Prediction of sustainable aviation fuel properties for liquid hydrocarbons from hydrotreating biomass catalytic fast pyrolysis derived organic intermediates†
Received
13th January 2023
, Accepted 11th April 2023
First published on 11th April 2023
Abstract
Catalytic fast pyrolysis (CFP) of wood, woody residues, and agricultural waste has the potential to produce organic liquid intermediates that can be hydroprocessed to sustainable aviation fuels (SAF). In this article we present results of property predictions for two SAF fuels produced from hydroprocessing of CFP-based oxygenated organic intermediates followed by distillation to obtain the jet boiling range cuts. The two intermediates, in turn, were produced using ZSM-5 and Pt/TiO2 catalysts. The objective is to assess the applicability of recently developed prediction methods and other well-established methods to CFP-derived SAF fuels, focusing on five properties that are important for jet fuel certification, namely density, flash point, net heat of combustion, freezing point, and distillation temperatures (10% distilled and final boiling point). For flash point and net heat of combustion, several of the methods tested gave comparable results with mean absolute errors (MAE) that are within the reproducibility limit of the data. The API and ASTM D7215 methods provided the best results for flash point, while the ASTM D3338 correlation gave the lowest MAE for net heat of combustion. For density at 15 °C, the MWA method of Shi et al. gave the lowest MAE of 0.0033 g cm−3, however, it is still larger than the reproducibility limit of 0.0005 g cm−3. The accuracy of the prediction methods for freezing point could not be assessed since the experimental data of the two SAF fuels were given as <−70 °C. The distillation temperatures were not well predicted by the method tested.
Introduction
Ambitious goals are being set for ramping up sustainable aviation fuels (SAF) to help reduce greenhouse gas (GHG) emissions from aviation. Those include the US SAF Grand Challenge to increase supplies to 3 and 35 billion gallons per year by 2030 and 2050, respectively1 and the European objective of deriving 63% of fuels for air transport from sustainable sources by 2050.2 Significant biomass resources exist in the US,3 and with the development of those feedstock supplies and synergistic conversion processes, sufficient feedstocks will be available to meet the 2050 SAF volumetric goals.4 The most efficient conversion and use of feedstocks towards meeting SAF specifications and GHG goals is an important consideration. Various conversion technologies, including gasification and the conversion of bio-derived ethanol to jet are promising near-term options.4 Pyrolysis based processes promise higher yields but have inherent challenges towards meeting the stringent requirements for aviation fuels, stemming primarily from the high oxygen content, corrosiveness, and chemical instability of the bio-oil intermediate. Thousands of different compounds are released during pyrolytic breakdown of biomass5 and funneling them towards specific molecules is difficult. In addition, variations in feedstock compositions and process conditions also manifest themselves as variations in the pyrolysis products. Towards the goal of getting a more stable and consistent slate of products, catalysts are used to deoxygenate and stabilize pyrolysis vapors in a process known as catalytic fast pyrolysis (CFP).6,7 Further hydrotreatment of condensed CFP oils is necessary to produce hydrocarbons for SAF, and this step provides a critical boost towards compositional compatibility and predictability of fuel quality. Enabling SAF from CFP will require careful study and understanding of product properties relevant for jet fuel certification. CFP oils contain aromatic structures (hydrocarbons, phenolics and higher hydroxyaromatics) that could be converted to cycloparaffins, which have been identified as desirable SAF components.4 With suitable hydrotreating conditions, including temperature, it is possible to produce SAF with high fractions of cycloparaffins.8 The composition of the product differs from most other bio-based SAF fuels; high concentrations of cyclohexanes, octahydroindenes, and decahydronaphthalenes are present in this product. Towards the goal of advancing the future adoption of CFP-derived SAF, the aim of this work is to employ model-based methods from literature to evaluate and connect recently available experimental information8 with composition-based prediction methods. These property predictions and related understanding from experimental speciation can help accelerate the understanding and adoption of SAF from CFP and other conversion processes and help inform process and catalyst designs; prediction of key product properties using known compositional measurement techniques that require small sample quantities can facilitate those efforts. In this work we focus on evaluation of properties with available experimental measurements (density, flash point, net heating value, freezing point, distillation temperatures for 10% distilled, and final boiling point) and plan to discuss additional properties in future publications.
Catalytic fast pyrolysis for fuels production
A simple block flow diagram of the CFP process,6,7 including the hydroprocessing and product recovery steps is shown in Fig. 1. Biomass contains significant amounts of oxygen (∼40 wt% in dry wood), carbon, and hydrogen, as well as nitrogen and sulfur. Other components, including inorganic mineral matter in varying proportions, dictated by the biomass source and type, are also present. Efficient removal of all other atomic constituents while preserving carbon and hydrogen for making hydrocarbon molecules in the correct jet-fuel boiling range is the key for high yields of SAF. After thermal breakdown of biomass into solid char, permanent gases, and oxygenated vapors during fast pyrolysis, subsequent deoxygenation during catalytic upgrading of pyrolysis vapors is important for producing and retrieving (after condensation) a stabilized organic intermediate or CFP oil. This CFP oil can then be further hydroprocessed to hydrocarbon fuels and blendstocks via removal of the remaining oxygen, sulfur, and nitrogen. Note that nitrogen and sulfur are usually minor species (<0.2 wt%) in woody biomass, and efficient deoxygenation is the primary chemical challenge for CFP processes using woody feedstocks.
 |
| Fig. 1 Simplified block flow diagram of catalytic fast pyrolysis (CFP) process including hydroprocessing and product recovery steps. | |
CFP processes can provide high hydrocarbon yields with effective chemistry to preserve a large fraction of the carbon in biomass in larger molecules and not forming CO, CO2, and other light gases during catalytic upgrading; many processes propose the use of hydrogen for removing most of the oxygen via hydrodeoxygenation, thus reducing coke, CO, and CO2 formation.9 In addition to process challenges, especially with the design and maintenance of efficient catalysts with high target yields, making aviation-compatible molecules and the removal of contaminants are other challenges that need to be addressed to realize the full potential of this technology towards SAF production.
For the two CFP-derived fuel sample analyses used in this article, carbon efficiencies from biomass to hydrotreated products in the SAF boiling range were calculated to be 11% and 13% using ZSM-5 and Pt/TiO2 CFP catalysts, respectively. These overall carbon efficiencies are similar to those that can be estimated from other published work, e.g., 7–12% in the SAF range based on 25–42% overall carbon efficiencies and 28% of the distillation cuts in the jet boiling range.10 Carbon efficiencies are known to vary with oxygen contents of CFP oils; data points follow a pattern of reduced carbon efficiencies with increased deoxygenation during CFP.11 There are no standardized CFP oils and research efforts are currently focused on finding optimal catalysts and conditions for increasing the yields of specific fuels and products; our current work falls within the continuum of yields and oxygen contents,11 trying to address gaps and questions regarding the production of SAF via CFP.
Properties of interest for SAF
Aviation fuels and their properties have been studied and documented.12 Since SAF candidate products need to make near-term impact without disrupting the existing infrastructure or requiring any modifications to aircraft engines, SAF properties are held to similar standards as regular jet fuel. In cases where SAF from specific processes cannot meet the full set of aviation fuel properties, e.g., farnesane (2,6,10-trimethyldodecane, a C15 molecule derived from sugar fermentation), specific blend limits (10% for farnesane) are set to allow use within the current aviation fuel infrastructure.4 As a guide to the reader, some key properties, and their specifications of two commonly used jet fuels are reproduced in Table 1. The significance of these properties during aviation fuel use is discussed in the literature.4 Note that we will be focusing on a subset of the listed properties (density, flash point, net heating value, freezing point, distillation temperatures for 10% distilled, and final boiling point).
Table 1 Specifications for select jet fuel properties12
Property |
Jet Aa, 2018 |
JP-8b, 2018 |
Jet fuel commonly used in commercial aviation.
Jet fuel commonly used by the US military.
1 cSt = 1 mm2 s−1.
IBP, initial boiling point, FBP, final boiling point.
|
Density at 15 °C (g cm−3) |
0.775 to 0.84 |
0.775 to 0.84 |
Flash point (°C) |
38 min |
38 min |
Viscosity (cSt)c |
8 at −20 °C, max |
8 at −20 °C, max |
Freezing point (°C) |
−40 max (−47 for jet A-1) |
−47 max |
Aromatics (vol%) |
25, max |
25, max |
Heat of combustion, (MJ kg−1) |
42.8, min |
42.8, min |
Sulfur content (mass%) |
0.3 |
0.3 |
Mercaptan sulfur (mass%) |
0.003 |
0.002 |
Acid number |
0.1 |
0.015 |
Distillation (°C) |
IBPd |
10% |
205 |
205 |
20% |
50% |
90% |
FBPd |
300 |
300 |
Smoke point (mm) |
18 min |
18 min |
Importance of understanding the impact of chemical composition on properties
The importance and benefits of predicting properties of aviation fuels from chemical compositions was discussed by Vozka and Kilaz.13 Since chemical compositions can be measured using known methods and relatively small quantities of fuel, the ability to predict fuel properties from chemical compositions can enable rapid feedback during the development of conversion processes for alternative fuels production. In this context CFP processes that can vary significantly because of the numerous choices of feedstocks, process configurations, catalysts, and operating conditions can benefit from the identification of appropriate prediction methods and any necessary related developments to help accelerate the potential adoption of SAF from CFP through better understanding of fuel property variations due to chemical compositional changes of the fuel products. Although the eventual long-term testing is necessary for any SAF pathway certification,4 available experimental data corroborating property prediction methods can help build confidence that we have a good understanding of what it will take for the CFP family of processes to be compatible with SAF production.
Property predictions in literature
Yang et al.14 reviewed physicochemical properties of bio-jet fuels considering their chemical compositions. They suggested the inclusion of chemical compositions, including the classes of hydrocarbons and carbon chain lengths because they significantly influence fuel performance.
