The relationship between error beliefs in chemistry and chemistry learning outcomes: a chain mediation model investigation
Received
16th May 2023
, Accepted 1st August 2023
First published on 16th August 2023
Abstract
Errors are natural elements of the learning process and provide a high potential to promote students’ learning outcomes. In recent years, there has been much research about learning from errors. However, we know little about the relationship between students’ error beliefs in chemistry and chemistry learning outcomes at present. Thus, the aim of this study was to explore the mechanisms of chemistry behavioral and cognitive engagements, adaptive reactions towards errors in chemistry and error beliefs in chemistry, and offer suggestions to the improvement of students’ chemistry learning outcomes. We assessed all variables in eight different schools in China (N = 1352 students, Grade 10) and used structural equation modelling (SEM) to check the direct and indirect relationships between four variables. Our findings revealed that (1) chemistry behavioral and cognitive engagements, adaptive reactions towards errors in chemistry and error beliefs in chemistry significantly positively predicted students’ chemistry learning outcomes; (2) both (a) adaptive reactions towards errors in chemistry and (b) chemistry behavioral and cognitive engagements acted as significant mediators between error beliefs in chemistry and chemistry learning outcomes; (3) the chain mediating effect of error beliefs in chemistry → adaptive reactions towards errors in chemistry → chemistry behavioral and cognitive engagements → chemistry learning outcomes was significant. Finally, we discussed the important findings, pointed out the educational implications, acknowledged our study's limitations and suggested directions for future study.
Introduction
Chemistry, as a fundamental natural science, is regarded as a compulsory introductory course in STEM discipline fields (Gonzalez and Paoloni, 2015). And according to the chemistry curriculum standard of senior high school (Ministry of Education, P. R. China, 2017), chemistry is closely related to economic development, social civilization and talent cultivation, beneficial to students’ core competencies and problem-solving abilities. Thus, promoting students’ chemistry learning is necessary and meaningful.
However, in the chemistry learning process, making errors is unavoidable. Making errors is beneficial for students’ learning, since it provides a chance for students to gain new knowledge and skills (Bray and Santagata, 2014). Previous studies have reported that, although making errors could increase students’ fear of errors and then have a negative impact on learning outcomes (Grassinger and Dresel, 2017; Soncini et al., 2022), students’ error beliefs provided reflective learning opportunities and potential for personal improvement (Tulis et al., 2018), positively affecting students’ learning outcomes (Käfer et al., 2018). To date, the research about error beliefs and learning outcomes focus on the mathematics/German/English domain (Tulis and Ainley, 2011; Tulis et al., 2018), and there is no evidence indicating that error beliefs in chemistry are related with students’ chemistry learning outcomes. For this reason, the initial purpose of the current research is to figure it out so that we can provide practical advice for improving students’ chemistry learning outcomes by changing their error beliefs.
In addition, according to relevant research, learning outcomes, behavioral and cognitive engagements, adaptive reactions towards errors and error beliefs have been demonstrated to be positively correlated in pairs (Tulis and Ainley, 2011; Steuer et al., 2013; Tulis et al., 2018; Soncini et al., 2022; Guo et al., 2023). Positive beliefs towards errors can stimulate the intrinsic learning motivation, which is contributing to adaptive reactions towards errors (Maehr and Zusho, 2009), and thereby regulate behavioral and cognitive engagements, achieving the purpose of promoting learning (Tulis et al., 2016). But there is no study testing all the relationships in a comprehensive model so far. Therefore, in this study we hypothesized and tested a chain-mediating model, in which error beliefs in chemistry are the independent variable, both (a) adaptive reactions towards errors in chemistry and (b) chemistry behavioral and cognitive engagements are the mediating variables, and students’ chemistry learning outcomes are the dependent variable. The second purpose is to explore whether adaptive reactions towards errors in chemistry or chemistry behavioral and cognitive engagements mediate the relationship between error beliefs in chemistry and chemistry learning outcomes, thus coming up with the related education interventions to promote students’ chemistry learning outcomes.
Error beliefs in chemistry and chemistry learning outcomes
The term of error beliefs (see Table 1), “namely students’ belief that it is possible to learn from errors”, was introduced by Soncini et al. (2022) for the first time. Before this, in Arenas et al.'s (2006) study, “a positive view of errors” was a sub-dimension of error orientation which includes attitudes to errors and ways of coping with errors (Rybowiak et al., 1999). And Tulis and Ainley (2011) used “orientation to learning from errors” to describe the positive attitudes towards errors and errors’ benefits of obtaining competence (Diener and Dweck, 1980). Then, Käfer et al. (2018) expressed error beliefs as “students’ perception of mistakes as useful for learning”, one aspect of handling mistakes (Spychiger et al., 2006; Heinze et al., 2012). In the same year, Tulis et al. (2018) proposed the definition of “positive beliefs about errors”, whose core content was that errors can provide learning opportunities. In this study, our definition of error beliefs in chemistry is “beliefs that it is possible to learn from errors in chemistry”, such as errors in learning chemical concepts, errors in writing chemical balanced equations, errors in solving chemical numerical problems and so on (Kousathana and Tsaparlis, 2002; Papaphotis and Tsaparlis, 2008; Naah and Sanger, 2012). One important characteristic of errors in chemistry is that the errors are generally more easily detectable than in other liberal art subjects.
Table 1 Definition of the terms
Term |
Definition |
Error beliefs |
Belief that it is possible to learn from errors |
Adaptive reactions towards errors |
Adaptive self-regulated learning triggered by error detection |
Adaptive affective-motivational reactions towards errors |
Emotion activation and motivation maintenance following errors |
Adaptive action reactions towards errors |
Behavior following errors |
Engagements |
Degree of contribution to activities |
Behavioral engagements |
Observable behavior of engagements |
Cognitive engagements |
Psychological investment in engagements |
Emotional engagements |
Feeling about engagements |
Error beliefs have been showed to affect learning outcomes theoretically and empirically. For one thing, based on the achievement goal theory (Nicholls, 1984; Dweck, 1986; Elliot, 1999; Maehr and Zusho, 2009; Lewis, 2018), individuals with mastery goals orientations show positive attitudes in the face of errors and failures, which can stimulate the intrinsic learning motivation and further promote learning performance. Thus, it could be expected that error beliefs have a positive impact on learning outcomes. For the other thing, several previous studies have already demonstrated their correlation. Arenas et al. (2006) explored whether the error orientation could predict performance in a management task, obtaining the result that a positive view of errors guaranteed better long-term performance. Later, Tulis and Ainley's (2011) research suggested that students who hold error beliefs were more likely to hold positive emotions when receiving feedback about errors, which exerted positive effects on students’ academic achievements for most conditions (Pekrun, 2006). And Käfer et al. (2018) found that students’ learning outcomes were better when they regarded errors to be useful in the learning progress.
The potential mediating effect of adaptive reactions towards errors in chemistry
Students’ reactions towards errors (see Table 1) are defined as the self-regulated learning triggered by error detection, including adaptive reaction pattern and maladaptive reaction pattern (Steuer et al., 2013; Soncini et al., 2022). Besides, there are differences in students’ adaptive reactions towards errors during the learning process. Dresel et al. (2013) have already defined two types of adaptive reactions towards errors: adaptive affective-motivational reactions towards errors and adaptive action reactions towards errors. The affective-motivational part includes activating emotions and maintaining motivation (Krohne et al., 2002; Baker and Berenbaum, 2007; Tulis et al., 2018; Soncini et al., 2022). While the action part is conceptualized as the behaviors following errors, such as analysing the errors, carrying out specific actions to overcome the errors and using appropriate meta-cognitive strategies to avoid the same errors (Grassinger and Dresel, 2017; Tulis et al., 2018). The differentiation of adaptive affective-motivational reactions towards errors and adaptive action reactions towards errors is rooted in the model of self-regulated learning (Boekaerts, 1996; Boekaerts et al., 2000; Kadioglu-Akbulut and Uzuntiryaki-Kondakci, 2021). In this research, we primarily study students’ adaptive reactions towards errors in chemistry.
