DOI:
10.1039/D3RA01761C
(Paper)
RSC Adv., 2023,
13, 13971-13984
Exploring the therapeutic mechanisms of Gleditsiae Spina acting on pancreatic cancer via network pharmacology, molecular docking and molecular dynamics simulation†
Received
17th March 2023
, Accepted 19th April 2023
First published on 9th May 2023
Abstract
Pancreatic cancer is one of the most aggressive tumors and also has a low survival rate. The dried spines of Gleditsia sinensis Lam are known as “Gleditsiae Spina” and they mostly contain flavonoids, phenolic acids, terpenoids, steroids, and other chemical components. In this study, the potential active components and molecular mechanisms of Gleditsiae Spina for treating pancreatic cancer were systematically revealed by network pharmacology, molecular docking and molecular dynamics simulations (MDs). RAC-alpha serine/threonine-protein kinase (AKT1), cellular tumor antigen p53 (TP53), tumor necrosis factor α (TNFα), interleukin-6 (IL6) and vascular endothelial growth factor A (VEGFA) were common targets of Gleditsiae Spina, human cytomegalovirus infection signaling pathway, AGE-RAGE signaling pathway in diabetic complications, and MAPK signaling pathway were critical pathways of fisetin, eriodyctiol, kaempferol and quercetin in the treatment of pancreatic cancer. Molecular dynamics simulations (MDs) results showed that eriodyctiol and kaempferol have long-term stable hydrogen bonds and high binding free energy for TP53 (−23.64 ± 0.03 kcal mol−1 and −30.54 ± 0.02 kcal mol−1, respectively). Collectively, our findings identify active components and potential targets in Gleditsiae Spina for the treatment of pancreatic cancer, which may help to explore leading compounds and potential drugs for pancreatic cancer.
1. Introduction
Pancreatic cancer, specifically pancreatic ductal adenocarcinoma (PDAC), is among the most lethal forms of cancer and its prevalence is on the rise. The five-year survival rate for pancreatic cancer is below 5%.1,2 The majority of patients are diagnosed with advanced-stage disease that cannot be treated surgically, resulting in a life expectancy of typically less than six months.3,4 Pancreatic cancer is a multifactorial disease that arises from a combination of factors. Genetic factors play a role in the development of pancreatic cancer through direct effects or by interacting with environmental and epigenetic factors. Additionally, disorders of the pancreas such as chronic pancreatitis and diabetes, as well as certain systemic diseases, may expedite the progression of pancreatic cancer.5 These factors interact in a complex manner in the development of pancreatic cancer, and further research is needed to fully understand their intrinsic relationships and how they collaborate in the disease process. Despite the fact that surgical resection, chemotherapy, and chemo-radiotherapy have been shown to improve survival rates in pancreatic cancer patients, the prognosis for this disease remains extremely poor.6,7 Therefore, there is an urgent need for innovative therapeutic approaches for pancreatic cancer. Recent research suggests that the consumption of natural products may be associated with a reduction in the incidence of various diseases, including inflammation, immune disorders, and tumors.8,9 Therefore, the development of cancer prevention and treatment strategies based on natural products has the potential to enhance the quality of life of individuals with pancreatic cancer.
Gleditsiae Spina, which refers to the dried spines of Gleditsia sinensis Lam, is known by various names in China, including Zao Ci, Zao Jiao, and Tian Ding.10 Gleditsiae Spina has been traditionally used as an herbal remedy or formula for the treatment of colon, lung, and liver cancers.11 The primary chemical constituents of Gleditsiae Spina comprise a diverse array of compounds, including flavonoids, lignans, coumarins, terpenoids, steroids, phenolic acids, and saponins.12 Several flavonoids present in Gleditsiae Spina extract have been shown to possess anti-cancer, anti-bacterial, anti-necrotic, immunomodulatory, and anti-inflammatory properties.13 Fisetin, a key bioactive compound found in Gleditsiae Spina, has been demonstrated to play a significant role in the treatment of pancreatic cancer. Specifically, it induces DNA damage, inhibits NF-κB and PI3K/AKT/mTOR signaling, and triggers autophagy in pancreatic cancer cells.14–17 Quercetin is presently employed as an alternative or adjuvant therapeutic agent for pancreatic cancer treatment due to its ability to influence autophagy, cell growth, oxidative stress, apoptosis, epithelial–mesenchymal transition (EMT), and the sensitivity of chemotherapeutic agents.18 Kaempferol, a flavonoid found in Gleditsiae Spina, induces ROS-dependent apoptosis in pancreatic cancer cells by targeting tissue transglutaminase (TGM2)-mediated Akt/mTOR signaling pathway.19 However, the main active components and molecular mechanisms of Gleditsiae Spina against pancreatic cancer have not been fully elucidated.
Molecular docking is a widely employed approach in drug discovery and development, utilized to evaluate the binding of potential ligands with proteins.20 However, the docking codes, which are the backbone of virtual screening techniques, are founded on a series of simplifications that hinder a direct extrapolation to biological contexts. There exist additional physical–chemical properties, such as solubility, pKa, and log
P, that could influence the path that a ligand needs to traverse before reaching its target.21 Moreover, given the dynamic nature of protein–ligand binding in vivo, it is necessary to incorporate a preliminary stage of molecular dynamics (MD) to generate trajectories of the target.22 A set of representative structures can be selected for conducting MD simulations, which can also be used to verify the stability of the binding pose. Additionally, the use of more advanced theoretical approaches, such as ab initio or multiscale Quantum Mechanical/Molecular Mechanical (QM/MM), is of benefit to highlighting the compounds with therapeutic potential.23 Consequently, it is crucial to enhance both the accuracy and scrutiny of the resulting outputs.
This paper employs a comprehensive approach utilizing network pharmacology, molecular docking, and molecular dynamics simulations (MDs) to investigate the therapeutic mechanisms by which Gleditsiae Spina may act on pancreatic cancer.
2. Methods
2.1. Screening for potential active ingredients and targets of Gleditsiae Spina
The Traditional Chinese Medicine Systematic Pharmacology Database and Analysis Platform (TCMSP) was utilized to identify the bioactive components of Gleditsiae Spina, using the Chinese name “Zhaojiaoci” as the search term.24 Oral bioavailability (OB) is defined as the proportion of a drug that enters the systemic circulation following absorption through the gastrointestinal tract.25 Drug-likeness (DL) reflects the pharmacokinetic profile of compounds in humans. It is an important indicator for predicting the druggability and safety of potential drug candidates.26 We used OB ≥ 30% and DL ≥ 0.18 as criteria to screen potential active ingredients. To obtain the targets of these active ingredients, we utilized both the DRUGBANK database (https://www.drugbank.ca) and the Swiss Target Prediction database (http://www.swisstargetprediction.ch).27 For swisstarget, include data with probability * > 0.
2.2. Screening of pancreatic cancer related targets
Targets relevant to pancreatic cancer were obtained from the GeneCards Database (https://www.genecards.org), Therapeutic Target Database (http://db.idrblab.net/ttd/), and OMIM Database (https://www.omim.org). The keyword “pancreatic cancer” was used to search for genes, and the species was set as human to collect the relevant target information.28,29 The screening genes were standardized using the Uniprot KB in the UniProt database (https://www.uniprot.org) and the NCBI gene database (https://www.ncbi.nlm.nih.gov).
2.3. A protein–protein interaction (PPI) network construction
We utilized E Venn (http://www.ehbio.com/test/venn/#/) to analyze the candidate targets of Gleditsiae Spina and the potential targets of pancreatic cancer. The intersecting genes were considered potential targets of Gleditsiae Spina for pancreatic cancer. These intersecting genes were further analyzed using the STRING database (https://cn.string-db.org/) for PPI network analysis. Cytoscape 3.7.1 software was used for the construction and visualization of the PPI network analysis. The importance of the core targets was examined using CytoNCA, which is a network topology analysis plugin for Cytoscape. The “Degree, Betweenness, Closeness” values were used to assess the importance of the core targets.
2.4. GO function enrichment and KEGG pathway enrichment analysis
Gene Ontology (GO) function enrichment and Kyoto Encyclopedia of Genes and Genomes (KEGG) pathway enrichment analysis were performed using the DAVID database (https://david.ncifcrf.gov/).30 Biological Process (BP), Molecular Function (MF), and Cellular Component (CC) analyses are all included in the GO enrichment analysis. The KEGG database is a powerful bioinformatics tool for exploring the extent of gene enrichment in various signaling pathways.31 The false discovery rate (FDR) was set to be less than 0.05, and the significance level was set to be P < 0.05. To visualize the enrichment results, we used a bioinformatics online mapping website (http://www.bioinformatics.com.cn/) to generate a gradient color bar and a Sankey dot pathway enrichment analysis.
