DOI:
10.1039/D3RA02845C
(Paper)
RSC Adv., 2023,
13, 20282-20297
Computational formulation study of insulin on biodegradable polymers†
Received
30th April 2023
, Accepted 23rd May 2023
First published on 6th July 2023
Abstract
Insulin administered orally has a limited therapeutic profile due to factors such as digestion enzymes, pH, temperature, and acidic conditions in the gastrointestinal tract. Type 1 diabetes patients are typically restricted to use intradermal insulin injections to manage their blood sugar levels as oral administration is not available. Research has shown that polymers could enhance the oral bioavailability of therapeutic biologicals, but traditional methods for developing suitable polymers are time-consuming and resource-intensive. Although computational formulations can be used to identify the best polymers more quickly. The true potential of biological formulations has not been fully explored due to a lack of benchmarking studies. Therefore, molecular modelling techniques were used as a case study in this research to determine which polymer is most compatible among five natural biodegradable polymers to address insulin stability. Specially, molecular dynamics simulations were conducted in order to compare insulin-polymer mixtures at different pH levels and temperatures. Hormonal peptide morphological properties were analyzed in body and storage conditions to assess stability of insulin with and without polymers. According to our computational simulations and energetic analyses, polymer cyclodextrin and chitosan maintain insulin stability the most effectively, while alginate and pectin are less effective relatively. Overall, this study contributes valuable insight into the role of biopolymers in stabilizing hormonal peptides in biological and storage conditions. A study such as this could have a significant impact on the development of new drug delivery systems and encourage scientists to utilize them in the formulation of biologicals.
Introduction
A wide range of diseases have been successfully treated with use of bio-therapeutics such as cancer, autoimmune disorders, metabolic disorders, and infectious diseases.1 The medicines in this category include antibodies, peptides, nucleic acids, fusion proteins, enzymes, and vaccines that are used to prevent critical diseases and disorders in humans and animals.2 Biologicals are highly specific, whereas synthetic organic agents are likely to cause mild to moderate adverse reactions.3 Drugs can be administered in various ways, but oral delivery is the most common method due to the ease of compliance of patients. To reap the benefits of oral bioavailability, drugs must pass through the digestive system. Extreme conditions and temperatures are prevalent in this environment, so biological peptides must endure these conditions and temperatures to remain intact.4 Apparently, biologics are large, molecularly diverse, and poorly understood mechanisms of action make them hard topics to research upon.5,6 Currently, due to protein denaturation in oral route except for few biologicals, many bio-therapeutics routes of administration are limited to parenteral injections.7 Thus, the development of biological products is both an opportunity for the growth of revenue and a means to improve the health of individuals.8
Generally, diabetics is a common condition which has considerable impact on human life, and among the two types of diabetics; type 1 diabetes patients must manage their blood sugar levels periodically by injecting insulin.9–11 However, the fear of injection pain causes several patients to miss their intended doses. As a result, many patients fail to take their insulin shots, resulting in mismanagement of their blood sugar levels. Moreover, prolonged blood sugar levels pose a significant risk to human health due to nerve damage, vision impairment, kidney failure, and heart attacks.12 Thus, research on insulin formulation has great scope to improve drug delivery compliance. Encouragingly research has shown that excipients such as salts, polymers, amino acids, and surfactants can improve insulin's thermal stability.13,14 Moreover, researchers are further investigating several methods for increasing the bioavailability of oral insulin, including absorption enhancers, enzyme inhibitors and polymers enteric coatings.15,16
Polymers derived from natural sources exhibit biocompatibility, biodegradability, and mucoadhesion properties which make them suitable for drug delivery.17 Apparently, researchers in literature already used nanoparticle carrier adjuvants composed of nontoxic natural polymers along with biologicals are best suited to extreme gastric and thermal conditions like body.18 Obviously, a natural carrier polymer, combined with a biologically active ingredient, can ensure both shelf stability and a prolonged therapeutic window for active pharmaceutical ingredient. Natural polymers are among the most popular options for encapsulating drugs for oral administration. Performing such research using traditional experimental approaches shall be time consuming and involves large investment's due to handling of biologicals. Alternatively, computational chemistry approach was used in our current research to explore the effects of temperature and pH on insulin-polymer system in the body and in storage conditions. However, the temperature of the body is approximately 37 degrees Celsius or 310 K, while insulin is generally stored at 6 degrees Celsius or 280 K.19 Hence computational studies were designed to consider temperature of storage at 280 K in basic pH and body temperature of 310 K along with acidic pH. Performing rational experimental studies is ideal once computational formulations of hormones have been assessed at the atomic level of accuracy.
Polymers such as pectin, dextran, chitosan, alginate, and cyclodextrin have been extensively studied in industrial drug delivery, so we used them for our formulation research. In addition, the choice of these polymers in this study was guided by the following literature. First polymer cyclodextrin study in wet lab research by Yang et al. showed that hydrogel-based microparticles grafted with carboxymethyl cyclodextrin had delivered oral insulin effectively.20 Our second polymer chitosan is made by deacetylating sea shrimp chitin using sodium hydroxide to generate a natural biopolymer.21 Frequently chitosan or its derivates had been evaluated for drug delivery research such as Wong Tin W. et al. showcased how chitosan can be used in the design of insulin delivery systems to address diabetes drug delivery.22 Then, dextran is a natural polysaccharide synthesised by lactic acid bacterial enzymes using sucrose as a starting material.23 Research is already reported as formulation study conducted by Kishore B Chalasani et al. indicated that vitamin B12-coated dextran nanoparticles could deliver insulin orally to animal models.24 Then, alginate, this anionic polysaccharide is derived from two copolymers: guluronic acid and mannuronic acid and is usually found in brown algae.25 By integrating internal gelation with alginate microspheres, Catarina M. Silva and colleagues demonstrate the development of alginate microspheres has promising insulin stability.26 Finally, pectin are acidic hetero polysaccharides that are found in plant cell walls. In food and pharmaceutical formulation, the main use is for jellying agents or nano formulation drug delivery. Special literature mention of Schwartz et al. whose team examined the effects of sustained pectin intake on glucose tolerance and gastric emptying in non-insulin-dependent diabetics.27 Apparently experimental study typically requires a longer period to set up and is quite costly so alternatively we can use computational formulations. Overall, the project aims to develop a computational formulation benchmarking study to test the effects of various polymers on insulin stability and to determine which polymers will be most effective in delivering biologicals.