Regarding the relationships between error beliefs, adaptive reactions towards errors and learning outcomes, as argued by the achievement goal theory (Nicholls, 1984; Dweck, 1986; Elliot, 1999; Maehr and Zusho, 2009; Lewis, 2018), under the orientations of mastery goals, those who perceive errors and failures as the source of knowledge tend to attribute their errors and failures to effort or strategy, show less negative emotion, and increase their effort when facing errors and failures (Tulis and Ainley, 2011; Steuer et al., 2013). Therefore, we can expect that error beliefs are positively related with adaptive reaction towards errors and there has already been much evidence of their relationship. In Tulis and Ainley's (2011) study, students’ affect and motivation following errors were investigated in relation to orientation to learning from errors, and they have found a positive relationship between them. Tulis et al. (2018) have demonstrated that positive error beliefs predicted students’ affective-motivational and action adaptive reactions towards errors in three different school subjects. And in line with Tulis et al. (2018), Soncini et al.'s (2022) bivariate correlation results showed that the positive association between error beliefs and adaptive reactions towards errors was significant. In 2016, Tulis et al. proposed an integrated model, “namely process model of individual reactions to and learning from errors”, which comprises the personal determinants, contextual conditions and situational processes. And all the elements benefit the process of learning from errors. This model suggests that the perception of errors is the antecedent of adaptive reactions towards errors, which can promote the learning process and outcomes through emotional and motivational regulation. This model provides a theoretical basis for the relationship between adaptive reactions towards errors and learning outcomes, which have been supported empirically by several studies too. Grassinger et al. (2018) investigated how adaptive reactions towards errors could affect students’ learning outcomes. The findings showed that affective-motivational reactions towards errors encouraged action reactions towards errors which further promoted students’ learning outcomes. The same result was found in Schrader and Grassinger’ (2021) article; they examined whether students’ adaptive reactions towards errors mediated the effect of attributional feedback on achievement emotions and performance. The results indicated that students’ affective-motivational adaptive reactions towards errors exhibited improved learning outcomes. Recently, Soncini et al. (2022) tested the relationship between adaptive reactions towards errors and learning outcomes again, and got the finding that students’ individual adaptive reactions towards errors mediated the effect of positive error climate on math grades.
The potential mediating effect of chemistry behavioral and cognitive engagements
Connell (1990) defined engagements as interactions of the individual with the context, which were amenable to environmental change. Later, in Lawson, M. A. and Lawson, H. A.'s (2013) article, engagements referred to the systems that included social and psychological elements, under the guidance of social-ecological analysis and social-cultural theory. Nowadays, engagements (see Table 1) are defined as the degree of contribution to activities, which are the necessary elements of activities (Huang et al., 2022). Engagements are a holistic concept, including a multifaceted construct: behavioral engagements, cognitive engagements and emotional engagements. Behavioral engagements are the observable behaviors of engagements, including academic involvement (such as attendance, participation, effort, and persistence), social involvement and extracurricular involvement. Cognitive engagements are defined as the psychological investments in engagements, including the willingness, perseverance and strategy toward tasks. Emotional engagements are the term with the meaning of feeling about engagements. Researchers use the term to describe positive and negative reactions to people and things (Fredricks et al., 2004; Finn and Zimmer, 2012; Ding et al., 2017; Smith and Alonso, 2020). Chemistry is a discipline that emphasizes practice (Ministry of Education, P. R. China, 2017), so students’ engagements in chemistry learning are essential. The definition of each type of engagement resonates strongly with the context of chemistry, and we focus on the chemistry behavioral and cognitive engagements in this article. Students’ chemistry behavioral and cognitive engagements refer to behavioral involvements (such as attendance, effort, and persistence) in the chemistry course (Reid et al., 2021), chemistry laboratory experiments (Smith and Alonso, 2020) and chemistry extracurricular activities (Yan, 2011), as well as psychological investment (such as willingness, cognitive strategy and meta-cognitive strategy) in chemistry tasks (Smith and Alonso, 2020).
Besides the definition of behavioral and cognitive engagements, the relationship between behavioral and cognitive engagements, error beliefs and learning outcomes should also be noted. On the one hand, as stated by Pekrun's (2006) control-value theory of achievement emotions, achievement emotions are defined as emotions pertaining to achievement-related activities, such as the frustration and anger when facing errors. Appraisals of the activities’ (including making errors) values can trigger achievement emotions, and achievement emotions are important proximal determinants of engagements. Accordingly, the conjecture that error beliefs have a positive impact on behavioral and cognitive engagements is reasonable. But little empirical evidence has been provided in prior research until now. Keith and Frese (2005) found that students who hold the beliefs that errors are beneficial tend to persevere when facing setbacks, and have better self-regulation of emotions and cognitions during skill acquisition (Tulis et al., 2018). Tulis and Ainley (2011) investigated students’ on-task emotions after success and failure experiences and have found that the students’ positive attitudes towards learning from errors predicted positive emotion reactions in the face of errors, which in turn were considered to be important for learning behavior (such as persistence, preference for challenge) (Hidi et al., 2004). On the other hand, there are qualitative differences in the intensity and duration of engagements. The increase in intensity and duration will make engagements get ideal results, so it is reasonable to assume that engagements contribute to improvements in outcomes (Fredricks et al., 2004). And there have been a large body of studies concerning engagements and learning outcomes up to now. Skinner and Pitzer (2012) have provided substantial support for considering engagements as robust predictors of learning, grades, and achievement test scores. Huang et al. (2022) found that the learning strategies fostered students’ learning engagements by analyzing quantitatively and qualitatively, which enhanced academic achievement. The study by Guo et al. (2023) explored the association between engagements and learning outcomes by making use of the longitudinal data across a lag of 2.5 years. And the results confirmed the important role of engagements in the process of college student learning. As for the sub-dimensions of engagements, Marks (2000) has examined the positive correlation between behavioral engagements and learning outcomes. In the same year, Boekaerts et al. (2000) got the result that cognitive engagements were crucial for better learning outcomes.
The chain mediating effect of (a) adaptive reactions towards errors in chemistry and (b) chemistry behavioral and cognitive engagements
As to the link between (a) adaptive reactions towards errors and (b) behavioral and cognitive engagements, it could be inferred from Tulis et al.'s (2016) learning from errors model. Adaptive reactions towards errors provide the basis for the emotional and motivational regulation, while the regulation can facilitate or impede persistent learning engagements, the use of appropriate metacognitions and cognitive activities. This is why we assume that (a) adaptive reactions towards errors and (b) behavioral and cognitive engagements are closely linked, which has been demonstrated in prior studies. In Dresel and Ziegler's (2002) research, they pointed out that adaptive action reactions towards errors were an important precondition for general cognitive and meta-cognitive aspects of the learning process (Steuer et al., 2013). And Dresel et al. (2013) have provided evidence for the beneficial effects of adaptive reactions towards errors on student's effort and self-regulated in the learning process (Tulis et al., 2018). In analogy, the results of Steuer et al.'s (2013) study, which were based on a questionnaire-study with 1116 students from sixth and seventh grade, underpinned that adaptive reactions were positive predictors of students’ behavioral and cognitive engagements. However, there existed scarce research about the correlation between (a) adaptive reactions towards errors and (b) behavioral and cognitive engagements from 2014 to now.
The present study
The existing research suggests that error beliefs can influence learning outcomes (Käfer et al., 2018). While the relationship between error beliefs and learning outcomes is domain-specific (Tulis et al., 2018), the relationship between error beliefs in chemistry and chemistry learning outcomes was not well supported by prior research. Therefore, we aim to investigate the connection between error beliefs in chemistry and chemistry learning outcomes at first.
Furthermore, the enhancement of error beliefs can improve adaptive reactions towards errors (Soncini et al., 2022), and adaptive reactions towards errors can influence learning outcomes positively (Schrader and Grassinger, 2021) So, we assume that adaptive reactions towards errors in chemistry act as a mediator between error beliefs in chemistry and chemistry learning outcomes. Meanwhile, error beliefs also have a positive impact on behavioral and cognitive engagements (Tulis and Ainley, 2011). And the more of the behavioral and cognitive engagements there are, the better the learning outcomes (Guo et al., 2023). So, we assume that chemistry behavioral and cognitive engagements mediate the connection between error beliefs in chemistry and chemistry learning outcomes too.
Finally, since both (a) adaptive reactions towards errors and (b) behavioral and cognitive engagements mediate the effect of error beliefs on learning outcomes, and adaptive reactions towards errors can be a positive indicator of behavioral and cognitive engagements (Steuer et al., 2013), we assume that error beliefs in chemistry affect chemistry learning outcomes through the chain mediating effect of (a) adaptive reactions towards errors in chemistry and (b) chemistry behavioral and cognitive engagements.
In conclusion, we propose the following four hypotheses (see Fig. 1):
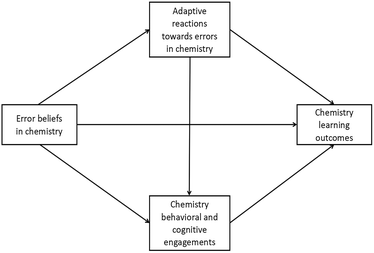 |
| Fig. 1 Hypothesized relationships among the variables. | |
Hypothesis 1: There is a positive relationship between error beliefs in chemistry and chemistry learning outcomes.
Hypothesis 2: Adaptive reactions towards errors in chemistry have a mediating effect between error beliefs in chemistry and chemistry learning outcomes.
Hypothesis 3: Chemistry behavioral and cognitive engagements act as a mediator between error beliefs in chemistry and chemistry learning outcomes.
Hypothesis 4: The (a) adaptive reactions towards errors in chemistry and (b) chemistry behavioral and cognitive engagements play a chain mediating role in the association between error beliefs in chemistry and chemistry learning outcomes.