2.5. ADME properties prediction
The development and design of medications are heavily reliant on the evaluation of molecular characteristics related to absorption, distribution, metabolism, and excretion (ADME).32 The selected compounds were evaluated using the SwissADME online tool (http://www.swissadme.ch/) to assess their total polar surface area (TPSA), water solubility, gastrointestinal (GI) absorption, and to identify Lipinski, Ghose, Veber, Egan, and Muegge violations in order to exclude interfering substances.33
2.6. Construction of the compounds–targets–pathway–pancreatic cancer network
The Cytoscape 3.7.1 software was used to construct a compound–target–pathway–pancreatic cancer network based on the findings from the DAVID database. This network provided a clear understanding of the properties of the various components, targets, and pathways of Gleditsiae Spina.
2.7. Molecular docking
The purpose of molecular docking is to predict the binding pattern and affinity of ligand molecules to protein receptors. AutoDock is a protein–ligand docking software widely used to identify lead compounds.34 We obtained the crystal structure of the protein from the RCSB Protein Data Bank (http://www.pdb.org/) and prepared it using Sybyl-X 2.0 software by removing ligands, water, and ions. The structures of fisetin, eriodyctiol (flavanone), kaempferol, and quercetin were drawn using Chemdraw software. AutoDock 4.2 was used for molecular docking analysis. The AutoDock tool was used to add gas-phase charges, merge non-polar hydrogen atoms, and set rotatable bonds for the ligand. The ligand and receptor structures were then converted to PDBQT format for AutoDock to read. The entire receptor was assigned polarity for AutoDock analysis.35 The resulting docking complex was subsequently analyzed using LigPlot and PyMOL software to investigate both the 2D and 3D polar interactions.
2.8. Molecular dynamics simulation (MDs)
This study employed the AMBER 22 program to perform molecular dynamics. Prior to MD simulation, the compounds were minimized using the HF/6-31G* optimization method in Gaussian 03, and the atomic partial charges were obtained from the Gaussian-derived electrostatic potential using the RESP fitting method in AMBER 22.36 The Antechamber and tleap modules were used to generate the parameters for the Generalized Amber Force Field 2 (GAFF2).37 The receptors were parameterized with the Ff19SB force field and any excess charge of the complex was neutralized by adding sodium or chloride ions. The complex was then solvated in a periodic box of TIP3P water molecules, with a distance of 10 Å between the edge of the box and the solute surface. To constrain the hydrogen bonds, the SHAKE method was employed. The PMEMD.CUDA module in AMBER 22 was utilized for accelerated simulation calculations.
The Sander module in Amber 22 was used for minimization and equilibration. Prior to MD simulations, three rounds of minimization were performed to relax each complex: solvent and ion (4000 steps); solution and side chain (5000 steps); and the entire system (10
000 steps). The energy minimization was carried out using a combination of the steepest descent method and conjugate gradient method. The system was then gradually heated from 0 to 300 K over a period of 50 ps. The density was stabilized using the NVT (constant particle number, volume, and temperature) ensemble. An unconstrained production phase was then initiated and run for 100 ns at an NPT (constant particle number, pressure, and temperature) ensemble of 1 atm and 300 K. The equilibrium of the complexes in MDs was assessed by evaluating the root mean square deviation (RMSD), root mean square fluctuations (RMSF), distances of important residues, and interactions between protein and ligand. The B-factor, which reflects the “diffusion” of the electron density of atoms in the crystal and indicates the uncertainty in the position of the atoms, was also used to assess the stability of the corresponding conformation. The relationship between B-factor and RMSF is as follows:
|
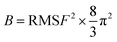 | (1) |
The MMPBSA.py script from the Amber Tools package was utilized to compute the binding free energy of all systems. This calculation was based on the solvent accessibility approach, the generalized Born solvation model, and the force field derived from molecular mechanics, which were used to determine the binding free energy of each snapshot from the MD trajectory.38 The total free energy binding was calculated using eqn (2)
|
ΔGbind = ΔGcomplex − (ΔGprotein + ΔGligand)
| (2) |
where, Δ
Gbind = binding free energy, Δ
Gcomplex = free energy of the complex, Δ
Gprotein = free energy of the protein, and Δ
Gligand = free energy of the ligand.
39
The free energy of each component complex, protein, and ligand was calculated by using eqn (3)
|
ΔGbind = ΔEMM + ΔEsol − TΔS = ΔEvdw + Eele + ΔEsol − TΔS
| (3) |
where, Δ
EMM indicates the gas-phase interaction energy between the receptor and ligand; Δ
Evdw = van der Waals energy; Δ
Eele = electrostatic energy contribution; Δ
Esol = the polar + non-polar solvation energy;
TΔ
S = represents the conformational entropy contribution at temperature
T.
40
2.9. Density functional theory (DFT) calculations
In density functional theory (DFT) based frontier molecular orbital (FMO) studies, the energies of the highest occupied molecular orbital (HOMO) and the lowest unoccupied molecular orbital (LUMO) of a molecule are important for many processes.41 A useful approach to identify electrostatic interactions between regions of molecules with electron-rich and electron-deficient areas is through the measurement of molecular electrostatic potential (MESP).42 It also aids in predicting potential sites for drug–molecule interactions as they bind to the active sites of proteins.43 This study utilized Gaussian 03 and GaussView 5 software to perform theoretical calculations using the DFT approach at the B3LYP level with a hybrid basis set comprising 6-31G(d).44 Following this, the present study calculated the molecular electrostatic potential (MESP), as well as the LUMO and HOMO energies for four compounds' molecular electrostatic properties. The results were represented using Multiwfn 3.8 and virtual molecular dynamics software (VMD 1.9.3).
3. Results
3.1. Five potential targets mediate Gleditsiae Spina therapy for pancreatic cancer
A total of 11 active ingredients were identified in Gleditsiae Spina using TCMSP. Detailed information on these active ingredients is listed in Table 1. We identified 294 drug targets and 12
706 disease targets, and found 265 intersection targets (Fig. 1A). We then screened and visualized the 265 core genes and their interacting lines (Fig. 1B). The top five targets ranked by degree value were RAC-alpha serine/threonine-protein kinase (AKT1), cellular tumor antigen p53 (TP53), tumor necrosis factor (TNF), interleukin-6 (IL6), and vascular endothelial growth factor A (VEGFA). The topological analysis results of these five potential targets are shown in Table 2.
