DOI:
10.1039/D3RA05606F
(Review Article)
RSC Adv., 2023,
13, 31795-31810
Recent progress in the genotyping of bovine tuberculosis and its rapid diagnosis via nanoparticle-based electrochemical biosensors
Received
17th August 2023
, Accepted 16th October 2023
First published on 30th October 2023
Abstract
Bovine tuberculosis (bTB) is considered a worldwide infectious zoonotic disease. Mycobacterium bovis causes bTB disease. It is one of the Mycobacterium tuberculosis complex (MTBC) members. MTBC is a clonal complex of close relatives with approximately 99.95% similarity. M. bovis is a spillover pathogen that can transmit from animals to humans and rarely from humans to animals with contact. Genotyping techniques are important to discriminate and differentiate between MTBC species. Spoligotyping and mycobacterial interspersed repetitive unit-variable number tandem repeat (MIRU-VNTR) are widely used but they have some limitations. As an alternative, whole genome sequencing approaches have been utilized due to their high-resolution power. They are employed in typing M. bovis and explain the evolutionary and phylogenetic relationships between isolates. The control of bTB disease has attracted a large amount of attention. Rapid and proper diagnosis is necessary for monitoring the disease as an initial step for its control and treatment. Nanotechnology has a potential impact on the rapid diagnosis and treatment of bTB through the use of nanocarrier and metal nanoparticles (NPs). Special attention has been paid to voltammetric and impedimetric electrochemical strategies as facile, sensitive, and selective methods for the efficient detection of tuberculosis. The efficacy of these sensors is enhanced in the presence of NPs, which act as recognition and/or redox probes. Gold, silver, copper, cobalt, graphene, and magnetic NPs, as well as polypyrrole nanowires and multiwalled carbon nanotubes have been employed for detecting tuberculosis. Overall, NP-based electrochemical sensors represent a promising tool for the diagnosis of bTB.
Introduction
Tuberculosis (TB) is an important zoonotic disease of animals and humans.1 Despite advancements in its prevention and management, TB still ranks as one of the top infectious agents in terms of mortality and has a devastating impact on populations of bovine livestock and wildlife worldwide.2 A World Health Organization (WHO) study from 2022 reported new cases of nearly ten million and 1.6 million fatalities as a result of initial infection, reinfection, or re-activation of latent infection (Tuberculosis Deaths and Disease Increase during the COVID-19 Pandemic, n.d.), and the increasing incidence of antimicrobial resistance (AMR) is a hazard to public health.3 WHO indicated that there are eight countries showing two-thirds of the novel cases: China, Nigeria, India, Bangladesh, Indonesia, South Africa, Pakistan, and the Philippines. Additionally, TB incidence was declining globally by an accumulated decreasing rate of 11%, which is less than half of the anticipated 20% drop from 2015 to 2020 outlined in the previous TB policy. Eradicating tuberculosis by 2030 is one of the first health goals of the United Nations Sustainable Development Goals (SDGs) (Tuberculosis, n.d.). Furthermore, bovine TB (bTB) is notifiable disease that must be informed to the OIE (World Organization for Animal Health). Reporting of the bTB status from 188 different countries from January 2017 to June 2018 revealed that the presence of the disease in animals was 44% with prevalence increasing in Africa, America and some regions of Asia.4 Regardless of successful worldwide notification calls, the real effect of bTB in livestock is rarely adequately reported, particularly in wild animals and in nations with undeveloped control measures.5
bTB is caused by M. bovis and it is considered to spill-over between hosts.6 It usually spreads to people after close contact with diseased animals or the consumption of soiled, unpasteurized dairy products.7 The symptoms of the human disease of M. bovis, which is resistant to PZA,8 one of the first TB medications, are like those caused by M. tuberculosis.9 It has been assessed that M. bovis accounts for less than 1.5% of all human cases of TB in areas outside of Africa and almost 2.8% of all TB disease in Africa.10
Effective programs to eradicate and control bTB usually depend on testing and slaughtering, post mortem inspection actions and movement restrictions, and before that proper diagnosis.11 To understand TB transmission, bacterial phylogeny and evolution has been considerably enhanced with the invention and application of molecular genotyping approaches for Mycobacterium species. Genotyping of Mycobacterium tuberculosis complex (MTBC) species is considered common practice for epidemiological tracking, and the analysis of regional and global strain population dynamics are of significant importance to investigate the spread of extensively drug-resistant (XDR) and multidrug-resistant (MDR) bacteria.12 Several methods for conventional genotyping can be used to examine several classes of genetic markers and produce strain-specific banding patterns (IS6110 DNA fingerprint), bar code-like signals (spoligotyping), or numerical patterns (24 locus-MIRU-VNTR typing).13 Nevertheless, quick advancements in whole-genome sequencing (WGS) methods offer more thorough insights into the underlying molecular and epidemiological techniques and result in improved diagnostic and treatment tools.14
In the past, M. bovis genotyping methods have mostly relied on PCR-based assays to evaluate a few of the genetic markers.15 Whole genome sequencing (WGS) of M. bovis will eventually substitute some challenging analyses as the cost per genome continues to drop, while also allowing for the required high resolution analysis of outbreaks.16 These genetic techniques offer valuable insights into the causes of infection, the transmission routes, the geographic localization, and the preferred hosts, as well as the dynamics and risk factors of the disease,17 thus promoting the reduction or elimination of the infection.18,19 Hence, this review will illustrate different genotyping methods, and WGS application in the detection of genetic diversity, and tackle the dynamic transmission of the M. bovis pathogen. On the other hand, the diagnosis of tuberculosis is necessary as a preliminary step for its control and treatment. In this review, a great focus has been put on electrochemical sensors as a rapid tool for the diagnosis of tuberculosis.
Electrochemical sensors represent an effective and rapid tool for determining various organic, inorganic, and biological molecules, as well as microorganisms.20–22 Electrochemical sensors can be classified into various types such as potentiometric, voltammetric, impedimetric, amperometric, and electrogenerated chemiluminescence sensors.20,23 The sensing process relies on the redox behavior of the targeted analytes at surfaces of the electrodes. For superior sensitivity and selectivity, as well as lower detection limits, the electrodes are modified with electroactive materials such as polymers, ferrocenes, and nanoparticles (NPs).20 Herein, a great focus has been put on NPs, such as gold (Au), silver (Ag), copper (Cu), cobalt (Co), ferric oxide (Fe3O4), graphene, poly pyrrole (PPY), and multiwalled carbon nanotubes (MWCNTs), which have been used for the electrochemical detection of tuberculosis. Additionally, NPs as nanocarriers for the delivery of antituberculosis drugs are discussed.
A concise summary of MTBC genomics
For the accurate interpretation of genotyping and WGS data, understanding the genetic profile of M. bovis is required. It is involved in the Mycobacterium tuberculosis complex (MTBC), a group containing 11 species of bacteria with variation in virulence and host tropism.24 MTBC genomes are very similar with higher than 99.95% homology at the level of nucleotides. These genomes contain ribosomal RNA genes (rRNA), while horizontal gene transfer (HGT) and significant recombining actions are thought to be missing.25 The only evolution occurring in the Mycobacterium pathogen can be due to indels (small insertions and deletions), single nucleotide polymorphisms (SNPs), deletions of about 26 kbp, duplication of a few paralogous genes and shuffling of insertion sequences (IS).26–28 Differential hybridization arrays and physical mapping have been carried out on M. tuberculosis H37Rv, M. bovis ATCC 19210 and M. bovis BCG Pasteur strains. Fourteen evolutionarily large deletion regions known as “regions of difference” (RD1–14) were characterized that had different sizes and presence in the strains.29,30 Identification of RDs is regarded as the gold standard technique to distinguish between MTBC species.31 Consequently, the deletion of RD4 and RD9 of M. bovis and RD1 from M. bovis BCG strains were used in the precise differentiated from other MTBC members.32
The M. bovis genome is about 4.3 Mb in size, covering around 4216 genes, with one copy of (5S, 16S, and 23S) rRNA genes, and 45 tRNAs with high GC content representing 65%, which infers proper sequencing for WGS library creation.33 One of the biggest difficulties in analyzing WGS data is the high proportion of repeated elements in MTBC genomes, including those of M. bovis. These involve IS, integrases, two phage sequences, clustered regularly interspaced short palindromic repeats (CRISPR), proline proline glutamate (PPE) or proline glutamate (PE) family genes, and genes from the 13 × 1012 repeat family. In particular, the family gene of PE–PPE is reported in about 10% of MTBC genomes, and has been linked with TB pathogenicity.34,35 As most widely used sequencing machines produce reads shorter than repeats, repetitive region handling becomes a challenge in genomic investigations. Traditional genotyping methods have been developed over time and are based in part on some of these repetitive regions.36 Table 1 shows the characteristics of genotyping and WGS.
Table 1 Different characteristics of genotyping techniques and WGS
Method |
Principle |
Minimum DNA input |
Time |
Advantages |
Disadvantages |
Ref |
Time can also vary depending on the number of tested samples. Total DNA input for WGS depends on the chosen library. Run time is highly dependent on the chosen protocol. |
REA |
Using three different restriction enzymes (PvuII, BstEII, and Bcll) in total DNA of M. bovis |
High (4–6 μg) |
2 days |
- Applied for strain typing in M. bovis, |
- Too many bands that are hard to resolve |
37 and 79 |
- Needs M. bovis isolation |
- Technically challenging |
- Standardized techniques |
PFGE |
Digestion DNA with rare cutting enzymes and the use of agarose gel to separate by electrical pulses |
High (2 μg) |
7 days |
- Able to differentiate between MTBC species |
- Labor intensive |
40 and 41 |
- Low intra-specific discrimination |
- Intact DNA is needed for restriction enzyme treatment |
- It is complicated getting agarose plugs |
- Requires isolation of M. bovis |
- Not defined standard method |
- Long turn-over time |
IS6110-RFLP |
Restriction enzymes digest M. bovis whole DNA, then electrophoresis on agarose gel, and southern blot with IS6110 probes |
High (2–3 μg) |
3 to 4 days |
- Standardized methods |
- No discriminatory power for M. bovis |
44 and 54 |
- Labor intensive |
- Specified for M. tuberculosis typing, but not M. bovis |
- Technically demanding |
- Requires M. bovis isolation |
PGRS-RFLP |
Restriction enzymes digest M. bovis in whole DNA, then electrophoresis on agarose gel, and southern blot with PGRS probes |
High (2–3 μg) |
3 to 4 days |
- Standardized methods |
- Too many bands that are hard to resolve |
57 |
- Labor intensive |
- Used in typing M. bovis |
- Technically demanding |
Spoligotyping |
PCR dependent method detecting the existence of 43 distinctive short DNA sequences (or spacers) in the DR position |
Very low (10 fg) (even from extracted infected tissue) |
2 to 3 days (up to 40 samples) |
- Standardized procedures |
- Homoplasy |
59 and 69 |
- Extracted DNA can be directly used |
- Lower resolution power compared to REA and MIRU-VNTR |
- Automatization |
- High throughput |
- Labor intensive |
- Used in typing M. bovis |
MIRU-VNTR PCR |
PCR-based amplification of VNTR loci and visualization following gel electrophoresis, MIRU-VNTR PCR targets many genomic loci |
Very low (10 fg) (even from extracted infected tissue) |
1 to 2 days for a few samplesa |
- Standardized procedures |
- Variable discriminating power depending on the chosen loci |
59 and 69 |
- Extracted DNA can be directly used |
- Automatization |
- Homoplasy |
- High throughput |
- Used in typing M. bovis |
MST |
Based on an examination of many intergenic regions from a single sequence |
2 μL target DNA |
Fast |
- Widely use in M. tuberculosis |
- Limited in M. bovis |
78 and 80 |
- Sequencing and accessibility of analyzed sequences in public databases |
- Infers evolutionary relationships and correlate geographical location |
WGS |
DNA is digested or sheared, and all fragments are simultaneously sequenced |
Low to highb (1 ng to 1 μg) |
2 to 4 daysc (∼24 to 96 samples) |
- Standardized techniques for sequencing |
- Requires M. bovis isolation |
70 |
- High output |
- Reproducible |
- High resolution |
- Detects spoligotypes and MIRU-VNTR patterns |
- Used in typing M. bovis |
- Repeats are hard to resolve using short reads |
- Used for evolutionary research of M. bovis |
- Technically demanding |
- Needs more advanced bioinformatic skills |
- Data analysis is needed |
Conventional genotyping techniques of M. bovis
The conventional genotyping techniques of M. bovis intervention include restriction endonuclease analysis and pulsed-field gel electrophoresis, IS6110-RFLP, PGRS-RFLP, spoligotyping, variable number tandem repeat (VNTR), and multispacer sequence typing (Fig. 1).