Method
Participants
In this study, a total of 1352 students (47.49% males; 52.51% females) in Grade 10 were selected from China by the stratified purposive sampling method, with all students aged from 15 to 17 years (Mean = 15.81; SD = 0.55). Firstly, according to the main classification criteria of the comprehensive economic strength, Chinese cities are stratified into first, second, and third-tier cities and other cities (first-tier cities refer to cities with the highest economic strength) (D’Acci, 2021; Xu et al., 2022). We chose two schools from first, second, and third-tier cities and other cities, respectively, totaling eight schools. Secondly, in China, students of the same grades in each school are divided into three levels based on their academic performance: the high, medium, and low. In this study, we selected about 56 students from each level in each school. All participants have already studied chemistry for two years from Grade 9, so they have a certain understanding of chemistry. Furthermore, the reason for choosing the 10th-grade students as the participants is that these students should decide whether they will keep learning chemistry in future, and students’ chemistry learning outcomes (the dependent variable of this study) will affect their choices to a certain degree.
Survey administration
We conducted the study via an electronic questionnaire. The collection of the questionnaire was completed in eight different schools, taking a total of one week. Before collecting data, both the Academic Ethics Committee of Southwestern University in China, all appropriate authorities and students’ parents approved this research. In addition, the study's aim and the data's application were explained to all participants prior to the investigation beginning, and the personal information of the participants was promised to be kept strictly confidential. All students participated voluntarily, with no additional awards.
Survey instruments
We selected the scales which have demonstrated evidence of high validity and reliability and have been widely used in the previous studies as our measurement tools. The existing scales were in English, but our subjects were Chinese students. So, in order to make it easier for students to understand exactly what the question means, we translated all the English questions into Chinese guided by Brislin's (1970) back-translation method (Liu et al., 2016). First, three authors majoring in pedagogy who were native Chinese and good at English translated all the items independently, and then discussed together to determine the final version. Next, we translated the Chinese questions back to English by translation software and compared them with the original English ones. Finally, we carried out cognitive interviews with 20 10th-grade students, and adjusted the expressions of some items based on students’ feedback, aiming to ensure that the participants could correctly understand all the questions and complete them independently.
Error beliefs in chemistry.
We assessed error beliefs in chemistry using the measurement instrument developed by Tulis et al. (2018). Since error beliefs in chemistry is defined similarly to error beliefs in Mathematics/German/English, we modified the expressions of the items by simply changing the original “in Mathematics/German/English” to “in Chemistry”. The scale consisted of 5 items (e.g., “I can learn something from my errors in chemistry.”), and we scored them with a 5-point Likert scale ranging from 1 (strongly disagree) to 5 (strongly agree). Higher scores indicated higher error beliefs in chemistry.
Adaptive reactions towards errors in chemistry.
To measure adaptive reactions towards errors in chemistry, the questionnaire designed by Dresel et al. (Dresel et al., 2013; Soncini et al., 2022) was used, which was translated from German to English by Tulis et al. (2018). The adaptive reactions towards errors in mathematics and adaptive reactions towards errors in chemistry have similar definitions and characteristics, so we adapted the questionnaire developed to measure adaptive reactions towards errors in mathematics to our study in chemistry. The questionnaire covered two dimensions. The dimension of adaptive affective-motivational reactions included 6 items (e.g., “When I say something wrong in chemistry, then the class is ruined as far as I am concerned.”, reversed item), while the other dimension which was called adaptive action reactions consisted of 7 items (e.g., “When I make a mistake in chemistry, then I know where I will have to focus my efforts next time around.”). All the items were evaluated by a 5-point Likert scale ranging from 1 (strongly disagree) to 5 (strongly agree). Items reflected the less adaptive reactions towards errors in chemistry were reverse-scored, and we converted them before calculating. So, the higher the scores were, the more adaptive reactions towards errors in chemistry.
Chemistry behavioral and cognitive engagements.
Chemistry behavioral and cognitive engagements were measured with a scale designed and validated by Reeve and Tseng (Reeve and Tseng, 2011; Huang et al., 2022). The original scale comprised four aspects of engagements: agentic engagements, behavioral engagements, emotional engagements and cognitive engagements, from which we chose two dimensions: behavioral and cognitive engagements. Since engagements in different subjects have similar characteristics, we directly used the existing scale to measure student's behavioral and cognitive engagements in chemistry class. There were 5 items (e.g., “I listen carefully in class.”) in the behavioral engagements and 8 items (e.g., “When I’m working on my schoolwork, I stop once in a while and go over what I have been doing.”) in the cognitive engagements. The scale to measure the items was a 5-point Likert scale, which changed from 1 (strongly disagree) to 5 (strongly agree). In this case, higher scores reflected greater chemistry behavioral and cognitive engagements.
Chemistry learning outcomes.
In the Chinese education system, there are two summative evaluations for students during the school year: one is at the end of the first semester (January), and the other is at the end of the school year (June). The summative grades are the means of all the grades in various formal examinations during one semester or one school year. The contents in every test varied depending on students’ learning progress, and were selected from the senior high school textbooks of chemistry compulsory one and two (Wang and Bi, 2019a,b). In this study, students’ school year summative chemistry grades in Grade 10 (obtained in July 2022) were provided by their teachers. And we took them as the indicators of students’ chemistry learning outcomes.
Data analysis procedures and tools
In this study, we chose SPSS 25.0 and Mplus 8.3 to analyse data (Reyes et al., 2022; Pratt et al., 2023). The analysis procedures were as follows:
(1) Inspection of measuring tools. The measuring tools employed in this research were modified and translated from the well-known scales, so it's necessary to examine the reliability and validity of the questionnaires before the formal test.
The exploratory factor analysis (EFA) and the confirmatory factor analysis (CFA) were used to test the three scales. We randomly divided collected data in the pretest (n = 420) into two equal parts. At first, EFA was used in SPSS 25.0 for Part 1 (n = 210) to explore whether the scale's factor structure was consistent with the original predefined (Lee et al., 2008). Specifically, the number of factors extracted was determined based on principal component analysis, and the factor matrix was analysed using the maximum variance method. Then, we modified the questionnaires according to the results of EFA and implemented CFA on Part 2 (n = 210) using Mplus 8.3 to check the adapted scales’ reliability (Velayutham and Aldridge, 2012). Finally, the omega coefficient (ω) (McDonald, 1999) has been showed to be more applicable and more accurate than the alpha coefficient (α) (Cronbach, 1951) in the reliability test (Dunn et al., 2013; Komperda et al., 2018). Therefore, in the third stage, we used the omega coefficient (ω) in SPSS 25.0 as a more sensible index of internal consistency (McNeish, 2018; Granziera et al., 2022; Montes et al., 2022).
For the EFA result, the Kaiser–Meyer–Olkin (KMO) should be higher than 0.6, and the p value of Bartlett's sphericity test should be less than 0.05 (Bartlett, 1951; Guo et al., 2022). Items would be retained if their factor loading scores were more than 0.4 and cross-loading scores were less than 0.32 (Tabachnick and Fidell, 2001; Costello and Osborne, 2005; Wei et al., 2020). And the recommendations for the values of the fit index in CFA are: χ2/df < 5, RMSEA < 0.08, SRMR < 0.08, CFI > 0.9 and TLI > 0.9 (Browne and Cudeck, 1992; Marsh et al., 2004; Hair et al., 2006; Lee et al., 2008; Opperman et al., 2013). Finally, if the omega coefficient (ω) of each questionnaire is higher than 0.7, the scales’ reliability will be accepted (Green and Yang, 2015; Li et al., 2019).
(2) Validation of model hypotheses. Before testing the hypothesis model, the questionnaires were tested using a Harman single factor test to make sure that there doesn’t exist common method bias (Harris and Mossholder, 1996; Podsakoff et al., 2003; Aguirre-Urreta and Hu, 2019). If the first factor's explained variance was no more than 40% and less than half of the cumulative interpretation total variance, there was no common method bias (Mo et al., 2019). Then, since our model was a saturated model (Nurkhaidarov and Shochat, 2011; Steeger and Gondoli, 2013; Zhang et al., 2019), we didn’t report how well the complete model fitted the data, but only considered the path coefficient (Guo et al., 2022). The direct and mediating effects in the hypothesized model were tested using the structural equation model (SEM) with Mplus 8.3 (Zhang et al., 2022). To be more specific, since all the variables in this study were regarded as observable variables, we chose the path analysis to explore the associations among variables and the mediating effects (Jonnada and Fegley, 1974; Granziera et al., 2022). We used the mean score of each instrument in the correlation and mediation analysis process (Steuer et al., 2013; Soncini et al., 2022; Xiong et al., 2023). In a simple mediation model, the mediating effect can be either fully or partially mediated. If both the direct and the indirect effects are significant, the influence of the independent variable on the dependent variable is partially mediated; when the indirect effect is significant and the direct effect is non-significant, it means that the relationship between independent and dependent variables is fully mediated (Gonzalez and Paoloni, 2015).
