive X-ray (EDX) spectroscopic analysis to ensure the anticipated coating in Fig. S1.†
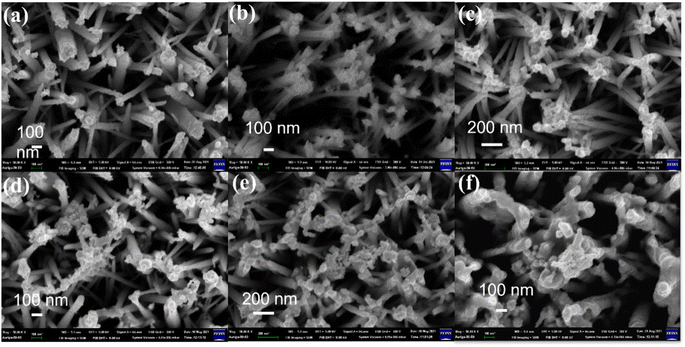 |
| Fig. 2 SEM top view images of (a) S–Au/ZnONRs, (b) S–Au/ZnONRs, (c) ULA/S–Au/ZnONRs, (d) S–Au–Ag (575)/ULA/S–Au/ZnONRs, (e) S–Au–Ag (448)/S–Au–Ag (575)/ULA/S–Au/ZnONRs, and (f) S–Ag/S–Au–Ag (448)/S–Au–Ag (575)/ULA/S–Au/ZnONRs. | |
Surface plasmon resonance and design of ML methods
To enable light absorption in the visible light and the NIR region for the heterostructure, NMNPs of different plasmonic resonances were synthesized. The UV-vis absorbances of the plasmonic NPs used for the deposition on ZnONRs are illustrated in Fig. 3a–e. In Fig. 3a, AuNRs suspended in water (2nd layer) displayed two bands, respectively at 590 nm (transverse electron oscillation) and 736 nm (longitudinal electron oscillation) as their unique morphologies induced the special surface plasmon resonance effect. However, the solution of ULA (3rd layer), S–Au–Ag (4th layer), S–Au–Ag (5th layer), and S–Ag (6th layer) all showed a single band respectively at 620 nm, 575 nm, 448 nm and 402 nm illustrated in Fig. 4b–e. UV-vis spectroscopy was used to investigate the light absorption properties of multilayer NMNPs decorated ZnONRs in Fig. 3f. With the deposition of S–AuNPs, the result manifested plasmonic resonance happening at 522 nm, and the light absorption band located in the 400–800 nm range, ascribed to the surface plasmon resonance of S–AuNPs. But the plasmonic peaks shifted from 522 nm to 544 nm due to the coupling effect of AuNRs and S–AuNPs, and the plasmonic resonance contributed by the deposited AuNRs in the red line of Fig. 3f. Meanwhile, the resonance peak appeared at 808 nm caused by the slight aggregation of AuNRs suspended in ethylene glycol, which has a longitudinal surface plasmonic resonance shift from 736 nm to 808 nm. With the deposition of ULA NPs and S–Au–Ag (NPs) by the electrophoretic deposition (EPD) method, the peak at 537 nm further showed a red shift to 560 nm, which was attributed to the NPs of a plasmonic resonance at a longer wavelength. Moreover, the deposition of S–Au–Ag (448) and S–AgNPs by a self-assembly method led to plasmonic resonances at the wavelengths respectively of 452 nm and 420 nm for 5-layer and 6-layer NMNPs. The plasmonic resonance exhibited a blue shift due to the plasmonic resonance of S–AgNPs suspended in ethylene glycol at 402 nm. In summary, with the multilayer coating of NMNPs of different plasmonic resonances deposited layer by layer on ZnONRs, the overall light absorption ability can be highly enhanced and extended to a wider region including the visible light and the NIR region thanks to the surface plasmon resonance effect.
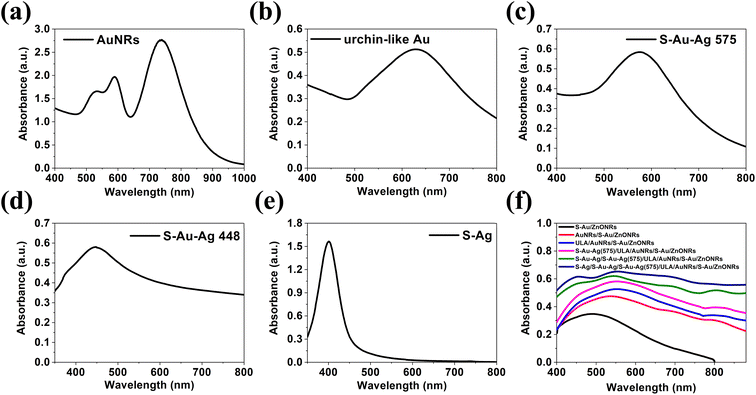 |
| Fig. 3 UV-vis absorbance of (a) Au nanorods suspended in water, (b) urchin-like Au suspended in water, (c) S–Au–Ag (molar ratio of Au/Ag = 3) suspended in water, (d) S–Au–Ag (molar ratio of Au/Ag = 1/3) suspended in water, (e) S–Ag suspended in ethylene glycol, and (f) S–Ag/S–Au–Ag (448)/S–Au–Ag (575)/ULA/AuNRs/S–Au/ZnONRs in air. | |
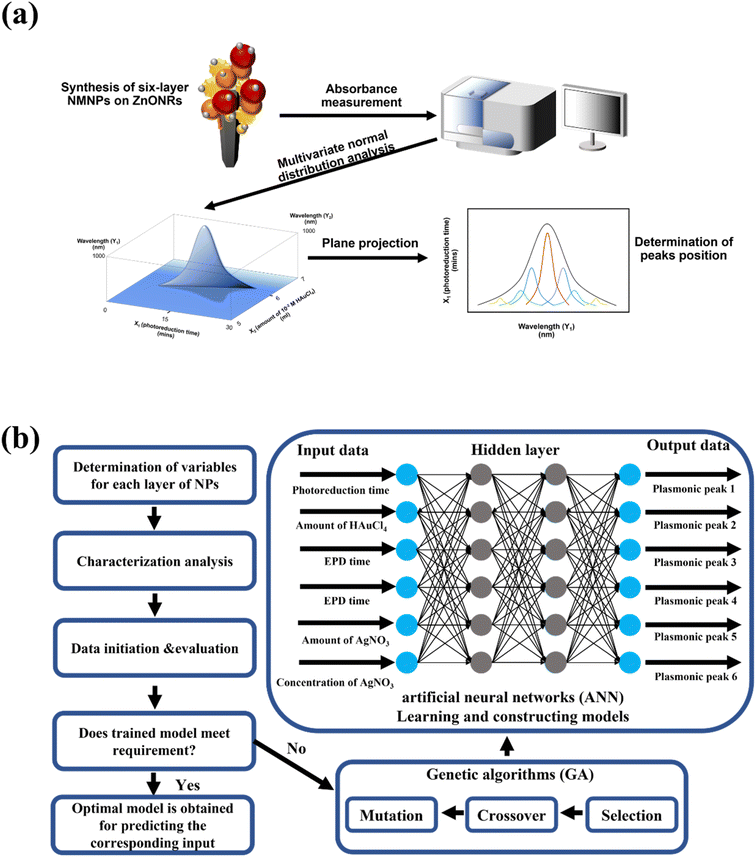 |
| Fig. 4 (a) Schematic representation of the analysis procedure for the UV-vis absorption spectrum of six-layer NMNPs on ZnONRs, and (b) flow chart of the model training by GANN for the plasmon resonance prediction of the heterostructure. | |
The entire analysis of the six-layer NMNPs on ZnONRs is shown in Fig. 4a. After a successful coating of NMNPs on ZnONRs, the samples' absorption spectra were measured step-by-step from 1-layer to 6-layer to overview the synergistic plasmonic resonances. The absorption spectra of every NMNP layer fabricated by tuning parameters served as the database for investigating the respective multivariate normal distributions of the wavelength scheme, leading to a seven-dimensional graph. To gain insight into the plasmonic resonance of every NMNP layer, the projection of multivariate normal distribution was applied to obtain a univariate normal distribution. The projected planes can herein derive selected univariate parameters–wavelength graphs to facilitate recognition of surface plasmon resonance for the selected fabrication parameters. This concept is further elaborated in Fig. 5. The established database was further employed to achieve a machine learning model by applying GANN, leading to a successful prediction and optimization to develop the multilayer NMNPs coated on ZnONRs. The developed model can moreover provide an understanding of the interaction between plasmonic resonances and coating parameters for every layer (Fig. 4b). The coating variables for every layer were primarily organized through the selection concept of protein taxonomy as in Table 1. The optical properties of every coated layer, analogous to the protein taxonomy caused by protein folding, were measured by the UV-vis absorption spectra and analyzed by multivariate normal distribution to understand the impact of every individual coating layer. Therefore, we considered the photoreduction time (X1), amount of 10−2 M HAuCl4 (X2), EPD time for depositing ULA NPs (X3), EPD time for depositing S–Au–Ag NPs (X4), amount of 10−3 M AgNO3 (X5), and concentration of AgNO3 (X6) as inputs, and plasmonic resonance of every layer as outputs, to train the GANN models.
