DOI:
10.1039/D3RA00732D
(Paper)
RSC Adv., 2023,
13, 24291-24308
Molecular dynamics simulation approach of hybrid chalcone–thiazole complex derivatives for DNA gyrase B inhibition: lead generation†
Received
3rd February 2023
, Accepted 31st July 2023
First published on 14th August 2023
Abstract
Compounds bearing thiazole and chalcone groups have been reported to be excellent leads for antibacterial, antitubercular and anticancer activities. In view of this, we performed quantitative structure–activity relationship studies using QSARINS for dataset preparation and for developing validated QSAR models that can predict novel series of thiazole–chalcone hybrids and further evaluate them for bioactivities. The molecular descriptors AATS8i, AVP-1, MoRSEE17 and GATSe7 were found to be active in predicting the structure–activity relationship. Molecular docking and dynamics simulation studies of the developed leads have shown insights into structural analysis. Furthermore, computational studies using AutoDock and Desmond predicted the key binding interactions responsible for the activity and the SwissADME tool computed the in silico drug likeliness properties. The lead compound 178 generated through this study creates a route for the optimization and development of novel drugs against tuberculosis infections. RMSD, RMSF, RoG, H-bond and SASA analysis confirmed the stable binding of compound 178 with the 6J90 structure. In addition, MM-PBSA and MM-GBSA also confirm the docking results. We propose the designed compound 178 as the best theoretical lead, which may further be experimentally studied for selective inhibition.
Introduction
Although most pharmaceuticals have heterocyclic rings, their chemistry is crucial in the development of therapeutic compounds.1 This illustrates how heterocyclic chemistry has influenced the design and development of potential therapeutics. Due to their many biological actions, organic and medicinal chemists have researched thiazole derivatives in great detail.2,3 Many anti-cancer medications have a thiazole ring, including bleomycin, dasatinib, tiazofuran, and epothiolone B. Other thiazole-based drugs include sulfathiazole, ravuconazole, abafungin, acinitrazole, micrococcin, penicillins, cephalosporins, nitazoxanide, ritonavir, meloxicam, febuxostat, famotidine, nizatidine, pramipexole, chlormethiazole and thiamine. A class of open chain natural flavonoids called chalcones has potential biological properties. Chalcones are diaryl vinyl ketones serving as a crucial synthon for augmenting the pharmacological value, in addition to being responsible for the biological action of chalcones.4,5
A wide range of bioactivities, including anticancer,5–19 antitubercular,20–31 antifungal,32–39 antioxidant40–45 and antibacterial,46–54 are present in thiazole and chalcone derivatives. The degree of a particular biological activity is determined by the type of aryl ring attached to the chalcone and the variety of substituents on the thiazole ring. Fluoro and chloro-substituted chalcones exhibit increased biological activity. Interestingly, the kinetic properties of drug-like candidates are improved by the insertion of halogen-modified aryl rings and heterocyclic structures.55 Molecular hybridization for joining biologically active pharmacophoric groups is a strategy that will result in compounds with greater bioactivity.56 In the past, thiazole–chalcone hybrids with therapeutic properties have been described.57,58 In light of the aforementioned information, we generated and validated novel thiazole–chalcone hybrids incorporating biologically energetic thiazole and chalcone pharmacophores in an effort to identify new lead compounds with enhanced anticipated biological activities.
Experiment and methods
Multiple linear regression models were developed and generally validated by options available within the software QSARINS according to the chemometric approach.59–61 The dataset series of forty-seven chalcone derivatives showing Staphylococcus aureus inhibition value were taken from the literature reported by Liaras et al.62 and Geronikaki et al.63 The dataset compounds were subjected to drug likeliness property checking by Lipinski's rule using the DRULITO tool. The IC50 values of the dataset compounds were converted to their respective logarithmic values; pIC50 and numbering were reported for ease against literature reference papers.62,63 Table 1 displays the dataset compounds along with their IC50 and pIC50 values.
Table 1 Antibacterial activity of the dataset compounds against Staphylococcus aureus: structure, and experimental IC50 and pIC50 values
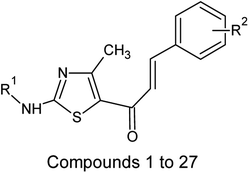
|
Sl. no |
R1 |
R2 |
Sl. no |
R1 |
R2 |
1 |
CH3 |
H |
15 |
CH3 |
2,3-diCl |
2 |
CH3 |
4-NO2 |
16 |
C2H5 |
H |
3 |
CH3 |
3-NO2 |
17 |
C2H5 |
4-NO2 |
4 |
CH3 |
4-Cl |
18 |
C2H5 |
3-NO2 |
5 |
CH3 |
3-Cl |
19 |
C2H5 |
4-Cl |
6 |
CH3 |
2-Cl |
20 |
C2H5 |
3-Cl |
7 |
CH3 |
4-OCH3 |
21 |
C2H5 |
2-Cl |
8 |
CH3 |
2-OCH3 |
22 |
C2H5 |
4-F |
9 |
CH3 |
2,6-diCl |
23 |
C2H5 |
3-F |
10 |
CH3 |
2,4-diCl |
24 |
C2H5 |
3-Br |
11 |
CH3 |
4-F |
25 |
C2H5 |
4-OCH3 |
12 |
CH3 |
3-F |
26 |
C2H5 |
2-OCH3 |
13 |
CH3 |
3-Br |
27 |
C3H7 |
H |
14 |
CH3 |
4-CH3 |
|
|
|
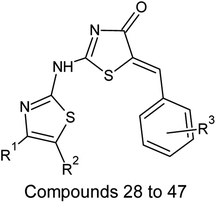
|
Sl. no |
R1 |
R2 |
R3 |
28 |
H |
H |
3-OH |
29 |
H |
H |
3,5-Dimethoxy, 4-OH |
30 |
H |
H |
2-Methoxy |
31 |
H |
H |
2,5-Dimethoxy |
32 |
H |
H |
4-CH3 |
33 |
H |
H |
3-F |
34 |
H |
H |
4-F |
35 |
H |
H |
3-Br |
36 |
H |
H |
4-Br |
37 |
H |
H |
4-Dimethyl amino |
38 |
H |
H |
2,6-diCl |
39 |
H |
H |
2,3-diCl |
40 |
H |
H |
2,3-diCl |
41 |
CH3 |
H |
2-Cl |
42 |
CH3 |
H |
4-Cl |
43 |
H |
CH3 |
4-NO2 |
44 |
Phenyl |
H |
4-Cl |
45 |
Phenyl |
H |
2-Cl |
46 |
Phenyl |
H |
3-Cl |
47 |
Phenyl |
H |
4-Cl |
Molecule |
IUPAC name |
Exp IC50 (nM) |
pIC50 |
1 (Training) |
(2E)-1-[4-methyl-2-(methylamino)-1,3-thiazol-5-yl]-3-phenylprop-2-en-1-one |
329.4 |
6.48 |
2 (Training) |
(2E)-1-[4-methyl-2-(methylamino)-1,3-thiazol-5-yl]-3-(4-nitrophenyl)prop-2-en-1-one |
247.5 |
6.61 |
3 (Training) |
(2E)-1-[4-methyl-2-(methylamino)-1,3-thiazol-5-yl]-3-(3-nitrophenyl)prop-2-en-1-one |
280.5 |
6.56 |
4(Test) |
(2E)-3-(4-chlorophenyl)-1-[4-methyl-2-(methylamino)-1,3-thiazol-5-yl]prop-2-en-1-one |
290.5 |
6.54 |
5 (Training) |
(2E)-3-(3-chlorophenyl)-1-[4-methyl-2-(methylamino)-1,3-thiazol-5-yl]prop-2-en-1-one |
290.5 |
6.54 |
6 (Training) |
(2E)-3-(2-chlorophenyl)-1-[4-methyl-2-(methylamino)-1,3-thiazol-5-yl]prop-2-en-1-one |
305.8 |
6.51 |
7 (Training) |
(2E)-3-(4-methoxyphenyl)-1-[4-methyl-2-(methylamino)-1,3-thiazol-5-yl]prop-2-en-1-one |
341.8 |
6.47 |
8 (Training) |
(2E)-3-(2-methoxyphenyl)-1-[4-methyl-2-(methylamino)-1,3-thiazol-5-yl]prop-2-en-1-one |
347.2 |
6.46 |
9 (Training) |
(2E)-3-(2,6-dichlorophenyl)-1-[4-methyl-2-(methylamino)-1,3-thiazol-5-yl]prop-2-en-1-one |
305.8 |
6.51 |
10 (Training) |
(2E)-3-(2,4-dichlorophenyl)-1-[4-methyl-2-(methylamino)-1,3-thiazol-5-yl]prop-2-en-1-one |
305.8 |
6.64 |
11 (Training) |
(2E)-3-(4-fluorophenyl)-1-[4-methyl-2-(methylamino)-1,3-thiazol-5-yl]prop-2-en-1-one |
54.3 |
7.27 |
12 (Training) |
(2E)-3-(3-fluorophenyl)-1-[4-methyl-2-(methylamino)-1,3-thiazol-5-yl]prop-2-en-1-one |
72.5 |
7.14 |
13(Test) |
(2E)-3-(3-bromophenyl)-1-[4-methyl-2-(methylamino)-1,3-thiazol-5-yl]prop-2-en-1-one |
59.3 |
7.23 |
14 (Training) |
(2E)-1-[4-methyl-2-(methylamino)-1,3-thiazol-5-yl]-3-(3-methylphenyl)prop-2-en-1-one |
73.5 |
7.13 |
15 (Training) |
(2E)-3-(2,3-dichlorophenyl)-1-[4-methyl-2-(methylamino)-1,3-thiazol-5-yl]prop-2-en-1-one |
61.2 |
7.21 |
16 (Training) |
(2E)-1-[2-(ethylamino)-4-methyl-1,3-thiazol-5-yl]-3-phenylprop-2-en-1-one |
36.8 |
7.43 |
17(Test) |
(2E)-1-[2-(ethylamino)-4-methyl-1,3-thiazol-5-yl]-3-(4-nitrophenyl)prop-2-en-1-one |
47.3 |
7.36 |
18 (Training) |
(2E)-1-[2-(ethylamino)-4-methyl-1,3-thiazol-5-yl]-3-(3-nitrophenyl)prop-2-en-1-one |
47.3 |
7.36 |
19 (Training) |
(2E)-3-(4-chlorophenyl)-1-[2-(ethylamino)-4-methyl-1,3-thiazol-5-yl]prop-2-en-1-one |
49.0 |
7.31 |
20 (Training) |
(2E)-3-(3-chlorophenyl)-1-[2-(ethylamino)-4-methyl-1,3-thiazol-5-yl]prop-2-en-1-one |
49.1 |
7.31 |
21 (Training) |
(2E)-3-(2-chlorophenyl)-1-[2-(ethylamino)-4-methyl-1,3-thiazol-5-yl]prop-2-en-1-one |
48.9 |
7.31 |
22 (Training) |
(2E)-1-[2-(ethylamino)-4-methyl-1,3-thiazol-5-yl]-3-(4-fluorophenyl)prop-2-en-1-one |
51.7 |
7.29 |
23 (Training) |
(2E)-1-[2-(ethylamino)-4-methyl-1,3-thiazol-5-yl]-3-(3-fluorophenyl)prop-2-en-1-one |
51.7 |
7.29 |
24 (Training) |
(2E)-3-(3-bromophenyl)-1-[2-(ethylamino)-4-methyl-1,3-thiazol-5-yl]prop-2-en-1-one |
57.1 |
7.24 |
25(Test) |
(2E)-1-[2-(ethylamino)-4-methyl-1,3-thiazol-5-yl]-3-(4-methoxyphenyl)prop-2-en-1-one |
49.7 |
7.3 |
26 (Training) |
(2E)-1-[2-(ethylamino)-4-methyl-1,3-thiazol-5-yl]-3-(2-methoxyphenyl)prop-2-en-1-one |
33.1 |
7.48 |
27 (Training) |
E(2E)-1-[4-methyl-2-(propylamino)-1,3-thiazol-5-yl]-3-phenylprop-2-en-1-one |
70.0 |
7.15 |
28(Test) |
(5Z)-5-[(3-hydroxyphenyl)methylidene]-2-[(1,3-thiazol-2-yl)amino]-1,3-thiazol-4(5H)-one |
110 |
6.96 |
29(Test) |
(5Z)-5-[(4-hydroxy-3,5-dimethoxyphenyl)methylidene]-2-[(1,3-thiazol-2-yl)amino]-1,3-thiazol-4(5H)-one |
31.3 |
7.5 |
30 (Training) |
(5Z)-5-[(2-methoxyphenyl)methylidene]-2-[(1,3-thiazol-2-yl)amino]-1,3-thiazol-4(5H)-one |
115 |
6.94 |
31 (Training) |
(5Z)-5-[(2,5-dimethoxyphenyl)methylidene]-2-[(1,3-thiazol-2-yl)amino]-1,3-thiazol-4(5H)-one |
33.0 |
7.48 |
32 (Training) |
(5Z)-5-[(4-methylphenyl)methylidene]-2-[(1,3-thiazol-2-yl)amino]-1,3-thiazol-4(5H)-one |
65.4 |
7.18 |
33 (Training) |
(5Z)-5-[(3-fluorophenyl)methylidene]-2-[(1,3-thiazol-2-yl)amino]-1,3-thiazol-4(5H)-one |
32.7 |
7.49 |
34 (Training) |
(5Z)-5-[(4-fluorophenyl)methylidene]-2-[(1,3-thiazol-2-yl)amino]-1,3-thiazol-4(5H)-one |
14.6 |
7.84 |
35(Test) |
(5Z)-5-[(3-bromophenyl)methylidene]-2-[(1,3-thiazol-2-yl)amino]-1,3-thiazol-4(5H)-one |
27.3 |
7.56 |
36 (Training) |
(5Z)-5-[(4-bromophenyl)methylidene]-2-[(1,3-thiazol-2-yl)amino]-1,3-thiazol-4(5H)-one |
15.2 |
7.81 |
37 (Training) |
(5Z)-5-{[4-(dimethylamino)phenyl]methylidene}-2-[(1,3-thiazol-2-yl)amino]-1,3-thiazol-4(5H)-one |
28.1 |
7.55 |
38 (Training) |
(5Z)-5-[(2,6-dichlorophenyl)methylidene]-2-[(1,3-thiazol-2-yl)amino]-1,3-thiazol-4(5H)-one) |
28.1 |
7.55 |
39 (Training) |
(5Z)-5-[(2,3-dichlorophenyl)methylidene]-2-[(1,3-thiazol-2-yl)amino]-1,3-thiazol-4(5H)-one |
28.1 |
7.55 |
40 (Training) |
(5Z)-5-[(2,3-dichlorophenyl)methylidene]-2-[(1,3-thiazol-2-yl)amino]-1,3-thiazol-4(5H)-one |
119.2 |
6.92 |
41 (Training) |
(5Z)-5-[(2-chlorophenyl)methylidene]-2-[(4-methyl-1,3-thiazol-2-yl)amino]-1,3-thiazol-4(5H)-one |