Vozka and Kilaz13 provided an overview of chemical composition–property correlation techniques from 1955 to 2019 covering four categories of analytical measurement techniques: nuclear magnetic resonance (NMR) spectroscopy, infrared (IR) spectroscopy, Raman spectroscopy, and gas chromatography (GC, one and two-dimensional). These techniques and their predictive capabilities were compared using measures of the uncertainty for future predictions. Many characteristics were considered, including smoke point, hydrogen content, net heat of combustion, freezing point, density at 15 °C, flash point, viscosities at −20 °C and −40 °C, calculated cetane index, and acid number. It was observed that chemical compositions from two-dimensional gas chromatography (GC × GC) measurements yielded the best correlations for most property predictions in comparison with other measurement techniques. For smoke point, the most successful correlation was based on NMR results, although it was noted that GC × GC method was not tested. The viscosity prediction was most successful using GC, implying that GC × GC would work as well. For density, the GC × GC method of Shi et al.15 gave a coefficient of determination (R2) of 0.9999, while that of Vozka et al.16 yielded an R2 of 0.9967 for their respective sets of aviation fuels evaluated; it was noted that the Shi et al. method predicted density at 20 °C instead of 15 °C. For freezing point, the Shi et al. method gave an R2 of 0.9866; only the GC × GC evaluation was reported.13 For net heat of combustion (NHC), Shi et al. was also the only GC × GC based evaluation reported, with an R2 of 0.9993. The Shi et al. GC × GC based method predicted flash point with an R2 of 0.9968. Vozka and Kilaz13 also reviewed correlations based on fuel properties. These correlations are predominantly ASTM methods, which are based either on the other fuel properties or on chemical composition. For example, in the ASTM D7215 (ref. 17) method, flash point is calculated as a function of simulated distillation data, which, in turn, is determined from chemical composition obtained from GC or GC × GC. The ASTM D3338 (ref. 18) method for estimating net heat of combustion of aviation fuels uses density and average distillation data as inputs.
Wang et al.19 published a comprehensive review of the effects of fuel composition and hydrocarbon molecular structure on the fuel physicochemical properties, including density, NHC, low-temperature fluidity (viscosity and freezing point), flash point, and thermal-oxidative stability. They reviewed many correlations and developed correlations for estimating density, NHC, and viscosity using hydrogen/carbon ratios and molecular weights.
Flora et al.20 performed a computational study of optimum blending ratios for conventional and alternative fuels that may be certifiable. The work described blending rules for calculating properties of binary fuel blends from different fractions of the two parent fuels. The properties included density, viscosity, flash point, aromatic concentration, NHC, and derived cetane number (DCN).
Since the GC × GC composition-based prediction methods of Shi et al.,15 as reviewed by Vozka and Kilaz13 provided good prediction results for many specification properties of interest for SAF, they are further described here. Shi et al.15 developed four statistical algorithms for the prediction of density at 20 °C, freezing point, flash point, and NHC of aviation fuels using detailed hydrocarbon composition obtained from the GC × GC-MS/FID measurements of 17 petroleum-based and synthetic aviation fuels. The four methods include the weighted average (WA) method, partial least squares analysis (PLS), genetic algorithm (GA), and modified weighted average (MWA) method. The correlations were based on the total content (wt%) of ten hydrocarbon classes for each carbon number, which could range from C7 to C19. A short description of all four Shi et al. methods can be found in the ESI.†
Further discussion of property predictions in this work
Predictive correlations that make use of chemical composition are of primary interest in this work since detailed composition determined using GC × GC with a mass spectrometer and a flame ionization detector (GC × GC-MS/FID) are available for two potential SAF-compatible fuels. The following sub-sections discuss prediction of the properties including liquid density, flash point, NHC, freezing point, distillation temperatures for 10% distilled, and FBP. They are the focus of this article because of the availability of experimental data.
Liquid density
According to Vozka et al.,16 the density of SAF is an important property and a key indicator of fuel quality. Density also determines the fuel load for a certain volume of fuel and hence the range of the aircraft. It is necessary for key operational requirements such as flow calculations, fuel gauging, metering device adjustments, and fuel thermal expansion calculations.21 Chemical composition impacts density;15 the latter increases with carbon number within a class of hydrocarbons. For a certain carbon number, the density decreases in the order aromatics > cycloparaffins > alkanes. Carbon number and hydrocarbon class are thus important indicators of density.
As discussed in the preceding section, Shi et al.15 developed four statistical algorithms to estimate density at 20 °C using data for 17 petroleum-based and synthetic aviation fuels. They reported that the PLS method gave the best results with an R2 of 0.9999 and a mean absolute percentage error (MAPE) of 0.06%.15
As pointed out by Vozka et al.,13 the limitation of Shi's work was the small number of the fuels tested. In addition, the density correlations were for the temperature of 20 °C instead of 15 °C, which is the temperature required by aviation fuel standards.
To overcome the temperature problem of Shi's density correlation, Vozka et al.16 developed correlations for density at 15 °C predicted from chemical composition. Composition data obtained from GC × GC-time-of-flight (TOF)-MS and GC × GC-FID were used to construct a composition matrix with seven hydrocarbon classes;16,22 each of those classes included carbon numbers ranging from C7 to C20. They used 50 samples that included both alternate fuel blending components and petroleum-derived fuels. In addition, they used the density of a single compound to represent each carbon number for their calculations instead of the average density used in the method of Shi et al.15 In their work, they used the PLS method and the regularized support vector machines (SVM) method, which uses high dimensional spaces. For the PLS and SVM methods, two alternative correlations were developed, one using the composition matrix and another one using the product matrix. Therefore, a total of four correlations were presented: PLS composition, PLS product, SVM composition, and SVM product. The SVM composition method gave an R2 of 0.9967 and the lowest MAPE of 0.1%. A short description of the Vozka et al. density method can be found in the ESI.†
Liquid density ρL of mixtures can be approximated by using specific volume νL with a linear mixing rule; excess volume contribution is neglected.23
| 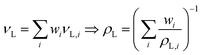 | (1) |
where
wi is mass fraction of component
i. According to Gmehling,
23 this simplified density calculation typically leads to small errors. Reiter
et al.24 used the equation towards the generation and evaluation of surrogate diesel fuels. Dahmen and Marquardt
25 used it in their work on formulation of biofuel blends.
Flash point
The flash point is the lowest temperature at which the vapors above a flammable liquid will ignite upon the application of an ignition source, i.e., spark or flame.26 At the flash point temperature, just enough liquid has vaporized to bring the vapor-air space over the liquid above the lower flammability limit.27 Therefore, it is an important property for hydrocarbon fuels used in aviation and is used to determine their volatilization and flammability characteristics.15
The minimum flash point is generally 38 °C for kerosene-type fuels, with higher minimums allowed with specific agreements with the purchaser.
The low-end boiling point temperatures of the distillation curve dictate the flash point.12 In the API technical databook,28 flash point (TF) is correlated with the ASTM D86 (ref. 29) 10% temperature (t10) for petroleum fractions:
|  | (2) |
A simpler correlation was later proposed:26
| TF (°C) = −64.542 + 0.70704t10 (°C). | (3) |
The ASTM D7215 method17 predicts flash point equivalent to the test methods ASTM D56 (ref. 30) and D93 (ref. 31) from simulated distillation data obtained using ASTM D2887.32 The equation for the D56 test method was developed using over one hundred samples of petroleum-derived diesel and jet fuel using PLS regression and is given as:
| TF (°C) = −55.5 + 0.164TIBP (°C) + 0.095T5 (°C) + 0.453T10 (°C) | (4) |
where
TIBP is the initial boiling point temperature, and
T5 and
T10 are the temperatures at which 5 and 10 wt% of the sample are recovered, respectively.
TIBP,
T5 and
T10 are data from the simulated distillation measurement. Eqn
(4) was used by Vozka
et al.33 to predict flash point of blends of hydroprocessed esters and fatty acids (HEFA) feedstocks sourced from camelina (CAME), tallow (TALL), and mixed fat (MFAT) with Jet A. Since the ASTM D7215 equation was not developed using mixtures of Jet A/A-1 with alternative fuel blending components such as HEFA, a new correlation was developed to improve the results using flash point data of the blends.
33 The revised parameters for the ASTM D56 equation are:
| TF (°C) = −39.244 + 0.246TIBP (°C) − 0.058T5 (°C) + 0.428T10 (°C) | (5) |
Additional alternative blending mixes (Fischer–Tropsch hydroprocessed synthesized paraffinic kerosene (FT-SPK), HEFA, synthesized iso-paraffins from hydroprocessed fermented sugars (SIP), and alcohol-to-jet synthetic paraffinic kerosene (ATJ)) with Jet A were later used to further validate the equation.34
Flash point can also be predicted or calculated from detailed chemical composition.15,33,35–37 In the work of Shi et al.,15 flash point was correlated using the same four methods described in the density section above and the ESI.† Of the 17 aviation fuels tested, the MWA method was reported to provide the best results with an R2 of 0.9968 and MAPE of 1.24%.15
Net heat of combustion (NHC)
NHC is a combustion performance specification for aviation fuels representing the energy contained in the fuel. It is the released energy obtained from the complete combustion of fuel, with products being in the gaseous state.26 NHC can be reported on gravimetric or volumetric basis, and it determines the distance and payload of a flight.19 Civil aircrafts, usually constrained by payload weight, use fuels with high gravimetric NHC. Flight vehicles such as rockets and missiles are limited by the small design sizes of their fuel tanks to leave sufficient space for avionics; in such cases a higher volumetric NHC is desirable.38,39
For aviation and SAF applications, gravimetric NHC is of interest. According to ASTM D3338,18 NHC of jet fuels should range from 40.19 to 44.73 MJ kg−1. For convenience and brevity, the remainder of the paper shall refer to gravimetric NHC simply as NHC.