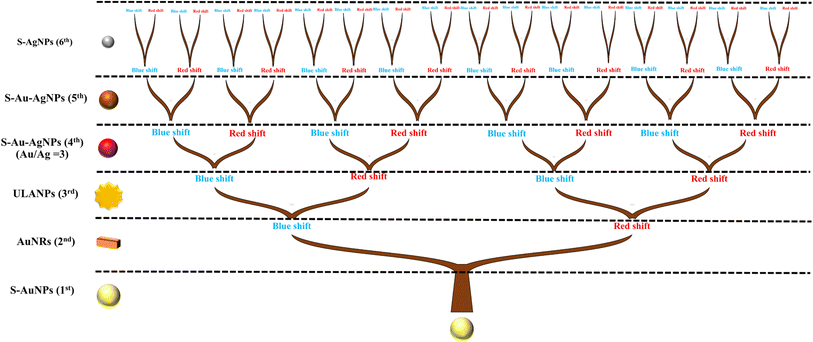 |
| Fig. 5 Evolutionary tree of plasmonic resonance shift of S–AuNPs, AuNRs, ULANPs, S–Au–AgNPs (575), and S–Au–AgNPs, induced by the coupling effect by multiple species of plasmonic NPs on every layer. | |
Table 1 Experimental parameters of samples for machine learning
X
1 (UV treatment time) |
X
2 (amount of 10−3 M HAuCl4) |
X
3 (EPD time) |
X
4 (EPD time) |
X
5 (amount of 10−3 M AgNO3) |
X
6 (concentration of AgNO3) |
10 min |
5 mL |
0.5 h |
0.5 h |
3 mL |
0.1 M |
30 min |
7 mL |
1 h |
1 h |
6 mL |
0.01 M |
Analysis of plasmonic coupling effects
The plasmonic coupling effect is a significant factor in impacting the plasmonic resonance of the various NPs due to their multilayer coating. Such a plasmonic coupling effect happens when the plasmonic NPs are close to each other at a distance much smaller than their size.71,72 Namely, the near field of one particle interacts with that of the adjacent particles. Therefore, the surface plasmon resonance of two or more NPs can strongly hybridize, strengthening the electromagnetic field near the surface and that of the interface. Surface plasmon resonance is additionally very sensitive to the medium of the surroundings. The surface plasmon resonance of each single layer is different from that in the layer-by-layer assembled surroundings, which can further cause the generation of multilayer conditions to predict surface plasmon resonance. To further demonstrate the plasmonic resonance shift of NPs induced by the coupling effect, the evolutionary tree of S–AuNPs (1st), AuNRs (2nd), ULANPs (3rd), S–Au–AgNPs (575) (4th), S–Au–Ag (5th) hybridizing with other NPs is schematically illustrated in Fig. 5. Surface plasmon resonance can be affected by the surrounding dielectric medium and geometry. The shift of plasmonic resonance is based on the surrounding dielectric medium and geometry non-linearly. Thus, the evolutionary tree system embraces many kinds of information related to evolutionary change, cladogenesis, and anagenesis. The tree branches reflect the relative relationships, which reveal the common ancestor of the NPs. However, evolution can cause species to become extinct or extant, corresponding to the plasmon resonance shift. The coupling effect in Fig. 5 between S-AuNPs and AuNRs induced the plasmonic resonance shift of S–AuNPs when AuNRs were coated on the S–AuNPs outer layer, namely, one evolutionary process for the S–AuNPs. The plasmonic resonance shift of the NPs was divided into blue and red shifts compared to the plasmonic resonance of the previous NP layer. And the data processing of absorption spectra converting the multivariate normal distribution to the univariate normal distribution can derive the overall curve of the univariate normal distribution composed of multiple curves, revealing every individual univariate normal distribution. These individual univariate normal distributions revealed the plasmonic resonance in their own curves, indicating that the plasmonic resonances were also affected by the post-depositional NPs. Hence, when the AuNRs (2nd) were coated on S–AuNPs (1st), simultaneously, some plasmonic resonance shifted to a longer wavelength (red shift), and some shifted to a shorter wavelength (blue shift), manifesting an evolution process. Similarly, when the ULANPs (3rd) were coated on S–AuNPs and AuNRs, the evolution for both NPs repeated, namely the plasmonic resonance shifts. Therefore, after completing the six-layer coating of NMNPs, the plasmonic resonances of S–AuNPs evolved five times and split into 32 species. As for AuNRs, ULANPs, S–Au–AgNPs (575), S–Au–AgNPs, and S–AgNPs, their plasmonic resonance would split into 16, 8, 4, 2, and 1 species, respectively, in the evolutionary process. However, applying the plane projection (the plane can be selected) to evaluate the relationship between the multivariate normal distribution of plasmonic resonance and experimental parameters can only differentiate blue shift from red shift by comparison with the original peaks of S–AuNPs on ZnONRs. Nevertheless, the main plasmonic resonance of NMNPs tended to red shift in the evolutionary process, implying that the red shift of the plasmonic resonance was the main characteristic of the surviving species, and the blue shift was that of the most extinct species.