30.0 |
7.52 |
42 (Training) |
((5Z)-5-[(4-chlorophenyl)methylidene]-2-[(4-methyl-1,3-thiazol-2-yl)amino]-1,3-thiazol-4(5H)-one |
29.0 |
6.54 |
43 (Training) |
(5Z)-2-[(5-methyl-1,3-thiazol-2-yl)amino]-5-[(4-nitrophenyl)methylidene]-1,3-thiazol-4(5H)-one |
27.0 |
6.57 |
44(Test) |
(5Z)-5-benzylidene-2-[(4-phenyl-1,3-thiazol-2-yl)amino]-1,3-thiazol-4(5H)-one |
25.0 |
6.6 |
45 (Training) |
(5Z)-5-[(2-chlorophenyl)methylidene]-2-[(4-phenyl-1,3-thiazol-2-yl)amino]-1,3-thiazol-4(5H)-one |
25.2 |
6.59 |
46 (Training) |
(5Z)-5-[(3-chlorophenyl)methylidene]-2-[(4-phenyl-1,3-thiazol-2-yl)amino]-1,3-thiazol-4(5H)-one |
50.0 |
7.3 |
47 (Training) |
(5Z)-5-[(4-chlorophenyl)methylidene]-2-[(4-phenyl-1,3-thiazol-2-yl)amino]-1,3-thiazol-4(5H)-one |
17.0 |
7.77 |
Molecule structure preparation and 3D geometry optimization
Compound structures were drawn and subjected to the geometry optimization tool of Avogadro V1.2.0 by applying MMFF94, molecular mechanics force field and steepest descent algorithm.64 Various molecular formats were obtained from Open Babel V2.4.1 wherever necessary.65 The best conformer for each dataset compound was obtained from Avogadro's genetic algorithm tool, using the ‘energy’ scoring function.
Data setup. The aforementioned molecules were subjected to PaDEL software and other descriptor calculation tools of Chemdes-Chemopy servers to calculate their respective values.66 The variables were pre-filtered and organized by removing all-zero value, missing value and semi-constant or near constant value (>50%) descriptors. The pairwise correlation was utilized to filter out 786 descriptors with >0.85 value. A total of 38 variables having >0.40 cut-off correlation value were selected for the study. For compound selection, a cut-off value of 0.85 pairwise correlations was considered, from which 47 compounds were included in the study, partitioned into training and test sets in a 4
:
1 ratio based on the response order. Out of many models obtained from different trials, the best model follow-up procedure is discussed here.
Variable selection and model calculation. Various options in the QSARINS software explore the possible combinations of descriptors selected.59–61 The genetic algorithm was employed for the structure bioactivity relationship and utilized for model fitness evaluation. A LOF smoothness level of 1.0, population size of 200, mutation probability of 0.1 and maximum generations of 5000 were chosen to explore more combinations.
Model validation. QSARINS models were subjected to validation criteria, both internal and external, along with applicability domain checking. Q2LMO was internally evaluated 5000 times with 30% of objects left randomly from the training set each time. The Y-scrambling procedure was set to 5000 iterations, which involve shuffling of the response data, to avoid chance correlation, meaning that the R2 and Q2loo values must be logically higher than the scrambled ones and the RMSE under prediction should be smaller than the scrambled ones. For predictivity of the models, various external validation parameters, implemented in QSARINS, were analyzed.67 The leverage approach is represented by the subsequent Williams plot. The leverage or critical value (hat) was calculated by hi = xi (XT X)−1 xTi (i = 1, 2, …, m), where xi = the query compound's descriptor row-value and m = the number of query compounds. X was an n*p matrix for the training set, where n = training set samples count and p = model descriptors count. The limit of model domain, h* is 3 (p + 1)/n, which was the leverage cut-off value. A leverage greater than h* for the training set means that the compound is highly significant in model determination and in the test set (X outlier), the prediction will be model extrapolation. Compounds with a standardized residual greater than 2.5 σ (2.5 standard deviation units) were considered as Y outliers.
In silico ADMET studies. The compounds with the best predicted model activity values were considered for in silico ADMET predictions using SWISS-ADME,68 ADMESAR69 and ProToxII70 servers. The results are discussed in detail. Furthermore, docking studies were carried out for all the selected compounds.
Molecular docking studies. Autodock V4.2.6 was used to assess the affinities and interactions of DNA gyrase B inhibitors in conjunction with the created QSAR model.71 The protein data bank provides the initial structure of the 3D structure of the DNA GyrB, pdb id: 6J90 (https://w3.rcsb.org). Missing residues were corrected using the Modeller V9.23 programme,72 along with the addition of hydrogen atoms and the removal of preexisting ligands. To find docking parameters useful for docking-specified molecules, co-crystallized ligand to 6J90 redocking was carried out. All of the ligand structures were geometry optimized using Avogadro V1.2.0's steepest descent technique with a convergence parameter of 10 × 10−7 and the MMFF94 force field. Wherever necessary, file format conversions were made using Open Babel V2.4.1.Proteins were created by geometrically adding polar hydrogens and assigning Kollman's united atom charges to create a pdbqt file. Along with the addition of polar hydrogens, the ligand is prepared by adding gasteiger charges. Torsions in the ligands were discovered, and a pdbqt file was created. The grid size was set to 60*60*60 points with a spacing of 0.375 Å and a distance-dependent function of the dielectric constant was used to calculate the energetic map. The autogrid option renders a selection of active sites. The grid box contains the enzyme's active binding site and has room for the ligand's rotational and translational movement.
For investigating ligand conformation poses and orientations inside the active site of DNA gyrase B, a Lamarckian evolutionary approach was employed. The following were the optimised parameters: the maximum number of energy assessments per run was increased to 25,000,000, the population size was set at 150, the maximum number of generations was 2700, and the gene mutation rate was set at 0.02. The default settings were used for all other variables. In a positional RMSD, results that varied by two points were grouped together. The representative of each group was the lowest binding energy configuration with the highest percentage frequency. The Discovery studio visualizer 2020 version 20.1.0.19295 (ref. 73) application created and displayed representations of ligand postures and interactions displayed.
Molecular dynamics simulations
Based on the Autodock binding energy of protein–ligand complexes, the best ligands from the designer dataset as well as reference compounds were identified and taken over for molecular dynamics simulations to study the physical changes in the atoms and molecules upon interacting with the solvent environment using the Desmond package of the Schrodinger 2021 molecular modelling suite.74
Results and discussion
Model information
The selected dataset molecules were optimized for geometry and by using MMFF94. ∼3000 descriptors were calculated using PaDEL software, on the Chemopy-chemdes (RDKit and Bluedesc) server. The dataset was divided into training and test sets based on chemical and biological diversity. Several QSAR model equations were generated using QSARINS. Some of the models displayed higher R2 and Q2LOO values, but their external validation was not good along with too many outliers. Generally, the QSAR model should be cross validated to check its internal performance (robustness) and external performance given to be predictive capacity (predictability).
Model 1 developed with the aforementioned options gave optimal statistical values along with one outlier (compound 43 from training set) in the Williams plot at the 2.5 standard deviation unit level, and high ‘RMSE’ value and low Q2LOO and Q2LMO values. Subsequently, model 2 was generated and analyzed for outliers and betterment of the statistical values. Finally, the outliers (compounds 43, 33 and 27 from training set) were removed from the dataset and analyzed further for generating final validated model 3. The statistical parameters of models 1 and 2 are presented in ESI Table 1.†
Model 3:
pIC50 = −9.1545 + 0.0798 (AATS8i) + 10.0218 (AVP-1) + 0.3957 (MoRSEE17) + 0.5990 (GATSe7).
ntr = 36, npred = 08, R2 = 0.7065, R2adj = 0.6687, R2–R2adj = 0.0379, LOF = 0.0894, RMSEtr = 0.2325, MAEtr = 0.1873, RSStr = 1.9466, CCCtr = 0.8280, s = 0.2506, F = 18.6577, Q2LOO = 0.6013, Q2LMO = 0.5701, R2Yscr = 0.1150, Q2Yscr = −0.1966, RMSEcv = 0.2710, MAEcv = 0.2182, PRESScv = 2.6444, CCCcv = 0.7686, R2ext = 0.7540, MAEext = 0.1911, PRESSext = 0.3199, RMSEext = 0.200, CCCext = 0.8509, Q2F1 = 0.7022, Q2F2 = 0.7015, Q2F3 = 0.7830.
Model 3 showed good fitting criteria with validation values. Compared to previous models, model 3 showed better internal validation parameters with no outliers in the Williams plot. The model 3 descriptor correlation matrix is represented in Table 2. The scatter plot denotes the experimental vs. calculated inhibitory activities of chalcone derivatives and Fig. 1 displays predicted values similar to parallel experimental values. The Kxy, the inter-correlation among descriptors and response vs. Q2LMO of model 3 are plotted in ESI Fig. 1† showing the LMO parameter values around the model parameters, meaning that the model is robust and stable. ESI Fig. 2† displays the Y-scramble plot of Kxy vs. R2Yscr and Q2Yscr, which means that the correlation coefficients of model 3 are much greater than those after endpoint scrambling and a broken relationship can be noticed between the structure and responses. Standardized residuals vs. leverage values shown in Fig. 2 as the Williams plot illustrate the applicability domain of the model, by which one can depict whether the molecules are located in the applicability domain of the model or not. From the plot, the leverage values were found to be lower than the warning h* of 0.417. The Q2F1, Q2F2 and Q2F3 values are near to the threshold value of 0.70. These results state that there is no chance correlation and truly there is a meaningful relationship between the chalcone derivatives with corresponding inhibitory activity. Model 3 containing molecular descriptors contributed structural information relating to the predicted bioactivity and the information obtained is compared to structure activity relationship studies of the dataset. The molecular descriptors contributing to model 3 were discussed in detail in ref. 75–77. Table 3 displays residual information of compound bioactivity compared with the experimental vs. QSAR best model.