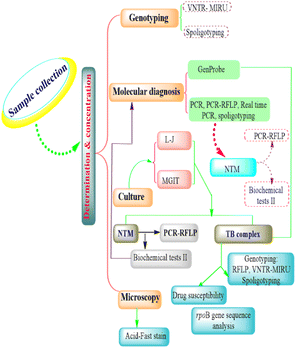 |
| Fig. 1 Flow chart showing conventional genotyping techniques of M. bovis intervention. | |
Restriction endonuclease analysis and pulsed-field gel electrophoresis
The initial approach to M. bovis intraspecific typing, restriction endonuclease analysis (REA), involves the rapid digestion of large quantities of whole DNA from M. bovis samples using three separate enzymes (PvuII, BstEII, and Bcll), followed by the visualization of band patterns on agarose gels.37 In spite of its use in molecular epidemiology investigations, it seems to have significant technical drawbacks because it utilizes too many little, difficult-to-reconstruct DNA fragments.38 Recently, it has been no longer used other than as a reference in New Zealand, where it was created, and it was last employed for normal M. bovis typing in 2011.39
The third-generation molecular technique for bacteria typing, known as pulsed-field gel electrophoresis (PFGE), eventually emerged for M. tuberculosis as well as the other MTBC species. Its resolution patterns were better than REA (fewer and bigger bands).40 Nonetheless, PFGE had two major drawbacks: (1) the lipid rich cell wall of the mycobacterium hinders the PFGE's lytic enzymes from working as intended, impairing the utilization of the agarose plugs;41 (2) comparative investigations revealed that the PFGE of M. tuberculosis strains had a low discrimination power versus later developed genotyping methods. PFGE has certain inherent disadvantages, like the fact that it is time consuming and labor intensive.42,43
Considering the failure of REA and PFGE to distinguish between M. bovis and M. tuberculosis strains, seeking polymorphic and stable genetic markers permitted the validation of more accurate genotyping methods. Now, there are genetic markers applied commonly including the direct repeat (DR) region, IS6110 (for M. tuberculosis), the variable number tandem repeats (VNTR) sequences and the poly (GC) rich sequences (PGRS). Each marker has its typing style that goes with it.
IS6110-RFLP
IS6110 is a particular insertion sequence (1358 bp) of the MTBC, and variations in its position and number of copies are what differentiate between isolates.44 This insertion sequence can serve like a mobile promoter, induce structural variation (insertion, deletion or inversion), and encourage gene silencing.45,46 In a case in Spain, M. bovis initiated a significant multidrug resistant tuberculosis (MDR TB) outburst in humans linked to gene overexpression and increased virulence by IS6110 in phoP promoter.47 Moreover, the association between the number of copies of the M. bovis strain and the high prevalence of bTB resulted from genomic alterations driven from IS6110 transposition.48 Also, the high IS6110 content in the M. tuberculosis Beijing lineage enhanced its virulence, antibiotic resistance, and environmental adaptability.49,50
For identifying IS6110 in the M. tuberculosis strain, the standard frequently utilized approach is IS6110-RFLP (IS6110-restriction fragment length polymorphism).51 Briefly, the approach entails high concentrations (2–3 g) of entire bacterial DNA extraction, PvuII endonuclease digestion, and standard agarose gel electrophoresis. Then, the gel is applied to complete a Southern blotting, as the fragments of DNA have been moved to a membrane and probes complimentary to the 3′ end prime of the IS6110 sequence. This is followed by hybridization to disclose the size of the generated fragments and the number of IS elements using chemiluminescence (originally radiolabeling).52,53
A main disadvantage of the IS6110-RFLP method is that the M. bovis strain has a single or few copies of the insertion element54 and this affects the discrimination power of this approach due to the same IS6110-RFLP pattern.55 Furthermore, this technique is labor intensive and needs large amounts of DNA, just like REA and PFGE. For these reasons, despite being often utilized for M. TB, M. bovis genotyping does not benefit much from IS6110-RFLP.
PGRS-RFLP
GC content is a valuable parameter for classification and differentiation of MTBC members.56 Polymorphic GC repeat sequence (PGRS) is performed to improve the power of identification of IS6110 isolates for M. bovis, but this also demands large DNA quantities and highly technical skills.57
Spoligotyping
Spoligotyping is a rapid PCR dependent technique that detects the existence of 43 distinctive short DNA sequences (or spacers) in the DR position.58 This approach relies on amplification of the DR, followed by hybridization of the amplified products with membrane bound oligonucleotides that are related to the variable spacer areas located between the DRs.59 The hybridization signals are recognized by chemiluminescence via biotin labeling of the PCR products and a streptavidin–peroxidase conjugate system and then observed using autoradiography.60 Fig. 2 illustrates tuberculosis (TB) spoligotyping intervention.
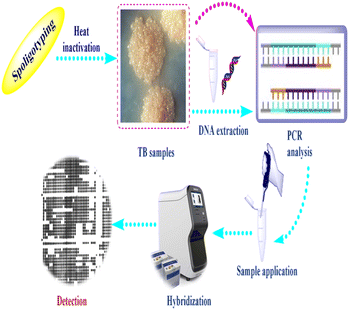 |
| Fig. 2 Flow chart showing tuberculosis (TB) spoligotyping intervention. | |
Patterns of spoligotypes are defined by their identity and the number of spacers. These patterns can be used to distinguish between similar or dissimilar strains. The lack of (3, 9, 16, and 39–43) spacers in M. bovis help in species distinction.61 This method has the benefit of being able to use DNA isolated from infected tissue samples without the need for bacterial isolation.62
Further automatization and development of this method has been directed to employ recognition systems based on microbeads, like Luminex platforms,63 multiplexed primer extension depending on spoligotyping assay using matrix-assisted laser desorption ionization time of flight mass spectrometry (MALDI-TOF MS),64 microarray,65,66 melting curve analysis and ligation based amplification.58 One of the most important advantages of spoligotyping is its ability to be used directly in clinical samples without the requirement for preparatory culture due to its true sensitivity estimated at 10 fg of chromosomal DNA, or the DNA of 2–3 bacterial cells.67 Also, it has shown promise in the characterization of nonviable cultures, Ziehl–Neelsen smear slides, and paraffin-embedded tissue sections.68 The drawback of spoligotyping is homoplasy as irrelevant lineages might exhibit similar patterns due to lack of sequences of spacers, which makes spoligotypes poor predictors of phylogenetic relatedness.69 In addition, it has limitations in the detection of the spread of the disease on the farm scale because of the poor resolution.70 Despite these considerations, spoligotyping is still the best practical genotyping method in M. bovis research.
Variable number tandem repeat (VNTR)
An arrangement of tandem repeats that are orientated in the same direction and clustered together is known as a VNTR locus. The locus's size (measured in base pairs) fluctuates depending on how often the nucleotide sequence is repeated. Recombination or replication defects allow for the addition or deletion of each repeat, resulting in alleles with various repeat counts.71 In contrast to the one DR site used for spoligotyping, there are numerous VNTR loci in MTBC that can be found using PCR. The count of repeats in every position is reflected by the size of the PCR products that are produced. There are several types of VNTR, including MIRU, Mtu and QUB.72,73
MIRU-VNTR needs small amounts of non-purified DNA and produces results in a digital format that may be transferred and used to build national and global databases for routine and research use.74 It makes use of repeated sequence changes that are not subject to selection pressure and evolve quite quickly, making them appropriate for future molecular epidemiological investigations and surveillance.75 A 24-loci MIRU-VNTR PCR is frequently employed, and it is reported to have poor accuracy in distinguishing between samples of M. tuberculosis, but this is not proven for M. bovis .76 The MIRU-VNTR approach provides a greater level of discrimination than spoligotyping, is quicker than RFLP analysis, and involves simple interpretation, as well as being familiar to technicians.77 MIRU-VNTR is also affected by homoplasy69 and assessment of the 24 loci of M. bovis for preventing homoplasy is time consuming and laborious.70
Multispacer sequence typing
Multispacer sequence typing (MST) is a sequencing dependent approach which can help in the epidemiological research of M. bovis by linking several polymorphic markers and utilizing intergenic regions sensitive to high mutation levels given the weak selection power. MST is employed for the M. tuberculosis strain, and not for the M. bovis strain. In the first report, Sales et al. identified the variability of M. bovis isolates using the MST approach in the short term. They also accompanied MST with the spoligotyping method, and the findings strengthen MST as a substitute technique for M. bovis genotyping. It has the benefits of sequencing and accessibility of analyzed sequences in public databases, inference of evolutionary relationships, and usage as a tool for further study by specialists worldwide.78
Risk factors and prevalence of M. bovis in Egypt
Kasir et al. revealed that M. bovis has been reported in animals and humans in only eight nations in the Middle East and North Africa (MENA): Egypt, Algeria, Iran, Iraq, Sudan, Morocco, Tunisia and Turkey. In other MENA nations, Djibouti, Lebanon, Saudi Arabia and Palestine, only human cases of zoonotic TB (zTB) have been recorded.81 The variation of M. bovis prevalence is influenced by the population size and characteristics, geographical region, and applied diagnostic and examination schemes. In addition, bTB prevalence is also linked to other factors, like BCG vaccination status, contaminated dairy product consumption, and the effectiveness of bTB control measures.82 The prevalence of M. bovis seems to be significantly influenced by a variety of bTB risk factors. Age, sex, animal body condition, crowding, grazing practices, cross-species transmission, immunosuppression, environmental conditions, feeding systems, and pathological and physiological differences are powerful parameters affecting the spread M. bovis. Because of parturition, gestation and lactation, female animals are more susceptible to bTB than male animals.16,83 Cross species transmission between (cattle and buffalo) and (cattle and goats) has been correlated to grazing locations and shared drinking water in Iran and Algeria, respectively.84,85 Shared water transport has great zoonotic importance for the transmission of infectious diseases and acquired AMR.86,87 Furthermore, unregulated animal migrations and international trade have been cited as major factors contributing to the spread of bTB.88 Workers (e.g., veterinarians, farmers, slaughterhouse workers) who had a close relationship with livestock, especially dairy cattle, or who dealt with wild animals, were more vulnerable to M. bovis infection.89 Cattle and buffalo were the two animal species in which active bTB was most frequently recorded; rare instances among other species were also noted. In Egypt, M. bovis was recorded in a mongoose,90 camels and pigs.91 Numerous research revealed that the prevalence in Egypt was between 0.1%92 and 16.4%.93 Furthermore, studies with limited population sizes revealed a greater incidence, ranging from 22.2% (ref. 94) to 82.6% (ref. 95) in Egypt. Over time, bTB prevalence patterns have changed; in Egypt, they have ranged between 0.2% (ref. 96) and 4.3%.97 Moreover, the majority of cases of bTB in both humans and animals have been found in the lungs and extrapulmonary regions.81 In humans, an investigation demonstrated that out of 45 pulmonary and 67 extrapulmonary tuberculosis cases, only one was a result of M. bovis in Egypt.98,99 Additionally, when directed at occupational risk groups, accessible data revealed high percentages of zTB, reaching 5.36% in Egypt.100
Whole genome sequencing (WGS) process