A wide range of industries and academic institutions are already embracing computationally driven drug discovery, physics-based simulations are in use for regular research.28,29 By using computational formulations, rapid and cost-effective drug formulation can be achieved. In the following section, we highlight a few notable studies that have been conducted research by computational drug delivery. For example, a molecular modelling study was conducted by Pálma Bucur and colleagues evaluated the most suitable type of cyclodextrin derivative to form a complex with an insulin monomer.30 Erma Fatiha Muhammad et al. also investigated molecular modelling techniques for the monomer insulin–CD complex for insulin drug delivery.31 The general approach taken in most previous papers on computational formulation of insulin is based on using molecular docking and molecular dynamics to check drug delivery. Alternatively, in this study we had built the 5 polymeric system along with biologicals and checked for thermodynamic stability of biologicals. Our study evaluated effects with biologicals in the presence of polymers for thermostability and tracked the changes in physisorption and computational chemistry properties. As temperature and pH are known to have a significant influence on insulin fibrillation, hence we evaluated the thermodynamic stability of the insulin–polymer systems.32 To emphasis, this research paper performed a comparative bio-formulation analysis considering insulin stability in presence of different natural polymers as formulation adjuvant. Additionally, post simulation the trajectory data was used to assess insulin morphology stability via properties such as insulin's solvent accessible surface area (SASA), root mean square deviations (RMSD), root mean square fluctuations (RMSF), and radius of gyration (RG). Further separate energetics profile analysis was performed to access electrostatic, van der Waals energetics, inter and intra h-bonding analysis has provided in depth understanding on insulin stability.33 Considering the current research, we anticipate that other formulation scientists will be encouraged to use computational formulation properties to obtain regular atomic level details to make better rational decisions. Apparently, insulin is a protein molecule and maintaining its three-dimensional structure is critical for insulin to work effectively and safely in medical treatments. To summarize the workflow, we conducted this research study on insulin polymers and present the findings in two stages. Firstly, we evaluated the morphological biological properties of monomeric insulin and secondly, we assessed the molecular dynamics trajectory analysis of the computational formulation of insulin-polymer combo to address hormonal stability in storage and body temperature.
Results and discussion
In a computational analysis of insulin monomers, the morphology of insulin was evaluated in terms of molecular surface, ionization states, and hormonal thermal stability. The following paragraphs provide an overview of the results of our study.
Computational biology analysis of insulin
In this research computational biological properties such as protein aggregation surface analysis and protein–protein non-bonding interactions are analysed. An analysis of the protein insulin reveals that the monomer unit consists of chains A and B with a molecular weight of 5.70 Dalton. Protein aggregation, which is a major obstacle in the production and development of biologicals.34 Further, this phenomenon can take place at various stages, including manufacturing, storage, and administration, and may result in a range of problems such as decreased efficacy, immunogenicity, and safety issues. To develop biological therapeutics that are both safe and effective, it is essential to understand and regulate protein aggregation. As per outcomes in protein surface analyser in BioLuminate the protein accumulative positive surface area is the largest with a value of 1664.86 Å2. Further, the results showed all negative surfaces area is equal to sum of 1075.87 Å2. Moreover, the sum of donor surface area along with accumulative acceptor surface is equal to 559.66 Å2. Hence insulin is positively charged peptide with predominant patches of negative surfaces. Specifically hydrophobic exposure self-aggregation is observed in residue types of isoleucine, cysteine, serine, leucine, and tyrosine of chain A; and glutamine, leucine, tyrosine, valine, cystine, and glycine of chain B. Thus, we propose that amino acids part of aggregation patch of 10 to 15 in both chain A & B may play an important role in insulin protein self-aggregation.
Non-bonding interactions play a significant role in the recognition and binding of proteins to each other.35 Protein–protein hydrogen bonds interactions are formed between amino acid side chains or between amino acid side chains and the backbone of another protein. As per protein–protein interaction analysis on monomeric crystal structure of 1LPH that contains heteromeric A and B chains. Fig. 1 illustrates the interactions in both 2D and 3D views. As per through non-bonding interaction analysis between insulin heteromeric chains, we found that chain A with Tyr 19 has an H-bond with the B chain Phe 25, and chain Asn 21 has an H-bond with the B chain Gly 23. We also observed a significant H-bond network between amino acid residues Cys6, Cys7 in chain A and His5 and Leu6 in chain B. Furthermore, salt bridges or ionic interactions between oppositely charged amino acid side chains are also crucial interactions. They contribute to the stability of the protein–protein complex by providing electrostatic interactions between the proteins. In this protein–protein complex Asn 21 (chain A) and Arg 22 chain B form salt bridges that contribute to the affinity of both chains.
 |
| Fig. 1 (A) 2D and (B) 3D non-bonding Interactions (purple and yellow arrows) between monomeric insulin proteins chains A and B. | |
Ionization states of insulin amino acids as a function of pH
Proteins can be affected by changes in pH, which can impact their solubility and aggregation.36 The charge distribution of a protein can be influenced by the pH environment, particularly the ionization of side chains. Researchers must consider the ionization and protonation properties of amino acid residues in proteins to optimize their stability. By understanding the pH-dependent behaviour of proteins and the factors that impact their stability, researchers can create effective formulations and manufacturing processes that maintain protein stability and activity over time. To better understand this, we examined the protonation state of insulin amino acids under neutral pH to replicate storage conditions, and acidic pH for gastric environments. The protein preparation tool in Schrodinger suite helped to set environments for pH conditions. Further insulin pH patterns under both acidic (pH 1) and neutral (pH 7) conditions, we found that amino acid residues in both Chain A and Chain B were charged positively or negatively at neutral pH and acidic pH. Unique amino acids to acidic environments were found in Chain A residue Asn18 and Chain B residues His5, His10, Asn18, and Thr30. Based on our findings, we can hypothesize that protonation of amino acid residues such as Chain A (Asn18) and Chain B (His5, His10, Asn18, Thr30) which tend to change their protonation states upon exposure to environmental pH changes may play an important role in insulin fibrillation. The outcomes of all amino acid's protonation states are summarized in the Table 1 with lists of all the residue codes and their charge states.
Table 1 Amino acids changes in insulin monomeric protein–protein charge due to pH changes
S. no |
Insulin pH |
Protein chain name – charged amino acids, formal charge |
(Unique) protein chain name – charged amino acids, formal charge |
1 |
Neutral |
A-Gly1, −1 |
A-Asn21, −1 |
A-Asn21, −1 |
B-Phe1, −1 |
B-His5, 1 |
B-His5, −1 |
B-Arg22, −1 |
B-Lys28, −1 |
B-Thr30, −1 |
2 |
Acidic |
A-Gly1, 1 |
B-Glu21, −1 |
A-Glu4,−1 |
B-Thr30, −1 |
A-Glu17, −1 |
A-Glu4, −1 |
A-Asn21, −1 |
|
B-Phe1, 1 |
|
B-Glu13, −1 |
|
B-Glu21, −1 |
|
B-Arg22, 1 |
|
B-Lys28, 1 |
|
B-Thr30, −1 |
|
Monomeric insulin's thermophysical analysis
Thermophysical properties refer to physical attributes of biological substances that dictate their behaviour under varied thermal conditions, including temperature and pressure.37 Computational molecular dynamics techniques are employed to study these characteristics. The thermal expansion coefficient is an indicator of the extent to which a substance's dimensions alter with temperature. It is estimated as the fractional variation in length or volume of biological materials per unit temperature shift. Insulin types vary in their stability at different temperatures. It is crucial to comprehend how insulin behaves under different temperature settings to store it properly and enhance its therapeutic efficacy. We conducted a thermal hormone stability assessment to determine the effects of temperature on insulin fibrillation. We used the Schrodinger material science suite to perform molecular dynamics simulations between 200 K to 400 K at 5 K intervals to compute the coefficient of thermal expansion of insulin. Our simulation data was accumulated to plot insulin density associated with respective temperatures which are shown in Table 2 and Fig. 2. The graph indicates that insulin density has been greatly influenced in the region of 310 to 320 K, which is the temperature range where hormonal fibrillation is likely to occur. Our study suggests that higher temperatures, such as body temperatures of 310 K, may be a contributing factor to hormonal fibrillation.