Table 1 Eleven active components were screened from Gleditsiae Spina
Molecule name |
Formula |
CAS |
MW |
OB (%) |
DL |
Fisetin |
C15H10O6 |
528-48-3 |
286.25 |
52.6 |
0.24 |
Fustin |
C15H12O6 |
20725-03-5 |
288.27 |
50.91 |
0.24 |
(−)-Taxifolin |
C15H12O7 |
111003-33-9 |
304.27 |
60.51 |
0.27 |
Eriodyctiol (flavanone) |
C15H12O6 |
4049-38-1 |
288.27 |
41.35 |
0.24 |
Beta-sitosterol |
C29H50O |
83-46-5 |
414.79 |
36.91 |
0.75 |
Sitosterol |
C29H50O |
83-46-5 |
414.79 |
36.91 |
0.75 |
Kaempferol |
C15H10O6 |
520-18-3 |
286.25 |
41.88 |
0.24 |
Stigmasterol |
C29H48O |
83-48-7 |
412.77 |
43.83 |
0.76 |
Stigmast-4-ene-3,6-dione |
C29H46O2 |
23670-94-2 |
426.75 |
39.12 |
0.79 |
Ent-epicatechin |
C15H14O6 |
35323-91-2 |
290.29 |
48.96 |
0.24 |
Quercetin |
C15H10O7 |
117-39-5 |
302.25 |
46.43 |
0.28 |
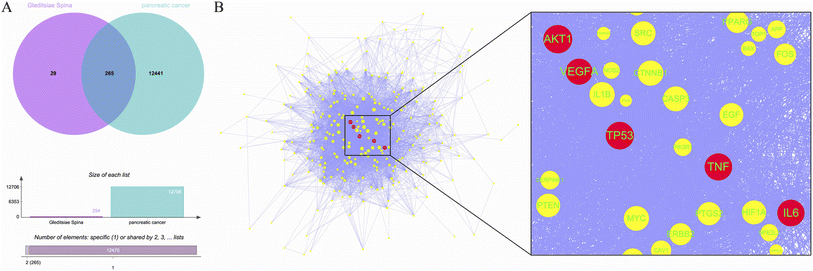 |
| Fig. 1 Core targets of Gleditsiae Spina are depicted in the figure. (A) The Venn diagram shows the overlap of Gleditsiae Spina and pancreatic cancer disease targets, and the elemental analysis. (B) The network topology analysis is shown with blue lines representing protein–protein interactions. The top 5 target proteins with degree values are represented as red circles, with the size of the node indicating the degree value. A local magnification of the protein interaction is presented on the right. | |
Table 2 Topological analysis of the top five genes by degree value
Gene name |
Degree |
Betweenness |
Closeness |
AKT1 |
162 |
3956.71 |
0.71 |
TP53 |
155 |
3378.50 |
0.70 |
TNF |
150 |
2601.46 |
0.69 |
IL6 |
146 |
2282.03 |
0.68 |
VEGFA |
140 |
1552.73 |
0.67 |
3.2. A variety of biological processes and signaling pathways are involved
To analyze the mechanism of action of the core targets, we performed GO and KEGG enrichment analyses. We identified 10 biological processes (BP), 7 molecular functions (MF), 3 cellular components (CC), and 10 pathways (Fig. 2A). The results revealed that BP terms were mainly related to the regulation of gene expression, transcription from RNA polymerase II promoter, and peptidyl-serine phosphorylation. CC terms were mainly related to macromolecular complexes, extracellular space, and extracellular regions. MF terms were mainly related to identical protein binding, cytokine activity, and protein phosphatase 2A binding. The top 10 significantly enriched pathways of Gleditsiae Spina in pancreatic cancer are shown in Fig. 2B, including the human cytomegalovirus infection, AGE-RAGE signaling pathway in diabetic complications, and MAPK signaling pathway. Our analysis suggests that the human cytomegalovirus infection signaling pathway may be a critical pathway for the treatment of pancreatic cancer using Gleditsiae Spina (ESI Fig. 1†).
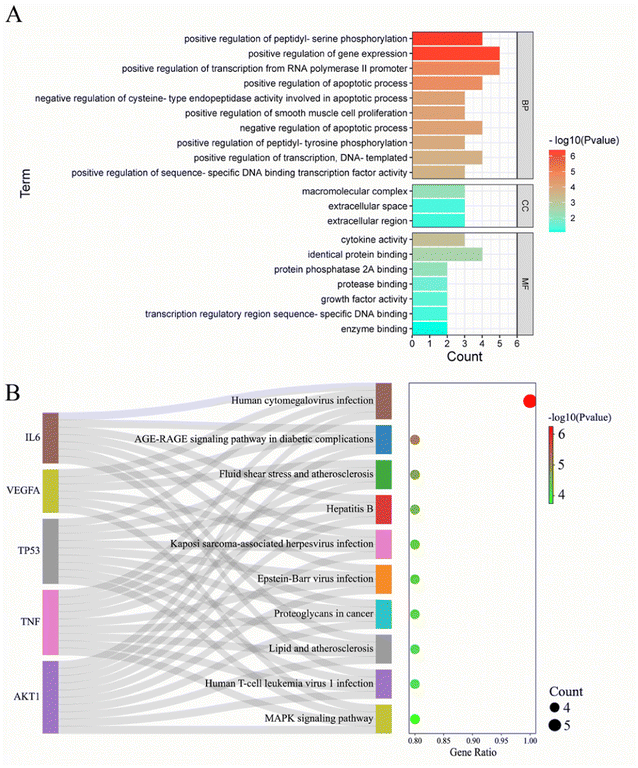 |
| Fig. 2 GO and KEGG enrichment analysis of core targets. (A) The bar with color gradient of GO function enrichment. (B) The sankey dot of KEGG pathway enrichment. | |
3.3. Seven kinds of drug molecules in the active ingredients of Gleditsiae Spina
As the negative ADME characteristics of the compounds limit their clinical use, we analyzed the ADME properties of the 11 identified active ingredients. Molecular and pharmacodynamic properties of these compounds were calculated. The total polar surface area (TPSA) of the compounds should range from 20 to 130 Å. Only quercetin slightly exceeds this limit. The water solubility range of the compounds should be ≥6, indicating that 7 of these compounds have good solubility in water. Gastrointestinal absorption (GI) of 7 molecules was significant, while that of 4 molecules was low. These seven compounds exhibit a high degree of drug likeness, as none of their attributes deviate from the guidelines established by Lipinski, Ghose, Veber, Muegge, and Egan (Table 3). Fisetin, fustin, (−)-taxifolin, eriodyctiol (flavanone), kaempferol, ent-epicatechin, and quercetin were found to have better ADMET parameters compared to other compounds, and therefore, are more likely to be developed as therapeutic molecules.
Table 3 ADME properties of 11 active components in Gleditsiae Spina
Molecule |
TPSA |
ESOL log S |
GI absorption |
Lipinski #violations |
Ghose #violations |
Veber #violations |
Egan #violations |
Muegge #violations |
Fisetin |
111.13 |
−3.35 |
High |
0 |
0 |
0 |
0 |
0 |
Fustin |
107.22 |
−2.53 |
High |
0 |
0 |
0 |
0 |
0 |
(−)-Taxifolin |
127.45 |
−2.66 |
High |
0 |
0 |
0 |
0 |
0 |
Eriodyctiol (flavanone) |
107.22 |
−3.26 |
High |
0 |
0 |
0 |
0 |
0 |
Beta-sitosterol |
20.23 |
−7.90 |
Low |
1 |
3 |
0 |
1 |
2 |
Sitosterol |
20.23 |
−7.90 |
Low |
1 |
3 |
0 |
1 |
2 |
Kaempferol |
111.13 |
−3.31 |
High |
0 |
0 |
0 |
0 |
0 |
Stigmasterol |
20.23 |
−7.46 |
Low |
1 |
3 |
0 |
1 |
2 |
Stigmast-4-ene-3,6-dione |
34.14 |
−7.36 |
Low |
1 |
3 |
0 |
1 |
1 |
Ent-epicatechin |
110.38 |
−2.22 |
High |
0 |
0 |
0 |
0 |
0 |
Quercetin |
131.36 |
−3.16 |
High |
0 |
0 |
0 |
0 |
0 |
3.4. Gleditsiae Spina acts on pancreatic cancer through multiple active ingredients, multiple targets, multiple pathways
We constructed a network of compounds, targets, pathways, and pancreatic cancer based on the active ingredients connected to the core genes. This network comprises 95 nodes and 312 edges, including 4 compounds, 5 targets, 85 pathways, and 1 disease. Through this analysis, we identified four key components, namely fisetin, eriodyctiol (flavonoid), kaempferol, and quercetin, that may serve as potential therapeutic agents in the treatment of pancreatic cancer (Fig. 3).
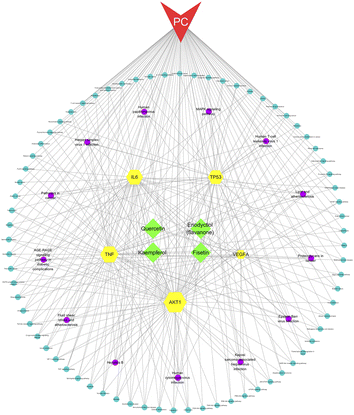 |
| Fig. 3 The compounds–targets–pathway–pancreatic cancer network. The gray connecting lines indicate the node interactions. Green represents the compounds; red represents the disease; and yellow represent the target protein. Signaling pathways are shown in circles, where pathways based on degree values ≥5 are shown in purple. | |
3.5. Four high affinity complexes among Gleditsiae Spina acting on pancreatic cancer
We performed molecular docking analysis to investigate the binding patterns between the four key active ingredients and five core targets. The target proteins used as receptors were AKT1 (PDBID 1UNQ), TP53 (PDBID 5O1E), TNF (PDBID 2E7A), IL6 (PDBID 1ALU) and VEGFA (PDBID 3V2A). The affinity value indicates the stability of the binding between the receptor and ligand, with a lower affinity indicating a more stable binding conformation. As shown in Fig. 4, the affinity values of the complexes between fisetin-TP53, eriodyctiol-TNF, kaempferol-TNF, and quercetin-IL6 were close to −7.0 kcal mol−1, indicating that these complexes were more stable. Therefore, we selected these four high-affinity complexes for further analysis.