NHC can be calculated from chemical composition, such as n-paraffins, iso-paraffins, cycloparaffins, aromatic content, or the other properties, such as density and distillation temperatures. A list of these methods was given by Wang et al.19 Since NHC of aviation fuels are strongly influenced by the hydrogen content and the total number of carbon atoms, they correlated NHC of 64 fuels to hydrogen-to-carbon ratio (H/C) and molar mass (M).
The ASTM D3338 method utilizes distillation data, aromatic content (vol%), and density. It is the officially permitted calculation method and is listed in many jet fuel specifications worldwide:33
|  | (6) |
where NHC is net heat of combustion (MJ kg
−1), sulfur-free basis,
A is aromatics vol%,
ρ15 is density (kg m
−3) at 15 °C, and
T (°C) is volatility. The latter is the average of 10%, 50%, and 90% points determined according to the D86 method.
29 Eqn
(6) was derived from 241 fuels, most of which conforming with aviation gasoline and jet fuel specifications; stepwise linear regression methods were used for the derivation.
Vozka et al.33,34 applied the D3338 method to alternative aviation fuels and reported that it was well suited for HEFA/Jet A mixture and other alternate blending component mixtures. Results were compared with data using ASTM D4809;40 the differences were below the reproducibility and the repeatability of the experimental values.
NHC can also be calculated from the detailed chemical composition15,37 of a fuel, such as composition obtained from GC × GC data. In the simplest form, NHC is related to composition via the following equation.
| 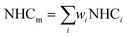 | (7) |
where
wi is mass fraction of component
i, NHC
m is the NHC of the mixture and NHC
i is NHC of the constituent component
i. Vozka
et al.33 used this equation to predict NHC of blends of HEFA-CAME, -TALL, and -MFAT with Jet A using NHC values of Jet A and the neat HEFA blend components. Flora
et al.20 used it for blends of Jet A/FT-SPK (Syntroleum GTL), Jet A/HEFA-SPK (Sasol Iso-Paraffinic Kerosene (IPK)), Jet A/Gevo ATJ, Jet A/LanzaTech ETJ, and Jet A/Farnesane. Here, ETJ and GTL stand for the ethanol-to-jet and gas-to-liquid fuels, respectively.
Shi et al.15 correlated NHC with detailed composition of 17 aviation fuels obtained from GC × GC-MS/FID measurements by four statistical algorithms as described in the density section and the ESI.† The MWA method was reported to give the best results with R2 of 0.9993 and mean absolute error (MAE) of 0.0102 MJ kg−1.
Freezing point
Freezing point is a vital low-temperature fluidity characteristic for aviation fuels, especially in low temperature environments such as at high altitudes. Freezing point is the temperature at which the last wax crystal melts, when warming a fuel that has previously been cooled until wax crystals form.41,49 The freezing points of Jet A and Jet A-1 are specified to be −40 °C and −47 °C, respectively (Table 1). Typically, the requirement is to maintain the fuel with a temperature of at least 3 °C above its freezing point.20,42
Freezing point is influenced by molecular structure and symmetry. The freezing point of hydrocarbons is affected by carbon number33,43 and hydrocarbon class.14,33,44 Yang et al.14 listed three key factors that significantly impact the freezing point of bio-jet fuel; they are iso-paraffins content, alkylated aromatics content, and the carbon chain length of bio-paraffins. n-Paraffins have the highest freezing point within a carbon number. Increasing iso-paraffin content in aviation fuels was found to lower freezing point.14,43,44 A definite trend was not found for cycloparaffins,43 however, generally low freezing points were observed for alkyl-substituted cycloparaffins.45,46 Certain aromatic compounds can reduce the freezing point of bio-jet fuels47 and blends of jet fuels with a gas-to-liquid-derived synthetic paraffinic kerosene (GTL SPK).48 Average carbon number of a fuel also influences its freezing point; fuels with lower average carbon number have lower freezing points.19
Cookson et al. correlated freezing point with chemical composition detected by high-performance liquid chromatography (HPLC), GC, and 13C NMR.49–52 One equation related freezing point to the total amount of n-paraffins:49
| FP (°C) = 60.7w(n) − 62.0 | (8) |
The other equation used the amount of n-paraffins C12–C14:49
| FP (°C) = 85.5w (C12 to C14) − 60.3 | (9) |
The third equation used the total amount of n-paraffins, branched and cyclic paraffins and aromatics.49,52
| FP (°C) = −0.8w(n) − 63.8w(BC) − 55.9w(Ar) | (10) |
The freezing point data of kerosene fuels used to develop eqn (8)–(10) ranged from −50 °C to −32 °C.
Distillation temperatures were later introduced.51 For the equation below, the freezing point data of jet fuels used ranged from −70 °C to −33.5 °C.
| FP (°C) = 81.1w(n) + 53.6w(Ar) + 0.255T10 (°C) + 0.338T90 (°C) − 206.2 | (11) |
In eqn (8)–(11), w(n), w(BC), and w(Ar) are weight fractions of n-paraffins, branched + cyclic paraffins, and aromatics, respectively. w(C12–C14) is weight fraction of the C12 to C14 n-paraffins. T10 and T90 were obtained from GC or simulated distillation method.51
Vozka et al.33 evaluated the four Cookson equations for predicting the freezing point of blends of Jet A and three HEFAs: CAME, TALL, and MFAT. They reported that since the freezing point values used in the development of the Cookson equations were in the range of −50 °C to −32 °C and the fuels used originated from different sources, these equations could not accurately predict the freezing points of the HEFA blends. The experimental freezing points of Jet A/HEFA blends range from −59 °C to −48.5 °C; with the lowest value lying outside the correlation limit of −50 °C. They applied the Cookson equations to predict freezing point of their mixtures anyway and concluded that none of the equations produced accurate results for TALL/Jet A blends, while eqn (8) predicted the freezing point within 2 °C of experiments for CAME/Jet A and most of MFAT/Jet A blends.
Shi et al.15 also correlated freezing point with detailed composition of 17 aviation fuels obtained from GC × GC-MS/FID measurements by different statistical algorithms as discussed previously. The freezing point data used ranged from −67 °C to −37.5 °C. The MWA method was reported to give the best results with R2 of 0.9866 and MAE of 0.82 °C.
Wang et al.19 and Vozka et al.13 listed many other methods used to estimate freezing point of aviation and SAF fuels. They are not considered in this work.
Distillation temperature
According to ASTM D7566,53 distillation temperatures of aviation fuels refer to the D86 distillation temperatures, which can be obtained using the ASTM D86 (ref. 29) or ASTM D2887 (ref. 32) procedures. For the D2887 procedure, the simulated distillation data are first obtained, then converted to the D86 temperatures using the correlation given in Appendix X4 of the ASTM D2887 standard.32 For this correlation, the test method D86 correlated data is calculated from the test method D2887 data using the following equation, | tn = a0 + a1·Tn−1 + a2·Tn + a3·Tn+1 | (12) |
where tn is the nth boiling point temperature of test method D86 correlated, ai is the ith coefficient given in Table X4.1 of the ASTM D2887 standard, and Tn is the nth boiling point temperature of D2887.
The distillation temperatures of an aviation fuel can be predicted by calculating the equivalent D2887 temperatures using the compositions (weight percent) and normal boiling point temperatures of the constituent compounds in the fuel. A spline fit of the sorted normal boiling point temperatures versus the cumulative weight percent of the components is used to obtain the equivalent D2887 temperatures. Eqn (12) is then used to calculate the distillation temperature from the D2887 temperatures.
Experimental measurements for jet fuel cuts of hydrotreated CFP oils
CFP-derived liquid hydrocarbons were produced via pilot-scale or bench-scale CFP to generate CFP oil, followed by hydrotreating (HT) of the oil. The steps mirror the conceptual process described above (Fig. 1). The hydrotreated product was further distilled to obtain the jet fuel boiling range cuts used for compositional analysis and property measurements. Those results were used in this work to evaluate how well the composition-based, and ASTM prediction methods discussed in the preceding section perform compared to the experimental data.
The jet range fuels used in this work were produced from hydrotreating of two different CFP intermediates that had been produced using different CFP catalysts, ZSM-5 and Pt/TiO2. The first CFP intermediate was produced in the thermochemical pilot-scale process development unit at NREL54 with a feed rate of 10 kg h−1 of pine in an ex situ configuration at 550 °C, over a ZSM-5 catalyst from Equilibrium Catalyst, Inc.,55 and the second CFP intermediate was produced in a bench-scale ex situ fluidized-bed pyrolyzer and fixed bed upgrading reactor combination with a feed rate of 150 g h−1 of a mixture of pine and forest residues over a 0.5 wt% Pt/TiO2 catalyst.9 Both CFP oils were hydroprocessed in a continuous trickle bed reactor in a staged process with a maximum temperature of 385 °C and a pressure of 125 bar over a sulfided NiMo/Al2O3 catalyst without plugging issues.8 The hydrotreated product was further fractionated via distillation in a micro spinning band distillation apparatus, and the cut obtained at 145–245 °C was evaluated for jet fuel properties. For convenience, the two resultant jet fuels will be referred to as “ZSM-5 CFP, HT fuel” and “Pt/TiO2 CFP, HT fuel”, respectively, for the remainder of the paper.