Table 2 The model 3 descriptor correlation matrix
|
AATS8i |
AVP-1 |
MoRSEE17 |
GATSe7 |
AATS8i |
1.0000 |
|
|
|
AVP-1 |
−0.1249 |
1.0000 |
|
|
MoRSEE17 |
0.0949 |
0.0704 |
1.0000 |
|
GATSe7 |
0.0330 |
0.3081 |
0.1359 |
1.0000 |
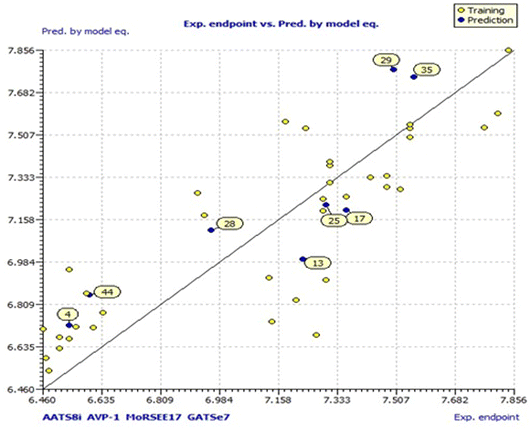 |
| Fig. 1 Model 3 scatter plot. | |
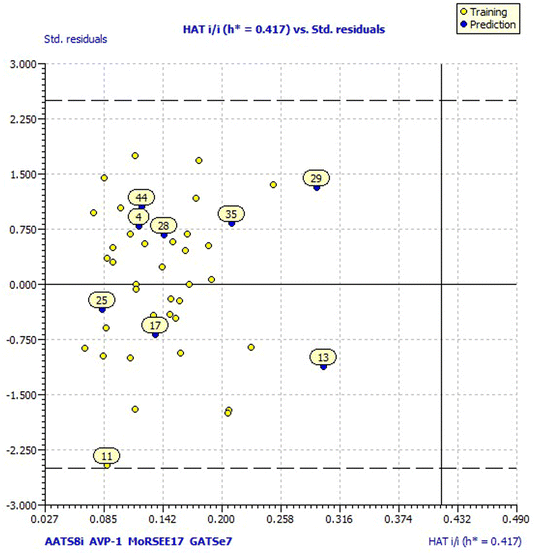 |
| Fig. 2 Model 3 Williams plot. | |
Table 3 pIC50 values of the dataset predicted by model 3 along with residuals
Compound |
Exp pIC50 |
Pred pIC50 |
Residuals |
1 |
6.4800 |
6.5367 |
0.0567 |
2 |
6.6100 |
6.7171 |
0.1071 |
3 |
6.5600 |
6.7181 |
0.1581 |
4 (Test) |
6.5400 |
6.7263 |
0.1863 |
5 |
6.5400 |
6.6708 |
0.1308 |
6 |
6.5100 |
6.6745 |
0.1645 |
7 |
6.4700 |
6.5912 |
0.1212 |
8 |
6.4600 |
6.7083 |
0.2483 |
9 |
6.5100 |
6.6308 |
0.1208 |
10 |
6.6400 |
6.7761 |
0.1361 |
11 |
7.2700 |
6.6836 |
−0.5864 |
12 |
7.1400 |
6.7416 |
−0.3984 |
13 (Test) |
7.2300 |
6.9963 |
−0.2337 |
14 |
7.1300 |
6.9212 |
−0.2088 |
15 |
7.2100 |
6.8289 |
−0.3811 |
16 |
7.4300 |
7.3348 |
−0.0952 |
17 (Test) |
7.3600 |
7.2007 |
−0.1593 |
18 |
7.3600 |
7.2547 |
−0.1053 |
19 |
7.3100 |
7.3843 |
0.0743 |
20 |
7.3100 |
7.3115 |
0.0015 |
21 |
7.3100 |
7.3981 |
0.0881 |
22 |
7.2900 |
7.2469 |
−0.0431 |
23 |
7.2900 |
7.1971 |
−0.0929 |
24 |
7.2400 |
7.5364 |
0.2964 |
25 (Test) |
7.3000 |
7.2208 |
−0.0792 |
26 |
7.4800 |
7.3396 |
−0.1404 |
27 |
7.1500 |
7.1012 |
−0.0487 |
28 (Test) |
6.9600 |
7.1157 |
0.1557 |
29 (Test) |
7.5000 |
7.7784 |
0.2784 |
30 |
6.9400 |
7.1773 |
0.2373 |
31 |
7.4800 |
7.2930 |
−0.1870 |
32 |
7.1800 |
7.5631 |
0.3831 |
33 |
7.4900 |
7.0890 |
−0.4002 |
34 |
7.8400 |
7.8563 |
0.0163 |
35 (Test) |
7.5600 |
7.7473 |
0.1873 |
36 |
7.8100 |
7.5975 |
−0.2125 |
37 |
7.5500 |
7.4993 |
−0.0507 |
38 |
7.5500 |
7.5352 |
−0.0148 |
39 |
7.5500 |
7.5508 |
0.0008 |
40 |
6.9200 |
7.2694 |
0.3494 |
41 |
7.5200 |
7.2850 |
−0.2350 |
42 |
6.5400 |
6.9531 |
0.4131 |
43 |
6.5700 |
6.880 |
0.3103 |
44 (Test) |
6.6000 |
6.8491 |
0.2491 |
45 |
6.5900 |
6.8574 |
0.2674 |
46 |
7.3000 |
6.9113 |
−0.3887 |
47 |
7.7700 |
7.5388 |
−0.2312 |
The molecular descriptors influencing the inhibitory activity are:
(i) AATS8i: This is the “averaged Moreau–Broto autocorrelation of lag 8 weighted by ionization potential”. This descriptor positively affects the activity as per final model information.
(ii) AVP-1: This is “average valence path order 1”, which is a topological descriptor that gives information regarding the connectivity of various atoms in the molecule and is referred to as a connectivity index calculated using the Chi operator. This descriptor depends on the number of lone pair and π electrons. According to the model, this descriptor positively affects the activity.
(iii) MoRSEE17: This is a 3D molecular representation of the structure based on electron diffraction data upon weightage of 17 value for Sanderson electronegativity.
(iv) GATSe7: This is “Geary coefficient of lag 1 weighted by Sanderson electronegativities”. It negatively affects the activity.
SAR studies of the dataset
On analyzing the bioactivity results from the original dataset as shown in Table 1, compounds 1 to 27 having a thiazole ring attached to the chalcone have shown activity compared to the others. Similarly compounds 28 to 47 with substitutions on the R1, R2 and R3 positions also have shown good inhibitory activity. Substitution with electron withdrawing groups at the R2 position of the phenyl ring, such as nitro, decreases the activity, but substitution with fluorine at the ortho, meta and para positions increases the activity gradually. Di-substitution of halogens increases the activity on the phenyl ring. Substitution of methoxy, hydroxy and methyl groups on the phenyl ring also increases the activity. Substitution of heterocyclic groups drastically decreases the activity. Substitution of bulkier groups at the R1 position increases the activity. Based on SAR studies of the original dataset and model equation parameters, two hundred compounds were designed and checked for predicted bioactivity using model 3. Table 4 displays the designed compounds' structural information and Table 5 displays the model predicted bioactivity of the designed compounds.
Table 4 Designer series molecules having chalcone and thiazole hybrid moieties
R2 |
Sl. no |
Group |
Sl. no |
Group |
Sl. no |
Group |
1, 41, 81, 121, 161 |
2-OH |
16, 56, 96, 136, 176 |
2-OH, 6-OH |
31, 71, 111, 151, 191 |
2-CH3, 6-CH3 |
2, 42, 82, 122, 162 |
2-NO2 |
17, 57, 97, 137, 177 |
2-OH, 5-CH3 |
32, 72, 112, 152, 192 |
2-OCH3, 3-OCH3, 5-Br |
3, 43, 83, 123, 163 |
2-F |
18, 58, 98, 138, 178 |
3-CH3, 4-OH |
33, 73, 113, 153, 193 |
2-OCH3, 3-OCH3, 5-Cl |
4, 44, 84, 124, 164 |
2-CF3 |
19, 59, 99, 139, 179 |
2-OH, 3-CH3 |
34, 74, 114, 154, 194 |
2-OCH3, 6-OCH3, 4- |
5, 45, 85, 125, 165 |
2-CH3 |
20, 60, 100, 140, 180 |
2-OH, 4-OH, 6-CH3 |
35, 75, 115, 155, 195 |
2-OCH3, 4-OCH3, 5-Br |
6, 46, 86, 126, 166 |
2-OC2H5 |
21, 61, 101, 141, 181 |
2-CH3, 4-OH |
36, 76, 116, 156, 196 |
2-OCH3, 4-OCH3 |
7, 47, 87, 127, 167 |
3-OC2H5 |
22, 62, 102, 142, 182 |
2-OH, 3-CH3, 5-F |
37, 77, 117, 157, 197 |
3-OCH3, 4-OCH3 |
8, 48, 88, 128, 168 |
4-OC2H5 |
23, 63, 103, 143, 183 |
3-CH3, 5-CH3 |
38, 78, 118, 158, 198 |
2-OCH3, 6-OCH3 |
9, 49, 89, 129, 169 |
4-C3H7 |
24, 64, 104, 144, 184 |
3-CH3, 4-OH, 5-CH3 |
39, 79, 119, 159, 199 |
3-OCH3, 5-OCH3 |
10, 50, 90, 130, 170 |
4- |
25, 65, 105, 145, 185 |
3-CH3, 5-CH3, 4- |
40, 80, 120, 160, 200 |
2-OCH3, 3-OCH3 |
11, 51, 91, 131, 171 |
4- |
26, 66, 106, 146, 186 |
2-OH, 3-CH3, 5-CH3 |
|
|
12, 52, 92, 132, 172 |
4-C2H5 |
27, 67, 107, 147, 187 |
3-CH3, 4-F, 5-CH3 |
|
|
13, 53, 93, 133, 173 |
2-OH, 3-OH |
28, 68, 108, 148, 188 |
2-CH3, 5-CH3 |
|
|
14, 54, 94, 134, 174 |
2-OH, 4-OH |
29, 69, 109, 149, 189 |
2-OH, 3-CH3, 4-CH3 |
|
|
15, 55, 95, 135, 175 |
2-OH, 5-OH |
30, 70, 110, 150, 190 |
2-CH3, 4-CH3 |
|
|
Table 5 Model 3 predicted pIC50 values for the designed compounds
Compound |
AATS8i |
AVP-1 |
MoRSEE17 |
GATSe7 |
pIC50 |
C1 |
154.6222 |
0.329386 |
−1.458 |
0.965 |
6.126499 |
C2 |
156.3947 |
0.354614 |
0.873 |
0.759 |
7.319758 |
C3 |
155.1452 |
0.341639 |
0.53 |
1.03 |
7.116621 |
C4 |
155.3775 |
0.350394 |
0.719 |
0.709 |
7.105405 |
C5 |
158.4803 |
0.340196 |
0.971 |
0.829 |
7.422403 |
C6 |
157.8188 |
0.362151 |
0.985 |
1.138 |
7.780273 |
C7 |
158.1324 |
0.353156 |
1.432 |
0.769 |
7.67099 |
C8 |
158.0306 |
0.344036 |
1.29 |
0.814 |
7.542242 |
C9 |
157.5324 |
0.353156 |
1.278 |
0.826 |
7.596317 |
C10 |
159.1588 |
0.351713 |
1.194 |
0.826 |
7.678412 |
C11 |
155.4385 |
0.362411 |
0.899 |
0.79 |
7.350451 |
C12 |
157.4171 |
0.353405 |
0.962 |
0.842 |
7.474156 |
C13 |
153.281 |
0.327647 |
0.523 |
0.88 |
6.735005 |
C14 |
155.3199 |
0.362411 |
0.629 |
0.932 |
7.319209 |
C15 |
153.0582 |
0.362671 |
0.545 |
0.88 |
7.076943 |
C16 |
157.2212 |
0.363802 |
0.525 |
0.998 |
7.483249 |
C17 |
157.5538 |
0.347839 |
0.81 |
1.155 |
7.556633 |
C18 |
160.0419 |
0.330409 |
0.937 |
1.06 |
7.573848 |
C19 |
160.1868 |
0.363802 |
0.65 |
1.155 |
7.86341 |
C20 |
160.6462 |
0.342408 |
0.778 |
0.875 |
7.568591 |
C21 |
158.208 |
0.342408 |
0.844 |
0.798 |
7.354022 |
C22 |
160.2244 |
0.342648 |
0.456 |
0.94 |
7.448853 |
C23 |
161.0717 |
0.342169 |
1.08 |
1.568 |
8.134755 |
C24 |
156.0422 |
0.353276 |
1.255 |
1.244 |
7.719884 |
C25 |
157.7357 |
0.342648 |
1.732 |
0.912 |
7.738393 |
C26 |
151.5597 |
0.338894 |
1.088 |
1.326 |
7.201085 |
C27 |
152.0352 |
0.329295 |
1.239 |
1.065 |
7.046242 |
C28 |
154.5617 |
0.337509 |
0.855 |
1.18 |
7.247114 |
C29 |
154.1035 |
0.323774 |
0.917 |
1.138 |
7.072279 |
C30 |
149.674 |
0.349949 |
1.203 |
0.816 |
6.901419 |
C31 |
154.5698 |
0.34995 |
1.538 |
0.793 |
7.410891 |
C32 |
155.1016 |
0.349728 |
1.231 |
0.999 |
7.453021 |
C33 |
152.1663 |
0.349728 |
1.174 |
0.944 |
7.163285 |
C34 |
152.3011 |
0.363089 |
1.696 |
1.23 |
7.68581 |
C35 |
155.3243 |
0.320381 |
0.955 |
1.045 |
7.095017 |
C36 |
153.74 |
0.358745 |
0.745 |
1.042 |
7.268184 |
C37 |
151.1758 |
0.335595 |
1.036 |
0.776 |
6.787358 |
C38 |
155.4989 |
0.366629 |
1.338 |
1.226 |
7.83242 |
C39 |
152.2192 |
0.331254 |
0.898 |
0.737 |
6.749158 |
C40 |
152.2154 |
0.331024 |
1.238 |
0.993 |
7.034434 |
C41 |
152.2192 |
0.331024 |
1.067 |
1.155 |
7.064107 |
C42 |
151.4043 |
0.331254 |
1.461 |
0.885 |
6.995564 |
C43 |
152.5082 |
0.341655 |
1.031 |
1.142 |
7.171673 |
C44 |
153.2633 |
0.341655 |
1.355 |
0.753 |
7.12713 |
C45 |
152.5082 |
0.341885 |
1.225 |
1.18 |
7.273507 |
C46 |
155.3194 |
0.320096 |
1.48 |
1.312 |
7.459452 |
C47 |
150.25 |
0.334196 |
1.581 |
0.973 |
7.033128 |
C48 |
150.3495 |
0.341655 |
1.52 |
1.024 |
7.122228 |
C49 |
153.9338 |
0.332914 |
1.736 |
1.192 |
7.506753 |
C50 |