As for the mediation analysis method, the bias-corrected percentile Bootstrap method has been demonstrated to be one of the best ways, because the Bootstrap method does not require normality hypothesis or large samples, and there is no need for standard error to estimate the mediating effect interval (Preacher and Hayes, 2008; Taylor et al., 2008; Fang et al., 2014). Therefore, in this study, we used the bias-corrected percentile Bootstrap method to sample the formal data 2000 times with a 95% confidence interval, with the aim of testing the significance of the mediating effect (Guo et al., 2022). If the 95% Bootstrap confidence interval (CI) doesn’t cover zero, the mediating effect is significant (Lau and Cheung, 2012; Fang et al., 2014; Hayes, 2015).
Suitability for measurement instruments
Error beliefs in chemistry.
After the EFA, the five items of the error beliefs in chemistry were extracted as two factors. And we found that one item had strong loadings on both factors, so we deleted it. The second round of EFA of the retained 4 items revealed a good EFA result: KMO = 0.815[>0.6]; Bartlett's test of sphericity showed χ2 = 449.261 (p < 0.001); the total explained variance of all items was 73.320%; the factor loadings of all items varied from 0.796 to 0.910 [>0.4]. Then we conducted a CFA to assess the measurement model. The results showed that the model fits well: χ2/df =1.300 [<5]; RMSEA = 0.038 [<0.08]; CFI = 0.999 [>0.9]; TLI = 0.996 [>0.9] and SRMR = 0.011 [<0.08]. The omega coefficient of the whole questionnaire was 0.876 [>0.7], indicating that the scale had a high level of reliability (see Table 2).
Table 2 Reliability and validity of the measurement tools
|
Factor loadings of items |
χ
2/df |
RMSEA |
CFI |
TLI |
SRMR |
ω
|
Error beliefs in chemistry |
0.796–0.910 |
1.300 |
0.038 |
0.999 |
0.996 |
0.011 |
0.876 |
Adaptive reactions towards errors in chemistry |
0.671–0.889 |
2.273 |
0.078 |
0.963 |
0.951 |
0.011 |
0.847 |
Chemistry behavioral and cognitive engagements |
0.753–0.898 |
2.262 |
0.078 |
0.958 |
0.949 |
0.044 |
0.925 |
Adaptive reactions towards errors in chemistry.
The scale could be divided into two dimensions as expected, and the EFA results extracted two factors. After three rounds, we eliminated three questions because they had significant loadings on two factors. Through the final EFA, we got the KMO coefficient of 0.884 [>0.6], the Bartlett's spherical test coefficient of 1186.112 (p < 0.001), the two-factor total explained variance of 69.430%, and the factor loadings of 0.671–0.889 [>0.4]. The CFA results provided a good-fit model: χ2/df = 2.273 [<5]; RMSEA = 0.078 [<0.08]; CFI = 0.963 [>0.9]; TLI = 0.951 [>0.9] and SRMR = 0.011 [<0.08]. The omega coefficient of the whole scale was 0.847 [>0.7], while the omega coefficients of the two dimensions were 0.861 and 0.911 [>0.7] (see Table 2).
Chemistry behavioral and cognitive engagements.
We used EFA to measure the components of chemistry behavioral and cognitive engagements. In the EFA model, there were two factors including five and eight items. The KMO coefficient was 0.924 [>0.6], the Bartlett's test of sphericity showed χ2 = 1951.708 (p < 0.001), the total explained variance of the two factors was 69.976%, and the factor loadings of all questions were from 0.753 to 0.898 [>0.4]. The CFA results were as follows: χ2/df = 2.262 [<5]; RMSEA = 0.078 [<0.08]; CFI = 0.958 [>0.9]; TLI = 0.949 [>0.9] and SRMR = 0.044 [<0.08], which supported that the measurement model of engagements was acceptable. The omega coefficient of the whole measurement tool was 0.925 [>0.7], and the two dimensions had the omega coefficients of 0.911 and 0.928 [>0.7] (see Table 2).
Results
Common method deviation test
The Harman single factor test's results showed that there was no common method bias in our measurement tools. Five factors’ initial eigenvalues were above one, and the first factor's explained variance was 37.391% [<40%]. In addition, the cumulative interpretation total variance was 69.327%, which is more than twice the explained variance of the first factor (Mo et al., 2019).
Descriptive statistics and bivariate correlations
The mean and standard deviation (SD) of each variable were displayed in Table 3. In addition, as presented in Table 3, the skewness of the variables in the present study ranged from −0.96 to 0.01, and the kurtosis was in the range of −0.56 to 2.21, all of which were within 3, indicating that the data were normally distributed (Kline, 2005). Finally, Table 3 also displays the bivariate correlations between variables in this study. The results suggested that the correlations between all variables were significant. To be specific, error beliefs in chemistry was significantly positively correlated with (a) adaptive reactions towards errors in chemistry (r = 0.462, p < 0.001) and (b) chemistry behavioral and cognitive engagements (r = 0.457, p < 0.001), as well as chemistry learning outcomes (r = 0.344, p < 0.001). The adaptive reactions towards errors in chemistry positively correlated with chemistry behavioral and cognitive engagements (r = 0.642, p < 0.001) and chemistry learning outcomes significantly (r = 0.379, p < 0.001). Furthermore, chemistry behavioral and cognitive engagements positively correlated with chemistry learning outcomes significantly too (r = 0.380, p < 0.001).
Table 3 Descriptive statistics and bivariate correlations
Variable |
Mean |
SD |
Min |
Max |
Skew |
Kurt |
1 |
2 |
3 |
Notes: ***p < 0.001. SD = standard deviation. 1, 2, 3 represent error beliefs in chemistry, adaptive reactions towards errors in chemistry, chemistry behavioral and cognitive engagements. |
Error beliefs in chemistry |
4.02 |
0.74 |
1.00 |
5.00 |
−0.96 |
2.21 |
— |
|
|
Adaptive reactions towards errors in chemistry |
3.80 |
0.65 |
1.30 |
5.00 |
−0.05 |
−0.19 |
0.462*** |
— |
|
Chemistry behavioral and cognitive engagements |
3.83 |
0.66 |
2.00 |
5.00 |
0.01 |
−0.36 |
0.457*** |
0.642*** |
— |
Chemistry learning outcomes |
90.19 |
32.62 |
0.00 |
150.00 |
−0.42 |
−0.56 |
0.344*** |
0.379*** |
0.380*** |
The chain mediating effects analyses
Given that prior research has found that the process of learning from errors might be different due to students’ gender (Soncini et al., 2022), the gender was entered as a covariate in the model, and the path analysis results are displayed in Fig. 2.
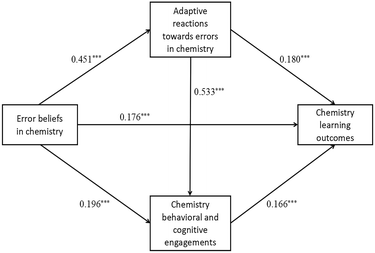 |
| Fig. 2 Significant effects from path model: standardized beta coefficients. Notes: ***p < 0.001. | |
Firstly, the results indicated that error beliefs in chemistry had a positive significant effect on adaptive reactions towards errors in chemistry (β = 0.451, p < 0.001). Then, after including chemistry behavioral and cognitive engagements into the regression equation, it showed that both error beliefs in chemistry and adaptive reactions towards errors in chemistry had a positive significant effect on chemistry behavioral and cognitive engagements (β = 0.196, p < 0.001; β = 0.533, p < 0.001). Finally, when we added chemistry learning outcomes to the regression equation, we got the result that all the chemistry behavioral and cognitive engagements, adaptive reactions towards errors in chemistry and error beliefs in chemistry had a positive significant effect on the chemistry learning outcomes (β = 0.166, p < 0.001; β = 0.180, p < 0.001; β = 0.176, p < 0.001).
Then we employed the Bootstrap method to examine the mediating effects, and the results are shown in Table 4. The 95% confidence interval of all the paths did not include 0, indicating significant mediating effects (Hayes, 2015). The total effect between error beliefs in chemistry and chemistry learning outcomes was 0.330 (95% CI was 0.283–0.377), while the direct effect between error beliefs in chemistry and chemistry learning outcomes was 0.176 (95% CI was 0.115–0.242), supporting hypothesis 1. Error beliefs in chemistry influence chemistry learning outcomes through three indirect pathways: the indirect effect of error beliefs in chemistry → adaptive reactions towards errors in chemistry → chemistry learning outcomes was 0.081 (95% CI was 0.052–0.117), supporting hypothesis 2; the indirect effect of error beliefs in chemistry → chemistry behavioral and cognitive engagements → chemistry learning outcomes was 0.033 (95% CI was 0.017–0.054), supporting hypothesis 3; the indirect effect of error beliefs in chemistry → adaptive reactions towards errors in chemistry → chemistry behavioral and cognitive engagements → chemistry learning outcomes was 0.040 (95% CI was 0.023–0.058), supporting hypothesis 4. The effects of direct and indirect paths were all significant, indicating that (a) adaptive reactions towards errors in chemistry and (b) chemistry behavioral and cognitive engagements only partially mediated the relationship between error beliefs in chemistry and chemistry learning outcomes.