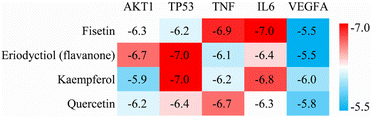 |
| Fig. 4 Heat map of docking affinity between four active components and five core targets. Affinity values are provided as kcal mol−1. | |
3.6. Hydrogen bonds of key residues of three complexes can act stably for a long time
To evaluate the dynamic stability and sampling validity of the four complexes, we studied the RMSD values of the four complex skeleton atoms over time during 100 ns MDs. Fig. 5A–D displays that the RMSD of the fisetin-TNF, fisetin-IL6, eriodyctiol-TP53, and kaempferol-TP53 remained stable around 2.0 Å. The RMSD values reached a plateau, indicating that the system reached equilibrium after 20 ns of simulation. Additionally, Fig. 5E–H demonstrated that the RMSF values of the receptor ranged from 2.0 to 14.0 Å during the 100 ns simulation, which was associated with the B-factor of the crystallography and the interaction with the binding site residues. Fig. 5E revealed that the most flexible fragment was located between ARG24 and ASN27 in the fisetin-TNF system. Similarly, Fig. 5F showed three highly flexible regions in the fisetin-IL6 system: between ASN27 and ASN30, between GLN48 and PHE51, and between ASN128 and TRP130. Fig. 5G and H indicated that the same areas of high flexibility were present in the eriodyctiol-TP53 and kaempferol-TP53 systems: between GLU129 and SER132. To better demonstrate the conformational stability and flexibility of the amino acid residues of the receptor protein, we performed conformational coloring according to RMSF values and displayed it in Fig. 5I–L. All molecular dynamics modeling methods showed excessively high values in the terminal residues and loop regions. This suggests that the four systems' complexes are stable and repeatable, and not the result of a computational error.
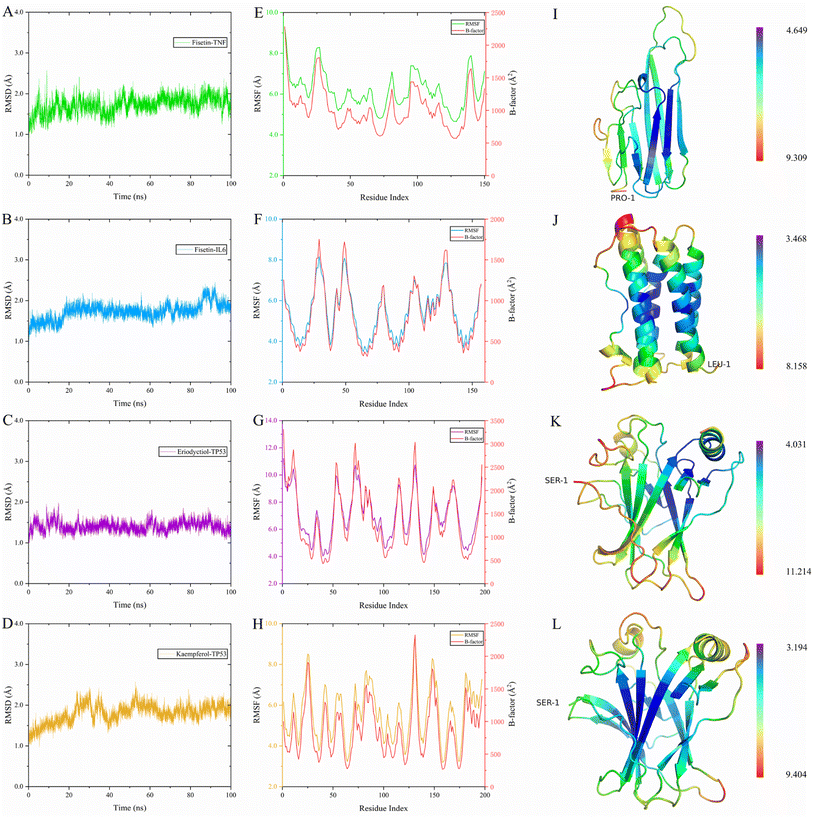 |
| Fig. 5 The RMSD value of the complex was simulated for 100 ns, and the receptor RMSF, B-factor and 3D structure were colored according to the RMSF value for the last 4 ns. (A) 100 ns RMSD of fisetin-TNF. (B) 100 ns RMSD of fisetin-IL6. (C) 100 ns RMSD of eriodyctiol-TP53. (D) 20 ns RMSD of kaempferol-TP53. (E) Last 10 ns RMSF and B-factor of fisetin-TNF. (F) Last 10 ns RMSF and B-factor of fisetin-IL6. (G) Last 10 ns RMSF and B-factor of eriodyctiol-TP53. (H) Last 10 ns RMSF and B-factor of kaempferol-TP53. (I) 3D structure of the TNF colored by RMSF in E. (J) 3D structure of the IL6 colored by RMSF in F. (K) 3D structure of the TP53 colored by RMSF in G. (L) 3D structure of the TP53 colored by RMSF in H. | |
In Fig. 6A–D, the stable conformations obtained after 100 ns of MD simulations were selected for interaction analysis and visualized using Pymol and Ligplot. To estimate the H-bond interactions of these complexes, H-bond distance analysis was performed on 10
000 snapshots of the last 10 ns simulations of each complex. The hydrogen bond distances were analyzed for each bond along the trajectory and are shown in Fig. 7. For more accurate analysis, the average length and occupancy of each hydrogen bond were also analyzed and presented in Table 4. The results indicate that long-time stable hydrogen bonds appear in three systems: ASP16-OD2/Fis-H8 and ASP16-OD2/Fis-H9 in fisetin-IL6 (with 100.00% occupancy), THR135-OG1/Eri-H7 in eriodyctiol-TP53 (with 99.07% occupancy), and LEU50-O/Kae-H10 in kaempferol-TP53 (with 99.63% occupancy). These results suggest that hydrogen bonding plays a crucial role in the conformational stability of these three complexes.