The process of WGS begins with DNA extraction and purification. There are different generations of sequencing technologies; second-generation sequencing technologies, such as Illumina, produce short reads on the order of 50–200 base pairs and have low error rates of around 0.5–2%, with the errors chiefly being substitution errors; third-generation technologies, such as PacBio, and fourth-generation technologies, such as Oxford Nanopore, provide read lengths in the thousands or tens of thousands but have much higher error rates of around 10–20%, with errors being chiefly insertions and deletions.101 Next, the ends are blunted and joined to oligonucleotides known as adapters. Usually, PCR is used to amplify these fragments before the final DNA library is applied to the sequencer. Two readings are produced for each genome after the run is complete. These readings must be put together; this process aims to overlap reads to create a segment of the DNA sequenced.102 The result file, which contains a number of contigs (DNA sequences) that the assembler was unable to shut, will be created after the assembling process is complete. Depending on the library preparation, genomic nature, assembly workflow, etc., the number of contigs per sample varies not only between different samples but also between the same sample and different runs. As a result, they are typically utilized to create the genome reference, followed by resequencing on one of the previous mentioned platforms (such as Illumina). The enormous amount of output data generated requires specialist bioinformaticians to perform proper analysis.103
WGS insights into the dynamic transmission of M. bovis
The dynamic transmission of M. bovis has various modes, which need an ecosystem level approach. In this approach, the environment, people, and animals within society must all be taken into account.104 Since the pathogen can be excreted by a variety of routes—including vaginal secretions, milk, feces, urine, and/or semen—age, hygiene practices, and environment all have an impact on the cattle's infection routes.105 Interspecies transmissions have occurred in nations where M. bovis is known to exist in wildlife.106 The wildlife reservoir hosts of M. bovis include the European badger in Ireland and Great Britain, the brush-tailed possum in New Zealand, African buffalo in South Africa, white-tailed deer in Michigan, USA, wild boar in Peninsula,107 and wild boar, goats,108 fallow deer, red deer, and roe deer in Europe.109
Despite some challenges of whole genome sequencing (WGS) in low and middle income countries,110 it has great impact on the discrimination and clarification of the entire DNA sequence. It allows a great degree of resolution, and comprehensive genetic insights including all potential genomic targets, transmission, genetic evolutionary, virulence determining factors, and drug resistance character.111 A molecular typing and WGS investigation was conducted in Egypt's Nile Delta to address the dynamic transmission of M. bovis among dairy cattle. The results found that a prominent spoligotype (SB0268) emerged between 2013 and 2015, signifying a current clonal expansion of this isolate inside the Nile Delta. Remarkably, two isolates belonged to the M. bovis BCG group, although Egypt does not permit animal vaccination. The remaining isolates belonged to the dangerous M. bovis clonal complex European 2, which is present in various European and Latin American nations.112 Furthermore, in Turkey, a clonal similarity study was carried out between cattle and human isolates using the spoligotyping and MIRU-VNTR techniques. The positive results hinted that M. bovis may be correlated to lung tuberculosis in humans.113 In Brazil, 11 spoligotype patterns were detected from 40 (25%) isolates of M. bovis, with a predominance of SB0121 (25.0%), SB1142 (37.5%), and SB1145 (10.0%). Other patterns, SB1050, SB0295, SB1144, SB1802, SB0881, SB0120, SB0140, and SB0849, with different values from 2.5 to 7.5%, were unevenly disseminated throughout the cities and provided epidemiological evidence of their potential exchange.114
M. bovis diversity values are highest in Northern and Eastern Africa, followed by Central Africa, and are lowest in Southern and Western Africa. It has been proposed that M. bovis originated in the Near East, travelled throughout Africa with domesticated animals, and resulted in the acquired ability to digest milk biologically as an adult .115 Moreover, a study carried out in Ethiopia (September 2018 to June 2019) showed that bTB is caused by M. bovis SB0033 and SB1176 strains with weak recurrence.116
A systematic assessment of 15 research studies revealed that M. bovis was the causative agent of all verified instances of zTB in humans in the range 0% to 28%. The predominant exposures to zTB were from cattle and raw dairy. Eight of the 15 studies sampled livestock, mostly cattle, and found that zTB prevalence ranged from 0% to 23%.117 Another meta-analysis review was performed among humans to estimate the M. bovis prevalence from 20 April 2009 to 17 April 2019. It showed that human M. bovis cases occurred globally, and this told us that zTB remains important in all regions. Methodological discrepancies and the requirement for additional molecular studies make it challenging to comprehend the full picture of illness prevalence.118 Kwaghe and his collaborators recommended necessary set up control measures to eliminate TB in cattle and humans in Nigeria. SB1025 and SB0944 were revealed as the patterns of M. bovis strains in cattle, while Haarlem families and LAM 10 were related to M. tuberculosis strains in humans.119,120 However, in Ghana, Acquah et al.'s research revealed that about 29% of M. bovis strains causing bTB are uncharacterized spoligotypes, so more control is needed for bTB in Ghana.121 Sandoval-Azuara et al. performed WGS and SNPs for cattle and human M. bovis isolates. They found that all isolates from humans and cattle were matched in the spoligotype patterns, and all human isolates shared common ancestors with cattle in Baja California. This implies that most tuberculosis reports in humans caused by M. bovis are obtained from travelling cattle.122
Applying WGS for M. bovis isolates from populations of elk, deer, and cattle revealed that bTB in elk and cattle gathered in the same clade, and this implies either intra-species transmission, or the sharing of a common source.123 In South Africa, goats are considered as a potential source of M. bovis for humans, cattle, and wildlife.124 Another novel record of bTB transmission is between cattle populations and badgers in Great Britain.125 Lombard et al. proved that MTBC could be transmitted from humans to cattle in three cases in the USA.89 In Poland, inter-species transmission of M. bovis between a farmer and his cattle has been reported.126 Other WGS studies have been used to exhibit the dynamic transmission of M. bovis isolates between cattle and wildlife populations in different epidemiological settings, including in the UK,127 Ireland,128 New Zealand,79 United States of America,123 Mexico,129 Italy,130 Spain131 and Bulgaria.132 All the above demonstrate the value of WGS research of M. bovis transmission at the livestock–wildlife interface, presenting insights into bTB control.133,134 Fig. 3 shows the interspecies transmission of bTB for livestock–human–wildlife.
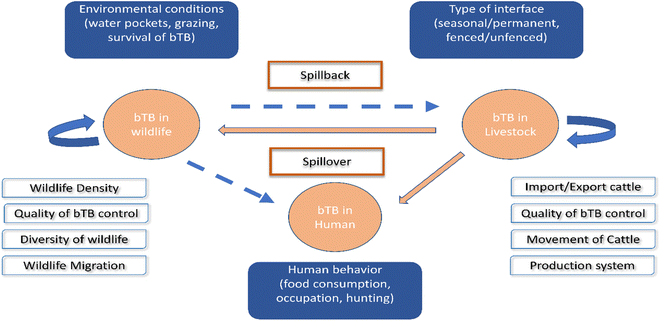 |
| Fig. 3 Interspecies transmission of bTB for livestock–human-wildlife. The bTB can remain in livestock and in wildlife and is encouraged by the different factors included in the white boxes. The danger of bTB is spillover from livestock to human or wildlife, as well as spillback from wildlife to livestock, as shown in the blue boxes.119 | |
WGS insights into the genetic diversity of M. bovis
Knowledge of the genetic diversity of M. bovis is necessary for detecting its routes of transmission.135 Single nucleotide polymorphism (SNP) analysis within virulence genes, which appear with the highest number of SNPs, indicated that M. bovis was continuously modifying and adapting specific mechanisms to sustain dissemination among environments and different hosts.136 The identification of non-synonymous SNPs on the virulence genes allowed for the differentiation of various groups.137 The Mycomar T7 phagemid delivery system was used to produce whole genome transposon libraries in laboratory strains of M. bovis and M. tuberculosis species to compare the gene status during exposure to identical in vitro conditions. In addition, CRISPRi was successfully utilized in these two species to determine the effects of silencing genes with Rv2182c/Mb2204c, a gene included in glycerophospholipid metabolism and wag31, a gene involved in peptidoglycan synthesis, and the results showed that inhibition expression of Mb2204c in M. bovis displayed a considerably smaller growth effect than silencing its orthologue (Rv2182c) in M. tuberculosis. Given that glycerophospholipid metabolism is a confirmed pathway for antimicrobials.137 Furthermore, a comparative genomic analysis of MTBC strains focused on the three features of pathogenicity: virulence, epitope variations, and host association, and the results provided insights into the pathogenic mechanisms, which helps with prevention, management and treatment of the disease.138
The pan-genome is made up of all of a species' genes, and its examination is a very instructive method that allows stratification into: (1) the core genome containing genes that all strains share; (2) the accessory genome containing unnecessary genes not found in all strains; (3) singletons, which are distinct genes that are peculiar to a given strain. The core-genome controls the fundamental phenotype and contains the genes required for the biological processes. The accessory genome is important for genetic diversity of species and adaptation to various environmental survival, new hosts, immune evasion or other tasks that are advantageous over other species.139 A comparative analysis of 70 M. bovis genomes revealed the core and accessory genome components; with 2736 CDS for the former, while the accessory part consists of 3897 CDS, of which 2656 are confined to one/two genomes only. The functional annotation of that genome analysis categorized each CDS into one or several COG (clusters of orthologous groups) categories; lipid metabolism (n = 242), transcription (n = 258), unknown function (n = 876) and energy production (n = 214). Another study disclosed M. bovis lineages by applying pangenome analysis, and the results showed 3900 core genes and only 96 accessory genes.48
Ceres and other scientists have proven that: (1) the accessory genome of M. bovis is noticeably smaller than previously believed, and this finding supports continuing clonal evolution and a closed pangenome with low gene content fluctuation throughout outbreaks; (2) several genes have frameshift mutations, including glpK, which has recently been linked to antibiotic tolerance in M. tuberculosis; (3) indels are the primary cause of the minor gene content variation that develops over brief time periods among closely related sequences; (4) multiple genes have potentially reversible homopolymeric tract alterations, indicating that phase variation may be a common method of gene regulation in MTBC.140
Nanotechnology based targeted drug delivery systems
Nanotechnology is considered a potential tool for the diagnosis and treatment of disease. Nanoparticles can be used as appropriate displacers of the traditional drugs used for the treatment of tuberculosis. Nanocapsules containing antimicrobial drugs are instantly taken up by macrophages, which play an important role, to efficiently reach the active targeted sites.141 Recently, M. tuberculosis DNA biomarker and biosensing platform studies have been involved in the rapid diagnosis of TB and rifampicin-resistant TB (RR-TB).142,143 Several anti-TB agent-based nanomaterials have attracted much attention to be applied for rapid diagnosis and effective treatment, encompassing noble metals (e.g. Au, Ag, Zn), semi-conducting materials such as metal oxides, and carbon nanotubes.144 These nanomaterials showed a high tendency to reveal the signal after recognizing the targeted DNA biomarker.144
Fig. 4 shows a summary of different nanotechnology based targeted drug delivery systems.