Table 2 Thermodynamic thermal expansion of insulin at varying temperature
S. no |
Temperature (K) |
Insulin density (g cm−3) |
1 |
200 |
1.06965628 |
2 |
205 |
1.06881703 |
3 |
210 |
1.06766359 |
4 |
215 |
1.06632376 |
5 |
220 |
1.06396675 |
6 |
225 |
1.06168213 |
7 |
230 |
1.06005707 |
8 |
235 |
1.05766749 |
9 |
240 |
1.05467688 |
10 |
245 |
1.05163743 |
11 |
250 |
1.04853601 |
12 |
270 |
1.03453353 |
13 |
275 |
1.03089259 |
14 |
280 |
1.02650461 |
15 |
285 |
1.02319003 |
16 |
290 |
1.01887248 |
17 |
295 |
1.01457159 |
18 |
300 |
1.01041339 |
19 |
305 |
1.0056236 |
20 |
310 |
1.00172637 |
21 |
315 |
0.99642214 |
22 |
320 |
0.99148239 |
23 |
325 |
0.98688688 |
24 |
330 |
0.98174712 |
25 |
335 |
0.9770193 |
26 |
340 |
0.97157881 |
27 |
345 |
0.9655102 |
28 |
350 |
0.9611951 |
29 |
355 |
0.95481551 |
30 |
360 |
0.94915691 |
31 |
365 |
0.94416139 |
32 |
370 |
0.93834663 |
33 |
375 |
0.9320304 |
34 |
380 |
0.92622288 |
35 |
385 |
0.92015512 |
36 |
390 |
0.91315635 |
37 |
395 |
0.90627807 |
38 |
400 |
0.90018394 |
 |
| Fig. 2 Insulin thermal stability (Tg) temperature probability distribution. | |
Insulin–polymer formulation system molecular dynamics trajectory analysis
To emphasis high temperatures can cause insulin molecules to unfold, making them inactive or harmful hence, it's essential to understand the role of temperature in insulin stability when developing and storing insulin-based therapies. Perhaps factors like storage temperature, exposure time, and pH can all impact insulin stability. In this research using molecular dynamics simulations for 100 nanoseconds on polymers such as pectin, dextran, chitosan, alginate, and cyclodextrin along with insulin thermodynamically. Overall, 12 explicit molecular simulations of each 100 ns were done on insulin and insulin associated polymers was performed. Insulin polymer formulation summary after system building for molecular dynamics is showcased in Table 3. Molecular dynamics simulation representee frames polymeric formulations are shown in Fig. 3. All the molecular dynamics has great quality outcomes which has stable total energy and potential energy. As per system building of insulin-polymer formulations outcomes are summarized in Table 4. Where we noticed stable potential and total energy suggesting that molecular simulations ran with best quality (images in supplementary). Molecular modelling properties such as radius of gyration (RG), root mean square deviations (RMSD), root mean square fluctuations (RMSF), Solvent accessible surface area (SASA), simulation energetics covering van der Waals, electrostatic, inter & intra H-bonding and protein principal component analysis was carried out on molecular dynamics trajectory. The crucial parameters for assessing the stability of hormone peptides based on computational simulation-based on morphology and energy data of formulations systems are analysed and discussed below.
Table 3 The energetics of 100 ns molecular dynamics simulations for the assessment of quality
S. no |
Polymer |
Average total energy full (kcal mol−1) |
Average potential energy full (kcal mol−1) |
280 K |
1 |
Cyclodextrin |
−129536.455 |
−162868.875 |
2 |
Dextran |
−99457.449 |
−123029.718 |
3 |
Pectin |
−137506.379 |
−168875.921 |
4 |
Alginate |
−258164.548 |
−320502.506 |
5 |
Chitosan |
−302370.762 |
−376046.478 |
![[thin space (1/6-em)]](https://www.rsc.org/images/entities/char_2009.gif) |
310 K |
1 |
Cyclodextrin |
−154863.026 |
−202087.979 |
2 |
Dextran |
−84549.188 |
−110038.35 |
3 |
Pectin |
−113144.237 |
−145930.19 |
4 |
Alginate |
−231631.794 |
−302008.995 |
5 |
Chitosan |
−187755.335 |
−244709.695 |
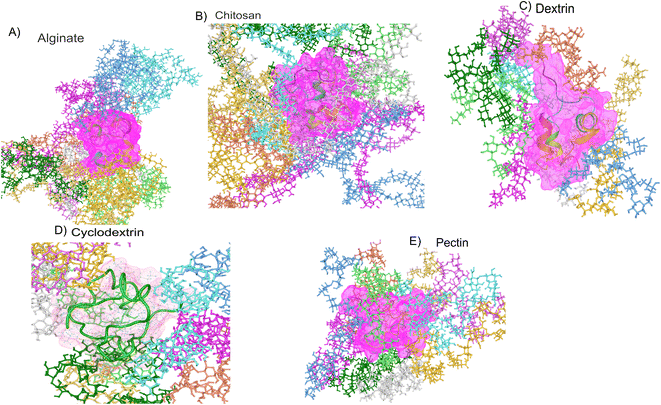 |
| Fig. 3 Representative polymer–insulin formulation frames of molecular dynamics simulation. | |
Table 4 Insulin polymer formulation summary after system building for molecular dynamics
S. no |
Component |
Molecular weight |
Number of polymer units in system |
Number of atoms in a monomer |
Total number of atoms in formulation |
1 |
Insulin |
5805.675 |
1 |
802 |
|
2 |
Cyclodextrin |
4856 |
10 |
622 |
75471 |
3 |
Dextran |
2514 |
10 |
322 |
54035 |
4 |
Pectin |
3844.545 |
10 |
422 |
71843 |
5 |
Alginate |
8326.36 |
10 |
882 |
109702 |
6 |
Chitosan |
7624.347 |
10 |
1027 |
130220 |
Insulin radius of gyration in polymer formulation
An ensemble's radius of gyration distance in molecular dynamics trajectory can be used to predict elemental level compatibility packing of a bio-formulation.38 In a varying thermodynamic simulation environment, a protein's radius of gyration (RG) is calculated as the sum of its principal moments. Thus, A radius of gyration is the distance between a rotation point and the system's overall potential energy. In our research molecular dynamics of formulation system along with RG is showcased in (Fig. 4). The study had calculations on radius of gyration of insulin in the presence of natural polymers was calculated using 100 ns bio-formulation simulations at 280 K and 310 K. The RG parameters can determine the stability of biologicals and polymer formulation systems. Further the analysis indicates that amorphous poly(alginate), amorphous poly(pectin), and amorphous poly(cyclodextrin) are relatively more stable. Furthermore, as per analysis we found at both 280 and 310 K, amorphous poly(alginate) maintained insulin RG of 10.0 Å. Furthermore, at 280 K, the amorphous poly(pectin) indicated a slight variation of 10.3 to 10.5 Å and at 310 K insulin showed values between 10.6 and 10.5 Å. Meanwhile, amorphous poly(dextran) and amorphous poly(chitosan) exhibited less stability as deviation RG increased. Amorphous poly(chitosan) had RG values between 10.2 and 10.5 Å at 280 K and between 10.00 and 10.5 Å at 310 K. At 280 K, amorphous poly(dextran) exhibited a declining RG from 10.65 to 10.2 Å, and at 310 K formulation showed an ascending insulin RG from 10.2 to 10.6 Å. The RG shall be important in determining the biological compactness of peptides where in the analysis amorphous poly(alginate) maintained least deviations in the range of 10.0 Å in both 310 K and 280 K.