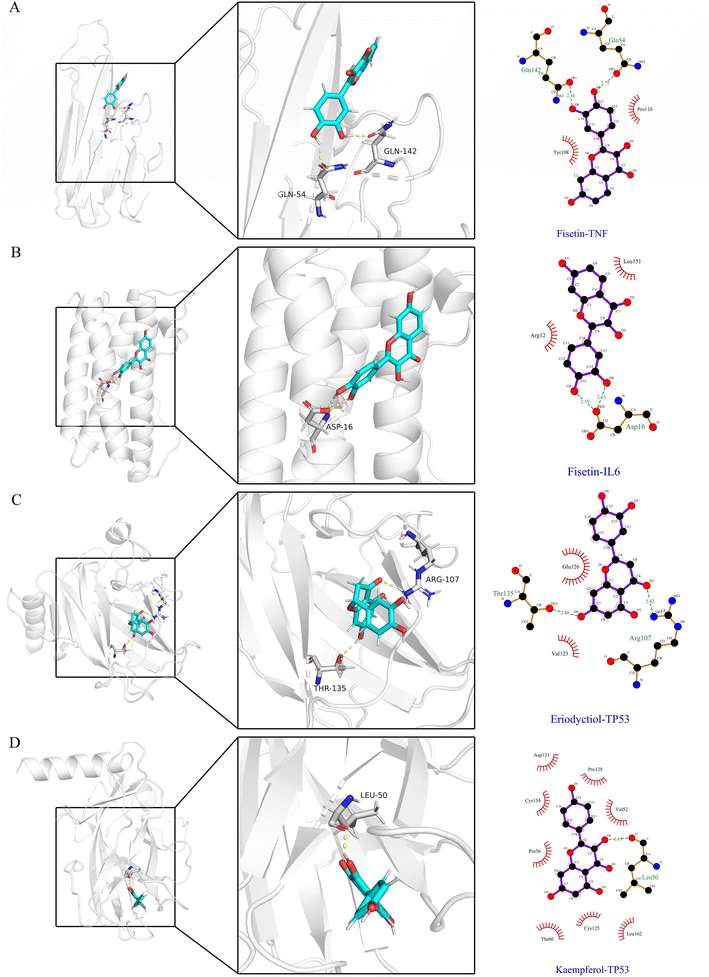 |
| Fig. 6 At 100 ns, compound binding site in four systems and amplification of such site. Blue colors in both the complete receptor structure and amplification of the binding site represent the ligand structure while the yellow dotted lines indicate H-bond interactions. The 2D diagram of the interaction shows all the interaction forces. (A) Fisetin-TNF. (B) Fisetin-IL6. (C) Eriodyctiol-TP53. (D) Kaempferol-TP53. | |
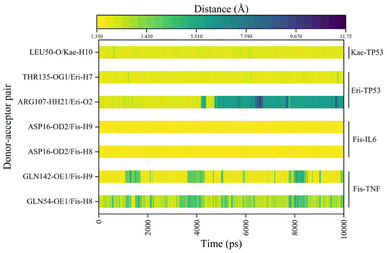 |
| Fig. 7 The distance between the hydrogen bonds in each system for the last 10 ns. | |
Table 4 Average hydrogen bond lengths and occupancy rates of key residues in MDs of the four systems
System |
Donor–acceptor pair |
Average bond length (Å) |
Occupancy (%) |
Fisetin-TNF |
GLN54-OE1/Fis-H8 |
3.13 ± 1.08 |
51.82% |
GLN142-OE1/Fis-H9 |
2.41 ± 1.14 |
75.08% |
Fisetin-IL6 |
ASP16-OD2/Fis-H8 |
1.67 ± 0.10 |
100.00% |
ASP16-OD2/Fis-H9 |
1.64 ± 0.10 |
100.00% |
Eriodyctiol-TP53 |
ARG107-HH21/Eri-O2 |
4.35 ± 2.16 |
44.33% |
THR135-OG1/Eri-H7 |
1.91 ± 0.24 |
99.07% |
Kaempferol-TP53 |
LEU50-O/Kae-H10 |
2.00 ± 0.23 |
99.63% |
3.7. Among the four complexes, TP53 has higher binding free energy as a receptor
Molecular mechanics Poisson–Boltzmann surface area (MMPBSA) and MMGBSA are commonly used to analyze molecular dynamics results, however, it has been shown that the GB solvent model provides more accurate results than the PB model.45 A residue-based energy decomposition analysis was performed using the MMGBSA method to further characterize the detailed mechanism of key residue interactions and to assess the effect of energy on the contribution of each residue in the binding site pocket. To determine the energy stability of these complexes, an energy convergence analysis was performed on 4000 snapshots of the last 4 ns simulations of each complex. Table 5 shows the calculated binding free energy for each complex. The ΔGbind of fisetin-IL6 (−24.84 ± 0.02 kcal mol−1), eriodyctiol-TP53 (−23.64 ± 0.03 kcal mol−1), and kaempferol-TP53 (−30.54 ± 0.02 kcal mol−1) were higher than fisetin-TNF (−18.93 ± 0.03 kcal mol−1), indicating that the affinity of fisetin-TNF is lower than that of the other systems, which is consistent with the result that the hydrogen bonds are not stabilized for a long time. Specifically, electrostatic interactions and gas phase energy were outstanding components in fisetin-IL6 and eriodyctiol-TP53, while van der Waals energy and gas phase energy contributed significantly to the system energy in kaempferol-TP53. The polar component of solvation free energy was unfavorable for all systems. To determine the key amino acids that contribute the most to ΔGbind, ΔGbind was broken down into each amino acid, and Fig. 8A–D shows the top ten energy-contributing amino acid residues in each system. The key residues of each receptor were determined by combining the free energy calculation method, providing a reference for the discovery of different inhibitors targeting the receptor, and a basis for the treatment of pancreatic cancer with the effective components of Gleditsiae Spina.
Table 5 Binding energies of the four systems. Energy values are provided as kcal mol−1
Systems |
ΔEvdw |
ΔEele |
ΔGgas |
ΔEsol |
ΔGbind |
Fisetin-TNF |
−14.75 ± 0.03 |
−35.72 ± 0.08 |
−50.47 ± 0.07 |
31.54 ± 0.04 |
−18.93 ± 0.03 |
Fisetin-IL6 |
−13.03 ± 0.03 |
−54.00 ± 0.05 |
−67.03 ± 0.04 |
42.19 ± 0.03 |
−24.84 ± 0.02 |
Eriodyctiol-TP53 |
−18.70 ± 0.03 |
−41.98 ± 0.09 |
−60.69 ± 0.09 |
37.05 ± 0.07 |
−23.64 ± 0.03 |
Kaempferol-TP53 |
−36.03 ± 0.02 |
−15.80 ± 0.05 |
−51.83 ± 0.04 |
21.29 ± 0.03 |
−30.54 ± 0.02 |
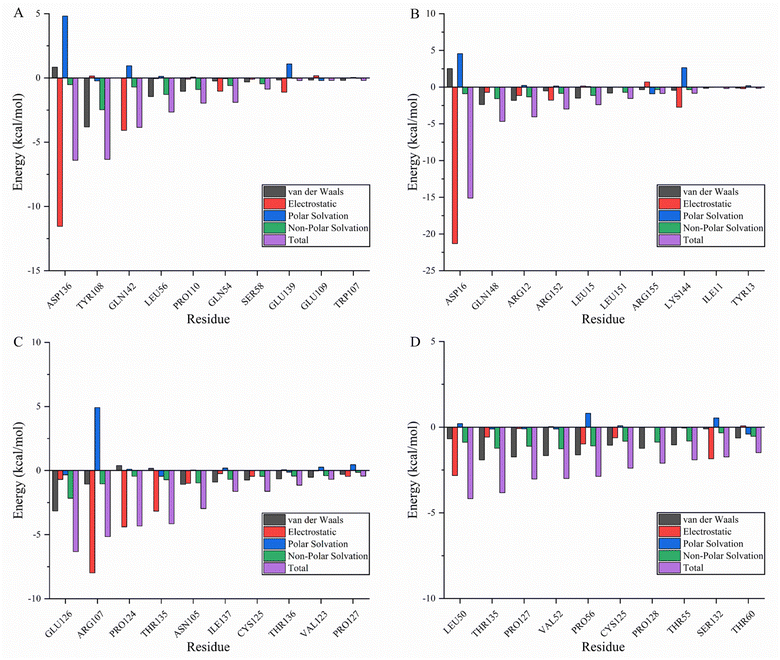 |
| Fig. 8 Energy decomposition of the top ten residues (including van der Waals: black; electrostatic: red; polar solvation: blue; non-polar solvation: green; total: purple). (A) Fisetin-TNF. (B) Fisetin-IL6. (C) Eriodyctiol-TP53. (D) Kaempferol-TP53. | |
3.8. Kaempferol is the most stable of the four active ingredients of Gleditsiae Spina
The figures presented in Fig. 9 illustrate the HOMO, LUMO, and MESP of four active ingredients found in Gleditsiae Spina. It is observed that the HOMO and LUMO orbitals of kaempferol, eriodyctiol, and fisetin are distributed more extensively throughout the molecule. The energy levels of the HOMO orbital of kaempferol are reported to be −5.57 eV, while the energy level of the LUMO orbital is −1.82 eV, as shown in Table 6. Furthermore, the HOMO–LUMO energy gap value of kaempferol is 3.75 eV, which is relatively larger than that of the other molecules, making the entitled molecules more kinetically stable.