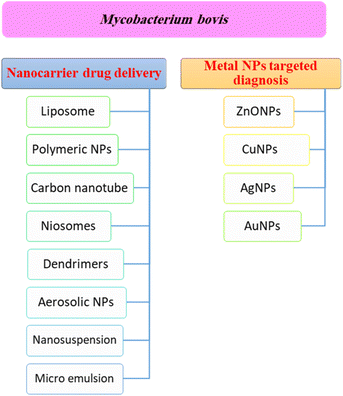 |
| Fig. 4 A summary of different nanotechnology based targeted drug delivery systems. The blue color represents the different nanocarrier drug delivery methods while the orange color represents the metal nanoparticle targeted diagnosis of M. bovis. | |
Nanoparticles for electrochemical detection of H. tuberculosis
Various biochemical methods including physical examination as well as sputum smear microscopy, chest photofluorography, and nucleic acid amplification tests have been used for the assessment of H. tuberculosis.145 Unfortunately, these methods are considered complicated, expensive, and time consuming. Thus, there has been a great need for developing novel methods and techniques for efficient determination of H. tuberculosis. Recently, electrochemical sensors have been investigated as a rapid tool for the diagnosis of tuberculosis. For example, gold particle-decorated graphene as modified paper has been used for the rapid diagnosis of tuberculosis.146 Additionally, rapid electrochemical detection of tuberculosis has been achieved by measuring Ag85 activity with disposable carbon sensors.147
NP-based electrochemical sensors have attracted attention, especially in the biomedical field, because of their high sensitivity, stability, and selectivity.148 Various electrochemical sensors including voltammetric, amperometric, and impedimetric sensors have been extensively investigated for targeting many organic and inorganic pollutants as well as biological molecules and drugs.23 In the present review, we highlight the role of different NP-based electrochemical sensors as valuable tools for targeting H. tuberculosis. The most reported electrochemical sensors used for H. tuberculosis are based on biosensing strategies. These strategies depend on using biological components as recognition elements. The NPs are used for enhancing the performance of these biosensors. They can be used as reporter probes or as enhancers of the performance of other redox probes. To the best of our knowledge, only one study has reported the use of NPs as recognition elements for H. tuberculosis.145
A DNA-based sensor or aptasensor depends on the formation of a DNA double helix between the probe and the target DNA. The hybridization results in signal amplification or inhibition of the NPs. This signal can be monitored using voltammetric techniques such as cyclic voltammetry (CV), differential pulse voltammetry (DPV) and square wave voltammetry (SWV), or the electrochemical impedance spectroscopy (EIS) technique. The amplification signal of voltammetric techniques and the inhibition signal of EIS are attributed to the enhancement of the conductivity or decrease of the impedance at the electrode surface, respectively. In contrast, the inhibition signal of voltammetric techniques and amplification signal of EIS are attributed to the decrease of the conductivity or increase of the impedance at the electrode surface, respectively (Fig. 5). Similarly, antigen-antibody-based sensors or immunosensors are based on the interaction of antigens immobilized on the electrode surface and the antibody of H. tuberculosis. This interaction affects the oxidation current of the NP redox probe at the electrode surface. Different NP-based electrochemical sensors used for targeting H. tuberculosis are listed in Table 2.
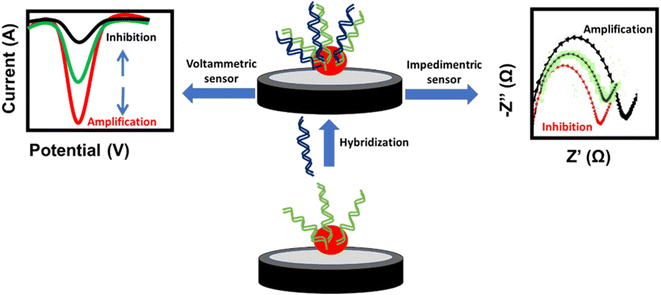 |
| Fig. 5 Schematic representation of the sensing mechanisms of DNA-based sensors. | |
Table 2 NP-based electrochemical sensors for detecting H. tuberculosisa
NP |
Modified electrode |
NP-modification technique |
Type of sensor |
Sensing technique |
Sensing mechanism |
LOD |
Ref. |
ABEI: N-(aminobutyl)-N-(ethylisoluminol), AuNPs: gold nanoparticles, AuSPE: gold screen-printed electrode, ECL: electrogenerated chemiluminescence, CS: chondroitin sulfate, CV: cyclic voltammetry, DPV: differential pulse voltammetry, GCE: glassy carbon electrode, GPNP: graphene nanoparticle powder, HAPNPs: hydroxyapatite nanoparticles, ITO: indium tin oxide, LOD: limit of detection, MWCNTs: multi-walled carbon nanotubes, rGO: reduced graphene oxide, PEI: polyethylenimine, PPY: polypyrrole, SPGE: screen-printed graphene electrode, SWV: square wave voltammetry. |
Au |
ABEI-AuNPs/Au electrode |
Drop casting |
Aptasensor |
ECL |
Signal amplification |
3.3 × 10−16 M |
153 |
AuNPs/Ce-MOFs/GCE |
Electrochemical reduction |
Aptasensor |
DPV |
Signal amplification |
67.6 fg mL−1 |
156 |
Dextrin-AuNPs/SPCE |
Drop casting |
Aptasensor |
DPV |
Signal amplification |
0.01 ng μL−1 |
152 |
AuNPs/PEI/CS/GCE |
Electrochemical reduction |
Aptasensor |
DPV |
Signal inhibition |
0.03 fM |
154 |
rGO nanoribbons/AuNPs/ITO |
Drop casting |
Aptasensor |
CV |
Signal amplification |
0.1 fM |
155 |
Ag |
Graphene quantum dots/Fe3O4/AgNPs/GCE |
Drop casting |
Immunosensor |
DPV |
Signal amplification |
0.33 ng mL−1 |
160 |
Cu |
CuNPs/Pt electrode |
Electrochemical reduction |
Aptasensor |
DPV |
Signal inhibition |
10−9 M |
164 |
Co |
CoNPs/rGO |
Drop casting |
Non-biological sensor |
DPV |
Signal inhibition |
4 × 10−7 M |
145 |
Fe3O4 |
PPY-Fe3O4/AuSPE |
Adsorption in presence of magnet |
Aptasensor |
SWV |
Signal inhibition |
1 fM |
169 |
Graphene |
GPNP/SPGE |
Electrolytic exfoliation method |
Genosensor |
CV |
Signal inhibition |
1 pg |
170 |
PPY nanowire |
PPY nanowire/Au electrode |
Electrodeposition |
Aptasensor |
SWV |
Signal inhibition |
3.6 × 10−19 M |
175 |
MWCNTs |
HAPNPs/PPY/MWCNTs/GCE |
Drop casting |
Aptasensor |
DPV |
Signal amplification |
0.141 × 10−9 M |
177 |
Gold nanoparticles (AuNPs) for electrochemical detection of H. tuberculosis
AuNPs are considered to be excellent electrode modifiers because of their stability and superior conductivity. Additionally, they can improve the sensitivity of electrochemical sensors.149–151 Various AuNP-based electrochemical sensors have been used for detecting H. tuberculosis (Table 2). For example, dextrin-AuNPs were previously studied as an electrochemical reporter for detecting M. tuberculosis using a DNA-based biosensor.152 The sensing strategy is based on the electrochemical detection of AuNPs. Additionally, N-(aminobutyl)-N-(ethylisoluminol) functionalized AuNPs as an electrochemical label were used for the detection of M. tuberculosis using electrogenerated chemiluminescence (EIS).153 Recently, an electrochemical sensor fabricated using the layer-by-layer assembly of AuNPs, polyethylenimine (PEI), and chondroitin sulfate (CS) has been used for the selective determination of the genes of M. tuberculosis using the DPV technique.154 The synergistic effect of PEI and CS enhance the antifouling properties of the sensor towards single proteins. The sensor performance is based on the signal amplification strategy. Additionally, AuNPs modified with 5′SH-ssDNA have been immobilized over reduced graphene oxide nanoribbons for the electrochemical detection of M. tuberculosis using the CV technique.155 On the other hand, urchin-like Ce-MOFs have been used for loading large amounts of AuNPs on the surface of GCE leading to dense immobilization of the capture probe. The mechanism of sensing was based on signal amplification using anthraquinone-2-carboxylic acid as a redox nanoprobe.156
Silver nanoparticles (AgNPs) for the electrochemical detection of H. tuberculosis
Silver nanoparticles (AgNPs) have been extensively investigated as recognition and redox probes for detecting inorganic and organic molecules.23,157–159 Interestingly, they have been studied as electrode modifiers for detecting M. tuberculosis. For example, GCE modified with a nanotriplex of graphene quantum dots, Fe3O4, and AgNPs has been reported as a novel electrochemical immunosensor of M. tuberculosis, where AgNPs prevent the aggregation of Fe3O4 and enhance the electrical conductivity, which facilitates the electron transport at the electrode surface.160
Copper nanoparticles (CuNPs) for the electrochemical detection of H. tuberculosis
Copper nanoparticles (CuNPs) are a promising candidate for fabricating electrochemical sensors due to their high surface area and strong adsorption capability, as well as redox and catalytic properties.161–163 Interestingly, CuNPs have been used for enhancing the electron transfer at the electrode surface for detecting H. tuberculosis DNA. First, CuNPs were electrochemically deposited at the platinum electrode using the chronoamperometric technique, where Cu2+ was reduced at −0.39 V. After electrode modification, ssDNA was immobilized on the CuNPs via incubation, then 6-mercapto-1-hexanol was utilized for the construction of a well-aligned DNA monolayer. The sensing principal is based on the change of the ferrocyanide oxidation current after the hybridization of the ssDNA immobilized on the modified electrode with its complementary DNA. The signal inhibition of the ferrocyanide redox probe upon increasing the concentration of the DNA was used for plotting the calibration curve.164
Cobalt nanoparticles (CoNPs) for the electrochemical detection of H. tuberculosis
Cobalt nanoparticles (CoNPs), especially those fabricated using biological molecules, have exceptional stability, good electroactive sites and electrical conductivity, which make them an appropriate candidate for many electrochemical sensing applications.165,166 They have been used as recognition probes for detecting methyl nicotinate, which is a metabolite of M. tuberculosis. The CoNPs were incorporated with reduced graphene oxide (rGO) dispersed N-doped phenolic polymer precursor-based carbon film for constructing the sensor. Additionally, the carbon film was doped with N-heteroatom using melamine to increase the conductivity of the film.145
Magnetic NPs for the electrochemical detection of H. tuberculosis