 |
| Fig. 4 Insulin radius of gyration (RG) along with natural biopolymers at 280 and 310 K temperature. | |
Insulin residue root mean square fluctuations (RMSF)
Protein root mean square fluctuation in molecular dynamics is a metric that determines the average variation of individual amino acids side chains within a protein structure during a molecular dynamic's simulation.39 To compute RMSF, the root mean square of each atom's displacement from its first trajectory frame position relative to throughout the simulation of 100 ns. Moreover (Fig. 5), illustrate root mean square fluctuation for insulin amino acids with respect to temperature conditions. The RMSF per residue is typically plotted against the residue number, and it is useful for identifying which amino acids in a protein handle the protein's structural motion during formulation thermodynamics movements. Next best was poly(pectin) that provided a consistent environment to insulin, which indicated comparable trends in both the study temperatures. Moreover, poly(chitosan). As well, poly(alginate) maintained insulin with relatively lower fluctuations in 310 and 280 K. Data from simulations at 280 K indicate that chain A Gly1, Ile2, Cys20, and Val3 have the least RMSF. In contrast, chain B had Cys7, Asn3, Gly8, Pro29, and Thr30 have the highest fluctuations. However, the highest fluctuations occurred in chain A, Ile10, Asn18, Tyr14, Asn21, while in chain B, Glu21, Asn3. Additionally, the least amount of fluctuation was observed with Gly1, Ile2 in chain A and with Val3 in chain B. The fluctuations at 310 and 280 K were controlled with poly(chitosan) polymer being the most effective in retaining residues intact below 7.5 Å. Next best was poly(pectin) that provided a consistent environment to insulin, which indicated comparable trends in both the study temperatures. Thus, the RMSF analysis of insulin amino acids throughout the simulation will provide insight into the trends in amino acids fluctuations.
 |
| Fig. 5 Comparative amino acids Root Mean Square Fluctuations (RMSF) of monomeric insulin in formulation at (A) 280 and (B) 310 Kelvin with orange color (raw insulin), light green color (poly alginate), pink (poly pectin), blue (poly chitosan), poly dextran as orange color and dark green color (poly cyclodextrin). | |
Biologics protein backbone root mean square deviation
Molecular dynamics trajectory is analysed for protein Root Mean Square Deviation (RMSD) is commonly applied in molecular dynamics simulations to compare protein structures under different conditions or at different time points.40 By using RMSD analysis, researchers can effectively study protein structure and function, which can provide crucial insights for protein engineering, drug design, and other applications. Protein backbone root-mean-square deviation (RMSD) is a measure of the average distance between atoms on the backbone of a protein between a superimposed ensemble of proteins of the first frame. A similarity measure is commonly used to analyse macromolecular molecule structure and dynamics. With raw insulin and natural polymer loaded as formulation system, root mean square deviations (RMSD) were observed on insulin. Nearly 12 biopolymer formulation of insulin simulations lasting 100 ns were conducted at 280 K and 310 K showcased in (Fig. 6). All molecular dynamics observations were checked. According to the image, all bio-formulations were stable at 280 K, except for insulin, which showed a slight upward trend from 2.0 to 3.5 Å. To ensure that the simulation was consistent, perhaps replica runs of all polymer's formulations were conducted five times and were included in the ESI data.† The RMSD plot clearly showcased that alginate and dextran biopolymer formulations influenced insulin and achieved stability at 2.3 Å. Other formulations, such as poly(chitosan, pectin), also achieved insulin stability at 3 to 3.2 Å. Lastly, poly(cyclodextrin) reached stability at 3.5 Å. There was a range of deviations of insulin between 2 and 5.5 at 310 K, suggesting that insulin is unstable at this temperature. However, bio-formulation insulin exhibited a deviation range of 2 to 3.5 Å. Poly(chitosan) exhibited the lowest deviation within 2.4 to 2.0 Å. Poly(alginate, dextran, and pectin) had similar RMSDs within 2.5 to 3.0 Å. Finally, poly(cyclodextrin) exhibited the highest RMSD of 3.3 to 3.8 Å. Overall. At 280 and 310 K, all polymers indicated insulin stability, whereas raw insulin was relatively less stable without polymers support.
 |
| Fig. 6 Comprehensive protein backbone Root Mean Square Deviations (RMSD) line plot at (A) 280 K and (B) 310 K of insulin root mean square deviations plots in bioformulation where raw insulin (brown color), poly alginate (purple), polypectin (red). | |
Biologics solvent accessible surface area (SASA)
The molecular surface formed by water covering the van der Waals surface of a biomolecule is called Solvent accessible surface area or SASA.41 Less the SASA suggests the capacity of the polymer encapsulation on insulin. Thus, we obtain to know encapsulation of biologics via polymers. Protein folding and stability studies have always considered the SASA of proteins to be of vital importance. The polymers with least mean values in both temperatures of SASA were with chitosan and Pectin. Moreover, highest was with alginate and cyclodextrin. Interestingly standard deviations among all polymers were similar with range of 400 to 600 Å2. The violin plot in (Fig. 7) depicts that insulin solvent accessible surface area was between 3500 to 4000 Å2 at 280 K, and 3800 to 4800 Å2 at 310 K, compared with the reference native insulin. It should be noted that poly(pectin) had better efficiency in retaining the SASA between 1500 and 2000 Å2 at both temperatures. Furthermore, all other polymers (alginate, chitosan, cyclodextrin, dextran, pectin) were within a range of 1500 to 3500 Å2. This values clearly indicates evidence of polymer encapsulation ability with and without polymers. Table 5 summarizes the SASA summary statistics of protein all atoms as they move through the dynamic's simulation.