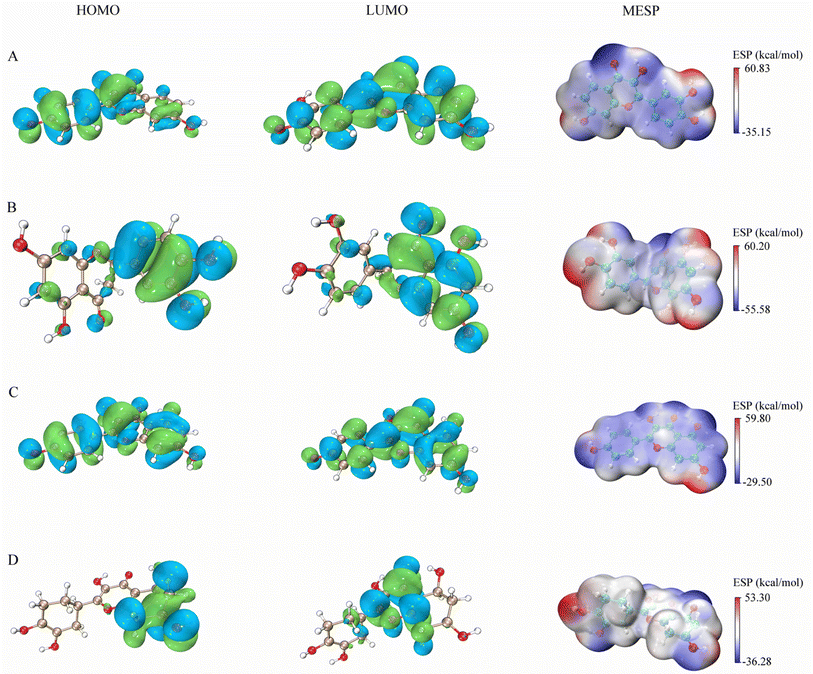 |
| Fig. 9 HOMO, LUMO and MESP on four active ingredients of Gleditsiae Spina. (A)fisetin. (B) Eriodyctiol. (C) Kaempferol. (D) Quercetin. | |
Table 6 Global reactivity descriptors on four active ingredients of Gleditsiae Spina
Parameters |
Fisetin |
Eriodyctiol |
Kaempferol |
Quercetin |
HOMO (eV) |
−5.38 |
−5.38 |
−5.57 |
−4.60 |
LUMO (eV) |
−1.74 |
−1.74 |
−1.82 |
−1.40 |
HOMO−LUMO gap (eV) |
3.64 |
3.64 |
3.75 |
3.20 |
The MESP images presented in this study utilize a color scheme where blue represents electron-rich (negative) regions, red represents electron-deficient (positive) regions, and white represents neutral regions. Notably, the MESP of kaempferol displays a smaller red region and a larger blue region. Further analysis through molecular docking and MDs revealed that these negative and positive ion centers play a crucial role in the formation of non-bond interactions, particularly hydrogen bonds, within the ligand–receptor complex.
4. Discussion
Understanding the binding mechanism between receptors and ligands is essential for drug discovery and optimization. If experimental validation of compound activity is unfeasible, several alternative methods can be considered to further improve the accuracy of molecular docking predictions. One such method is to combine multiple scoring functions to generate a consensus ranking list. Scoring function is another important factor affecting the accuracy of molecular docking, which mainly evaluates the affinity between the ligand and the receptor molecules, and can be used for ranking different conformations of the same ligand or different ligands to achieve the goal of identifying the correct binding conformation or finding ligands with higher affinity.46 Shengyong Yang and colleagues have developed a target-specific scoring function, based on a commonly used empirical scoring function, which significantly improves the scoring performance for protein targets. By using this method, they successfully screened and identified JAK3 and YopH activators.47 The AutoDock Vina program features an iterated local search global optimizer, while the determination of binding energy combines both knowledge-based and empirical scoring functions.20 In this study, the complex structures predicted by AutoDock vina were re-scored by MMPB(GB)SA to evaluate the binding mode between the active components from Gleditsiae Spina and the target protein. Compared with traditional universal scoring functions (AutoDock vina), the inadequate consideration of solvent effects can adversely affect the performance of the scoring function. MM-GBSA and MM-PBSA, which was widely used for calculating binding energies based on simulation trajectories, introduce of continuous solvent models such as Poisson–Boltzmann (PB) and Generalized Born (GB), result in improving the solvent effect calculations and ultimately enhancing scoring functions.22,40 In addition, the calculated binding free energy can be decomposed into multiple energy terms with clear physical meanings, which has some reference value for guiding molecular design. In addition to solvent effects, entropy effects are another important factor that needs to be considered. Considering both entropy effects is likely to be one of the future development trends of such scoring functions.48
The utilization of MM/PB(GB)SA methods to predict binding free energy and pinpoint the native structure as the conformation with the lowest energy is notably more theoretically rigorous than the majority of scoring functions.49 Methods in this category are typically considered to have higher computational accuracy since their calculated values are not entirely dependent on a single initial complex structure, but their computational demands are usually higher. Despite the significant reduction in computation time due to advances in software and hardware, especially the widespread use of GPUs in MD in recent years, such calculations are still difficult to predict for thousands of systems in a short period.50,51 In contrast, docking score energies can directly estimate binding free energies for a given single complex structure, making them computationally faster and often applied in virtual screening processes involving tens of thousands of ligands. In recent years, the emergence of scoring functions based on quantum mechanics (QM) methods has brought new ideas and new hopes for improving scoring performance.52 Therefore, the development of new high-precision universal scoring functions and the rational selection and combined use of existing scoring functions (consistent scoring) are still key points that need to be focused on and worthy of further exploration in molecular docking research. In the present study, the authors demonstrated binding free energy values of (−18.93 ± 0.03, −24.84 ± 0.02, −23.64 ± 0.03, and −30.54 ± 0.02 kcal mol−1) for the four systems, which are significantly more meaningful than the affinities (−6.9, −7.0, −7.0, and −7.0 kcal mol−1) obtained using AutoDock Vina. This implies that the combined application of molecular docking and molecular dynamics simulations is theoretically more accurate for the discovery of potential active compounds and drug targets.
The pathogenesis of pancreatic cancer involves a complex and multifaceted biological process. Among the various factors involved, four main driver genes have been identified, namely KRAS, CDKN2A, TP53, and SMAD4.53 Among these four driver genes, it has been reported that mutations in the tumor suppressor gene TP53 occur in approximately 50–75% of pancreatic cancers.54 Previous studies have demonstrated that mutations in TP53 promote the expression of oncogene Wnt1 inducible signaling pathway protein-1 (Wisp-1) in mouse PDAC cells. However, drug therapy targeting TP53 in pancreatic cancer remains rare.55 Gleditsiae Spina has shown remarkable potential in the treatment of various types of cancers, making it an important resource in cancer research and therapy.56 Studies have shown that the extract of Gleditsiae Spina has the potential to prevent colon cancer in vitro and in vivo through the induction of G2/M cell cycle arrest and activation of extracellular signal-regulated kinase 1/2 (ERK1/2). Furthermore, in vivo studies have revealed that Gleditsiae Spina can prevent cervical cancer through the down-regulation of proliferating cell nuclear antigen (PCNA) and mutant p53.57,58 Gleditsiae Spina is recognized as containing diverse chemical components such as flavonoid glycosides, phenolic compounds, amino acids, palmitic acid, and other compounds.12,13 The aforementioned major chemical components of Gleditsiae Spina exhibit varying degrees of anticancer effects, with flavonoids demonstrating the strongest efficacy. Current pharmacological studies have demonstrated the anti-tumor pharmacological activities of Gleditsiae Spina and its chemical constituents.59 In this study, we conducted a comprehensive analysis of the interactions between network parameters associated with pancreatic cancer and potential compounds found in Gleditsiae Spina, with the aim of exploring their potential in the treatment of pancreatic cancer.
We collected and screened multiple databases to obtain 11 bioactive compounds in Gleditsiae Spina and 265 Gleditsiae Spina-pancreatic cancer target genes. Interestingly, we found that four important compounds were consistent with ADMET prediction in the compounds–targets–pathway–pancreatic cancer network. These active ingredients could lead to the discovery of existing drug leads for targeting pancreatic cancer. Flavonoids have been shown to possess a wide variety of anticancer effects, such as modulating reactive oxygen species (ROS)-scavenging enzyme activities, participating in cell cycle arrest, inducing apoptosis and autophagy, and suppressing cancer cell proliferation and invasiveness.8,60 Our study comprehensively analyzed the potential anti-pancreatic cancer effects of flavonoid compounds, including fisetin, eriodyctiol, kaempferol, and quercetin, which are active components of Gleditsiae Spina. Among these compounds, fisetin, eriodyctiol, and kaempferol showed the strongest potential effects. Previous studies have shown that fisetin can induce DNA damage, inhibit the PI3K/AKT/mTOR signaling pathway, suppress NF-κB activation, and induce autophagy in pancreatic cancer cells. Molecular docking and molecular dynamics simulation results revealed that fisetin can bind to IL6, which suggests a potential mechanism for its anti-pancreatic cancer activity. Kaempferol was found to be the major active component contributing to the anti-pancreatic cancer activity and can induce ROS-dependent apoptosis in pancreatic cancer cells via TGM2-mediated Akt/mTOR signaling. Furthermore, our GO function and KEGG pathway enrichment analysis showed that Gleditsiae Spina may be associated with human cytomegalovirus infection signaling pathway, AGE-RAGE signaling pathway in diabetic complications, and MAPK signaling pathway in the treatment of pancreatic cancer. Notably, the human cytomegalovirus infection signaling pathway has been reported to be closely related to the pathological process of pancreatic cancer.61 Previous studies have demonstrated that human pancreatic cells, primary β cells, and cytomegalovirus insulinoma cells are susceptible to human cytomegalovirus infection, replication, and expression of viral proteins.62 This finding implies that kaempferol, as an active compound of Gleditsiae Spina, is associated with the human cytomegalovirus infection signaling pathway in the treatment of pancreatic cancer. Moreover, our results suggest that Gleditsiae Spina can induce apoptosis in pancreatic cancer cells through the AGE-RAGE signaling pathway, which is characterized by an increase in ROS levels and a decrease in mitochondrial membrane potential. Furthermore, our study found that the MAPK signaling pathway is closely linked to the development and progression of human pancreatic cancer and may be targeted by Gleditsiae Spina in the treatment of this disease.