The plasmonic resonance investigated from one specific layer can be impacted by the other layers due to the coupling effect. The prediction of plasmon resonances through the well-trained GANN model is displayed in Fig. 6 and 7. Such a model can further be exploited to reveal the plasmon resonance information for the variables in the given region. We further illustrated the predicted data by 2D contour plotting to visualize the correlation between the fabrication parameters and the plasmonic properties of the coating layer. The shift of plasmonic resonance of S–Au (1st layer), AuNRs (2nd layer), and ULA (3rd layer) impacted by the variable parameters for coating the NP layers was evaluated in Fig. 6. We focused on examining the integrated impact on the plasmonic resonance and its respective shift caused by the contacting layers, matched by the model training through the GANN ML method. We measured the plasmonic resonance shifts of S–AuNPs (1st layer) after coating AuNRs and ULANPs through tuning X1, X2, and X3, as shown in Fig. 6a and b. The plasmonic resonance of the S–Au on ZnONRs manifested a red shift from 512 nm to 522 nm when the photoreduction time (X1) was increased (Fig. 3f). Moreover, the coating of AuNRs on S–Au led to a red shift of the plasmonic resonance from 530 nm to 540 nm. Meanwhile, a higher length/width ratio of AuNRs coated on S–Au/ZnONRs can be yielded with an increasing amount of 10−2 M HAuCl4 (X2), causing a shift of plasmonic resonance to a longer wavelength due to the coupling effect. Therefore, a longer wavelength where plasmonic resonance happened due to the increase in X1 and X2 was roughly 536 nm, revealed by Fig. 6a. The plasmonic resonance of S–AuNPs as evaluated in Fig. 6b was regulated by the amount of HAuCl4 (X2) especially when the ULANPs were coated on the AuNRs/S–Au/ZnONRs, indicating that the EPD time (X3) for depositing ULA NPs was less influential in this respect. This was impacted by the shielding of the AuNRs coated on the surface of S–AuNPs. Hence most of the coated ULANPs tended not to interact with S–AuNPs. The impact of X1, X2, X3, and X4 on the shift of plasmonic resonance of AuNRs (2nd layer) was examined in Fig. 6c–e. The plasmonic resonance of the AuNRs coated on S–AuNPs was mainly influenced by the amount of HAuCl4 (X2) instead of photoreduction time (X1), as displayed in Fig. 6c. The increasing amount of HAuCl4 caused a big length-to-width ratio of AuNRs, exhibiting a strong red shift of longitudinal plasmonic resonance from 736 nm to 844 nm in Fig. 3a. This observation indicated a strong aggregation of AuNRs on S–Au/ZnO coated through the self-assembly method. However, the coating of ULANPs on AuNRs slightly influenced the plasmonic resonance, as revealed in Fig. 6d, which might be attributed to the high aggregation of AuNRs, causing a nonsignificant coupling effect. Moreover, the shift of plasmonic resonance after the S–Au/ZnONRs were coated by the AuNRs (2nd layer) was examined at two EPD times (X3 and X4) as investigated in Fig. 6e, revealing that X3 and X4 were less influential in the shift of the plasmonic resonance than the high aggregation of AuNRs. We further studied the impacts of X2, X3, X4, and X5 on the shift of the plasmonic resonance after a coating of ULANPs (3rd layer) on AuNRs/S–Au/ZnONRs (Fig. 6f–i). The plasmonic resonance in Fig. 6f manifested nearly no shift when X1 and X2 varied. But the small amount of HAuCl4 (X2) and the increasing EPD time (X3) led to a prominent red shift (Fig. 6g). We noticed that the plasmonic resonance of ULANPs was mainly controlled by the EPD time (X3) but was only slightly influenced by the coating of Au–Ag (575) NPs. Additionally, we found the shift of the plasmonic resonance of the ULANPs was regulated by EPD time (X4) and the amount of 10−3 M AgNO3 (X5) (Fig. 6i). We, therefore, found the coating of S–Au–Ag (575) (4th layer) instead of S–Au–Ag (5th layer) played a crucial role in the shift of the plasmonic resonance of ULANPs. Furthermore, X3 was pivotal in shifting the plasmonic resonance of ULANPs (3rd layer) (Fig. 6f–i), indicating that increasing the EPD time and increasing the amount of ULANPs coated on ZnONRs have a significant impact on the self-induced coupling effect.
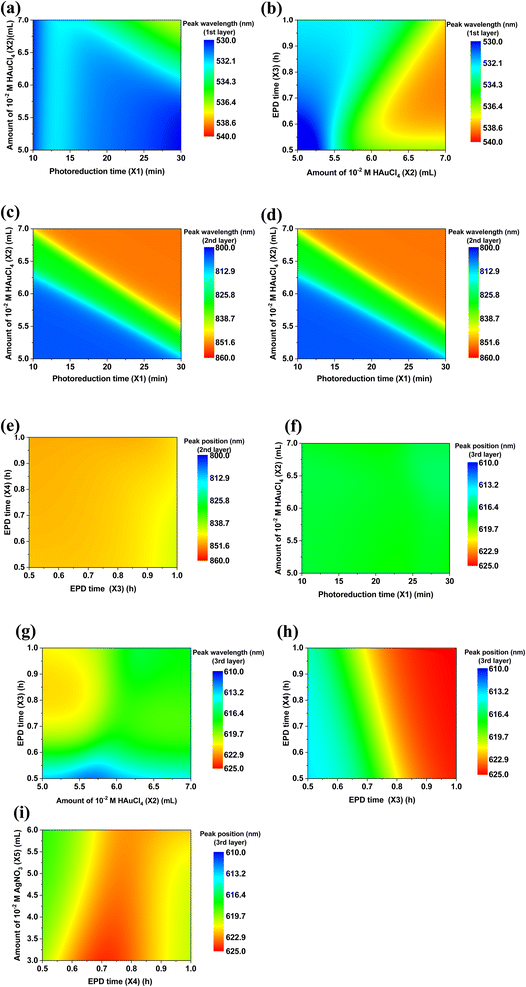 |
| Fig. 6 Plasmonic peaks prediction of S–AuNPs on ZnONRs with tuning (a) X1 and X2, and (b) X2 and X3. Peaks prediction of AuNRs on ZnONRs with tuning (c) X1 and X2, (d) X2 and X3, and (e) X3 and X4. Peaks prediction of urchin-like Au on ZnONRs with tuning (f) X1 and X2, (g) X2 and X3, (h) X3 and X4, and (i) X4 and X5 using the GANN method. | |
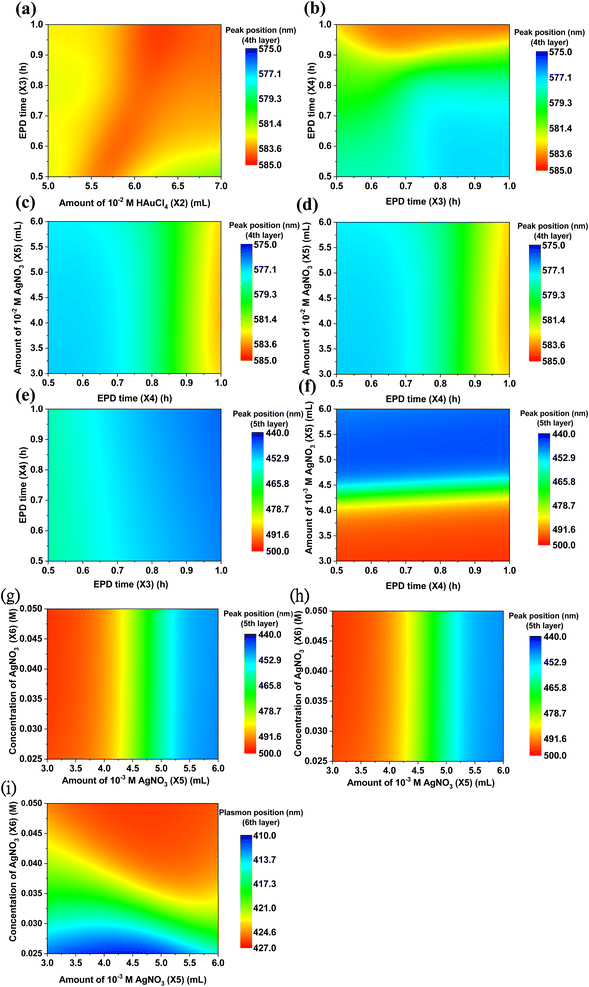 |
| Fig. 7 Plasmonic peaks prediction of S–Au–Ag (575) on ZnONRs with tuning (a) X2 and X3, (b) X3 and X4, (c) X4 and X5, and (d) X5 and X6. Peak prediction of S–Au–Ag (448) on ZnONRs with tuning (e) X3 and X4, (f) X4 and X5, and (g) X5 and X6. Peaks prediction of S–Ag on ZnONRs with tuning (h) X4 and X5 and (i) X5 and X6 using the GANN method. | |
The influence of the adjusted fabrication parameters on the shift of the plasmonic resonance of S–Au–Ag (575) (4th layer), S–Au–Ag (5th layer), and S–Ag (6th layer) is illustrated in Fig. 7. The plasmonic resonance of S–Au–Ag (575) was mainly affected by EPD time (X4) for depositing S–Au–Ag NPs (Fig. 7a–d). Increasing parameter X4 up to 1 hour led to the plasmonic resonance of S–Au–Ag (575) on ULA/AuNRs/S–Au/ZnO shifting to a longer wavelength up to 583 nm. X5 and X6, as revealed in Fig. 7d, had less influence than X4, caused by the same phenomenon as ULANPs. The adjustment of the fabrication parameters of X3, X4, X5, and X6 caused the plasmonic resonance shift of S–Au–Ag (5th layer) (Fig. 7e–g). EPD time X3 and EPD time X4 had little effect on the plasmonic resonance of S–Au–Ag (5th layer) (Fig. 7e). The similar results in Fig. 7f–g suggested that the amount of 10−3 M AgNO3 (X5) was the key to the plasmonic resonance of S–Au–AgNPs (5th layer), while the EPD time (X4) and concentration of AgNO3 (X6) displayed an implicit impact. Hence the coating procedure had significant modulation of the yielded plasmonic resonance of S–Au–AgNPs (5th), consequently generating an unremarkable coupling effect between the S–Au–AgNPs and the NMNPs of other layers. The plasmonic resonance evolution of S–AgNPs (6th layer) on top of the previous five layers was investigated by adjusting the fabrication parameters of X4, X5, and X6 (Fig. 7h and i). But we found that the plasmonic resonance of S–AgNPs (6th layer) manifested an unregular shift when the amount of 10−3 M AgNO3 (X5) and EPD time (X4) increased (Fig. 7h). Nevertheless, X6 had a crucial impact on modulating the plasmonic resonance of S–AgNPs (6th layer) (Fig. 7i). The increased concentration of AgNO3 (X6) led to a higher level of S–AgNPs (6th layer) aggregation, consequently causing a red shift of the plasmonic resonance after a successful coating.