153.4696 |
0.352055 |
1.635 |
1.192 |
7.621577 |
C51 |
153.9632 |
0.344433 |
1.228 |
1.153 |
7.400165 |
C52 |
155.8359 |
0.338089 |
1.505 |
1.208 |
7.628591 |
C53 |
153.2788 |
0.344433 |
0.757 |
1.007 |
7.071725 |
C54 |
155.0042 |
0.343151 |
0.739 |
1.06 |
7.221181 |
C55 |
150.7438 |
0.352285 |
0.881 |
1.007 |
6.99719 |
C56 |
153.1473 |
0.344655 |
1.187 |
1.116 |
7.298892 |
C57 |
155.3243 |
0.320096 |
1.238 |
1.326 |
7.372464 |
C58 |
152.1195 |
0.354211 |
1.135 |
1.244 |
7.368744 |
C59 |
148.0179 |
0.352515 |
1.177 |
1.326 |
7.090184 |
C60 |
153.0541 |
0.35458 |
0.906 |
1.003 |
7.212046 |
C61 |
153.4321 |
0.340268 |
0.796 |
1 |
7.053457 |
C62 |
158.1649 |
0.325888 |
1.065 |
1.022 |
7.406644 |
C63 |
156.8756 |
0.35458 |
1.428 |
1.857 |
8.235106 |
C64 |
157.3901 |
0.335149 |
1.476 |
1.411 |
7.833266 |
C65 |
154.1755 |
0.335149 |
1.935 |
1.07 |
7.554108 |
C66 |
156.9177 |
0.335362 |
1.182 |
1.483 |
7.724505 |
C67 |
157.8667 |
0.334935 |
1.422 |
1.176 |
7.70702 |
C68 |
154.2766 |
0.320381 |
1.189 |
1.493 |
7.37236 |
C69 |
153.6387 |
0.335362 |
1.209 |
1.312 |
7.371095 |
C70 |
153.3833 |
0.311854 |
1.343 |
1.166 |
7.080681 |
C71 |
153.7329 |
0.304529 |
1.398 |
1.129 |
7.034779 |
C72 |
155.9892 |
0.310522 |
1.659 |
1.108 |
7.365585 |
C73 |
155.4626 |
0.300053 |
1.319 |
1.041 |
7.043977 |
C74 |
151.6464 |
0.322485 |
2.021 |
1.301 |
7.397769 |
C75 |
155.677 |
0.324447 |
1.521 |
1.154 |
7.453169 |
C76 |
156.1458 |
0.324233 |
1.344 |
1.161 |
7.422593 |
C77 |
153.6774 |
0.324233 |
1.493 |
0.915 |
7.13722 |
C78 |
153.7141 |
0.337117 |
1.516 |
1.328 |
7.525756 |
C79 |
155.4186 |
0.333257 |
1.509 |
0.874 |
7.348385 |
C80 |
154.9298 |
0.332928 |
1.643 |
1.111 |
7.501063 |
C81 |
152.6653 |
0.313728 |
1.568 |
1.081 |
7.080285 |
C82 |
154.5577 |
0.331084 |
1.784 |
0.836 |
7.343959 |
C83 |
153.8906 |
0.305499 |
1.182 |
1.071 |
6.93687 |
C84 |
153.8874 |
0.305278 |
1.551 |
0.717 |
6.868359 |
C85 |
153.8906 |
0.305278 |
1.434 |
1.108 |
7.056528 |
C86 |
153.1922 |
0.305499 |
1.6 |
1.242 |
7.148966 |
C87 |
154.041 |
0.315514 |
1.826 |
0.918 |
7.212425 |
C88 |
154.6947 |
0.315514 |
1.754 |
0.962 |
7.262457 |
C89 |
154.041 |
0.315736 |
1.927 |
1.121 |
7.376208 |
C90 |
156.3708 |
0.333257 |
1.734 |
1.121 |
7.661352 |
C91 |
152.033 |
0.309256 |
1.462 |
1.095 |
6.951449 |
C92 |
152.1723 |
0.315514 |
1.855 |
1.132 |
7.202965 |
C93 |
155.2751 |
0.308019 |
0.906 |
0.948 |
6.889715 |
C94 |
154.7942 |
0.32553 |
1.034 |
0.993 |
7.104429 |
C95 |
155.1906 |
0.319127 |
1.187 |
0.948 |
7.105486 |
C96 |
156.3913 |
0.318583 |
1.097 |
1.051 |
7.22193 |
C97 |
154.5906 |
0.319127 |
1.602 |
1.252 |
7.403919 |
C98 |
156.1342 |
0.31789 |
1.265 |
1.169 |
7.331638 |
C99 |
152.4139 |
0.325751 |
1.55 |
1.252 |
7.276025 |
C100 |
154.4753 |
0.319341 |
1.309 |
0.947 |
7.098224 |
C101 |
155.4186 |
0.333542 |
1.507 |
0.94 |
7.389982 |
C102 |
152.2953 |
0.325751 |
1.003 |
0.97 |
6.881198 |
C103 |
150.0336 |
0.325973 |
1.837 |
1.758 |
7.504959 |
C104 |
154.3579 |
0.330622 |
1.512 |
1.337 |
7.515857 |
C105 |
154.6906 |
0.316788 |
2.309 |
1.043 |
7.54302 |
C106 |
158.5296 |
0.30773 |
1.548 |
1.41 |
7.677302 |
C107 |
157.6303 |
0.330622 |
1.659 |
1.115 |
7.702183 |
C108 |
158.0897 |
0.311035 |
1.712 |
1.413 |
7.742015 |
C109 |
155.3448 |
0.311035 |
1.291 |
1.242 |
7.253953 |
C110 |
157.6679 |
0.311242 |
1.285 |
1.099 |
7.353377 |
C111 |
158.5152 |
0.310829 |
1.635 |
1.069 |
7.537376 |
C112 |
155.0877 |
0.31847 |
1.672 |
1.056 |
7.347296 |
C113 |
152.4747 |
0.324485 |
1.562 |
0.993 |
7.117799 |
C114 |
154.8724 |
0.311242 |
1.476 |
1.272 |
7.3095 |
C115 |
152.6969 |
0.333257 |
2.35 |
1.097 |
7.597547 |
C116 |
157.2161 |
0.322912 |
1.476 |
1.1 |
7.510455 |
C117 |
156.3779 |
0.346134 |
1.462 |
0.864 |
7.529397 |
C118 |
156.8736 |
0.337049 |
1.457 |
1.267 |
7.717314 |
C119 |
157.4161 |
0.331617 |
1.661 |
0.828 |
7.523929 |
C120 |
156.0313 |
0.337049 |
1.577 |
1.056 |
7.571195 |
C121 |
158.2808 |
0.335475 |
−1.46 |
0.97 |
6.481681 |
C122 |
152.9979 |
0.346419 |
0.808 |
0.782 |
6.954621 |
C123 |
155.8694 |
0.337321 |
0.839 |
0.971 |
7.218061 |
C124 |
158.7334 |
0.327655 |
0.561 |
0.68 |
7.065426 |
C125 |
152.8296 |
0.346419 |
0.973 |
0.954 |
7.109505 |
C126 |
149.6179 |
0.346704 |
0.95 |
1.119 |
6.945804 |
C127 |
155.6547 |
0.349925 |
1.182 |
0.83 |
7.378509 |
C128 |
156.1167 |
0.332632 |
1.271 |
0.863 |
7.297051 |
C129 |
159.8323 |
0.317914 |
1.052 |
0.962 |
7.418706 |
C130 |
159.8327 |
0.349925 |
1.35 |
0.962 |
7.857454 |
C131 |
160.4451 |
0.326067 |
0.726 |
0.942 |
7.408336 |
C132 |
157.0253 |
0.326067 |
1 |
0.969 |
7.260031 |
C133 |
159.8828 |
0.326327 |
0.614 |
0.874 |
7.281018 |
C134 |
161.0124 |
0.325807 |
0.282 |
0.909 |
7.255543 |
C135 |
157.5813 |
0.31222 |
0.638 |
0.874 |
6.965482 |
C136 |
156.3693 |
0.326327 |
0.637 |
0.966 |
7.064848 |
C137 |
160.1081 |
0.340399 |
1.029 |
1.127 |
7.755786 |
C138 |
160.2586 |
0.329 |
0.647 |
1.048 |
7.455079 |
C139 |
163.2627 |
0.33875 |
0.684 |
1.127 |
7.854488 |
C140 |
161.943 |
0.322572 |
0.356 |
0.874 |
7.305694 |
C141 |
157.7387 |
0.353561 |
0.789 |
0.848 |
7.436524 |
C142 |
161.1574 |
0.353247 |
0.341 |
0.904 |
7.562462 |
C143 |
161.6664 |
0.352987 |
0.994 |
1.498 |
8.214671 |
C144 |
159.4841 |
0.352987 |
0.917 |
1.202 |
7.832754 |
C145 |
159.1825 |
0.368672 |
1.33 |
0.963 |
7.986136 |
C146 |
151.9438 |
0.343206 |
0.554 |
1.273 |
7.031901 |
C147 |
160.2699 |
0.363573 |
1.247 |
1.019 |
8.022507 |
C148 |
157.814 |
0.336277 |
1.05 |
1.213 |
7.591227 |
C149 |
160.3564 |
0.362702 |
1.313 |
1.119 |
8.106705 |
C150 |
160.4329 |
0.331303 |
1.146 |
1.094 |
7.717072 |
C151 |
160.4293 |
0.331031 |
1.066 |
0.928 |
7.582972 |
C152 |
160.4329 |
0.331031 |
1.044 |
0.988 |
7.610491 |
C153 |
159.6609 |
0.331303 |
0.628 |
0.932 |
7.353459 |
C154 |
160.1691 |
0.343594 |
1.375 |
1.217 |
7.983493 |
C155 |
160.8871 |
0.343594 |
1.269 |
1.021 |
7.881442 |
C156 |
160.1691 |
0.343866 |
0.754 |
1.013 |
7.618292 |
C157 |
157.4539 |
0.343819 |
0.984 |
0.796 |
7.362178 |
C158 |
157.7753 |
0.334754 |
0.937 |
1.048 |
7.429325 |
C159 |
158.1166 |
0.343594 |
0.878 |
0.768 |
7.35409 |
C160 |
161.5245 |
0.333249 |
1.025 |
0.978 |
7.706323 |
C161 |
160.6147 |
0.355885 |
0.743 |
0.987 |
7.75438 |
C162 |
160.8729 |
0.346771 |
1.212 |
0.789 |
7.750623 |
C163 |
159.4946 |
0.339005 |
0.906 |
0.97 |
7.550146 |
C164 |
160.2191 |
0.346771 |
0.879 |
0.672 |
7.496606 |
C165 |
162.0784 |
0.345266 |
0.913 |
1.019 |
7.851196 |
C166 |
158.0147 |
0.356157 |
0.983 |
1.135 |
7.733245 |
C167 |
160.0935 |
0.347031 |
1.144 |
0.86 |
7.70666 |
C168 |
158.1017 |
0.343547 |
1.181 |
0.892 |
7.546605 |
C169 |
157.8852 |
0.356157 |
1.442 |
1.033 |
7.843439 |
C170 |
155.4147 |
0.356429 |
0.982 |
1.033 |
7.466996 |
C171 |
159.8031 |
0.358354 |
0.878 |
1.021 |
7.788141 |
C172 |
160.1646 |
0.341753 |
1.533 |
1.037 |
7.919384 |
C173 |
161.4762 |
0.32527 |
0.42 |
0.878 |
7.323211 |
C174 |
162.6989 |
0.358354 |
0.547 |
0.91 |
7.821762 |
C175 |
163.1936 |
0.335842 |
0.542 |
0.878 |
7.614478 |
C176 |
160.8757 |
0.335842 |
0.743 |
0.965 |
7.561161 |
C177 |
162.7394 |
0.336091 |
0.878 |
1.14 |
7.870625 |
C178 |
163.6518 |
0.335593 |
0.975 |
1.064 |
7.931297 |
C179 |
154.8149 |
0.343547 |
1.075 |
1.14 |
7.390926 |
C180 |
160.3623 |
0.336091 |
0.594 |
0.88 |
7.412812 |
C181 |
156.9037 |
0.347654 |
0.794 |
0.875 |
7.328842 |
C182 |
157.1416 |
0.336125 |
0.489 |
0.899 |
7.125976 |
C183 |
159.6289 |
0.34608 |
0.99 |
1.542 |
8.007633 |
C184 |
158.7664 |
0.329669 |
0.98 |
1.215 |
7.574506 |
C185 |
154.9502 |
0.360217 |
0.789 |
0.999 |
7.37116 |
C186 |
158.5026 |
0.359362 |
1.022 |
1.283 |
7.908383 |
C187 |
158.9714 |
0.359112 |
1.269 |
1.023 |
7.885294 |
C188 |
156.7452 |
0.359112 |
0.882 |
1.269 |
7.701861 |
C189 |
156.6161 |
0.374144 |
0.637 |
1.135 |
7.664984 |
C190 |
154.7802 |
0.359945 |
1.385 |
1.015 |
7.600291 |
C191 |
157.7187 |
0.369257 |
1.318 |
0.995 |
7.889613 |
C192 |
155.4542 |
0.342124 |
1.256 |
0.988 |
7.408255 |
C193 |
157.5823 |
0.368672 |
1.228 |
0.93 |
7.798315 |
C194 |
157.2993 |
0.338637 |
1.867 |
1.219 |
7.900692 |
C195 |
157.2961 |
0.338377 |
0.651 |
1.019 |
7.296857 |
C196 |
157.2993 |
0.338377 |
1.231 |
1.014 |
7.523625 |
C197 |
156.6009 |
0.338637 |
1.143 |
0.808 |
7.312281 |
C198 |
157.2462 |
0.350394 |
1.257 |
1.169 |
7.742952 |
C199 |
157.8999 |
0.350394 |
0.897 |
0.781 |
7.420255 |
C200 |
157.2462 |
0.350654 |
1.215 |
0.982 |
7.616927 |
Study of the applicability domain of the QSAR model to the newly designed compounds
The designed compounds by SAR analysis predicted for pIC50 applying model 3 have been analyzed for their distribution in the chemical space of the original dataset defined by the model descriptors by applying a Principal Component Analysis (PCA).