Table 4 The indirect effect of (a) adaptive reactions towards errors in chemistry and (b) chemistry behavioral and cognitive engagements
Path |
Effect |
SE |
CI95 |
p
|
LL |
UL |
Note: SE = standard error, CI95 = 95% confidence interval, LL = lower level, UL = upper level. Confidence intervals were calculated using 2000 bootstraps, all variables used in this table have been standardized. |
Direct effect |
|
|
|
|
|
Error beliefs in chemistry → Chemistry learning outcomes |
0.176 |
0.032 |
0.115 |
0.242 |
P < 0.001 |
Indirect effects |
|
|
|
|
|
Error beliefs in chemistry → adaptive reactions towards errors in chemistry → Chemistry learning outcomes |
0.081 |
0.016 |
0.052 |
0.117 |
P < 0.001 |
Error beliefs in chemistry → chemistry behavioral and cognitive engagements → Chemistry learning outcomes |
0.033 |
0.009 |
0.017 |
0.054 |
P < 0.001 |
Error beliefs in chemistry → adaptive reactions towards errors in chemistry → chemistry behavioral and cognitive engagements → Chemistry learning outcomes |
0.040 |
0.009 |
0.023 |
0.058 |
P < 0.001 |
Total effect |
|
|
|
|
|
Error beliefs in chemistry → Chemistry learning outcomes |
0.330 |
0.025 |
0.283 |
0.377 |
P < 0.001 |
Discussion and conclusion
As previously stated, according to Tulis et al.'s (2016) model, making errors plays an important role in the learning process, and the process is affected by students’ error beliefs (Tulis et al., 2018). Therefore, to determine teaching interventions that could improve students’ chemistry learning outcomes by changing error beliefs in chemistry, this research investigated how students’ chemistry learning outcomes were associated with chemistry behavioral and cognitive engagements, adaptive reactions towards errors in chemistry and error beliefs in chemistry.
The relationship between error beliefs in chemistry and chemistry learning outcomes
The study's initial contribution is the discovery that error beliefs in chemistry have an impact on students’ chemistry learning outcomes. The research's findings verified that error beliefs in chemistry positively affected chemistry learning outcomes significantly (direct effect = 0.176). This finding verifies the relationship between error beliefs and students’ learning outcomes which has been demonstrated in some theories and previous studies. The achievement goal theory suggests that the positive attitudes towards errors can stimulate students’ learning outcomes (Maehr and Zusho, 2009). And several studies have demonstrated the positive correlation between error beliefs and students’ learning outcomes in recent years (Arenas et al., 2006; Tulis and Ainley, 2011; Käfer et al., 2018). But if we focus on the domains where previous research applied, we can find that the relationship between error beliefs in chemistry and chemistry learning outcomes is still an open question, which can be supplemented by our study.
The mediating roles of (a) adaptive reactions towards errors in chemistry and (b) chemistry behavioral and cognitive engagements
The second contribution of this research is the study of the mediating effects of (a) adaptive reactions towards errors in chemistry and (b) chemistry behavioral and cognitive engagements between error beliefs in chemistry and chemistry learning outcomes. The direct and indirect effects between error beliefs in chemistry and chemistry learning outcomes were all significant (direct effect = 0.176; total indirect effect = 0.154), indicating that both (a) adaptive reactions towards errors in chemistry and (b) chemistry behavioral and cognitive engagements acted as a partial mediator in the association between error beliefs in chemistry and chemistry learning outcomes.
In the present study, the positive connection between error beliefs in chemistry and students’ chemistry learning outcomes was partly mediated through adaptive reactions towards errors in chemistry (β = 0.081): error beliefs positively affected adaptive reactions towards errors (Tulis et al., 2018), while adaptive reactions towards errors had a strong influence on students’ learning outcomes (Grassinger et al., 2018). If we go to the origin of the relation between these variables, the above conclusions also have the theory root. According to the achievement goal theory, for students with strong error beliefs, errors provide information for one's own learning, accompanied by positive emotions and sufficient efforts (Maehr and Zusho, 2009; Steuer et al., 2013; Soncini et al., 2022). What's more, as stated by Tulis et al.'s (2016) theoretical model, adaptive reactions towards errors affect emotional regulation and motivational regulation and further have a positive impact on students’ learning outcomes in most instances. However, to the best of our knowledge, there has been no research on the combination of error beliefs, adaptive reactions towards errors and students’ learning outcomes, while our study investigates the mediating role of adaptive reactions towards errors in chemistry.
Additionally, the research suggested that students’ chemistry behavioral and cognitive engagements mediated the relationship between error beliefs in chemistry and chemistry learning outcomes too (β = 0.033). This showed that error beliefs in chemistry could positively affect chemistry behavioral and cognitive engagements, while the chemistry behavioral and cognitive engagements had a strong impact on students’ chemistry learning outcomes. The result is in accordance with the theories, according to Pekrun's (2006) control-value theory of achievement emotions, appraisals of the activities’ (including making errors) values are positively related with emotions, and positive emotions are important antecedents of engagements. Furthermore, Fredricks et al.'s (2004) theoretical analysis suggests that engagements are assumed to exert a broad influence on learning outcomes. And all the positive effects of error beliefs on behavioral and cognitive engagements (Soncini et al., 2022), behavioral and cognitive engagements on learning outcomes (Guo et al., 2023), error beliefs on learning outcomes (Käfer et al., 2018) have been demonstrated by previous empirical research. But there was no study on the integration of behavioral and cognitive engagements, error beliefs and learning outcomes.
The chain mediating effect of (a) adaptive reactions towards errors in chemistry and (b) chemistry behavioral and cognitive engagements
The third contribution of this study concerns the significant chain mediating effect of error beliefs in chemistry → adaptive reactions towards errors in chemistry → chemistry behavioral and cognitive engagements → chemistry learning outcomes (β = 0.040). This finding suggested that adaptive reactions towards errors in chemistry played a mediating role in the impact of error beliefs in chemistry on chemistry behavioral and cognitive engagements, whereas chemistry behavioral and cognitive engagements mediated the connection between adaptive reactions towards errors in chemistry and chemistry learning outcomes. As for the relationships between all study variables, previous studies have provided enough evidence. Tulis et al. (2018) focused on students’ error beliefs as an important element of adaptive reactions towards errors, and found the different influence of error beliefs on adaptive reactions in the subjects of mathematics, German and English. And in Käfer et al.'s (2018) study, they investigated students’ perception and reaction towards mistakes (errors), and got the result that students’ adaptive reactions towards mistakes (errors) have been shown to affect students’ behavioral and cognitive engagements in a favorable way. In addition, Kobicheva (2022) conducted online surveys to reveal the influence of the engagements on academic outcomes, comparing the levels of engagement and academic outcomes for males and females, and undergraduate and postgraduate students. Therefore, our study combined all four relative variables, and have indicated the existence of the chain mediating effect of (a) adaptive reactions towards errors and (b) behavioral and cognitive engagements in chemistry.
Implications for practice
The findings of current research show that error beliefs in chemistry have a positive impact on chemistry learning outcomes, so it's practicable to improve students’ chemistry learning outcomes by changing error beliefs. According to the behaviorist theories, errors have a negative impact on learning, and thus need to be avoided (Dormann and Frese, 1994; Santagata 2005). In Thorndike's (1901) method of trial and error, learning is a process of reducing errors and increasing success (Catania, 1999). Skinner's (1968) programmed learning represents that error avoidant training leads to better performance. In contrast, the constructivist method is based on Piaget's theory of cognitive development (Piaget, 1964), and have advocated student-centred learning methods, emphasizing students’ active participation in the construction of new knowledge (Paris and Byrnes, 1989; Zarotladou and Tsaparlis, 2000). From a constructivist perspective on learning, students’ mistakes (errors) are unavoidable and seen as the tools for learning (Gelman, 1994). Our results support the viewpoint of constructivism, holding the point that error beliefs are beneficial for students’ learning. In Oser and Spychiger's (2005) research, they also provided empirical evidence that errors are major sources for learning which led to improving and gaining knowledge. More specifically, learning from errors is associated with reflection on underlying misconceptions and self-explanations (Siegler, 2002; Van Lehn, et al., 2003). Consistent with earlier research, Tulis et al. (2018) explained that learning from errors requires cognitive and meta-cognitive processes. In a word, learning from errors is necessary and meaningful, so students should show positive beliefs towards errors. Meanwhile, we have noted that teachers’ attitudes towards errors and error-management behaviours will shape students’ perceptions of errors, and influence students’ error beliefs, finally changing students’ learning outcomes (Santagata, 2005; Tulis and Fulmer, 2013). Thus, as Error Management Training (EMT) argued, errors should be seen as learning opportunities and dealt with proactively (Grief and Keller, 1990; Frese et al., 1991). Chemistry teachers should show preferable attitudes towards students’ errors in class, allow students to make mistakes, not tie errors to evaluation, and provide appropriate ways of handling errors, to promote students’ learning outcomes finally.