Magnetic nanoparticles have useful features such as large surface area, low toxicity, small particle size, and superparamagnetic behavior, which make them an excellent electrode modifier for constructing electrochemical sensors.167,168 An electrochemical sensor based on Fe3O4 incorporated with polypyrrole and a naphthoquinone probe was previously constructed for detecting H. tuberculosis. For modifying the surface of the gold screen-printed electrode with the magnetic particles, a permanent magnet was used, and the potential was swept from −0.4 to +0.8 V vs. Ag/AgCl reference electrode with a scan rate of 50 mV s−1 for ten cycles. The presence of magnetic nanoparticles enhances the response of the redox probe. The hybridization between a probe and the complementary DNA target led to a large decrease in the naphthoquinone redox oxidation current which was monitored using the SWV technique.169
Graphene NPs for the electrochemical detection of H. tuberculosis
Graphene is considered an attractive material for the biosensing process due to its superb structural, optical, and electronic features, as well as its biocompatibility and mechanical stability.170 Graphene-based nanomaterials have been widely used in sensing applications, both directly or as substrates for other materials.171 Previously, a screen printed graphene electrode has been modified with graphene NPs using the electrolytic exfoliation method for targeting the DNA of H. tuberculosis. The presence of graphene NPs increases the electron transfer at the electrode surface.170
Polypyrrole nanowires for the electrochemical detection of H. tuberculosis
Polypyrrole has been widely used due to its good environmental stability and extraction ability of polar compounds.172 Polypyrrole nanowires are applied in many applications due to their good chemical and physical properties.173,174 The features of polypyrrole nanowires, such as the hydrophilic character and the large surface area, enhance the electron transfer at the electrode surface. They have been previously used for enhancing the performance of the ferrocenyl group as a redox probe for detecting the genomic DNA of H. tuberculosis. The sensing principal is based on the decrease of the redox current of the ferrocenyl oxidation, which was monitored using the SWV technique. This decrease is attributed to the small amount of electron transfer occurring after the hybridization of the complementary DNA and the formation of a double strand on the surface.175
Multi-walled carbon nanotubes (MWCNTs) for the electrochemical detection of H. tuberculosis
Carbon-based electrode materials with diverse porous structures show enhanced electrochemical sensing abilities due to their superior conductivity, as well as the absorption and enrichment of analytes from porous structures.176 Previously, a PCR-free DNA electrochemical biosensor was constructed based on multi-walled carbon nanotubes (MWCNTs), polypyrrole, and hydroxyapatite NPs for detecting the DNA of H. tuberculosis. MWCNTs were essential for improving the electrical conductivity, increasing the surface area of the electrode, and providing an ideal immobilization material for the DNA of H. tuberculosis. The sensing principal was based on signal amplification, which was monitored using DPV.177
Conclusion
bTB is an important zoonotic disease that threatens humans and animals worldwide. M. bovis is considered a spillover pathogen, which can be transmitted from livestock to humans to wildlife. More molecular epidemiological studies are needed to determine the dynamic transmission of bTB, to define the dominant genotypes between humans and animals, and to understand the phylogenetic relationships of the strains. Spoligotyping and MIRU-VNTR are widely used to discriminate between M. bovis isolates. Some of the disadvantages of these two techniques are homoplasy and lower resolution power in spoligotyping, and WGS solved these problems. WGS is a valuable tool due to its high-resolution for the analysis of M. bovis genomic diversity, and it provides insights into the role of recombination and positive selection as evolutionary driving forces in a pathogen affecting a large range of host species, with economical and biodiversity impacts across the world. The current review also summarizes the NP-based electrochemical sensors that have been used for detecting tuberculosis. Notably, most of the reported electrochemical sensors are based on biological elements as recognition probes. NPs act as reporter probes or as enhancers of the performance of other redox probes. The sensing principal is based on tuberculosis DNA (aptasensor), antigens (immunosensor), or biomarkers. The aptasensor depends on the formation of a DNA double helix between the probe and the target DNA leading to signal amplification or inhibition of the NPs which can be monitored by voltammetric and impedimetric methods. The immunosensor is based on the interaction of antigens immobilized on the electrode surface and the antibodies of tuberculosis. Overall, NP-electrochemical sensors present an efficient tool for the detection of tuberculosis. They offer a good opportunity for the simple, sensitive, and rapid detection of tuberculosis. However, there can be some challenges, such as the occurrence of non-specific binding with other biological molecules in real-life applications. Therefore, we recommend the integration of microfluidics with electrochemical sensors for reliable results.
Conflicts of interest
There are no conflicts to declare.
Notes and references
- G. Matope, M. B. Gadaga, B. Bhebhe, P. T. Tshabalala and P. V. Makaya, Vet. Med. Sci., 2023, 9, 1327–1337 CrossRef CAS.
- A. P. Barnes, A. Moxey, S. Brocklehurst, A. Barratt, I. J. McKendrick, G. Innocent and B. V. Ahmadi, Prev. Vet. Med., 2023, 211, 105808 CrossRef PubMed.
- F. Admassu, E. Abera, A. Gizachew, T. Sedoro and T. Gari, BMJ Open, 2023, 13, e061836 CrossRef.
- K. Murai, P. Tizzani, L. Awada, L. Mur, N. J. Mapitse and P. CÃCeres, Bulletin de l'OIE, 2019, 2019, 1–3 CrossRef.
- O. Andrievskaia, A. Garceac, D. Lloyd, M. Savic and M.-O. Duceppe, Microbiol. Resour. Announce., 2023, 12, e0121322 CrossRef.
- P. R. Sichewo, T. M. Hlokwe, E. M. C. Etter and A. L. Michel, BMC Microbiol., 2020, 20, 1–9 CrossRef.
- T. T. Deneke, A. Bekele, H. L. Moore, T. Mamo, G. Almaw, G. A. Mekonnen, A. Mihret, R. Tschopp, L. Yeheyis, C. Hodge, J. L. N. Wood, S. Berg, A. Aseffa, B. Tessema, B. Belachew, E. Fekadu, F. Melese, G. Gemechu, H. Taye, S. Haile, S. Ayalew, T. Hailu, C. Yirga, M. Ambaw, T. Solomon, T. Teklewold, S. Gebre, G. Gari, M. Sahle, A. Aliy, A. Olani, A. Sirak, G. Mekonnen, M. Tamiru, S. Guta, J. Wood, A. Conlan, A. Clarke, C. Smith, R. G. Hewinson, M. Vordermeier, J. Nunez-Garcia, G. Ameni, B. Bayissa, A. Zewude, A. Worku, L. Terfassa, M. Chanyalew, T. Mohammed and M. Zeleke, BMC Public Health, 2022, 22, 222 CrossRef.
- M. Krajewska-Wędzina, Ł. Radulski, W. R. Waters, A. Didkowska, A. Zabost, E. Augustynowicz-Kopeć, S. Brzezińska and M. Weiner, Pathogens, 2022, 11, 1170 CrossRef.
- R. Gopalaswamy, V. N. A. Dusthackeer, S. Kannayan and S. Subbian, Journal of Respiration, 2021, 1, 141–164 CrossRef.
- B. Müller, S. Dürr, S. Alonso, J. Hattendorf, C. J. M. Laisse, S. D. C. Parsons, P. D. van Helden and J. Zinsstag, Emerging Infect. Dis., 2013, 19, 899–908 CrossRef.
- E. Borja, L. F. Borja, R. Prasad, T. Tunabuna and J. A. L. M. L. Toribio, Front. Vet. Sci., 2018, 5, 270 CrossRef.
- S. Niemann and P. Supply, Cold Spring Harbor Perspect. Med., 2014, 4, a021188 CrossRef.
- A. C. Schürch and D. van Soolingen, Infect., Genet. Evol., 2012, 12, 602–609 CrossRef.
- A. Roetzer, R. Diel, T. A. Kohl, C. Rückert, U. Nübel, J. Blom, T. Wirth, S. Jaenicke, S. Schuback and S. Rüsch-Gerdes, PLoS Med., 2013, 10, e1001387 CrossRef PubMed.
- D. Couvin, I. Cervera-Marzal, A. David, Y. Reynaud and N. Rastogi, Database, 2022, 2022, 1–8 CrossRef.
- F. Tazerart, J. Saad, N. Sahraoui, D. Yala, A. Niar and M. Drancourt, Pathogens, 2021, 10, 802 CrossRef CAS.
- R. Avoi and Y. C. Liaw, Int. J. Environ. Res. Public Health, 2021, 18, 9740 CrossRef PubMed.
- A. El-Sayed, S. El-Shannat, M. Kamel, M. A. Castañeda-Vazquez and H. Castañeda-Vazquez, Zoonoses Public Health, 2016, 63, 251–264 CrossRef CAS PubMed.
- É. Réveillaud, S. Desvaux, M. L. Boschiroli, J. Hars, É. Faure, A. Fediaevsky, L. Cavalerie, F. Chevalier, P. Jabert, S. Poliak, I. Tourette, P. Hendrikx and C. Richomme, Front. Vet. Sci., 2018, 5, 262 CrossRef PubMed.
- M. Zahran, Heliyon, 2023, e19943 CrossRef CAS.
- Z. Khalifa, M. Zahran, M. A. Zahran and M. A. Azzem, RSC Adv., 2020, 10, 37675–37682 RSC.
- O. Simoska and K. J. Stevenson, Analyst, 2019, 144, 6461–6478 RSC.
- M. M. Zahran, Z. Khalifa, M. Zahran and M. A. Azzem, Mater. Adv., 2021, 7350–7365 RSC.
- H. Zhang, M. Liu, W. Fan, S. Sun and X. Fan, Front. Public Health, 2022, 10, 994745 CrossRef.
- M. Lasserre, P. Fresia, G. Greif, G. Iraola, M. Castro-Ramos, A. Juambeltz, Á. Nuñez, H. Naya, C. Robello and L. Berná, BMC Genomics, 2018, 19, 2 CrossRef PubMed.
- D. Brites, C. Loiseau, F. Menardo, S. Borrell, M. B. Boniotti, R. Warren, A. Dippenaar, S. D. C. Parsons, C. Beisel, M. A. Behr, J. A. Fyfe, M. Coscolla and S. Gagneux, Front. Microbiol., 2018, 9, 2820 CrossRef PubMed.
- S. Gagneux, Nat. Rev. Microbiol., 2018, 16, 202–213 CrossRef CAS PubMed.
- C. V. Mulholland, A. C. Shockey, H. L. Aung, R. T. Cursons, R. F. O’Toole, S. S. Gautam, D. Brites, S. Gagneux, S. A. Roberts, N. Karalus, G. M. Cook, C. S. Pepperell and V. L. Arcus, Front. Microbiol., 2019, 10, 2778 CrossRef PubMed.
- Z. Liu, Z. Jiang, W. Wu, X. Xu, Y. Ma, X. Guo, S. Zhang and Q. Sun, Front. Microbiol., 2022, 13, 3419 Search PubMed.
- H. Ru, X. Liu, C. Lin, J. Yang, F. Chen, R. Sun, L. Zhang and J. Liu, Front. Cell. Infect. Microbiol., 2017, 7, 239 CrossRef.
- D. Bespiatykh, J. Bespyatykh, I. Mokrousov and E. Shitikov, mSphere, 2021, 6, e0053521 CrossRef CAS.
- R. M. Warren, N. C. Gey van Pittius, M. Barnard, A. Hesseling, E. Engelke, M. de Kock, M. C. Gutierrez, G. K. Chege, T. C. Victor, E. G. Hoal and P. D. van Helden, Int. J. Tuberc. Lung Dis., 2006, 10, 818–822 CAS.