The PCA loading plot of the original dataset with 44 molecules for model 3 descriptors is presented in ESI Fig. 3† having a Principal Component 1 (PC1) value of 37.20% and PC2 value of 25.54%. The score plot of the original dataset with 44 molecules for model 3 presented in Fig. 3 showcases the chemical space ranging from the X-axis: −3.139 to 2.289 and Y-axis: −2.425 to 2.304 data points.
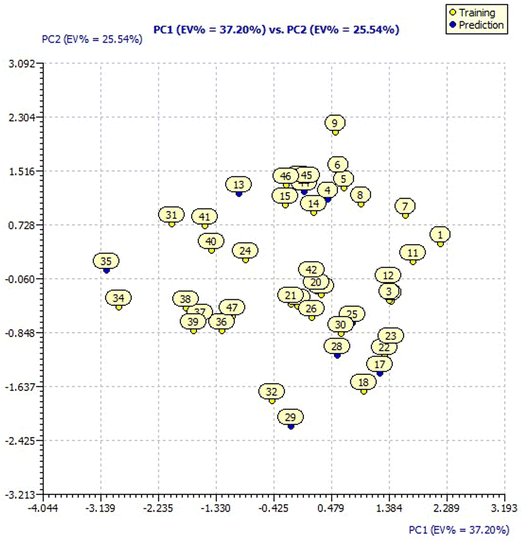 |
| Fig. 3 PCA score plot of model 3 with compound names. | |
For a better understanding of the structural diversity of the designed compounds, the Williams plot and scatter plot are presented in Fig. 4 and 5, respectively. Fig. 4 envisages the h* value of 0.076 notified using model 3 for all the compounds. C1 and C121 of the designer series were identified as X-outliers, distant from the applicability domain, and C63, C103, 31, 33, 34, 35 and 43 were identified as Y-outliers as explored by model 3. In Fig. 5, the scatter plot for all the dataset compounds and designed molecules shows 33 and C1, C121 distant from the regression line of model 3.
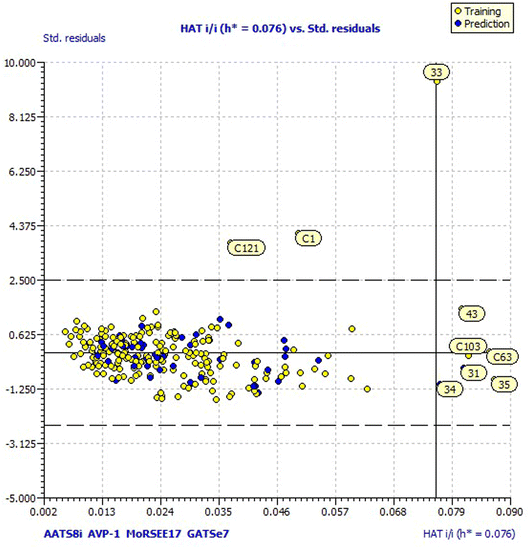 |
| Fig. 4 Williams plot of the designed and dataset compounds. | |
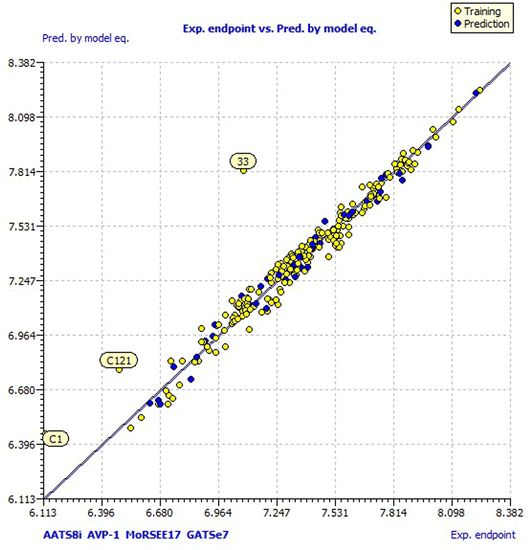 |
| Fig. 5 Scatter plot of the designed and dataset compounds. | |
Similarly, the loading plot of both the designed and dataset compounds for the model 3 descriptors presented a PC1 value of 36.39% and PC2 value of 23.82% in ESI Fig. 4.† The score plots of both the designed and dataset compounds for model 3 presented in Fig. 6 showcase the chemical space ranging from the X-axis: −2.497 to 3.307 and Y-axis: −2.170 to 2.938 data points.
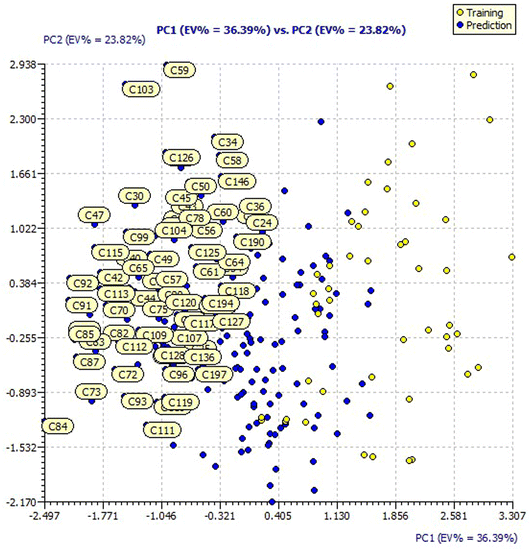 |
| Fig. 6 PCA score plot of the designed and dataset compounds. | |
It is evident that the majority of designed molecules have been part of interpolation of the dataset and inside the structural applicability domain. The designed compounds having substitutions of 3-methyl, 3-methoxy or their combination at the R2 position were found to be out of the applicability domain of the proposed model and therefore their predictions were extrapolated. The data predicted for these compounds are, at least, less reliable than those of the other compounds. It's noteworthy that 2-hydroxyl groups and compounds having a combination of groups at the R2 position have shown excellent predicted activity as well as structural domain applicability (compounds C53 and C54, C93 and C94, C133 and C134, C173 and C174). In a similar case, the applicability region specified that a 5-Br group at the R2 position is not beneficial (on comparing the pIC50 values of 35 and 36, 75 and 76, 115 and 116, 155 and 156, and 195 and 196). Overall, the applicability domain of the designed compounds projected that 2-hydroxyl, 2-nitro, 2-ethoxy and 2-methyl groups were beneficial at the R2 position following methyl group, thiazole and pyridyl rings at the R1 position. Model 3 was promising in this regard as the probability of accordance between the predicted and actual values is as high as that for the training set compounds (Table 3).
In silico ADMET studies
The twelve compounds with >7.9 pIC50 values listed in ESI Tables 2 and 3† were studied for their ADMET properties using SWISSADME, ADMESAR & Protox Studies. The key outcomes are listed below:
(1) According to SWISS ADME studies all of the selected 12 compounds pass the Lipinski rule of five.
(2) All the compounds have molar refractivity in the range of 40–130.
(3) All the compounds have a total polar surface area in the range of 20–130.
(4) Lipophilicity log P is less than 5.
(5) Out of the 12 compounds, 9 are moderately soluble, and 7 have high intestinal absorption.
(6) None of the compounds was a PGP substrate, so the intestinal efflux is low, and a small dose will be sufficient for drug activity.
(7) All compounds were CYP450 enzyme inhibitors, so first pass metabolism will be less and even with a low dose the bioavailability will be high.
The intestinal absorption of the molecule upon oral administration is given by the HIA score and was found to be between 0.956 and 0.1 for the best designed compounds, indicating that the compounds were well absorbed from the intestines. The predicted cell permeability Caco2 lies in the range of (0.50–0.801), which helps in better intestinal absorption and is also found to be in the acceptable range (−1 to +1). The designed compounds show a blood–brain barrier probability score of (0.9715–0.979), which implies that they cross the blood–brain barrier, producing an effect on CNS activity.
The best designed compounds are devoid of toxicological endpoints; for example, the carcinogenicity binary, immunotoxicity and cytotoxicity are within acceptable ranges, which was identified by using Protox-II. The acute oral toxicity values reveal that all 12 compounds comes under class IV drugs of toxicity, showing LD50 values in the range of (300–2000 mg kg−1). The solubility values range from −3.303 to 4.775, and the more negative the value, the higher the solubility up to −10. All 12 compounds have shown high plasma binding protein capacity, which in-turn influences the drug biological half-life. The bound portion acts as a reservoir from which the drug will be slowly released as unbound form, and then the unbound form is metabolized and excreted rapidly. ESI Tables 1 and 2 detail† the ADMET results from various servers.
Molecular docking studies
H-bond interactions were seen in compound 143 with the residues Cys268, Gln275 and Ile266 of the 6J90 protein. Compound 143 with a thiazole group at the R1 position interacted with Gln275 and Ile266 by hydrogen bonding followed by the nitrogen of the ligand thiazole ring with Cys268. The best active site conformation from Autodock results in a binding affinity of compound 143 with 6J90 protein of −9.7 kcal mol−1. Similarly, H-bond interactions were predicted in compound 145 with residues Gln335, Tyr26, His38 and Lys103 of the 6J90 protein. Compound 145 display H-bonds near to the R1 position with Tyr26, followed by oxygen of chalcone interaction with Gln335. His38 interacted with the thiazole N atom forming a favorable H-bond. The oxygen atom on the methoxy phenyl group of compound 145 interacted well with Lys103. The best active site conformation from Autodock results in a binding affinity of compound 145 with the 6J90 protein of −10.47 kcal mol−1.