Furthermore, the results of this study show that error beliefs in chemistry also have an indirect effect on chemistry learning outcomes through (a) adaptive reactions towards errors in chemistry and (b) chemistry behavioral and cognitive engagements. Both (a) adaptive reactions towards errors and (b) behavioral and cognitive engagements were demonstrated to be positively related with error climate (Steuer et al., 2013; Soncini et al., 2022). Steuer et al. (2013) defined a positive error climate as a classroom climate tolerating errors and regarding errors as indispensable elements of learning. Students’ perceptions of error climate depend on three aspects: “teachers’ attitudes and behaviours towards errors, classmates’ reaction and practical use of errors during learning activities” (Steuer et al., 2013; Soncini et al., 2022). At present, we focus on the part of classmates’ reactions, including “absence of negative classmate reactions and taking the error risk”. Positive classmate reactions (belonging to error climate) help students to show adaptive reactions towards errors (Grassinger et al., 2018) and can improve students’ behavioral and cognitive engagements (Steuer et al., 2013). At the same time, our study has suggested that students’ (a) adaptive reactions towards errors in chemistry and (b) chemistry behavioral and cognitive engagements mediate the relationship between error beliefs in chemistry and chemistry learning outcomes. As a result, classmate reactions towards others’ errors in chemistry class can be seen as an important part of the instructional interventions to use errors as essential components of study as well. To be more specific, chemistry teachers are supposed to conduct students to show error beliefs towards their classmates’ errors.
Finally, positive discipline, as a contemporary approach to upbringing, holds the belief that teachers need to understand, respect and encourage every student, as well as teaching students in accordance with their aptitude (Durrant, 2013; Zuković and Stojadinović, 2021). By implementing positive discipline in class, positive class climate (climate where there is full of acceptance, respect, and encouragement), problem solving and academic success are the outcomes (Bej, 2016). According to the approach of positive discipline, errors are natural elements of learning, providing opportunities for learning and improving (Charles and Senter, 2005). Following this idea, Nelsen et al. (2000) emphasize the solutions towards errors and believe that students can learn important skills by cooperating with classmates to find positive solutions to errors. Accordingly, in order to encourage students to learn from errors, managing the class with the principles of positive discipline can be seen as a meaningful education strategy. Chemistry teachers ought to respect all students, encourage students to learn from errors, provide skills and opportunities, and create positive and harmonious class climates, which will effectively raise the quality of education and contribute to students’ learning outcomes eventually.
Limitations and future directions
The current research was focused on the students in Grade 10, and mainly discussed the relationship between error beliefs in chemistry and chemistry learning outcomes. However, when broadening and applying our findings, some limitations ought to be noticed.
The first limitation is the cross-sectional design of the present study, by which only correlations and possible causal relationships among several variables can be stated (Rozgonjuk et al., 2020). Although the hypothesized causal relationships are theoretically grounded in the relevant literature, in order to confirm the causal relationships, future longitudinal studies will be essential. Besides, all variables in the study were investigated using self-reported data, which may lead to some method bias. So future studies need to take more objective measurement methods such as simultaneous surveys of students, parents, teachers and the combination of questionnaire, interview and daily observation. In addition, this study only tested the relationship between error beliefs and learning outcomes among tenth-grade students in some cities of China, limiting the results by the samples. In order to enhance the results’ universality and representativeness, future studies should survey students in different grades and cultural backgrounds. Finally, previous studies have verified that both error climates and error beliefs could influence students’ learning outcomes (Tulis et al., 2018; Soncini et al., 2022), while our study only explored the influence of error beliefs on chemistry learning outcomes. Thus, future studies can also research how error climates influence students’ chemistry learning outcomes, providing practical clues on the promotion of students’ chemistry learning outcomes by comprehensively considering both error climates and error beliefs.
Conflicts of interest
There are no conflicts to declare.
Acknowledgements
This study was financially supported by the Funds of the Ministry of Education of Humanities and Social Science Project (21YJA880018). We express our sincere thanks to the teachers and students who participated in this study.
References
- Aguirre-Urreta M. I. and Hu J., (2019), Detecting Common Method Bias: Performance of the Harman's Single-Factor Test, DATA BASE Adv. Inf. Syst., 50(2), 45–70.
- Arenas A., Tabernero C. and Briones E., (2006), Effects of goal orientation, error orientation and self-efficacy on performance in an uncertain situation, Soc. Behav. Pers., 34(5), 569–586.
- Baker J. P. and Berenbaum H., (2007), Emotional approach and problem-focused coping: a comparison of potentially adaptive strategies, Cognition Emotion, 21(1), 95–118.
- Bartlett M. S., (1951), The Effect of Standardization on a χ2 Approximation in Factor Analysis, Biometrika, 38(3/4), 337–344.
- Bej M., (2016), Social Skills and Programs of Positive Discipline in School Environment-A Literature Review, Mediterr. J. Soc. Sci., 7(2 S1), 84–87.
- Boekaerts M., (1996), Self-regulated learning at the junction of cognition and motivation, Eur. Psychol., 1(2), 100–112.
- Boekaerts M., Pintrich P. R. and Zeidner M., (2000), Handbook of self-regulation: Theory, research and applications, San Diego, CA: Academic Press.
- Bray W. and Santagata R., (2014), Making mathematical errors springboards for learning, in Karp K. and McDuffie A. R. (ed.), Annual perspectives in mathematics education: Using research to improve instruction, National Council of Teachers of Mathematics, pp. 239–248.
- Brislin R. W., (1970), Back-translation for cross-cultural research, J. Cross-Cult. Psychol., 1(3), 185–216.
- Browne M. W. and Cudeck R., (1992), Alternative Ways of Assessing Model Fit, Soc. Methods Res., 21(2), 230–258.
- Catania A. C., (1999), Thorndike's legacy: learning, selection, and the law of effect, J. Exp. Anal. Behav., 72(3), 425–428.
- Charles C. M. and Senter G. W., (2005), Building classroom discipline, Boston: Pearson.
- Connell J. P., (1990), Context, self, and action: a motivational analysis of self-system processes across the life-span, in Cicchetti D. (ed.), The self in transition: Infancy to childhood, Chicago: University of Chicago Press, pp. 61–97.
- Costello A. B. and Osborne J. W., (2005), Best practices in exploratory factor analysis: four recommendations for getting the most from your analysis, Practical Assessment, Res., Evaluation, 10(7), 1–9.
- Cronbach L. J., (1951), Coefficient alpha and the internal structure of tests, Psychometrika, 16(3), 297–334.
- D’Acci L. S., (2021), Preferring or Needing Cities? (Evolutionary) Psychology, Utility and Life Satisfaction of Urban Living, City, Culture Soc., 24, 100375.
- Diener C. and Dweck C., (1980), An analysis of learned helplessness: II. The processing of success, J. Pers. Soc. Psychol., 39(5), 940–952.
- Ding L., Kim C. and Orey M., (2017), Studies of student engagement in gamified online discussions, Comput. Educ., 115, 126–142.
- Dormann T. and Frese M., (1994), Error Training: Replication and the Function of Exploratory Behavior, Int. J. Human Comput. Int., 6(4), 365–372.
- Dresel M. and Ziegler A., (2002), Failure as an element of adaptive learning, Paper presented on the 8. Biennial Conference of the European Association for Research on Adolecence, Oxford, England.
- Dresel M., Schober B., Ziegler A., Grassinger R. and Steuer G., (2013), Affektiv-motivational adaptive undlandlungsadaptive Reaktionen auf Fehler im Lernprozess, Zeitschrift für Padagogische Psychologie, 27(4), 255–271.
- Dunn T. J., Baguley T. and Brunsden V., (2013), From alpha to omega: a practical solution to the pervasive problem of internal consistency estimation, Br. J. Psychol., 105(3), 399–412.
- Durrant J. E., (2013), Positive discipline in everyday parenting, Ottawa, ON: Save the Children Sweden.
- Dweck C., (1986), Motivational processes affecting learning, Am. Psychol., 41(10), 1040–1048.
- Elliot A. J., (1999), Approach and avoidance motivation and achievement goals, Educ. Psychol., 34(3), 169–189.
- Fang J., Wen Z. L., Zhang M. Q. and Sun P. Z., (2014), The analyses of multiple mediation effects based on structural equation modelling, J. Psychol. Sci., 37(7), 735–741.
- Finn J. D. and Zimmer K. S., (2012), Student engagement: What is it? Why does it matter? in Christenson, S. L., Reschly, A. L. and Wylie, C. (ed.), The Handbook of Research on Student Engagement, New York: Springer Science, pp. 97–131.
- Fredricks J. A., Blumenfeld P. C. and Paris A. H., (2004), School Engagement: Potential of the Concept, State of the Evidence, Rev. Educ. Res., 74(1), 59–109.
- Frese M., Brodbeck F., Heinbokel T., Mooser C., Schleiffenbaum E. and Thiemann P., (1991), Errors in training computer skills: on the positive function of errors, Human Computer Interaction, 6, 77–93.
- Gelman R., (1994), Constructivism and supporting environments, in Tirosh, D. (ed.), Human Development: Implicit and explicit knowledge: An educational approach, Norwood, NJ: Ablex Publishing Corporation, vol. 6.