- C. K. Zimpel, P. E. Brandão, A. F. de Souza Filho, R. F. de Souza, C. Y. Ikuta, J. S. F. Neto, N. C. Soler Camargo, M. B. Heinemann and A. M. S. Guimarães, Front. Microbiol., 2017, 8, 2389 CrossRef PubMed.
- G. Delogu, M. J. Brennan and R. Manganelli, Adv. Exp. Med. Biol., 2017, 1019, 191–207 CrossRef CAS PubMed.
- C. K. Zimpel, J. S. L. Patané, A. C. P. Guedes, R. F. de Souza, T. T. Silva-Pereira, N. C. S. Camargo, A. F. de Souza Filho, C. Y. Ikuta, J. S. F. Neto, J. C. Setubal, M. B. Heinemann and A. M. S. Guimaraes, Front. Microbiol., 2020, 11, 843 CrossRef PubMed.
- O. K. Tørresen, B. Star, P. Mier, M. A. Andrade-Navarro, A. Bateman, P. Jarnot, A. Gruca, M. Grynberg, A. V. Kajava, V. J. Promponas, M. Anisimova, K. S. Jakobsen and D. Linke, Nucleic Acids Res., 2019, 47, 10994–11006 CrossRef PubMed.
- D. M. Collins and G. W. De Lisle, J. Gen. Microbiol., 1984, 130, 1019–1021 CAS.
- R. Hodgeman, R. Mann, K. Savin, N. Djitro, S. Rochfort and B. Rodoni, BMC Microbiol., 2021, 21, 1–16 CrossRef.
- M. Price-Carter, S. Rooker and D. M. Collins, Vet. Microbiol., 2011, 150, 107–114 CrossRef CAS.
- H. m. Neoh, X. E. Tan, H. F. Sapri and T. L. Tan, Infect., Genet. Evol., 2019, 74, 103935 CrossRef CAS.
- C. Ghazaei, J. Res. Med. Sci., 2018, 23, 63 CrossRef PubMed.
- S. Jeon, N. Lim, S. Park, M. Park and S. Kim, J. Microbiol. Biotechnol., 2018, 28, 338–346 CrossRef CAS PubMed.
- H. Ravansalar, K. Tadayon and K. Ghazvini, Iran. J. Microbiol., 2016, 8, 338 Search PubMed.
- A. Kechin, I. Oscorbin, A. Cherednichenko, E. Khrapov, Y. Schwartz, N. Stavitskaya and M. Filipenko, Arch. Microbiol., 2023, 205, 71 CrossRef CAS.
- H. Alonso, J. I. Aguilo, S. Samper, J. A. Caminero, M. I. Campos-Herrero, B. Gicquel, R. Brosch, C. Martín and I. Otal, Tuberculosis, 2011, 91, 117–126 CrossRef CAS PubMed.
- T. Roychowdhury, S. Mandal and A. Bhattacharya, Sci. Rep., 2015, 5, 1–10 Search PubMed.
- C. Y. Soto, M. C. Menéndez, E. Pérez, S. Samper, A. B. Gómez, M. J. García and C. Martín, J. Clin. Microbiol., 2004, 42, 212–219 CrossRef CAS PubMed.
- C. Charles, C. Conde, F. Biet, M. L. Boschiroli and L. Michelet, Front. Microbiol., 2022, 13, 1868 Search PubMed.
- H. Alonso, S. Samper, C. Martín and I. Otal, BMC Genomics, 2013, 14, 422 CrossRef CAS PubMed.
- K. Kremer, J. R. Glynn, T. Lillebaek, S. Niemann, N. E. Kurepina, B. N. Kreiswirth, P. J. Bifani and D. Van Soolingen, J. Clin. Microbiol., 2004, 42, 4040–4049 CrossRef CAS PubMed.
- C. J. Augusto, W. d. S. Carvalho, I. N. d. Almeida, L. J. d. A. Figueiredo, N. G. T. Dantas, P. N. Suffys and S. S. d. Miranda, Braz. J. Microbiol., 2018, 49, 641–646 CrossRef PubMed.
- S. M. d. Almeida, A. C. Malaspina, C. Q. F. Leite and M. H. F. Saad, Rev. do Inst. Med. Trop. São Paulo, 2019, 61, e10 CrossRef PubMed.
- E. Green, L. C. Obi, A. I. Okoh, M. Nchabeleng, B. E. De Villiers, T. Letsoalo, A. A. Hoosen, P. O. Bessong and R. N. Ndip, J. Health Popul. Nutr., 2013, 31, 1 Search PubMed.
- J. Gonzalo-Asensio, I. Pérez, N. Aguiló, S. Uranga, A. Picó, C. Lampreave, A. Cebollada, I. Otal, S. Samper and C. Martín, PLoS Genet., 2018, 14, e1007282 CrossRef.
- S. Thabet, A. Karboul, N. Dekhil and H. Mardassi, Int. J. Infect. Dis., 2014, 29, 211–218 CrossRef PubMed.
- B. G. Eimani, M. Seyyedi, L. Sahebi, A. Monfaredan and M. Khalili, Int. J. Mycobact., 2015, 4, 123 CrossRef.
- S. Desikan and S. Narayanan, Indian J. Med. Res., 2015, 141, 761 CrossRef CAS PubMed.
- X. Zeng, H. Li, R. Zheng, N. Kurepina, B. N. Kreiswirth, X. Zhao, Y. Xu and Q. Lia, J. Clin. Microbiol., 2016, 54, 2384 CrossRef CAS PubMed.
- C. Genestet, E. Hodille, A. Bernard, M. Vallée, G. Lina, A. Le Meur, G. Refrégier and O. Dumitrescua, Microbiol. Spectrum, 2022, 10, e0022322 CrossRef PubMed.
- J. Zhang, E. Abadia, G. Refregier, S. Tafaj, M. L. Boschiroli, B. Guillard, A. Andremont, R. Ruimy and C. Sola, J. Med. Microbiol., 2010, 59, 285–294 CrossRef CAS PubMed.
- N. H. Smith, S. V. Gordon, R. de la Rua-Domenech, R. S. Clifton-Hadley and R. G. Hewinson, Nat. Rev. Microbiol., 2006, 4, 670–681 CrossRef CAS PubMed.
- A. G. M. Van Der Zanden, A. H. Hoentjen, F. G. C. Heilmann, E. F. Weltevreden, L. M. Schouls and J. D. A. Van Embden, Mol. Pathol., 1998, 51, 209 CrossRef CAS PubMed.
- K. Masoud, G. F. Araj, L. Reslan, S. Fadlallah, M. Wehbe, L. Y. Itani, A. Z. Avedissian, G. Dbaibo, A. Saade, G. Refrégier, C. Sola and G. M. Matar, J. Infect. Dev. Countries, 2020, 14, 878–885 CrossRef CAS PubMed.
- V. Lorente-Leal, E. Liandris, J. Bezos, M. Pérez-Sancho, B. Romero and L. d. Juan, Front. Vet. Sci., 2022, 9, 827702 CrossRef PubMed.
- A. M. Cabibbe, P. Miotto, R. Moure, F. Alcaide, S. Feuerriegel, G. Pozzi, V. Nikolayevskyy, F. Drobniewski, S. Niemann, K. Reither, D. M. Cirillo, P. Di Pietro, F. San Biagio, E. Alessi, T. G. Barbuzzi, S. Tafaj, E. Bachiyska, I. Kontsevaya, Y. Balabanova, E. Lazzeri, J. Sserunkuma, F. Aloi, M. Nsubuga and M. Sasamalo, J. Clin. Microbiol., 2015, 53, 3876–3880 CrossRef CAS PubMed.
- J. Li, Y. Wang, L. Yan, C. Zhang, Y. He, J. Zou, Y. Zhou, C. Zhong and X. Zhang, Microbes Infect., 2022, 24, 105002 CrossRef CAS PubMed.
- S. Kulkarni, C. Sola, I. Filliol, N. Rastogi and G. Kadival, Res. Microbiol., 2005, 156, 588–596 CrossRef CAS PubMed.
- J. R. Driscoll, M. A. McGarry and H. W. Taber, J. Clin. Microbiol., 1999, 37, 274 CrossRef CAS PubMed.
- J. F. Reyes, C. H. S. Chan and M. M. Tanaka, Infect. Genet. Evol., 2012, 12, 811–818 CrossRef PubMed.
- A. M. S. Guimaraes and C. K. Zimpel, Microorganisms, 2020, 8, 667 CrossRef CAS PubMed.
- R. Ramazanzadeh and R. McNerney, Pak. J. Biol. Sci., 2007, 10, 2612–2621 CrossRef CAS PubMed.
- M. Ghavidel, K. Tadayon, N. Mosavari, K. Nourian, H. R. BahramiTaghanaki, G. R. Mohammadi, M. Rashtibaf and K. Ghazvini, Rep. Biochem. Mol. Biol., 2019, 8, 335 Search PubMed.
- M. Kargarpour Kamakoli, S. Hadifar, S. Khanipour, G. Farmanfarmaei, A. Fateh, S. D. Siadat and F. Vaziri, Infect. Dis., 2019, 51, 659–667 CrossRef CAS PubMed.
- M. Kato-Maeda, J. Z. Metcalfe and L. Flores, Future Microbiol., 2011, 6, 203–216 CrossRef CAS PubMed.
- T. Jagielski, J. Van Ingen, N. Rastogi, J. Dziadek, P. K. Mazur and J. Bielecki, BioMed Res. Int., 2014, 2014, 645802 Search PubMed.
- D. H. Wyllie, J. A. Davidson, E. Grace Smith, P. Rathod, D. W. Crook, T. E. A. Peto, E. Robinson, T. Walker and C. Campbell, EBioMedicine, 2018, 34, 122–130 CrossRef PubMed.
- F. Belakehal, S. A. Barth, C. Menge, H. T. Mossadak, N. Malek and I. Moser, PLoS One, 2022, 17, e0262390 CrossRef CAS PubMed.
- É. B. Sales, A. A. Fonseca, C. M. Gonçalves, A. P. Lage, G. I. Andrade, P. N. Suffys, H. M. Gomes, N. L. Dias, J. S. Ferreira Neto, A. M. d. S. Guimarães and M. B. Heinemann, Front. Vet. Sci., 2021, 8, 396 CrossRef PubMed.
- M. Price-Carter, R. Brauning, G. W. de Lisle, P. Livingstone, M. Neill, J. Sinclair, B. Paterson, G. Atkinson, G. Knowles, K. Crews, J. Crispell, R. Kao, S. Robbe-Austerman, T. Stuber, J. Parkhill, J. Wood, S. Harris and D. M. Collins, Front. Vet. Sci., 2018, 5, 272 CrossRef PubMed.
- Z. Djelouadji, C. Arnold, S. Gharbia, D. Raoult and M. Drancourt, PLoS One, 2008, 3, e2433 CrossRef PubMed.
- D. Kasir, N. Osman, A. Awik, I. El Ratel, R. Rafei, I. Al Kassaa, D. El Safadi, R. Salma, K. El Omari and K. J. Cummings, Diseases, 2023, 11, 39 CrossRef PubMed.
- P. R. Bapat, R. S. Dodkey, S. D. Shekhawat, A. A. Husain, A. R. Nayak, A. P. Kawle, H. F. Daginawala, L. K. Singh and R. S. Kashyap, Journal of Epidemiology and Global Health, 2017, 7, 277–283 CrossRef PubMed.