Again, H-bond interactions were seen in compound 172 with the residues Cys268 and Gln275 of the 6J90 protein. Compound 172 with a pyridyl group at the R1 position does not show any interactions, followed by H-bonding of the amino group near the thiazole ring interacting with Cys268 and Gln275. The best active site conformation from Autodock results in the binding affinity of compound 172 with the 6J90 protein of −8.79 kcal mol−1. Similarly, H-bond interactions were predicted in compound 178 with the residues Cys268, Ile266, Arg276 and Lys189 of the 6J90 protein. Compound 178 displays H-bonds near to the amine group of the thiazole ring R1 position with Lys189. The oxygen of the chalcone interacted with the amine group of Arg276, and the hydroxyl group interacted with Cys268 and Ile266. The best active site conformation from Autodock results in the binding affinity of compound 17 with the 3ZKD protein of −8.65 kcal mol−1.
With various substituents at the R1 and R2 positions, the designed series gave a few best leads based on predicted values using QSAR model 3. These leads were evaluated for H-bond interactions and binding scores using molecular docking studies. The results are presented in Table 4 with interacting residues, and binding affinity scores from Autodock. The results are favorable giving importance to model equation descriptors supporting QSAR studies. In general, the molecular descriptors (weighted or unweighted) of the obtained QSAR model and type of molecular docking interactions (charged, hydrogen bond, dipole–dipole, van der Waals, pi-cation, etc.), were correlated and found to be in association with each other. ESI Fig. 5a–d† (5a-ligand 143, 5b-ligand 145, 5c-ligand 172, and 5d-ligand 178) show the docking interactions of the best compounds with 6J90 from Discovery studio visualizer 2020. To confirm the interactions and stability in the active site of DNA gyrase B, compounds 143, 145, 172 and 178 of the designed compounds were taken over for molecular dynamics simulations using the DESMOND package.
Molecular dynamics simulations
Compounds 143, 145, 172 and 178, and reference compound vosaroxin, were subjected to molecular dynamics simulations to analyze the stability of the ligands in the active-site cavity. However, it was observed that compounds 143 and 145 show fluctuations in their conformations during the simulation time (ESI Fig. 6d and 7d†). This clearly denotes instability of the ligands and furthermore, the RMSD graph of compound 172 showed fluctuations leading to an unsteady graph (ESI Fig. 8a†). Thus, compound 172 in the 6J90 active-site cannot be considered as best-fit. Analyzing further, compound 178 in the active-site of 6J90 has shown good stability, with no fluctuations in the H-bond network with the residues.
DNA gyrase 6J90 with compound 178: detailed investigation. The backbone structure and C-alpha residues were analyzed from the simulation data. The ligand fit protein in Fig. 7a displayed fluctuations up to 10 nanoseconds (0.6–2.6 Å) and displayed incremental stability until 100 nanoseconds. The RMSD average value of the protein backbone is 2.4 Å, which lies within the standard limit (<3 Å) and for the protein-reference complex, the average and maximum value was found to be 2.403 Å and 2.971 Å at 22 nanoseconds.
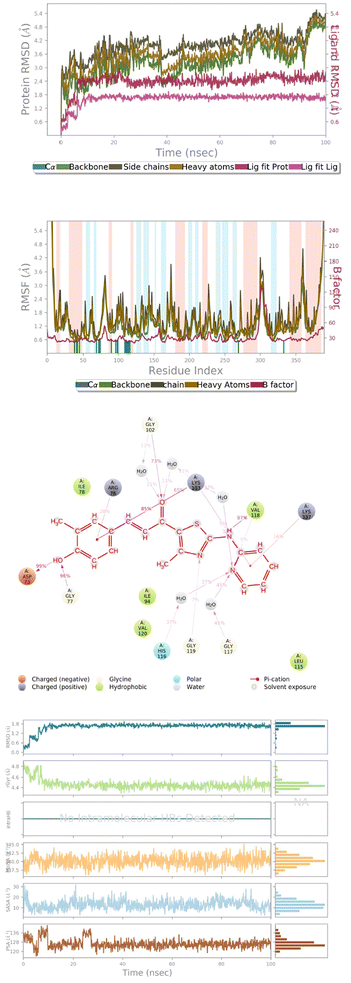 |
| Fig. 7 (a) RMSD graph of ligand 178 with 6j90. (b) RMSF graph of ligand 178 with 6j90. (c) H-bond contacts of ligand 178 with 6j90. (d) Surface analysis of ligand 178 with 6j90. | |
Dynamic properties of the protein. The RMSF graph indicates that there are no local changes along the protein chain during the thermal motion. The fluctuations are seen mildly in helices and moderately in loops. The ‘N’ and ‘C’ end terminals fluctuated higher than the other regions in the protein. The more rigid secondary structure elements like α-helices and β-strands are less fluctuated compared to the loop region residues, which participated in 70% of the simulation time (Fig. 7b).
H-bond analysis. The complex conformation displayed water bridges, ‘H’ bonds, hydrophobic contacts and ionic interactions. There are four H-bond interactions at residues Asn46, Asp73, Gly77 and Gly119 at the maximum of 99% of the simulation time. The residues Asp43 interact with the hydroxyl group on the phenyl ring, exhibiting a H-bond of about 99% of the simulation time. The residues Gly102, Val118 and His116 showed H-bond interactions with water bridges towards the chalcone oxygen, amine bridge and pyridyl nitrogen. Lys103 have shown H-bonds along with a water-bridge and hydrophobic interactions with the amine-bridge. All these vital residues play a key role for ligand binding and stabilization of the reference molecule complex (Fig. 7c).
Percentage residue of H-bond analysis. The ‘H’ bond analysis in the protein ligand contact denotes aromatic stacking interactions of the thiazole nitrogen with Gly119 for 7% simulation time. The other residues Asp73 and Gly77 showed 99% and 96% interaction during the simulation run with the hydroxyl group of the phenyl ring, respectively. His116 has shown interaction along the water-bridge for 37% of the simulation time with the pyridyl nitrogen. Similarly, Gly117 has shown interaction along the water-bridge for 41% of the simulation time with the pyridyl nitrogen. It was noticed that Gly102 has shown direct interaction with the chalcone oxygen for 73% of the simulation time. From ESI Fig. 9c† and the interactions, it is clear that ‘H’ bonding along with additional water bridges favors the molecular stability throughout the simulation time.
Ligand properties
Radius of gyration (RoG) determines the compactness of the protein during simulation. The maximum and the average values for the ROG are 4.811 Å and 4.432 Å. The solvent exposed surface area determines the changes in conformation in terms of MolSA, SASA, and PSA. The average and the minimum values of MolSA were 340.23 Å and 345.22 Å. The minimum and the average value of the solvent accessible surface area is 1.296 Å and 10.98 Å, respectively. The PSA determines the polarity induced by electronegative atoms, and the minimum and average value of PSA is 112.16 Å and 124.92 Å (Fig. 7d).
DNA gyrase 6J90 with the reference compound. The ligand fit protein in ESI Fig. 9a† displayed fluctuations and displayed incremental stability occasionally until 100 nanoseconds. The RMSD average value of the protein backbone is 1.9 Å, which lies within the standard limit (<3 Å) and for the protein-reference complex, the average and maximum value were found to be 1.912 Å and 3.012 Å at 11 nanoseconds.
Dynamic properties of the protein. Fluctuations are seen in the helices and heavily in the loops. The more rigid secondary structure elements like α-helices and β-strands fluctuated less compared to the loop region residues, which participated in 90% of the simulation time (ESI Fig. 9b†).
Percentage residue of H-bond analysis. The ‘H’ bond analysis in the protein ligand contact denotes aromatic stacking interactions of the thiazole ring with Lys103 for 28% of the simulation time. The other residues, Thr165, Asp73 and Val43, showed 6%, 61% and 9% interaction during the simulation run with the methyl-amine substituent, respectively. Tyr109 showed interaction for 19% of the simulation time with the nitrogen-containing ring system. Similarly, Ser108 has shown interaction for 7% of the simulation time with a carboxylic acid group. From ESI Fig. 9c† and the interactions, it is clear that ‘H’ bonding along with the additional water bridges favors the molecular stability throughout the simulation time.
Ligand properties
The maximum and the average values for the Radius of Gyration are 4.256 Å and 4.392 Å. The average and the minimum values of MolSA were 352.12 Å and 341.56 Å. The minimum and the average value of solvent accessible surface area is 41.2 Å and 18.2 Å, respectively. The PSA determines the polarity induced by electronegative atoms, and the minimum and average value of PSA is 159.02 Å and 172.4 Å (ESI Fig. 9d†).
Estimation of the binding free energy of the compound 178_6J90 complex. The molecular mechanics-generalized born surface area (MM-GBSA) analysis's calculation of Gibbs free energy reveals a high correlation between the anticipated and experimental binding affinities. The OPLS forcefield-based molecular mechanics energies with the variable dielectric generalised born 2.0 (VSGB) solvation model, which takes into account residue-dependent effects, was used for the polar solvation term. Solvent-Accessible Surface Area (SASA), a nonpolar solvation term, was included in the Prime MM-GBSA approach along with van der Waals interactions to estimate the binding free energy (ΔGbind) of the finished docked complex. The OPLS-2005 force field was used to optimise the docked conformations. In Prime MM-GBSA, the binding free energy of the 5FL4 protein and ligand complex was measured (molecular mechanics generalised born surface area). The Schrodinger suite's Prime module and the OPLS-2005 force field were used to compute the binding free energies of the receptor–ligand complexes. Using the free energy difference between the complex and the sum of the individual free energies of the protein and ligand, the binding free energy was computed.
ΔG (binding) = ΔG (complex) − ΔG (protein) − ΔG (ligand) |
where the binding free energy is denoted by ΔG (binding) and the free energy of the complex, protein, and ligand was denoted by ΔG (complex), ΔG (protein) and ΔG (ligand), respectively (Table 6).
Table 6 Docking interactions of the designed compounds
Compound no |
Docking score (kcal mol−1) |
Residue interactions and hydrogen bond distance in Å |
23 |
−7.85 |
Arg276 (1.9 Å), Arg276 (2.3 Å) |
63 |
−7.79 |
Arg276 (1.9 Å) |
143 |
−9.7 |
Cys268 (2.0 Å), Gln275 (1.9 Å), Ile266 (1.8 Å) |
145 |
−10.47 |
Gln335 (1.9 Å), Tyr26 (2.6 Å), His38 (2.1 Å), Lys103 (2.1 Å) |
147 |
−8.2 |
Lys337 (2.73 Å) |
149 |
−8.03 |
Arg276 (2.0 Å), His38 (2.2 Å), Asp45 (3.1 Å) |
154 |
−8.78 |
Lys337 (2.2 Å), Gln335 (2.2 Å), Met25 (2.0 Å) |
172 |
−8.79 |
Cys268 (1.8 Å), Gln275 (2.0 Å) |
178 |
−8.65 |
Cys268 (2.1 Å), Ile266 (1.8 Å), Arg276 (2.0 Å) Arg276 (2.2 Å), Lys189 (1.9 Å) |
183 |
−7.46 |
Cys268 (1.9 Å), Cys268 (2.2 Å) |
186 |
−8.47 |
Arg276 (2.79 Å), His38 (2.9 Å) |
194 |
−8.57 |
Ile186 (2.0 Å), Arg276 (2.1 Å), Glu193 (1.9 Å) |
Using the MMGBSA method and the MM-PBSA.py tool, binding free energies were calculated using 250 snapshots taken at evenly spaced intervals over the last 20 ns of the simulation. The H-bond interactions between the optimised lead molecules and the residues Arg76, Lys103, Lys337, Asp73, Val118, and His116 were demonstrated to remain constant. The RMSD increment of the ligand 178 according to Desmond calculations suggests a new binding mode, which was subsequently investigated to see if it had any impact on the binding energy using the MM-PBSA (Poisson Boltzmann surface analysis) and the MMGBSA calculations. Table 7 lists the binding free energies for the complex 6J90 compound 178 in MM-GBSA and MM-PBSA. Van der Waals (−41.4585 kcal mol−1) favoured the complex interactions more than electrostatic interactions in both techniques (−8.1254 kcal mol−1). The polar solvation energy was found to be advantageous in the development of protein-inhibitor complexes (−16.4589 kcal mol−1 in MM-GBSA and −18.3599 kcal mol−1 in MM-PBSA). When compared to the complex stability achieved by MM-PBSA, the net binding energy for the complex anticipated by MM-GBSA was −74.0394 kcal mol−1 (−73.1054 kcal mol−1). The new kind of conformation pose in binding can be more energetically beneficial, as seen by the increased binding energy in MM-GBSA. It was discovered that the net energy disparities between the MM-PBSA and MM-GBSA approaches were reliable.