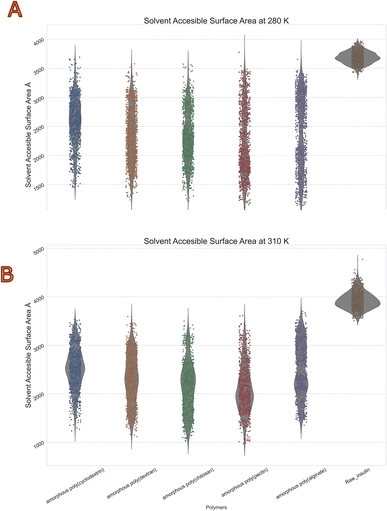 |
| Fig. 7 Consolidated solvent accessible surface area (Å2) violin plot of insulin in bio-polymer formulation at (A) 280 K and (B) 310 K. | |
Table 5 Summary statistics of insulin solvent accessible surface area (Å2) in diverse polymers
|
(Chitosan) Å2 |
(Cyclodextrin) Å2 |
(Dextran) Å2 |
(Alginate) Å2 |
(Pectin) Å2 |
Temperature (K) |
Mean |
2133.20 |
2520.87 |
2298.21 |
2509.651 |
2107.09 |
310 |
Std |
522.4148799 |
434.266877 |
515.19 |
556.11 |
487.72 |
Min |
1020.62541 |
1231.724751 |
1114.07 |
1349.10 |
970.79 |
Mean |
2321.476206 |
2589.353996 |
2346.45 |
2377.28 |
2218.96 |
280 |
Std |
459.2778638 |
419.0688639 |
485.35 |
646.80 |
597.109 |
Min |
1221.529827 |
1455.26749 |
1236.51 |
1143.66 |
930.87 |
Bio-polymer formulation simulation free energy profile
Computational energetics is a highly useful technique that aids in analysing the energetics of biological systems, thereby offering significant insights into the molecular mechanisms of biological processes. This information can be utilized to create better drugs and therapies, enhance protein engineering and biomanufacturing processes, and enrich our understanding of the fundamental principles of biology. Molecular dynamics simulations utilize classical mechanics to simulate the behaviour of a system over time, enabling researchers to investigate the dynamics and energetics of biological systems. This method is ideal for examining protein folding, protein–ligand binding, and other biological processes at a molecular level. After the simulated systems were subjected to an energetic analysis, the following results were obtained. In Fig. 8, we have summarized the results and consolidated the energy. This image contains two subsections, (A) demonstrating the results obtained at 280 K, and (B) showcasing the results obtained from the analysis of formulation systems at 310 K which showcases electrostatic and van der Waals energetics. At a temperature of 280 K, cyclodextrin displayed lower energetics in the formulation systems compared to other polymers. The electrostatic energy centroids of all polymers ranged from less than −250 kcal mol−1 to −650 kcal mol−1 with moderate stability. Both cyclodextrin and alginate showed the van der Waals energy of −150 kcal mol−1. Pectin, alginate, and chitosan displayed electrostatic energetics peaks ranging from −650 to −380 kcal mol−1. When the temperature was increased to 310 K, all polymers showed changes in their energetics patterns. Chitosan and pectin demonstrated great stability and minimal deviations in their energetics, with chitosan having electrostatic energy peaks of −400 kcal mol−1 and −250 kcal mol−1 Van der waals energy, followed closely by pectin, which exhibited −300 kcal mol−1 of electrostatic energy and −250 kcal mol−1 of van der Waals energetics. Binding free energy shall be great indicator as to access stability of insulin with various polymer. To determine which polymer is the most effective accommodative biological, it is important to consider the binding free energy of insulin in various polymer formulations. The transfer free energy or absolute free energy of insulin is calculated with and without formulation in a molecular dynamic's trajectory. As shown in Table 6, cyclodextrin had a pretty good binding energy for insulin transfer in both temperature regimes, with an average of −149.08 kcal mol−1 and −146.98 kcal mol−1. Except for cyclodextrin, all other polymers had fluctuating free energies. According to Fig. 9 which illustrates the total energy versus delta free energy of insulin in all polymer formulations at (A) 280 K and (B) 310 K. The binding free energy of cyclodextrin is among the top polymers whose bins are most with display least energy in the range of −100 to −200 kcal mol−1 for insulin, particularly at higher temperatures. It is important to note that at 280 K, all polymers responded to insulin with an average −100 kcal mol−1, but that as the temperature varies, cyclodextrin retained the least binding free energy. In general, all polymers bind negatively to insulin, but cyclodextrin is among the best polymers for accommodating insulin effectively.
 |
| Fig. 8 Molecular dynamics 100 ns insulin–polymer formulation energetics profile at (A) 280 K and (B) 310 K. | |
Table 6 Absolute free energy profiles of insulin and in various polymer formulations at 280 K and 310 K
S. no |
System of study |
Average total potential energy (kcal mol−1) |
Average free energy of insulin ΔG (kcal mol−1) |
Temperature (280 K) |
1 |
Insulin |
2680.70 |
NA |
2 |
Insulin in cyclodextrin formulation |
2829.78 |
−149.08 |
3 |
Insulin in dextran formulation |
2828.07 |
−147.37 |
4 |
Insulin in pectin formulation |
2756.21 |
−75.51 |
5 |
Insulin in alginate formulation |
2709.96 |
−29.26 |
6 |
Insulin in chitosan formulation |
2777.13 |
−96.43 |
![[thin space (1/6-em)]](https://www.rsc.org/images/entities/char_2009.gif) |
Temperature (310 K) |
1 |
Insulin |
2777.97 |
NA |
2 |
Insulin in cyclodextrin formulation |
2924.96 |
−146.98 |
3 |
Insulin in dextran formulation |
2837.91 |
−59.94 |
4 |
Insulin in pectin formulation |
2835.33 |
−57.35 |
5 |
Insulin in alginate formulation |
2886.18 |
−108.21 |
6 |
Insulin in chitosan formulation |
2805.04 |
−27.07 |
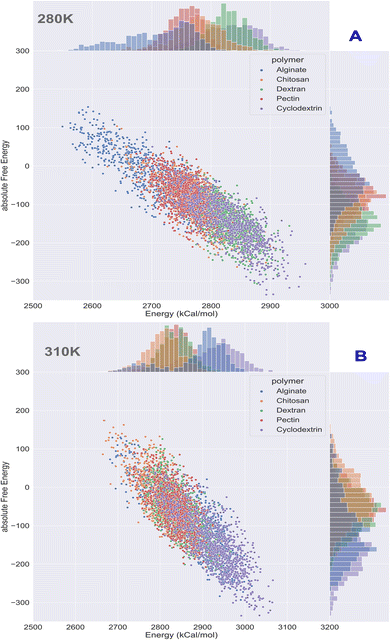 |
| Fig. 9 Binding energy analysis of insulin in polymeric formulation at (A) 280 K and (B) 310 K. | |
Insulin inter and intra H-bond and principal component analysis
Hydrogen bonds, both inter and intra, are crucial in stabilizing and determining the functionality of proteins. Inter H-bonds form between various parts of the protein, while intra H-bonds occur within a single peptide backbone. A study conducted on different types of polymers found that inter H-bonding with insulin was highest with dextran and lowest with cyclodextrin and chitosan. On the other hand, intra H-bonding analysis revealed that insulin had an average interaction of 100–120 counts with all natural polymers. Interestingly, the intra H-bond counts of insulin remained the same at higher temperatures, indicating the maintenance of predominant inter and intra H-bonding showcased in Fig. 10. Additionally, protein Principal Component Analysis (PCA) is a statistical technique commonly utilized for analysing molecular dynamics simulations. Through PCA, researchers can identify significant movements or alterations in protein structures that take place over time. Our investigation revealed that the cross-correlation matrix of PCA analysis for both 280 and 310 K remained largely consistent, with similar patterns, though the intensity of variance varied slightly. Overall, our findings suggest that the variance magnitude in protein PCA analysis was not particularly noticeable (image in the ESI data†).
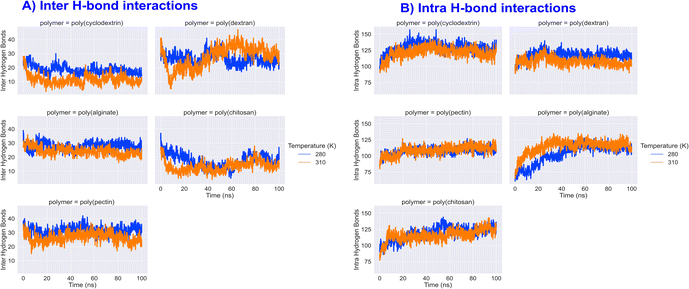 |
| Fig. 10 Formulation insulin inter and intra H-bond interactions counts during 100 ns molecular dynamics simulation. | |
Materials and methods
Computers and software
To conduct this study, we used following hardware and software; An Intel Xeon processor with 2.3 GHz, 32 GB of RAM, and a Quadro K620 GPU was used to perform this study on a PC with operating system running Linux Centos 6. Furthermore, NVIDIA Tesla K20 graphics cards were used to simulate the molecular dynamics of bio-formulations. Schrodinger Suite42 was used to solve all simulation tasks related to computational biology and materials science.2,43 We have generated the comparison plots using Python modules such as Matplotlib, Pyplot, Seaborn, and Schrodinger software.