Compositions of these jet range fuels were obtained using GC × GC-MS/FID method on a LECO Pegasus 4D system.56 Components were identified based on mass spectral matching using LECO ChromaTOF® software56 and the NIST 2014 Mass Spectral Library,57 characteristic spectral features, and retention times. The GC × GC/FID results were quantified based on calculated response factors (using effective carbon number) and normalized to 100%; note that the boiling range of the jet fuel cut is in a temperature range which fully evaporates in the GC inlet and elutes through the column for detection. Details of the analysis are included in the ESI.†Table 2 shows the normalized mass percentage of compounds found by class. Both fuels were dominated by cycloparaffins. The Pt/TiO2 CFP, HT fuel contained more than twice the amount of mono-branched mono-cycloparaffins and about 5 wt% less of the other types of cycloparaffins compared to the ZSM-5 CFP, HT fuel. For other compound classes, the overall compositions of the two fuels were similar. Detailed compositions of compounds identified for ZSM-5 CFP, HT fuel and Pt/TiO2 CFP, HT fuel can be found in Tables S2 and S3, respectively in the ESI.† The heaviest compound identified in ZSM-5 CFP, HT fuel was C13H22 ((3aα, 6aα, 9aα, 9bβ)-perhydrophenalene), while that for Pt/TiO2 CFP, HT fuel was C14H26 (1,1′-(1,2-ethanediyl)biscyclohexane). No C7 compounds were identified in the Pt/TiO2 CFP, HT fuel.
Table 2 Mass percentage of compound groups found in the jet fuel cuts from the distillation of the hydrotreated products from CFP oils obtained using two different catalysts (ZSM-5 and Pt/TiO2)
Class |
ZSM-5 CFP, HT fuel |
Pt/TiO2 CFP, HT fuel |
n-Paraffins |
1.84 |
2.57 |
Iso-paraffins |
2.74 |
2.13 |
One-branched monocycloparaffins |
16.74 |
31.33 |
Multi-branched monocycloparaffins |
36.32 |
30.51 |
Di- and tri-cycloparaffins |
37.51 |
29.63 |
Alkylbenzenes |
2.02 |
2.26 |
Cycloaromatics |
2.82 |
1.57 |
Measured properties for the fuels included liquid density at 15 °C, freezing point, NHC, D86 distillation temperature at 10%, D86 distillation FBP, and flash point. The measurements are summarized in Table 3. The measured properties are well within the specification limits of aviation fuels shown in Table 1.12
Table 3 Measured properties of the jet fuel cuts from the distillation of the hydrotreated products from ZSM-5 and Pt/TiO2 CFP oils
|
Densitya at 15 °C (kg m−3) |
Freezing pointa (°C) |
NHCb (MJ kg−1) |
Distillationc, 10% (°C) |
Distillationc, FBP (°C) |
Flash pointa (°C) |
Measured at an ambient pressure of 0.08 MPa.
Refers to t = 25 °C and p = 101.3 kPa.
Estimated D86 temperature obtained from D2887 data using eqn (12).
Expanded uncertainties for 0.95 level of confidence. Estimated as reproducibility values taken from the standards. For distillation data, the values are for D2887 data.
|
ASTM D756653/D405458 |
775 to 840 |
Max −40 |
>42.8 |
≤205 |
≤300 |
>38 |
ZSM-5 CFP, HT fuel |
834 |
<−70 |
43 |
174.3 |
249.2 |
49.6 |
Pt/TiO2 CFP, HT fuel |
833 |
<−70 |
43 |
170.2 |
256.8 |
46.6 |
Reproducibilityd |
0.5 |
0.8 |
0.234 |
2.4 |
11.8 |
3.1 |
Pure properties and methods used for mixture property predictions
We collected pure component properties of the compounds (Tables S2 and S3†) identified in the ZSM-5 CFP, HT fuel and Pt/TiO2 CFP, HT fuel because pure component properties inform mixture property predictions in most of the methods. The pure-component properties collected included liquid density at 15 °C, freezing point, NHC, flash point, and normal boiling point; these were necessary for the mixture properties reported in this paper.
Thermodynamic properties of pure compounds were found with the NIST ThermoData Engine (TDE)59 software. In this software, the dynamic data evaluation concept is implemented to obtain thermodynamic and thermophysical properties of compounds and mixtures. The available data are used as input parameters for single-property or multi-property equations. If the experimental property values are not available, the gaps are filled with the predicted ones. Details on the TDE procedures for pure compounds have been published.60–62
The pure-compound freezing points were found as the crystal-liquid triple-point temperatures. This quantity was evaluated by TDE, and the values were reported only if experimental results were available. To fill gaps in the experimental data, saturated liquid densities were predicted by the Yamada-Gunn method using the modified Rackett equation.63
NHC of compound CaHbOc is the enthalpy (isobaric heat of reaction) of its quantitative combustion in oxygen where the products are gaseous H2O and CO2. The specific net heats of combustion at T = 25 °C and P = 0.1 MPa were calculated with the equation:
|  | (13) |
where Δ
fH(CO
2(g)) = −393.51 kJ mol
−1 and Δ
fH(H
2O(g)) = −241.826 kJ mol
−1 were recommended by CODATA
64 and
M is the molar mass of the target compound. The enthalpy of formation of the compound, Δ
fH(C
aH
bO
c), was found with one of three procedures described below. If experimental data were available, the Δ
fH value in the liquid phase evaluated by TDE was used. Otherwise, the gas-phase value was estimated using the group-contribution procedure by Verevkin
et al.65 The enthalpies of vaporization evaluated by TDE were subtracted from the estimated value to obtain the liquid-phase values. For some compounds, the experimental data appeared to be unreliable and/or the above predictive procedure could not be used. In this case, the gas-phase enthalpy of formation was predicted using the aLL5 variation of the
ab initio-based protocol by Paulechka and Kazakov
66 and converted to the liquid phase value as described above.
The normal boiling point temperatures were derived from the temperature-dependent vapor pressures interpolated by the Wagner 25 equation.67
|  | (14) |
where
T and
Tc are the temperature and critical temperature in °C, respectively, and
pc is the critical pressure.
Tc values were found using the experimental data or predictive procedures.
68–72 The critical pressure and vapor pressures were obtained from simultaneous regression of multiple properties (
p,
pc, enthalpy of vaporization, liquid and gas heat capacities,
etc.). The source of the input
pc values was either experimental or one of the predictive methods.
68–71,73 The predicted
pc values given in Table S4
† are the ones used as the inputs. The evaluated
pc values are close, but not necessarily equal, to them.
For the compounds considered here, the experimental vapor pressures were not measured over the whole temperature range from the triple point to the critical point or were not available at all. To fill the gaps, predictions with the Ambrose–Walton73 method were used. An acentric factor is required in this method. In some cases, it was found from the critical parameters and the normal boiling point temperature predicted with the Nannoolal–Rarey–Ramjugernath–Cordes74 or Constantinou–Gani72 methodologies.
Flash points were calculated by a two-step procedure. The flash-point pressure of a liquid CaHbOc was estimated using the equation:75
| 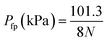 | (15) |
where
N =
a + (
b − 2
c)/4 is the number of moles of O
2 required for complete combustion of 1 mol of the compound. Then, the flash-point temperature was found using eqn
(14).
Selected properties of the compounds are presented in Table S4 in the Excel file of the ESI.†
Results and discussion
Properties of ZSM-5 CFP, HT fuel and Pt/TiO2 CFP, HT fuel were predicted using composition-based correlations as well as the ASTM methods discussed above and compared to experimental measurements shown in Table 3. Results are discussed in this section.
Liquid density
Liquid density at 15 °C was predicted using the statistical methods of Shi et al.15 including WA, PLS, GA, and MWA methods; the methods of Vozka et al.16 with 98 predictors including PLS product, PLS composition, SVM product, and SVM composition methods; and eqn (1). Although the methods of Shi et al. were developed using data at 20 °C, we included them for comparison. In applying these methods for density prediction (as well as flash point, freezing point, and NHC), the composition matrices of the CFP-derived SAF fuels were constructed using the weight % data in Tables S2 and S3† and by mapping each compound in the table to one of the 10 classes defined in the methods. The resulting composition matrices are summarized in Tables S5 and S6.† The density matrix from the Shi et al. paper15 with average density values for each carbon number in the class was used instead of the density value for each individual component of the fuel. For the methods of Vozka et al.,16 the composition and density matrices were constructed in the same way as the Shi's methods with compounds mapped to one of the seven classes defined in the methods, but with a representative density value for each carbon number in the class, as recommended by the authors. The resulting composition matrices for the fuels are shown in Tables S7 and S8 of the ESI.† When applying eqn (1), the density value for each individual component shown in Table S4 of the ESI† was used. The prediction results using these methods shown in table are compared with the experimental data from table. For each method, error, mean absolute error (MAE), percent error, and mean absolute percent error (MAPE) are reported (Table 4).
Table 4 Comparison between experimental (exp.) density data (g cm−3) at 15 °C and predictions for the two jet range fuels produced from hydrotreated ZSM-5 and Pt/TiO2 CFP oils
Fuel name |
Exp. |
Prediction |
WAa,b |
PLSa,b |
GAa,b |
MWAa,b |
PLS_P |
PLS_C |
SVM_P |
SVM_C |
Eqn (1) |
Estimation methods of Shi et al.:15 WA = weighted average, PLS = partial least squares, GA = genetic algorithm, MWA = modified weighted average. Estimation methods of Vozka et al.:16 PLS_P = partial least squares product, PLS_C = partial least squares composition, SVM_P = support vector machine product, SVM_C = support vector machine composition.
Estimation methods of Shi et al.15 are for density at 20 °C but are included for comparison.
MAE = mean absolute error.