Table 7 The binding free energy details of the complex 6J90_compound 178
Energy component |
Average |
Std. deviation |
Std. error of mean |
Generalized born |
Van der Waals |
−41.4585 |
2.6789 |
0.267 |
EEL |
−8.1254 |
2.8745 |
0.287 |
EGB |
−16.4589 |
1.8956 |
0.189 |
ESURF |
−7.9966 |
0.2565 |
0.256 |
ΔGgas |
−49.5839 |
2.8978 |
0.289 |
ΔGsolv |
−24.4555 |
1.5236 |
0.152 |
ΔGtotal |
−74.0394 |
1.6523 |
0.165 |
![[thin space (1/6-em)]](https://www.rsc.org/images/entities/char_2009.gif) |
Poisson Boltzmann |
Van der Waals |
−41.4585 |
2.6789 |
0.266 |
EEL |
−8.1254 |
2.8745 |
0.287 |
EPB |
−18.3599 |
1.9856 |
0.198 |
ENPOLAR |
−5.1616 |
0.2689 |
0.268 |
EDISPER |
0.0000 |
0.0000 |
0.000 |
ΔGgas |
−49.5839 |
2.9923 |
0.299 |
ΔGsolv |
−23.5215 |
1.6895 |
0.168 |
ΔGtotal |
−73.1054 |
1.9875 |
0.198 |
Conclusion
The current study focused on performing QSAR analysis of 47 chalcone derivatives utilizing validation criteria of the QSARINS software. The molecular descriptors utilized to develop the validated model favored designing compounds based on dataset structure–activity relationship information. The applicability domain of the designed 200 compounds was explored in the QSAR model region and the leads were obtained. Further ADMET profiles for the compounds with the best predicted bioactivity were analyzed and molecular docking studies were performed for active-site analysis. The stability of lead compounds 143, 145, 172 and 178 was determined using molecular dynamics simulations and upon analysis, compound 178 emerged as the best lead candidate, which can be explored further. The binding free energy decomposition analysis further indicates that residues Lys103, Asp73, Arg76, Lys337, Val118 and His116 are essential for the high selectivity of DNA gyrase B inhibition. Overall, these results serve as a significant guideline for the discovery and design of novel DNA gyrase B inhibitors.
Conflicts of interest
There are no conflicts to declare.
Acknowledgements
The authors are thankful to the Research Council of GITAM and VISTAS, and the QSARINS software license provider Dr Gramatica P.
References
- A. Ayati, S. Emami, A. Asadipour, A. Shafiee and A. Foroumadi, Recent applications of 1,3-thiazole core structure in the identification of new lead compounds and drug discovery, Eur. J. Med. Chem., 2015, 5, 699–718 CrossRef PubMed.
- T. M. Chhabria, S. Patel, P. Modi and S. P. Brahmkshatriya, Thiazole: a review on chemistry, synthesis and therapeutic importance of its derivatives, Curr. Top. Med. Chem., 2016, 16, 2841–2862 CrossRef PubMed.
- Z. Chunlin, Z. Wen, S. Chunquan, Z. Wannian, X. Chengguo and M. Zhenyuan, Chalcone: a Privileged Structure in Medicinal Chemistry, Chem. Rev., 2017, 117, 7762–7810 CrossRef PubMed.
- K. S. Yazdan, G. V. Sagar and B. A. Shaik, Biological and synthetic potentiality of chalcones: a review, J. Chem. Pharm. Res., 2015, 7, 829–842 Search PubMed.
- T. I. De Santana, B. M. De Oliveira, G. P. A. De Moraes, A. C. Da Cruz, T. G. Da Silva and A. C. Leite, Synthesis, anticancer activity and mechanism of action of new thiazole derivatives, Eur. J. Med. Chem., 2018, 20, 874–886 CrossRef PubMed.
- S. M. Gomha, M. M. Edrees and F. M. A. Altalbawy, Synthesis and Characterization of Some New Bis-Pyrazolyl-Thiazoles Incorporating the Thiophene Moiety as Potent Anti-Tumor Agents, Int. J. Mol. Sci., 2016, 17, 1499 CrossRef PubMed.
- P. Takac, M. Kello, M. Vilkova, J. Vaskova, R. Michalkova, G. Mojzisova and J. Mojzis, Antiproliferative Effect of Acridine Chalcone is Mediated by Induction of Oxidative Stress, Biomolecules, 2020, 10, 345 CrossRef CAS PubMed.
- S. M. Gomha, S. A. Ahmed and A. O. Abdelhamid, Synthesis and Cytotoxicity Evaluation of Some Novel Thiazoles, Thiadiazoles, and Pyrido [2,3-d] [1,2,4] triazole [4,3 – a] pyrimidin – 5(1H)- ones Incorporating Triazole Moiety, Molecules, 2015, 20, 1357–1376 CrossRef PubMed.
- S. Patel, R. Patle, P. Parameswaran, A. Jain and A. Shard, Design, computational studies, synthesis and biological evaluation of thiazole – based molecules as anticancer agents, Eur. J. Pharm. Sci., 2019, 134, 20–30 CrossRef PubMed.
- S. Abu-Melha, M. M. Edrees, H. H. Salem, N. A. Kheder, S. M. Gomha and M. R. Abdelaziz, Synthesis and biological evaluation of some novel thiazole-based heterocycles as potential anticancer and antimicrobial agents, Molecules, 2019, 24, 539 CrossRef PubMed.
- T. A. Farghaly, G. S. Masaret, Z. A. Muhammad and M. F. Harras, Discovery of thiazole based chalcones and 4-hetarylthiazoles as potent anticancer agents: synthesis, docking study and anticancer activity, Bioorg. Chem., 2020, 98, 103761 CrossRef CAS PubMed.
- M. D. Altıntop, B. Sever, Ç. G. Akalın and A. Özdemir, Design, synthesis, and evaluation of a new series of thiazole-based anticancer agents as potent Akt inhibitors, Molecules, 2018, 23, 1318 CrossRef PubMed.
- V. Spanò, A. Attanzio, S. Cascioferro, A. Carbone, A. Montalbano, P. Barraja, L. Tesoriere, G. Cirrincione, P. Diana and B. Parrino, Synthesis and Antitumor Activity of New Thiazole Nortopsentin Analogs, Mar. Drugs, 2016, 14, 226 CrossRef PubMed.
- A. B. Shaik, R. R. Bhandare, S. Nissankararao, B. V. Lokesh, S. Shahanaaz and M. M. Rahman, Synthesis, and biological screening of chloropyrazine conjugated benzothiazepine derivatives as potential antimicrobial, antitubercular and cytotoxic agents, Arabian J. Chem., 2021, 14, 102915 CrossRef CAS.
- Y. Wang, W. Zhang, J. Dong and J. Gao, Design, synthesis and bioactivity evaluation of coumarin–chalcone hybrids as potential anticancer agents, Bioorg. Chem., 2020, 95, 103530 CrossRef CAS PubMed.
- A. Shaik, R. R. Bhandare, K. Palleapati, S. Nissankararao, V. Kancharlapalli and S. Shaik, Antimicrobial, Antioxidant, and Anticancer Activities of Some Novel Isoxazole Ring Containing Chalcone and Dihydropyrazole Derivatives, Molecules, 2020, 25, 1047 CrossRef CAS PubMed.
- S. Madhavi, R. Sreenivasulu, J. P. Yazala and R. R. Raju, Synthesis of chalcone incorporated quinazoline derivatives as anticancer agents, Saudi Pharm. J., 2017, 25, 275–279 CrossRef PubMed.
- H. R. M. Rashdan, A. H. Abdelmonsef, I. A. Shehadi, S. M. Gomha, A. M. M. Soliman and H. K. Mahmoud, Synthesis, Molecular Docking Screening and Anti-Proliferative Potency Evaluation of Some New Imidazo[2,1-b]Thiazole Linked Thiadiazole Conjugates, Molecules, 2020, 25, 4997 CrossRef CAS PubMed.
- S. Madhavi, R. Sreenivasulu, A. M. Yousuf, A. M. Jawed and R. R. Ramesh, Synthesis, biological evaluation and molecular docking studies of pyridine incorporated chalcone derivatives as anticancer agents, Lett. Org. Chem., 2016, 13, 682–692 CrossRef CAS.
- Y. K. Abhale, A. Shinde, K. K. Deshmukh, L. Nawale, D. Sarkar and P. C. Mhaske, Synthesis, antitubercular and antimicrobial potential of some new thiazole substituted thiosemicarbazide derivatives, Med. Chem. Res., 2017, 26, 2557–2567 CrossRef CAS.
- S. T. Dhumal, A. R. Deshmukh, M. R. Bhosle, V. M. Khedkar, L. U. Nawale, D. Sarkar and R. A. Mane, Synthesis and antitubercular activity of new 1,3,4-oxadiazoles bearing pyridyl and thiazolyl scaffolds, Bioorg. Med. Chem. Lett., 2016, 26, 3646–3651 CrossRef CAS PubMed.
- N. U. Güzeldemirci, B. Karaman and Ö. Küçükbasmaci, Antibacterial, antitubercular and antiviral activity evaluations of some arylidene hydrazide derivatives bearing imidazo [2,1-b] thiazole moiety, Turk. J. Pharm. Sci., 2017, 14, 157 CrossRef PubMed.
- M. Yan, L. Xu, Y. Wang, J. Wan, T. Liu, W. Liu, Y. Wan, B. Zhang, R. Wang and Q. Li, Opportunities and challenges of using five-membered ring compounds as promising antitubercular agents, Drug Dev. Res., 2020, 81, 402–418 CrossRef CAS PubMed.
- A. Kryshchyshyn, O. Roman, A. Lozynskyi and R. Lesyk, Thiopyrano [2,3-d]Thiazoles as New Efficient Scaffolds in Medicinal Chemistry, Sci. Pharm., 2018, 86, 26 CrossRef PubMed.
- G. B. Gundlewad and B. R. Patil, Synthesis and Evaluation of Some Novel 2-Amino-4-Aryl Thiazoles for Antitubercular Activity, J. Heterocycl. Chem., 2018, 55, 769–774 CrossRef CAS.
- A. B. Shaik, R. R. Bhandare, S. Nissankararao, Z. Edis, N. R. Tangirala, S. Shahanaaz and M. M. Rahman, Design, facile synthesis and characterization of dichloro substituted chalcones and dihydropyrazole derivatives for their antifungal, antitubercular and antiproliferative activities, Molecules, 2020, 25, 3188 CrossRef CAS PubMed.
- S. Pola, K. K. Banoth, M. Sankaranarayanan, R. Ummani and A. Garlapati, Design, synthesis, in silico studies, and evaluation of novel chalcones and their pyrazoline derivatives for antibacterial and antitubercular activities, Med. Chem. Res., 2020, 29, 1819–1835 CrossRef CAS.
- S. Burmaoglu, O. Algul, A. Gobek, A. D. Aktas, M. Ulger, B. G. Erturk, E. Kaplan, A. Dogen and G. Aslan, Design of potent fluoro – substituted chalcones as antimicrobial agents, J. Enzyme Inhib. Med. Chem., 2017, 32, 490–495 CrossRef CAS PubMed.
- A.-M. Borcea, I. Ionut, O. Cris and O. Oniga, An Overview of the Synthesis and Antimicrobial, Antiprotozoal, and Antitumor Activity of Thiazole and Bisthiazole Derivatives, Molecules, 2021, 26, 624 CrossRef CAS PubMed.
- P. Kishor, K. V. Ramana and A. B. Shaik, Antitubercular evaluation of isoxazolyl chalcones, Res. J. Pharm., Biol. Chem. Sci., 2017, 8, 730–735 CAS.
- B. V. Lokesh, Y. R. Prasad and A. B. Shaik, Synthesis and biological activity of novel 2,5-dichloro-3-acetylthiophene chalcone derivatives, Indian J. Pharm. Educ. Res., 2017, 51, 679–690 CrossRef.
- A. M. Asiri and S. A. Khan, Synthesis and Anti-Bacterial Activities of a Bis-Chalcone Derived from Thiophene and Its Bis-Cyclized Products, Molecules, 2011, 16, 523–531 CrossRef CAS PubMed.
- A. J. Kadhim, J. H. Mohammed and N. M. Aljamali, Thiazole Amide Derivatives (Synthesis, Spectral Investigation, Chemical Properties, Antifungal Assay), NeuroQuantology, 2020, 18, 16 Search PubMed.
- M. Kucerova-Chlupacova, V. Vyskovska-Tyllova, L. Richterova-Finkova, J. Kunes, V. Buchta, M. Vejsova, P. Paterova, L. Semelkova, O. Jandourek and V. Opletalova, Novel Halogenated Pyrazine-Based Chalcones as Potential Antimicrobial Drugs, Molecules, 2016, 21, 1421 CrossRef PubMed.