Monomeric insulin preparation
Computational study used insulin molecule identified as 1LPH obtained from the protein data bank for molecular modeling purposes. Moreover, any crystal structure downloaded from pdb is recommended to preprocessed, as our X-ray PDB structures required to assign bond order, add hydrogens. Further we retained only monomeric units of insulin chains A & B. To optimize H-bonds assignments and maintain protein pH, it is necessary to create a highly acidic environment where ASP, GLU, and HIS can protonate, as well as a neutral pH condition with ProPka. All the tasks were performed by Schrodinger protein preparation wizard, that simplifies the protein preparation process.44 The workflow has prepared protein to be minimized to converge heavy atoms to 0.3 Å, while keeping the experimental coordinates intact. We used the OPLS4 Force Field for our molecular modeling tasks. To ensure the protein is properly minimized prior to using it for any study, the potential energy of the structure is checked for negative values to ensure steric clash absence and energetically fit.
Protocols for protein aggregation surface and pH protonation states
Fully prepared Insulin's pdb (protein data bank) id 1LPH monomer computational biological properties were calculated using a protein surface analyser (Schrödinger Release 2022-3: BioLuminate).45 As it has inbuilt analysis panels to visualize qualitative and quantitative outcomes on aggregations outcomes. As a result of our computational formulation study, insulin was required to have both an acidic and neutral pH. In the refine tab, the program could modify residue ionization or tautomer states to optimize hydrogen bond networks by selecting the “Use PROPKA” settings. Furthermore, the program used relative penalties for different protonation states based on estimates of pKa. As a result, these options have been extensively used to simulate insulin in neutral and acidic pH environments.
Thermophysical properties of insulin
We used the monomeric chain of insulin 1LPH (ref. 46 and 47) in Schrodinger material science suite (2021). To be able to understand insulin thermophysical properties, we carried out a series of molecular dynamics simulations in an NPT ensemble in Desmond.48–50 This was done to arrive at a converged density of insulin molecules obtained during molecular dynamics simulation in this research. The output was a plot as a function of temperature in the 200 to 400 K range with 5 K interval, we examined the density of the hormonal peptide simulation system. The simulation data was used as a function of the thermal expansion of the hormonal peptide simulation system at all temperatures. The output of trajectory is used to generate histogram plot to assess insulin fibrillations.
Polymer building
Each monomeric unit of polymer is sketched as per PubChem description. First sketched monomeric units of polymers analysed are minimised in terms of conformational energy. We used the macromodel tool for polymer conformational search that utilizes the OPLS 4 force field.51 The tool used energy minimization of polymers with a specific method called truncated newton conjugate gradient minimization protocol from macromodel. Moreover, this method involved 2500 iterations with 0.05 kcal energy-based convergence. Additionally, the settings were to set for systematic torsional sampling technique with an energy window to save the structure coordinates at 5.02 kcal mol−1. Molecular mechanics optimized monomers of polymers The quantum mechanical properties of polymers computed were used to fit the force field parameters in OPLS force field builder. Using Jaguar, the least energy structure conformer was calculated quantum mechanically.52 Settings used density functional theory with B3LYP -D3 and basis set for the atoms was 6-31G with 100 iterations before a convergence energy threshold of 5.0 × 10−5 Eh. We optimized the polymer from monomer units by using the polymer builder and macromodel for all atom molecular dynamics. The linear polymers used in our study were derived from monomeric units of pectin, dextran, chitosan, alginate, and cyclodextrin. The central core of the polymer monomeric unit was used to create the initiator and terminator based on default software settings. The software has options create amorphous cubic cell consisting of multiple polymer chains, with varying compositions of polymers that required further stabilization and formulation with a biological molecule of interest.
Disordered system building
We constructed a bioformulation using the natural polymer and insulin prepared in the preceding steps using the disordered system builder in MS suite (Schrodinger Material science Suite). A formulation system was constructed using 10 units of polymer, one unit of insulin, and the appropriate water solvent. Specifically, minimal insulin was used as a substrate with an appropriate number of polymers to submerge biological systems. As part of the preparation of formulation systems, the force field OPLS4 and amorphous state were considered, along with Monte Carlo simulation of annealing energy and steric pack minimization. A disordered system is directly appropriate for use in molecular dynamics production runs. All formulations resulting in a disordered system were further subjected to 100 ns of storage at 280 K and 310 K for 100 seconds using MS multistage molecular dynamics panel.
Molecular dynamics using multiple multistage workflow
A material science multistage molecular dynamics simulation workflow is comprised of three different stages, as explained below. Firstly, material relaxation, then the relaxation by Brownian minimization protocol, and then production explicit molecular dynamics of 100 ns. The workflow has special settings to address materials relaxation protocol in polymer science applications. During the materials relaxation process, the temperature and pressure of the simulation ensemble are set by using a Noose–Hover chain thermostat and Martyna–Tobias–Klein barostat for maintaining pressure theoretically. This simulation algorithm includes a NVT ensemble along with Brownian minimization for 20 ps at 10 K, followed by a NPT Brownian minimization for 20 ps at 100 K, and then a 100 ps NPT MD stage at 300 K. A separate max simulation time of 100 ps will be given for the Brownian motion simulation in order to facilitate system relaxation. In the third stage, Desmond has performed molecular dynamics simulations using a protocol that permits simulations of molecular systems to be conducted using multistage simulation workflow. The output of Brownian minimization is used as an input for molecular dynamics simulations. In this study, we have simulated 12 systems and analysed them based on their morphological properties as per morphological properties of insulin. The workflow uses an 8 stage stabilization phase protocol to relax the system, before performing a production run for the NPT ensemble of each 100 ns full system. The final analysis of the trajectory is done separately.
Thermodynamics properties of insulin formulations
Each formulation system trajectory data was subjected to analysis with option in the trajectory player to generate respective properties using plot properties over trajectory option. Later all data was merged for plotting for comparative analysis using Seaborn and Matplotlib python libraries. The molecular dynamics data was used All properties, such as RMSD, RMSF, SASA, RG of insulin protein were calculated using Maestro GUI.53 Energetics analysis of two systems that is protein and polymers were performed using specialized scripts. As part of our study, we computed interaction energies between polymers and proteins using the analyze_simulation.py script within a Desmond trajectory system. Results were compared by temperature for all polymers and discussed in the results section bio-polymer energetic analysis. The molecular dynamics trajectory of Desmond is used to calculate the transfer free energy or absolute free energy of insulin. For each trajectory, 1000 frames were analysed using OPLS4 with a special energy analysis script produced by maestro for scoring insulin's total energy (bonded and unbonded terms). To arrive at the total energy, we subtracted each frame of raw insulin total energy without formulation polymer from respective frames of insulin total energy in formulation. This resulted in the transfer free energy or the absolute free energy of insulin. Insulin's affinity for polymers can be determined by these values.
Conclusions
To sum up, our analysis of 5.70 Dalton insulin's monomer unit with two chains, A and B. We found the protein had a patch of residues including isoleucine, cysteine, serine, leucine, tyrosine in chain A and glutamine, leucine, tyrosine, valine, cystine, and glycine in chain B are critical for the protein's self-aggregation and stability. Hence this residues shall be taken care to avoid exposure to hydrophilic environment to avoid fibrillations. We observed a significant H-bond network between amino acids Cys6, Cys7 in chain A and His5 and Leu6 in chain B. We also observed that amino acids, such as Chain A residue Asn18 and Chain B residues His5, His10, Asn18, and Thr30, were unique to the acidic environment due to which affinity between chains may vary. Moreover, as per the thermal stability of insulin through molecular dynamics simulations in considering the coefficient of thermal expansion. Where the simulations at regular interval between 200 K and 400 K showed that insulin density varies most between 310 K and 320 K, indicating that insulin fibrillation is more likely to occur at higher temperatures.