- M. K. Ghebremariam, V. P. M. G. Rutten, J. C. M. Vernooij, K. Uqbazghi, T. Tesfaalem, T. Butsuamlak, A. M. Idris, M. Nielen and A. L. Michel, BMC Vet. Res., 2016, 12, 1–7 CrossRef PubMed.
- H. Damene, D. Tahir, M. Diels, A. Berber, N. Sahraoui and L. Rigouts, PLoS Neglected Trop. Dis., 2020, 14, 1–18 CrossRef PubMed.
- K. Tadayon, N. Mosavari, A. H. Shahmoradi, F. Sadeghi, A. Azarvandi and K. Forbes, J. Vet. Med., Ser. B, 2006, 53, 41–42 CrossRef.
- L. A. Dagher, J. Hassan, S. Kharroubi, H. Jaafar and I. I. Kassem, Antibiotics, 2021, 10, 883 CrossRef CAS PubMed.
- I. I. Kassem and H. Jaafar, Water Int., 2020, 45, 423–429 CrossRef.
- M. S. A. E. Elsayed, Infect., Genet. Evol., 2019, 71, 211–214 CrossRef CAS PubMed.
- J. E. Lombard, E. A. Patton, S. N. Gibbons-Burgener, R. F. Klos, J. L. Tans-Kersten, B. W. Carlson, S. J. Keller, D. J. Pritschet, S. Rollo, T. V. Dutcher, C. A. Young, W. C. Hench, T. C. Thacker, C. Perea, A. D. Lehmkuhl and S. Robbe-Austerman, Front. Vet. Sci., 2021, 8, 748 Search PubMed.
- A. Matos, L. Figueira, M. Martins, M. Matos, M. Morais, A. Dias and A. Coelho, Vet. Rec., 2013, 173, 376 CrossRef CAS PubMed.
- Y. F. Elnaker, M. S. Diab, N. A. Ibrahim, A. El-Gedawy, R. S. Zaki and A. Radwan, Vet. World, 2019, 12, 1180 Search PubMed.
- G. S. Abdellrazeq, M. M. Elnaggar, H. S. Osman, W. C. Davis and M. Singh, Transboundary Emerging Dis., 2016, 63, 497–507 CrossRef CAS PubMed.
- I. Elsohaby, H. A. Ahmed, M. M. El-Diasty, A. A. Elgedawy, E. Mahrous and F. I. El Hofy, J. Appl. Microbiol., 2020, 129, 1207–1219 CrossRef CAS PubMed.
- Y. F. Elnaker, N. T. Elsharawy, M. S. Daib, A. A. Elgedawy and N. A. Ibrahim, Assiut Vet. Med. J., 2018, 64, 179–186 CrossRef.
- N. A. Hassanain, M. A. Hassanain, Y. A. Soliman, A. A. Ghazy and Y. A. Ghazyi, Adv. J. Microbiol. Res., 2014, 446–450 Search PubMed , https://internationalscholarsjournals.com.
- M. Lobna and E. Nasr, Int. J. Adv. Res., 2015, 3, 693–701 CAS.
- M. Sabry and A. Elkerdasy, J. Pharm. BioAllied Sci., 2014, 6, 115–121 CrossRef PubMed.
- S. Abbadi, G. El Hadidy, N. Gomaa and R. Cooksey, Int. J. Infect. Dis., 2009, 13, 236–242 CrossRef CAS PubMed.
- R. C. Cooksey, S. H. Abbadi, C. L. Woodley, D. Sikes, M. Wasfy, J. T. Crawford and F. Mahoney, J. Clin. Microbiol., 2002, 40, 1651–1655 CrossRef CAS PubMed.
- H. A. Abdelsadek, H. M. Sobhy, K. F. Mohamed, S. H. A. Hekal, A. N. Dapgh and A. S. Hakim, Vet.
World, 2020, 13, 2150 CAS.
- Y. Yoshinaga, C. Daum, G. He and R. O'Malley, Fungal Genomics: Methods and Protocols, 2018, 37–52 CAS.
- T. Zhang, J. Zhou, W. Gao, Y. Jia, Y. Wei and G. Wang, Briefings Bioinf., 2022, 23, bbac305 CrossRef PubMed.
- R. Vaser, I. Sović, N. Nagarajan and M. Šikić, Genome Res., 2017, 27, 737–746 CrossRef CAS PubMed.
- M. Q. Wilber, K. M. Pepin, H. Campa, S. E. Hygnstrom, M. J. Lavelle, T. Xifara, K. C. VerCauteren and C. T. Webb, J. Appl. Ecol., 2019, 56, 1471–1481 CrossRef.
- M. Domingo, E. Vidal and A. Marco, Res. Vet. Sci., 2014, 97, S20–S29 CrossRef CAS PubMed.
- B. Z. Katale, E. V. Mbugi, K. K. Siame, J. D. Keyyu, S. Kendall, R. R. Kazwala, H. M. Dockrell, R. D. Fyumagwa, A. L. Michel, M. Rweyemamu, E. M. Streicher, R. M. Warren, P. van Helden and M. I. Matee, Transboundary Emerging Dis., 2017, 64, 815 CrossRef CAS PubMed.
- M. V. Palmer, Transboundary Emerging Dis., 2013, 60, 1–13 CrossRef PubMed.
- S. Berg, M. C. Garcia-Pelayo, B. Müller, E. Hailu, B. Asiimwe, K. Kremer, J. Dale, M. B. Boniotti, S. Rodriguez, M. Hilty, L. Rigouts, R. Firdessa, A. MacHado, C. Mucavele, B. N. R. Ngandolo, J. Bruchfeld, L. Boschiroli, A. Müller, N. Sahraoui, M. Pacciarini, S. Cadmus, M. Joloba, D. Van Soolingen, A. L. Michel, B. Djønne, A. Aranaz, J. Zinsstag, P. Van Helden, F. Portaels, R. Kazwala, G. Källenius, R. G. Hewinson, A. Aseffa, S. V. Gordon and N. H. Smith, J. Bacteriol., 2011, 193, 670–678 CrossRef CAS PubMed.
- C. Gortázar, R. J. Delahay, R. A. McDonald, M. Boadella, G. J. Wilson, D. Gavier-Widen and P. Acevedo, Mamm Rev., 2012, 42, 193–206 CrossRef.
- C. D. Foo, P. Shrestha, L. Wang, Q. Du, A. L. García-Basteiro, A. S. Abdullah and H. Legido-Quigley, PLoS Med., 2022, 19, e1003899 CrossRef PubMed.
- I. D. Otchere, A. J. van Tonder, A. Asante-Poku, L. Sánchez-Busó, M. Coscollá, S. Osei-Wusu, P. Asare, S. Y. Aboagye, S. A. Ekuban, A. I. Yahayah, A. Forson, A. Baddoo, C. Laryea, J. Parkhill, S. R. Harris, S. Gagneux and D. Yeboah-Manu, PLoS One, 2019, 14, e0209395 CrossRef CAS PubMed.
- H. F. M. Abdelaal, D. Spalink, A. Amer, H. Steinberg, E. A. Hashish, E. A. Nasr and A. M. Talaat, Sci. Rep., 2019, 9, 1–15 CrossRef CAS PubMed.
- T. G. Gökmen, G. Yakici, Y. Kalayci, N. Turut, M. M. Ocal, M. Haligür, E. Günaydin and F. Köksal, Iran. J. Vet. Res., 2022, 23, 210 Search PubMed.
- C. D'Souza, U. Kishore and A. G. Tsolaki, Immunobiology, 2022, 228, 1–19 Search PubMed.
- O. F. Inlamea, P. Soares, C. Y. Ikuta, M. B. Heinemann, S. J. Achá, A. Machado, J. S. F. Neto, M. Correia-Neves and T. Rito, PLoS Neglected Trop. Dis., 2020, 14, e0008081 CrossRef PubMed.
- M. Ejo, B. Haile, T. Tariku, S. Nigatu, E. Kebede, A. B. Bitew, Y. Demessie, G. Getaneh, A. Alebie, M. Girma, F. Ota and A. Nuru, BMC Microbiol., 2021, 21, 1–10 CrossRef PubMed.
- S. A. Luciano and A. Roess, Zoonoses Public Health, 2020, 67, 97 CrossRef PubMed.
- H. Taye, K. Alemu, A. Mihret, J. L. N. Wood, Z. Shkedy, S. Berg and A. Aseffa, Zoonoses Public Health, 2021, 68, 704–718 CrossRef PubMed.
- A. V. Kwaghe, J. A. Ameh, C. A. Kudi, A. G. Ambali, H. K. Adesokan, V. O. Akinseye, O. D. Adelakun, J. G. Usman and S. I. Cadmus, BMC Microbiol., 2023, 23, 7 CrossRef CAS PubMed.
- A. V. Kwaghe, J. A. Ameh, C. A. Kudi, A.-G. Ambali, H. K. Adesokan, V. O. Akinseye, O. D. Adelakun and J. G. Usman, Molecular Characterization of Mycobacterium tuberculosis Complex in Cattle and Humans, Maiduguri, Borno State, Nigeria: a Cross-sectional Study, 2022, DOI:10.21203/RS.3.RS-1549668/V1.
- S. E. K. Acquah, P. Asare, E. K. Danso, P. Tetteh, A. Y. Tetteh, D. Boateng, S. Osei-Wusu, T. Afum, Y. I. Ayamdooh and E. A. Akugre, PLoS Neglected Trop. Dis., 2022, 16, e0010649 CrossRef PubMed.
- S. E. Sandoval-Azuara, R. Muñiz-Salazar, R. Perea-Jacobo, S. Robbe-Austerman, A. Perera-Ortiz, G. López-Valencia, D. M. Bravo, A. Sanchez-Flores, D. Miranda-Guzmán, C. A. Flores-López, R. Zenteno-Cuevas, R. Laniado-Laborín, F. L. de la Cruz and T. P. Stuber, Int. J. Infect. Dis., 2017, 63, 48–56 CrossRef CAS PubMed.
- L. C. M. Salvador, D. J. O'Brien, M. K. Cosgrove, T. P. Stuber, A. M. Schooley, J. Crispell, S. V. Church, Y. T. Gröhn, S. Robbe-Austerman and R. R. Kao, Mol. Ecol., 2019, 28, 2192–2205 CrossRef CAS PubMed.
- D. M. Cooke, W. J. Goosen, T. Burgess, C. Witte and M. A. Miller, Vet. Immunol. Immunopathol., 2023, 257, 110559 CrossRef CAS PubMed.
- A. J. Van Tonder, M. J. Thornton, A. J. K. Conlan, K. A. Jolley, L. Goolding, A. P. Mitchell, J. Dale, E. Palkopoulou, P. J. Hogarth, R. G. Hewinson, J. L. N. Wood and J. Parkhill, PLoS Pathog., 2021, 17, e1010075 CrossRef CAS PubMed.
- M. Krajewska-Wędzina, Ł. Radulski, W. R. Waters, A. Didkowska, A. Zabost, E. Augustynowicz-Kopeć, S. Brzezińska and M. Weiner, Pathogens, 2022, 11, 1170 CrossRef PubMed.
- J. Crispell, C. H. Benton, D. Balaz, N. De Maio, A. Ahkmetova, A. Allen, R. Biek, E. L. Presho, J. Dale, G. Hewinson, S. J. Lycett, J. Nunez-Garcia, R. A. Skuce, H. Trewby, D. J. Wilson, R. N. Zadoks, R. J. Delahay and R. R. Kao, eLife, 2019, 8, e45833 CrossRef CAS PubMed.