Conclusion
Multilayer NMNPs coated ZnONRs, the S–Ag/S–Au–Ag (448)/S–Au–Ag (575)/ULA/AuNRs/S–Au/ZnONRs heterostructure, were successfully synthesized by the layer-by-layer method. Numerous plasmonic bands and enhancement of light absorption ability of the heterostructures contributed by the expression of six-layer NMNPs can be observed in optical absorbance as the NMNP coating layers of various shapes and sizes contained specific plasmonic bands in the visible light and NIR regions due to the LSPR effect and coupling effect. To investigate the relationship between the plasmonic resonances and each NP coating layer by utilizing the concept of biological evolution, we synergized the multivariate normal distribution method and GANN to construct a prediction model, which was trained with a low data set by considering the yielded plasmonic resonance of every coating layer as protein taxonomy and the respective experimental parameters, i.e., photoreduction time (X1), amount of 10−2 M HAuCl4 (X2), EPD time X3, EPD time X4, amount of 10−3 M AgNO3 (X5), and the concentration of AgNO3 (X6). We found the plasmonic resonance of every layer can be affected by the NPs in the respective proximity. Therefore, we achieved an optimization model through the GANN method to accurately predict the plasmonic resonance. Furthermore, the ML model using GANN to explore the light-to-plasmon properties of a six-layer coating of NMNPs on ZnO nanorods enables an essential optimization in the design of the solar-to-hydrogen water-splitting solar cell. Moreover, ML facilitated by GANN can be extensively applied to analyze and predict more complicated heterostructures ensuring a fast and anticipated design of materials for hydrogen production.
Materials and methods
Materials
Polyvinylpyrrolidone (PVP, 55
000 g mol−1), hydroquinone (C6H6O2, 99%), zinc acetate dihydrate (Zn(CH3COO)2·2H2O, 99%), sodium borohydride (NaBH4, 98%), and L-ascorbic acid (C6H8O6, 99.7–100.5%) were all obtained from Sigma-Aldrich. Silver nitrate (AgNO3, 99%) was purchased from Honeywell Fluka. 1,6-Hexanedithiol (HS(CH2)6SH, 97%), hexamethylenetetramine (HMTA, 99%), and (1-hexadecyl)trimethylammonium bromide (CTAB, 98%) were purchased from Alfa Aesar. 1-Hexanethiol (CH3(CH2)5SH, 96%) and hydrogen tetrachloroaurate(III) trihydrate (HAuCl4, 99.99%) were purchased from Acros Organics. Hexane (C6H14, 98.5%) was obtained from Fisher Chemical.
Preparation of ZnONRs and S–Au/ZnONRs heterostructure
20 mL of 0.025 M Zn(CH3COO)2 containing equimolar HMTA solution was prepared to obtain the ZnO seed solution, and a droplet of the seed solution was added to clean indium-tin-oxide (ITO). Then, the sample was heated to 350 °C for 10 min to obtain the seed layer. The ZnO seed layer was immersed vertically in 100 mL of 0.02 M Zn(CH3COO)2 and HMTA solution, followed by chemical bath deposition at 95 °C for 3 h. Afterward, the sample was heated to 350 °C for 30 min to obtain ZnONRs. A spherical Au/ZnONRs heterostructure was prepared by the photoreduction method. The ZnONRs were immersed in an aqueous solution of 50 mL 10−4 HAuCl4, followed by irradiation with UV light for 10–30 min to grow Au nanoparticles on ZnONRs. Then, the sample was rinsed and dried with N2. The photoreduction time was the variable of the first layer for machine learning, labeled as X1.
Preparation of Au nanorods/S–Au/ZnONRs heterostructure
The Au nanorods (AuNRs) were synthesized by a seed-mediated method, and the experimental process is described in the ESI† in detail. The plasmonic peaks of AuNRs were tuned by the amount of 10−2 M HAuCl4, 5–7 mL, used for machine learning. The coating of AuNRs on S–Au/ZnONRs was prepared by the self-assembly method. The S–Au/ZnONRs were immersed in 0.5% 1,6-hexanedithiol and 0.5% 1-hexanethiol in hexane at room temperature for one day to form the sulfhydryl group on the S–Au NPs and ZnONRs, and then rinsed with alcohol. Subsequently, the thiol and dithiol-mediated S–Au/ZnONRs were immersed again in the 2 mL AuNRs solution suspended in ethylene glycol for one day, then rinsed with alcohol and dried with N2. The amount of 10−2 M HAuCl4 was the variable of the second layer for machine learning, labeled as X2.
Preparation of urchin-like Au (ULA)/AuNRs/S–Au/ZnONRs and S–Au–Ag (575)/ULA/AuNRs/S–Au/ZnONRs heterostructures
The synthesis process of urchin-like Au (ULA) NPs and spherical Au–Ag (575) (S–Au–Ag (575)) NPs is described in the ESI† in detail. The coating of ULA and S–Au–Ag was performed by the electrophoretic deposition (EPD) method. The experimental setup comprised AuNRs/S–Au/ZnONRs as a working electrode and silver wire as a reference and a counter electrode. The two electrodes, AuNRs/S–Au/ZnONRs and silver wire, were both immersed in ULA solution and then S–Au–Ag solution before and after by applying +2 V on the sample for 0.5 h to 1 h. The sample was subsequently rinsed with deionized water and dried with N2. After finishing the process, ULA/AuNRs/S–Au/ZnONRs and S–Au–Ag (575)/ULA/AuNRs/S–Au/ZnONRs were obtained. The EPD time of 0.5–1 h was the variable of the third and fourth layers for machine learning, labeled as X3 and X4.