MAPE = mean absolute percent error.
|
ZSM-5 CFP, HT fuel |
0.8345 |
0.8208 |
0.8187 |
−0.5380 |
0.8336 |
0.8415 |
0.8439 |
0.7784 |
0.7666 |
0.8363 |
Pt/TiO2 CFP, HT fuel |
0.8332 |
0.8138 |
0.8099 |
−1.5786 |
0.8389 |
0.8348 |
0.8377 |
0.8123 |
0.8126 |
0.8263 |
![[thin space (1/6-em)]](https://www.rsc.org/images/entities/char_2009.gif) |
|
|
Error
|
ZSM-5 CFP, HT fuel |
|
−0.0137 |
−0.0158 |
−1.3725 |
−0.0009 |
0.0070 |
0.0094 |
−0.0561 |
−0.0679 |
0.0018 |
Pt/TiO2 CFP, HT fuel |
|
−0.0194 |
−0.0233 |
−2.4118 |
0.0057 |
0.0016 |
0.0045 |
−0.0209 |
−0.0206 |
−0.0069 |
|
MAEc |
0.0165 |
0.0195 |
1.8921 |
0.0033 |
0.0043 |
0.0070 |
0.0385 |
0.0443 |
0.0044 |
![[thin space (1/6-em)]](https://www.rsc.org/images/entities/char_2009.gif) |
|
|
% Error
|
ZSM-5 CFP, HT fuel |
|
−1.64 |
−1.89 |
−164.47 |
−0.1 |
0.83 |
1.1 |
−6.72 |
−8.14 |
0.21 |
Pt/TiO2 CFP, HT fuel |
|
−2.33 |
−2.80 |
−289.46 |
0.68 |
0.20 |
0.54 |
−2.51 |
−2.47 |
−0.83 |
|
MAPEd |
1.98 |
2.34 |
226.96 |
0.39 |
0.51 |
0.84 |
4.62 |
5.31 |
0.52 |
The MWA, PLS_P, and eqn (1) methods predicted liquid density at 15 °C with MAPE of ∼0.5%, with the MWA method having the lowest MAPE of 0.39%. The GA method predicted negative density for both fuels. The negative density prediction likely indicates that the method was developed using too few fuel samples (17), given the large number of model coefficients involved (20) compared to the PLS or MWA methods, which used 10 coefficients each. All four methods of Shi et al. underpredicted density at 15 °C of both fuels, except MWA, which overpredicted density of Pt/TiO2 CFP, HT fuel. Since these methods were developed using density data at 20 °C, the under-prediction of data at the lower temperature of 15° is reasonable. While the SVM_C method had been shown to provide the most accurate results (MAPE of 0.0984%) for 50 samples of petroleum-derived fuels and alternative fuel blending components,16 it underpredicted liquid density of the two CFP-derived SAF fuels, resulting in MAPE of 5.31%. It is likely that the large discrepancy in accuracy is caused by the especially high contents of cycloparaffins present in the two fuels (>90 wt%) compared to those used in the development of the SVM_C method. The PLS product and PLS composition methods performed better than the SVM product and SVM composition methods for these fuels with MAPE of 0.51% and 0.84%, respectively. The simple weighted average method of eqn (1) also provided good predictions with MAPE of 0.52%. Similar results of ∼0.5% by the three best methods (MWA, PLS_P, and eqn (1)) can be considered quite good. However, they are not good enough since the MAEs of ∼0.004 g cm−3 are larger than the reproducibility of the measured density data of 0.0005 g cm−3.
Flash point
Flash points of the CFP-derived SAF fuels were predicted using the methods of Shi et al.,15 the API correlation (eqn (2),28), the ASTM D7215 method (eqn (4),17), and the modified ASTM method (eqn (5),33). For the Shi et al. methods, the same composition matrices used in the density predictions were used. The flash point property matrix from the paper15 with average flash point value for each carbon number in the class was used. The revised coefficients for the MWA method as shown in Table S10 of the ESI were used since the values presented in table 9 of the original paper15 for flash point were incorrect because they were swapped with those for freezing point method.76
For eqn (2), the ASTM D86 10% temperature, t10, was calculated using the method described in the distillation temperature section above, and from composition of the constituent compounds of the fuels shown in Tables S2 and S3† and normal boiling point temperatures in Table S4.† The distillation temperatures TIBP, T5, and T10 required in the ASTM D7215 method, eqn (4) and its modification, eqn (5) were calculated from composition shown in Tables S2 and S3† and normal boiling point temperatures in Table S4.† A spline fit of the sorted normal boiling point temperatures versus the cumulative weight percent of the components is used to obtain the required temperatures. Prediction results using these methods are compared to the experimental data in Table 5.
Table 5 Comparison between experimental data (exp.) and predicted flash points (°C) for the two jet range fuels produced from hydrotreated ZSM-5 and Pt/TiO2 CFP oils
Fuel name |
Exp. |
Prediction |
WAa |
PLSa |
GAa |
MWAa |
API |
ASTM 1 |
ASTM 2 |
Estimation methods of Shi et al.:15 WA = weighted average, PLS = partial least squares, GA = genetic algorithm, MWA = modified weighted average. API = eqn (2);28 ASTM 1 = ASTM D7215 – eqn (4);17 ASTM 2 = modified ASTM D7215 – eqn (5).33
MAE = mean absolute error.
MAPE = mean absolute percent error.
|
ZSM-5 CFP, HT fuel |
49.6 |
47.1 |
28.8 |
172.7 |
52.2 |
46.2 |
47.4 |
47.9 |
Pt/TiO2 CFP, HT fuel |
46.6 |
42.1 |
−1.3 |
17.4 |
49.9 |
46.6 |
48.0 |
48.9 |
![[thin space (1/6-em)]](https://www.rsc.org/images/entities/char_2009.gif) |
|
|
Error
|
ZSM-5 CFP, HT fuel |
|
−2.5 |
−20.8 |
123.1 |
2.6 |
−3.4 |
−2.2 |
−1.7 |
Pt/TiO2 CFP, HT fuel |
|
−4.5 |
−47.9 |
−29.2 |
3.3 |
0.0 |
1.4 |
2.3 |
|
MAEb |
3.5 |
34.3 |
76.2 |
2.9 |
1.7 |
1.8 |
2.0 |
![[thin space (1/6-em)]](https://www.rsc.org/images/entities/char_2009.gif) |
|
|
% Error
|
ZSM-5 CFP, HT fuel |
|
−5.0 |
−41.9 |
248.2 |
5.1 |
−6.8 |
−4.4 |
−3.3 |
Pt/TiO2 CFP, HT fuel |
|
−9.6 |
−103 |
−62.7 |
7.1 |
0.0 |
3.1 |
5.0 |
|
MAPEc |
7.3 |
72.3 |
156 |
6.1 |
3.4 |
3.8 |
4.2 |
Four prediction methods, MWA, API, ASTM 1, and ASTM 2, gave MAE within the reproducibility of the measured flash point data of 3.1 °C. The API method (eqn (2)) gave the lowest MAE and MAPE among the methods tested. It underpredicted flash point of the ZSM-5 CFP, HT fuel by 3.4 °C, but predicted the property of the Pt/TiO2 CFP, HT fuel exactly, resulting in an MAE of 1.7 °C and an MAPE of 3.4%. The ASTM D7215 method (ASTM 1) gave a slightly larger MAE of 1.8 °C and MAPE of 3.8%, with a smaller error for ZSM-5 CFP, HT fuel, and a larger error for the Pt/TiO2 CFP, HT fuel. The ASTM 2 method provided the next best results with MAE and MAPE of 2.0 °C, and 4.2%, respectively. The recommended MWA method of Shi et al. (with a reported MAPE of 1.24%)15 had the lowest MAE and MAPE among the four methods of Shi et al. However, it overpredicted flash point of Pt/TiO2 CFP, HT fuel compared to the API, ASTM 1, and ASTM 2 methods, hence larger MAE (2.9 °C) and MAPE (6.1%). The significantly larger errors of the MWA method for the two CFP-derived SAF fuels compared to literature15 may be caused by the significantly higher contents of cycloparaffins present in these fuels (>90 wt%) compared to the values of ∼50 wt% for most of the fuels used in the development of the method.15 The WA method provided the next best results among the Shi et al. methods. The PLS method predicted flash points that are significantly lower than the minimum of 38 °C allowed for aviation fuels (see Table 1). The GA method significantly overpredicted flash point of ZSM-5 CFP, HT fuel, but underpredicted Pt/TiO2 CFP, HT fuel. The erratic behavior may be attributed to overfitting of the data as previously discussed for density.
Net heat of combustion (NHC)
NHC of the two CFP-derived SAF fuels was predicted using the methods of Shi et al.,15 the ASTM D3338 method, eqn (6),18 and the simple weight fraction average of pure component NHC, eqn (7). For the Shi et al. methods, the same composition matrices used in the density and flash point predictions were used. The NHC property matrix from the paper15 with average property value for each carbon number in the class was also used. For eqn (6), the aromatics volume%, A, was computed from the composition data of aromatics compounds shown in Tables S2 and S3 of the ESI.† Liquid density at 15 °C, ρ15, was calculated using the Shi's MWA method, which provided the best prediction of liquid density at 15 °C for these two fuels. The volatility, T, was calculated from the average of test method D86 10%, 50%, and 90% points. When applying eqn (7), the NHC value for each individual component shown in Table S4 of the ESI† was used. The prediction results using these methods are compared against the experimental data in Table 6.
Table 6 Comparison between experimental data (exp.) and predicted NHC (MJ kg−1) for the two jet range fuels produced from hydrotreated ZSM-5 and Pt/TiO2 CFP oils
Fuel name |
Exp. |
Prediction |
WAa |
PLSa |
GAa |
MWAa |
ASTM |
Eqn (7) |
Estimation methods of Shi et al.:15 WA = weighted average, PLS = partial least squares, GA = genetic algorithm, MWA = modified weighted average. ASTM = ASTM D3338 – eqn (6).18
MAE = mean absolute error.