- Gonzalez A. and Paoloni P., (2015), Perceived autonomy support, expectancy, value, metacognitive strategies and performance in chemistry: a structural equation model in undergraduates, Chem. Educ. Res. Pract., 16(3), 640–653.
- Granziera H., Liem G. A. D., Chong W. H., Martin A. J., Collie R. J., Bishop M. and Tynan L., (2022), The role of teachers' instrumental and emotional support in students' academic buoyancy, engagement, and academic skills: a study of high school and elementary school students in different national contexts, Learn. Instruction, 80, 101619.
- Grassinger R. and Dresel M., (2017), Who learns from errors on a class test? Antecedents and profiles of adaptive reactions to errors in a failure situation, Learn. Individual Differences, 53, 61–68.
- Grassinger R., Scheunpflug A., Zeinz H. and Dresel M., (2018), Smart is who makes lots of errors? The relevance of adaptive reactions to errors and a positive error climate for academic achievement, High Ability Studies, 29(1), 37–49.
- Green S. B. and Yang Y., (2015), Evaluation of Dimensionality in the Assessment of Internal Consistency Reliability: Coefficient Alpha and Omega Coefficients, Educ. Measurement: Issues Practice, 34(4), 14–20.
- Grief S. and Keller H., (1990), Innovation and the design of work and learning environments: the concept of explanation of exploration in human-computer interaction, in West M. A. and Farr J. L. (ed.), Innovation and creativity at work, New York: Wiley, pp. 231–249.
- Guo X. P., Deng W. B., Hu K. F., Lei W. N., Xiang S. Q., and Hu W. P., (2022), The effect of metacognition on students’ chemistry identity: the chain mediating role of chemistry learning burnout and chemistry learning flow, Chem. Educ. Res. Pract., 23(2), 408–421.
- Guo J. P., Lv S., Wang S. C., Wei S. M., Guo Y. R. and Yang L. Y., (2023), Reciprocal modeling of university students’ perceptions of the learning environment, engagement, and learning
outcome: a longitudinal study, Learn. Instruct., 83, 101692.
- Hair J. F., Black W. C., Babin B. J., Anderson R. E. and Tatham R. L., (2006), Multivariate data analysis, Pearson Education India. Handbook of research on science education, Mahwah, NJ: Lawrence Erlbaum Associates Publishers, pp. 125–167.
- Harris T. G. and Mossholder K. W., (1996), The affective implications of perceived congruence with culture dimensions during organizational transformation, J. Management, 22(4), 527–547.
- Hayes A. F., (2015), An Index and Test of Linear Moderated Mediation. Multivariate Behav. Res., 50(1), 1–22.
- Heinze A., Ufer S., Rach S. and Reiss K., (2012), The student perspective on dealing with errors in mathematics class, in Wuttke E. and Seifried J. (ed.), Research in vocational education: Learning from Errors at School and at Work, Opladen: Budrich, vol. 1, pp. 65–80.
- Hidi S., Renninger K. A. and Krapp A., (2004), Interest. A motivational construct that combines affective and cognitive functioning, in Dai D. and Sternberg R. (ed.), Motivation, emotion and cognition: Integrative perspectives on intellectual functioning and development, Hillsdale, NJ: Erlbaum, pp. 88–115.
- Huang Y. M., Silitonga L. M. and Wu T. T., (2022), Applying a business simulation game in a flipped classroom to enhance engagement, learning achievement, and higher-order thinking skills, Comput. Educ., 183, 104494.
- Jonnada R. K. and Fegley K. A., (1974), Path Analysis in Systems Science, IEEE Trans. Systems Man Cybernetics, SMC-4(5), 418–424.
- Kadioglu-Akbulut C. and Uzuntiryaki-Kondakci E., (2021), Implementation of self-regulatory instruction to promote students’ achievement and learning strategies in the high school chemistry classroom, Chem. Educ. Res. Pract., 22(1), 12–29.
- Käfer J., Kuger S., Klieme E. and Kunter M., (2018), The significance of dealing with mistakes for student achievement and motivation: results of doubly latent multilevel analyses, Eur., J. Psychol. Educ., 34(4), 731–753.
- Keith N. and Frese M., (2005), Self–regulation in error management training: emotion control and metacognition as mediators of performance effects, J. Appl. Psychol., 90(4), 677–691.
- Kline R. B., (2005), Methodology in the social sciences, Principles and Practice of Structural Equation Modeling, 2nd edn, New York: Guilford Press.
- Kobicheva A., (2022), Comparative study on students' engagement and academic outcomes in live online learning at university, Educ. Sci., 12, 371.
- Komperda R., Pentecost T. C. and Barbera J., (2018), Moving beyond Alpha: A Primer on Alternative Sources of Single-Administration Reliability Evidence for Quantitative Chemistry Education Research, J. Chem. Educ., 95(9), 1477–1491.
- Kousathana M. and Tsaparlis G., (2002), STUDENTS’ ERRORS IN SOLVING NUMERICAL CHEMICAL-EQUILIBRIUM PROBLEMS, Chem. Educ. Res. Pract., 3(1), 5–17.
- Krohne H. W., Pieper M., Knoll N. and Breimer N., (2002), The cognitive regulation of emotions: the role of success versus failure experience and coping dispositions, Cognition Emotion, 16(2), 217–243.
- Lau R. S. and Cheung G. W., (2012), Estimating and comparing specific mediation effects in complex latent variable models, Org. Res.Methods, 15(1), 3–16.
- Lawson M. A. and Lawson H. A., (2013), New Conceptual Frameworks for Student Engagement Research, Policy, and Practice, Rev. Educ. Res., 83(3), 432–479.
- Lee M. H., Johanson R. E. and Tsai C. C., (2008), Exploring Taiwanese high school students' conceptions of and approaches to learning science through a structural equation modeling analysis, Sci. Educ., 92(2), 191–220.
- Lewis S. E., (2018), Goal orientations of general chemistry students via the achievement goal framework, Chem. Educ. Res. Pract., 19(1), 199–212.
- Li R. X., Yao M. L., Liu H. R. and Chen Y. X., (2019), Chinese Parental Involvement and Adolescent Learning Motivation and Subjective Well-Being: more is not Always Better, J. Happiness Studies, 21(7), 2527–2555.
- Liu S. G., Hallinger P. and Feng D., (2016), Supporting the professional learning of teachers in China: Does principal leadership make a difference? Teach. Teacher Educ., 59, 79–91.
- Maehr M. L., and Zusho A., (2009), Achievement goal theory: the past, present, and future, in Wentzel K. R. and Wigfield A. (ed.), Handbook of motivation at school, New York, NY: Routledge, pp. 77–104.
- Marks H. M., (2000), Student engagement in instructional activity: patterns in the elementary, middle, and high school years, Am. Educ. Res. J., 37(1), 153–184.
- Marsh H. W., Hau K. T. and Wen, Z., (2004), In search of golden rules: comment on hypothesis-testing approaches to setting cutoff values for fit indexes and dangers in overgeneralizing Hu and Bentler's (1999) findings, Struct. Equation Modeling, 11(3), 320–341.
- McDonald R. P., (1999), Test theory: A unified treatment, Mahwah, NJ: Lawrence Erlbaum Associates.
- McNeish D., (2018), Thanks coefficient alpha, we’ll take it from here, Psychol. Methods, 23(3), 412–433.
- Ministry of Education, P. R. China, (2017), Chemistry curriculum standards of senior high school, Beijing: People's Education Press.
- Mo J., Wang C., Niu X., Jia X., Liu T. and Lin L., (2019), The relationship between impulsivity and self-injury in Chinese undergraduates: the chain mediating role of stressful life events and negative affect, J. Affective Disord., 256, 259–266.
- Montes L. H., Ferreira R. A. and Rodriguez C., (2022), The attitude to learning chemistry instrument (ALChI): linking sex, achievement, and attitudes, Chem. Educ. Res. Pract., 23(3), 686–697.
- Naah B. M. and Sanger M. J., (2012), Student misconceptions in writing balanced equations for dissolving ionic compounds in water, Chem. Educ. Res. Pract., 13(3), 186–194.
- Nelsen J., Lott L. and Glenn H., (2000), Positive Discipline in the classroom, 3rd edn, the United States: Harmony.
- Nicholls J., (1984), Achievement motivation: conceptions of ability, subjective experience, task choice, and performance, Psychol. Rev., 91, 328–346.
- Nurkhaidarov E. S. and Shochat E., (2011), Automorphisms of Saturated and Boundedly Saturated Models of Arithmetic, Notre Dame J. Formal Logic, 52(3), 315–329.
- Opperman E. A., Benson L. E. and Milhausen R. R., (2013), Confirmatory factor analysis of the female sexual function index, J. Sex. Res., 50(1), 29–36.
- Oser F. and Spychiger M., (2005), Lernen ist schmerzhaft-Zur Theorie des Negativen Wissens und zur Praxis der Fehlerkultur [Learning is painful-On the theory of negative knowledge and a practice and error culture], Weinheim: Beltz DOI:10.1080/03057240600874687.