Preparation of S–Au–Ag (448)/S–Au–Ag (575)/ULA/AuNRs/S–Au/ZnONRs and S–Ag/S–Au–Ag (448)/Au–Ag (575)/ULA/AuNRs/S–Au/ZnONRs heterostructures
The synthesis of S–Au–Ag (448) NPs, S–Au–Ag (500), S–Ag (402), and S–Ag (405) NPs is described in the ESI† in detail. Both the coatings of S–Au–Ag (448) NPs and S–Ag NPs were prepared by self-assembly, the same process as the coating of AuNRs. S–Au–Ag (448)/S–Au–Ag (575)/ULA/AuNRs/S–Au/ZnONRs were immersed in 0.5% 1,6-hexanedithiol and 0.5% 1-hexanethiol in hexane at room temperature for one day, followed by rinsing the sample with alcohol. Subsequently, the thiol and dithiol-mediated S–Au–Ag (448)/S–Au–Ag (575)/ULA/AuNRs/S–Au/ZnONRs were immersed again in 2 mL S–Au–Ag (448) solution to obtain the S–Au–Ag (448)/S–Au–Ag (575)/ULA/AuNRs/S–Au/ZnONRs. The S–Ag/S–Au–Ag (448)/Au–Ag (575)/ULA/AuNRs/S–Au/ZnONRs were obtained by repeating the above process but using the S–Ag solution instead of the S–Au–Ag (448) solution. Here, the amount of AgNO3 (3–6 mL) and the concentration of AgNO3 (0.025–0.05 M) were the variables of the fifth layer and sixth layer, respectively, utilized for machine learning, labeled as X5 and X6.
Determination of plasmonic resonances through the multivariate normal distribution method
The multivariate normal distribution is a generalization of a one-dimensional normal distribution to a multi-dimensional distribution (Gaussian distribution). The Gaussian distribution in one dimension can be defined as | 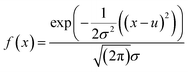 | (1) |
where x represents the plasmonic peaks in one dimension, u is the mean value of the wavelength for the plasmonic resonance, and σ is the standard deviation. Then, the generalization of the one-dimensional normal distribution to multiple dimensions can be written as | 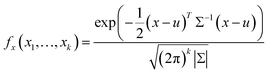 | (2) |
where x is a real k-dimensional column vector, u is the mean vector in k-dimensions, ∑ is the symmetric covariance matrix and ∑−1 is the inverse matrix defined as
and
X
1 (photoreduction time), X2 (the amount of 10−2 M HAuCl4), X3 (EPD time for depositing ULA NPs), X4 (EPD time for depositing S–Au–Ag NPs), X5 (the amount of AgNO3), X6 (the concentration of AgNO3), and X7 (the corresponding wavelength for the plasmonic resonance of the NPs coated on the ZnO and the previously coated NPs revealed by the UV-vis absorption spectra) are inserted into eqn (2). The complex multivariate normal distribution diagram can be obtained by constructing the 7-axis graph, which is composed of multiple parameters versus X7. Then, the projection plane method was used for the diagram by selecting the specific planes for projection, and the Xn (only one parameter)–wavelength (X7) dependent diagram (one-dimensional normal distribution) was drawn in a plane. Therefore, the determination of the main peaks of integrated peaks can be visualized to provide the input data for machine learning.
Integration of the artificial neural network (ANN) and genetic algorithm (GA)
The predictive model was established by hybridization of GANN by using Super PCNeuron 5.0 as previously reported.39,40,73 The variables of heterostructures by tuning photoreduction time (X1), amount of HAuCl4 (X2), EPD time X3, EPD time X4, the amount of 10−3 M AgNO3 (X5), and the concentration of AgNO3 (X6) were input to neural network software for training and testing steps, which further influenced the weight xi for every datapoint to learn the interaction between variables and plasmonic resonance (the 6 outputs) by ANN. Furthermore, a GA was employed to optimize the computational model by the evolutionary process, i.e., repeatedly selective, mutation, and crossover, which provided the selection of optimal parameters from the whole population. In the training mode, double hidden layers of 6 neurons were used in the GANN training as the best performance, and the lowest root mean square error (RMSE) (0.022) was achieved. The training and testing sets used in the ML model training consist of 2160 and 1080 datapoints, respectively. Moreover, the setting of the models contained 1 × 106 learning cycles, the lowest root mean square error (RMSE) of 0.022, a crossover rate of 0.7, and a mutation rate of 0.07.
Characterization
The morphology of six different noble metal NPs on ZnONR arrays was evaluated using a field emission scanning electron microscope (ZEISS AURIGA AFE-SEM). The optical properties were measured using a UV-vis spectrophotometer (PerkinElmer LAMBDA 950 UV/VIS/IR). Transmission electron microscopy (TEM)74,75 was conducted using a JEOL JEM-3010 Analytical Scanning Transmission Electron Microscope.76–78
Data availability
The data supporting this study are available from the corresponding authors upon reasonable request.
Author contributions
Yu-Kai Liao: conceptualization, investigation, methodology, project administration, software, validation, visualization, writing – original draft, writing – review & editing. Yi-Sheng Lai: conceptualization, formal analysis, investigation, methodology, project administration, resources, software, supervision, validation, writing – review & editing. Fei Pan: conceptualization, formal analysis, investigation, methodology, project administration, resources, software, supervision, validation, visualization, writing – original draft, writing – review & editing. Yen-Hsun Su: conceptualization, data curation, formal analysis, funding acquisition, investigation, methodology, project administration, resources, software, supervision, validation, visualization, writing – original draft, writing – review & editing.
Conflicts of interest
There are no conflicts to declare.
Acknowledgements
The authors gratefully acknowledge the support of National Cheng Kung University and the National Science and Technology Council, Taiwan, ROC for projects 109-2221-E-006-024-MY3, 111-2224-E-006-005-, 111-2622-8-006-027, 110-2224-E-006-007-, and 111-2923-E-006-008-MY3.
References
- E. W. Edwards, M. Chanana, D. Wang and H. Mohwald, Stimuli-responsive reversible transport of nanoparticles across water/oil interfaces, Angew. Chem., Int. Ed., 2008, 47(2), 320–323, DOI:10.1002/anie.200702597.
- C. W. Cheng, E. J. Sie, B. Liu, C. H. A. Huan, T. C. Sum, H. D. Sun and H. J. Fan, Surface plasmon enhanced band edge luminescence of ZnO nanorods by capping Au nanoparticles, Appl. Phys. Lett., 2010, 96(7), 071107, DOI:10.1063/1.3323091.
- Y. K. Mishra, S. Mohapatra, R. Singhal, D. K. Avasthi, D. C. Agarwal and S. B. Ogale, Au–ZnO: A tunable localized surface plasmonic nanocomposite, Appl. Phys. Lett., 2008, 92(4), 043107, DOI:10.1063/1.2838302.
- P. Fageria, S. Gangopadhyay and S. Pande, Synthesis of ZnO/Au and ZnO/Ag nanoparticles and their photocatalytic application using UV and visible light, RSC Adv., 2014, 4(48), 24962–24972, 10.1039/C4RA03158J.
- Y. Wang, X. Li, N. Wang, X. Quan and Y. Chen, Controllable synthesis of ZnO nanoflowers and their morphology-dependent photocatalytic activities, Sep. Purif. Technol., 2008, 62(3), 727–732, DOI:10.1016/j.seppur.2008.03.035.