- A. B. Shaik, R. P. Yejella and S. Shaik, Synthesis, Antimicrobial, and Computational Evaluation of Novel Isobutylchalcones as Antimicrobial Agents, Int. J. Med. Chem., 2017, 2017, 6873924 Search PubMed.
- G. Singh, A. Arora, P. Kalra, I. K. Maurya, C. E. Ruizc, M. A. Estebanc, S. Sinha, K. Goyal and R. Sehgal, A strategic approach to the synthesis of ferrocene appended chalcone linked triazole allied organo silatranes: antibacterial, antifungal, antiparasitic and antioxidant studies, Bioorg. Med. Chem., 2019, 27, 188–195 CrossRef CAS PubMed.
- A. B. Shaik, S. V. Lohitha, S. B. Puttagunta, A. Shaik, K. Supraja and H. K. Sai, Synthesis and screening of novel lipophilic diarylpropeones as prospective antitubercular, antibacterial and antifungal agents, Biointerface Res. Appl. Chem., 2019, 9, 3912–3918 CAS.
- M. Mellado, L. Espinoza, A. Madrid, J. Mella, E. Chávez-Weisser, K. Diaz and M. Cuellar, Design, synthesis, antifungal activity, and structure–activity relationship studies of chalcones and hybrid dihydrochromane–chalcones, Mol. Diversity, 2019, 3, 1–3 Search PubMed.
- S. B. Lagu, P. Y. Rajendra, N. Srinath and B. S. Afzal, Synthesis, antibacterial, antifungal antitubercular activities and molecular docking studies of nitrophenyl derivatives, Int. J. Life Sci. Pharma Res., 2019, 9, 54–64 Search PubMed.
- M. Djukic, M. Fesatidou, I. Xenikakis, A. Geronikaki, V. T. Angelova, V. Savic, M. Pasic, B. Krilovic, D. Djukic and B. Gobeljic, et al., In vitro antioxidant activity of thiazolidinone derivatives of 1,3-thiazole and 1,3,4-thiadiazole, Chem.-Biol. Interact., 2018, 286, 119–131 CrossRef CAS PubMed.
- V. A. Adole, R. A. More, B. S. Jagdale, T. B. Pawar and S. S. Chobe, Efficient synthesis, antibacterial, antifungal, antioxidant and cytotoxicity study of 2-(2-hydrazineyl) thiazole derivatives, ChemistrySelect, 2020, 5, 2778–2786 CrossRef CAS.
- C. Nastasa, B. Tiperciuc, M. Duma, D. Benedec and O. Oniga, New hydrazones bearing thiazole scaffold: synthesis, characterization, antimicrobial, and antioxidant investigation, Molecules, 2015, 20, 17325–17338 CrossRef CAS PubMed.
- E. Polo, N. Ibarra-Arellano, L. Prent-Peñaloza, A. Morales-Bayuelo, J. Henao, A. Galdámez and M. Gutiérrez, Ultrasound-assisted synthesis of novel chalcone, heterochalcone and bis-chalcone derivatives and the evaluation of their antioxidant properties and as acetylcholinesterase inhibitors, Bioorg. Chem., 2019, 90, 103034 CrossRef CAS PubMed.
- J. Wang, L. Huang, C. Cheng, G. Li, J. Xie, M. Shen, Q. Chen, W. Li, W. He and P. Qiu, et al., Design, synthesis and biological evaluation of chalcone analogues with novel dual antioxidant mechanisms as potential anti-ischemic stroke agents, Acta Pharm. Sin. B, 2019, 9, 335–350 CrossRef PubMed.
- N. A. Al Zahrani, R. M. El-Shishtawy, M. M. Elaasser and A. M. Asiri, Synthesis of Novel Chalcone-Based Phenothiazine Derivatives as Antioxidant and Anticancer Agents, Molecules, 2020, 25, 4566 CrossRef CAS PubMed.
- N. Sun, Y. J. Lu, F. Y. Chan, R. L. Du, Y. Y. Zheng, K. Zhang, L. Y. So, R. Abagyan, C. Zhuo and Y. C. Leung, et al., A thiazole orange derivative targeting the bacterial protein FtsZ shows potent antibacterial activity, Front. Microbiol., 2017, 8, 855 CrossRef PubMed.
- E. Abdel-Latif, A. S. Almatari and G. E. Abd-ElGhani, Synthesis and Antibacterial Evaluation of Some New Thiazole-Based Polyheterocyclic Ring Systems, J. Heterocycl. Chem., 2019, 56, 1978–1985 CrossRef CAS.
- S. R. Vegesna, Y. R. Prasad and B. S. Afzal, Antimicrobial evaluation of some novel pyrazine based chalcones, Int. J. Adv. Pharm. Sci., 2017, 8, 11–18 CAS.
- A. Özdemir, M. D. Altıntop, B. Sever, H. K. Gençer, H. A. Kapkaç, Ö. Atlı and M. Baysal, A New Series of Pyrrole-Based Chalcones: Synthesis and Evaluation of Antimicrobial Activity, Cytotoxicity, and Genotoxicity, Molecules, 2017, 22, 2112 CrossRef PubMed.
- X. Tang, S. Su, M. Chen, J. He, R. Xia, T. Guo, Y. Chen, C. Zhang, J. Wang and W. Xue, Novel chalcone derivatives containing a 1,2,4-triazine moiety: design, synthesis, antibacterial and antiviral activities, RSC Adv., 2019, 9, 6011–6020 RSC.
- S. B. Lagu, R. P. Yejella, R. R. Bhandare and A. B. Shaik, Design, Synthesis, and Antibacterial and Antifungal Activities of Novel Trifluoromethyl and Trifluoromethoxy Substituted Chalcone Derivatives, Pharmaceuticals, 2020, 13, 375 CrossRef CAS PubMed.
- P. V. Reddy, M. Hridhay, K. Nikhil, S. Khan, P. N. Jha, K. Shah and D. Kumar, Synthesis and investigations into the anticancer and antibacterial activity studies of β-carboline chalcones and their bromide salts, Bioorg. Med. Chem. Lett., 2018, 28, 1278–1282 CrossRef PubMed.
- S. A. Khan and A. M. Asiri, Green synthesis, characterization and biological evaluation of novel chalcones as antibacterial agents, Arabian J. Chem., 2017, 10, S2890–S2895 CrossRef CAS.
- M. Zhang, A. M. Prior, M. M. Maddox, W. J. Shen, K. E. Hevener, D. F. Bruhn, R. B. Lee, A. P. Singh, J. Reinicke and C. J. Simmons, et al., Pharmacophore modeling, synthesis, and antibacterial evaluation of chalcones and derivatives, ACS Omega, 2018, 3, 18343–18360 CrossRef CAS PubMed.
- A. Gomtsyan, Heterocycles in drugs and drug discovery, Chem. Heterocycl. Compd., 2012, 48, 7–10 CrossRef CAS.
- C. Viegas-Junior, A. Danuello, V. da Silva Bolzani, E. J. Barreiro and C. A. M. Fraga, Molecular hybridization: a useful tool in the design of new drug prototypes, Curr. Med. Chem., 2007, 14, 1829–1852 CrossRef CAS PubMed.
- K. V. Sashidhara, K. B. Rao, P. Kushwaha, R. K. Modukuri, P. Singh, I. Soni, P. K. Shukla, S. Chopra and M. Pasupuleti, Novel chalcone–thiazole hybrids as potent inhibitors of drug resistant Staphylococcus aureus, ACS Med. Chem. Lett., 2015, 6, 809–813 CrossRef CAS PubMed.
- S. Sinha, S. L. Manju and M. Doble, Chalcone–Thiazole Hybrids: Rational Design, Synthesis, and Lead Identification against 5-Lipoxygenase, ACS Med. Chem. Lett., 2019, 10, 1415–1422 CrossRef CAS PubMed.
- P. Gramatica, N. Chirico, E. Papa, S. Cassani and S. Kovarich, QSARINS: a new software for the development, analysis, and validation of QSAR MLR models, J. Comput. Chem., 2013, 34, 2121–2132, DOI:10.1002/jcc.23361.
- P. Gramatica, Principles of QSAR Modeling: Comments and Suggestions from Personal Experience, Int. J. Quant. Struct.-Prop. Relat., 2020, 5(3), 61–97, DOI:10.4018/IJQSPR.20200701.oa1.
- P. Gramatica, N. Chirico and S. Cassani, QSARINS-chem: Insubria datasets and new QSAR/QSPR models for environmental pollutants in QSARINS, J. Comput. Chem., 2014, 35, 1036–1044, DOI:10.1002/jcc.23576.
- K. Liaras, A. Geronikaki, J. Glamočlija, A. Ćirić and M. Soković, Thiazole-based
chalcones as potent antimicrobial agents. Synthesis and biological evaluation, Bioorg. Med. Chem., 2011, 19(10), 3135–3140 CrossRef CAS PubMed.
- C. Tratrat, M. Haroun, E. Tsolaki, A. Petrou, A. Gavalas and A. Geronikaki, Thiazole-based Chalcone Derivatives as Potential Anti-inflammatory Agents: Biological Evaluation and Molecular Modelling, Curr. Top. Med. Chem., 2021, 21(4), 257–268, DOI:10.2174/1568026621999201214232458.
- M. D. Hanwell, D. E. Curtis and D. C. Lonie, et al., Avogadro: an advanced semantic chemical editor, visualization and analysis platform, J. Cheminf., 2012, 4, 17, DOI:10.1186/1758-2946-4-17.
- N. M. O’Boyle, M. Banck and C. A. James, et al., Open Babel: an open chemical toolbox, J. Cheminf., 2011, 3, 33, DOI:10.1186/1758-2946-3-33.
- J. Dong, D. S. Cao and H. Y. Miao, et al., ChemDes: an integrated web-based platform for molecular descriptor and fingerprint computation, J. Cheminf., 2015, 7, 60, DOI:10.1186/s13321-015-0109-z.
- P. Gramatica and A. Sangion, A Historical Excursus on the Statistical Validation Parameters for QSAR Models: A Clarification Concerning Metrics and Terminology, J. Chem. Inf. Model., 2016, 56(6), 1127–1131, DOI:10.1021/acs.jcim.6b00088.
- A. Daina, O. Michielin and V. Zoete, SwissADME: a free web tool to evaluate pharmacokinetics, drug-likeness and medicinal chemistry friendliness of small molecules, Sci. Rep., 2017, 7, 42717, DOI:10.1038/srep42717.
- F. Cheng, W. Li, Y. Zhou, J. Shen, Z. Wu, G. Liu, P. W. Lee and Y. Tang, admetSAR: A Comprehensive Source and Free Tool for Assessment of Chemical ADMET Properties, J. Chem. Inf. Model., 2012, 52(11), 3099–3105, DOI:10.1021/ci300367a.
- P. Banerjee, A. O. Eckert, A. K. Schrey and R. Preissner, ProTox-II: a webserver for the prediction of toxicity of chemicals, Nucleic Acids Res., 2018, 46(W1), W257–W263, DOI:10.1093/nar/gky318.
- J. Eberhardt, D. Santos-Martins, A. F. Tillack and S. Forli, AutoDock Vina 1.2.0: New Docking Methods, Expanded Force Field, and Python Bindings, J. Chem. Inf. Model., 2021, 61(8), 3891–3898, DOI:10.1021/acs.jcim.1c00203.
- N. Eswar, B. Webb, M. A. Marti-Renom, M. S. Madhusudhan, D. Eramian, M. Y. Shen, U. Pieper and A. Sali, Comparative protein structure modeling using Modeller, Curr. Protoc. Bioinf., 2006 DOI:10.1002/0471250953.bi0506s15.
- https://www.3ds.com/products-services/biovia/products/molecular-modeling-simulation/biovia-discovery-studio/visualization/.
- L. Ivanova, J. Tammiku-Taul, A. T. García-Sosa, Y. Sidorova, M. Saarma and M. Karelson, Molecular Dynamics Simulations of the Interactions between Glial Cell Line-Derived Neurotrophic Factor Family Receptor GFRα1 and Small-Molecule Ligands, ACS Omega, 2018, 3(9), 11407–11414, DOI:10.1021/acsomega.8b01524.
- P. Broto, G. Moreau and C. Vandycke, Molecular structures: perception, autocorrelation descriptor and sar studies. Autocorrelation descriptor, Eur. J. Med. Chem., 1984, 19, 66–70 CAS.
- https://www.epa.gov/sites/default/files/2015-05/documents/moleculardescriptorsguide-v102.pdf.
- O. Devinyak, D. Havrylyuk and L. Roman, 3D-MoRSE descriptors explained, J. Mol. Graphics Modell., 2014, 54, 194–203, DOI:10.1016/j.jmgm.2014.10.006.
|
This journal is © The Royal Society of Chemistry 2023 |