The molecular simulations analysis of insulin's Radius of Gyrations at 280 K and 310 K showed that amorphous poly(alginate) maintained a stable RG of 10.0 Å at both temperatures. Amorphous poly(pectin) showed a slight variation in RG, ranging from 10.3 to 10.5 Å at 280 K and between 10.6 to 10.5 Å at 310 K. On the other hand, amorphous poly(dextran) and amorphous poly(chitosan) were less stable as their gyration radius increased compared to other polymers. Amorphous poly(alginate) was the most stable, showing the least deviation in the range of 10.0 Å at both 310 K and 280 K. Furthermore, the RMSF analysis provided insight into the trends in amino acid fluctuations. Poly(alginate), poly(pectin), and poly(chitosan) maintained insulin with relatively lower fluctuations at 310 and 280 K. The data from simulations at 280 K indicated that chain A Gly1, Ile2, Cys20, and Val3 had the least RMSF, while chain B had Cys7, Asn3, Gly8, Pro29, and Thr30 with the highest fluctuations. Thus for 310 and 280 K, poly(chitosan) polymer was found to be most effective in maintaining residues as it maintained insulin fluctuations below 7.5 Å, followed by poly(pectin). The simulation data SASA analysis suggests that chitosan and pectin exhibited the least mean values at both temperatures, while alginate and cyclodextrin showed the highest for insulin. Overall the insulin SASA was between 3500 and 4000 Å2 at 280 K and 3800 to 4800 Å2 at 310 K, compared to native insulin. Poly(pectin) was efficient in retaining SASA between 1500 and 2000 Å2 at both temperatures. All other polymers (alginate, chitosan, cyclodextrin, dextran, pectin) had a range of 1500 to 3500 Å2. These values demonstrate evidence of polymer encapsulation ability with and without polymers greatly varies. The standard deviations among all polymers were similar, ranging from 400 to 600 Å2. As per analysis on RMSD data we found that all biopolymer formulations were stable at 280 K, except for raw insulin without polymer encapsulation, which showed a slight upward or unstable trend. At 310 K, again raw insulin was unstable, but bio-formulation insulin exhibited a deviation range of 2 to 3.5 Å with sustained stability. Poly(chitosan) had the lowest deviation, while poly(cyclodextrin) exhibited the highest RMSD. Overall, at both temperatures, all polymers supported insulin stability, whereas raw insulin was found to be unstable. As per intra and inter insulin H-bonding and PCA analysis we were able to see that all polymers relatively kept the bioformulations intact as per non-bonding interaction counts and energetics is concerned. The results of analysis of the energetic profile of formulation systems at 280 K and 310 K indicated that cyclodextrin displayed lower energetics than other polymers at 280 K. However, at 310 K, all polymers displayed changes in their energetics patterns, with chitosan and pectin displaying the greatest stability. It has been shown that chitosan has electrostatic energy peaks ranging from −400 kcal mol−1 to −250 kcal mol−1 van der Waals energy. Additionally, pectin exhibited electrostatic energy of −300 kcal mol−1 and van der Waals energy of −250 kcal mol−1. A more stable insulin formulation was achieved by using chitosan as its energetics were relatively stable in both temperatures. In comparison with all other polymers, insulin's absolute free energy in cyclodextrin was among the best at both temperatures.
Concluding, we sought to demonstrate throughout computational study as how molecular modelling properties could be incorporated into bioformulation to gain an understanding of the packing of biologics on an atomic level using molecular modelling approaches. Due to molecular simulation of formulation systems, we got sufficient research data to extrapolate our understanding of polymers on insulin. Hopefully, our study will provide guidelines to other researchers on how to approach such interdisciplinary research on computational formulation of biologics. We have found that biologics that are biologics encapsulated in polymers exhibit greater stability than those that are not encapsulated insulin. Specifically, our study indicates that natural polymers have the potential to maintain the stability of insulin during storage and under gastric conditions in the stomach. However, it is imperative that more rigorous studies be conducted using hybrid polymers to address the issue of insulin's oral bioavailability. We encourage researchers to use computational techniques to gain a deeper understanding of oral drug delivery, which can aid in the identification of optimal polymers and the formulation of compatible strategies for better patient compliance.
Author contributions
All authors took part in (a) conception and design, or analysis and interpretation of the data; (b) drafting the article or revising for important intellectual content; and (c) approval of the definitive version.
Conflicts of interest
The authors declare that there are no conflicts of interest associated with the research.
Acknowledgements
We would like to express my gratitude to Mr Raghu R, Dr Pritesh Bhat, Dr Sudharsan Pandiyan, and Dr Alexander Goldberg for their suggestions and discussions. The study would like to express its appreciation to Schrodinger software for its continuous support of software accessibility throughout the study.
References
- A. Baumann, Curr. Drug Metab., 2005, 7, 15–21 CrossRef PubMed.
- A. N. Zelikin, C. Ehrhardt and A. M. Healy, Nat. Chem., 2016, 11, 997–1007 CrossRef PubMed.
- F. Yakushiji, Yakugaku Zasshi, 2022, 142, 1–7 CrossRef CAS PubMed.
- D. C. Whitcomb and M. E. Lowe, Dig. Dis. Sci., 2007, 52, 1–17 CrossRef CAS PubMed.
- R. Kannan and A. Przekwas, Int. J. Numer. Method Biomed. Eng., 2020, 36, e3403 Search PubMed.
- Y. Cao, P. Rewatkar, R. Wang, S. Z. Hasnain, A. Popat and T. Kumeria, Trends Pharmacol. Sci., 2021, 42, 957–972 CrossRef CAS PubMed.
- D. Christensen, L. Bøllehuus Hansen, R. Leboux, W. Jiskoot, J. P. Christensen, P. Andersen and J. Dietrich, ACS Nano, 2019, 13, 1116–1126 CAS.
- M. Goodman, Nat. Rev. Drug Discovery, 2009, 11, 837 CrossRef PubMed.
- J. P. Palmer, C. M. Asplin, P. Clemons, K. Lyen, O. Tatpati, P. K. Raghu and T. L. Paquette, Science, 1983, 222, 1337–1339 CrossRef CAS PubMed.
- R. N. Brogden and R. C. Heel, Drugs, 1987, 34, 350–371 CrossRef CAS PubMed.
- A. D. Association, Diabetes Care, 2004, 27, s106–s107 CrossRef PubMed.
- E. W. Gregg, N. Sattar and M. K. Ali, Lancet Diabetes Endocrinol., 2016, 4, 537–547 CrossRef PubMed.
- W. Leobandung, H. Ichikawa, Y. Fukumori and N. A. Peppas, J. Controlled Release, 2002, 80, 357–363 CrossRef CAS PubMed.
- T. T. Andreassen, K. Seyer-Hansen and A. J. Bailey, Biochim. Biophys. Acta, Gen. Subj., 1981, 677, 313–317 CrossRef CAS PubMed.
- H. Iyer, A. Khedkar and M. Verma, Diabetes, Obes. Metab., 2010, 12, 179–185 CrossRef CAS PubMed.