- Papaphotis G. and Tsaparlis G., (2008), Conceptual versus algorithmic learning in high school chemistry: the case of basic quantum chemical concepts Part 2. Students' common errors, misconceptions and difficulties in understanding, Chem. Educ. Res. Pract., 9(4), 332–340.
- Paris S. G. and Byrnes J., (1989), The constructivist approach to self-regulation and learning in the classroom, in Zimmerman B. J. and Schunk D. H. (ed.), Self-regulated learning and academic achievement: theory research and practice, Springer-Verlag New York Inc, pp. 169–200.
- Pekrun R. (2006), The Control-Value Theory of Achievement Emotions: Assumptions, Corollaries, and Implications for Educational Research and Practice, Educ. Psychol. Rev., 18(4), 315–341.
- Piaget J., (1964), Development and learning, J. Res. Sci. Teach., 2, 176–186.
- Podsakoff P. M., Mackenzie S. B., Lee J. Y. and Podsakoff N. P., (2003), Common method biases in behavioral research: a critical review of the literature and recommended remedies, J. Appl. Psychol., 88(5), 879–903.
- Pratt J. M., Stewart J. L., Reisner B. A., Bentley A. K., Lin S., Smith S. R. and Raker J. R., (2023), Measuring student motivation in foundation-level inorganic chemistry courses: a multi-institution study, Chem. Educ. Res. Pract., 24(1), 143–160.
- Preacher K. J. and Hayes A. F., (2008), Asymptotic and resampling strategies for assessing and comparing indirect effects in multiple mediator models, Behav. Res. Methods, 40(3), 879–891.
- Reeve J. and Tseng C. M., (2011), Agency as a fourth aspect of students’ engagement during learning activities, Contemp. Educ. Psychol., 36(4), 257–267.
- Reid J. W., Gunes Z. D. K., Fateh S., Fatima A., Macrie-Shuck M., Nennig H. T., Quintanilla F., States N. E., Syed A., Cole R., Rushton G. T., Shah L. and Talanquer V., (2021), Investigating patterns of student engagement during collaborative activities in undergraduate chemistry courses, Chem. Educ. Res. Pract., 23(1), 173–188.
- Reyes C. T., Lawrie G. A., Thompson C. D. and Kyne S. H., (2022), “Every little thing that could possibly be provided helps”: analysis of online first-year chemistry resources using the universal design for learning framework, Chem. Educ. Res. Pract., 23(2), 385–407.
- Rozgonjuk D., Kraav T., Mikkor K., Orav-Puurand K. and Tht K., (2020), Mathematics anxiety among STEM and social sciences students: the roles of mathematics self-efficacy, and deep and surface approach to learning, Int. J. STEM Educ., 7(1), 46.
- Rybowiak V., Garst H., Frese M. and Batinic B., (1999), Error Orientation Questionnaire (EOQ): reliability, validity, and different language equivalence, J. Org. Behav., 20(4), 527–547.
- Santagata R., (2005), Practices and beliefs in mistake-handling activities: a video study of Italian and US mathematics lessons, Teach. Teacher Educ., 21(5), 491–508.
- Schrader C. and Grassinger R., (2021), Tell me that I can do it better. The effect of attributional feedback from a learning technology on achievement emotions and performance and the moderating role of individual adaptive reactions to errors, Comput. Educ., 161, 104028.
- Siegler R. S., (2002), Microgenetic studies of self-explanation, in Granott N. and Parziale J. (ed.), Microdevelopment. transition processes in development and learning, Cambridge: Cambridge University Press, pp. 31–58.
- Skinner B. F., (1968), The technology of teaching, New York: Appleton-Century-Crofts.
- Skinner E. A. and Pitzer J. R., (2012), Developmental dynamics of student engagement, coping, and everyday resilience, in Christenson S. L., Reschly A. L. and Wylie, C. (ed.), The Handbook of Research on Student Engagement, New York: Springer Science, pp. 21–44.
- Smith K. C. and Alonso V., (2020), Measuring student engagement in the undergraduate general chemistry laboratory, Chem. Educ. Res. Pract., 21(1), 399–411.
- Soncini A., Visintin E. P., Matteucci M. C., Tomasetto C. and Butera F., (2022), Positive error climate promotes learning outcomes through students’ adaptive reactions towards errors, Learn. Instruct., 80, 101627.
- Spychiger M. B., Kuster R. and Oser F., (2006), Dimensionen von Fehlerkultur in der Schule und deren Messung: Der Schülerfragebogen zur Fehlerkultur im Unterricht für Mittel-und Oberstufe [Dimensions of Mistake Culture in School and their Measurement: The Student Questionnaire on Mistake Culture in Secondary School Classes], Schweizerische Zeitschrift für Bildungswissenschaften, 28(1), 87–110.
- Steeger C. M. and Gondoli D. M., (2013), Mother-adolescent conflict as a mediator between adolescent problem behaviors and maternal psychological control, Dev. Psychol., 49(4), 804–814.
- Steuer G., Rosentritt-Brunn G. and Dresel M., (2013), Dealing with errors in mathematicss classrooms: structure and relevance of perceived error climate, Contemp. Educ. Psychol., 38(3), 196–210.
- Tabachnick B. G. and Fidell L. S., (2001), Using Multivariate Statistics, Boston: Allyn and Bacon.
- Taylor A. B., MacKinnon D. P. and Tein J. Y., (2008), Tests of the three-path mediated effect, Org. Res. Methods, 11(2), 241–269.
- Thorndike E. L., (1901), The human nature club: An introduction to the study of mental life, 2nd edn, New York: Macmillan, pp. 38–39.
- Tulis M. and Ainley M., (2011), Interest, enjoyment and pride after failure experiences? Predictors of students’ state-emotions after success and failure during learning mathematics, Educ. Psychol., 31(7), 779–807.
- Tulis M. and Fulmer S. M., (2013), Students’ motivational and emotional experiences and their relationship to persistence during academic challenge in mathematics and reading, Learn. Individual Differences, 27, 35–46.
- Tulis M., Steuer G. and Dresel M., (2016), Learning from errors: a model of individual processes, Front. Learn. Res., 4(2), 12–26.
- Tulis M., Steuer G. and Dresel M., (2018), Positive beliefs about errors as an important element of adaptive individual dealing with errors during academic learning, Educ. Psychol., 38(2), 139–158.
- Van Lehn K., Siler S., Murray C., Yamauchi T. and Baggett W., (2003), Why do only some events cause learning during human tutoring? Cognition Instruct., 21(3), 209–249.
- Velayutham S. and Aldridge J. M., (2012), Influence of psychosocial classroom environment on students’ motivation and self-regulation in science learning: a structural equation modeling approach, Res. Sci. Educ., 43(2), 507–527.
- Wang J. and Bi H. L., (2019a), Senior high school textbook of chemistry compulsory one, Beijing: People's Education Press.
- Wang J. and Bi H. L., (2019b), Senior high school textbook of chemistry compulsory two, Beijing: People's Education Press.
- Wei J., Treagust D. F., Mocerino M., Vishnumolakala V. R., Zadnik M. G., Lucey A. D. and Lindsay E. D., (2020), Design and Validation of an Instrument to Measure Students’ Interactions and Satisfaction in Undergraduate Chemistry Laboratory Classes. Res. Sci. Educ., 51(4), 1039–1053.
- Xiong W., Huang M. J. and Chen S. Y., (2023), The effect of tourist-generated content characteristics on travel intention: the chain mediation of social comparison emotions, Tourism Tribune, 38(2), 81–91.
- Xu L. L., Wang L. and Nygaard C., (2022), Locational decisions and subjective well-being: an empirical study of Chinese urban migrants, Appl. Econ., 54(27),
3180–3195.
- Yan L., (2011), Engaging students in chemistry, Chemistry, Teach. Learn., 9, 44–47.
- Zarotladou E. and Tsaparlis G., (2000), Teaching Lower-Secondary Chemistry with a Piagetian Constructivist and an Ausbelian Meaningful-Receptive Method: A Longitudinal Comparison, Chem. Educ. Res. Pract., 1(1), 37–50.
- Zhang L., Xue X. J. and Zhao J. X., (2019), Discrimination perception, Depression, and Academic Achievement in Rural Left-behind Children: A Longitudinal Mediation Model, J. Psychol. Sci., 42(3),584–590.
- Zhang L., Fu B. B., Xu Y., Zhang Q., Peng S. Z. and Tan X. D., (2022), Association Between Social Support and Perceived Stress: A Cross-Sectional Study on Staffs of the Epidemic Prevention During the Covid-19 Epidemic in China, Front. Public Health, 10, 844139.
- Zuković S. and Stojadinović D., (2021), Applying positive discipline in school and adolescents’ self-esteem, International Journal of Cognitive Research in Science, Eng. Educ. (IJCRSEE), 9(1), 1–11.
|
This journal is © The Royal Society of Chemistry 2023 |