MAPE = mean absolute percent error.
|
ZSM-5 CFP, HT fuel |
42.99 |
43.01 |
43.63 |
37.21 |
43.53 |
42.95 |
43.03 |
Pt/TiO2 CFP, HT fuel |
43.02 |
43.11 |
44.53 |
34.48 |
44.38 |
43.03 |
43.13 |
![[thin space (1/6-em)]](https://www.rsc.org/images/entities/char_2009.gif) |
|
|
Error
|
ZSM-5 CFP, HT fuel |
|
0.02 |
0.64 |
−5.78 |
0.53 |
−0.04 |
0.04 |
Pt/TiO2 CFP, HT fuel |
|
0.09 |
1.51 |
−8.54 |
1.35 |
0.01 |
0.11 |
|
MAEb |
0.05 |
1.08 |
7.16 |
0.94 |
0.02 |
0.07 |
![[thin space (1/6-em)]](https://www.rsc.org/images/entities/char_2009.gif) |
|
|
% Error
|
ZSM-5 CFP, HT fuel |
|
0.04 |
1.5 |
−13.4 |
1.2 |
−0.09 |
0.1 |
Pt/TiO2 CFP, HT fuel |
|
0.2 |
3.51 |
−19.9 |
3.14 |
0.02 |
0.24 |
|
MAPEc |
0.1 |
2.50 |
16.7 |
2.2 |
0.05 |
0.2 |
The WA method of Shi et al., the ASTM D3338 correlation, and eqn (7) predicted NHC of the two CFP-derived SAF fuels with MAPE well below 1% and with MAE within the reproducibility of the measured data of 0.234 MJ kg−1. The difference between the WA method and eqn (7) lies in the fact that the former uses an average value of NHC for each of the 13 carbon numbers in the 10 compound classes while for eqn (7), NHC for each individual compound was used. However, the predicted NHCs of the fuels themselves are essentially the same. MWA, the best method from Shi et al.,15 overpredicted the data and gave MAPE of 2.2%. The PLS method also overpredicted the data, resulting in an MAPE of 2.5%. The GA method underpredicted NHC with values that are well below the minimum value of 42.8 MJ kg−1 required for aviation fuels (see Table 1). The ASTM D3338 correlation gave the lowest MAE (0.02 MJ kg−1) and MAPE (0.05%) for these fuels.
Freezing point
Freezing points of the two CFP-derived SAF fuels were predicted using the methods of Shi et al.15 and Cookson et al. (eqn (8)–(11)). For the Shi et al. methods, the composition matrices from the density predictions were used. The freezing point property matrix from the paper15 with average freezing point value for each carbon number in the class was also used. The revised coefficients for the MWA method as shown in Table S10 of the ESI† were required since the values presented in Table 9 of the original paper15 were swapped with those for the flash point method.76 For the Cookson et al. correlations, eqn (8)–(11), the parameters w(n), w(BC), and w(Ar) were computed from the weight fractions of n-paraffins, branched + cyclic paraffins, and aromatics, respectively, of the CFP-derived SAF fuels (Table 2). w(C12 to C14) was determined from the sum of the weight fractions of all C12 to C14 n-paraffins. T10 and T90 were determined using the same procedure employed in computing the distillation temperatures for flash point prediction via the ASTM D7215 method (eqn (4)).
Prediction results using these methods are compared with the experimental data in Table 7. Since the experimental freezing points of both fuels were reported as <−70 °C, actual errors and % errors could not be determined. However, a value of −70 °C allowed the comparison of predicted results against this upper limit.
Table 7 Comparison between experimental data (exp.) and predicted freezing points (°C) for the two jet range fuels produced from hydrotreated ZSM-5 and Pt/TiO2 CFP oils
Fuel name |
Exp. |
Prediction |
WAa |
PLSa |
GAa |
MWAa |
Eqn (8) |
Eqn (9) |
Eqn (10) |
Eqn (11) |
Estimation methods of Shi et al.:15 WA = weighted average, PLS = partial least squares, GA = genetic algorithm, MWA = modified weighted average.
Errors and errors% were computed assuming that the experimental freeze point was −70 °C.
MAE = mean absolute error.
MAPE = mean absolute percent error.
|
ZSM-5 CFP, HT fuel |
<−70 |
−64 |
−88 |
−75 |
−88 |
−61 |
−60 |
−62 |
−94 |
Pt/TiO2 CFP, HT fuel |
<−70 |
−71 |
−106 |
−69 |
−89 |
−60 |
−60 |
−62 |
−95 |
![[thin space (1/6-em)]](https://www.rsc.org/images/entities/char_2009.gif) |
|
|
Error
|
ZSM-5 CFP, HT fuel |
|
6 |
−18 |
−5 |
−18 |
9 |
10 |
8 |
−24 |
Pt/TiO2 CFP, HT fuel |
|
−1 |
−36 |
1 |
−19 |
10 |
10 |
8 |
−25 |
|
MAEc |
4 |
27 |
3 |
19 |
9 |
10 |
8 |
24 |
![[thin space (1/6-em)]](https://www.rsc.org/images/entities/char_2009.gif) |
|
|
% Error
|
ZSM-5 CFP, HT fuel |
|
9 |
−26 |
−7 |
−26 |
13 |
15 |
11 |
−34 |
Pt/TiO2 CFP, HT fuel |
|
−1 |
−51 |
2 |
−27 |
14 |
14 |
12 |
−36 |
|
MAPEd |
5 |
38 |
4 |
27 |
13 |
14 |
11 |
35 |
All the methods tested predicted meaningful freezing points for the two fuels, without any erratic results as observed for some of the density and flash point methods. In addition, no predicted values are above the specification limits of −40 °C for Jet A and −47 °C for Jet A-1. For the ZSM-5 CFP, HT fuel, the WA, eqn (8)–(10) predicted freezing points that are higher than the −70 °C limit of the data by more than 5 °C, with eqn (8) and (9) over-predicting by 9 and 10 °C, respectively. The remaining methods predicted freezing points that are lower than the upper limit of −70 °C. The GA method and eqn (8)–(10) overpredicted the freezing point data of the Pt/TiO2 CFP, HT fuel. However, both the WA and GA methods predicted freezing points that are close to the upper value of −70 °C for this fuel. The remaining three methods (PLS, MWA, eqn (11)) predicted freezing points that are well below the upper limit of −70 °C. Since the actual value of freezing point was not reported, it is not possible to judge the relative merits of each prediction method. However, it is reasonable to state that the methods that predicted freezing points below and close to −70 °C are better than those that overpredicted it.
Distillation temperature
Distillation temperatures t10 and tFBP of the two CFP-derived SAF fuels were calculated using the method described in the distillation temperature section above, and compositions and normal boiling points of the constituent compounds of the two fuels shown in Tables S2–S4 of the ESI.† Prediction results are summarized in Table 8. The procedure underpredicted t10 of the ZSM-5 CFP, HT fuel and Pt/TiO2 CFP, HT fuel by 9.4 °C and 4.8 °C, respectively. The resulting MAE of 7.1 °C is well above the reproducibility limit of 2.1 °C. For tFBP, the method underpredicted the data by 24.7 °C and 39.7 °C, respectively, resulting in MAE of 32.2 °C, which is also well above the reproducibility limit of 11.8 °C. The significant underprediction is likely caused by the estimated normal boiling points of the compounds in the heavy ends of the fuels.
Table 8 Comparison between experimental data (exp.) and predicted distillation temperatures (°C) for the two jet range fuels produced from hydrotreated ZSM-5 and Pt/TiO2 CFP oils
Fuel name |
t
10
|
t
FBP
|
Exp. |
ASTMa |
Exp. |
ASTMa |
ASTM D2887 conversion procedure described above.32
MAE = mean absolute error.
MAPE = mean absolute percent error.
|
ZSM-5 CFP, HT fuel |
174.3 |
164.9 |
249.2 |
224.5 |
Pt/TiO2 CFP, HT fuel |
170.2 |
165.4 |
256.9 |
217.1 |
![[thin space (1/6-em)]](https://www.rsc.org/images/entities/char_2009.gif) |
|
|
Error
|
|
Error
|
ZSM-5 CFP, HT fuel |
|
−9.4 |
|
−24.7 |
Pt/TiO2 CFP, HT fuel |
|
−4.8 |
|
−39.7 |
|
MAEb |
7.1 |
|
32.2 |
![[thin space (1/6-em)]](https://www.rsc.org/images/entities/char_2009.gif) |
|
|
% Error
|
|
% Error
|
ZSM-5 CFP, HT fuel |
|
−5.4 |
|
−9.9 |
Pt/TiO2 CFP, HT fuel |
|
−2.8 |
|
−15.4 |
|
MAPEc |
4.1 |
|
12.7 |
Conclusion
This work showed that flash point, and NHC of jet range fuels from two different CFP catalysts can be well predicted using many of the available prediction methods with mean absolute errors that are within the reproducibility limit of the property data. The API correlation (eqn (2)), and the ASTM D3338 correlation (eqn (6)) provided the lowest mean absolute error for flash point, and NHC, respectively. Density at 15 °C can be predicted with mean absolute percent error of ∼0.5%, which is quite good, but not accurate enough. For freezing point, all the methods tested predicted meaningful results for the two fuels. However, the accuracy of the prediction methods could not be assessed since the experimental data of the two fuels were given as <−70 °C. To facilitate model evaluation, any future measurements of freezing point data for CFP-derived SAF fuels should report actual numerical values. The t10 and tFBP distillation temperatures were not well predicted by the method tested, which significantly underpredicted the experimental data. The shortcomings are likely caused by the uncertainty of the estimated normal boiling points of the compounds in the fuels.
While results for density, flash point, and NHC are encouraging, the evaluation was based on only two sets of data. Additional data points would be useful in improving the confidence in the applicability of the methods. The scarcity of data was due to the efforts and resources necessary for producing the required samples, along with the associated analytical measurements, within the time window of the initial experimental work. There are ongoing efforts at NREL to produce additional CFP-derived SAF samples for compositional and property analysis. These data, when they become available, will be useful in further evaluation effort and to update of the knowledge gained in this work. Our future work will also report on the prediction of some additional properties shown in Table 1 to help advance the development of CFP-derived SAF.