5. Conclusion
For the first time, we have systematically elucidated the pivotal active ingredients and mechanisms of Gleditsiae Spina in the treatment of pancreatic cancer. Fisetin, eriodyctiol, kaempferol, and quercetin might target AKT1, TP53, TNF, IL6, VEGFA, and other targets, participating in the human cytomegalovirus infection signaling pathway, AGE-RAGE signaling pathway, and MAPK signaling pathway. Molecular docking, MD simulations, and DFT calculations further revealed the binding mode and interaction mechanisms of fisetin, eriodyctiol, and kaempferol with TP53, TNF, and IL6. TP53 may be the most potential target for Gleditsiae Spina bioactive compounds in pancreatic cancer. This data may contribute to further studies in the search for potential drugs for pancreatic cancer.
Author contributions
Hongtao Duan and Rui Zhang designed the study, performed the main experiments and drafted the manuscript. Lu Yuan, Yiyuan Liu, and Aiminuer Asikaer did literature search and analyzed the data. Yang Liu revised the manuscript. Yan Shen obtained the financial support for the project leading to this publication, supervised the experiments, and revised the manuscript. All authors read and approved the final manuscript.
Conflicts of interest
The authors declare no potential conflicts of interest with respect to the research, authorship, and/or publication of this article.
Acknowledgements
This work was supported by Natural Science Foundation of Chongqing, China (CSTB2022NSCQ-MSX1493), National Natural Science Foundation of China (No. 82100684), and Chongqing University of Technology Postgraduate Innovation Project (gzlcx 20223340).
References
- H. Zhu, T. Li, Y. Du and M. Li, BMC Med., 2018, 16, 214 CrossRef CAS PubMed.
- H. Yao, W. Song, R. Cao, C. Ye, L. Zhang, H. Chen, J. Wang, Y. Shi, R. Li, Y. Li, X. Liu, X. Zhou, R. Shao and L. Li, Nat. Commun., 2022, 13, 5506 CrossRef CAS PubMed.
- A. Saluja and A. Maitra, Gastroenterology, 2019, 156, 1937–1940 CrossRef PubMed.
- R. L. Siegel, K. D. Miller and A. Jemal, Ca-Cancer J. Clin., 2018, 68, 7–30 CrossRef PubMed.
- A. McGuigan, P. Kelly, R. C. Turkington, C. Jones, H. G. Coleman and R. S. McCain, World J. Gastroenterol., 2018, 24, 4846–4861 CrossRef PubMed.
- C. F. Song, Y. H. Hu, Z. G. Mang, Z. Ye, H. D. Chen, D. S. Jing, G. X. Fan, S. R. Ji, X. J. Yu, X. W. Xu and Y. Qin, Acta Pharmacol. Sin., 2022, 44, 865–876 CrossRef PubMed.
- A. L. Miller, P. L. Garcia and K. J. Yoon, Pharmacol. Res., 2020, 155, 104740 CrossRef CAS PubMed.
- D. M. Kopustinskiene, V. Jakstas, A. Savickas and J. Bernatoniene, Nutrients, 2020, 12, 457 CrossRef CAS PubMed.
- D. Aune, Adv. Nutr., 2019, 10, S404–S421 CrossRef PubMed.
- M. Zeng, M. Qi, Y. Wang, R. Xu, Y. Wu, M. Li, X. Zheng and W. Feng, Int. Immunopharmacol., 2020, 80, 106194 CrossRef CAS PubMed.
- S. J. Lee, S. S. Park, W. J. Kim and S. K. Moon, Am. J. Chin. Med., 2012, 40, 373–386 CrossRef PubMed.
- L. Zhou, D. Li, J. Wang, Y. Liu and J. Wu, Nat. Prod. Res., 2007, 21, 283–291 CrossRef CAS PubMed.
- J. Li, K. Jiang, L. J. Wang, G. Yin, J. Wang, Y. Wang, Y. B. Jin, Q. Li and T. J. Wang, J. Sep. Sci., 2018, 41, 1752–1763 CrossRef CAS PubMed.
- S. Jia, X. Xu, S. Zhou, Y. Chen, G. Ding and L. Cao, Cell Death Dis., 2019, 10, 142 CrossRef CAS PubMed.
- G. Ding, X. Xu, D. Li, Y. Chen, W. Wang, D. Ping, S. Jia and L. Cao, Cell Death Dis., 2020, 11, 893 CrossRef CAS PubMed.
- L. Y. Xiao and Z. Gao, Aging, 2021, 13, 24753–24767 CrossRef PubMed.
- I. Murtaza, V. M. Adhami, B. B. Hafeez, M. Saleem and H. Mukhtar, Int. J. Cancer, 2009, 125, 2465–2473 CrossRef CAS PubMed.
- P. Asgharian, A. P. Tazehkand, S. R. Soofiyani, K. Hosseini, M. Martorell, V. Tarhriz, H. Ahangari, N. Cruz-Martins, J. Sharifi-Rad, Z. M. Almarhoon, A. Ydyrys, A. Nurzhanyat, A. Yessenbekova and W. C. Cho, Oxid. Med. Cell. Longevity, 2021, 2021, 4393266 Search PubMed.
- F. Wang, L. Wang, C. Qu, L. Chen, Y. Geng, C. Cheng, S. Yu, D. Wang, L. Yang, Z. Meng and Z. Chen, BMC Cancer, 2021, 21, 396 CrossRef CAS PubMed.
- C. Blanes-Mira, P. Fernandez-Aguado, J. de Andres-Lopez, A. Fernandez-Carvajal, A. Ferrer-Montiel and G. Fernandez-Ballester, Molecules, 2022, 28, 175 CrossRef PubMed.
- J. P. Ceron-Carrasco, ChemMedChem, 2022, 17, e202200278 CAS.
- P. Schmidtke, A. Bidon-Chanal, F. J. Luque and X. Barril, Bioinformatics, 2011, 27, 3276–3285 CrossRef CAS PubMed.
- C. A. Ramos-Guzman, J. J. Ruiz-Pernia and I. Tunon, ACS Catal., 2021, 11, 4157–4168 CrossRef CAS PubMed.
- C. Xie, H. Tang, G. Liu and C. Li, Front. Aging Neurosci., 2022, 14, 940166 CrossRef CAS PubMed.
- X. Xu, W. Zhang, C. Huang, Y. Li, H. Yu, Y. Wang, J. Duan and Y. Ling, Int. J. Mol. Sci., 2012, 13, 6964–6982 CrossRef CAS PubMed.
- H. Lin, X. Wang, M. Liu, M. Huang, Z. Shen, J. Feng, H. Yang, Z. Li, J. Gao and X. Ye, Phytother. Res., 2021, 35, 2651–2664 CrossRef CAS PubMed.
- S. Hu, M. Ge, S. Zhang, M. Jiang, K. Hu and L. Gao, Front. Oncol., 2022, 12, 854596 CrossRef PubMed.
- T. He, M. Wang, J. Kong, Q. Wang, Y. Tian, C. Li, Q. Wang, C. Liu and J. Huang, J. Ethnopharmacol., 2022, 284, 114784 CrossRef CAS PubMed.
- X. Li, H. Tang, Q. Tang and W. Chen, Front. Cell Dev. Biol., 2021, 9, 638366 CrossRef PubMed.