- F. Pan, Y.-H. Su, J. Augusto, W.-S. Hwang and H.-L. Chen, Optical inclusion transformation with different amount of cerium addition during solidification of SS400 steel, Opt. Quantum Electron., 2016, 48(12) DOI:10.1007/s11082-016-0795-4.
- P.-Y. Kung, F. Pan and Y.-H. Su, Spintronic hydrogen evolution induced by surface plasmon of silver nanoparticles loaded on Fe- and Co-doped ZnO nanorods, J. Mater. Chem. A, 2021, 9(44), 24863–24873, 10.1039/D1TA04949F.
- A. Wahyuono Ruri, C. Schmidt, A. Dellith, J. Dellith, M. Schulz, M. Seyring, M. Rettenmayr, J. Plentz and B. Dietzek, ZnO nanoflowers-based photoanodes: aqueous chemical synthesis, microstructure and optical properties, Open Chem., 2016, 14, 158 Search PubMed.
- P.-Y. Kung, S.-L. Cai, F. Pan, T.-W. Shen and Y.-H. Su, Photonic fano resonance of multishaped Cu2O nanoparticles on ZnO nanowires modulating efficiency of hydrogen generation in water splitting cell, ACS Sustainable Chem. Eng., 2018, 6(5), 6590–6598, DOI:10.1021/acssuschemeng.8b00381.
- P.-Y. Kung, F. Pan and Y.-H. Su, Gold nanoparticles on TM:ZnO (TM: Fe, Co) as spinplasmon-assisted electro-optic reaction modulator in solar-to-hydrogen water splitting cell, ACS Sustainable Chem. Eng., 2020, 8(39), 14743–14751, DOI:10.1021/acssuschemeng.0c03610.
- Y.-S. Lai, M. A. J. V. G. Del Rosario, W.-F. Chen, S.-C. Yen, F. Pan, Q. Ren and Y.-H. Su, Energy-yielding mini heat thermocells with WS2 water-splitting dual system to recycle wasted heat, ACS Appl. Energy Mater., 2019, 2(10), 7092–7103, DOI:10.1021/acsaem.9b01010.
- Y.-S. Lai, F. Pan and Y.-H. Su, Firefly-like water splitting cells based on FRET phenomena with ultrahigh performance over 12%, ACS Appl. Mater. Interfaces, 2018, 10(5), 5007–5013, DOI:10.1021/acsami.7b18003.
- M.-Y. Tseng, Y.-H. Su, Y.-S. Lai, F. Pan and P.-Y. Kung, Cobalt−citrate metal−organic-framework UTSA-16 on TiO2 nanoparticles, IOP Conf. Ser.: Mater. Sci. Eng., 2020, 720, 012008, DOI:10.1088/1757-899x/720/1/012008.
- R. Yu, F. Pan, C. Schreine, X. Wang, D. M. Bell, G. Qiu and J. Wang, Quantitative determination of airborne redox-active compounds based on heating-induced reduction of gold nanoparticles, Anal. Chem., 2021, 93(44), 14859–14868, DOI:10.1021/acs.analchem.1c03823.
- F. Pan, S. Altenried, F. Zuber, R. S. Wagner, Y.-H. Su, M. Rottmar, K. Maniura-Weber and Q. Ren, Photo-activated titanium surface confers time dependent bactericidal activity towards Gram Positive and Negative bacteria, Colloids Surf., B, 2021, 206, 111940 CrossRef CAS PubMed.
- T.-S. Pan, J. Sharma, C.-C. Chu and Y. Tai, Evidences of plasmonic effect in an organic–inorganic hybrid photovoltaic device using flower-like ZnO@Au nanoparticles, J. Nanopart. Res., 2014, 16(10), 2637, DOI:10.1007/s11051-014-2637-2.
- P. Li, Z. Wei, T. Wu, Q. Peng and Y. Li, Au–ZnO hybrid nanopyramids and their photocatalytic properties, J. Am. Chem. Soc., 2011, 133(15), 5660–5663, DOI:10.1021/ja111102u.
- C. Zhang, M. Shao, F. Ning, S. Xu, Z. Li, M. Wei, D. G. Evans and X. Duan, Au nanoparticles sensitized ZnO nanorod@nanoplatelet core–shell arrays for enhanced photoelectrochemical water splitting, Nano Energy, 2015, 12, 231–239, DOI:10.1016/j.nanoen.2014.12.037.
- L. Wang, S. Wang, M. Xu, X. Hu, H. Zhang, Y. Wang and W. Huang, A Au-functionalized ZnO nanowire gas sensor for detection of benzene and toluene, Phys. Chem. Chem. Phys., 2013, 15(40), 17179–17186, 10.1039/C3CP52392F.
- X.-j. Wang, W. Wang and Y.-L. Liu, Enhanced acetone sensing performance of Au nanoparticles functionalized flower-like ZnO, Sens. Actuators, B, 2012, 168, 39–45, DOI:10.1016/j.snb.2012.01.006.
- A. Milionis, A. Tripathy, M. Donati, C. S. Sharma, F. Pan, K. Maniura-Weber, Q. Ren and D. Poulikakos, Water-based scalable methods for self-cleaning antibacterial ZnO-nanostructured surfaces, Ind. Eng. Chem. Res., 2020, 59(32), 14323–14333, DOI:10.1021/acs.iecr.0c01998.
- I. Gonzalez-Valls and M. Lira-Cantu, Vertically-aligned nanostructures of ZnO for excitonic solar cells: a review, Energy Environ. Sci., 2009, 2(1), 19–34, 10.1039/b811536b.
- X. H. Lu, G. M. Wang, S. L. Xie, J. Y. Shi, W. Li, Y. X. Tong and Y. Li, Efficient photocatalytic hydrogen evolution over hydrogenated ZnO nanorod arrays, Chem. Commun., 2012, 48(62), 7717–7719, 10.1039/c2cc31773g.
- S. Hoang and P. X. Gao, Nanowire array structures for photocatalytic energy conversion and utilization: a review of design concepts, assembly and integration, and function enabling, Adv. Energy Mater., 2016, 6(23) DOI:10.1002/aenm.201600683.
- Z. K. Tang, G. K. L. Wong, P. Yu, M. Kawasaki, A. Ohtomo, H. Koinuma and Y. Segawa, Room-temperature ultraviolet laser emission from self-assembled ZnO microcrystallite thin films, Appl. Phys. Lett., 1998, 72(25), 3270–3272, DOI:10.1063/1.121620.
- F. L. Su, T. Wang, R. Lv, J. J. Zhang, P. Zhang, J. W. Lu and J. L. Gong, Dendritic Au/TiO2 nanorod arrays for visible-light driven photoelectrochemical water splitting, Nanoscale, 2013, 5(19), 9001–9009, 10.1039/c3nr02766j.
- R. B. Wei, P. Y. Kuang, H. Cheng, Y. B. Chen, J. Y. Long, M. Y. Zhang and Z. Q. Liu, Plasmon-enhanced photoelectrochemical water splitting on gold nanoparticle decorated ZnO/CdS nanotube arrays, ACS Sustainable Chem. Eng., 2017, 5(5), 4249–4257, DOI:10.1021/acssuschemeng.7b00242.
- X. J. Wang, W. Wang and Y. L. Liu, Enhanced acetone sensing performance of Au nanoparticles functionalized flower-like ZnO, Sens. Actuators, B, 2012, 168, 39–45, DOI:10.1016/j.snb.2012.01.006.