Author contributions
SW: literature data collection, mixture property predictions modeling, technical coordination, writing – initial draft and subsequent review/edits. EP: data collection, estimation and compilation of pure properties, writing/review/edits. KI: experimental work and providing related data, writing/review/edits. EC: analytical measurements of CFP products and related review/edits. CM: NIST coordination and group lead, review/edits. AD: conceptualization and coordination as NREL project lead for thermochemical analysis, writing/review/edits.
Conflicts of interest
The authors declare that they have no known competing financial interests or personal relationships than could have appeared to influence the work reported in this paper.
Acknowledgements
Trade names are provided only to specify procedures adequately and do not imply endorsement by the National Institute of Standards and Technology or the National Renewable Energy Laboratory. Similar products by other manufacturers may be found to work as well or better. This work was authored in part by the National Renewable Energy Laboratory, operated by Alliance for Sustainable Energy, LLC, for the U.S. Department of Energy (DOE) under Contract No. DE-AC36-08GO28308. Funding provided by U.S. Department of Energy Office of Energy Efficiency and Renewable Energy Bioenergy Technologies Office. The views expressed in the article do not necessarily represent the views of the DOE or the U.S. Government. The U.S. Government retains and the publisher, by accepting the article for publication, acknowledges that the U.S. Government retains a nonexclusive, paid-up, irrevocable, worldwide license to publish or reproduce the published form of this work, or allow others to do so, for U.S. Government purposes. The authors wish to thank Dr Calvin Mukarakate, Dr Michael Griffin, Dr Joshua Schaidle, Michael Talmadge, Dr Richard French, Scott Palmer, Kellene Orton, Rebecca Jackson, Andy Young, Katie Gaston, Kristin Smith, and many other members of the Thermochemical Conversion team at NREL, and personnel from other National Laboratories who played important roles towards our research advancements in CFP of biomass.
References
-
Memorandum of Understanding Sustainable Aviation Fuel Grand Challenge, 2021, available at: https://www.energy.gov/sites/default/files/2021-09/S1-Signed-SAF-MOU-9-08-21_0.pdf, accessed July 24, 2022 Search PubMed.
-
Fit for 55 and ReFuelEU Aviation, available at: https://www.easa.europa.eu/light/topics/fit-55-and-refueleu-aviation, accessed July 24, 2022 Search PubMed.
-
Billion Ton Report, 2016, https://www.energy.gov/eere/bioenergy/2016-billion-ton-report Search PubMed.
-
Sustainable Aviation Fuels: Review of Technical Pathways, Bioenergy Technologies Office, DOE/EE-2041, September 2020, available at: https://www.energy.gov/sites/prod/files/2020/09/f78/beto-sust-aviation-fuel-sep-2020.pdf, accessed July 28, 2022 Search PubMed.
- D. Mohan, C. U. Pittman and P. H. Steele, Pyrolysis of Wood/Biomass for Bio-oil: A Critical Review, Energy Fuels, 2006, 20, 848–889 CrossRef CAS
.
- R. H. A. Venderbosch, Critical View on Catalytic Pyrolysis of Biomass, ChemSusChem, 2015, 8, 1306–1316 CrossRef CAS PubMed
.
-
A. Dutta, A. Sahir, E. Tan, D. Humbird, L. Snowden-Swan, P. Meyer, J. Ross, D. Sexton, R. Yap and J. Lukas, Process Design and Economics for the Conversion of Lignocellulosic Biomass to Hydrocarbon Fuels—Thermochemical Research Pathways with in Situ and Ex Situ Upgrading of Fast Pyrolysis Vapors, National Renewable Energy Laboratory, Golden, CO, NREL/TP-5100-62455, PNNL-23823, 2015, https://www.nrel.gov/docs/fy15osti/62455.pdf Search PubMed
.
-
K. Iisa; E. Christensen; K. Orton; C. Mukarakate; M. B. Griffin; K. Smith; K. Gaston; L. Tuxworth and M. J. Watson, Sustainable Aviation Fuel via Hydroprocessing of Catalytic Fast Pyrolysis Oil, TC Biomass, Denver, Colorado, 2022. https://www.gti.energy/wp-content/uploads/2022/05/08-tcbiomass2022-Presentation-Kristiina-Iisa.pdf Search PubMed
.
- M. B. Griffin, K. Iisa, H. Wang, A. Dutta, K. A. Orton, R. J. French, D. M. Santosa, N. Wilson, E. Christensen, C. Nash, K. M. van Allsburg, F. G. Baddour, D. A. Ruddy, E. C. D. Tan, H. Cai, C. Mukarakate and J. A. Schaidle, Driving towards cost-competitive biofuels through catalytic fast pyrolysis by rethinking catalyst selection and reactor configuration, Energy Environ. Sci., 2018, 11, 2901–2918 RSC
.
- D. M. Santosa, C. Zhu, F. A. Agblevor, B. Maddi, B. Q. Roberts, I. V. Kutnyakov, S. Lee and H. Wang, In Situ Catalytic Fast Pyrolysis Using Red Mud Catalyst: Impact of Catalytic Fast Pyrolysis Temperature and Biomass Feedstocks, ACS Sustainable Chem. Eng., 2020, 8, 5156–5164 CrossRef CAS
.
- G. Yildiz and W. Prins, Perspectives of Biomass Catalytic Fast Pyrolysis for Co-refining: Review and Correlation of Literature Data from Continuously Operated Setups, Energy Fuels, 2023, 37, 805–832 CrossRef CAS
.
-
J. T. Edwards, Jet Fuel Properties, Air Force Research Laboratory, Report AFRL-RQ-WP-TR-2020-0017, 2020 Search PubMed
.
- P. Vozka and G. Kilaz, A review of aviation turbine fuel chemical composition-property relations, Fuel, 2020, 268, 117391 CrossRef CAS
.
- J. Yang, Z. Xin, Q. S. He, K. Corscadden and H. Niu, An overview on performance characteristics of bio-jet fuels, Fuel, 2019, 237, 916–936 CrossRef CAS
.
- X. Shi, H. Li, Z. Song, X. Zhang and G. Liu, Quantitative composition-property relationship of aviation hydrocarbon fuel based on comprehensive two-dimensional gas chromatography with mass spectrometry and flame ionization detector, Fuel, 2017, 200, 395–406 CrossRef CAS
.
- P. Vozka, B. A. Modereger, A. C. Park, W. T. J. Zhang, R. W. Trice, H. I. Kenttamaa and G. Kilaz, Jet fuel density via GC × GC-FID, Fuel, 2019, 235, 1052–1060 CrossRef CAS
.
- Standard Test Method for Calculated Flash Point from Simulated Distillation Analysis of Distillate Fuels, ASTM D7215.
-
Standard Test Method for Estimation of Net Heat of Combustion of Aviation Fuels, ASTM D3338, ASTM International, Pennsylvania Search PubMed
.
-
X. Wang, T. Jia, L. Pan, Q. Liu, Y. Fang, J.-J. Zou and X. Zhang, Review on the Relationship between Liquid Aerospace Fuel Composition and Their Physicochemical Properties, Transactions of Tianjin University, 2021, vol. 27, pp. 87–109 Search PubMed
.
-
G. Flora, S. Kosir, L. Behnke, R. Stachler, J. Heyne, S. Zabarnick, et al., Properties Calculator and Optimization for Drop-In Alternative Jet Fuel Blends, 2019, conference: AIAA Scitech 2019 Forum, DOI:10.2514/6.2019-2368
.
-
Coordinating Research Council, Handbook of Aviation Fuel Properties, Defense Technical Information Center, 1983 Search PubMed
.
- R. C. Striebich, L. M. Shafer, R. K. Adams, Z. J. West, M. J. DeWitt and S. Zabarnick, Hydrocarbon group-type analysis of petroleum-derived and synthetic fuels using two-dimensional gas chromatography, Energy Fuels, 2014, 28, 5696–5706 CrossRef CAS
.
-
J. Gmehling
Chemical Thermodynamics for Process Simulation; Wiley-VCH: Weinheim, Germany, 2012 Search PubMed
.
- A. M. Reiter, T. Wallek, A. Pfennig and M. Zeymer, Surrogate Generation and Evaluation for Diesel Fuel, Energy Fuels, 2015, 29, 4181–4192 CrossRef CAS
.
- M. Dahmen and W. Marquardt, Model-Based Formulation of Biofuel Blends by Simultaneous Product and Pathway Design, Energy Fuels, 2017, 31, 4096–4121 CrossRef CAS
.
-
M. R. Riazi, Characterization and Properties of Petroleum Fractions, ASTM Stock Number: MNL50, ASTM, West Conshohocken, PA, 2005 Search PubMed
.
-
Aviation Fuels Technical Review, Chevron Products Company, 2007, https://www.chevron.com/-/media/chevron/operations/documents/aviation-tech-review.pdf, accessed April 12, 2022 Search PubMed.
-
M. R. Riazi, API Databook, 5th edn, procedure 2B7.1, 1986 Search PubMed
.
- Standard Test Method for Distillation of Petroleum Products and Liquid Fuels at Atmospheric Pressure, ASTM D86.
- Standard Test Method for Flash Point by Tag Closed Cup Tester, ASTM D56.
- Standard Test Methods for Flash Point by Pensky-Martens Closed Cup Tester, ASTM D93.
-
Standard Test Method for Boiling Range Distribution of Petroleum Fractions by Gas Chromatography, ASTM D2887-9a, ASTM International, Pennsylvania Search PubMed.
- P. Vozka, P. Šimáček and G. Kilaz, Impact of HEFA Feedstocks on Fuel Composition and Properties in Blends with Jet A, Energy Fuels, 2018, 32, 11595–11606 CrossRef CAS
.
- P. Vozka, D. Vrtiška, P. Šimaček and G. Kilaz, Impact of Alternative Fuel Blending Components on Fuel Composition and Properties in Blends with Jet A, Energy Fuels, 2019, 33, 3275–3289 CrossRef CAS
.