- J. Crispell, S. Cassidy, K. Kenny, G. McGrath, S. Warde, H. Cameron, G. Rossi, T. Macwhite, P. C. L. White, S. Lycett, R. R. Kao, J. Moriarty and S. V. Gordon, Microb. Genomics, 2020, 6, 1–8 CAS.
- A. P. Ortiz, C. Perea, E. Davalos, E. F. Velázquez, K. S. González, E. R. Camacho, E. A. García Latorre, C. S. Lara, R. M. Salazar, D. M. Bravo, T. P. Stuber, T. C. Thacker and S. Robbe-Austerman, Front. Vet. Sci., 2021, 8, 674307 CrossRef PubMed.
- C. Marianelli, B. Amato, M. B. Boniotti, M. Vitale, F. P. Ciarello, M. L. Pacciarini and V. D. M. Lo Presti, PLoS Neglected Trop. Dis., 2019, 13, e0007546 CrossRef PubMed.
- P. Pozo, V. Lorente-Leal, S. Robbe-Austerman, J. Hicks, T. Stuber, J. Bezos, L. de Juan, J. L. Saez, B. Romero and J. Alvarez, Front. Microbiol., 2022, 13, 915843 CrossRef PubMed.
- V. Valcheva, C. Perea, T. Savova-Lalkovska, A. Dimitrova, L. Radulski, I. Mokrousov, K. Marinov, H. Najdenski and M. Bonovska, BMC Vet. Res., 2022, 18, 1–12 CrossRef PubMed.
- N. M. Zetola, P. K. Moonan, E. Click, J. E. Oeltmann, J. Basotli, X.-J. Wen, R. Boyd, J. L. Tobias, A. Finlay and C. Modongo, Emerging Infect. Dis., 2021, 27, 835 CrossRef CAS PubMed.
- M. De Garine-Wichatitsky, A. Caron, R. Kock, R. Tschopp, M. Munyeme, M. Hofmeyr and A. Michel, Epidemiol. Infect., 2013, 141, 1342–1356 CrossRef CAS PubMed.
- G. Almaw, A. Mihret, T. Abebe, G. Ameni, B. Gumi, A. Olani, M. Tamiru, T. Koran, A. Aliy, M. Sombo, S. Ayalew, A. Yesuf, H. Taye, J. L. N. Wood, S. Berg, A. Aseffa, B. Tessema, B. Belachew, E. Fekadu, F. Melese, G. Gemechu, R. Tschopp, S. Haile, T. Hailu, A. Bekele, C. Yirga, M. Ambaw, T. Mamo, T. Solomon, T. Teklewold, S. Gebre, G. Gari, M. Sahle, A. Sirak, G. Mekonnen, S. Guta, J. Wood, A. Conlan, A. Clarke, H. L. Moore, C. Hodge, R. G. Hewinson, M. Vordermeier, J. Nunez-Garcia, B. Bayissa, A. Zewude, A. Worku, L. Terfassa, M. Chanyalew, T. Mohammed and Y. Zeleke, Zoonoses Public Health, 2022, 69, 663–672 CrossRef CAS PubMed.
- C. Perea, G. Ciaravino, T. Stuber, T. C. Thacker, S. Robbe-Austerman, A. Allepuz and B. P. de Val, Microorganisms, 2021, 9, 1629 CrossRef PubMed.
- A. Hauer, L. Michelet, T. Cochard, M. Branger, J. Nunez, M. L. Boschiroli and F. Biet, Front. Microbiol., 2019, 10, 955 CrossRef PubMed.
- X. Jia, L. Yang, M. Dong, S. Chen, L. Lv, D. Cao, J. Fu, T. Yang, J. Zhang, X. Zhang, Y. Shang, G. Wang, Y. Sheng, H. Huang and F. Chen, Front. Cell. Infect. Microbiol., 2017, 7, 88 Search PubMed.
- G. Vernikos, D. Medini, D. R. Riley and H. Tettelin, Curr. Opin. Microbiol., 2015, 23, 148–154 CrossRef CAS PubMed.
- K. M. Ceres, M. J. Stanhope and Y. T. Gröhn, Microb. Genomics, 2022, 8, 000839 CAS.
- L. Muthukrishnan, Chem.-Biol. Interact., 2021, 337, 109397 CrossRef CAS PubMed.
- K. C. Januarie, O. V. Uhuo, E. Iwuoha and U. Feleni, Anal. Bioanal. Chem., 2022, 414, 907 CrossRef CAS PubMed.
- A. Rabti, A. Raouafi and N. Raouafi, Nanotechnology Based Approaches for Tuberculosis Treatment, 2020, pp. 207–230 Search PubMed.
- M. Gupta and L. S. Meena, Journal of Nanotechnology and Nanomaterials, 2020, 1, 46–56 Search PubMed.
- P. K. Bairagi, A. Goyal and N. Verma, Sens. Actuators, B, 2019, 297, 126754 CrossRef CAS.
- T. Pornprom, N. Phusi, P. Thongdee, B. Pakamwong, J. Sangswan, P. Kamsri, A. Punkvang, K. Suttisintong, J. Leanpolchareanchai and P. Hongmanee, Talanta, 2023, 125210 Search PubMed.
- E. Barbier, T. Fouchet, A. Hartmann, E. Cambau, F. Mougari, C. Dubois, M. Lubetzki and M. Rochelet, Talanta, 2023, 253, 123927 CrossRef CAS PubMed.
- F. Mollarasouli, E. Zor, G. Ozcelikay and S. A. Ozkan, Talanta, 2021, 226, 122108 CrossRef CAS PubMed.
- D. Örenli, C. K. Selvi, F. Öztürk, P. E. Erden and E. Kılıç, Anal. Biochem., 2023, 662, 115002 CrossRef PubMed.
- X. Zhang, J. Zhu, Z. Wu, W. Wen, X. Zhang and S. Wang, Anal. Chim. Acta, 2023, 1239, 340743 CrossRef CAS PubMed.
- G. Zhao, T. Pham, Y. Chen, M. Sedki and A. Mulchandani, Microchem. J., 2023, 187, 108376 CrossRef CAS.
- E. Torres-Chavolla and E. C. Alocilja, Biosens. Bioelectron., 2011, 26, 4614–4618 CrossRef CAS PubMed.
- X. Yu, Y. Chai, J. Jiang and H. Cui, J. Photochem. Photobiol., A, 2012, 241, 45–51 CrossRef CAS.
- C. Wu, Y. Ma and L. Chen, J. Electroanal. Chem., 2022, 907, 116013 CrossRef CAS.
- N. K. Mogha, V. Sahu, R. K. Sharma and D. T. Masram, J. Mater. Chem. B, 2018, 6, 5181–5187 RSC.
- Y. Li, D. Peng, S. Guo, B. Yang, J. Zhou, J. Zhou, Q. Zhang and L. Bai, Bioelectrochemistry, 2022, 147, 108209 CrossRef CAS PubMed.
- M. Zahran, Z. Khalifa, M. A. Zahran and M. A. Azzem, Microchem. J., 2021, 106173 CrossRef CAS.
- M. Zahran, Z. Khalifa, M. A.-H. Zahran and M. Abdel Azzem, ACS Appl. Nano Mater., 2020, 3, 3868–3875 CrossRef CAS.
- M. Zahran, Z. Khalifa, M. A.-H. Zahran and M. A. Azzem, Electrochim. Acta, 2020, 356, 136825 CrossRef CAS.
- L. T. Tufa, S. Oh, J. Kim, K.-J. Jeong, T. J. Park, H.-J. Kim and J. Lee, Electrochim. Acta, 2018, 290, 369–377 CrossRef CAS.
- M. S. de Oliveira, E. A. de Oliveira Farias, A. M. S. de Sousa, N. A. Dionísio, P. R. S. Teixeira, A. S. d. N. M. Teixeira, D. A. da Silva and C. Eiras, Surf. Interfaces, 2021, 25, 101245 CrossRef CAS.
- M. Shalauddin, S. Akhter, W. J. Basirun, M. Akhtaruzzaman, M. A. Mohammed, N. M. M. Abd Rahman and N. M. Salleh, Surf. Interfaces, 2022, 34, 102385 CrossRef CAS.
- C. Guang-Lei, J.-C. Huang, Y. Jia-Qi, G. Ye-Min, L. Ming, Y.-Y. Zhang and S. Xia, Chin. J. Anal. Chem., 2021, 49, 1–9 Search PubMed.
- D. V. P. Thao, D. T. T. Ngan, D. Van Tuan, H. Lan, N. T. Nguyet, V. Van Thu, V.-P. Hung and P. D. Tam, Mater. Today Commun., 2022, 33, 104161 CrossRef CAS.
- Y. Liu, X. Yan, Y. Tang, N. Lu, T. Zhang, Z. Xu, Y. Xing, P. Zhao, M. Liu and Y. Zhu, J. Electroanal. Chem., 2022, 926, 116932 CrossRef CAS.
- A. K. Singh, Curr. Res. Green Sustainable Chem., 2022, 100270 CrossRef CAS.
- D. Manoj, L. Gnanasekaran, S. Rajendran, A. Jalil, M. N. Siddiqui, F. Gracia and M. Soto-Moscoso, Environ. Res., 2023, 222, 115358 CrossRef CAS PubMed.
- R. Zokhtareh, M. Rahimnejad, G. Najafpour-Darzi and H. Karimi-Maleh, Environ. Res., 2023, 216, 114643 CrossRef CAS PubMed.
- M. Haddaoui, C. Sola, N. Raouafi and H. Korri-Youssoufi, Biosens. Bioelectron., 2019, 128, 76–82 CrossRef CAS PubMed.
- W. Jaroenram, J. Kampeera, N. Arunrut, C. Karuwan, A. Sappat, P. Khumwan, S. Jaitrong, K. Boonnak, T. Prammananan and A. Chaiprasert, J. Pharm. Biomed. Anal., 2020, 186, 113333 CrossRef CAS PubMed.
- S. R. Benjamin and E. J. M. R. Junior, Current Opinion in Environmental Science & Health, 2022, 100381 Search PubMed.
- Y. Hou, Y. Li, W. Huang, M. Tsunoda, Y. Zhang, X. Xie, R. Wang, L. Li, W. Hu and Y. Song, J. Chromatogr. A, 2020, 1632, 461609 CrossRef CAS PubMed.
- C. P. McCarthy, K. M. Herdman, D. Rooney, B. Alcock-Earley and C. B. Breslin, Synth. Met., 2021, 280, 116881 CrossRef CAS.
- Y. Yan, P. Zhou, S. Zhang, X. Yin, X. Zeng, P. Pi, Y. Nong and X. Wen, J. Water Proc. Eng., 2022, 49, 102942 CrossRef.
- R. Khoder and H. Korri-Youssoufi, Mater. Sci. Eng. C, 2020, 108, 110371 CrossRef CAS PubMed.
- H. Ali, A. Yadav and N. Verma, Chem. Eng. Process., 2022, 180, 108656 CrossRef CAS.
- K. S. Rizi, B. Hatamluyi, M. Rezayi, Z. Meshkat, M. Sankian, K. Ghazvini, H. Farsiani and E. Aryan, Talanta, 2021, 226, 122099 CrossRef CAS PubMed.
|
This journal is © The Royal Society of Chemistry 2023 |