- Y. Su, H. Guo, Z. S. Wang, Y. M. Long, W. F. Li and Y. F. Tu, Au@Cu2O core-shell structure for high sensitive non-enzymatic glucose sensor, Sens. Actuators, B, 2018, 255, 2510–2519, DOI:10.1016/j.snb.2017.09.056.
- L. S. Huang, D. Y. Bao, X. Q. Jiang, J. H. Li, L. X. Zhang and X. S. Sun, Fabrication of stable high-performance urchin-like CeO2/ZnO@Au hierarchical heterojunction photocatalyst for water remediation, J. Colloid Interface Sci., 2021, 588, 713–724, DOI:10.1016/j.jcis.2020.11.099.
- Y. Ding, Y. X. Liu, J. Parisi, L. C. Zhang and Y. Lei, A novel NiO-Au hybrid nanobelts based sensor for sensitive and selective glucose detection, Biosens. Bioelectron., 2011, 28(1), 393–398, DOI:10.1016/j.bios.2011.07.054.
- W. W. Zhang, W. Z. Wang, H. L. Shi, Y. J. Liang, J. L. Fu and M. Zhu, Surface plasmon-driven photoelectrochemical water splitting of aligned ZnO nanorod arrays decorated with loading-controllable Au nanoparticles, Sol. Energy Mater. Sol. Cells, 2018, 180, 25–33, DOI:10.1016/j.solmat.2018.02.020.
- Z. J. Lin, X. H. Wang, J. Liu, Z. Y. Tian, L. C. Dai, B. B. He, C. Han, Y. G. Wu, Z. G. Zeng and Z. Y. Hu, On the role of localized surface plasmon resonance in UV-vis light irradiated Au/TiO2 photocatalysis systems: pros and cons, Nanoscale, 2015, 7(9), 4114–4123, 10.1039/c4nr06929c.
- W. Li, D. D. Huang, T. T. Wang, C. Zheng, X. Q. Xiao, S. G. Cai and W. Z. Chen, Au nanoparticle decorated WO3 nanorods with enhanced optical limiting activity, Opt. Mater. Express, 2020, 10(10), 2655–2668, DOI:10.1364/OME.403617.
- S. M. Majhi, P. Rai and Y.-T. Yu, Facile approach to synthesize Au@ZnO core–shell nanoparticles and their application for highly sensitive and selective gas sensors, ACS Appl. Mater. Interfaces, 2015, 7(18), 9462–9468, DOI:10.1021/acsami.5b00055.
- Y. S. Xie, N. Zhang, Z. R. Tang, M. Anpo and Y. J. Xu, Tip-grafted Ag-ZnO nanorod arrays decorated with Au clusters for enhanced photocatalysis, Catal. Today, 2020, 340, 121–127, DOI:10.1016/j.cattod.2018.09.010.
- H. X. Huang, J. C. Li and C. L. Xiao, A proposed iteration optimization approach integrating backpropagation neural network with genetic algorithm, Expert Syst. Appl., 2015, 42(1), 146–155, DOI:10.1016/j.eswa.2014.07.039.
- D. Venkatesan, K. Kannan and R. Saravanan, A genetic algorithm-based artificial neural network model for the optimization of machining processes, Neural Comput. Appl., 2009, 18(2), 135–140, DOI:10.1007/s00521-007-0166-y.
- Y.-S. Lai and Y.-H. Su, Over 9% water splitting nature dyes solar cells via artificial intelligent selected combination, ACS Agric. Sci. Technol., 2022, 2(3), 615–624, DOI:10.1021/acsagscitech.2c00043.
- F. Pan, C.-C. Wu, Y.-L. Chen, P.-Y. Kung and Y.-H. Su, Machine learning ensures rapid and precise selection of gold sea-urchin-like nanoparticles for desired light-to-plasmon resonance, Nanoscale, 2022, 14, 13532–13541, 10.1039/D2NR03727K.
- V. Khezri, E. Yasari, M. Panahi and A. Khosravi, Hybrid artificial neural network-genetic algorithm-based technique to optimize a steady-state gas-to-liquids plant, Ind. Eng. Chem. Res., 2020, 59(18), 8674–8687, DOI:10.1021/acs.iecr.9b06477.
- C.-C. Wu, F. Pan and Y.-H. Su, Surface plasmon resonance of gold nano-sea-urchins controlled by machine-learning-based regulation in seed-mediated growth, Adv. Photonics Res., 2021, 2(9), 2170031, DOI:10.1002/adpr.202170031.
- C. Poloni, A. Giurgevich, L. Onesti and V. Pediroda, Hybridization of a multi-objective genetic algorithm, a neural network and a classical optimizer for a complex design problem in fluid dynamics, Comput. Methods Appl. Mech. Eng., 2000, 186(2), 403–420, DOI:10.1016/S0045-7825(99)00394-1.
- C. Y. Shen, L. X. Wang and Q. Li, Optimization of injection molding process parameters using combination of artificial neural network and genetic algorithm method, J. Mater. Process. Technol., 2007, 183(2–3), 412–418, DOI:10.1016/j.jmatprotec.2006.10.036.
- J. Morshed and J. J. Kaluarachchi, Application of artificial neural network and genetic algorithm in flow and transport simulations, Adv. Water Resour., 1998, 22(2), 145–158, DOI:10.1016/S0309-1708(98)00002-5.
- K. Gupta, M. Parasnis, R. Jain and P. Dandekar, Vector-related stratagems for enhanced monoclonal antibody production in mammalian cells, Biotechnol. Adv., 2019, 37(8) DOI:10.1016/j.biotechadv.2019.107415.
- T. Matsuda, T. Tanijima, A. Hirose, K. Masumi-Koizumi, T. Katsuda and H. Yamaji, Production of influenza virus-like particles using recombinant insect cells, Biochem. Eng. J., 2020, 163, 107757, DOI:10.1016/j.bej.2020.107757.
- H. Waegeman and W. Soetaert, Increasing recombinant protein production in Escherichia coli through metabolic and genetic engineering, J. Ind. Microbiol. Biotechnol., 2011, 38(12), 1891–1910, DOI:10.1007/s10295-011-1034-4.
-
D. Mattanovich, P. Branduardi, L. Dato, B. Gasser, M. Sauer, and D. Porro, Recombinant Protein Production in Yeasts, in Recombinant Gene Expression: Reviews and Protocols, ed. A. Lorence, 3rd edn, 2012, vol. 824, pp. 329–358 Search PubMed.
- D. Hegemann, B. Hanselmann, F. Zuber, F. Pan, S. Gaiser, P. Rupper, K. Maniura-Weber, K. Ruffieux and Q. Ren, Plasma-deposited AgOx-doped TiOx coatings enable rapid antibacterial activity based on ROS generation, Plasma Processes Polym., 2022, 19(7), e2100246, DOI:10.1002/ppap.202100246.
- F. Pan, S. Altenried, M. Liu, D. Hegemann, E. Bülbül, J. Moeller, W. W. Schmahl, K. Maniura-Weber and Q. Ren, A nanolayer coating on polydimethylsiloxane surfaces enables a mechanistic study of bacterial adhesion influenced by material surface physicochemistry, Mater. Horiz., 2020, 7(1), 93–103 RSC.
- F. Pan, A. Amarjargal, S. Altenried, M. Liu, F. Zuber, Z. Zeng, R. M. Rossi, K. Maniura-Weber and Q. Ren, Bioresponsive hybrid nanofibers enable controlled drug delivery through glass transition switching at physiological temperature, ACS Appl. Bio Mater., 2021, 4(5), 4271–4279 CrossRef CAS PubMed.
- F. Pan, G. Giovannini, S. Zhang, S. Altenried, F. Zuber, Q. Chen, L. F. Boesel and Q. Ren, pH-responsive silica nanoparticles for triggered treatment of skin wound infections, Acta Biomater., 2022, 145, 172–184, DOI:10.1016/j.actbio.2022.04.009.