- J. A. Cramer, M. H. Hammond, K. M. Myers, T. N. Loegel and R. E. Morris, Novel data abstraction strategy utilizing gas chromatography-mass spectrometry data for fuel property modeling, Energy Fuels, 2014, 28, 1781–1791 CrossRef CAS
.
- K. J. Johnson, R. E. Morris and S. L. Rose-Pehrsson, Evaluating the predictive powers of spectroscopy and chromatography for fuel quality assessment, Energy Fuels, 2006, 20, 727–733 CrossRef CAS
.
- G. Liu, L. Wang, H. Qu, H. Shen, X. Zhang and S. Zhang,
et al., Artificial neural network approaches on composition-property relationships of jet fuels based on GC-MS, Fuel, 2007, 86, 2551–2559 CrossRef CAS
.
- S. Blakey, C. W. Wilson and M. Farmery,
et al, Fuel effects on range versus payload for modern jet aircraft, Aeronaut. J., 2011, 115, 627–634 CrossRef
.
- C. J. Chuck and J. Donnelly, The compatibility of potential bioderived fuels with Jet A-1 aviation kerosene, Appl. Energy, 2014, 118, 83–91 CrossRef CAS
.
- Standard Test Method for Heat of Combustion of Liquid Hydrocarbon Fuels by Bomb Calorimeter (Precision Method), ASTM D4809.
-
Standard Test Method for Freezing Point of Aviation Fuels, ASTM D2386-19 (2019), ASTM International, Pennsylvania Search PubMed.
-
FAA Aviation Rulemaking Advisory Committee, Fuel Properties – Effect on Aircraft and Infrastructure, FAA, 1998 Search PubMed
.
- R. L. J. Coetzer, T. S. Joubert and C. L Viljoen,
et al., Response surface models for synthetic jet fuel properties, Appl. Petrochem. Res., 2018, 8, 39–53 CrossRef CAS
.
- E. E. Elmalik, B. Raza and S. Warrag,
et al., Role of hydrocarbon building blocks on gas-to-liquid derived synthetic jet fuel characteristics, Ind. Eng. Chem. Res., 2014, 53, 1856–1865 CrossRef CAS
.
- P. J. Han, G. K. Nie and J. J. Xie,
et al., Synthesis of high-density biofuel with excellent low-temperature properties from lignocellulose-derived feedstock, Fuel Process. Technol., 2017, 163, 45–50 CrossRef CAS
.
- G. K. Nie, X. W. Zhang and L. Pan,
et al., One-pot production of branched decalins as high-density jet fuel from monocyclic alkanes and alcohols, Chem. Eng. Sci., 2018, 180, 64–69 CrossRef CAS
.
- T. D. Hong, T. H. Soerawidjaja and I. K. Reksowardojo,
et al., A study on developing aviation biofuel for the tropics: production process: experimental and theoretical evaluation of their blends with fossil kerosene, Chem. Eng. Process., 2013, 74, 124–130 CrossRef CAS
.
- I. A. Al-Nuaimi, M. Bohra and M. Selam,
et al., Optimization of the aromatic/paraffinic composition of synthetic jet fuels, Chem. Eng. Technol., 2016, 39, 2217–2228 CrossRef CAS
.
- D. J. Cookson, C. P. Lloyd and B. E. Smith, Investigation of the chemical basis of kerosene (jet fuel) specification properties, Energy Fuels, 1987, 1, 438–447 CrossRef CAS
.
- D. J. Cookson and B. E. Smith, Calculation of jet and diesel fuel properties using carbon-13 NMR spectroscopy, Energy Fuels, 1990, 4, 152–156 CrossRef CAS
.
- D. J. Cookson, P. Iliopoulos and B. E. Smith, Composition-property relations for jet and diesel fuels of variable boiling range, Fuel, 1995, 74, 70–78 CrossRef CAS
.
- D. J. Cookson and B. E. Smith, Observed and predicted properties of jet and diesel fuels formulated from coal liquefaction and fischer-tropsch feedstocks, Energy Fuels, 1992, 6, 581–585 CrossRef CAS
.
- Standard Specification for Aviation Turbine Fuel Containing Synthesized Hydrocarbons, ASTM D7566.
-
K. Gaston, Pilot-scale CFP Commissioning: Creative Problem Solving and Lessons Learned, TC Biomassplus2019, Rosemont, Illinois, 2019, https://www.nrel.gov/docs/fy20osti/75187.pdf Search PubMed
.
-
Equilibrium Catalyst, Inc., https://equilibriumcatalyst.com/, website accessed March 7, 2023.
-
LECO Corporation, Pegasus® 4D GCxGC-MS (TOF), ChromaTOF software 4.51.6.0 optimized for Pegasus, 2020, https://www.leco.com/product/pegasus-bt-4d/, website accessed March 7, 2023 Search PubMed.
-
NIST Standard Reference Database 1A, Software Version: 2.2, NIST/EPA/NIH Mass Spectral Library, 2014 Search PubMed
.
- Standard Practice for Evaluation of New Aviation Turbine Fuels and Fuel Additives, ASTM D4054.
-
V. Diky, R. D. Chirico, M. Frenkel, A. Bazyleva, J. W. Magee, E. Paulechka, A. Kazakov, E. W. Lemmon, C. D. Muzny and A. Y. Smolyanitsky, NIST ThermoData Engine, NIST Standard Reference Database 103b, version 10.4.3, 2022, https://www.nist.gov/mml/acmd/trc/thermodata-engine/srd-nist-tde-103b Search PubMed.
- M. Frenkel, R. D. Chirico, V. Diky, X. Yan, Q. Dong and C. Muzny, ThermoData Engine (TDE): Software Implementation of the Dynamic Data Evaluation Concept, J. Chem. Inf. Model., 2005, 45, 816–838 CrossRef CAS PubMed
.
- V. Diky, C. D. Muzny, E. W. Lemmon, R. D. Chirico and M. Frenkel, ThermoData Engine (TDE): Software Implementation of the Dynamic Data Evaluation Concept. 2. Equations of State on Demand and Dynamic Updates over the Web, J. Chem. Inf. Model., 2007, 47, 1713–1725 CrossRef CAS PubMed
.
- V. Diky, R. D. Chirico, C. D. Muzny, A. F. Kazakov, K. Kroenlein, J. W. Magee, I. Abdulagatov and M. Frenkel, ThermoData Engine (TDE): Software Implementation of the Dynamic Data Evaluation Concept. 9. Extensible Thermodynamic Constraints for Pure Compounds and New Model Developments, J. Chem. Inf. Model., 2013, 53, 3418–3430 CrossRef CAS PubMed
.
- T. Yamada and R. D. Gunn, Saturated Liquid Molar Volumes. The Rackett Equation, J. Chem. Eng. Data, 1973, 18, 234–236 CrossRef CAS
.
-
J. D. Cox, D. D. Wagman and V. A. Medvedev, ed. CODATA Key Values for Thermodynamics, Hemisphere, New York, 1989 Search PubMed
.
- S. P. Verevkin, V. N. Emel’yanenko, V. Diky, C. D. Muzny, R. D. Chirico and M. Frenkel, New Group-Contribution Approach to Thermochemical Properties of Organic Compounds: Hydrocarbons and Oxygen-Containing Compounds, J. Phys. Chem. Ref. Data, 2013, 42, 033102 CrossRef
.
- E. Paulechka and A. Kazakov, Efficient Estimation of Formation Enthalpies for Closed-Shell Organic Compounds with Local Coupled-Cluster Methods, J. Chem. Theory Comput., 2018, 14, 5920–5932 CrossRef CAS PubMed
.
-
B. E. Poling, J. P. O'Connell and J. Prausnitz, The Properties of Gases and Liquids, McGraw-Hill, New York, 2001 Search PubMed
.
- K. G. Joback and R. C. Reid, Estimation of Pure-Component Properties from Group-Contributions, Chem. Eng. Commun., 1987, 57, 233–243 CrossRef CAS
.
- J. Marrero-Morejon and E. Pardillo-Fontdevila, Estimation of Pure Compound Properties Using Group-Interaction Contributions, AIChE J., 1999, 45, 615–621 CrossRef CAS
.
-
G. M. Wilson and L. V. Jasperson, Critical Constants Tc, Pc, Estimation Based on Zero, First, Second-Order Methods, AIChE Meeting, New Orleans, LA, 1996 Search PubMed
.
- A. Kazakov, C. D. Muzny, V. Diky, R. D. Chirico and M. Frenkel, Predictive correlations based on large experimental datasets: Critical constants for pure compounds, Fluid Phase Equilib., 2010, 298, 131–142 CrossRef CAS
.
- L. Constantinou and R. Gani, New Group-Contribution Method for Estimating Properties of Pure Compounds, AIChE J., 1994, 40, 16971710 CrossRef
.
- D. Ambrose and J. Walton, Vapor-Pressures up to their Critical Temperatures of Normal Alkanes and Alkanols, Pure Appl. Chem., 1989, 61, 1395–1403 CrossRef CAS
.
- Y. Nannoolal, J. Rarey, D. Ramjugernath and W. Cordes, Estimation of pure component properties: Part 1. Estimation of the normal boiling point of non-electrolyte organic compounds via group contributions and group interactions, Fluid Phase Equilib., 2004, 226, 45–63 CrossRef CAS
.
-
E. H. Leslie and J. C. Geniesse, in Flash Points of Saturated Vapors of Combustible Liquids, ed. E. W. Washburn, C. J. West, N. E. Dorsey, F. R. Bichowsky, A. Klemenc, International Critical Tables of Numerical Data, Physics, Chemistry, and Technology, National Research Council (U.S.), 1927, vol. 2, pp. 161–162 Search PubMed
.
- G. Liu, Corresponding author of Shi et al, Fuel, 2017, 200, 395–406 CrossRef
.
|
This journal is © The Royal Society of Chemistry 2023 |
Click here to see how this site uses Cookies. View our privacy policy here.