- C. Wu, Z. H. Huang, Z. Q. Meng, X. T. Fan, S. Lu, Y. Y. Tan, L. M. You, J. Q. Huang, A. Stalin, P. Z. Ye, Z. S. Wu, J. Y. Zhang, X. K. Liu, W. Zhou, X. M. Zhang and J. R. Wu, China's Med., 2021, 16, 121 CrossRef CAS PubMed.
- J. An, H. Fan, M. Han, C. Peng, J. Xie and F. Peng, Front. Pharmacol., 2022, 13, 961012 CrossRef CAS PubMed.
- X. Wang, Y. Sun, L. Ling, X. Ren, X. Liu, Y. Wang, Y. Dong, J. Ma, R. Song, A. Yu, J. Wei, Q. Fan, M. Guo, T. Zhao, R. Dao and G. She, Front. Pharmacol., 2021, 12, 704040 CrossRef CAS PubMed.
- R. L. Bakal, R. D. Jawarkar, J. V. Manwar, M. S. Jaiswal, A. Ghosh, A. Gandhi, M. E. A. Zaki, S. Al-Hussain, A. Samad, V. H. Masand, N. Mukerjee, S. Nasir Abbas Bukhari, P. Sharma and I. Lewaa, Saudi Pharm. J., 2022, 30, 693–710 CrossRef CAS PubMed.
- T. Gaillard, J. Chem. Inf. Model., 2018, 58, 1697–1706 CrossRef CAS PubMed.
- X. Y. Zhang, H. J. Huang, D. L. Zhuang, M. I. Nasser, M. H. Yang, P. Zhu and M. Y. Zhao, Infect. Dis. Poverty, 2020, 9, 99 CrossRef PubMed.
- D. A. Case, H. M. Aktulga, K. Belfon, I. Y. Ben-Shalom, J. T. Berryman, S. R. Brozell, D. S. Cerutti, T. E. III Cisneros, V. W. D. Cruzeiro, T. A. Darden, R. E. Duke, G. Giambasu, M. K. Gilson, H. Gohlke, A. W. Goetz, R. Harris, S. Izadi, S. A. Izmailov, K. Kasavajhala, M. C. Kaymak, E. King, A. Ko- valenko, T. Kurtzman, T. S. Lee, S. LeGrand, P. Li, C. Lin, J. Liu, T. Luchko, R. Luo, M. Machado, V. Man, M. Manathunga, K. M. Merz, Y. Miao, O. Mikhailovskii, G. Monard, H. Nguyen, K. A. O’Hearn, A. Onufriev, F. Pan, S. Pantano, R. Qi, A. Rahnamoun, D.R. Roe, A. Roitberg, C. Sagui, S. Schott-Verdugo, A. Shajan, J. Shen, C. L. Simmerling, N. R. Skrynnikov, J. Smith, J. Swails, R. C. Walker, J. Wang, J. Wang, H. Wei, R. M. Wolf, X. Wu, Y. Xiong, Y. Xue, D. M. York, S. Zhao, and P. A. Kollman, Amber 2022, University of California, San Francisco, 2022 Search PubMed.
- J. Fang, P. Wu, R. Yang, L. Gao, C. Li, D. Wang, S. Wu, A. L. Liu and G. H. Du, Acta Pharm Sin B, 2014, 4, 430–437 CrossRef PubMed.
- M. Manish, S. Mishra, A. Anand and N. Subbarao, Comput. Biol. Med., 2022, 150, 106125 CrossRef CAS PubMed.
- S. Jupudi, K. Rajagopal, S. Murugesan, B. K. Kumar, K. Raman, G. Byran, J. Chennaiah, V. P. Muthiah, P. B. Dasan and S. Sankaran, S. Afr. J. Bot., 2021, 151, 82–91 CrossRef PubMed.
- S. Genheden and U. Ryde, Expert Opin. Drug Discovery, 2015, 10, 449–461 CrossRef CAS PubMed.
- F. L. Li and Q. He, et al., J. Mol. Struct., 2021, 1243, 130688 CrossRef.
- C. S. Abraham, S. Muthu, J. C. Prasana, S. J. Armakovic, S. Armakovic, B. F. Rizwana and A. S. B. Geoffrey, Comput. Biol. Chem., 2018, 77, 131–145 CrossRef CAS PubMed.
- M. Khnifira, W. Boumya, M. Abdennouri, M. h. Sadiq, M. Achak, G. Serdaroğlu, S. Kaya, S. Şimşek and N. Barka, Int. J. Biol. Macromol., 2021, 166, 707–721 CrossRef CAS PubMed.
- C. S. Abraham, S. Muthu, J. C. Prasana, S. Armakovic, S. J. Armakovic, B. F. Rizwana, B. Geoffrey and R. H. David, Spectrochim. Acta, Part A, 2019, 222, 117188 CrossRef CAS PubMed.
- C. Wang, D. A. Greene, L. Xiao, R. Qi and R. Luo, Front. Mol. Biosci., 2018, 4, 87 CrossRef PubMed.
- J. Liu and R. Wang, J. Chem. Inf. Model., 2015, 55, 475–482 CrossRef CAS PubMed.
- W. J. Wang, Q. Huang, J. Zou, L. L. Li and S. Y. Yang, Chem. Biol. Drug Des., 2015, 86, 1–8 CrossRef CAS PubMed.
- S. K. Mishra and J. Koca, J. Phys. Chem. B, 2018, 122, 8113–8121 CrossRef CAS PubMed.
- S. Zhong, K. Huang, S. Luo, S. Dong and L. Duan, Phys. Chem. Chem. Phys., 2020, 22, 4240–4251 RSC.
- T. S. Lee, Y. Hu, B. Sherborne, Z. Guo and D. M. York, J. Chem. Theory Comput., 2017, 13, 3077–3084 CrossRef CAS PubMed.
- D. J. Mermelstein, C. Lin, G. Nelson, R. Kretsch, J. A. McCammon and R. C. Walker, J. Comput. Chem., 2018, 39, 1354–1358 CrossRef CAS PubMed.
- P. Chaskar, V. Zoete and U. F. Rohrig, J. Chem. Inf. Model., 2014, 54, 3137–3152 CrossRef CAS PubMed.
- T. Kamisawa, L. D. Wood, T. Itoi and K. Takaori, Lancet, 2016, 388, 73–85 CrossRef CAS PubMed.
- W. Wu, X. Liu, L. Wei, T. Li, Y. Zang, Y. Qian, T. Bai, J. Li, M. Xie, Y. Zhu, Q. Wang and L. Wang, Front. Pharmacol., 2018, 9, 857 CrossRef PubMed.
- Q. Wang, H. Jiang, C. Ping, R. Shen, T. Liu, J. Li, Y. Qian, Y. Tang, S. Cheng, W. Yao and L. Wang, Pharm. Res., 2014, 32, 793–805 CrossRef PubMed.
- J. P. Zhang, X. H. Tian, Y. X. Yang, Q. X. Liu, Q. Wang, L. P. Chen, H. L. Li and W. D. Zhang, J. Ethnopharmacol., 2016, 178, 155–171 CrossRef CAS PubMed.
- S. J. Lee, K. Park, S. D. Ha, W. J. Kim and S. K. Moon, Phytother. Res., 2010, 24, 1870–1876 CrossRef CAS PubMed.
- P. J. Yi and S. M. Oh, BMC Complementary Altern. Med., 2012, 12, 243 CrossRef PubMed.
- J. H. P. Jae-Chul Lim, M. Budesinsky, A. Kasal, Y.-H. Han, B.-S. Koo, S.-I. Lee and D.-U. Lee, Chem. Pharm. Bull., 2005, 53, 561–564 CrossRef PubMed.
- D. Raffa, B. Maggio, M. V. Raimondi, F. Plescia and G. Daidone, Eur. J. Med. Chem., 2017, 142, 213–228 CrossRef CAS PubMed.
- K. Schlick, M. Grundbichler, J. Auberger, J. M. Kern, M. Hell, F. Hohla, G. Hopfinger and R. Greil, Infect. Agents Cancer, 2015, 10, 45 CrossRef PubMed.
- M. M. F. Maaike, J. Smelt, B. J. d. Haan, C. Draijer, G. C. G. Hugenholtz, A. de Haan, M. A. Engelse, E. J. P. d. Koning and P. d. Vos, Pancreas, 2012, 41, 39–49 CrossRef PubMed.
|
This journal is © The Royal Society of Chemistry 2023 |