- S. Sharma, A. Mittal and A. Mehra, Pharm. Pat. Anal., 2022, 11(6), 199–212 CrossRef CAS PubMed.
- Y. Y. Luo, X. Y. Xiong, Y. Tian, Z. L. Li, Y. C. Gong and Y. P. Li, Drug Delivery, 2015, 23, 1882–1891 Search PubMed.
- S. K. Panigrahy and A. Kumar, J. Biomater. Sci., Polym. Ed., 2022, 33, 2145–2164 CrossRef CAS PubMed.
- J. Brange and L. Langkjoer, Pharm. Biotechnol., 1993, 5, 315–350 CAS.
- Y. Yang, Y. Liu, S. Chen, K. L. Cheong and B. Teng, Carbohydr. Polym., 2020, 246, 116617 CrossRef CAS PubMed.
- S. (Gabriel) Kou, L. M. Peters and M. R. Mucalo, Int. J. Biol. Macromol., 2021, 169, 85–94 CrossRef CAS PubMed.
- T. Wong, Recent Pat. Drug Delivery Formulation, 2009, 3, 8–25 CrossRef CAS PubMed.
- T. Heinze, T. Liebert, B. Heublein and S. Hornig, Adv. Polym. Sci., 2006, 205, 199–291 CrossRef CAS PubMed.
- K. B. Chalasani, G. J. Russell-Jones, A. K. Jain, P. V. Diwan and S. K. Jain, J. Controlled Release, 2007, 122, 141–150 CrossRef CAS PubMed.
- K. Y. Lee and D. J. Mooney, Prog. Polym. Sci., 2012, 37, 106–126 CrossRef CAS PubMed.
- C. M. Silva, A. J. Ribeiro, I. V. Figueiredo, A. R. Gonçalves and F. Veiga, Int. J. Pharm., 2006, 311, 1–10 CrossRef CAS PubMed.
- S. E. Schwartz, R. A. Levine, R. S. Weinstock, S. Petokas, C. A. Mills and F. D. Thomas, Am. J. Clin. Nutr., 1988, 48, 1413–1417 CrossRef CAS PubMed.
- G. Sliwoski, S. Kothiwale, J. Meiler and E. W. Lowe, Pharmacol. Rev., 2014, 66, 334–395 CrossRef PubMed.
- H. Kubinyi, Curr. Opin. Drug Discovery Dev., 1998, 1, 16–27 CAS.
- P. Bucur, I. Fülöp and E. Sipos, Molecules, 2022, 27, 465 CrossRef CAS PubMed.
- (15) (PDF) Computational Investigation of the Monomer Insulin-β-CD Complex for the New Insulin Formulation, https://www.researchgate.net/publication/284180328_Computational_Investigation_of_the_Monomer_Insulin-b-CD_Complex_for_the_New_Insulin_Formulation, accessed 23 October 2022 Search PubMed.
- V. A. Koivisto, S. Fortney, R. Hendler and P. Felig, Metabolism, 1981, 30, 402–405 CrossRef CAS PubMed.
- J. D. Durrant and J. A. McCammon, BMC Biol., 2011, 9, 1–9 CrossRef PubMed.
- A. Morriss-Andrews and J. E. Shea, Annu. Rev. Phys. Chem., 2015, 66, 643–666 CrossRef CAS PubMed.
- G. Rabbani, M. H. Baig, K. Ahmad and I. Choi, Curr. Protein Pept. Sci., 2018, 19, 948–957 CrossRef CAS PubMed.
- V. Z. Spassov and L. Yan, J. Comput. Chem., 2016, 37, 2573–2587 CrossRef CAS PubMed.
- R. C. Bernardi, M. C. R. Melo and K. Schulten, Biochim. Biophys. Acta, 2015, 1850, 872–877 CrossRef CAS PubMed.
- R. Funari, N. Bhalla and L. Gentile, ACS Meas. Sci. Au, 2022, 2, 547–552 CrossRef CAS PubMed.
- P. Śledź and A. Caflisch, Curr. Opin. Struct. Biol., 2018, 48, 93–102 CrossRef PubMed.
- K. Sargsyan, C. Grauffel and C. Lim, J. Chem. Theory Comput., 2017, 13, 1518–1524 CrossRef CAS PubMed.
- H. Chen and A. Z. Panagiotopoulos, Langmuir, 2019, 35, 2443–2450 CrossRef CAS PubMed.
- Schrödinger Release 2022-3: Desmond Molecular Dynamics System, D. E. Shaw Research, New York, NY, 2023, Maestro-Desmond Interoperability Tools, Schrödinger, New York, NY, 2022 Search PubMed.
- A. D. Bochevarov, E. Harder, T. F. Hughes, J. R. Greenwood, D. A. Braden, D. M. Philipp, D. Rinaldo, M. D. Halls, J. Zhang and R. A. Friesner, Int. J. Quantum Chem., 2013, 113, 2110–2142 CrossRef CAS.
- G. M. Sastry, M. Adzhigirey, T. Day, R. Annabhimoju and W. Sherman, J. Comput. Aid. Mol. Des., 2013, 27(3), 221–234 CrossRef PubMed.
- BioLuminate, Schrödinger, LLC, New York, NY, 2021 Search PubMed.
- RCSB PDB – 1LPH: LYS, (B28)PRO(B29)-Human insulin, https://www.rcsb.org/structure/1LPH, accessed 20 April 2023 Search PubMed.
- E. Ciszak, J. M. Beals, B. H. Frank, J. C. Baker, N. D. Carter and G. D. Smith, Structure, 1995, 3, 615–622 CrossRef CAS PubMed.
- F. C. Ezebuo and I. C. Uzochukwu, J. Biomol. Struct. Dyn., 2020, 995–1009 Search PubMed.
- K. J. Bowers, D. E. Chow, H. Xu, R. O. Dror, M. P. Eastwood, B. A. Gregersen, J. L. Klepeis, I. Kolossvary, M. A. Moraes, F. D. Sacerdoti, J. K. Salmon, Y. Shan and D. E. Shaw, 2007, 43.
- D. E. Shaw, M. M. Deneroff, R. O. Dror, J. S. Kuskin, R.
H. Larson, J. K. Salmon, C. Young, B. Batson, K. J. Bowers, J. C. Chao, M. P. Eastwood, J. Gagliardo, J. P. Grossman, C. R. Ho, D. J. Lerardi, I. Kolossváry, J. L. Klepeis, T. Layman, C. McLeavey, M. A. Moraes, R. Mueller, E. C. Priest, Y. Shan, J. Spengler, M. Theobald, B. Towles and S. C. Wang, Commun. ACM, 2008, 51, 91–97 CrossRef.
- C. Lu, C. Wu, D. Ghoreishi, W. Chen, L. Wang, W. Damm, G. A. Ross, M. K. Dahlgren, E. Russell, C. D. Von Bargen, R. Abel, R. A. Friesner and E. D. Harder, J. Chem. Theory Comput., 2021, 17, 4291–4300 CrossRef CAS PubMed.
- A. D. Bochevarov, E. Harder, T. F. Hughes, J. R. Greenwood, D. A. Braden, D. M. Philipp, D. Rinaldo, M. D. Halls, J. Zhang and R. A. Friesner, Int. J. Quantum Chem., 2013, 113, 2110–2142 CrossRef CAS.
- Maestro, Schrödinger, LLC, New York, NY, 2021 Search PubMed.
|
This journal is © The Royal Society of Chemistry 2023 |
Click here to see how this site uses Cookies. View our privacy policy here.