- F. Pan, M. Liu, S. Altenried, M. Lei, J. Yang, H. Straub, W. W. Schmahl, K. Maniura-Weber, O. Guillaume-Gentil and Q. Ren, Uncoupling bacterial attachment on and detachment from polydimethylsiloxane surfaces through empirical and simulation studies, J. Colloid Interface Sci., 2022, 622, 419–430 CrossRef CAS PubMed.
-
F. Pan, Fighting Antimicrobial Resistant (AMR) Bacteria: from Bacteriophage-Based Specific Capture to Controlled Killing, ETH Zurich, 2022 Search PubMed.
- F. Guo, F. Pan, W. Zhang, T. Liu, F. Zuber, X. Zhang, Y. Yu, R. Zhang, M. Niederberger and Q. Ren, Robust antibacterial activity of xanthan-gum-stabilized and patterned CeO2–x–TiO2 antifog films, ACS Appl. Mater. Interfaces, 2022, 14(39), 44158–44172, DOI:10.1021/acsami.2c11968.
- F. Pan, S. Zhang, S. Altenried, F. Zuber, Q. Chen and Q. Ren, Advanced antifouling and antibacterial hydrogels enabled by the controlled thermo-responses of a biocompatible polymer composite, Biomater. Sci., 2022, 10, 6146–6159, 10.1039/D2BM01244H.
- S. M. G. Hayat, N. Farahani, B. Golichenari and A. Sahebkar, Recombinant protein expression in Escherichia coli (E. coli): what we need to know, Curr. Pharm. Des., 2018, 24(6), 718–725, DOI:10.2174/1381612824666180131121940.
- F. J. M. Mergulhao, D. K. Summers and G. A. Monteiro, Recombinant protein secretion in Escherichia coli, Biotechnol. Adv., 2005, 23(3), 177–202, DOI:10.1016/j.biotechadv.2004.11.003.
- H. P. Sorensen and K. K. Mortensen, Advanced genetic strategies for recombinant protein expression in Escherichia coli, J. Biotechnol., 2005, 115(2), 113–128, DOI:10.1016/j.jbiotec.2004.08.004.
- N. M. Mahmoud, M. H. Mahmoud, S. Alamery and H. Fouad, Structural modeling and phylogenetic analysis for infectious disease transmission pattern based on maximum likelihood tree approach, J. Ambient Intell. Hum. Comput., 2021, 12(3), 3479–3492, DOI:10.1007/s12652-020-02702-8.
- T. T. Li, D. X. Liu, Y. D. Yang, J. L. Guo, Y. J. Feng, X. M. Zhang, S. L. Cheng and J. Feng, Phylogenetic supertree reveals detailed evolution of SARS-CoV-2, Sci. Rep., 2020, 10(1) DOI:10.1038/s41598-020-79484-8.
- W.-T. Jin, D. S. Gernandt, C. Wehenkel, X.-M. Xia, X.-X. Wei and X.-Q. Wang, Phylogenomic and ecological analyses reveal the spatiotemporal evolution of global pines, Proc. Natl. Acad. Sci. U. S. A., 2021, 118(20), e2022302118, DOI:10.1073/pnas.2022302118.
- B. S. Godoy, L. M. Camargos and S. Lodi, When phylogeny and ecology meet: modeling the occurrence of Trichoptera with environmental and phylogenetic data, Ecol. Evol., 2018, 8(11), 5313–5322, DOI:10.1002/ece3.4031.
- T. J. Davies, S. A. Fritz, R. Grenyer, C. D. L. Orme, J. Bielby, O. R. P. Bininda-Emonds, M. Cardillo, K. E. Jones, J. L. Gittleman and G. M. Mace,
et al., Phylogenetic trees and the future of mammalian biodiversity, Proc. Natl. Acad. Sci. U. S. A., 2008, 105, 11556–11563, DOI:10.1073/pnas.0801917105.
- X. M. Zhang, Y. L. Chen, R. S. Liu and D. P. Tsai, Plasmonic photocatalysis, Rep. Prog. Phys., 2013, 76(4) DOI:10.1088/0034-4885/76/4/046401.
- K. M. Mayer and J. H. Hafner, Localized surface plasmon resonance sensors, Chem. Rev., 2011, 111(6), 3828–3857, DOI:10.1021/cr100313v.
- E. Petryayeva and U. J. Krull, Localized surface plasmon resonance: nanostructures, bioassays and biosensing-a review, Anal. Chim. Acta, 2011, 706(1), 8–24, DOI:10.1016/j.aca.2011.08.020.
- J. Wei, C. Zhu, Z. Zeng, F. Pan, F. Wan, L. Lei, G. Nyström and Z. Fu, Bioinspired cellulose-integrated MXene-based hydrogels for multifunctional sensing and electromagnetic interference shielding, Interdiscip. Mater., 2022, 1(4), 495–506 CrossRef.
- H. Yuan, W. Ma, C. Chen, J. Zhao, J. Liu, H. Zhu and X. Gao, Shape and SPR evolution of thorny gold nanoparticles promoted by silver ions, Chem. Mater., 2007, 19(7), 1592–1600, DOI:10.1021/cm062046i.
- P. K. Jain, W. Y. Huang and M. A. El-Sayed, On the universal scaling behavior of the distance decay of plasmon coupling in metal nanoparticle pairs: a plasmon ruler equation, Nano Lett., 2007, 7(7), 2080–2088, DOI:10.1021/nl071008a.
- S. K. Ghosh and T. Pal, Interparticle coupling effect on the surface plasmon resonance of gold nanoparticles: from theory to applications, Chem. Rev., 2007, 107(11), 4797–4862, DOI:10.1021/cr0680282.
- C.-C. Wu, F. Pan and Y.-H. Su, Surface plasmon resonance of gold nano-sea-urchins controlled by machine-learning-based regulation in seed-mediated growth, Adv. Photonics Res., 2021, 2(9), 2100052, DOI:10.1002/adpr.202100052.
- F. Pan, S. Altenried, S. Scheibler, A. H. C. Anthis and Q. Ren, Specific capture of Pseudomonas aeruginosa for rapid detection of antimicrobial resistance in urinary tract infections, Biosens. Bioelectron., 2023, 222, 114962 CrossRef CAS PubMed.
- F. Pan, S. Altenried, S. Scheibler, I. Rodriguez Fernandez, G. Giovannini and Q. Ren, Ultrafast determination of antimicrobial resistant Staphylococcus aureus specifically captured by functionalized magnetic nanoclusters, ACS Sens., 2022, 7(11), 3491–3500 CrossRef CAS PubMed.
- J. Wei, R. Wang, F. Pan and Z. Fu, Polyvinyl alcohol/graphene oxide conductive hydrogels via the synergy of freezing and salting out for strain sensors, Sensors, 2022, 22(8), 3015 CrossRef CAS PubMed.
- Y. Yang, N. Wu, B. Li, W. Liu, F. Pan, Z. Zeng and J. Liu, Biomimetic porous MXene sediment-based hydrogel for high-performance and multifunctional electromagnetic interference shielding, ACS Nano, 2022, 16(9), 15042–15052, DOI:10.1021/acsnano.2c06164.
- F. Pan, H.-L. Chen, Y.-H. Su, Y.-H. Su and W.-S. Hwang, Inclusions properties at 1673 K and room temperature with Ce addition in SS400 steel, Sci. Rep., 2017, 7, 2564, DOI:10.1038/s41598-017-02478-6.
|
This journal is © The Royal Society of Chemistry 2023 |
Click here to see how this site uses Cookies. View our privacy policy here.